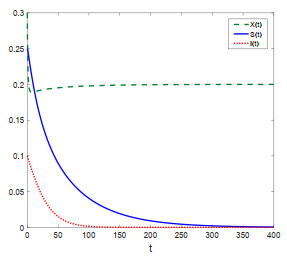
Citation: Juliet Lee. Insights into cell motility provided by the iterative use of mathematical modeling and experimentation[J]. AIMS Biophysics, 2018, 5(2): 97-124. doi: 10.3934/biophy.2018.2.97
[1] | Kawkab Al Amri, Qamar J. A Khan, David Greenhalgh . Combined impact of fear and Allee effect in predator-prey interaction models on their growth. Mathematical Biosciences and Engineering, 2024, 21(10): 7211-7252. doi: 10.3934/mbe.2024319 |
[2] | Saheb Pal, Nikhil Pal, Sudip Samanta, Joydev Chattopadhyay . Fear effect in prey and hunting cooperation among predators in a Leslie-Gower model. Mathematical Biosciences and Engineering, 2019, 16(5): 5146-5179. doi: 10.3934/mbe.2019258 |
[3] | Dirk Stiefs, Ezio Venturino, Ulrike Feudel . Evidence of chaos in eco-epidemic models. Mathematical Biosciences and Engineering, 2009, 6(4): 855-871. doi: 10.3934/mbe.2009.6.855 |
[4] | Yuhong Huo, Gourav Mandal, Lakshmi Narayan Guin, Santabrata Chakravarty, Renji Han . Allee effect-driven complexity in a spatiotemporal predator-prey system with fear factor. Mathematical Biosciences and Engineering, 2023, 20(10): 18820-18860. doi: 10.3934/mbe.2023834 |
[5] | Yuanfu Shao . Bifurcations of a delayed predator-prey system with fear, refuge for prey and additional food for predator. Mathematical Biosciences and Engineering, 2023, 20(4): 7429-7452. doi: 10.3934/mbe.2023322 |
[6] | Hongqiuxue Wu, Zhong Li, Mengxin He . Dynamic analysis of a Leslie-Gower predator-prey model with the fear effect and nonlinear harvesting. Mathematical Biosciences and Engineering, 2023, 20(10): 18592-18629. doi: 10.3934/mbe.2023825 |
[7] | Ranjit Kumar Upadhyay, Swati Mishra . Population dynamic consequences of fearful prey in a spatiotemporal predator-prey system. Mathematical Biosciences and Engineering, 2019, 16(1): 338-372. doi: 10.3934/mbe.2019017 |
[8] | Shunyi Li . Hopf bifurcation, stability switches and chaos in a prey-predator system with three stage structure and two time delays. Mathematical Biosciences and Engineering, 2019, 16(6): 6934-6961. doi: 10.3934/mbe.2019348 |
[9] | Rongjie Yu, Hengguo Yu, Chuanjun Dai, Zengling Ma, Qi Wang, Min Zhao . Bifurcation analysis of Leslie-Gower predator-prey system with harvesting and fear effect. Mathematical Biosciences and Engineering, 2023, 20(10): 18267-18300. doi: 10.3934/mbe.2023812 |
[10] | Wanxiao Xu, Ping Jiang, Hongying Shu, Shanshan Tong . Modeling the fear effect in the predator-prey dynamics with an age structure in the predators. Mathematical Biosciences and Engineering, 2023, 20(7): 12625-12648. doi: 10.3934/mbe.2023562 |
The effect of disease on eco-epidemiology system is a significant topic from both mathematical and ecological perspectives. The disease factor usually leads to a more complex and diverting dynamics than those in the disease-free system [1,2]. Within the interactions between predator and prey, the disease could only spread in prey or predator population, also could spread between prey and predator [3,4,5]. Birds (particularly pelicans) infect vibrio and die by preying on vibrio-infected fish (particularly tilapia) at the Salton Sea in the desert of Southern California [3], which is an example of disease spreads amongst the prey. For the disease in predator, taking fox rabies as an example, foxes (Vulpis) infect rabies and transmit to other foxes or their prey rabbits by biting in Europe and North America [6]. More relevant examples could be found in [7]. From the mathematical epidemiology point of view, one needs much more attention in the dynamics of infected predator to observe whether the presence of the prey allows the survival of a part of the predator population [8].
A variety of diseased predator models have been proposed to study the complex interaction between prey and predator with infected diseases [2,9,10] and the reference therein. Most common epidemic model applied in predator-prey interactions is the $ SI $-type, i.e., the predator population $ Y(t) $ is divided into two sub-classes, namely susceptible predator $ S(t) $ and infected predator $ I(t) $, respectively [10,11,12]. The infection term could be mass-action term (bilinear form) $ \beta S I $ or saturation form $ \frac{\beta S I}{S+I} $ [4]. The infected predators usually behave differently with susceptible ones, and suffer an additional death rate. In a epidemic model, the global dynamics are usually determined by the basic reproduction number $ \mathcal{R}_0 $, i.e., the disease will dies out in the population when $ \mathcal{R}_0\leq 1 $, and the disease will persist in the population when $ \mathcal{R}_0 > 1 $. However, the basic reproduction number is no longer a threshold parameter determining the global dynamics in diseased predator models, on the contrary, the dynamics are relatively comprehensive and unexpected.
Predation is the key force in a prey-predator interaction, which could affect the size of prey population by direct hunting [9,13,14,15], and elicit a variety of anti-predator responses [16,17,18]. Consequently, prey tends to alter behaviors in a certain extent, such as change of habitat, foraging activity, vigilance, physiological changes. This anti-predator behaviors accelerate the extinction, evolution and development of prey population in the long run. Under the risk of predation, prey may reduce its foraging activity in order to stay alert, leading to starvation which impacts on population growth [19,20]. Therefore, an immediately result of anti-predator behaviors is the reduction of prey growth rate, which is the cost for prey in prey defense [19,21,22,23,24,25,26].
Consider a simple birth-death process of the prey $ X(t) $ with the cost of anti-predator behaviors [27]:
$ \frac{{\mathrm{d}}X}{{\mathrm{d}}t} = [F(k,Y)a]X-dX, $ |
where $ X, Y $ represent the density of the prey and predator, respectively. $ a $ is the birth rate of prey, $ d $ is the natural death rate of prey. $ F(k, Y) $ accounts for the cost of anti-predator defence due to fear, the parameter $ k $ reflects the level of fear which drives anti-predator behaviors of prey. The fear factor $ F(k, Y) $ has some specific assumptions under the ecological motions, for details see [20,27].
To derive a simple diseased predator model incorporating the anti-predator defence due to fear, we adopted the following fear effect term $ F(k, Y) $:
$ F(k, Y) = \frac{1}{1+kY} = \frac{1}{1+k(S+I)}. $ |
Based on the results in [4,9,11], we can obtain the eco-epidemiological system with cost of anti-predator behaviors as following system of nonlinear differential equations:
$ {dXdt=rX1+k(S+I)−rX2K−aXS1+bX,dSdt=eaXS1+bX−d1S−βSI,dIdt=βSI−d2I, $
|
(1.1) |
where $ X, S, I $ represent the density of prey, susceptible predator and infected predator at time $ t $, respectively. $ r $ is the intrinsic growth rate of prey, $ K $ is the carrying capacity of the prey, $ a $ is the predation coefficient, $ b $ is the predators handling time of a prey, $ e $ is the biomass conversion constant, $ \beta $ is the transmissibility coefficient. $ d_1 $ and $ d_2 $ are the mortality rates of the susceptible predator and infected predator, and naturally $ d_1 < d_2 $.
This paper consists of six sections. In the next section, we prove the positivity and boundedness of the solution of system (1.1). In Section 3, we provide the existence conditions of the equilibria of the model. We analyze the stability of equilibria and show the occurrence of Hopf bifurcation in Section 4. In Section 5, the correctness of the theoretical proof is illustrated by numerical simulation. Finally, we summarize our results with ecological interpretations in Section 6.
In view of the ecological significance, we only consider the solutions $ (X(t), S(t), I(t)) $ of system (1.1) on
$ {\mathbb R}_{+}^{3} = \{(X(t), S(t), I(t))\in {\mathbb R}_+^3:X(t)\geq0,S(t)\geq0,I(t)\geq0\}. $ |
Theorem 2.1. Each solution of system (1.1) with initial value $ (X(0), S(0), I(0))\in {\mathbb R}^{3}_{+} $ is positive and ultimately bounded.
Proof. Since the right-hand side of system (1.1) is completely continuous and locally Lipschitzian on $ {\mathbb R}^{3}_{+} $, the solution $ (X(t), S(t), I(t)) $ with initial condition $ (X(0), S(0), I(0))\in {\mathbb R}^{3}_{+} $ exists and is unique on $ {\mathbb R}^{3}_{+} $.
By integrating, it follows from system (1.1) that
$ X(t)=X(0)exp{t∫0(r1+k(S(τ)+I(τ))−rX(τ)K−aS(τ)1+bX(τ))dτ}≥0,S(t)=S(0)exp{t∫0(eaX(τ)1+bX(τ)−d1−βI(τ))dτ}≥0,I(t)=I(0)exp{t∫0(βS(τ)−d2)dτ}≥0. $
|
Hence, the solution $ (X(t), S(t), I(t)) $ of system (1.1) with the initial condition $ (X(0), S(0), I(0))\in {\mathbb R}^{3}_{+} $ remains positive.
From the first equation of (1.1), we can obtain
$ \frac{{\mathrm{d}}X}{{\mathrm{d}}t} = \frac{rX}{1+k(S+I)}- \frac{rX^2}{K}- \frac{aXS}{1+bX}\leq rX- \frac{rX^2}{K} = rX\left(1- \frac{X}{K}\right), $ |
then
$ \limsup\limits_{t\rightarrow \infty}X(t)\leq K. $ |
Let $ N(t) = eX(t)+S(t)+I(t) $, we can get
$ dNdt=erX1+k(S+I)−erX2K−d1S−d2I≤erX−erX2K−d1S−d2I≤erX(1−XK)+ed1X−d1N≤eK(r+d1)24r−d1N, $
|
then
$ \limsup\limits_{t\rightarrow \infty}N(t)\leq \frac{eK(r+d_1)^2}{4rd_1}. $ |
This ends the proof.
Remark 2.2. From Theorem 2.1, we know that all positive solutions of system (1.1) with initial conditions $ (X(0), S(0), I(0))\in \mathbb{R}_+^3 $ are defined in the following positive bounded invariant:
$ \Gamma: = \left\{(X(t),S(t),I(t))\in {\mathbb R}^{3}_{+}:0\leq X(t)\leq K,0\leq eX(t)+S(t)+I(t)\leq \frac{eK(r+d_1)^2}{4rd_1}\right\}. $ |
System (1.1) possesses at most three boundary equilibria:
(i) Trivial equilibrium: $ E_0 = (0, 0, 0); $
(ii) Axial equilibrium: $ E_1 = (K, 0, 0); $
(iii) Planar equilibrium: $ E_2 = (X_2, S_2, 0) $ exists if $ ea-bd_1 > 0 $ and $ K > \frac{d_1}{ea-bd_1} $, where
$ X2=d1ea−bd1,S2=−[K(ea−bd1)2+rd1ke]+√[K(ea−bd1)2−rd1ke]2+4K2kre(ea−bd1)32Kk(ea−bd1)2. $
|
(3.1) |
For epidemic models, the most critical problem is the threshold property for the extinction and persistence of the disease, which is generally governed by the basic reproduction number $ \mathcal{R}_0 $. The basic reproduction number can be interpreted as the expected number of secondary cases produced, in a completely susceptible population, by a typical infected individual during its entire period of infectiousness. Following [28], we define the basic reproduction number for the predator population in the system (1.1) by
$ \mathcal{R}_0: = \dfrac{\beta S_2}{d_2}, $ |
where $ S_2 $ is given by (3.1).
Next, we mainly focus on the existence of positive equilibrium $ E_3 = (X_3, S_3, I_3) $ of system (1.1). The coordinates $ X_3, S_3, I_3 $ are positive solutions to the following system of equilibrium equations:
$ {r1+k(S3+I3)−rX3K−aS31+bX3=0,eaX31+bX3−d1−βI3=0,βS3−d2=0. $
|
Thus,
$ S_3 = \frac{d_2}{\beta},\;\;\;I_3 = {\frac {X_3(ea-bd_{{1}})-d_{{1}}}{ \left( bX_3+1 \right) \beta}}, $ |
and $ X_3 $ is the positive root of (3.2) in $ \left(X_2, +\infty\right) $:
$ Q(X)=m3X3+m2X2+m1X+m0=0, $
|
(3.2) |
where
$ m3:=−bβr(k(ea−bd1)+b(kd2+β)),m2:=βr(Kb2β−k(ea−bd1)−2b(kd2+β)+bkd1),m1:=(−kd2a(ea−bd1)−b(akd22+aβd2−2β2r))K−βr(−kd1+kd2+β),m0:=−K(−ad2(kd1−kd2−β)−β2r). $
|
If $ ea-bd_1 > 0 $ and $ r > \dfrac{ad_2(\beta+k(d_2-d_1))}{\beta^2} $, we have
$ m_3 < 0,\;\;\;m_0 > 0. $ |
By Descartes' rule of signs, system (1.1) has at least one positive equilibrium $ E_3. $
Hence, we have the following results on the existence of the positive equilibrium. It is worthy to note that the positive equilibrium is not unique due to the impact of fear effect $ k $.
Theorem 3.1. If $ ea-bd_1 > 0 $ and $ r > \frac{ad_2(\beta+k(d_2-d_1))}{\beta^2} $, then system (1.1) has at least one positive equilibrium $ E_3 = (X_3, S_3, I_3), $ where $ S_3 = \frac{S_2}{\mathcal{R}_0} $, $ I_3 = {\frac {X_3(ea-bd_{{1}})-d_{{1}}}{ \left(bX_3+1 \right) \beta}} $ and $ X_3 $ is the positive root of (3.2) in $ \left(X_2, +\infty\right) $.
Regarding the local stability of trivial equilibrium $ E_0 $ and axial equilibrium $ E_1 $, we have the following results. The proof is standard, so we omit it here.
Theorem 4.1. For system (1.1),
(i) The trivial equilibrium $ E_0 = (0, 0, 0) $ is unstable;
(ii) If one of the following inequalities holds:
(ii-1) $ ea-bd_1 < 0 $;
(ii-2) $ ea-bd_1 > 0 $ and $ K < \frac{d_1}{ea-bd_1} $,
then the axial equilibrium $ E_1 = (K, 0, 0) $ is stable; while $ E_1 = (K, 0, 0) $ is unstable if $ ea-bd_1 > 0 $ and $ K > \frac{d_1}{ea-bd_1} $.
Secondly, we will show the local stability of the planar equilibrium $ E_2 $ of system (1.1). For convenience, set
$ r1:=d2(ea−bd1)βe,r2:=d2(ea−bd1)(ea+bd1)aβe2,K1:=ea+bd1b(ea−bd1),K2:=βerd1(ea−bd1)(βer−d2(ea−bd1)),k1:=Kb(ea−bd1)2(Kb(ea−bd1)−(ea+bd1))ae2r(ea+bd1),k2:=−β((ea−bd1)(d2(ea−bd1)−βer)K+βerd1)(Kd2(ea−bd1)2+βerd1)d2. $
|
(4.1) |
Theorem 4.2. For system (1.1), assume that $ ea-bd_{{1}} > 0 $. If one of the following inequalities holds:
(Ⅰ) $ r\leq r_1 $ and one of the following inequalities holds:
(Ⅰ-1) $ \frac{d_1}{ea-bd_1} < K\leq K_1 $;
(Ⅰ-2) $ K > K_1 $ and $ k > k_1 $;
(Ⅱ) $ r_1 < r < r_2 $ and one of the following inequalities holds:
(Ⅱ-1) $ \frac{d_1}{ea-bd_1} < K\leq K_1 $;
(Ⅱ-2) $ K_1 < K $ and $ k > \max\{k_1, k_2\} $;
(Ⅲ) $ r > r_2 $ and one of the following inequalities holds:
(Ⅲ-1) $ \frac{d_1}{ea-bd_1} < K\leq K_2 $;
(Ⅲ-2) $ K_2 < K $ and $ k > \max\{k_1, k_2\} $,
then equilibrium $ E_2 $ is stable; otherwise, it is unstable.
Proof. The Jacobian matrix of system (1.1) at $ E_2 $ is given by
$ J2=(a11a12a13a210−βS200βS2−d2), $
|
where
$ a11:=X2(−rK+abS2(1+bX2)2),a12:=−krX2(1+kS2)2−aX21+bX2,a13:=−krX2(1+kS2)2,a21:=eaS2(1+bX2)2. $
|
Hence, the characteristic equation of $ J_2 $ is given as
$ f(λ)(λ−βS2+d2)=0, $
|
(4.2) |
where
$ f(\lambda): = \lambda^2-a_{11}\lambda-a_{12}a_{21}. $ |
Clearly, one can see that $ J_2 $ has three eigenvalues $ \lambda_1 $, $ \lambda_2 $ and $ \lambda_3 = \beta S_2-d_2. $ Since $ a_{12} < 0, a_{21} > 0 $, then $ -a_{12}a_{21} > 0 $.
From (3.1), we can obtain
$ a11=X2(−rK+abS2(1+bX2)2)=X2Φ2Kka2e2, $
|
where
$ \Phi: = ab\sqrt { \left( K \left( ea-bd_{{1}} \right) ^{2}-rd_{{1}}ke \right) ^{ 2}+4\,{K}^{2}kre \left( ea-bd_{{1}} \right) ^{3}} \\- ab(K(ea-bd_1)^2+rd_1ke)-2rka^2e^2. $ |
Note that the sign of $ \Phi $ depends on
$ ˜Φ:=a2b2((K(ea−bd1)2−rd1ke)2+4K2kre(ea−bd1)3)−(ab(K(ae−bd1)2+rd1ke)+2rka2e2)2=4a2kerP(k), $
|
where
$ P(k): = -a{e}^{2}r \left( ea+bd_{{1 }} \right)k+Kb(ea-bd_1)^2(Kb(ea-bd_1)-(ea+bd_1)) . $ |
One can obtain that $ P(k) $ is decreasing with respect to $ k $. If $ K\leq{\frac {ea+bd_{{1}}}{b \left(ea-bd_{{1}} \right) }} $ holds, we have $ P(0)\leq0 $, which means that $ P(k) < 0 $ for all $ k > 0 $; if $ K > {\frac {ea+bd_{{1}}}{b \left(ea-bd_{{1}} \right) }} $ and $ k > k_1 $ hold, we can get $ P(k) < 0 $. Therefore, when one of the following inequalities holds:
(i) $ K\leq{\frac {ea+bd_{{1}}}{b \left(ea-bd_{{1}} \right) }} $;
(ii) $ K > {\frac {ea+bd_{{1}}}{b \left(ea-bd_{{1}} \right) }} $ and $ k > k_1 $,
we can obtain $ a_{11} < 0 $, which implies that the real parts of $ \lambda_1 $ and $ \lambda_2 $ are all negative.
It follows from system (3.1) that
$ βS2−d2=−β[K(ea−bd1)2+rd1ke]+β√[K(ea−bd1)2−rd1ke]2+4K2kre(ea−bd1)32Kk(ea−bd1)2−d2=Θ2Kk(ea−bd1)2, $
|
where
$ \Theta: = -K \left( ea-bd_{{1}} \right) ^{2} \left( 2\,kd_{{2}}+\beta \right) -\beta\,ekrd_{{1}}+\beta\,\sqrt { \left( K \left( ea-bd_{{1}} \right) ^{2}-rd_{{1}}ke \right) ^{2}+4\,{K}^{2}kre \left( ea-bd_{{1}} \right) ^{3}}. $ |
Note that the sign of $ \Theta $ depends on
$ ˜Θ:=β2(K(ea−bd1)2−rd1ke)2+4β2K2kre(ea−bd1)3−(K(ea−bd1)2(2kd2+β)+βekrd1)2=−4Kk(ae−bd1)2[(Kd2(ea−bd1)2+βerd1)d2k+β((ea−bd1)(d2(ea−bd1)−βer)K+βerd1)]. $
|
Then if one of the following inequalities holds:
(Ⅰ) $ ea-bd_{{1}} > 0 $ and $ r\leq\frac{d_2(ea-bd_1)}{\beta e} $;
(Ⅱ) $ ea-bd_{{1}} > 0 $, $ r > \frac{d_2(ea-bd_1)}{\beta e} $ and one of the following inequalities:
(Ⅱ-1) $ K\leq\frac{\beta erd_1}{(ea-bd_1)(\beta er-d_2(ea-bd_1))} $;
(Ⅱ-2) $ K > \frac{\beta erd_1}{(ea-bd_1)(\beta er-d_2(ea-bd_1))} $ and $ k > k_2: = -\frac{\beta((ea-bd_1)(d_2(ea-bd_1)-\beta er)K+\beta erd_1)}{(Kd_2(ea-bd_1)^2+\beta erd_1)d_2} $,
we have $ \lambda_3 = \beta S_2-d_2 < 0. $
Thus, we can arrive at the conclusion.
It should be pointed out that another way to state Theorem 4.2 is as follows.
Remark 4.3. For system (1.1), assume that $ ea-bd_1 > 0 $ and $ \mathcal{R}_0 < 1 $. If one of the following inequalities:
(Ⅰ) $ \dfrac{d_1}{ea-bd_1} < K\leq K_1; $
(Ⅱ) $ K > K_1 $ and $ k > k_1 $
holds, then the planar equilibrium $ E_2 $ is stable; otherwise, it is unstable.
Next, we will show the local stability of the positive equilibrium $ E_3 $ of system (1.1).
The Jacobian matrix of system (1.1) at $ E_3 $ is given by
$ J_3 = \left( b11b12b13b210−d20βI30 \right), $
|
where
$ b11=X3(−rK+abS3(1+bX3)2),b12=−krX3(1+k(S3+I3))2−aX31+bX3<0,b13=−krX3(1+k(S3+I3))2<0,b21=eaS3(1+bX3)2>0. $
|
(4.3) |
The characteristic equation of $ J_3 $ is given as
$ λ3+A1λ2+A2λ+A3=0, $
|
(4.4) |
where
$ A1=−b11,A2=βd2I3−b12b21,A3=−b11βd2I3−b13b21βI3. $
|
(4.5) |
Note that if $ A_1 > 0 $ holds, then $ b_{11} < 0 $, which means that $ A_3 > 0. $ According to Routh-Hurwitz criterion, the positive equilibrium $ E_3 $ is locally asymptotically stable when $ A_1 > 0 $ and $ A_1A_2-A_3 > 0 $.
Therefore, we can establish the following statement.
Theorem 4.4. Assume that $ ea-bd_1 > 0 $ and $ r > \frac{ad_2(\beta+k(d_2-d_1))}{\beta^2} $ hold. The positive equilibrium $ E_3 $ of system (1.1) is locally asymptotically stable if $ A_1 > 0 $ and $ A_1A_2-A_3 > 0 $, where $ A_i, i = 1, 2, 3 $ is defined as in (4.5). Otherwise, it is unstable.
Remark 4.5. Theorem 4.4 gives a sufficient condition about the stability of the positive equilibrium $ E_3 $ for system (1.1). However, the complexity of model (1.1) leads to the failure to theoretically demonstrate how the fear factor affects the stability of the positive equilibrium. This will be discussed later through numerical simulations.
In this subsection, we take $ k $ as the bifurcation parameter. The characteristic equation of system (1.1) at $ E_3 $ is (4.4), and $ A_i(k), i = 1, 2, 3 $ are defined as (4.5).
Theorem 4.6. Hopf bifurcation near the positive equilibrium $ E_3 $ for system (1.1) occurs whenever the critical parameter $ k $ attains the value $ k = k_h $ in the domain:
$ \Omega = \left\{k_h\in \mathbb{R}^+: \Delta(k_h): = [A_1(k)A_2(k)-A_3(k)]|_{k = k_h} = 0\; with\; A_2(k_h) > 0,\; \left[\frac{{\mathrm{d}}\Delta(k)}{{\mathrm{d}}k}\right]\Bigg|_{k = k_h}\neq0\right\}. $ |
Proof. If $ k = k_h $, the characteristic Eq (4.4) equals
$ λ3+A1(kh)λ2+A2(kh)λ+A3(kh)=0, $
|
(4.6) |
then (4.6) can be factorized as
$ (λ2+A2(kh))(λ+A1(kh))=0. $
|
(4.7) |
Clearly, (4.7) has three roots: $ \lambda_1 = i\sqrt{A_2(k_h)} $, $ \lambda_2 = -i\sqrt{A_2(k_h)} $ and $ \lambda_3 = -A_1(k_h) $. The roots are of the form $ \lambda_1 = p_1(k)+ip_2(k) $, $ \lambda_2 = p_1(k)-ip_2(k) $ and $ \lambda_3 = -p_3(k) $, where $ p_i(k) (i = 1, 2, 3) $ are real numbers.
From the characteristic Eq (4.4), we can get
$ dλdk=−λ2A′1+λA′2+A′33λ2+2A1λ+A2, $
|
(4.8) |
where $ ' = \frac{{\mathrm{d}}}{{\mathrm{d}}k} $. Substituting $ \lambda = i\sqrt{A_2} $ into (4.8), we obtain that
$ A′3−A2A′1+iA′2√A22(A2−iA1√A2)=−dΔ(k)dk2(A21+A2)+i[√A2A′22A2−A1√A2dΔ(k)dk2A2(A21+A2)], $
|
which implies that
$ \left[\dfrac{{\mathrm{d}}{\mathrm{Re}}(\lambda)}{{\mathrm{d}}k}\right]\Big|_{k = k_h} = -\dfrac{\frac{{\mathrm{d}}\Delta(k)}{{\mathrm{d}}k}}{2(A_1^2+A_2)}\Big|_{k = k_h}. $ |
By using monotonicity condition in the real part of the complex root $ \frac{{\mathrm{d}} {\mathrm{Re}}(\lambda)}{{\mathrm{d}}k}|_{k = k_h}\neq0 $, the transversality condition $ \frac{{\mathrm{d}}\Delta(k)}{{\mathrm{d}}k}|_{k = k_h}\neq0 $ can be obtained to ensure the existence of Hopf bifurcation.
Results from numerical simulations are provided in this section to demonstrate our theoretical results. As we will show, the observations shed lights on the impact of fear factor. We choose the parameters of system (1.1) as follows:
$ r=0.8,a=0.2,b=0.1,e=0.9,d1=0.05,β=0.1,d2=0.053. $
|
(5.1) |
Then we have
$ ea−bd1=0.175>0,d1ea−bd1=0.286,r1=d2(ea−bd1)βe=0.103,r2=d2(ea−bd1)(ea+bd1)aβe2=0.106,K1=ea+bd1b(ea−bd1)=10.571,K2=βerd1(ea−bd1)(βer−d2(ea−bd1))=0.328. $
|
Example 5.1 (The stability of $ E_1 $).
We adopt $ K = 0.2, k = 0.01 $, then system (1.1) has trivial equilibrium $ E_0 = (0, 0, 0) $ and axial equilibrium $ E_1 = (0.2, 0, 0) $. In this case, one can know that the conditions of Theorem 4.1 are satisfied, which means that $ E_1 $ is locally asymptotically stable. The numerical results are shown in Figure 1.
Example 5.2 (The impacts of $ K $ and $ k $ on the stability of $ E_2 $).
In this example, we will choose three values of carrying capacity $ K $ for numerical experiments. We conclude that the carrying capacity and fear effect are other key factors related to the extinction of infected predators, in addition to the basic reproduction number $ \mathcal{R}_0 $.
Firstly, we take $ K = 0.3 < K_2 $, then we have $ k = 0.1 $ which yields that $ \mathcal{R}_0 = 0.263 < 1 $. In this case, system (1.1) has trivial equilibrium $ E_0 = (0, 0, 0) $, axial equilibrium $ E_1 = (0.3, 0, 0) $, and planar equilibrium $ E_2 = (0.286, 0.139, 0) $. By Theorem 4.5, $ E_2 $ is locally asymptotically stable, see Figure 2(a). Thus, when the carrying capacity of the prey $ K $ is small, no matter what the level of fear $ k $ is, the small size of prey population will lead to the extinction of infected predators.
Secondly, for comparison, we take $ K_2 < K = 15 $, then
$ k1=Kb(ea−bd1)2(Kb(ea−bd1)−(ea+bd1))ae2r(ea+bd1)=0.149,k2=−β((ea−bd1)(d2(ea−bd1)−βer)K+βerd1)(Kd2(ea−bd1)2+βerd1)d2=10.873. $
|
Choosing $ k = 0.1 < \max\{k_1, k_2\} $ which yields $ \mathcal{R}_0 = 5.792 > 1 $, then we have
$ A_1 = 0.25791 > 0,\;A_1A_2-A_3 = 0.00523 > 0. $ |
In this case, system (1.1) has trivial equilibrium $ E_0 = (0, 0, 0) $, axial equilibrium $ E_1 = (15, 0, 0) $, planar equilibrium $ E_2 = (0.286, 3.070, 0) $, and positive equilibrium $ E_3 = (7.351, 0.530, 7.125) $. By Theorem 4.5, $ E_2 = (0.286, 3.070, 0) $ is unstable. On the contrary, $ E_3 = (7.351, 0.530, 7.125) $ is locally asymptotically stable. The numerical simulation is shown in Figure 2(b).
Finally, we take $ K_2 < K = 60 $, then we have
$ k1=Kb(ea−bd1)2(Kb(ea−bd1)−(ea+bd1))ae2r(ea+bd1)=6.629,k2=−β((ea−bd1)(d2(ea−bd1)−βer)K+βerd1)(Kd2(ea−bd1)2+βerd1)d2=12.238. $
|
Choosing $ k = 30 > \max\{k_1, k_2\} $ which yields that $ \mathcal{R}_0 = 0.649 < 1 $, system (1.1) has trivial equilibrium $ E_0 = (0, 0, 0) $, axial equilibrium $ E_1 = (60, 0, 0) $, and planar equilibrium $ E_2 = (0.286, 0.344, 0) $. By Theorem 4.5, $ E_2 $ is locally asymptotically stable, see Figure 2(c). Thus, when the carrying capacity of the prey $ K $ is relatively large, a high level of fear $ k $ will lead to the extinction of infected predators.
Example 5.3 (The impact of $ k $ on the stability of $ E_3 $). We adopt $ K = 60 $, then we have $ k_h = 0.26 $. In the next, we will choose three values of $ k $, corresponding to the local stability of $ E_3 $, Hopf bifurcation, and instability of $ E_3 $, to illustrate the impact of fear factor on the population dynamics.
Firstly, we take $ k = 0.1 < k_h $ which yields that $ \mathcal{R}_0 = 5.881 > 1 $, then system (1.1) has trivial equilibrium $ E_0 = (0, 0, 0) $, axial equilibrium $ E_1 = (60, 0, 0) $, planar equilibrium $ E_2 = (0.286, 3.117, 0) $ and a unique positive equilibrium $ E_3 = (24.047, 0.530, 12.213) $. In this case, we obtain that
$ A_1 = 0.29863 > 0,\;A_1A_2-A_3 = 0.00065 > 0, $ |
which means that $ E_3 $ is local asymptotically stable. The numerical results are shown in Figure 3.
Secondly, we take $ k = 0.26 = k_h $ which yields that $ \mathcal{R}_0 = 4.682 > 1 $, then system (1.1) has trivial equilibrium $ E_0 = (0, 0, 0) $, axial equilibrium $ E_1 = (60, 0, 0) $, planar equilibrium $ E_2 = (0.286, 2.481, 0) $ and a unique positive equilibrium $ E_3 = (12.975, 0.530, 9.665) $. In this case, we obtain that
$ A_1 = 0.14694 > 0,\;A_1A_2-A_3 = 0, $ |
which means that system (1.1) undergoes a Hopf bifurcation and there is a limit cycle around $ E_3 $. The numerical results and the bifurcation diagrams of system (1.1) with respect to the parameter $ k $ are shown in Figures 4 and 5, respectively. Comparing Figures 3 and 4(a), one can see that there are two different implications induced by the fear factor $ k $: the first is that the stability of $ E_3 $ converts from stable into unstable, and the second is the decrease of values of $ X_3 $ and $ I_3 $ of $ E_3 $.
Finally, we take $ k = 0.5 > k_h $ which yields that $ \mathcal{R}_0 = 3.821 > 1 $, then system (1.1) has trivial equilibrium $ E_0 = (0, 0, 0) $, axial equilibrium $ E_1 = (60, 0, 0) $, planar equilibrium $ E_2 = (0.286, 2.025, 0) $ and a unique positive equilibrium $ E_3 = (7.685, 0.530, 7.322) $. In this case, we can obtain that
$ A_1 = 0.07642 > 0,\;A_1A_2-A_3 = -0.00051 < 0, $ |
which means that $ E_3 $ is unstable. The numerical results are shown in Figure 6. One can find that the difference between Figures 4 and 6 is the decrease of values of $ E_3 $ from $ (12.975, 0.530, 9.665) $ to $ (7.685, 0.530, 7.322), $ which is induced by the impact of the feat factor.
In this paper, we explored a predator-prey model that incorporates infectious disease in predator population and the cost of anti-predator behaviors. The cost of anti-predator behaviors is measured by a fear effect $ k $ leading to an reduction of prey's birth rate. We fulfill a complete stability analysis of equilibria for system (1.1) and show that the system (1.1) exhibits the Hopf bifurcation. Biologically, we focus on the impact of fear effect on the population dynamics. As we will see later, the cost of a high level of fear effect is disastrously. The main findings are summarized in the following.
$ 1) $ Small size of prey population leads to the extinction of infected predators.
If the carrying capacity $ K $ is relatively small, the planar equilibrium $ E_2 $ is stable, see Figure 2(a). Thus, no matter what the level of fear effect $ k $ is, a small size of prey population will lead to the extinction of infected predators.
$ 2) $ Low level of the fear effect doesn't impact on the population dynamics.
If the level of fear effect $ k < k_h $, the positive (coexistence) equilibrium $ E_3 $ is stable, see Figure 3. Hence, we conclude that a small fear effect $ k $ is not the key disturbance and does not change the coexistence dynamics of system (1.1). However, the densities of the prey and infected predator gradually decrease as $ k $ increasing.
$ 3) $ Certain medium level of the fear effect lead to periodic oscillation.
If $ k = k_h $, the fear effect can destabilize the stability of $ E_3 $ and will benefit the occurrence of periodic oscillation. In other words, system (1.1)undergoes a limit cycle, see Figures 4 and 5.
$ 4) $ High level of the fear effect leads to complex dynamics and the infected predator can go to extinction.
If $ k > k_h $, $ E_3 $ is unstable, see Figure 6. Therefore, a large fear effect $ k $ persistently and dramatically influence the population dynamics of prey and predator. Furthermore, if the level of the fear factor $ k $ is extremely high, the planar equilibrium $ E_2 $ is stable, see Figure 2(c). The prey will respond to perceived predation risk and show a variety of anti-predator responses, dramatically decreasing the recruitment of susceptible predator, which will lead to an extinction of infected predator.
The authors would like to thank the editor and the referees for their helpful comments. This research was supported by the National Natural Science Foundation of China (Grant No. 12171192, 12031020 and 12071173), the Science and Technology Research Projects of the Education Office of Jilin Province, China (JJKH20211033KJ), the Technology Development Program of Jilin Province, China (20210508024RQ) and Huaian Key Laboratory for Infectious Diseases Control and Prevention, China (HAP201704).
The authors declare that there are no conflicts of interest regarding the publication of this paper.
[1] |
Pollard TD, Borisy GG (2003) Cellular motility driven by assembly and disassembly of actin filaments. Cell 112: 453–465. doi: 10.1016/S0092-8674(03)00120-X
![]() |
[2] |
Pollard TD (2003) The cytoskeleton, cellular motility and the reductionist agenda. Nature 422: 741–745. doi: 10.1038/nature01598
![]() |
[3] |
Holmes WR, Edelsteinkeshet L (2012) A comparion of computational models for eukaryotic cell hape and motility. PLoS Comput Biol 8: e1002793. doi: 10.1371/journal.pcbi.1002793
![]() |
[4] |
Danuser G, Allard J, Mogliner A (2013) Mathematical modeling of eukaryotic cell migration: Insights beyond experiments. Annu Rev Cell Dev Biol 29: 501–528. doi: 10.1146/annurev-cellbio-101512-122308
![]() |
[5] |
Mogiler A (2009) Mathematics of cell motility: Have we got its number? J Math Biol 58: 105–134. doi: 10.1007/s00285-008-0182-2
![]() |
[6] | Mogilner A, Keren AK (2009) The shape of motile cells. Curr Biol 15: 762–771. |
[7] | Oelz D, Schmeiser C (2011) Simulation of lamellipodial fragments. J Math Biol 64: 513–528. |
[8] | Adler Y, Givli S (2013) Closing the loop: Lamellipodia dynamics from the perspective of front propagation. Phys Rev E 88: 042708. |
[9] |
Recho P, Putelat T, Truskinovsky L (2013) Contraction-driven cell motility. Phys Rev Lett 111: 108102. doi: 10.1103/PhysRevLett.111.108102
![]() |
[10] |
Tjhung E, Tiribocchi A, Marenduzzo D, et al. (2015) A minimal physical model captures the shapes of crawling cells. Nat Commun 6: 5420. doi: 10.1038/ncomms6420
![]() |
[11] | Ambrosi D, Zanzottera A (2016) Mechanics and polarity in cell motility. Physica D 330: 58–66. |
[12] |
Raynaud F, Ambühl ME, Gabella C, et al. (2016) Minimal models for spontaneous cell polarization and edge activity in oscillating, rotating and migrating cells. Nat Phys 12: 367–374. doi: 10.1038/nphys3615
![]() |
[13] |
Pollard TD, Cooper JA (2009) Actin, a central player in cell shape and movement. Science 326: 1208–1212. doi: 10.1126/science.1175862
![]() |
[14] |
Rafelski SM, Theriot JA (2004) Crawling toward a unified model of cell motility: Spatial and temporal regulation of actin dynamics. Annu Rev Biochem 73: 209–239. doi: 10.1146/annurev.biochem.73.011303.073844
![]() |
[15] | Mogilner A (2006) On the edge: Modeling protrusion. Curr Opin Cell Biol 18: 32–39. |
[16] |
Svitkina TM, Verkhovsky AB, Mcquade KM, et al. (1997) Analysis of the actin-myosin II system in fish epidermal keratocytes: Mechanism of cell body translocation. J Cell Biol 139: 397–415. doi: 10.1083/jcb.139.2.397
![]() |
[17] |
Clainche CL, Carlier MF (2008) Regulation of actin assembly associated with protrusion and adhesion in cell migration. Physiol Rev 88: 489–513. doi: 10.1152/physrev.00021.2007
![]() |
[18] |
Vincente-Manzaneres M, Choi CK, Horwitz AR (2009) Integrins in cell migration-the actin connection. J Cell Sci 122: 199–206. doi: 10.1242/jcs.018564
![]() |
[19] |
Gardel ML, Schneider IC, Aratyn-Schaus Y, et al. (2010) Mechanical integration of actin and adhesion dynamics in cell migration. Annu Rev Cell Dev Biol 26: 315–333. doi: 10.1146/annurev.cellbio.011209.122036
![]() |
[20] |
Suter DM, Forscher P (2000) Substrate-cytoskeletal coupling as a mechanism for the regulation of growth cone motility and guidance. Dev Neurobiol 44: 97–113. doi: 10.1002/1097-4695(200008)44:2<97::AID-NEU2>3.0.CO;2-U
![]() |
[21] | Vincente-Manzaneres M, Ma X, Adelstein RS, et al. (2009) Non-muscle myosin II takes centre stage in cell adhesion and migration. Nat Rev Mol Cell Biol 10: 788–790. |
[22] |
Kirfel G, Rigort A, Borm B, et al. (2004) Cell migration: Mechanisms of rear detachment and the formation of migration tracks. Eur J Cell Biol 83: 717–724. doi: 10.1078/0171-9335-00421
![]() |
[23] |
Lee J, Ishihara A, Oxford G, et al. (1999) Regulation of cell movement is mediated by stretch-activated calcium channels. Nature 400: 382–386. doi: 10.1038/22578
![]() |
[24] |
Huttenlocher A, Palecek SP, Lu Q, et al. (1997) Regulation of cell migration by the calcium-dependent protease calpain. J Biol Chem 272: 32719–32722. doi: 10.1074/jbc.272.52.32719
![]() |
[25] |
Wolfenson H, Bershadsky A, Henis Y, et al. (2011) Actomyosin-generated tension controls the molecular kinetics of focal adhesions. J Cell Sci 124: 1425–1432. doi: 10.1242/jcs.077388
![]() |
[26] | Verkhovsky AB, Svitkina T, Borisy GG (1999) Self-polarization and directional motility of cytoplasm. Curr Biol 9: 11–20. |
[27] |
Ridley AJ, Horwitz AR (2003) Cell migration: Integrating signals from front to back. Science 302: 1704–1709. doi: 10.1126/science.1092053
![]() |
[28] | Friedl P, Sahai E, Weiss S, et al. (2012) New dimensions in cell migration. Nat Rev Mol Cell Biol 13: 743–747. |
[29] |
Lo CM, Wang HB, Dembo M, et al. (2000) Cell movement is guided by the rigidity of the substrate. Biophys J 79: 144–152. doi: 10.1016/S0006-3495(00)76279-5
![]() |
[30] |
Abercrombie M (1961) The bases of locomotory behaviour of fibroblasts. Exp Cell Res 8: 188. doi: 10.1016/0014-4827(61)90348-2
![]() |
[31] |
Allan RB, Wilkinson PC (1978) A visual analysis of chemotactic and chemokinetic locomotion of human neutrophil leukocytes. Exp Cell Res 111: 191–203. doi: 10.1016/0014-4827(78)90249-5
![]() |
[32] | Lee J, Ishihara A, Jacobson K (1993) The fish epidermal keratocyte as a model system for the study of cell locomotion. Symp Soc Exp Biol 47: 73–89. |
[33] |
Tranquillo RT, Lauffenburger DA, Zigmond SH (1988) A stochastic model for leukocyte random motility and chemotaxis bases on receptor binding fluctuations. J Cell Biol 106: 303–309. doi: 10.1083/jcb.106.2.303
![]() |
[34] | Mogilner A, Verzi DW (2003) A Simple 1-D physical model for the crawling nematode sperm cell. J Stat Phys 110: 1169–1189. |
[35] |
Herant M, Dembo M (2010) Form and function in cell motility: From fibroblasts to keratocytes. Biophys J 98: 1408–1417. doi: 10.1016/j.bpj.2009.12.4303
![]() |
[36] |
Satulovsky J, Lui R, Wang Yl (2008) Exploring the control circuit of cell migration by mathematical modeling. Biophys J 94: 3671–3683. doi: 10.1529/biophysj.107.117002
![]() |
[37] |
Grimm HP, Verkhovsky AB, Mogilner A, et al. (2003) Analysis of actin dynamics at the leading edge of crawling cells: Implications for the shape of the keratocyte lamellipodia. Eur Biophys J 32: 563–577. doi: 10.1007/s00249-003-0300-4
![]() |
[38] | Hellewell SB, Taylor DL (1979) The contractile basis of amoeboid movements. VI. The solation-contraction coupling hypothesis. J Cell Biol 83: 633–648. |
[39] |
Chen WT (1979) Induction of spreading during fibroblast movement. J Cell Biol 81: 684–691. doi: 10.1083/jcb.81.3.684
![]() |
[40] |
Harris A, Dunn G (1972) Centripetal transport of attached particles on both surfaces of moving fibroblasts. Exp Cell Res 73: 519–523. doi: 10.1016/0014-4827(72)90084-5
![]() |
[41] |
Lee J, Ishihara A, Teriot JA, et al. (1993) Principles of locomotion for simple-shaped cells. Nature 362: 167–171. doi: 10.1038/362167a0
![]() |
[42] |
Keren K, Pincus Z, Allen GM, et al. (2008) Mechanism of shape determination in motile cells. Nature 453: 475–480. doi: 10.1038/nature06952
![]() |
[43] |
Rubenstein B, Jacobson K, Moglilner A (2005) Multiscale two-dimensional modeling of a motile simple-shaped cell. Multiscale Model Sim 3: 413–439. doi: 10.1137/04060370X
![]() |
[44] | Barnhart EL, Lee KC, Keren K, et al. (2011) An adhesion-dependent switch between mechanisms that determine motile cell shape. PLoS Biol 9: 1–16. |
[45] |
Wolgemuth C, Stajic J, Mogliner A (2011) Redundant mechanisms for stable cell locomotion revealed by minimal models. Biophys J 101: 545–553. doi: 10.1016/j.bpj.2011.06.032
![]() |
[46] | Bottino D, Moglilner A, Roberts T, et al. (2001) How nematode sperm crawl. J Cell Sci 115: 367–384. |
[47] |
Roberts T, Stewart M (2000) Acting like actin: The dynamics of the nematode major sperm protein (MSP) cytoskeleton indicate a push-pull mechanism for amoeboid cell motility. J Cell Biol 149: 7–12. doi: 10.1083/jcb.149.1.7
![]() |
[48] |
Janson LW, Kolega J, Taylor DL (1991) Modulation of contraction by gelation/solation in a reconstituted motile model. J Cell Biol 114: 1005–1015. doi: 10.1083/jcb.114.5.1005
![]() |
[49] |
Forscher P, Smith SJ (1988) Actions of cytochalasins on the organization of actin filaments and microtubules in a neuronal growth cone. J Cell Biol 107: 1505–1516. doi: 10.1083/jcb.107.4.1505
![]() |
[50] |
Theriot JA, Mitchison TJ (1991) Actin microfilament dynamics in locomoting cells. Nature 352: 126–131. doi: 10.1038/352126a0
![]() |
[51] |
Harris AK, Wild P, Stopal D (1980) Silicone rubber substrata: A new wrinkle in the study of cell locomotion. Science 208: 177–179. doi: 10.1126/science.6987736
![]() |
[52] |
Wang JHC, Shang JS (2007) Cell traction force and measurement methods. Biomech Model Mechan 6: 361–371. doi: 10.1007/s10237-006-0068-4
![]() |
[53] |
Beningo KA, Dembo M, Kverina I, et al. (2001) Nascent focal adhesions are responsible for the generation of strong propulsive forces in migrating fibroblasts. J Cell Biol 153: 881–887. doi: 10.1083/jcb.153.4.881
![]() |
[54] |
Hind LE, Dembo M, Hammer DA (2015) Macrophage motility is driven by frontal-towing with a force magnitude dependent on substrate stiffness. Integr Biol 7: 447–453. doi: 10.1039/C4IB00260A
![]() |
[55] |
Doyle A, Marganski W, Lee J (2004) Calcium transients induce spatially coordinated increases in traction force during the movement of fish keratocytes. J Cell Sci 117: 2203–2214. doi: 10.1242/jcs.01087
![]() |
[56] |
Loisel TP, Boujemaa R, Pantaloni D, et al. (1999) Reconstitution of actin-based motility of Listeria and Shigella using pure proteins. Nature 401: 613–616. doi: 10.1038/44183
![]() |
[57] |
Roy P, Rajfur Z, Jones D (2001) Local photorelease of caged thymosin beta 4 in locomoting keratocytes causes cell turning. J Cell Biol 153: 1035–1047. doi: 10.1083/jcb.153.5.1035
![]() |
[58] |
DiMilla PA, Barbee K, Lauffenburger DA (1991) Mathematical model for the effects of adhesion and mechanics on cell migration speed. Biophys J 60: 15–37. doi: 10.1016/S0006-3495(91)82027-6
![]() |
[59] |
Gupton SL, Waterman-Storer CM (2006) Spatiotemporal feedback between actomyosin and focal-adhesion systems optimizes rapid cell migration. Cell 125: 1361–1374. doi: 10.1016/j.cell.2006.05.029
![]() |
[60] | Jurado C, Haserick JR, Lee J (2005) Slipping or gripping? Fluorescent speckle microscopy in fish keratocytes reveals two different mechanisms for generating a retrograde flow of actin. Mol Biol Cell 16: 507–518. |
[61] |
Wilson CA, Tsuchida MA, Allen GM, et al. (2010) Myosin II contributes to cell-scale actin network treadmilling through network disassembly. Nature 465: 373–377. doi: 10.1038/nature08994
![]() |
[62] |
Fournier MF, Sauser S, Ambrosi D, et al. (2010) Force transimission in migrating cells. J Cell Biol 188: 287–297. doi: 10.1083/jcb.200906139
![]() |
[63] |
Lee J, Leonard M, Oliver T, et al. (1994) Traction forces generated by locomoting keratocytes. J Cell Biol 127: 1957–1964. doi: 10.1083/jcb.127.6.1957
![]() |
[64] |
Hartwell LH, Hopfield JJ, Leibler S, et al. (1999) From molecular to modular cell biology. Nature 402: C47–C52. doi: 10.1038/35011540
![]() |
[65] |
Italiano JE, Stewart M, Roberts TM (2001) How the assembly dynamics of the nematode major sperm protein generate amoeboid cell motility. Int Rev Cytol 202: 1–34. doi: 10.1016/S0074-7696(01)02002-2
![]() |
[66] |
Friedl P, Wolf K (2010) Plasticity of cell migration: A multiscale tuning model. J Cell Biol 188: 11–19. doi: 10.1083/jcb.200909003
![]() |
[67] |
Doyle AD, Lee J (2005) Cyclic changes in keratocyte speed and traction stress arise from Ca2+-dependent regulation of cell adhesiveness. J Cell Sci 118: 369–379. doi: 10.1242/jcs.01590
![]() |
[68] |
Paku S, Tóvári J, Lörincz Z, et al. (2003) Adhesion dynamics and cytoskeletal structure of gliding human fibrosarcoma cells: A hypothetical model of cell migration. Exp Cell Res 290: 246–253. doi: 10.1016/S0014-4827(03)00334-3
![]() |
[69] |
Huang C, Rajfur Z, Borchers C, et al. (2003) JNK phosphorylates paxillin and regulates cell migration. Nature 424: 219–223. doi: 10.1038/nature01745
![]() |
[70] |
Asano Y, Mizuno T, Kon T, et al. (2004) Keratocyte-like locomotion in amiB-null Dictyostelium cells. Cell Motil Cytoskel 59: 17–27. doi: 10.1002/cm.20015
![]() |
[71] |
Ma L, Janetopoulos C, Yang L, et al. (2004) Two complementary, local excitation, global inhibition mechanisms acting in parallel can explain the chemoattractant-induced regulation of PI(3,4,5)P-3 response in Dictyostelium cells. Biophys J 87: 3764–3774. doi: 10.1529/biophysj.104.045484
![]() |
[72] |
Yam PT, Wilson CA, Ji L, et al. (2007) Actin-myosin network reorganization breaks symmetry at the cell rear to spontaneously initiate polarized cell motility. J Cell Biol 178: 1207–1221. doi: 10.1083/jcb.200706012
![]() |
[73] | Lee J, Jacobson K (1997) The composition and dynamics of cell-substratum adhesions in locomoting fish keratocytes. J Cell Sci 110: 2833–2844. |
[74] |
Anderson KI, Cross R (2000) Contact dynamics during keratocyte motility. Curr Biol 10: 253–260. doi: 10.1016/S0960-9822(00)00357-2
![]() |
[75] |
Morin TR, Ghassemzadeh SA, Lee J (2014) Traction force microscopy in rapidly moving cells reveals separate roles for ROCK and MLCK in the mechanics of retraction. Exp Cell Res 326: 280–294. doi: 10.1016/j.yexcr.2014.04.015
![]() |
[76] |
Shao D, Levine H, Rappel WJ (2012) Coupling actin flow, adhesion, and morphology in a computational cell motiliy model. PNAS 109: 6851–6856. doi: 10.1073/pnas.1203252109
![]() |
[77] |
Ziebert F, Aranson IS (2014) Modular approach for modeling cell motility. Eur Phys J-Spec Top 223: 1265–1277. doi: 10.1140/epjst/e2014-02190-2
![]() |
[78] |
Camely BA, Zhao Y, Li B, et al. (2017) Crawling and turning in a minimal reaction-diffusion cell motility model: Coupling cell shape and biochemistry. Phys Rev E 95: 012401. doi: 10.1103/PhysRevE.95.012401
![]() |
[79] |
Barnhart EL, Allen GM, Julicher F, et al. (2010) Bipedal locomotion in crawling cells. Biophys J 98: 933–942. doi: 10.1016/j.bpj.2009.10.058
![]() |
[80] |
Sanz-Morena V, Gadea G, Paterson H, et al. (2008) Rac activation and inactivation control plasticity of tumor cell movement. Cell 135: 510–523. doi: 10.1016/j.cell.2008.09.043
![]() |
1. | Chunmei Zhang, The effect of the fear factor on the dynamics of an eco-epidemiological system with standard incidence rate, 2024, 9, 24680427, 128, 10.1016/j.idm.2023.12.002 | |
2. | Hongqiuxue Wu, Zhong Li, Mengxin He, Dynamic analysis of a Leslie-Gower predator-prey model with the fear effect and nonlinear harvesting, 2023, 20, 1551-0018, 18592, 10.3934/mbe.2023825 | |
3. | Zhuoying Zhao, Xinhong Zhang, Unraveling the transmission mechanism of animal disease: Insight from a stochastic eco-epidemiological model driven by Lévy jumps, 2025, 191, 09600779, 115859, 10.1016/j.chaos.2024.115859 | |
4. | Huazhou Mo, Yuanfu Shao, Stability and bifurcation analysis of a delayed stage-structured predator–prey model with fear, additional food, and cooperative behavior in both species, 2025, 2025, 2731-4235, 10.1186/s13662-025-03879-y |