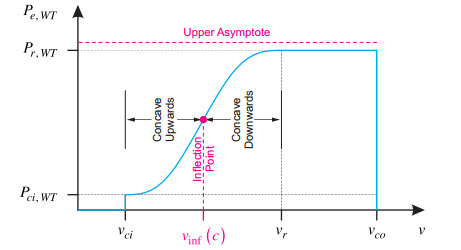
Major issues with logistic functions (LFs) in modeling wind turbine power-speed characteristics (WTPSCs) include: 1. low accuracy near cut-in and rated wind speeds due to lack of continuity; 2. difficulties in fitting their parameters because of ill-conditioning; 3. no guaranteed monotonicity; 4. no systematic way to determine upper and lower limits for their parameters. The literature also reports that six parameter LFs may sometimes provide less accurate results than five, four, and three parameter models, implying: 1. they are unsuitable for WTPSC modeling; 2. lack of systematic method to determine upper and lower limits for optimization algorithms to search in. In this paper, we propose a new six parameter LF then employ subspace trust-region (STIR) algorithm to estimate its parameters. We compare the accuracy of our six parameter model to others from the literature. With 42 on-shore and off-shore WTs database of ratings varying from 275 to 8000 kW, we the comprehensiveness of our model. The results show an average mean absolute percent error (MAPE) of 2.383 × 10−3. Furthermore, our model reduces average and median normalized root mean square error (NRMSE) by 32.3% and 38.5%, respectively.
Citation: Al-Motasem Aldaoudeyeh, Khaled Alzaareer, Di Wu, Mohammad Obeidat, Salman Harasis, Zeyad Al-Odat, Qusay Salem. A novel monotonic wind turbine power-speed characteristics model[J]. AIMS Energy, 2023, 11(6): 1231-1251. doi: 10.3934/energy.2023056
[1] | Nour Khlaifat, Ali Altaee, John Zhou, Yuhan Huang . A review of the key sensitive parameters on the aerodynamic performance of a horizontal wind turbine using Computational Fluid Dynamics modelling. AIMS Energy, 2020, 8(3): 493-524. doi: 10.3934/energy.2020.3.493 |
[2] | Rashid Al Badwawi, Mohammad Abusara, Tapas Mallick . Speed control of synchronous machine by changing duty cycle of DC/DC buck converter. AIMS Energy, 2015, 3(4): 728-739. doi: 10.3934/energy.2015.4.728 |
[3] | Stephen K. Musau, Kathrin Stahl, Kevin Volkmer, Nicholas Kaufmann, Thomas H. Carolus . A design and performance prediction method for small horizontal axis wind turbines and its application. AIMS Energy, 2021, 9(5): 1043-1066. doi: 10.3934/energy.2021048 |
[4] | Hassam Nasarullah Chaudhry, John Kaiser Calautit, Ben Richard Hughes . The Influence of Structural Morphology on the Efficiency of Building Integrated Wind Turbines (BIWT). AIMS Energy, 2014, 2(3): 219-236. doi: 10.3934/energy.2014.3.219 |
[5] | Majid Deldar, Afshin Izadian, Sohel Anwar . Analysis of a hydrostatic drive wind turbine for improved annual energy production. AIMS Energy, 2018, 6(6): 908-925. doi: 10.3934/energy.2018.6.908 |
[6] | Ammar E. Ali, Nicholas C. Libardi, Sohel Anwar, Afshin Izadian . Design of a compressed air energy storage system for hydrostatic wind turbines. AIMS Energy, 2018, 6(2): 229-244. doi: 10.3934/energy.2018.2.229 |
[7] | Saiyad S. Kutty, M. G. M. Khan, M. Rafiuddin Ahmed . Estimation of different wind characteristics parameters and accurate wind resource assessment for Kadavu, Fiji. AIMS Energy, 2019, 7(6): 760-791. doi: 10.3934/energy.2019.6.760 |
[8] | Azevedo Joaquim, Mendonça Fábio . Small scale wind energy harvesting with maximum power tracking. AIMS Energy, 2015, 2(3): 297-315. doi: 10.3934/energy.2015.3.297 |
[9] | Navid Majdi Nasab, Jeff Kilby, Leila Bakhtiaryfard . Integration of wind and tidal turbines using spar buoy floating foundations. AIMS Energy, 2022, 10(6): 1165-1189. doi: 10.3934/energy.2022055 |
[10] | Ayman B. Attya, T. Hartkopf . Wind Turbines Support Techniques during Frequency Drops — Energy Utilization Comparison. AIMS Energy, 2014, 2(3): 260-275. doi: 10.3934/energy.2014.3.260 |
Major issues with logistic functions (LFs) in modeling wind turbine power-speed characteristics (WTPSCs) include: 1. low accuracy near cut-in and rated wind speeds due to lack of continuity; 2. difficulties in fitting their parameters because of ill-conditioning; 3. no guaranteed monotonicity; 4. no systematic way to determine upper and lower limits for their parameters. The literature also reports that six parameter LFs may sometimes provide less accurate results than five, four, and three parameter models, implying: 1. they are unsuitable for WTPSC modeling; 2. lack of systematic method to determine upper and lower limits for optimization algorithms to search in. In this paper, we propose a new six parameter LF then employ subspace trust-region (STIR) algorithm to estimate its parameters. We compare the accuracy of our six parameter model to others from the literature. With 42 on-shore and off-shore WTs database of ratings varying from 275 to 8000 kW, we the comprehensiveness of our model. The results show an average mean absolute percent error (MAPE) of 2.383 × 10−3. Furthermore, our model reduces average and median normalized root mean square error (NRMSE) by 32.3% and 38.5%, respectively.
Abbreviations: DE: Differential evolution; EA: Evolutionary algorithm; EP: Evolutionary programming; GA: Genetic algorithm; GCR: Generator control region; GWEC: Global wind energy council; LF: Logistic function; LSE: Least-Square error; MAE: Mean absolute error; MAPE: Mean absolute percent error; NRMSE: Normalized root mean square error; PDF: Power distribution function; PSO: Particle swarm optimization; RMSE: Root mean square error; STIR: Subspace trust-region; TI: Turbulence intensity; WT: Wind turbine; WTPSC: Wind turbine power-speed characteristics; SDS: Significant downwards shifting; SUS: Significant upwards shifting; MDS: Minor downwards shifting; MUS: Minor upwards shifting; CBS: Curve becomes steeper; CBF: Curve becomes flatter; NDS: Negligible downwards shifting; NUS: Negligible upwards shifting; DbS: Decrease (in q(→θ,v)) by horizontal shifting; IoD: Increase or decrease (in q(→θ,v)) by horizontal shifting
WT installations experienced an unprecedented annual growth of 53% in 2020. GWEC reports that, with 93 GW of new installations in 2020, the global cumulative capacity reached 743 GW [1].
Such growth is attributed to factors such as: 1. innovations in blades design and fabrication [2]; 2. improvement in nacelle components reliability (e.g., gears with fatigue and wear resistance and new softwares for system level modeling of the nacelle) [2]; 3. improved sensors and control algorithms [2]; 4. political support (e.g., low carbon energy goals, feed-in tariffs, or guaranteed access to the transmission grid) [3,4].
WTPSCs play significant roles in: 1. risk assessment [5]; 2. wind energy yield and WT selection [5]; 3. condition monitoring [6]; 4. sizing storage capacity for wind power integration [7]; 5. predictive control optimization [8]; 6. WTs troubleshooting [8]; 7. detection of degradation because of aging [6]; 8. optimal dispatching of wind farms [6].
Near cut-in wind speed, WTPSCs are difficult to model [9]. Although we theoretically expect WTs power to exhibit a cubic relationship with wind (when it is below rated speed), this is incorrect. The conversion efficiency of WTs varies with speed, which is most remarkable near cut-in and rated wind speeds. Just slightly above cut-in speed, virtually all WTs exhibit a steep growth in efficiency. Near rated wind speeds, WTs 'spill' wind energy [10], decreasing the efficiency. The result is complex and nonlinear relationship between wind speed and the WT power output [11].
The general shape of LFs (sometimes referred to as logistic distributions or activation functions) made them meaningfully suitable for scientific modeling of bacteria and plant growth since a growing population 'competes' for resources, placing an upper limit on the number of bacteria/plants [12]. LFs can also be useful for certain biological, chemical, linguistic, political science topics [13].
Since both of LFs and WTPSCs are 'S'-shaped, LFs are (at least in principle) candidates for accurate WTPSC mathematical models. LFs are parametric models (they are based on mathematical expressions with a fixed number of parameters), bringing potential to offer some analytical advantages. For instance, in wind energy assessment, it is possible to use them along with Weibull distribution to obtain explicit PDFs [14]. Lydia et al. [15] are among the earliest researchers to attempt modeling WTPSCs curves using LFs. They propose a 5-parameter LF and estimate its parameters using GA, EP, PSO and DE. Many researchers have sought new methods to estimate the parameters of the LF proposed by Lydia et al. [15] (e.g., [6,16,17]). Sohoni et al. [9] reported that certain LFs (due to explicitly including an inflection point in their parameters) have the potential to increase accuracy and improve online-monitoring using LFs. Pei and Li [11] confirmed this by parameterizing various WTPSC models and comparing them using statistical metrics, such as MAE and RMSE.
Jing et al. [18] improved the model of Lydia et al. [15] by introducing 'quantile dependency' to its parameters. Jing et al. [18] parameterized the model proposed by Lydia et al. [15] using PSO for three wind farms and validating the accuracy of their work using MAPE and NRMSE.
Villanueva and Feijóo [19] proposed two 'generalized' 6-parameter LFs and estimate their parameters using evolutionary optimization techniques. The proposed models show substantial accuracy improvement, but Villanueva and Feijóo [19] reported difficulties in optimizing their parameters.
Zou et al. [17] reviewed three, four, five, and six parameter LFs and their accuracy using MAE and RMSE. Results show that six parameter LF may sometimes provide lower accuracy than four and three parameter LF. Thus, adding new parameters to LFs does not necessarily contribute to increased accuracy. Villanueva and Feijóo [19] reported similar results.
In Section 2, we provide a mathematical background on WTPSC and LFs and highlight their limitations and our contributions. Section 3 proposes a new LF model, demonstrates its curve fitting merits, and develops upper and lower limits on its parameters. We validate our model in Section 4 by providing graphical and numerical accuracy results. To ensure a suitable comparison, we compare our work with other 6-parameter LFs. Section 5 draws final remarks, discusses the limitations of the study and puts forth suggestions for future investigations. A summary of the literature gap and the most significant contributions are then mentioned in Section 6.
For stall or pitch-controlled WT, it is possible to characterize the WTPSC curves mathematically as [5]
Pe,WT(v)={0v<vciqe(→θ,v)vci≤v≤vrPrvr<v≤vco0v>vco | (1) |
where v is the wind speed. vci, vr, and vco are the cut-in, rated, and cut-out speeds of the WT, respectively. Pr is the rated output power of the WT. qe(→θ,v) is a mathematical expression which should (ideally) accurately estimate the manufacturer-provided data (i.e., empirical power-speed pairs in the GCR). Such estimation is typically done by fitting a vector of parameters →θ using the least-squares method [5].
Figure 1 depicts a WTPSC curve. When estimating →θ parameters to fit the manufacturer datasheet, some points of interest assist us in establishing its limits.
1. The curve is monotonic. This means it always increases (or at least remains the same) with v. Mathematically, this means the first derivative must remain greater than or equal to zero, as Eq 2:
dqe(→θ,v)dv≥0,∀v≥0 | (2) |
2. For virtually all WTPSC curves, an inflection point exists. This is the point at which the curve switches from concave upwards to concave downwards. Mathematically, this means the second derivative must be zero, as Eq 3:
d2qe(→θ,v)v2|v=vinf=0 | (3) |
Where vinf is the inflection point.
3. One major attribute of LFs is that they should approach an upper asymptote when their dependent variable approaches infinity. Mathematically, this means
limv→∞qe(→θ,v)≈Pr,WT |
Ideally, the upper asymptote of WTPSC should be close to Pr. This trend of approaching an upper asymptote is obvious in virtually all WTPSC curves when v→vr. In practice, v is never ∞, but this mathematical formulation benefits in establishing upper and lower limits for →θ parameters, which, in turn, assists the optimization algorithm by searching in a suitable region instead of arbitrarily determining it by trial and error.
6-parameter LFs are discussed in Villanueva and Feijóo [19] and have one of the following forms
q(→θ,v)=d+a−d(ε+[vc]−b)g6PL | (4) |
q(→θ,v)=d+a−d(ε+e−b(v−c))g6PLE | (5) |
where a and d are the upper and lower asymptote, respectively. b is the growth rate (sometimes called 'hill slope') around point c. g is called the 'asymmetry factor' because it tunes the degree of asymmetry around point c [11,15]. c is the inflection point (the point at which q(→θ,v) turns from concave upward to concave downward). ε has no special meaning, but has a value around one [8].
Disadvantages of fits in Eqs 4 and 5:
1. With some WTs, they inaccurately fit the WTPSC, especially near vci and vr. This is reported by as a disadvantage for piecewise models, which is the conventional way to account for the effect of turning off the WT when v<vci and v>vco [9]. Yan et al. [20] reports such inaccuracy for LFs even with continuous WTPSC;
2. Contain a high number of parameters compared to other fits with fewer parameters, but better accuracy. For example, Zou et al. [17] review three, four, five, and six parameter LF and tested their accuracy using MAE and RMSE. Results showed that 6-parameter LF may sometimes provide lower accuracy than three, four and five parameter LFs. Pei and Li [11] report similar results. Thus, adding new parameters does not contribute to increased accuracy. Intuitively, adding new parameters should provide certain advantages, such as accuracy improvement;
3. Their monotonicity is not guaranteed when the parameters are estimated using EAs. We overcome this disadvantage in Aldaoudeyeh et al. [8];
4. Sometimes LFs have the possibility of becoming ill-conditioned, making the estimation of →θ parameters difficult [9].
This paper contain the following contributions:
1. We propose a new 6-parameter LF model for WTPSC curves; 2. We develop constraints on the parameters of our model to guarantee its monotonicity; 3. The model exhibits excellent fit that is at least as accurate as 6PL and 6PLE for some WTs, but also provides significant MAPE and NRMSE improvements. It also provides high accuracy near vci and vr; 4. The parameters of our model are easily optimized with the STIR algorithm.
Our proposed model is
q(→θ,v)=d+a−d(1+[vc]ζe−b(v−c))g | (6) |
where a is the upper asymptote. d is a parameter to tune the lower asymptote near vci (i.e., d is not the lower asymptote, but it drastically influences it). b is the growth rate around point c. g is called the 'asymmetry factor' because it tunes the degree of asymmetry around point c. c is the inflection point. ζ is the steepness tuning factor.
We call this model 6PLEZ. At a glance, 6PLEZ model resembles 6PL and 6PLE (Eqs 4 and 5), but, as we will see in Section 4, it is more accurate. To our best knowledge, the literature does not contain this model. Villanueva and Feijóo [21] and Zou et al. [17] review WTPSC models (including LFs models), but the 6PLEZ model does not appear on any of them.
We estimate →θ parameters with the same method mentioned in Aldaoudeyeh et al. [8] (LSEs objective function minimized using STIR algorithm).
In this subsection, we illustrate the effect of →θ parameters variation on q(→θ,v) shape. Unless otherwise is specified, the parameters are a=1, b=1.25, c=6, d=0, g=1, and ζ=0. The dependent parameter (v) is shown from 2.5 (typical vci) to 12 (typical vr).
Figure 2 shows the effect of varying a and d on the shape of q(→θ,v). Clearly, increasing a slightly shifts the curve upwards near vci. This effect becomes more pronounced the closer we get to vr, where the upper asymptote varies linearly with a. The final value of q(→θ,v) is a. We thus call it the upper asymptote. We also note that increasing a steepens the curve near c.
The effect of d is better illustrated with a=10 and when we extend the curve from 0 to vr. Increasing d shifts the upwards curve near vci and its effect diminishes at vr. We also note that increasing d flattens the curve near c.
Thus, most of a and d effect is to scale the maximum and minimum asymptotes of the LF curve, respectively. By contrast, b, c, g, and ζ (as we will discuss now) define the shape of the LF.
Figure 3 shows the effects of b, c, g, and ζ variation on q(→θ,v) shape. The increase in b shifts the curve downwards near vci and upwards near vr. However, it is also obvious from the curve that the effect is more pronounced near vci. As b increases, the curve becomes steeper near the inflection point (vinf=c).
The variation of c only shifts the curve to the left and right. In fact, it is worth mentioning that all curves for varying c are the same, but merely shifted horizontally. It is possible to conclude from Figure 3 that increasing c decreases q(→θ,v) near vci, and increases or decreases q(→θ,v) near vr.
The increase in g drastically downshifts the curve near vci. It also substantially downshifts the curve near vinf and makes it steeper, but has no effect on the power curve near c. As we increase ζ, q(→θ,v) shifts slightly upward and becomes flatter near c, but has no effect on q(→θ,v) near vr. In a sense, ζ adjusts the steepness around c while having a negligible effect minor increase effect vci and a negligible decrease near vr.
Note that b and ζ seem to provide the same effect. Remarkable differences, however, are: 1. b tunes the steepness while having significant effect near vci and a minor effect near vr; 2. ζ tunes the steepness while having minor effect near vci and a negligible effect near vr.
Table 1 shows a summary of the previously mentioned effects. In Figure 4, we list some examples on why our model provides higher accuracy. The dashed lines show curves with low accuracy in some region and the high accuracy in others, while solid lines are improvements due to variations in →θ. Circles are manufacturer-provided data.
Parameter | vci | vinf | vr | Notes |
a | MUS | SUS | SUS | the upper asymptote as v→∞ |
b | SDS | CBS | MDS | |
c | DbS | IoD | IoD | shifts the curve horizontally while the shape remains the same |
d | SUS | SUS | NUS | |
g | SDS | CBS | NDS | |
ζ | MUS | CBF | NDS |
In Figure 4a, we see low accuracy near vci and vinf. The error is positive and tends to decrease the closer we get to vr, but turns negative at vr. In this case, increasing b substantially increases the accuracy since it shifts the curve downward near vci, moderately downward near vinf, and slightly shifts the curve upward near vr. In Figure 4b, we see a case where substantial errors occurring near vci but they disappear as the speed increases and accuracy is very high near vr. A suitable solution is to increase g, which shifts the curve downward with larger shifting occur closer to vci, but no effect at vr. Figure 4c shows a curve providing high accuracy near vci and vr. The error, however, to the left/right of vinf is negative/positive, with a slope greater than the slope of manufacturer-provided data. In this case, increasing ζ improves the accuracy by flattening the curve near vinf.
The previously mentioned examples demonstrate the flexibility of the 6PLEZ model and why it provides significant accuracy improvements. Our model adapts to improving the accuracy in some regions (near vci, vinf or vr) without sacrificing the accuracy in the others.
In this section, we devise some limits on the 6PLEZ model parameters (Eq 6), which help the STIR algorithm minimize the objective function (i.e., LSEs). We define the main limits on →θ as (the subscripts min and max denote the minimum and maximum limits of each parameter, respectively)
amin≤a≤amax,bmin≤b≤bmaxcmin≤c≤cmax,dmin≤d≤dmaxgmin≤g≤gmax,ζmin≤ζ≤ζmax |
To guarantee increasing monotonicity, the derivative of q(→θ,v) must be positive or zero. Thus, differentiating Eq 6 we get
g[b[vc]ζe−b(v−c)−ζ[vc]ζ−1e−b(v−c)c](a−d)(1+[vc]ζe−b(v−c))g+1≥0∀v≥0 | (7) |
g[[vc]ζe−b(v−c)(bv−ζ)](a−d)v(1+[vc]ζe−b(v−c))g+1≥0∀v≥0 | (8) |
The inequality of Eq 8 is satisfied when
a≥dMonotonicity Condition 1b≥0Monotonicity Condition 2c≥0Monotonicity Condition 3g≥0Monotonicity Condition 4ζ≤bvrMonotonicity Condition 5 |
These monotonicity conditions mean that Eqs 9–13 must be true
amin≥dmax | (9) |
bmin=0 | (10) |
cmin=0 | (11) |
gmin=0 | (12) |
ζmax,1=bmaxvr | (13) |
Taking the limit of Eq 6 as v→∞ yields
limv→∞q(→θ,v)=a | (14) |
Equation 14 is the exact value of the upper asymptote of Eq 6. However, in practice, v<∞, but since we still want to help the STIR algorithm estimate a such that q(→θ,v) still fits the manufacturer-provided data well, we allow the value of a to vary within ±10% of Pr, resulting in the conditions
amin=0.9Pr | (15) |
amax=1.1Pr | (16) |
Equation 6 must be greater than 0 for all v>0. Thus, a−d must always be positive. This requires that
dmax=0 | (17) |
Note that the conditions in Eqs 15 and 17 embody the condition in Eq 9.
[vc]ζe−b(v−c) in Eq 6 must start to substantially decrease at some point between vci and vr. In other words, as v increases, [vc]ζe−b(v−c) should become much smaller than one at some point between vci and vr. Otherwise, the curve would never approach the upper asymptote (which is almost equal to Pr) when v→vr. Thus, the inflection point c must be somewhere between vci and vr. We formulate the limits on c as follows
cmin=vci | (18) |
cmax=vr | (19) |
Note that the condition in Eq 18 embodies the condition in Eq 11. vinf (i.e., c) of an LF is the point at which a curve switches from concave upward to concave downward (i.e., the second derivative must be zero). Thus, taking the second derivative of Eq 6 with respect to v and equating it with zero
[vc]ζe−b(v−c)(−b2v2+2bvζ−ζ2+ζ)(1+[vc]ζe−b(v−c))g+1=−[vc]2ζe−2b(v−c)(ζ−bv)2(g+1)(1+[vc]ζe−b(v−c))g+2 | (20) |
Substituting v=c in Eq 20 and simplifying
b2c2+2gbcζ+ζ2=gb2c2+2bcζ+gζ2+2ζ | (21) |
then solving Eq 21 for g as Eq 22
g=ζ2−2bcζ+b2c2−2ζζ2−2bcζ+b2c2=1−2ζζ2−2bcζ+b2c2 | (22) |
by taking the limits for various extreme values of ζ, we obtain Eq 23
limζ→+∞g=1limζ→−∞g=1limζ→0+g=1limζ→0−g=1 | (23) |
this suggests that
gmax=1 | (24) |
We solve Eq 22 for ζ
ζ=1+bc(1−g)+√1+2bc(1−g)1−g | (25a) |
ζ=1+bc(1−g)−√1+2bc(1−g)1−g | (25b) |
Equations 25a and 25b mean for every bc (the product of the two parameters) and g values, there are (in general) two ζ values to satisfy the condition d2qe(→θ,v)dv2|v=vinf=0 (Eq 3). By plotting both equations in 3D for g∼[0,0.95] and bc∼[0,100], we see that the maximum value of ζ is 180. Thus, we say
ζmax,2=200 | (26) |
Equations 13 and 26 dictate the upper limits for ζ to satisfy the monotonicity and the inflection point existence conditions, respectively. Since we must satisfy both conditions, it follows that
ζmax=minof(ζmax,1,ζmax,2)=minof(bmaxvr,200) | (27) |
where 'minof' means the minimum of the two choices.
Equation 10, Eqs 12, 15–19 and 24 and 27 define nine constraints necessary for the STIR algorithm to quickly and reliably parameterize →θ in the 6PLEZ model (Eq 6). The three limits in which we did not derive analytically (or with reasoning) are: (1) bmax and (2) dmin, (3) ζmin.
We determined such limits by extensive simulations with the WT database of various ratings as follows: (1) bmax=3 and (2) dmin=−0.25Pr, (3) ζmin=−500. Table 2 shows a summary of the limits for the 6PLEZ logistic model.
Lower limits →θmin | Upper limits →θmax | How obtained | |||
a | 0.9Pr | Eq 15 | 1.1Pr | Eq 16 | LL: analytically, UL: analytically |
b | 0 | Eq 10 | 3 | LL: analytically, UL: observation | |
c | vci | Eq 18 | vr | Eq 19 | LL: proper reasoning, UL: proper reasoning |
d | −0.25Pr | 0 | Eq 17 | LL: observation, UL: analytically | |
g | 0 | Eq 12 | 1 | Eq 24 | LL: analytically, UL: analytically |
ζ | −500 | min(bmaxvr,200), Eq 27 | LL: observation, UL: proper reasoning | ||
Notice: LL: Lower Limit; UL: Upper Limit; →θmin: a vector of minimum values for elements of →θ; →θmax: a vector of maximum values for elements of →θ |
The main function to be minimized is the LSE between the fits of Eqs 4–6 and empirical power-speed data provided by the manufacturer. This is given as Eq 28:
f(→θk)=n∑i=1[qe(→θk,vi)−qm(vi)]2 | (28) |
where qe(→θk,vi) are curves of Eqs 4–6; vi, qm(vi) are the ith wind speed and wind power output data point as provided by the manufacturer, respectively; →θk is the parameters of vector θ in Eqs 4–6 at the kth iteration; and n is the number of data points in the GCR (region between vci and vr in 1) as provided by the manufacturer. Aldaoudeyeh et al. [8] discussed further details on the application of STIR algorithm for WTPSC curve fitting.
Compared to other optimization algorithms, advantages of the STIR algorithm include: 1. an efficiency in solving large bound-constrained minimization problems [22,23]; 2. An appeal for solving non-linear problems [22]; 3. An ability to handle convex, nonconvex, and ill-conditioned objective functions with large number of variables [24].
We fit →θ parameters using STIR algorithm as described by Aldaoudeyeh et al. [8]. MAE, MAPE, RMSE and NRMSE as defined in [6,25] are the statistical metrics we use to demonstrate the accuracy of our proposed model.
To ensure as a fair comparison as possible, we compare our work to the closest one. Villanueva and Feijóo [19] provide fits for 6PL model (Eq 4) for multiple WTs. We choose four of them for comparison purposes: (1) Enercon E82 E2; (2) Repower MM82; (3) Siemens S82 SWT-2.3 82; (4) Vestas V164/8000.
Figure 5 shows curve fits for the 6PL model (as estimated by Villanueva and Feijóo [19]) and the 6PLEZ model (as estimated in this work). Although both models contain the same number of parameters, our model (despite being a parametric one) fits the WTPSC curve near vci and vr. Such inaccuracy is reported for LFs (see Yan et al. [20], for example), but it does not occur in our model.
We test our model with 42 on-shore and off-shore WTs from 29 manufacturers. Ratings of the WTs range from 275 to 8000 kW. Tables 3 and 4 list the estimated →θ parameters, while Figures 6 and 7 show statistical metrics graphically. For convenience of presentation, we sorted WTs from with descending order of accuracy improvement. Clearly, the 6PLEZ model is either more accurate or at least as accurate as the 6PLE model.
WT model | Rating (kW) | Estimated parameters | |||||
a | b | c | d | g | ε | ||
Windtec WT1650df/77 | 1650 | 1576 | 1.615 | 10.24 | −141.9 | 0.2114 | 0.7440 |
Windtec WT3000fc/91 | 3000 | 2866 | 1.227 | 11.46 | −346.7 | 0.2175 | 0.6613 |
Windflow 45-500 | 500 | 492.3 | 1.313 | 10.42 | −93.27 | 0.2258 | 0.9368 |
Vestas V100/1800 | 1800 | 1720 | 2.008 | 9.215 | −408.7 | 0.1359 | 0.7640 |
Vestas V112/3000 | 3000 | 2934 | 0.8737 | 11.30 | −306.0 | 0.3378 | 0.8916 |
Vestas V164/8000 | 8000 | 7636 | 1.778 | 10.88 | −1290 | 0.1481 | 0.7417 |
Vergnet GEV MP R | 275 | 264.6 | 0.9909 | 10.59 | −33.34 | 0.3180 | 0.6362 |
Sinovel SL 3000/90 | 3000 | 2854 | 2.087 | 11.48 | −426.8 | 0.1204 | 0.6230 |
Siemens S82 SWT-2.3 82 | 2300 | 2219 | 0.8866 | 10.94 | −245.5 | 0.3493 | 0.8966 |
Siemens SWT-3.6-107 | 3600 | 3439 | 1.053 | 10.70 | −389.9 | 0.2975 | 0.8619 |
Shandong Swiss Electric YZ78/1.5 | 1500 | 1426 | 2.783 | 9.826 | −103.6 | 0.1308 | 0.6990 |
Repower MM82 | 2000 | 1928 | 1.413 | 11.17 | −314.6 | 0.1712 | 0.8553 |
Regen Powertech Vensys V70 | 1500 | 1478 | 1.295 | 10.90 | −116.4 | 0.2450 | 0.9323 |
Nordex S82 | 1500 | 1469 | 1.063 | 9.517 | −182.0 | 0.3317 | 0.9083 |
Nordex N90/2500 LS offshore | 2500 | 2420 | 1.126 | 10.77 | −344.0 | 0.2439 | 0.8179 |
Nordex N90/2300 | 2300 | 2191 | 1.416 | 10.86 | −519.4 | 0.1639 | 0.7269 |
Made AE-52 | 800 | 800.0 | 0.5824 | 9.994 | −20.67 | 0.7892 | 0.6719 |
M Torres MT TWT 82/1650 | 1650 | 1630 | 0.7061 | 9.226 | −127.8 | 0.5598 | 0.9359 |
Leitwind LTW70-1700 | 1700 | 1622 | 2.083 | 11.49 | −154.5 | 0.1341 | 0.6356 |
JSW J82 | 2000 | 1898 | 1.600 | 11.23 | −434.7 | 0.1321 | 0.6680 |
Inox Wind DF 100 | 2000 | 1896 | 1.783 | 8.547 | −240.2 | 0.1932 | 0.6884 |
IMPSA IWP-70-1500 | 1500 | 1430 | 2.277 | 11.12 | −189.9 | 0.1230 | 0.6919 |
Hyosung HS90-2MW | 2000 | 1915 | 1.292 | 10.01 | −193.7 | 0.2847 | 0.7484 |
Hyosung HS50-750kW | 750 | 715.3 | 3.427 | 11.30 | −86.71 | 0.07944 | 0.6020 |
Guodian United Power UP77 | 1500 | 1424 | 2.262 | 10.41 | −203.4 | 0.1215 | 0.6182 |
Guangdong Mingyang MY1.5s | 1500 | 1430 | 1.591 | 10.29 | −140.7 | 0.2071 | 0.7068 |
Global Wind Power GWP82-2000 | 2000 | 1987 | 0.7925 | 10.25 | −129.5 | 0.4587 | 0.9103 |
Gamesa G52/850 | 850 | 814.9 | 1.035 | 10.85 | −123.6 | 0.2623 | 0.8458 |
Fuhrlander FL MD/7 | 1525 | 1490 | 1.079 | 10.27 | −235.2 | 0.2643 | 0.8742 |
Eviag ev100 | 2500 | 2376 | 1.756 | 9.938 | −227.4 | 0.1954 | 0.6965 |
Eviag ev2.93 | 2050 | 2003 | 0.9065 | 9.172 | −134.4 | 0.5021 | 0.8590 |
Enercon E92/2350 | 2350 | 2345 | 0.9208 | 9.526 | −76.27 | 0.5096 | 0.9880 |
Enercon E82 E2 | 2050 | 2016 | 0.9338 | 9.669 | −66.77 | 0.4910 | 0.9254 |
Enercon E33/330 | 335 | 332.4 | 0.7343 | 9.298 | −12.29 | 0.6540 | 0.9220 |
Enercon E53/800 | 810 | 788.1 | 1.179 | 9.874 | −37.54 | 0.3385 | 0.9183 |
EWT DirectWind 52/750 | 750 | 739.1 | 0.9819 | 10.15 | −44.36 | 0.3776 | 0.9304 |
Doosan WinDS3000/91 | 3000 | 2870 | 1.759 | 10.92 | −77.35 | 0.2260 | 0.8047 |
Dewind D8.2 | 2000 | 1994 | 0.7215 | 7.917 | −114.9 | 0.8369 | 0.8851 |
Clipper Liberty C93 | 2500 | 2436 | 1.249 | 10.76 | −244.9 | 0.2505 | 0.9016 |
Clipper Liberty C100 | 2500 | 2480 | 1.099 | 10.22 | −235.1 | 0.3076 | 0.9526 |
Clipper Liberty C89 | 2500 | 2447 | 1.173 | 10.81 | −93.36 | 0.3307 | 0.9347 |
AVIC Huide HD2000 | 2050 | 1957 | 1.674 | 9.901 | −258.7 | 0.1947 | 0.7736 |
WT model | Rating (kW) | Estimated parameters | |||||
a | b | c | d | g | ζ | ||
Windtec WT1650df/77 | 1650 | 1686 | 1.750 | 10.42 | −175.5 | 0.2082 | 1.406 |
Windtec WT3000fc/91 | 3000 | 3107 | 0.01857 | 12.15 | −46.62 | 0.1391 | −19.87 |
Windflow 45-500 | 500 | 501.3 | 0.5517 | 10.64 | −45.75 | 0.2094 | −8.888 |
Vestas V100/1800 | 1800 | 1799 | 1.833 | 9.354 | −343.6 | 0.1394 | −1.672 |
Vestas V112/3000 | 3000 | 3005 | 0 | 13.33 | 30.21 | 0.04814 | −126.8 |
Vestas V164/8000 | 8000 | 8015 | 0.3718 | 11.24 | −365.5 | 0.1215 | −19.24 |
Vergnet GEV MP R | 275 | 282.8 | 0 | 11.62 | −6.191 | 0.08386 | −49.43 |
Sinovel SL 3000/90 | 3000 | 2999 | 0 | 12.02 | −9.974 | 0.04348 | −92.05 |
Siemens S82 SWT-2.3 82 | 2300 | 2319 | 0 | 12.47 | −16.08 | 0.1377 | −36.09 |
Siemens SWT-3.6-107 | 3600 | 3613 | 0 | 11.62 | −47.49 | 0.1707 | −23.59 |
Shandong Swiss Electric YZ78/1.5 | 1500 | 1498 | 1.343 | 10.05 | −19.66 | 0.1096 | −18.46 |
Repower MM82 | 2000 | 1989 | 1.264 | 11.30 | −242.6 | 0.1747 | −1.768 |
Regen Powertech Vensys V70 | 1500 | 1503 | 0.09158 | 11.36 | −13.24 | 0.1722 | −16.39 |
Nordex S82 | 1500 | 1525 | 0.2547 | 10.01 | −65.12 | 0.2620 | −9.131 |
Nordex N90/2500 LS offshore | 2500 | 2526 | 0 | 11.74 | −36.94 | 0.1087 | −36.07 |
Nordex N90/2300 | 2300 | 2328 | 0 | 11.38 | −128.3 | 0.1323 | −20.00 |
Made AE-52 | 800 | 800.3 | 0 | 11.71 | 13.59 | 0.01353 | −374.9 |
M Torres MT TWT 82/1650 | 1650 | 1693 | 0 | 12.31 | 1.248 | 0.08053 | −65.60 |
Leitwind LTW70-1700 | 1700 | 1701 | 0 | 12.03 | 4.671 | 0.03713 | −101.4 |
JSW J82 | 2000 | 2019 | 0.2879 | 11.67 | −98.75 | 0.1174 | −17.89 |
Inox Wind DF 100 | 2000 | 2027 | 0 | 9.076 | −13.55 | 0.1121 | −28.12 |
IMPSA IWP-70-1500 | 1500 | 1505 | 1.463 | 11.33 | −88.78 | 0.1188 | −10.56 |
Hyosung HS90-2MW | 2000 | 2000 | 0 | 11.10 | 2.642 | 0.02119 | −318.6 |
Hyosung HS50-750kW | 750 | 748.3 | 3.388 | 11.45 | −84.47 | 0.07969 | −0.4132 |
Guodian United Power UP77 | 1500 | 1499 | 0 | 10.95 | −10.07 | 0.03476 | −111.0 |
Guangdong Mingyang MY1.5s | 1500 | 1503 | 0 | 11.02 | −9.377 | 0.05691 | −77.47 |
Global Wind Power GWP82-2000 | 2000 | 2036 | 0 | 12.10 | −5.765 | 0.1168 | −38.87 |
Gamesa G52/850 | 850 | 850.0 | 0 | 12.86 | 14.89 | 0.02416 | −278.7 |
Fuhrlander FL MD/7 | 1525 | 1547 | 0 | 11.27 | −24.56 | 0.1325 | −31.33 |
Eviag ev100 | 2500 | 2507 | 0 | 10.55 | −14.82 | 0.07318 | −55.92 |
Eviag ev2.93 | 2050 | 2055 | 0 | 11.20 | 23.19 | 0.03325 | −227.5 |
Enercon E92/2350 | 2350 | 2361 | 0.3550 | 10.08 | −14.94 | 0.3588 | −6.829 |
Enercon E82 E2 | 2050 | 2096 | 0.4879 | 10.18 | −17.17 | 0.3736 | −5.488 |
Enercon E33/330 | 335 | 352.4 | 0.1004 | 10.38 | −1.293 | 0.3939 | −7.691 |
Enercon E53/800 | 810 | 811.2 | 0.4786 | 10.29 | −6.623 | 0.2568 | −8.688 |
EWT DirectWind 52/750 | 750 | 758.6 | 0 | 10.96 | −5.549 | 0.2220 | −13.92 |
Doosan WinDS3000/91 | 3000 | 3019 | 1.822 | 11.04 | −91.61 | 0.2271 | 0.7850 |
Dewind D8.2 | 2000 | 2200 | 0 | 10.89 | −12.30 | 0.1473 | −44.29 |
Clipper Liberty C93 | 2500 | 2507 | 1.128 | 10.87 | −194.4 | 0.2501 | −1.414 |
Clipper Liberty C100 | 2500 | 2522 | 0.3279 | 10.61 | −61.88 | 0.2478 | −9.311 |
Clipper Liberty C89 | 2500 | 2505 | 1.173 | 10.87 | −93.22 | 0.3308 | 0.001366 |
AVIC Huide HD2000 | 2050 | 2071 | 1.633 | 10.06 | −245.6 | 0.1954 | −0.4215 |
Table 6 shows four statistical metrics (MAE, RMSE, MAPE, and NRMSE) with their mean, median, maximum and minimum values for the entire database of WTs. Villanueva and Feijóo [21] provided classifications of the accuracy of WTPSC models depending on MAPE values. Such categories are summarized in Table 5. Tables 5 and 6 show that: (1) Both mean and median MAPE values for the 6PLE and 6PLEZ models fall in the 'very high' category. However, the 6PLEZ model has a median MAPE of 0.002383, which is less than half the 'very high' category threshold and (2) both mean and median NRMSE for the 6PLEZ model are 32.3% and 38.5% less than for the 6PLE model. This is a substantial accuracy improvement while maintaining the same number of parameters.
Accuracy level | MAPE range |
Very high | <0.005 |
High | 0.005–0.025 |
Medium | 0.025–0.1 |
Low | 0.1–0.15 |
Mean | Median | Max | Min | |
MAE | ||||
6PLE | 8.250 | 7.145 | 31.01 | 489.6×10−3 |
6PLEZ | 5.577 | 4.751 | 27.47 | 0.3737 |
RMSE | ||||
6PLE | 10.07 | 8.470 | 37.27 | 613.1×10−3 |
6PLEZ | 6.949 | 5.570 | 34.43 | 0.5241 |
MAPE | ||||
6PLE | 4.175×10−3 | 3.866×10−3 | 8.983×10−3 | 9.791×10−4 |
6PLEZ | 2.782×10−3 | 2.383×10−3 | 6.959×10−3 | 9.721×10−4 |
NRMSE | ||||
6PLE | 5.103×10−3 | 4.666×10−3 | 1.153×10−3 | 1.226×10−4 |
6PLEZ | 3.452×10−3 | 2.866×10−3 | 8.285×10−3 | 1.165×10−4 |
One limitation of the study is the use of manufacturer-provided data. Because of several factors (e.g., wind power curtailment, accumulation of dirt and snow, sensor failures, and pitch angle control malfunctioning [26,27,28]), the real power-speed data points may not be exactly the same as the ones provided by the manufacturer. In short, the data was characterized by noise and outliers. According to the IEC 61400-12-1 standard, outliers are highly weighted by the ordinary LSE regression method [29]. This conclusion should also pertain to most WTPSC fitting methods, as virtually all of them employ the LSE as an objective function. Pei and Li [11] confirm that outliers compromise the accuracy machine-learning WTPSC fitting methods.
When fitting using the manufacturer datasheet, the data points were obtained under controlled conditions. For example, the air mass density was set to 1.225 kg m−3, the wake effect was neglected (because only one WT was tested at a time), and the TI was assumed to be 10% [30]. The conditions in the actual WT location are usually not the same. The implementation of test condition control promoted fairness in comparing different WTPSC fitting models or estimation methods.
When fitting with real power-speed data, the air mass and TI may fluctuate for similar wind speed measurements, while the wake effect was greatly affected by the distance between WTs and their yaw angles. Additionally, several data cleaning techniques were devised in the literature (see [6,20,31]), which could be incorporated in future research. The amount of data we must remove, however, changes depends on the WT itself, making the entire data cleaning process susceptible to subjectivity [32], and sometimes outliers may still be present in the processed data [28].
In summary, although many papers addressed the effect of outliers on the accuracy of WTPSC models and the sensitivity of their parameters (e.g., [32,33]), the literature contained only few for LFs WTPSC models and most of them were in the last five years (e.g., [11,18,20]). Bilendo et al. [31] provide a recent review of WTPSC, but with no conclusive remarks on the effect of outliers on the performance of LFs WTPSC models. Thus, we suggest the following as future work:
1. Development of new robust LFs WTPSC curve fitting techniques (or objective functions) to guarantee model resiliency against outliers;
2. Incorporating additional parameters into the model to account for typical factors affecting outliers;
3. Examining the correlation between data filtering techniques and criteria and the accuracy of LFs WTPSC modeling;
4. Formulating probabilistic LFs WTPSC curves to accommodate fluctuations in instantaneous turbulence in wind speeds or enhanced LFs WTPSC to guarantee that the 10-minute averaged power closely approximates the empirical data.
In this paper, we proposed the 6PLEZ model (Eq 6). We then derived upper and lower limits for its parameters (2) and estimated them using the STIR algorithm. The significance of this paper is:
1. Our model adapts to improving accuracy in some WTPSC regions without sacrificing the accuracy in others (3.1);
2. Unlike most parametric approaches, the 6PLEZ model provides accurate WTPSC modeling near vci and vr (4.1);
3. The limits on the parameters of the 6PLEZ model are clearly specified, which:
(a) guarantee the monotonicity of the model (Eq 8);
(b) provide robust and reliable estimation of the parameters with no difficulties in 'guessing' their range (Table 2);
4. It provides substantial accuracy improvement over the 6PLE model despite having the same number of parameters as the 6PL and 6PLE models (Tables 5 and 6).
The authors declare they have not used Artificial Intelligence (AI) tools in the creation of this article.
The authors declare that they have no known competing financial interests or personal relationships that could have appeared to influence the work reported in this paper.
[1] | Global Wind Energy Council (2021) Global wind report 2021, Brussels: Global Wind Energy Council, 6–7. |
[2] |
Willis DJ, Niezrecki C, Kuchma D, et al. (2018) Wind energy research: State-of-the-art and future research directions. Renewable Energy 125: 133–154. https://doi.org/10.1016/j.renene.2018.02.049 doi: 10.1016/j.renene.2018.02.049
![]() |
[3] |
Hitaj C, Löschel A (2019) The impact of a feed-in tariff on wind power development in germany. Resour Energy Econ 57: 18–35. https://doi.org/10.1016/j.reseneeco.2018.12.001 doi: 10.1016/j.reseneeco.2018.12.001
![]() |
[4] |
Lin BQ, Chen YF (2019) Impacts of policies on innovation in wind power technologies in China. Appl Energy 247: 682–691. https://doi.org/10.1016/j.apenergy.2019.04.044 doi: 10.1016/j.apenergy.2019.04.044
![]() |
[5] |
Al Motasem IA, Alzaareer K (2020) Evaluating the accuracy of wind turbine power-speed characteristics fits for the generator control region. Int J Renewable Energy Res 10: 1031–1041. https://doi.org/10.20508/ijrer.v10i2.10955.g7975 doi: 10.20508/ijrer.v10i2.10955.g7975
![]() |
[6] |
Hu Y, Xi YH, Pan CY, et al. (2020) Daily condition monitoring of grid-connected wind turbine via high-fidelity power curve and its comprehensive rating. Renewable Energy 146: 2095–2111. https://doi.org/10.1016/j.renene.2019.08.043 doi: 10.1016/j.renene.2019.08.043
![]() |
[7] |
Shokrzadeh S, Jozani MJ, Bibeau E (2014) Wind turbine power curve modeling using advanced parametric and nonparametric methods. IEEE Trans Sustainable Energy 5: 1262–1269. https://doi.org/10.1109/TSTE.2014.2345059 doi: 10.1109/TSTE.2014.2345059
![]() |
[8] |
Aldaoudeyeh AM, Alzaareer K, Harasis S, et al. (2021) A new method to fit logistic functions with wind turbines power curves using manufacturer datasheets. IET Renewable Power Gener 16: 287–299. https://doi.org/10.1049/rpg2.12309 doi: 10.1049/rpg2.12309
![]() |
[9] |
Sohoni V, Gupta SC, Nema RK (2016) A critical review on wind turbine power curve modelling techniques and their applications in wind based energy systems. J Energy 2016: 1–18. https://doi.org/10.1155/2016/8519785 doi: 10.1155/2016/8519785
![]() |
[10] | Aldaoudeyeh AMI, Alzaareer K (2020) Statistical analysis of wind power using weibull distribution to maximize energy yield. 2020 IEEE PES/IAS PowerAfrica 2020: 1–5. |
[11] |
Pei S, Li Y (2019) Wind turbine power curve modeling with a hybrid machine learning technique. Appl Sci 9: 4930. https://doi.org/10.3390/app9224930 doi: 10.3390/app9224930
![]() |
[12] |
Kawano T, Wallbridge N, Plummer C (2020) Logistic models for simulating the growth of plants by defining the maximum plant size as the limit of information flow. Plant Signaling Behav 15: 1709718. https://doi.org/10.1080/15592324.2019.1709718 doi: 10.1080/15592324.2019.1709718
![]() |
[13] |
Rahimi I, Chen F, Gandomi AH (2021) A review on covid-19 forecasting models. Neural Comput Appl 35: 23671–23681. https://doi.org/10.1007/s00521-020-05626-8 doi: 10.1007/s00521-020-05626-8
![]() |
[14] |
Al-Hinai A, Charabi Y, Aghay Kaboli SH (2021) Offshore wind energy resource assessment across the territory of oman: A spatial-temporal data analysis. Sustainability 13: 2862. https://doi.org/10.3390/su13052862 doi: 10.3390/su13052862
![]() |
[15] |
Lydia M, Selvakumar AI, Kumar SS, et al. (2013) Advanced algorithms for wind turbine power curve modeling. IEEE Trans Sustainable Energy 4: 827–835. https://doi.org/10.1109/TSTE.2013.2247641 doi: 10.1109/TSTE.2013.2247641
![]() |
[16] |
Taslimi-Renani E, Modiri-Delshad M, Elias MFM, et al. (2016) Development of an enhanced parametric model for wind turbine power curve. Appl Energy 177: 544–552. https://doi.org/10.1016/j.apenergy.2016.05.124 doi: 10.1016/j.apenergy.2016.05.124
![]() |
[17] |
Zou R, Yang J, Wang Y, et al. (2021) Wind turbine power curve modeling using an asymmetric error characteristic-based loss function and a hybrid intelligent optimizer. Appl Energy 304: 117707. https://doi.org/10.1016/j.apenergy.2021.117707 doi: 10.1016/j.apenergy.2021.117707
![]() |
[18] |
Jing B, Qian Z, Zareipour H, et al. (2021) Wind turbine power curve modelling with logistic functions based on quantile regression. Appl Sci 11: 3048. https://doi.org/10.3390/app11073048 doi: 10.3390/app11073048
![]() |
[19] |
Villanueva D, Feijóo A (2018) Comparison of logistic functions for modeling wind turbine power curves. Electr Power Syst Res 155: 281–288. https://doi.org/10.1016/j.epsr.2017.10.028 doi: 10.1016/j.epsr.2017.10.028
![]() |
[20] |
Yan J, Zhang H, Liu Y, et al. (2019) Uncertainty estimation for wind energy conversion by probabilistic wind turbine power curve modelling. Appl Energy 239: 1356–1370. https://doi.org/10.1016/j.apenergy.2019.01.180 doi: 10.1016/j.apenergy.2019.01.180
![]() |
[21] |
Villanueva D, Feijóo A (2020) A review on wind turbine deterministic power curve models. Appl Sci 10: 4186. https://doi.org/10.3390/app10124186 doi: 10.3390/app10124186
![]() |
[22] |
Branch MA, Coleman TF, Li Y (1999) A subspace, interior, and conjugate gradient method for large-scale bound-constrained minimization problems. SIAM J Sci Comput 21: 1–23. https://doi.org/10.1137/S1064827595289108 doi: 10.1137/S1064827595289108
![]() |
[23] |
Conn AR, Gould NIM, Toint PL (1998) Global convergence of a class of trust region algorithms for optimization with simple bounds. SIAM J Numer Anal 25: 433–460. https://doi.org/10.1137/0725029 doi: 10.1137/0725029
![]() |
[24] |
Kamandi A, Amini K, Ahookhosh M (2016) An improved adaptive trust-region algorithm. Optim Lett 11: 555–569. https://doi.org/10.1007/s11590-016-1018-4 doi: 10.1007/s11590-016-1018-4
![]() |
[25] |
Villanueva D, Feijóo AE (2016) Reformulation of parameters of the logistic function applied to power curves of wind turbines. Electr Power Syst Res 137: 51–58. https://doi.org/10.1016/j.epsr.2016.03.045 doi: 10.1016/j.epsr.2016.03.045
![]() |
[26] |
Lapira E, Brisset D, Ardakani HD, et al. (2012) Wind turbine performance assessment using multi-regime modeling approach. Renewable Energy 45: 86–95. https://doi.org/10.1016/j.renene.2012.02.018 doi: 10.1016/j.renene.2012.02.018
![]() |
[27] |
Lin Z, Liu X (2020) Wind power forecasting of an offshore wind turbine based on high-frequency scada data and deep learning neural network. Energy 201: 117693. https://doi.org/10.1016/j.energy.2020.117693 doi: 10.1016/j.energy.2020.117693
![]() |
[28] |
Wang Y, Hu Q, Li L, et al. (2019) Approaches to wind power curve modeling: A review and discussion. Renewable Sustainable Energy Rev 116: 109422. https://doi.org/10.1016/j.rser.2019.109422 doi: 10.1016/j.rser.2019.109422
![]() |
[29] | International Electrotechnical Commission (2007) Wind energy generation systems–-Part 12-1: Power performance measurements of electricity producing wind turbines. International Electrotechnical Commission (IEC), IEC Central Office, 3: 2017-03. |
[30] |
Sunderland K, Woolmington T, Blackledge J, et al. (2013) Small wind turbines in turbulent (urban) environments: A consideration of normal and weibull distributions for power prediction. J Wind Eng Ind Aerodyn 121: 70–81. https://doi.org/10.1016/j.jweia.2013.08.001 doi: 10.1016/j.jweia.2013.08.001
![]() |
[31] |
Bilendo F, Meyer A, Badihi H, et al. (2022) Applications and modeling techniques of wind turbine power curve for wind farms–-A review. Energies 16: 180. https://doi.org/10.3390/en16010180 doi: 10.3390/en16010180
![]() |
[32] |
Mehrjoo M, Jozani MJ, Pawlak M (2020) Wind turbine power curve modeling for reliable power prediction using monotonic regression. Renewable Energy 147: 214–222. https://doi.org/10.1016/j.renene.2019.08.060 doi: 10.1016/j.renene.2019.08.060
![]() |
[33] |
Pelletier F, Masson C, Tahan A (2016) Wind turbine power curve modelling using artificial neural network. Renewable Energy 89: 207–214. https://doi.org/10.1016/j.renene.2015.11.065 doi: 10.1016/j.renene.2015.11.065
![]() |
Parameter | vci | vinf | vr | Notes |
a | MUS | SUS | SUS | the upper asymptote as v→∞ |
b | SDS | CBS | MDS | |
c | DbS | IoD | IoD | shifts the curve horizontally while the shape remains the same |
d | SUS | SUS | NUS | |
g | SDS | CBS | NDS | |
ζ | MUS | CBF | NDS |
Lower limits →θmin | Upper limits →θmax | How obtained | |||
a | 0.9Pr | Eq 15 | 1.1Pr | Eq 16 | LL: analytically, UL: analytically |
b | 0 | Eq 10 | 3 | LL: analytically, UL: observation | |
c | vci | Eq 18 | vr | Eq 19 | LL: proper reasoning, UL: proper reasoning |
d | −0.25Pr | 0 | Eq 17 | LL: observation, UL: analytically | |
g | 0 | Eq 12 | 1 | Eq 24 | LL: analytically, UL: analytically |
ζ | −500 | min(bmaxvr,200), Eq 27 | LL: observation, UL: proper reasoning | ||
Notice: LL: Lower Limit; UL: Upper Limit; →θmin: a vector of minimum values for elements of →θ; →θmax: a vector of maximum values for elements of →θ |
WT model | Rating (kW) | Estimated parameters | |||||
a | b | c | d | g | ε | ||
Windtec WT1650df/77 | 1650 | 1576 | 1.615 | 10.24 | −141.9 | 0.2114 | 0.7440 |
Windtec WT3000fc/91 | 3000 | 2866 | 1.227 | 11.46 | −346.7 | 0.2175 | 0.6613 |
Windflow 45-500 | 500 | 492.3 | 1.313 | 10.42 | −93.27 | 0.2258 | 0.9368 |
Vestas V100/1800 | 1800 | 1720 | 2.008 | 9.215 | −408.7 | 0.1359 | 0.7640 |
Vestas V112/3000 | 3000 | 2934 | 0.8737 | 11.30 | −306.0 | 0.3378 | 0.8916 |
Vestas V164/8000 | 8000 | 7636 | 1.778 | 10.88 | −1290 | 0.1481 | 0.7417 |
Vergnet GEV MP R | 275 | 264.6 | 0.9909 | 10.59 | −33.34 | 0.3180 | 0.6362 |
Sinovel SL 3000/90 | 3000 | 2854 | 2.087 | 11.48 | −426.8 | 0.1204 | 0.6230 |
Siemens S82 SWT-2.3 82 | 2300 | 2219 | 0.8866 | 10.94 | −245.5 | 0.3493 | 0.8966 |
Siemens SWT-3.6-107 | 3600 | 3439 | 1.053 | 10.70 | −389.9 | 0.2975 | 0.8619 |
Shandong Swiss Electric YZ78/1.5 | 1500 | 1426 | 2.783 | 9.826 | −103.6 | 0.1308 | 0.6990 |
Repower MM82 | 2000 | 1928 | 1.413 | 11.17 | −314.6 | 0.1712 | 0.8553 |
Regen Powertech Vensys V70 | 1500 | 1478 | 1.295 | 10.90 | −116.4 | 0.2450 | 0.9323 |
Nordex S82 | 1500 | 1469 | 1.063 | 9.517 | −182.0 | 0.3317 | 0.9083 |
Nordex N90/2500 LS offshore | 2500 | 2420 | 1.126 | 10.77 | −344.0 | 0.2439 | 0.8179 |
Nordex N90/2300 | 2300 | 2191 | 1.416 | 10.86 | −519.4 | 0.1639 | 0.7269 |
Made AE-52 | 800 | 800.0 | 0.5824 | 9.994 | −20.67 | 0.7892 | 0.6719 |
M Torres MT TWT 82/1650 | 1650 | 1630 | 0.7061 | 9.226 | −127.8 | 0.5598 | 0.9359 |
Leitwind LTW70-1700 | 1700 | 1622 | 2.083 | 11.49 | −154.5 | 0.1341 | 0.6356 |
JSW J82 | 2000 | 1898 | 1.600 | 11.23 | −434.7 | 0.1321 | 0.6680 |
Inox Wind DF 100 | 2000 | 1896 | 1.783 | 8.547 | −240.2 | 0.1932 | 0.6884 |
IMPSA IWP-70-1500 | 1500 | 1430 | 2.277 | 11.12 | −189.9 | 0.1230 | 0.6919 |
Hyosung HS90-2MW | 2000 | 1915 | 1.292 | 10.01 | −193.7 | 0.2847 | 0.7484 |
Hyosung HS50-750kW | 750 | 715.3 | 3.427 | 11.30 | −86.71 | 0.07944 | 0.6020 |
Guodian United Power UP77 | 1500 | 1424 | 2.262 | 10.41 | −203.4 | 0.1215 | 0.6182 |
Guangdong Mingyang MY1.5s | 1500 | 1430 | 1.591 | 10.29 | −140.7 | 0.2071 | 0.7068 |
Global Wind Power GWP82-2000 | 2000 | 1987 | 0.7925 | 10.25 | −129.5 | 0.4587 | 0.9103 |
Gamesa G52/850 | 850 | 814.9 | 1.035 | 10.85 | −123.6 | 0.2623 | 0.8458 |
Fuhrlander FL MD/7 | 1525 | 1490 | 1.079 | 10.27 | −235.2 | 0.2643 | 0.8742 |
Eviag ev100 | 2500 | 2376 | 1.756 | 9.938 | −227.4 | 0.1954 | 0.6965 |
Eviag ev2.93 | 2050 | 2003 | 0.9065 | 9.172 | −134.4 | 0.5021 | 0.8590 |
Enercon E92/2350 | 2350 | 2345 | 0.9208 | 9.526 | −76.27 | 0.5096 | 0.9880 |
Enercon E82 E2 | 2050 | 2016 | 0.9338 | 9.669 | −66.77 | 0.4910 | 0.9254 |
Enercon E33/330 | 335 | 332.4 | 0.7343 | 9.298 | −12.29 | 0.6540 | 0.9220 |
Enercon E53/800 | 810 | 788.1 | 1.179 | 9.874 | −37.54 | 0.3385 | 0.9183 |
EWT DirectWind 52/750 | 750 | 739.1 | 0.9819 | 10.15 | −44.36 | 0.3776 | 0.9304 |
Doosan WinDS3000/91 | 3000 | 2870 | 1.759 | 10.92 | −77.35 | 0.2260 | 0.8047 |
Dewind D8.2 | 2000 | 1994 | 0.7215 | 7.917 | −114.9 | 0.8369 | 0.8851 |
Clipper Liberty C93 | 2500 | 2436 | 1.249 | 10.76 | −244.9 | 0.2505 | 0.9016 |
Clipper Liberty C100 | 2500 | 2480 | 1.099 | 10.22 | −235.1 | 0.3076 | 0.9526 |
Clipper Liberty C89 | 2500 | 2447 | 1.173 | 10.81 | −93.36 | 0.3307 | 0.9347 |
AVIC Huide HD2000 | 2050 | 1957 | 1.674 | 9.901 | −258.7 | 0.1947 | 0.7736 |
WT model | Rating (kW) | Estimated parameters | |||||
a | b | c | d | g | ζ | ||
Windtec WT1650df/77 | 1650 | 1686 | 1.750 | 10.42 | −175.5 | 0.2082 | 1.406 |
Windtec WT3000fc/91 | 3000 | 3107 | 0.01857 | 12.15 | −46.62 | 0.1391 | −19.87 |
Windflow 45-500 | 500 | 501.3 | 0.5517 | 10.64 | −45.75 | 0.2094 | −8.888 |
Vestas V100/1800 | 1800 | 1799 | 1.833 | 9.354 | −343.6 | 0.1394 | −1.672 |
Vestas V112/3000 | 3000 | 3005 | 0 | 13.33 | 30.21 | 0.04814 | −126.8 |
Vestas V164/8000 | 8000 | 8015 | 0.3718 | 11.24 | −365.5 | 0.1215 | −19.24 |
Vergnet GEV MP R | 275 | 282.8 | 0 | 11.62 | −6.191 | 0.08386 | −49.43 |
Sinovel SL 3000/90 | 3000 | 2999 | 0 | 12.02 | −9.974 | 0.04348 | −92.05 |
Siemens S82 SWT-2.3 82 | 2300 | 2319 | 0 | 12.47 | −16.08 | 0.1377 | −36.09 |
Siemens SWT-3.6-107 | 3600 | 3613 | 0 | 11.62 | −47.49 | 0.1707 | −23.59 |
Shandong Swiss Electric YZ78/1.5 | 1500 | 1498 | 1.343 | 10.05 | −19.66 | 0.1096 | −18.46 |
Repower MM82 | 2000 | 1989 | 1.264 | 11.30 | −242.6 | 0.1747 | −1.768 |
Regen Powertech Vensys V70 | 1500 | 1503 | 0.09158 | 11.36 | −13.24 | 0.1722 | −16.39 |
Nordex S82 | 1500 | 1525 | 0.2547 | 10.01 | −65.12 | 0.2620 | −9.131 |
Nordex N90/2500 LS offshore | 2500 | 2526 | 0 | 11.74 | −36.94 | 0.1087 | −36.07 |
Nordex N90/2300 | 2300 | 2328 | 0 | 11.38 | −128.3 | 0.1323 | −20.00 |
Made AE-52 | 800 | 800.3 | 0 | 11.71 | 13.59 | 0.01353 | −374.9 |
M Torres MT TWT 82/1650 | 1650 | 1693 | 0 | 12.31 | 1.248 | 0.08053 | −65.60 |
Leitwind LTW70-1700 | 1700 | 1701 | 0 | 12.03 | 4.671 | 0.03713 | −101.4 |
JSW J82 | 2000 | 2019 | 0.2879 | 11.67 | −98.75 | 0.1174 | −17.89 |
Inox Wind DF 100 | 2000 | 2027 | 0 | 9.076 | −13.55 | 0.1121 | −28.12 |
IMPSA IWP-70-1500 | 1500 | 1505 | 1.463 | 11.33 | −88.78 | 0.1188 | −10.56 |
Hyosung HS90-2MW | 2000 | 2000 | 0 | 11.10 | 2.642 | 0.02119 | −318.6 |
Hyosung HS50-750kW | 750 | 748.3 | 3.388 | 11.45 | −84.47 | 0.07969 | −0.4132 |
Guodian United Power UP77 | 1500 | 1499 | 0 | 10.95 | −10.07 | 0.03476 | −111.0 |
Guangdong Mingyang MY1.5s | 1500 | 1503 | 0 | 11.02 | −9.377 | 0.05691 | −77.47 |
Global Wind Power GWP82-2000 | 2000 | 2036 | 0 | 12.10 | −5.765 | 0.1168 | −38.87 |
Gamesa G52/850 | 850 | 850.0 | 0 | 12.86 | 14.89 | 0.02416 | −278.7 |
Fuhrlander FL MD/7 | 1525 | 1547 | 0 | 11.27 | −24.56 | 0.1325 | −31.33 |
Eviag ev100 | 2500 | 2507 | 0 | 10.55 | −14.82 | 0.07318 | −55.92 |
Eviag ev2.93 | 2050 | 2055 | 0 | 11.20 | 23.19 | 0.03325 | −227.5 |
Enercon E92/2350 | 2350 | 2361 | 0.3550 | 10.08 | −14.94 | 0.3588 | −6.829 |
Enercon E82 E2 | 2050 | 2096 | 0.4879 | 10.18 | −17.17 | 0.3736 | −5.488 |
Enercon E33/330 | 335 | 352.4 | 0.1004 | 10.38 | −1.293 | 0.3939 | −7.691 |
Enercon E53/800 | 810 | 811.2 | 0.4786 | 10.29 | −6.623 | 0.2568 | −8.688 |
EWT DirectWind 52/750 | 750 | 758.6 | 0 | 10.96 | −5.549 | 0.2220 | −13.92 |
Doosan WinDS3000/91 | 3000 | 3019 | 1.822 | 11.04 | −91.61 | 0.2271 | 0.7850 |
Dewind D8.2 | 2000 | 2200 | 0 | 10.89 | −12.30 | 0.1473 | −44.29 |
Clipper Liberty C93 | 2500 | 2507 | 1.128 | 10.87 | −194.4 | 0.2501 | −1.414 |
Clipper Liberty C100 | 2500 | 2522 | 0.3279 | 10.61 | −61.88 | 0.2478 | −9.311 |
Clipper Liberty C89 | 2500 | 2505 | 1.173 | 10.87 | −93.22 | 0.3308 | 0.001366 |
AVIC Huide HD2000 | 2050 | 2071 | 1.633 | 10.06 | −245.6 | 0.1954 | −0.4215 |
Accuracy level | MAPE range |
Very high | <0.005 |
High | 0.005–0.025 |
Medium | 0.025–0.1 |
Low | 0.1–0.15 |
Mean | Median | Max | Min | |
MAE | ||||
6PLE | 8.250 | 7.145 | 31.01 | 489.6×10−3 |
6PLEZ | 5.577 | 4.751 | 27.47 | 0.3737 |
RMSE | ||||
6PLE | 10.07 | 8.470 | 37.27 | 613.1×10−3 |
6PLEZ | 6.949 | 5.570 | 34.43 | 0.5241 |
MAPE | ||||
6PLE | 4.175×10−3 | 3.866×10−3 | 8.983×10−3 | 9.791×10−4 |
6PLEZ | 2.782×10−3 | 2.383×10−3 | 6.959×10−3 | 9.721×10−4 |
NRMSE | ||||
6PLE | 5.103×10−3 | 4.666×10−3 | 1.153×10−3 | 1.226×10−4 |
6PLEZ | 3.452×10−3 | 2.866×10−3 | 8.285×10−3 | 1.165×10−4 |
Parameter | vci | vinf | vr | Notes |
a | MUS | SUS | SUS | the upper asymptote as v→∞ |
b | SDS | CBS | MDS | |
c | DbS | IoD | IoD | shifts the curve horizontally while the shape remains the same |
d | SUS | SUS | NUS | |
g | SDS | CBS | NDS | |
ζ | MUS | CBF | NDS |
Lower limits →θmin | Upper limits →θmax | How obtained | |||
a | 0.9Pr | Eq 15 | 1.1Pr | Eq 16 | LL: analytically, UL: analytically |
b | 0 | Eq 10 | 3 | LL: analytically, UL: observation | |
c | vci | Eq 18 | vr | Eq 19 | LL: proper reasoning, UL: proper reasoning |
d | −0.25Pr | 0 | Eq 17 | LL: observation, UL: analytically | |
g | 0 | Eq 12 | 1 | Eq 24 | LL: analytically, UL: analytically |
ζ | −500 | min(bmaxvr,200), Eq 27 | LL: observation, UL: proper reasoning | ||
Notice: LL: Lower Limit; UL: Upper Limit; →θmin: a vector of minimum values for elements of →θ; →θmax: a vector of maximum values for elements of →θ |
WT model | Rating (kW) | Estimated parameters | |||||
a | b | c | d | g | ε | ||
Windtec WT1650df/77 | 1650 | 1576 | 1.615 | 10.24 | −141.9 | 0.2114 | 0.7440 |
Windtec WT3000fc/91 | 3000 | 2866 | 1.227 | 11.46 | −346.7 | 0.2175 | 0.6613 |
Windflow 45-500 | 500 | 492.3 | 1.313 | 10.42 | −93.27 | 0.2258 | 0.9368 |
Vestas V100/1800 | 1800 | 1720 | 2.008 | 9.215 | −408.7 | 0.1359 | 0.7640 |
Vestas V112/3000 | 3000 | 2934 | 0.8737 | 11.30 | −306.0 | 0.3378 | 0.8916 |
Vestas V164/8000 | 8000 | 7636 | 1.778 | 10.88 | −1290 | 0.1481 | 0.7417 |
Vergnet GEV MP R | 275 | 264.6 | 0.9909 | 10.59 | −33.34 | 0.3180 | 0.6362 |
Sinovel SL 3000/90 | 3000 | 2854 | 2.087 | 11.48 | −426.8 | 0.1204 | 0.6230 |
Siemens S82 SWT-2.3 82 | 2300 | 2219 | 0.8866 | 10.94 | −245.5 | 0.3493 | 0.8966 |
Siemens SWT-3.6-107 | 3600 | 3439 | 1.053 | 10.70 | −389.9 | 0.2975 | 0.8619 |
Shandong Swiss Electric YZ78/1.5 | 1500 | 1426 | 2.783 | 9.826 | −103.6 | 0.1308 | 0.6990 |
Repower MM82 | 2000 | 1928 | 1.413 | 11.17 | −314.6 | 0.1712 | 0.8553 |
Regen Powertech Vensys V70 | 1500 | 1478 | 1.295 | 10.90 | −116.4 | 0.2450 | 0.9323 |
Nordex S82 | 1500 | 1469 | 1.063 | 9.517 | −182.0 | 0.3317 | 0.9083 |
Nordex N90/2500 LS offshore | 2500 | 2420 | 1.126 | 10.77 | −344.0 | 0.2439 | 0.8179 |
Nordex N90/2300 | 2300 | 2191 | 1.416 | 10.86 | −519.4 | 0.1639 | 0.7269 |
Made AE-52 | 800 | 800.0 | 0.5824 | 9.994 | −20.67 | 0.7892 | 0.6719 |
M Torres MT TWT 82/1650 | 1650 | 1630 | 0.7061 | 9.226 | −127.8 | 0.5598 | 0.9359 |
Leitwind LTW70-1700 | 1700 | 1622 | 2.083 | 11.49 | −154.5 | 0.1341 | 0.6356 |
JSW J82 | 2000 | 1898 | 1.600 | 11.23 | −434.7 | 0.1321 | 0.6680 |
Inox Wind DF 100 | 2000 | 1896 | 1.783 | 8.547 | −240.2 | 0.1932 | 0.6884 |
IMPSA IWP-70-1500 | 1500 | 1430 | 2.277 | 11.12 | −189.9 | 0.1230 | 0.6919 |
Hyosung HS90-2MW | 2000 | 1915 | 1.292 | 10.01 | −193.7 | 0.2847 | 0.7484 |
Hyosung HS50-750kW | 750 | 715.3 | 3.427 | 11.30 | −86.71 | 0.07944 | 0.6020 |
Guodian United Power UP77 | 1500 | 1424 | 2.262 | 10.41 | −203.4 | 0.1215 | 0.6182 |
Guangdong Mingyang MY1.5s | 1500 | 1430 | 1.591 | 10.29 | −140.7 | 0.2071 | 0.7068 |
Global Wind Power GWP82-2000 | 2000 | 1987 | 0.7925 | 10.25 | −129.5 | 0.4587 | 0.9103 |
Gamesa G52/850 | 850 | 814.9 | 1.035 | 10.85 | −123.6 | 0.2623 | 0.8458 |
Fuhrlander FL MD/7 | 1525 | 1490 | 1.079 | 10.27 | −235.2 | 0.2643 | 0.8742 |
Eviag ev100 | 2500 | 2376 | 1.756 | 9.938 | −227.4 | 0.1954 | 0.6965 |
Eviag ev2.93 | 2050 | 2003 | 0.9065 | 9.172 | −134.4 | 0.5021 | 0.8590 |
Enercon E92/2350 | 2350 | 2345 | 0.9208 | 9.526 | −76.27 | 0.5096 | 0.9880 |
Enercon E82 E2 | 2050 | 2016 | 0.9338 | 9.669 | −66.77 | 0.4910 | 0.9254 |
Enercon E33/330 | 335 | 332.4 | 0.7343 | 9.298 | −12.29 | 0.6540 | 0.9220 |
Enercon E53/800 | 810 | 788.1 | 1.179 | 9.874 | −37.54 | 0.3385 | 0.9183 |
EWT DirectWind 52/750 | 750 | 739.1 | 0.9819 | 10.15 | −44.36 | 0.3776 | 0.9304 |
Doosan WinDS3000/91 | 3000 | 2870 | 1.759 | 10.92 | −77.35 | 0.2260 | 0.8047 |
Dewind D8.2 | 2000 | 1994 | 0.7215 | 7.917 | −114.9 | 0.8369 | 0.8851 |
Clipper Liberty C93 | 2500 | 2436 | 1.249 | 10.76 | −244.9 | 0.2505 | 0.9016 |
Clipper Liberty C100 | 2500 | 2480 | 1.099 | 10.22 | −235.1 | 0.3076 | 0.9526 |
Clipper Liberty C89 | 2500 | 2447 | 1.173 | 10.81 | −93.36 | 0.3307 | 0.9347 |
AVIC Huide HD2000 | 2050 | 1957 | 1.674 | 9.901 | −258.7 | 0.1947 | 0.7736 |
WT model | Rating (kW) | Estimated parameters | |||||
a | b | c | d | g | ζ | ||
Windtec WT1650df/77 | 1650 | 1686 | 1.750 | 10.42 | −175.5 | 0.2082 | 1.406 |
Windtec WT3000fc/91 | 3000 | 3107 | 0.01857 | 12.15 | −46.62 | 0.1391 | −19.87 |
Windflow 45-500 | 500 | 501.3 | 0.5517 | 10.64 | −45.75 | 0.2094 | −8.888 |
Vestas V100/1800 | 1800 | 1799 | 1.833 | 9.354 | −343.6 | 0.1394 | −1.672 |
Vestas V112/3000 | 3000 | 3005 | 0 | 13.33 | 30.21 | 0.04814 | −126.8 |
Vestas V164/8000 | 8000 | 8015 | 0.3718 | 11.24 | −365.5 | 0.1215 | −19.24 |
Vergnet GEV MP R | 275 | 282.8 | 0 | 11.62 | −6.191 | 0.08386 | −49.43 |
Sinovel SL 3000/90 | 3000 | 2999 | 0 | 12.02 | −9.974 | 0.04348 | −92.05 |
Siemens S82 SWT-2.3 82 | 2300 | 2319 | 0 | 12.47 | −16.08 | 0.1377 | −36.09 |
Siemens SWT-3.6-107 | 3600 | 3613 | 0 | 11.62 | −47.49 | 0.1707 | −23.59 |
Shandong Swiss Electric YZ78/1.5 | 1500 | 1498 | 1.343 | 10.05 | −19.66 | 0.1096 | −18.46 |
Repower MM82 | 2000 | 1989 | 1.264 | 11.30 | −242.6 | 0.1747 | −1.768 |
Regen Powertech Vensys V70 | 1500 | 1503 | 0.09158 | 11.36 | −13.24 | 0.1722 | −16.39 |
Nordex S82 | 1500 | 1525 | 0.2547 | 10.01 | −65.12 | 0.2620 | −9.131 |
Nordex N90/2500 LS offshore | 2500 | 2526 | 0 | 11.74 | −36.94 | 0.1087 | −36.07 |
Nordex N90/2300 | 2300 | 2328 | 0 | 11.38 | −128.3 | 0.1323 | −20.00 |
Made AE-52 | 800 | 800.3 | 0 | 11.71 | 13.59 | 0.01353 | −374.9 |
M Torres MT TWT 82/1650 | 1650 | 1693 | 0 | 12.31 | 1.248 | 0.08053 | −65.60 |
Leitwind LTW70-1700 | 1700 | 1701 | 0 | 12.03 | 4.671 | 0.03713 | −101.4 |
JSW J82 | 2000 | 2019 | 0.2879 | 11.67 | −98.75 | 0.1174 | −17.89 |
Inox Wind DF 100 | 2000 | 2027 | 0 | 9.076 | −13.55 | 0.1121 | −28.12 |
IMPSA IWP-70-1500 | 1500 | 1505 | 1.463 | 11.33 | −88.78 | 0.1188 | −10.56 |
Hyosung HS90-2MW | 2000 | 2000 | 0 | 11.10 | 2.642 | 0.02119 | −318.6 |
Hyosung HS50-750kW | 750 | 748.3 | 3.388 | 11.45 | −84.47 | 0.07969 | −0.4132 |
Guodian United Power UP77 | 1500 | 1499 | 0 | 10.95 | −10.07 | 0.03476 | −111.0 |
Guangdong Mingyang MY1.5s | 1500 | 1503 | 0 | 11.02 | −9.377 | 0.05691 | −77.47 |
Global Wind Power GWP82-2000 | 2000 | 2036 | 0 | 12.10 | −5.765 | 0.1168 | −38.87 |
Gamesa G52/850 | 850 | 850.0 | 0 | 12.86 | 14.89 | 0.02416 | −278.7 |
Fuhrlander FL MD/7 | 1525 | 1547 | 0 | 11.27 | −24.56 | 0.1325 | −31.33 |
Eviag ev100 | 2500 | 2507 | 0 | 10.55 | −14.82 | 0.07318 | −55.92 |
Eviag ev2.93 | 2050 | 2055 | 0 | 11.20 | 23.19 | 0.03325 | −227.5 |
Enercon E92/2350 | 2350 | 2361 | 0.3550 | 10.08 | −14.94 | 0.3588 | −6.829 |
Enercon E82 E2 | 2050 | 2096 | 0.4879 | 10.18 | −17.17 | 0.3736 | −5.488 |
Enercon E33/330 | 335 | 352.4 | 0.1004 | 10.38 | −1.293 | 0.3939 | −7.691 |
Enercon E53/800 | 810 | 811.2 | 0.4786 | 10.29 | −6.623 | 0.2568 | −8.688 |
EWT DirectWind 52/750 | 750 | 758.6 | 0 | 10.96 | −5.549 | 0.2220 | −13.92 |
Doosan WinDS3000/91 | 3000 | 3019 | 1.822 | 11.04 | −91.61 | 0.2271 | 0.7850 |
Dewind D8.2 | 2000 | 2200 | 0 | 10.89 | −12.30 | 0.1473 | −44.29 |
Clipper Liberty C93 | 2500 | 2507 | 1.128 | 10.87 | −194.4 | 0.2501 | −1.414 |
Clipper Liberty C100 | 2500 | 2522 | 0.3279 | 10.61 | −61.88 | 0.2478 | −9.311 |
Clipper Liberty C89 | 2500 | 2505 | 1.173 | 10.87 | −93.22 | 0.3308 | 0.001366 |
AVIC Huide HD2000 | 2050 | 2071 | 1.633 | 10.06 | −245.6 | 0.1954 | −0.4215 |
Accuracy level | MAPE range |
Very high | <0.005 |
High | 0.005–0.025 |
Medium | 0.025–0.1 |
Low | 0.1–0.15 |
Mean | Median | Max | Min | |
MAE | ||||
6PLE | 8.250 | 7.145 | 31.01 | 489.6×10−3 |
6PLEZ | 5.577 | 4.751 | 27.47 | 0.3737 |
RMSE | ||||
6PLE | 10.07 | 8.470 | 37.27 | 613.1×10−3 |
6PLEZ | 6.949 | 5.570 | 34.43 | 0.5241 |
MAPE | ||||
6PLE | 4.175×10−3 | 3.866×10−3 | 8.983×10−3 | 9.791×10−4 |
6PLEZ | 2.782×10−3 | 2.383×10−3 | 6.959×10−3 | 9.721×10−4 |
NRMSE | ||||
6PLE | 5.103×10−3 | 4.666×10−3 | 1.153×10−3 | 1.226×10−4 |
6PLEZ | 3.452×10−3 | 2.866×10−3 | 8.285×10−3 | 1.165×10−4 |