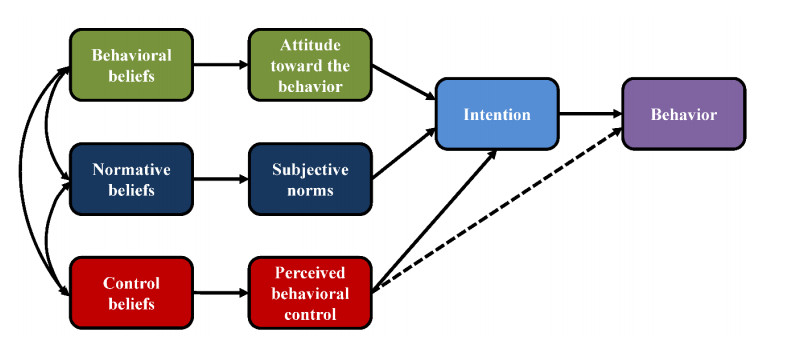
In this paper, Kou's method is extended to solve nonlinear systems. The convergence order of the iterative method is proved. Using fractal theory, we study the theoretical operators related to the iterative method, and analyze the stability of the iterative method. Properties related to strange fixed points and critical points are explored. The fractal results indicate that the iterative method is most stable when the parameter equals zero. The extended iterative method is applied to solve the Hammerstein equation and some nonlinear systems. The dynamic plane and numerical experiments show that the extended iterative method can solve the nonlinear system of equations with good convergence and stability.
Citation: Yantong Guo, Quansheng Wu, Xiaofeng Wang. An extension of high-order Kou's method for solving nonlinear systems and its stability analysis[J]. Electronic Research Archive, 2025, 33(3): 1566-1588. doi: 10.3934/era.2025074
[1] | Xiaojie Huang, Gaoke Liao . Identifying driving factors of urban digital financial network—based on machine learning methods. Electronic Research Archive, 2022, 30(12): 4716-4739. doi: 10.3934/era.2022239 |
[2] | Nuri Park, Junhan Cho, Juneyoung Park . Assessing crash severity of urban roads with data mining techniques using big data from in-vehicle dashcam. Electronic Research Archive, 2024, 32(1): 584-607. doi: 10.3934/era.2024029 |
[3] | Zhiqiang Yuan, Xi Deng, Tianzi Ding, Jing Liu, Qi Tan . Factors influencing secondary school teachers' usage behavior of dynamic mathematics software: A partial least squares structural equation modeling (PLS-SEM) method. Electronic Research Archive, 2023, 31(9): 5649-5684. doi: 10.3934/era.2023287 |
[4] | Mingyang Pei, Yi Hu, Lingshu Zhong . Applying an extended prototype willingness model to predict back seat safety belt use in China. Electronic Research Archive, 2023, 31(2): 961-984. doi: 10.3934/era.2023048 |
[5] | Xin Tang, Zhiqiang Yuan, Xi Deng, Liping Xiang . Predicting secondary school mathematics teachers' digital teaching behavior using partial least squares structural equation modeling. Electronic Research Archive, 2023, 31(10): 6274-6302. doi: 10.3934/era.2023318 |
[6] | Lijing Ma, Shiru Qu, Lijun Song, Junxi Zhang, Jie Ren . Human-like car-following modeling based on online driving style recognition. Electronic Research Archive, 2023, 31(6): 3264-3290. doi: 10.3934/era.2023165 |
[7] | Ying Luo, Yanyan Chen, Kaiming Lu, Jian Zhang, Tao Wang, Zhiyan Yi . On the driver's stochastic nature in car-following behavior: Modeling and stabilizing based on the V2I environment. Electronic Research Archive, 2023, 31(1): 342-366. doi: 10.3934/era.2023017 |
[8] | Jiaqi Chang, Xuhan Xu . Network structure of urban digital financial technology and its impact on the risk of commercial banks. Electronic Research Archive, 2022, 30(12): 4740-4762. doi: 10.3934/era.2022240 |
[9] | Meng Gao, Xiaohui Ai . A stochastic Gilpin-Ayala nonautonomous competition model driven by mean-reverting OU process with finite Markov chain and Lévy jumps. Electronic Research Archive, 2024, 32(3): 1873-1900. doi: 10.3934/era.2024086 |
[10] | Najmeddine Attia, Ahmed Ghezal . Global stability and co-balancing numbers in a system of rational difference equations. Electronic Research Archive, 2024, 32(3): 2137-2159. doi: 10.3934/era.2024097 |
In this paper, Kou's method is extended to solve nonlinear systems. The convergence order of the iterative method is proved. Using fractal theory, we study the theoretical operators related to the iterative method, and analyze the stability of the iterative method. Properties related to strange fixed points and critical points are explored. The fractal results indicate that the iterative method is most stable when the parameter equals zero. The extended iterative method is applied to solve the Hammerstein equation and some nonlinear systems. The dynamic plane and numerical experiments show that the extended iterative method can solve the nonlinear system of equations with good convergence and stability.
Globally, road traffic accidents result in approximately 1.3 million fatalities and an estimated 50 million injuries annually [1]. Road intersections are frequent sites of such accidents. In the United States alone, there were 9.14 million vehicle collisions in 2020, with 2.23 million occurring at intersections and 2.09 million being intersection-related incidents [2]. In China, around 35% of accidents occur at road intersections each year [3].
Urban intersections play a crucial role within the road traffic network, serving as crucial hubs for distributing traffic flow. Usually, during periods of heavy traffic, vehicles near intersections tend to move slowly and form queues. The formation and dissipation process of vehicle queue length at intersections is influenced by signal control and varies with the cycle state. Accurate estimation of vehicle queuing delay information can assist traffic engineers in effectively evaluating target control strategies and identifying issues in traffic management, thereby aiding decision makers in enhancing traffic control methods [4,5]. Simultaneously, it enables road users to make optimal travel decisions by selecting the best departure time and route to avoid unnecessary delays and wasted travel costs [4,5]. As an important indicator, queuing delay not only directly evaluates intersection operational efficiency, lane design rationality, fuel consumption, and emissions but also reflects the level of obstruction faced by road users as well as their perception of intersection service quality. Consequently, numerous studies have been conducted on queue length evaluation models [6,7,8,9], queue waiting time models [10,11,12], and queue dissipation time models [13,14,15] at intersections.
For an intersection with a vehicle queue present, the intersection clearing speed, which refers to the average speed of queued vehicles passing through the intersection from the front to the back, determines the duration required for dissipating the queue. Generally, a higher clearing speed during queue discharge indicates greater capacity for the intersection [16]. Various factors influence this clearing speed; among them is driver's behavior such as queue-jumping which significantly reduces intersection clearance speeds consequently increasing queuing vehicles' delay time.
Queue-jumping refers to the act of a vehicle bypassing a parking queue or slow-moving traffic, disregarding the established order and forcefully merging into the target lane by abruptly cutting in front of another vehicle. During this lane-changing, the queue-jumping vehicle fails to yield to vehicles already in the target lane, significantly disrupting their normal flow. Queue-jumping is considered as an uncivilized driving behavior and was identified as the most irritating driving habit among 2.52 million individuals surveyed in China [17]. From a legal standpoint, queue-jumping also constitutes an illegal driving behavior. Both China's Road Traffic Safety Law (RTSL) and Road Traffic Safety Law Implementation Regulations (RTSLIR) explicitly prohibit queue-jumping behavior. For instance, Article 45 of RTSL stipulates that "When encountering a motor vehicle parked or moving slowly ahead in line, it is prohibited to overtake or occupy the opposite lane or jump the queue." Similarly, Article 53 of RTSLIR states that "When a motor vehicle stops or moves slowly ahead in line, vehicles behind should wait their turn without interpenetration or overtaking on either side."
Despite being prohibited by traffic laws and regulations and provoking annoyance among other road users, a significant number of drivers persist in flouting these regulations through engaging in queue-jumping behavior. This behavior is particularly prevalent at urban intersections within medium-sized and small cities across the country in China. The high frequency of drivers' queue-jumping behavior at urban intersections has detrimental effects on road traffic. On the one hand, it can cause traffic jams and reduce traffic efficiency, and on the other hand, it can lead to traffic conflicts, which increases the probability of accidents and poses a significant safety threat to other traffic participants. According to statistics [17], traffic accidents caused by queue-jumping account for approximately 38.5% of the total number of minor accidents every year in China.
In China, the primary focus of traffic managers has been on effectively enforcing regulations against illegal driving behaviors observed at urban intersections, such as running red lights, exceeding speed limits, crossing solid lines, and disregarding designated guidance lanes. This enforcement is facilitated through the utilization of electronic police systems. However, there exist technical complexities in accurately detecting instances of queue-jumping behavior via these electronic police systems, potentially leading to inadequate penalties being imposed. At the same time, drivers have little understanding of the harm of queue-jumping at urban intersections, and they have received insufficient publicity and education of relevant laws and regulations. Despite the recognition of frequent queue-jumping in China, current management measures have not effectively addressed this issue. Therefore, more attention should be paid to such an improper driving behavior.
The driver plays a crucial role in road accidents as they serve as both the processor and decision-maker of traffic information. Traffic violations are identified as one of the primary causes of traffic accidents, with statistics indicating that approximately 90% of traffic crashes in China can be attributed to such violations [18]. Consequently, the driver becomes central to issues related to illegal and unsafe driving behavior. Therefore, when investigating queue-jumping behavior at urban intersections, it is essential to focus on analyzing drivers' social psychological factors. This analysis will provide a foundation for implementing behavioral interventions at urban intersections. However, previous research primarily focused on highlighting the harmfulness and adverse impact of drivers' queue-jumping behavior on road traffic [19,20,21,22,23,24,25,26,27,28,29,30,31], with only a few studies exploring their motivation. Wang and Wang [32] suggested that from a psychological perspective, anxiety arising from prolonged waiting is the main influencing factor leading drivers to jump queues. Yang et al. [33] discovered significant correlations between queue-jumping behavior and Type A personality traits, cognitions, and driver skills. Nevertheless, these studies did not analyze the relationship between queue-jumping behavior and drivers' demographic information while also neglecting certain underlying psychosocial factors.
In light of the aforementioned issues, we aim to investigate potential demographic differences in drivers' self-reported queue-jumping behavior at urban intersections through the analysis of questionnaire data. At the same time, we aim to identify the socio-psychological factors that influence such behavior. Furthermore, we aim to propose relevant traffic management, traffic safety education and publicity measures based on the above analysis results to promote intervention and mitigate the occurrence of this behavior. Specifically, we employ the TPB as an analytical framework to examine the determinants of queue-jumping behavior.
TPB offers a conceptual model that aids decision-makers in identifying influential factors affecting specific behavioral patterns and devising effective intervention strategies [34,35]. Notably, TPB has been extensively utilized within the transportation domain, encompassing studies on travel mode preference [36,37], satisfaction with bus services [38] and ride-hailing services [39], analysis of risky driving behaviors [40,41,42,43,44,45,46,47,48,49,50,51,52,53,54,55,56,57,58,59,60] among others, yielding promising outcomes. To enhance our comprehension of queue-jumping behavior, we extend the TPB model by incorporating two additional variables commonly utilized in investigations concerning other driving behaviors: moral norms and perceived risk. The contributions of this study are as follows:
1) This study statistically analyzes and confirms potential demographic differences (gender, age, driving experience, driving frequency, etc.) in drivers' queue-jumping behavior at urban intersections.
2) This study proposes an extended TPB model by introducing moral norms and perceived risk as supplementary factors to determine the significant influences on drivers' queue-jumping behavior and behavioral intention at urban intersections.
3) Based on the identified factors influencing drivers' queue-jumping behavior at urban intersections, this study presents corresponding coping strategies and management measures from three perspectives: traffic management, traffic safety education, and media publicity.
The following sections of this paper are structured as follows. First, there is a review and summary of relevant research work, which mainly includes two aspects: research on drivers' queue-jumping behavior and TPB related research on drivers' traffic violation behavior. The study framework and related research hypotheses are also proposed. Second, the materials and methods used in this paper are provided. Third, the results are described. Fourth, the findings and implications for intervention are discussed. Finally, the conclusions are summarized.
Many scholars have extensively investigated drivers' queue-jumping behavior on road sections. Hallmark et al. [19] and Sun et al. [20] conducted research on drivers' queue-jumping behavior at work zones, revealing that such behavior significantly increased the likelihood of sudden deceleration or even braking by vehicles behind, often leading to anger or aggressive responses from other drivers. Shang et al. [21] investigated queue-jumping behavior at expressway off-ramps using cellular automata simulation, demonstrating its adverse impact on average vehicle delay time. Ren et al. [22] demonstrated that queue-jumping behavior was one of the top three direct causes of road rage. The likelihood of queue-jumping leading to road rage was associated with the driver's weekly driving frequency and age; those who drove more frequently per week were more prone to experiencing road rage as a result of queue-jumping behavior. Ning et al.'s work [23,24] indicated a strong correlation between sideswipe collisions in urban expressway traffic accidents and queue-jumping behavior; furthermore, collisions related to queue-jumping had the most substantial impact on crash duration. Yang et al. [33] developed a questionnaire to investigate drivers' queue-jumping behavior (both on road sections and at intersections), and the findings revealed that attitude, risk perception, driving skill, and safety motivation were significant factors. Lajunen et al. [61] employed a British version of a driver anger scale that included items related to queue-jumping to examine the association between self-reported general aggressiveness, driver anger, and aggressive driving. The results indicated that queue-jumping could cause extreme aggression. Taking into full consideration the unique traffic and driving conditions in China, Xie et al. [62], Li et al. [63], and Li et al. [64] incorporated items related to queue-jumping behavior when modifying the driver behavior questionnaire (DBQ). Similarly, Abid et al. [65] developed an extended DBQ that specifically included items on drivers' queue-jumping behavior in Pakistan. Li et al. [66,67] argued that competitive driving during traffic jams (including queue-jumping) aimed at gaining spatial advantage was primarily influenced by social environment factors. Liew et al. [68] found no significant difference in terms of gender or driving experience regarding queue-jumping; however, male drivers were observed to engage in this practice more frequently than female drivers. On conducting a questionnaire survey among adolescents regarding their perception of potential causes of traffic accidents, Chee [69] identified that speeding, inattention, and queue-jumping ranked in the top three.
Some studies have focused on queue-jumping behavior at urban intersections. Liu [25] conducted a risk assessment study on different types of risky lane-changing behaviors at signalized intersections, revealing that sudden queue-jumping when starting up posed the highest level of risk for drivers. Yang and Jia [26] investigated queue-jumping behavior among vehicles at urban road-rail crossings, finding that larger vehicles exhibited higher rates of this behavior compared to smaller ones. At bottlenecks, queue-jumping could significantly impact traffic conditions and lead to rapid declines in capacity. Huang [27] demonstrated that both successful and unsuccessful attempts at queue-jumping had adverse effects on safe driving for surrounding vehicles, with failed attempts having greater impacts than successful ones. Hao et al. [28,29,30] reported that queue-jumping behavior accounted for 28.79% of the total number of risky lane-changing at intersections, and posed a high-risk level. Ahmed et al. [31] highlighted how motorbike riders' tendency toward queue-jumping behavior could affect travel time and traffic flow in micro simulations. Wang et al. [32] conducted an analysis of queue-jumping among drivers and found that the anxiety while waiting at traffic lights and large gaps between vehicles were significant influencing factors. Li et al.' study [70], which analyzed the queue-jumping behavior at signalized intersections based on prospect theory, identified three primary influencing factors: traffic management policy, the delayed, and audit probability. Li et al. [71] applied evolutionary game theory to analyze drivers' choice of queuing or queue-jumping strategies over a period of time and found that when traffic management departments promptly penalized drivers for illegal actions in a timely manner, greater benefits would be brought to all involved parties. Qin et al. [72,73] identified queue-jumping as one of the most common forms of aggressive driving at urban intersections, and drivers were more likely to engage in this behavior during peak hours than off-peak hours. Li et al. [74] showed that drivers with more aggressive tendencies are more likely to engage in high-risk lane-changing (including queue-jumping) behaviors at intersections.
In summary, the aforementioned studies on drivers' queue-jumping behavior, both on road sections and at intersections, indicate a frequent occurrence of this aggressive driving behavior which has detrimental effects on traffic flow, safety, and driver emotions. Furthermore, it is influenced by various factors; however, the mechanism of its occurrence remains unclear.
By enhancing the theory of reasoned action (TRA) [75], Azjen [34,35] put forward a well-known social cognitive theory called TPB to elucidate behavioral decision-making processes. In TPB (see Figure 1), intention can be predicted by attitude (AT), subjective norms (SN), and perceived behavioral control (PBC), and behavior can be directly predicted by behavioral intention (BI) and PBC.
As a comprehensive social theoretical model, TPB has been extensively applied in the field of safe driving research. For instance, it has been used to explain behaviors such as phone usage while driving [40,41,42,43,44,45,46,47,48,49,50,51,52], speeding [53,54,55], drunk driving [56,57], illegal lane-changing [58,59], and blocking ambulances [60], among others. The flexibility of TPB allows for the inclusion of additional factors like moral norms (MN) and perceived risk (PR) to enhance its explanatory power for specific behaviors. Table 1 summarizes the application of TPB along with these additional factors in studying illegal driving behavior.
Behavior | AT | SN | PBC | MN | PR | Country | Sample size | Ref. |
Phone usage while driving | √ | √# | √# | — | √d# | Australia | 796 | [40] |
× | √ | × | √ | — | Australia | 133 | [41] | |
√ | √ | √ | √ | — | Australia | 171 | [42] | |
√ | × | × | √ | — | UK | 150 | [43] | |
√ | √ | √ | √ | — | USA | 243 | [44] | |
√ | √ | √ | √ | — | Australia | 114 | [45] | |
√ | √# | √# | — | √c# | Poland | 298 | [46] | |
√# | ×# | √# | — | √c | Australia | 551/559 | [47] | |
√ | √ | √ | √ | — | Iran | 257 | [48] | |
× | √ | × | √ | — | USA | 259 | [49] | |
√ | √ | √ | √ | — | China | 286 | [50] | |
× | × | √ | — | √ | Ethiopia | 155 | [51] | |
√ | × | × | — | √# | UK | 314 | [52] | |
Speeding | √ | √ | √# | √ | — | UK | 83/303 | [53] |
√ | √ | √ | √ | √ | Australia | 126 | [54] | |
√ | × | × | — | √ | China | 952 | [55] | |
Drunk driving | √ | × | √ | √ | — | Norway | 879 | [56] |
√ | × | √ | √ | — | China | 459 | [57] | |
Illegal lane-changing | √ | √ | √ | — | — | China | 506 | [58] |
√ | √ | √ | × | √ | China | 515 | [59] | |
Not giving way to ambulances | √ | × | √ | √ | — | China | 423 | [60] |
Note: √: significant; : × non-significant. # Some driving scenarios. c Crash risk; d Apprehension risk. |
Through literature analysis presented in Table 1, it is evident that attitude, subjective norms, and PBC within the fundamental TPB model are closely associated with drivers' intentions and violations of traffic rules. Therefore, this study establishes a model for drivers' queue-jumping behavior based on the basic TPB model.
As depicted in Table 1, moral norms and perceived risk emerge as two significant factors influencing drivers' violation behavior. Moral norms pertain to an individual's assessment of whether a particular behavior is right or wrong based on societal values [76]. The behavior of queue-jumping at urban intersections is generally regarded as an uncivilized driving behavior. When drivers make decisions on queue-jumping, they are more or less likely to consider the ethical implications of this behavior. In the current study, we hypothesized that moral norms would also play a significant role in affecting drivers' queue-jumping behavior at urban intersections.
Perceived risk means a person's awareness of possible risks linked with specific behaviors, including crash risk and apprehension risk [40,46,47]. Considering that drivers would have certain subjective judgments on the possibility of being involved in a traffic crash and the likelihood of being pulled over by law enforcement when deciding to jump the queue at urban intersections, we introduced perceived risk as an additional factor.
Based on the above analysis of potential influencing factors and the summary of existing studies, we constructed an extended TPB model to describe drivers' queue-jumping behavior at urban intersections. Figure 2 shows the model framework.
The initial hypotheses to be examined in this study are as follows.
H1: Behavioral intention affects queue-jumping behavior at urban intersections.
H2: PBC affects queue-jumping behavior at urban intersections.
H3: Attitude affects queue-jumping behavioral intention.
H4: Subjective norms affect queue-jumping behavioral intention.
H5: PBC affects queue-jumping behavioral intention.
H6: Moral norms affect queue-jumping behavioral intention.
H7: Perceived risk affects queue-jumping behavioral intention.
H8: PBC indirectly affects queue-jumping behavior at urban intersections through behavioral intention.
A pilot survey was conducted among 15 drivers to identify items for inclusion in the preliminary questionnaire. Detailed participant information and relevant interview materials can be found in Supplementary. Open-ended questions were asked regarding participants' attitudes toward queue-jumping at urban intersections, including opinions about this behavior from their family members and friends, among others. The most frequently provided answers were selected to design corresponding items. For instance, concerning the question "Can you think of any advantages of queue-jumping when driving near an urban intersection?", five responses related to saving time or not wasting time while six responses mentioned arriving quickly at a destination or passing through the intersection as fast as possible. Subsequently, we developed corresponding items based on these responses and previous research. Pre-tests were also conducted to assess the items prior to the formal survey.
The formal questionnaire comprised three sections. The first section served as an introduction, expressing gratitude toward participants and providing information about the purpose, organizer, and utilization of relevant data. Participants were informed that their participation was voluntary, they could discontinue filling out the questionnaire at any time, and their information would be strictly confidential. Additionally, participants were provided with an explanation of queue-jumping at the investigated intersection and its regulation measures.
Personal attributes of participants including gender, age, education level, etc., were collected in the second section. The third section consisted of the questions corresponding to observed indexes of each latent variable in the model shown in Figure 2.
The formal questionnaire was administered online through the Wenjuanxing application—a professional online platform for questionnaire surveys, examinations, assessments, and voting in China. Participants were recruited via various popular social media chat-groups. Participation was limited to individuals aged 18 years or above who possessed a valid driver's license and regularly drove cars. Each Internet protocol address was restricted to one participation. The average completion time for the online survey was approximately 20 minutes. The survey took place over a duration of one month.
At the end, out of 550 invited drivers, 456 completed the questionnaire. However, after careful screening, 29 responses were deemed invalid. Incomplete responses or those with missing values in any questions were excluded. Some participants completed the survey faster than required, some participants consistently selected the same option for all questions, some participants' age was obviously inconsistent with their driving experience, some participants' age was obviously inconsistent with their educational background, and so on. The responses of these participants were also excluded. Therefore, a total of 427 valid questionnaires were collected.
The basic information of participants is shown in Table 2. Of the 427 participants, 274 were male (64.2%) and 153 were female (35.8%). Additionally, 35.8% of them fell within the age range of 30 to 39 years old. The majority (32.8%) reported driving a car for 4–6 hours per week, while 70.8% had a driving experience ranging from 2 to 10 years.
Items | Number | Percent | Items | Number | Percent | ||
Gender | Male | 274 | 64.2% | Education | < high school | 73 | 17.1% |
Female | 153 | 35.8% | high school | 130 | 30.4% | ||
Age (years) | 18–29 | 114 | 26.7% | Undergraduate | 155 | 36.3% | |
30–39 | 153 | 35.8% | > Undergraduate | 69 | 16.2% | ||
40–49 | 94 | 22.0% | Driving Frequency (hours/week) |
< 4 | 84 | 19.7% | |
≥ 50 | 66 | 15.5% | 4–6 | 140 | 32.8% | ||
Driving Experience (years) | < 2 | 102 | 23.9% | 7–10 | 86 | 20.1% | |
2–5 | 151 | 35.4% | 11–15 | 63 | 14.8% | ||
6–10 | 151 | 35.4% | > 15 | 54 | 12.6% | ||
> 10 | 23 | 5.3% |
In the third section of the questionnaire, 7 constructs and 19 corresponding items are compiled on the ground of pilot survey results as well as previous studies (see Table 1). Table 3 provides a detailed description of the questionnaire.
Constructs | Code | Items |
Attitude (AT) | AT1 | Jumping the queue near an urban intersection gives me a sense of pleasure. |
AT2 | Jumping the queue near an urban intersection gives me a sense of accomplishment. | |
AT3 | It saves me time by jumping the queue near an urban intersection. | |
AT4 | It makes me get to my destination faster by jumping the queue near an urban intersection. | |
Strongly disagree ○1 ○2 ○3 ○4 ○5 Strongly agree | ||
Subjective norms (SN) | SN1 | My family would not stop me from jumping the queue near an urban intersection. |
SN2 | My friends would not stop me from jumping the queue near an urban intersection. | |
Strongly disagree ○1 ○2 ○3 ○4 ○5 Strongly agree | ||
Perceived behavior control (PBC) | PBC1 | It is an easy driving task for me to jump the queue near an urban intersection. |
PBC2 | I am able to respond quickly to all kinds of emergencies when I conduct queue-jumping behavior near an urban intersection. | |
Strongly disagree ○1 ○2 ○3 ○4 ○5 Strongly agree | ||
Moral norms (MN) | MN1 | I would feel guilty if I jump the queue near an urban intersection. |
MN2 | For me, the act of queue-jumping near an urban intersection is within the scope of uncivilized driving. | |
Strongly disagree ○1 ○2 ○3 ○4 ○5 Strongly agree | ||
Perceived risk (PR) | PR1 | To what extent do you agree that it is likely you will have a crash if you conduct queue-jumping near an urban intersection? |
PR2 | To what extent do you agree that it is likely you will encounter an emergency if you conduct queue-jumping near an urban intersection? | |
PR3 | To what extent do you agree that it is likely you will be fined by the police if you conduct queue-jumping near an urban intersection? | |
PR4 | To what extent do you agree that it is likely you will be caught by the police or be captured by the video surveillance if you conduct queue-jumping near an urban intersection? | |
No extent at all ○1 ○2 ○3 ○4 ○5 A great extent | ||
Behavioral intention (BI) | BI1 | In the coming 3 months, I will plan to conduct queue-jumping behavior near an urban intersection. |
BI2 | In the coming 3 months, if conditions permit, I will plan to conduct queue-jumping behavior near an urban intersection. | |
BI3 | In the coming 3 months, in the event that I need to jump the queue, I will plan to conduct queue-jumping behavior near an urban intersection. | |
Strongly disagree ○1 ○2 ○3 ○4 ○5 Strongly agree | ||
Self-reported queue-jumping behavior (QJ) | QJ1 | I used to conduct queue-jumping behavior near an urban intersection when I needed to in the past 6 months. |
Strongly disagree ○1 ○2 ○3 ○4 ○5 Strongly agree | ||
QJ2 | How often have you conducted queue-jumping behavior near an urban intersection in the past 6 months? | |
Never ○1 ○2 ○3 ○4 ○5 Very often |
To examine potential demographic differences in drivers' self-reported queue-jumping behavior at urban intersections, we employed Pearson's chi-square test after data clearing was performed. We used Cronbach's alpha (α) values to test item consistency. To explore the questionnaire structure, explanatory factor analysis (EFA) using principal component factor analysis (PCA) with varimax-rotation was conducted. All these analyses were performed using SPSS 25.0.
There are two widely employed SEM methods: Covariance-based structural equation modeling (CB-SEM) and partial least squares based structural equation modeling (PLS-SEM). CB-SEM model is more suitable for factor-based models, whereas PLS-SEM is better suited for composite-based models [77]. In our study, the proposed conceptual model is a factor-based model; hence CB-SEM is deemed more appropriate.
A SEM model consists of two components: the measurement model and structural model [78,79,80,81,82,83]. The measurement model underwent confirmatory factor analysis (CFA) for testing purposes in this study. Both CFA and the structural model tests were conducted using AMOS 24.0. Selecting an appropriate method is crucial when evaluating CFA and the structural model. Bootstrapping, a non-parametric resampling technique, evaluates the variability of a statistic by analyzing sample data variability instead of relying on parametric assumptions to assess estimate precision [84]. Utilizing bootstrapping offers several advantages in this context [85,86]. First, this method is easily understandable and does not require extensive knowledge of mathematics or the probability theory. Second, the statistical assumptions underlying bootstrapping are quite flexible, which is particularly advantageous when dealing with empirical data that often deviate from restrictive assumptions like (multivariate) normality in many statistical models. These deviations can affect the validity of statistical inferences drawn from the data. Third, bootstrapping has wide-ranging applicability and serves as a viable solution in situations where conventional methods may be challenging or even unattainable to employ.
The use of CB-SEM with bootstrapping enables researchers to assess the stability of parameter estimates and can be applied in situations where assumptions of large sample size and multivariate normality may not hold. Previous research [87] also demonstrated that results obtained from CB-SEM with bootstrapping and variance-based SEM (also known under the term PLS-SEM) are essentially similar. Therefore, we utilized maximum likelihood estimation method along with bootstrapping technique employing 1000 samples to estimate both measurement model and structural model in AMOS 24.0.
Throughout the data analysis process described above, several evaluation criteria were employed. Table 4 lists the relevant evaluation criteria along with their recommended values [78,79,80,81,82,83].
Stage | Indices | Abbreviation | Recommended criteria |
EFA | Cronbach's Alpha | α | > 0.7 |
Bartlett's Test of Sphericity | BTS | p–Value < 0.05 | |
Kaiser-Meyer-Olkin test | KOM | > 0.6 | |
Factor Loadings | > 0.6 | ||
CFA | Standardized Regression Weights | > 0.6 | |
Composite Reliability | CR | > 0.7 | |
Average Variance Extracted | AVE | 0.5 < AVE < CR; > Inter-construct correlations |
|
Maximum Shared Variance | MSV | MSV < AVE | |
Average Shared Variance | ASV | ASV < AVE | |
CFA and structural model | Normed Chi-square | 2/df | 1 < 2/df < 3 |
Comparative Fit Index | CFI | > 0.90 | |
Tucker-Lewis Index | TLI | > 0.90 | |
Incremental Fit Index | IFI | > 0.90 | |
Root-Mean-Square Error of Approximation | RMSEA | < 0.06 | |
Test of Close Fit | PCLOSE | > 0.50 |
The analysis involved conducting a Pearson's chi-square test to explore potential demographic variations in drivers' self-reported queue-jumping behavior at urban intersections. The results indicated no significant differences among drivers' queue-jumping behavior based on their educational background, driving experience, or driving frequency. However, as shown in Table 5, significant differences were observed when considering the gender and age groups of drivers. Male drivers (M1 = 2.24, SD1 = 1.07; M2 = 1.92, SD2 = 0.94) exhibited a higher tendency to partake in queue-jumping at urban intersections compared to female drivers (M1 = 1.92, SD1 = 0.97; M2 = 1.73, SD2 = 0.89). Additionally, it was observed that individuals aged between 18-29 (M1 = 2.27, SD1 = 0.99; M2 = 1.97, SD2 = 0.97) exhibited a higher propensity for engaging in this behavior compared to the other three age groups.
Item | QJ1 | QJ2 | |||||||
(1) | (2)–(5) | M1 | SD1 | (1) | (2)–(5) | M2 | SD2 | ||
Gender | Male | 18.1% | 46.1% | 2.24 | 1.07 | 27.2% | 37.0% | 1.92 | 0.94 |
Female | 15.2% | 20.6% | 1.92 | 0.97 | 19.9% | 15.9% | 1.73 | 0.89 | |
Sum | 33.3% | 66.7% | 47.1% | 52.9% | |||||
= 13.473**, p = 0.009 | = 10.544*, p = 0.032 | ||||||||
Age | 18–29 | 6.6% | 20.1% | 2.27 | 0.99 | 10.8% | 15.9% | 1.97 | 0.97 |
30–39 | 10.5% | 25.3% | 2.21 | 1.06 | 14.3% | 21.5% | 1.87 | 0.95 | |
40–49 | 11.5% | 10.5% | 1.79 | 1.03 | 14.5% | 7.5% | 1.69 | 0.89 | |
≥ 50 | 4.7% | 10.8% | 2.17 | 1.05 | 7.5% | 8.0% | 1.83 | 0.81 | |
Sum | 33.3% | 66.7% | 47.1% | 52.9% | |||||
= 27.234**, p = 0.007 | = 22.037*, p = 0.037 | ||||||||
Note: (1) Never/Strongly Disagree; (2) Occasionally/Disagree; (3) Sometimes/Neutral; (4) Often/Agree; (5) Very often/Strongly Agree. * p0.05, ** p0.01. |
We performed an EFA using PCA with varimax rotation to investigate whether constructs loaded as expected, exhibited adequate correlation, and satisfied reliability and validity requirements. Prior to conducting the EFA, we assessed the questionnaire's suitability by employing Bartlett's test of sphericity and Kaiser-Meyer-Olkin (KMO) measure of sampling adequacy. The findings indicated that KMO = 0.826 > 0.6 and Bartlett's test was statistically significant at p = 0.000 with an Approx. Chi-square = 40673987 and degrees of freedom equal to 171, suggesting that performing a PCA was appropriate for our study.
Table 6 presents the factor loadings for each individual item which all exceeded a threshold of 0.6 ranging from 0.672 to 0.908 without any cross-loadings among them. Thus, no items required elimination from the questionnaire. Ultimately, our comprehensive seven-factor model accounted for a substantial proportion (77.45%) of the total variance. Moreover, all seven extracted factors exhibited eigenvalues greater than 1.0.
Constructs | Items | Loadings | Cronbach's α | Variance explained | Cumulative variance explained |
AT | AT1 | 0.672 | 0.797 | 13.83% | 13.83% |
AT2 | 0.783 | ||||
AT3 | 0.809 | ||||
AT4 | 0.788 | ||||
SN | SN1 | 0.874 | 0.802 | 8.92% | 22.75% |
SN2 | 0.869 | ||||
MN | MN1# | 0.908 | 0.866 | 9.29% | 32.04% |
MN2# | 0.883 | ||||
PBC | PBC1 | 0.829 | 0.797 | 8.51% | 40.55% |
PBC2 | 0.855 | ||||
PR | PR1# | 0.841 | 0.861 | 15.44% | 55.99% |
PR2# | 0.837 | ||||
PR3# | 0.754 | ||||
PR4# | 0.797 | ||||
BI | BI1 | 0.769 | 0.869 | 12.16% | 68.15% |
BI2 | 0.848 | ||||
BI3 | 0.779 | ||||
QJ | QJ1 | 0.876 | 0.868 | 9.30% | 77.45% |
QJ2 | 0.873 | ||||
Note: # The scores were reversed (the same below). |
To assess reliability for each factor, Cronbach's α coefficient was calculated resulting in values ranging from 0.797 to 0.869 as presented in Table 6. All factors exceeded the cutoff criterion of 0.7 for reliability values.
Figure 3 and Table 7 depict both the measurement model and the evaluation results. It showed that all fit indexes of the measurement model (see Figure 3) met the recommended criteria, which provided evidence of good model fit. Each construct in the measurement model exhibited significant associations with all of its items, and the standardized regression weight of each item exceeded 0.6 (see Table 7).
Constructs | Items | β | Std. Error | 95% Confidence Intervals | p | |
Lower | Upper | |||||
AT | AT1 | 0.680** | 0.039 | 0.592 | 0.749 | 0.003 |
AT2 | 0.704** | 0.036 | 0.635 | 0.779 | 0.002 | |
AT3 | 0.824** | 0.034 | 0.748 | 0.889 | 0.003 | |
AT4 | 0.757*** | 0.036 | 0.690 | 0.828 | 0.001 | |
SN | SN1 | 0.785** | 0.043 | 0.694 | 0.866 | 0.002 |
SN2 | 0.853** | 0.046 | 0.770 | 0.954 | 0.002 | |
MN | MN1 | 0.821** | 0.038 | 0.743 | 0.899 | 0.002 |
MN2 | 0.930** | 0.040 | 0.853 | 1.009 | 0.003 | |
PBC | PBC1 | 0.853** | 0.038 | 0.773 | 0.926 | 0.003 |
PBC2 | 0.779** | 0.036 | 0.700 | 0.846 | 0.003 | |
PR | PR1 | 0.830** | 0.019 | 0.791 | 0.866 | 0.002 |
PR2 | 0.873** | 0.018 | 0.834 | 0.905 | 0.002 | |
PR3 | 0.712** | 0.030 | 0.649 | 0.768 | 0.002 | |
PR4 | 0.743*** | 0.027 | 0.692 | 0.792 | 0.001 | |
BI | BI1 | 0.836** | 0.019 | 0.797 | 0.874 | 0.002 |
BI2 | 0.901** | 0.016 | 0.864 | 0.928 | 0.004 | |
BI3 | 0.772** | 0.023 | 0.722 | 0.812 | 0.002 | |
QJ | QJ1 | 0.869** | 0.030 | 0.806 | 0.929 | 0.002 |
QJ2 | 0.888** | 0.031 | 0.828 | 0.952 | 0.002 | |
Note: p0.01; *** p0.001. β (Standardized regression weight); Std. Error (Standardized error). |
To assess the convergent validity, we computed CR and AVE values. As depicted in Table 8, all CR values ranged from 0.800 to 0.876, consistently surpassing the minimum threshold value of 0.70, thereby indicating reliable measures. AVEs ranged from 0.553 to 0.772, exceeding the recommended level of 0.50, and confirming the convergent validity of the measurement model.
Constructs | CR | AVE | MSV | ASV | AT | SN | MN | PBC | PR | BI | QJ |
AT | 0.831 | 0.553 | 0.204 | 0.093 | 0.744 a | ||||||
SN | 0.804 | 0.672 | 0.142 | 0.087 | 0.185 b | 0.820 a | |||||
MN | 0.869 | 0.770 | 0.149 | 0.089 | 0.253 b | 0.243 b | 0.877 a | ||||
PBC | 0.800 | 0.667 | 0.169 | 0.124 | 0.366 b | 0.266 b | 0.274 b | 0.817 a | |||
PR | 0.870 | 0.628 | 0.175 | 0.112 | 0.212 b | 0.377 b | 0.274 b | 0.373 b | 0.792 a | ||
BI | 0.876 | 0.702 | 0.204 | 0.169 | 0.452 b | 0.364 b | 0.386 b | 0.411 b | 0.418 b | 0.838 a | |
QJ | 0.871 | 0.772 | 0.187 | 0.114 | 0.275 b | 0.236 b | 0.334 b | 0.394 b | 0.310 b | 0.433 b | 0.879 a |
Note: a ; b Inter-construct correlations. |
To effectively evaluate discriminant validity, the square root of AVE (on diagonal in Table 8) was compared with inter-construct correlations for each construct pairwise comparison. This analysis revealed that for each construct was greater than its corresponding inter-construct correlation coefficient, suggesting good discrimination validity. The MSV and ASV values were also calculated. As shown in Table 8, the results indicated that both MSV and ASV were lower than AVE values, further confirming good discrimination validity.
Taken together, both the obtained strong convergent and discriminative validities of the measurement model support further evaluation of the structural model.
The first step in structural evaluation is assessing collinearity within the structural model [88]. To test linearity, a regression analysis was conducted to examine relationships between variables directly connected by a path. As shown in Table 9, our findings demonstrated that both BI (β = 0.326, p = 0.000) and PBC (β = 0.260, p = 0.000) were positively related to QJ. Additionally, AT (β = 0.282, p = 0.000), SN (β = 0.163, p = 0.000), PBC (β = 0.139, p = 0.002), MN (β = 0.182, p = 0.000), and PR (β = 0.202, p = 0.000) were all positively correlated with BI.
Independent Variables | t | p | VIF | |
Dependent Variable: QJ | ||||
Intercept | 0.717*** | 5.849 | 0.000 | |
PBC | 0.260*** | 5.620 | 0.000 | 1.203 |
BI | 0.326*** | 7.041 | 0.000 | 1.203 |
Dependent Variable: BI | ||||
Intercept | –0.627*** | -3.879 | 0.000 | |
AT | 0.282*** | 6.819 | 0.000 | 1.197 |
SN | 0.163*** | 3.965 | 0.000 | 1.185 |
PBC | 0.139** | 3.178 | 0.002 | 1.332 |
MN | 0.182*** | 4.455 | 0.000 | 1.171 |
PR | 0.202*** | 4.722 | 0.000 | 1.282 |
Note: β (Standardized regression coefficients); VIF (Variance inflation factor). ** p0.01; *** p0.001. |
We also assessed whether multicollinearity was an issue by calculating variance inflation factor (VIF) values. Results showed that all VIF values were below 5.0, very close to 1.0, suggesting no presence of multilinearity.
The structural model is depicted in Figure 4, which demonstrates that all fit indices meet the recommended criteria, indicating a satisfactory fit of the data to the model. Path analysis was conducted to test all proposed hypotheses, as presented in Table 10.
Hypotheses | Paths | β | 95% Confidence Intervals | p | Results | |
Lower | Upper | |||||
H1 | QJBI | 0.391** | 0.265 | 0.508 | 0.002 | Supported |
H2 | QJPBC | 0.282** | 0.146 | 0.418 | 0.002 | Supported |
H3 | BIAT | 0.316** | 0.202 | 0.403 | 0.005 | Supported |
H4 | BISN | 0.175** | 0.060 | 0.282 | 0.002 | Supported |
H5 | BIPBC | 0.122* | 0.031 | 0.252 | 0.05 | Supported |
H6 | BIMN | 0.184** | 0.078 | 0.284 | 0.002 | Supported |
H7 | BIPR | 0.230*** | 0.128 | 0.349 | 0.001 | Supported |
H8 | QJPBC (Total) | 0.330** | 0.188 | 0.458 | 0.002 | Supported |
QJBIPBC (Indirect) | 0.048* | 0.001 | 0.102 | 0.037 | ||
QJPBC (Direct) | 0.282** | 0.146 | 0.418 | 0.002 | ||
Note: * p0.05; ** p0.01; *** p0.001. |
According to Table 10, it was found that all paths were statistically significant, providing support for all proposed hypotheses.
The standardized regression weight value between BI and QJ was 0.391 (p = 0.002), which was the largest value, suggesting that drivers' intention toward queue-jumping at urban intersections was the strongest predictor of this behavior. This confirmed that hypothesis H1 was supported. Another predictor of QJ was PBC with a path coefficient of 0.282 (p = 0.002). PBC was also positively associated with QJ, which supported hypothesis H2.
As hypothesized, AT (β = 0.316, p = 0.005), SN (β = 0.175, p = 0.002), PBC (β = 0.122, p = 0.05), MN (β = 0.184, p = 0.002), and PR (β = 0.230, p = 0.001) were all found to have a positive association with BI. Consequently, hypotheses H3-H7 were supported as well.
To test hypothesis H8, we conducted a mediation analysis using the bootstrapping method to examine whether PBC had an indirect effect on QJ through BI. As presented in Table 10, the total effect (β = 0.330, p = 0.002), indirect effect (β = 0.048, p = 0.037), and direct effect (β = 0.282, p = 0.002) were all statistically significant, confirming support for hypothesis H8.
Additionally, we also assessed the explanatory power of the structural model by calculating squared multiple correlations. The results indicated that the structural model explained 48.9% of the variance in behavioral intention (R2 = 0.489, p = 0.009) and 33.8% of the variance in queue-jumping behavior (R2 = 0338, p = 0.004). Therefore, the extended TPB model proposed could effectively explain drivers' behavioral intentions and behaviors related to queue-jumping at urban intersections.
Urban intersections are susceptible to traffic congestion and accidents, with drivers' queue-jumping behavior exacerbating the disorder of traffic operation and creating new safety hazards. Queue-jumping is an uncivilized and illegal conduct that disrupts normal traffic order, negatively impacts other road users, and undermines fairness in travel. This behavior has a high prevalence in many small and medium-sized cities in China, with studies [19,20,21,22,23,24,25,26,27,28,29,30,31] confirming its detrimental effects. The objective of this study was to investigate the influencing factors of queue-jumping at urban intersections from the perspective of drivers' social psychology based on an extended TPB. These findings can help traffic managers to better understand the causes behind this behavior to some extent, enabling them to implement targeted management strategies and interventions.
Previous studies [89,90,91,92,93,94,95,96,97,98] have demonstrated a correlation between drivers' age, gender, and other demographic factors with risky driving behaviors, traffic offences, and traffic accidents. In our study, we identified that gender and age as significant influencing factors for queue-jumping at urban intersections.
Liew et al. [68] reported that male drivers were more inclined toward queue-jumping compared to female drivers; however, the gender difference was not found to be statistically significant. Contrary to their findings, our study revealed significant disparities between male and female drivers in engaging in queue-jumping behavior. This discrepancy may stem from variations in social psychology between males and females. Specifically, research [99] has shown that males tend to exhibit a higher propensity for risk-taking than females. Moreover, males demonstrate greater competitiveness [100], lower inclination toward altruistic behavior [101,102], and less cooperation compared to their female counterparts [103]. Females also exhibit higher levels of integrity when it comes to honesty relative to males [104]. Lastly, when faced with moral dilemmas, females display a stronger aversion toward causing harm than males do [105].
Previous research has consistently demonstrated that young drivers are more prone to engaging in risky driving behaviors, including distracted driving [41,42,44,45,47,49], speeding [54,106,107,108], risky lane-changing [58,59], driving while intoxicated [109,110], driving while drowsy [111], driving without seat belts [112]. Consequently, they have a higher likelihood of being involved in traffic accidents. In our study, we observed a significant age disparity regarding queue-jumping behavior at intersections. Specifically, drivers aged between 18 and 29 exhibited a greater propensity for this improper behavior compared to other age groups. It is worth noting that young drivers generally engage in riskier driving behaviors [113] and possess an elevated level of confidence in their driving abilities [90]. Furthermore, they tend to hold more favorable attitudes toward violating lane change regulations [58,59].
Overall, the male and young driver group should be the focus of attention in the management of queue-jumping behavior at urban intersections.
As expected, the extended TPB was confirmed to be a model with high efficiency. It explained 48.9% of the variance in behavioral intention and 33.8% of the variance in the queue-jumping behavior at urban intersections. All the basic TPB factors and the additional TPB factors were significantly associated with drivers' behavioral intention and the behavior.
In our study, intention (β = 0.391, p = 0.002) emerged as the strongest predictor of the queue-jumping behavior in our study. Drivers who had a strong intention to conduct queue-jumping at urban intersections were more likely to do so. However, behavioral intention was more explained than queue-jumping behavior in our model. This is a common result that has been found in the application of TPB, which is taken as "the intention-behavior gap" [114,115]. Although research [116] argued that TPB could better explain behavioral intention than behavior, a strong intention could be also likely to predict the behavior.
PBC was a significant predictor of queue-jumping behavior (β = 0.282, p = 0.002) as well as behavioral intention (β = 0.122, p = 0.05). Drivers who viewed queue-jumping as easier to implement and easier to deal with unexpected situations were more inclined toward intending and engaging in such behavior. PBC reflects the driver's confidence in his/her driving skills. When the driver is too confident in his/her driving skills, he/she is more likely to underestimate the risk of driving violations. In our study, PBC could not only directly affect the queue-jumping behavior at urban intersections, but also indirectly affect the behavior through behavioral intention, which supported hypothesis H8. Therefore, the intervention measures for queue-jumping behavior at urban intersections should also fully consider the PBC.
Studies [33,40,42,43,44,45,46,48,50,52,53,54,55,56,57,58,59,60] showed that attitude was an important influencing factor of illegal driving. In our study, attitude (β = 0.316, p = 0.005) was the best predictor of behavioral intention. Attitude also had an indirect effect on queue-jumping behavior by behavioral intention. The drivers who hold a more positive attitude toward queue-jumping behavior at urban intersections, that was, they thought that it could bring them convenience and achievement, then the more inclined they were to plan to implement this behavior. Therefore, it was very important to help drivers establish a good and correct attitude toward the behavior of queue-jumping at urban intersections.
Subjective norms are considered to be the weakest predictors in the TPB [41]. Contrary to some studies [43,51,52,55,56,57,60] on risky driving behavior that showed that subjective norms were not a significant influencing factor, in our study, subjective norms (β = 0.175, p = 0.002) demonstrated significant association with behavioral intention and had an indirect effect on queue-jumping behavior at urban intersections. It meant that the attitudes or opinions of drivers' family members or friends more or less played a role in their queue-jumping decision-making.
Along with the factors mentioned above, we introduced two additional influencing factors. Previous studies have not considered the role of moral norms in drivers' queue-jumping behavior. However, our study found that moral norms (β = 0.184, p = 0.002) exhibited significant correlation with behavioral intention. The more a driver perceived queue-jumping was wrong, the less likely he/she planned to do so. Our findings also demonstrated that moral norms played an important role in decision-making in terms of social morality and behavior related to social merit and civilization [76].
According to previous studies [51,54,55,59], drivers' risky driving behavior could be predicted by perceived risk. Yang et al. [33] showed that risk perception could affect drivers' queue-jumping behavior. However, they only considered the crash risk and did not consider the apprehension risk. In fact, both types of the perceived risk would affect driving behavior [40,46,47]. Different from previous studies, in our study, both crash risk and apprehension risk were included in the questionnaire, and the results indicated that they both had a substantial impact (β = 0.230, p = 0.001) on behavioral intention toward queue-jumping. The less risk drivers were aware of, the more willing they were to jump the queue at urban intersections.
Numerous studies have proposed variety of methods to address risky driving behaviors such as traffic law enforcement [117,118,119,120], media publicity [121,122,123,124], and public education [125,126,127]. Our findings suggested that intervention strategies should target all fundamental TPB factors as well as the two additional TPB factors identified in this study. Additionally, particular attention should be given to male drivers and young drivers. Based on these findings, we proposed relevant intervention and coping strategies from three perspectives: traffic management, traffic safety education, and media publicity.
● Traffic management
Li et al. [71] showed that timely punishment by traffic management departments of queue-jumping at urban intersections could discourage drivers from this behavior. In China, traffic managers have primarily focused on strict enforcement of illegal driving behaviors at urban intersections such as red-light running, speeding, crossing solid lines, and not using designated guidance lanes with the assistance of the electronic police systems. However, there are technical challenges involved in identifying queue-jumping behavior through electronic police systems, which may result in insufficient punishment being imposed. Traffic management departments can enhance the deterrent effect on queue-jumping behavior by strengthening on-site patrol and law enforcement, installing dedicated cameras, and implementing other measures.
In addition, traffic management departments can install warning signs near some intersections where queue-jumping behavior is serious to remind drivers. There are usually two types of lane-changing near intersections for drivers: co-directional lane-changing and turning lane-changing [128]. In general, the frequency of queue-jumping behavior is higher due to turning lane-changing than co-directional lane-changing at urban intersections. Therefore, it is crucial for traffic management departments to enhance the existing lane selection guidance system, enabling drivers to promptly choose the appropriate lane at the correct place, which has been proven effective [129].
● Traffic safety education
In China, driver training primarily focuses on driving skills within driving schools. Although there is some traffic safety education provided, it requires further strengthening. Driving schools and instructors should employ various methods, such as simulation driving lessons, to raise students' awareness of the hazards associated with queue-jumping. Traffic management departments should also reinforce re-learning and retraining programs for drivers who have been punished for this offense in order to prevent recurrences.
Our findings indicated that family members and friends exert influence over drivers. Therefore, safety education should encompass not only drivers but also their relatives and friends. A positive family safety climate will significantly impact drivers in a beneficial manner [130]. Furthermore, previous studies [131,132,133] have demonstrated the positive impact of peer education on young drivers, making it a valuable component in education programs.
● Media publicity
To enhance its effectiveness, it is recommended to employ new media platforms such as micro-video to actively disseminate information about the dangers of queue-jumping behavior through reporting real-life traffic accident cases. Additionally, efforts should be made to strengthen public awareness regarding the penalties associated with queue-jumping by promoting RTSL and RTSLIR. This multifaceted approach aims to increase drivers' understanding of both the crash risk and apprehension risk from authorities, as well as the consequences and costs associated with engaging in such an improper behavior. Furthermore, traffic management departments can establish an online platform for exposing illegal driving behaviors, and regularly publish punishment cases related to queue-jumping incidents. Moreover, these departments can also set up positive role models to foster a favorable social atmosphere that promotes safe and civilized driving practices.
This study examined the factors that contributed to drivers' tendency to jump the queue at urban intersections, using an expanded TPB framework. The results revealed significant differences based on gender and age regarding this behavior. Specifically, male drivers and younger drivers were found to be more inclined toward queue-jumping compared to their female counterparts and older drivers, respectively. By incorporating moral norms and perceived risk as additional factors, the extended TPB model effectively accounted for drivers' queue-jumping behavior at urban intersections. Attitude, subjective norms, perceived behavioral control, moral norms, perceived risk, and behavioral intention all emerged as significant predictors of self-reported queue-jumping among drivers at urban intersections.
Our findings have some important implications for interventions aimed at reducing such behavior among drivers for traffic management departments and driving schools. It is recommended that traffic management departments should enhance the enforcement of queue-jumping behavior at urban intersections, optimize the design of relevant warning facilities and lane selection guidance systems, and intensify safety education for drivers while promoting awareness of pertinent laws and regulations through diverse means. Additionally, driving schools should improve training and educational content on queue-jumping behavior to ensure comprehensive understanding among driving students regarding its associated risks.
Several limitations exist in this study. First, expanding the sample size is necessary to ensure greater representation among respondents. Second, in the questionnaire, we did not collect the income, occupation and other relevant personal information of the participants. However, these factors may also influence drivers' queue-jumping behavior. Third, while moral norms and perceived risk were included as additional factors within the TPB, other potential significant predictors such as anticipated regret [42,45,47,53,76], social environment [66,67], driving habits [110,112,134] identified in previous research should be considered in future investigations. Finally, although TPB partially explains drivers' risky driving behavior, according to some studies [135,136], it has certain limitations due to its assumption that driver decisions are entirely rational and planned. Drivers not only engage in deliberative decision-making during actual driving but also make reactive decisions based on different situations. Therefore, it is crucial to consider drivers' reactive decisions regarding queue-jumping behavior at urban intersections in further research.
The authors declare they have not used Artificial Intelligence (AI) tools in the creation of this article.
This work was supported by the Fundamental Research Funds for the Central Universities of China (No. 2019-zy-062). The authors thank all the anonymous participants who took part in the online survey.
The authors declare there is no conflict of interest.
No. | Gender | Age | Education | Driving experience (years) | Driving frequency (hours per week) | Queue-jumping behavior |
1 | Male | 28 | 3 | 6 | 3 | 3 |
2 | Male | 26 | 2 | 3 | 4 | 5 |
3 | Female | 34 | 4 | 5 | 2 | 1 |
4 | Male | 55 | 1 | 12 | 5 | 4 |
5 | Male | 24 | 2 | 4 | 3 | 4 |
6 | Female | 27 | 3 | 2 | 1 | 1 |
7 | Male | 46 | 2 | 8 | 2 | 2 |
8 | Female | 57 | 4 | 13 | 3 | 2 |
9 | Male | 38 | 2 | 7 | 3 | 2 |
10 | Female | 22 | 2 | 1 | 1 | 1 |
11 | Female | 41 | 3 | 2 | 2 | 1 |
12 | Male | 62 | 1 | 15 | 2 | 3 |
13 | Male | 27 | 3 | 3 | 2 | 2 |
14 | Male | 36 | 3 | 6 | 3 | 3 |
15 | Female | 24 | 3 | 2 | 1 | 1 |
Note: Education (1 = "Below high school", 2 = "High school", 3= "Undergraduate", 4 = "Above undergraduate"); Driving frequency (1 = " < 4", 2 = "4-6", 3 = "7-10", 4 = "11-15", 5 = " > 15"/hours per week); Past queue-jumping behavior experience in the last 6 months (1 = "Never", 2 = "Occasionally", 3 = "Sometimes", 4 = "Often", 5 = "Very often"). |
Q1. Gender □ Male □ Female
Q2. Your age: ________.
Q3. Your education level:
□ Below high school □ High school □ Undergraduate □ Above undergraduate
Q4. Your driving experience (the time from you getting a driving license): years.
Q5. How many hours do you drive on average per week?
□ 0-4 h □ 4-6 h □ 7-10 h □ 11-15 h □ above 15 h
Q6. Your queue-jumping behavior experience at urban intersections in the past 6 months?
Never ○ 1 ○ 2 ○ 3 ○ 4 ○ 5 Very often
Q7. What do you think about queue-jumping when driving near an urban intersection?
Q8. Can you think of any advantages of queue-jumping when driving near an urban intersection?
Q9. Can you think of any disadvantages of queue-jumping when driving near an urban intersection?
Q10. Can you think of anyone who would approve of your behavior of queue-jumping when driving near an urban intersection?
Q11. Can you think of anyone who would disapprove of your behavior of queue-jumping when driving near an urban intersection?
Q12. Can you think of the challenge to driving skills during queue-jumping when driving near an urban intersection?
Q13. Can you think of the challenge to emergency response capability of driving during queue-jumping near an urban intersection?
Q14. What are your thoughts about the impact of queue-jumping near an urban intersection on the other drivers?
Q15. What do you think about the impact of queue-jumping near an urban intersection on the society?
Q16. What are your thoughts about the risk factors for traffic accidents of queue-jumping near an urban intersection?
Q17. Do you think people should be punished for queue-jumping near an urban intersection? Is it likely?
Q18. Have you ever been punished for queue-jumping near an urban intersection?
Q19. In what type of situation might a person conduct queue-jumping near an urban intersection?
Q20. Can you think of any reasons or circumstances that would motivate you to conduct queue-jumping near an urban intersection?
Q21. Can you think of any reasons or circumstances that would refrain you from queue-jumping near an urban intersection?
[1] |
C. Li, H. Zhang, X. Yang, A new linearized ADI compact difference method on graded meshes for a nonlinear 2D and 3D PIDE with a WSK, Comput. Math. Appl., 176 (2024), 349–370. https://doi.org/10.1016/j.camwa.2024.11.006 doi: 10.1016/j.camwa.2024.11.006
![]() |
[2] |
H. Zhang, X. Jiang, F. Wang, X. Yang, The time two-grid algorithm combined with difference scheme for 2D nonlocal nonlinear wave equation, J. Appl. Math. Comput., 70 (2024), 1127–1151. https://doi.org/10.1007/s12190-024-02000-y doi: 10.1007/s12190-024-02000-y
![]() |
[3] |
X. Yang, Z. Zhang, On conservative, positivity preserving, nonlinear FV scheme on distorted meshes for the multi-term nonlocal Nagumo-type equations, Appl. Math. Lett., 150 (2024), 108972. https://doi.org/10.1016/j.aml.2023.108972 doi: 10.1016/j.aml.2023.108972
![]() |
[4] |
Z. Chen, H. Zhang, H. Chen, Adi compact difference scheme for the two-dimensional integro-differential equation with two fractional Riemann–Liouville integral kernels, Fractal Fract., 8 (2024), 707. https://doi.org/10.3390/fractalfract8120707 doi: 10.3390/fractalfract8120707
![]() |
[5] |
J. Wang, X. Jiang, X. Yang, H. Zhang, A new robust compact difference scheme on graded meshes for the time-fractional nonlinear Kuramoto-Sivashinsky equation, Comput. Appl. Math., 43 (2024), 381. https://doi.org/10.1007/s40314-024-02883-4 doi: 10.1007/s40314-024-02883-4
![]() |
[6] |
X. Shen, X. Yang, H. Zhang, The high-order ADI difference method and extrapolation method for solving the two-dimensional nonlinear parabolic evolution equations, Mathematics, 12 (2024), 3469. https://doi.org/10.3390/math12223469 doi: 10.3390/math12223469
![]() |
[7] |
X. Yang, W. Wang, Z. Zhou, H. Zhang, An efficient compact difference method for the fourth-order nonlocal subdiffusion problem, Taiwan. J. Math., 29 (2025), 35–66. https://doi.org/10.11650/tjm/240906 doi: 10.11650/tjm/240906
![]() |
[8] | X. Yang, Z. Zhang, Superconvergence analysis of a robust orthogonal Gauss collocation method for 2D fourth-order subdiffusion equations, J. Sci. Comput., 100 (2024). https://doi.org/10.1007/s10915-024-02616-z |
[9] |
N. Sidorov, Solvability of Nonlinear equations with parameters: Branching, regularization, group symmetry and solutions blow-up, Symmetry, 14 (2022), 226. https://doi.org/10.3390/sym14020226 doi: 10.3390/sym14020226
![]() |
[10] |
Q. Zhu, Event-triggered sampling problem for exponential stability of stochastic nonlinear delay systems driven by Levy processes, IEEE Trans. Autom. Control, 70 (2025), 1176–1183. http://doi.org/10.1109/TAC.2024.3448128 doi: 10.1109/TAC.2024.3448128
![]() |
[11] |
X. Yang, Z. Zhang, Analysis of a new NFV scheme preserving DMP for two-dimensional sub-diffusion equation on distorted meshes, J. Sci. Comput., 99 (2024), 80. https://doi.org/10.1007/s10915-024-02511-7 doi: 10.1007/s10915-024-02511-7
![]() |
[12] | J. Wang, X. Jiang, X. Yang, H. Zhang, A compact difference scheme for mixed-type time-fractional black-scholes equation in European option pricing, Math. Methods Appl. Sci., 2025. https://doi.org/10.1002/mma.10717 |
[13] |
K. R. Nilay, A new semi-explicit atomistic molecular dynamics simulation method for membrane proteins, J. Comput. Methods Sci. Eng., 19 (2019), 259–286. https://doi.org/10.3233/jcm-180851 doi: 10.3233/jcm-180851
![]() |
[14] | X. Wang, N. Shang, Local convergence analysis of a novel derivative-free method with and without memory for solving nonlinear systems, Int. J. Comput. Math., (2025), 1–18. http://doi.org/10.1080/00207160.2025.2464701 |
[15] | D. Ruan, X. Wang, Y. Wang, Local convergence of seventh-order iterative method under weak conditions and its applications, Eng. Comput., 2025. http://doi.org/10.1108/EC-08-2024-0775 |
[16] |
D. Ruan, X. Wang, A high-order Chebyshev-type method for solving nonlinear equations: local convergence and applications, Electron. Res. Arch., 33 (2025), 1398–1413. https://doi.org/10.3934/era.2025065 doi: 10.3934/era.2025065
![]() |
[17] | X. Wang, W. Li, Fractal behavior of King's optimal eighth-order iterative method and its numerical application, Math. Comm., 29 (2024), 217–236. |
[18] |
X. Wang, T. Zhang, Y. Qin, Efficient two-step derivative-free iterative methods with memory and their dynamics, Int. J. Comput. Math., 93 (2015), 1423–1446. http://doi.org/10.1080/00207160.2015.1056168 doi: 10.1080/00207160.2015.1056168
![]() |
[19] |
A. Cordero, J. R. Torregrosa, M. P. Vassileva, Three-step iterative methods with optimal eighth-order convergence, J. Comput. Appl. Math., 235 (2011), 3189–3194. http://doi.org/10.1016/j.cam.2011.01.004 doi: 10.1016/j.cam.2011.01.004
![]() |
[20] |
A. Cordero, M. Moscoso-Martinez, J. R. Torregrosa, Chaos and stability in a new iterative family for solving nonlinear equations, Algorithms, 14 (2021), 101. http://doi.org/10.3390/a14040101 doi: 10.3390/a14040101
![]() |
[21] |
J. M. Ortega, W. C, Rheinboldt, Iterative solution of nonlinear equations in several variables, SIAM, 52 (2000), 521–572. https://doi.org/10.1137/1.9780898719468.bm doi: 10.1137/1.9780898719468.bm
![]() |
[22] | F. A. Potra, V. Pta'k, Nondiscrete induction and iterative processes. SIAM Rev., 1984. https://doi.org/10.1137/1029105 |
[23] |
A. Cordero, J. R. Torregrosa, On interpolation variants of Newton's method for functions of several variables, J. Comput. Appl. Math., 234 (2010), 34–43. https://doi.org/10.1016/j.cam.2009.12.002 doi: 10.1016/j.cam.2009.12.002
![]() |
[24] |
J. Kou, Y. Li, X. Wang, A composite fourth-order iterative method for solving non-linear equations, Appl. Math. Comput., 184 (2007), 471–475. http://doi.org/10.1016/j.amc.2006.05.181 doi: 10.1016/j.amc.2006.05.181
![]() |
[25] | K. Falconer, Fractal geometry: mathematical foundations and applications, Wiley, 2013. |
[26] | B. B. Mandelbrot, Fractal geometry: what is it, and what does it do?, Proc. R. Soc. Lond. A Math. Phys. Sci., 423 (1989), 3–16. https://doi.org/10.1098/rspa.1989.0038 |
[27] |
M. Y. Lee, Y. I. Kim, The dynamical analysis of a uniparametric family of three-point optimal eighth-order multiple-root finders under the Möbius conjugacy map on the Riemann sphere, Numer. Algorithms, 83 (2020), 1063–1090. https://doi.org/10.1007/s11075-019-00716-8 doi: 10.1007/s11075-019-00716-8
![]() |
[28] |
X. Wang, W. Li, Choosing the best members of the optimal eighth-order Petković's family by its fractal behavior, Fractal Fract., 6 (2022), 749. http://doi.org/10.3390/fractalfract6120749 doi: 10.3390/fractalfract6120749
![]() |
[29] |
S. Amat, S. Busquier, S. Plaza, Chaotic dynamics of a third-order Newton-type method, J. Math. Anal. Appl., 366 (2010), 24–32. https://doi.org/10.1016/j.jmaa.2010.01.047 doi: 10.1016/j.jmaa.2010.01.047
![]() |
[30] |
A. Cordero, T. Lotfi, K. Mahdiani, J. R. Torregrosa, A stable family with high order of convergence for solving nonlinear equations, Appl. Numer. Math., 254 (2015), 240–251. https://doi.org/10.1016/j.amc.2014.12.141 doi: 10.1016/j.amc.2014.12.141
![]() |
[31] |
H. H. H. Homeier, On Newton-type methods with cubic convergence, J. Comput. Appl. Math., 176 (2005), 425–432. https://doi.org/10.1016/j.cam.2004.07.027 doi: 10.1016/j.cam.2004.07.027
![]() |
[32] |
C. Chun, Some third-order families of iterative methods for solving nonlinear equations, Appl. Math. Comput., 188 (2007), 924–933. https://doi.org/10.1016/j.amc.2006.10.043 doi: 10.1016/j.amc.2006.10.043
![]() |
[33] |
A. Cordero, J. R. Torregrosa, Variants of Newton's method using fifth-order quadrature formulas, Appl. Math. Comput., 190 (2007), 686–698. https://doi.org/10.1016/j.amc.2007.01.062 doi: 10.1016/j.amc.2007.01.062
![]() |
[34] |
J. Kou, On Chebyshev-Halley methods with sixth-order convergence for solving non-linear equations, Appl. Math. Comput., 190 (2007), 126–131. https://doi.org/10.1016/j.amc.2007.01.011 doi: 10.1016/j.amc.2007.01.011
![]() |
[35] |
Y. I. Kim, R. Behl, S. S. Motsa, Higher-order efficient class of Chebyshev-Halley-type methods, Appl. Math. Comput., 273 (2016), 1148–1159. https://doi.org/10.1016/j.amc.2015.09.013 doi: 10.1016/j.amc.2015.09.013
![]() |
[36] |
S. Qureshi, A. Soomro, A. A. Shaikh, E. Hincal, N. Gokbulut, A novel multistep iterative technique for models in medical sciences with complex dynamics, Comput. Math. Methods Med., 2022 (2022), 7656451. https://doi.org/10.1155/2022%2F7656451 doi: 10.1155/2022%2F7656451
![]() |
[37] |
J. R. Sharma, S. Kumar, H. Singh, A new class of derivative-free root solvers with increasing optimal convergence order and their complex dynamics, SeMA J., 80 (2023), 333–352. https://doi.org/10.1007/s40324-022-00288-z doi: 10.1007/s40324-022-00288-z
![]() |
[38] |
O. S. Solaiman, I. Hashim, Two new efficient sixth order iterative methods for solving nonlinear equations, J. King Saud Univ. Sci., 31 (2019), 701–705. https://doi.org/10.1016/j.jksus.2018.03.021 doi: 10.1016/j.jksus.2018.03.021
![]() |
[39] |
J. Kou, Y. Li, X. Wang, A modification of Newton method with third-order convergence, Appl. Numer. Math., 181 (2019), 1106–1111. https://doi.org/10.1016/j.amc.2006.01.076 doi: 10.1016/j.amc.2006.01.076
![]() |
Behavior | AT | SN | PBC | MN | PR | Country | Sample size | Ref. |
Phone usage while driving | √ | √# | √# | — | √d# | Australia | 796 | [40] |
× | √ | × | √ | — | Australia | 133 | [41] | |
√ | √ | √ | √ | — | Australia | 171 | [42] | |
√ | × | × | √ | — | UK | 150 | [43] | |
√ | √ | √ | √ | — | USA | 243 | [44] | |
√ | √ | √ | √ | — | Australia | 114 | [45] | |
√ | √# | √# | — | √c# | Poland | 298 | [46] | |
√# | ×# | √# | — | √c | Australia | 551/559 | [47] | |
√ | √ | √ | √ | — | Iran | 257 | [48] | |
× | √ | × | √ | — | USA | 259 | [49] | |
√ | √ | √ | √ | — | China | 286 | [50] | |
× | × | √ | — | √ | Ethiopia | 155 | [51] | |
√ | × | × | — | √# | UK | 314 | [52] | |
Speeding | √ | √ | √# | √ | — | UK | 83/303 | [53] |
√ | √ | √ | √ | √ | Australia | 126 | [54] | |
√ | × | × | — | √ | China | 952 | [55] | |
Drunk driving | √ | × | √ | √ | — | Norway | 879 | [56] |
√ | × | √ | √ | — | China | 459 | [57] | |
Illegal lane-changing | √ | √ | √ | — | — | China | 506 | [58] |
√ | √ | √ | × | √ | China | 515 | [59] | |
Not giving way to ambulances | √ | × | √ | √ | — | China | 423 | [60] |
Note: √: significant; : × non-significant. # Some driving scenarios. c Crash risk; d Apprehension risk. |
Items | Number | Percent | Items | Number | Percent | ||
Gender | Male | 274 | 64.2% | Education | < high school | 73 | 17.1% |
Female | 153 | 35.8% | high school | 130 | 30.4% | ||
Age (years) | 18–29 | 114 | 26.7% | Undergraduate | 155 | 36.3% | |
30–39 | 153 | 35.8% | > Undergraduate | 69 | 16.2% | ||
40–49 | 94 | 22.0% | Driving Frequency (hours/week) |
< 4 | 84 | 19.7% | |
≥ 50 | 66 | 15.5% | 4–6 | 140 | 32.8% | ||
Driving Experience (years) | < 2 | 102 | 23.9% | 7–10 | 86 | 20.1% | |
2–5 | 151 | 35.4% | 11–15 | 63 | 14.8% | ||
6–10 | 151 | 35.4% | > 15 | 54 | 12.6% | ||
> 10 | 23 | 5.3% |
Constructs | Code | Items |
Attitude (AT) | AT1 | Jumping the queue near an urban intersection gives me a sense of pleasure. |
AT2 | Jumping the queue near an urban intersection gives me a sense of accomplishment. | |
AT3 | It saves me time by jumping the queue near an urban intersection. | |
AT4 | It makes me get to my destination faster by jumping the queue near an urban intersection. | |
Strongly disagree ○1 ○2 ○3 ○4 ○5 Strongly agree | ||
Subjective norms (SN) | SN1 | My family would not stop me from jumping the queue near an urban intersection. |
SN2 | My friends would not stop me from jumping the queue near an urban intersection. | |
Strongly disagree ○1 ○2 ○3 ○4 ○5 Strongly agree | ||
Perceived behavior control (PBC) | PBC1 | It is an easy driving task for me to jump the queue near an urban intersection. |
PBC2 | I am able to respond quickly to all kinds of emergencies when I conduct queue-jumping behavior near an urban intersection. | |
Strongly disagree ○1 ○2 ○3 ○4 ○5 Strongly agree | ||
Moral norms (MN) | MN1 | I would feel guilty if I jump the queue near an urban intersection. |
MN2 | For me, the act of queue-jumping near an urban intersection is within the scope of uncivilized driving. | |
Strongly disagree ○1 ○2 ○3 ○4 ○5 Strongly agree | ||
Perceived risk (PR) | PR1 | To what extent do you agree that it is likely you will have a crash if you conduct queue-jumping near an urban intersection? |
PR2 | To what extent do you agree that it is likely you will encounter an emergency if you conduct queue-jumping near an urban intersection? | |
PR3 | To what extent do you agree that it is likely you will be fined by the police if you conduct queue-jumping near an urban intersection? | |
PR4 | To what extent do you agree that it is likely you will be caught by the police or be captured by the video surveillance if you conduct queue-jumping near an urban intersection? | |
No extent at all ○1 ○2 ○3 ○4 ○5 A great extent | ||
Behavioral intention (BI) | BI1 | In the coming 3 months, I will plan to conduct queue-jumping behavior near an urban intersection. |
BI2 | In the coming 3 months, if conditions permit, I will plan to conduct queue-jumping behavior near an urban intersection. | |
BI3 | In the coming 3 months, in the event that I need to jump the queue, I will plan to conduct queue-jumping behavior near an urban intersection. | |
Strongly disagree ○1 ○2 ○3 ○4 ○5 Strongly agree | ||
Self-reported queue-jumping behavior (QJ) | QJ1 | I used to conduct queue-jumping behavior near an urban intersection when I needed to in the past 6 months. |
Strongly disagree ○1 ○2 ○3 ○4 ○5 Strongly agree | ||
QJ2 | How often have you conducted queue-jumping behavior near an urban intersection in the past 6 months? | |
Never ○1 ○2 ○3 ○4 ○5 Very often |
Stage | Indices | Abbreviation | Recommended criteria |
EFA | Cronbach's Alpha | α | > 0.7 |
Bartlett's Test of Sphericity | BTS | p–Value < 0.05 | |
Kaiser-Meyer-Olkin test | KOM | > 0.6 | |
Factor Loadings | > 0.6 | ||
CFA | Standardized Regression Weights | > 0.6 | |
Composite Reliability | CR | > 0.7 | |
Average Variance Extracted | AVE | 0.5 < AVE < CR; > Inter-construct correlations |
|
Maximum Shared Variance | MSV | MSV < AVE | |
Average Shared Variance | ASV | ASV < AVE | |
CFA and structural model | Normed Chi-square | 2/df | 1 < 2/df < 3 |
Comparative Fit Index | CFI | > 0.90 | |
Tucker-Lewis Index | TLI | > 0.90 | |
Incremental Fit Index | IFI | > 0.90 | |
Root-Mean-Square Error of Approximation | RMSEA | < 0.06 | |
Test of Close Fit | PCLOSE | > 0.50 |
Item | QJ1 | QJ2 | |||||||
(1) | (2)–(5) | M1 | SD1 | (1) | (2)–(5) | M2 | SD2 | ||
Gender | Male | 18.1% | 46.1% | 2.24 | 1.07 | 27.2% | 37.0% | 1.92 | 0.94 |
Female | 15.2% | 20.6% | 1.92 | 0.97 | 19.9% | 15.9% | 1.73 | 0.89 | |
Sum | 33.3% | 66.7% | 47.1% | 52.9% | |||||
= 13.473**, p = 0.009 | = 10.544*, p = 0.032 | ||||||||
Age | 18–29 | 6.6% | 20.1% | 2.27 | 0.99 | 10.8% | 15.9% | 1.97 | 0.97 |
30–39 | 10.5% | 25.3% | 2.21 | 1.06 | 14.3% | 21.5% | 1.87 | 0.95 | |
40–49 | 11.5% | 10.5% | 1.79 | 1.03 | 14.5% | 7.5% | 1.69 | 0.89 | |
≥ 50 | 4.7% | 10.8% | 2.17 | 1.05 | 7.5% | 8.0% | 1.83 | 0.81 | |
Sum | 33.3% | 66.7% | 47.1% | 52.9% | |||||
= 27.234**, p = 0.007 | = 22.037*, p = 0.037 | ||||||||
Note: (1) Never/Strongly Disagree; (2) Occasionally/Disagree; (3) Sometimes/Neutral; (4) Often/Agree; (5) Very often/Strongly Agree. * p0.05, ** p0.01. |
Constructs | Items | Loadings | Cronbach's α | Variance explained | Cumulative variance explained |
AT | AT1 | 0.672 | 0.797 | 13.83% | 13.83% |
AT2 | 0.783 | ||||
AT3 | 0.809 | ||||
AT4 | 0.788 | ||||
SN | SN1 | 0.874 | 0.802 | 8.92% | 22.75% |
SN2 | 0.869 | ||||
MN | MN1# | 0.908 | 0.866 | 9.29% | 32.04% |
MN2# | 0.883 | ||||
PBC | PBC1 | 0.829 | 0.797 | 8.51% | 40.55% |
PBC2 | 0.855 | ||||
PR | PR1# | 0.841 | 0.861 | 15.44% | 55.99% |
PR2# | 0.837 | ||||
PR3# | 0.754 | ||||
PR4# | 0.797 | ||||
BI | BI1 | 0.769 | 0.869 | 12.16% | 68.15% |
BI2 | 0.848 | ||||
BI3 | 0.779 | ||||
QJ | QJ1 | 0.876 | 0.868 | 9.30% | 77.45% |
QJ2 | 0.873 | ||||
Note: # The scores were reversed (the same below). |
Constructs | Items | β | Std. Error | 95% Confidence Intervals | p | |
Lower | Upper | |||||
AT | AT1 | 0.680** | 0.039 | 0.592 | 0.749 | 0.003 |
AT2 | 0.704** | 0.036 | 0.635 | 0.779 | 0.002 | |
AT3 | 0.824** | 0.034 | 0.748 | 0.889 | 0.003 | |
AT4 | 0.757*** | 0.036 | 0.690 | 0.828 | 0.001 | |
SN | SN1 | 0.785** | 0.043 | 0.694 | 0.866 | 0.002 |
SN2 | 0.853** | 0.046 | 0.770 | 0.954 | 0.002 | |
MN | MN1 | 0.821** | 0.038 | 0.743 | 0.899 | 0.002 |
MN2 | 0.930** | 0.040 | 0.853 | 1.009 | 0.003 | |
PBC | PBC1 | 0.853** | 0.038 | 0.773 | 0.926 | 0.003 |
PBC2 | 0.779** | 0.036 | 0.700 | 0.846 | 0.003 | |
PR | PR1 | 0.830** | 0.019 | 0.791 | 0.866 | 0.002 |
PR2 | 0.873** | 0.018 | 0.834 | 0.905 | 0.002 | |
PR3 | 0.712** | 0.030 | 0.649 | 0.768 | 0.002 | |
PR4 | 0.743*** | 0.027 | 0.692 | 0.792 | 0.001 | |
BI | BI1 | 0.836** | 0.019 | 0.797 | 0.874 | 0.002 |
BI2 | 0.901** | 0.016 | 0.864 | 0.928 | 0.004 | |
BI3 | 0.772** | 0.023 | 0.722 | 0.812 | 0.002 | |
QJ | QJ1 | 0.869** | 0.030 | 0.806 | 0.929 | 0.002 |
QJ2 | 0.888** | 0.031 | 0.828 | 0.952 | 0.002 | |
Note: p0.01; *** p0.001. β (Standardized regression weight); Std. Error (Standardized error). |
Constructs | CR | AVE | MSV | ASV | AT | SN | MN | PBC | PR | BI | QJ |
AT | 0.831 | 0.553 | 0.204 | 0.093 | 0.744 a | ||||||
SN | 0.804 | 0.672 | 0.142 | 0.087 | 0.185 b | 0.820 a | |||||
MN | 0.869 | 0.770 | 0.149 | 0.089 | 0.253 b | 0.243 b | 0.877 a | ||||
PBC | 0.800 | 0.667 | 0.169 | 0.124 | 0.366 b | 0.266 b | 0.274 b | 0.817 a | |||
PR | 0.870 | 0.628 | 0.175 | 0.112 | 0.212 b | 0.377 b | 0.274 b | 0.373 b | 0.792 a | ||
BI | 0.876 | 0.702 | 0.204 | 0.169 | 0.452 b | 0.364 b | 0.386 b | 0.411 b | 0.418 b | 0.838 a | |
QJ | 0.871 | 0.772 | 0.187 | 0.114 | 0.275 b | 0.236 b | 0.334 b | 0.394 b | 0.310 b | 0.433 b | 0.879 a |
Note: a ; b Inter-construct correlations. |
Independent Variables | t | p | VIF | |
Dependent Variable: QJ | ||||
Intercept | 0.717*** | 5.849 | 0.000 | |
PBC | 0.260*** | 5.620 | 0.000 | 1.203 |
BI | 0.326*** | 7.041 | 0.000 | 1.203 |
Dependent Variable: BI | ||||
Intercept | –0.627*** | -3.879 | 0.000 | |
AT | 0.282*** | 6.819 | 0.000 | 1.197 |
SN | 0.163*** | 3.965 | 0.000 | 1.185 |
PBC | 0.139** | 3.178 | 0.002 | 1.332 |
MN | 0.182*** | 4.455 | 0.000 | 1.171 |
PR | 0.202*** | 4.722 | 0.000 | 1.282 |
Note: β (Standardized regression coefficients); VIF (Variance inflation factor). ** p0.01; *** p0.001. |
Hypotheses | Paths | β | 95% Confidence Intervals | p | Results | |
Lower | Upper | |||||
H1 | QJBI | 0.391** | 0.265 | 0.508 | 0.002 | Supported |
H2 | QJPBC | 0.282** | 0.146 | 0.418 | 0.002 | Supported |
H3 | BIAT | 0.316** | 0.202 | 0.403 | 0.005 | Supported |
H4 | BISN | 0.175** | 0.060 | 0.282 | 0.002 | Supported |
H5 | BIPBC | 0.122* | 0.031 | 0.252 | 0.05 | Supported |
H6 | BIMN | 0.184** | 0.078 | 0.284 | 0.002 | Supported |
H7 | BIPR | 0.230*** | 0.128 | 0.349 | 0.001 | Supported |
H8 | QJPBC (Total) | 0.330** | 0.188 | 0.458 | 0.002 | Supported |
QJBIPBC (Indirect) | 0.048* | 0.001 | 0.102 | 0.037 | ||
QJPBC (Direct) | 0.282** | 0.146 | 0.418 | 0.002 | ||
Note: * p0.05; ** p0.01; *** p0.001. |
No. | Gender | Age | Education | Driving experience (years) | Driving frequency (hours per week) | Queue-jumping behavior |
1 | Male | 28 | 3 | 6 | 3 | 3 |
2 | Male | 26 | 2 | 3 | 4 | 5 |
3 | Female | 34 | 4 | 5 | 2 | 1 |
4 | Male | 55 | 1 | 12 | 5 | 4 |
5 | Male | 24 | 2 | 4 | 3 | 4 |
6 | Female | 27 | 3 | 2 | 1 | 1 |
7 | Male | 46 | 2 | 8 | 2 | 2 |
8 | Female | 57 | 4 | 13 | 3 | 2 |
9 | Male | 38 | 2 | 7 | 3 | 2 |
10 | Female | 22 | 2 | 1 | 1 | 1 |
11 | Female | 41 | 3 | 2 | 2 | 1 |
12 | Male | 62 | 1 | 15 | 2 | 3 |
13 | Male | 27 | 3 | 3 | 2 | 2 |
14 | Male | 36 | 3 | 6 | 3 | 3 |
15 | Female | 24 | 3 | 2 | 1 | 1 |
Note: Education (1 = "Below high school", 2 = "High school", 3= "Undergraduate", 4 = "Above undergraduate"); Driving frequency (1 = " < 4", 2 = "4-6", 3 = "7-10", 4 = "11-15", 5 = " > 15"/hours per week); Past queue-jumping behavior experience in the last 6 months (1 = "Never", 2 = "Occasionally", 3 = "Sometimes", 4 = "Often", 5 = "Very often"). |
Behavior | AT | SN | PBC | MN | PR | Country | Sample size | Ref. |
Phone usage while driving | √ | √# | √# | — | √d# | Australia | 796 | [40] |
× | √ | × | √ | — | Australia | 133 | [41] | |
√ | √ | √ | √ | — | Australia | 171 | [42] | |
√ | × | × | √ | — | UK | 150 | [43] | |
√ | √ | √ | √ | — | USA | 243 | [44] | |
√ | √ | √ | √ | — | Australia | 114 | [45] | |
√ | √# | √# | — | √c# | Poland | 298 | [46] | |
√# | ×# | √# | — | √c | Australia | 551/559 | [47] | |
√ | √ | √ | √ | — | Iran | 257 | [48] | |
× | √ | × | √ | — | USA | 259 | [49] | |
√ | √ | √ | √ | — | China | 286 | [50] | |
× | × | √ | — | √ | Ethiopia | 155 | [51] | |
√ | × | × | — | √# | UK | 314 | [52] | |
Speeding | √ | √ | √# | √ | — | UK | 83/303 | [53] |
√ | √ | √ | √ | √ | Australia | 126 | [54] | |
√ | × | × | — | √ | China | 952 | [55] | |
Drunk driving | √ | × | √ | √ | — | Norway | 879 | [56] |
√ | × | √ | √ | — | China | 459 | [57] | |
Illegal lane-changing | √ | √ | √ | — | — | China | 506 | [58] |
√ | √ | √ | × | √ | China | 515 | [59] | |
Not giving way to ambulances | √ | × | √ | √ | — | China | 423 | [60] |
Note: √: significant; : × non-significant. # Some driving scenarios. c Crash risk; d Apprehension risk. |
Items | Number | Percent | Items | Number | Percent | ||
Gender | Male | 274 | 64.2% | Education | < high school | 73 | 17.1% |
Female | 153 | 35.8% | high school | 130 | 30.4% | ||
Age (years) | 18–29 | 114 | 26.7% | Undergraduate | 155 | 36.3% | |
30–39 | 153 | 35.8% | > Undergraduate | 69 | 16.2% | ||
40–49 | 94 | 22.0% | Driving Frequency (hours/week) |
< 4 | 84 | 19.7% | |
≥ 50 | 66 | 15.5% | 4–6 | 140 | 32.8% | ||
Driving Experience (years) | < 2 | 102 | 23.9% | 7–10 | 86 | 20.1% | |
2–5 | 151 | 35.4% | 11–15 | 63 | 14.8% | ||
6–10 | 151 | 35.4% | > 15 | 54 | 12.6% | ||
> 10 | 23 | 5.3% |
Constructs | Code | Items |
Attitude (AT) | AT1 | Jumping the queue near an urban intersection gives me a sense of pleasure. |
AT2 | Jumping the queue near an urban intersection gives me a sense of accomplishment. | |
AT3 | It saves me time by jumping the queue near an urban intersection. | |
AT4 | It makes me get to my destination faster by jumping the queue near an urban intersection. | |
Strongly disagree ○1 ○2 ○3 ○4 ○5 Strongly agree | ||
Subjective norms (SN) | SN1 | My family would not stop me from jumping the queue near an urban intersection. |
SN2 | My friends would not stop me from jumping the queue near an urban intersection. | |
Strongly disagree ○1 ○2 ○3 ○4 ○5 Strongly agree | ||
Perceived behavior control (PBC) | PBC1 | It is an easy driving task for me to jump the queue near an urban intersection. |
PBC2 | I am able to respond quickly to all kinds of emergencies when I conduct queue-jumping behavior near an urban intersection. | |
Strongly disagree ○1 ○2 ○3 ○4 ○5 Strongly agree | ||
Moral norms (MN) | MN1 | I would feel guilty if I jump the queue near an urban intersection. |
MN2 | For me, the act of queue-jumping near an urban intersection is within the scope of uncivilized driving. | |
Strongly disagree ○1 ○2 ○3 ○4 ○5 Strongly agree | ||
Perceived risk (PR) | PR1 | To what extent do you agree that it is likely you will have a crash if you conduct queue-jumping near an urban intersection? |
PR2 | To what extent do you agree that it is likely you will encounter an emergency if you conduct queue-jumping near an urban intersection? | |
PR3 | To what extent do you agree that it is likely you will be fined by the police if you conduct queue-jumping near an urban intersection? | |
PR4 | To what extent do you agree that it is likely you will be caught by the police or be captured by the video surveillance if you conduct queue-jumping near an urban intersection? | |
No extent at all ○1 ○2 ○3 ○4 ○5 A great extent | ||
Behavioral intention (BI) | BI1 | In the coming 3 months, I will plan to conduct queue-jumping behavior near an urban intersection. |
BI2 | In the coming 3 months, if conditions permit, I will plan to conduct queue-jumping behavior near an urban intersection. | |
BI3 | In the coming 3 months, in the event that I need to jump the queue, I will plan to conduct queue-jumping behavior near an urban intersection. | |
Strongly disagree ○1 ○2 ○3 ○4 ○5 Strongly agree | ||
Self-reported queue-jumping behavior (QJ) | QJ1 | I used to conduct queue-jumping behavior near an urban intersection when I needed to in the past 6 months. |
Strongly disagree ○1 ○2 ○3 ○4 ○5 Strongly agree | ||
QJ2 | How often have you conducted queue-jumping behavior near an urban intersection in the past 6 months? | |
Never ○1 ○2 ○3 ○4 ○5 Very often |
Stage | Indices | Abbreviation | Recommended criteria |
EFA | Cronbach's Alpha | α | > 0.7 |
Bartlett's Test of Sphericity | BTS | p–Value < 0.05 | |
Kaiser-Meyer-Olkin test | KOM | > 0.6 | |
Factor Loadings | > 0.6 | ||
CFA | Standardized Regression Weights | > 0.6 | |
Composite Reliability | CR | > 0.7 | |
Average Variance Extracted | AVE | 0.5 < AVE < CR; > Inter-construct correlations |
|
Maximum Shared Variance | MSV | MSV < AVE | |
Average Shared Variance | ASV | ASV < AVE | |
CFA and structural model | Normed Chi-square | 2/df | 1 < 2/df < 3 |
Comparative Fit Index | CFI | > 0.90 | |
Tucker-Lewis Index | TLI | > 0.90 | |
Incremental Fit Index | IFI | > 0.90 | |
Root-Mean-Square Error of Approximation | RMSEA | < 0.06 | |
Test of Close Fit | PCLOSE | > 0.50 |
Item | QJ1 | QJ2 | |||||||
(1) | (2)–(5) | M1 | SD1 | (1) | (2)–(5) | M2 | SD2 | ||
Gender | Male | 18.1% | 46.1% | 2.24 | 1.07 | 27.2% | 37.0% | 1.92 | 0.94 |
Female | 15.2% | 20.6% | 1.92 | 0.97 | 19.9% | 15.9% | 1.73 | 0.89 | |
Sum | 33.3% | 66.7% | 47.1% | 52.9% | |||||
= 13.473**, p = 0.009 | = 10.544*, p = 0.032 | ||||||||
Age | 18–29 | 6.6% | 20.1% | 2.27 | 0.99 | 10.8% | 15.9% | 1.97 | 0.97 |
30–39 | 10.5% | 25.3% | 2.21 | 1.06 | 14.3% | 21.5% | 1.87 | 0.95 | |
40–49 | 11.5% | 10.5% | 1.79 | 1.03 | 14.5% | 7.5% | 1.69 | 0.89 | |
≥ 50 | 4.7% | 10.8% | 2.17 | 1.05 | 7.5% | 8.0% | 1.83 | 0.81 | |
Sum | 33.3% | 66.7% | 47.1% | 52.9% | |||||
= 27.234**, p = 0.007 | = 22.037*, p = 0.037 | ||||||||
Note: (1) Never/Strongly Disagree; (2) Occasionally/Disagree; (3) Sometimes/Neutral; (4) Often/Agree; (5) Very often/Strongly Agree. * p0.05, ** p0.01. |
Constructs | Items | Loadings | Cronbach's α | Variance explained | Cumulative variance explained |
AT | AT1 | 0.672 | 0.797 | 13.83% | 13.83% |
AT2 | 0.783 | ||||
AT3 | 0.809 | ||||
AT4 | 0.788 | ||||
SN | SN1 | 0.874 | 0.802 | 8.92% | 22.75% |
SN2 | 0.869 | ||||
MN | MN1# | 0.908 | 0.866 | 9.29% | 32.04% |
MN2# | 0.883 | ||||
PBC | PBC1 | 0.829 | 0.797 | 8.51% | 40.55% |
PBC2 | 0.855 | ||||
PR | PR1# | 0.841 | 0.861 | 15.44% | 55.99% |
PR2# | 0.837 | ||||
PR3# | 0.754 | ||||
PR4# | 0.797 | ||||
BI | BI1 | 0.769 | 0.869 | 12.16% | 68.15% |
BI2 | 0.848 | ||||
BI3 | 0.779 | ||||
QJ | QJ1 | 0.876 | 0.868 | 9.30% | 77.45% |
QJ2 | 0.873 | ||||
Note: # The scores were reversed (the same below). |
Constructs | Items | β | Std. Error | 95% Confidence Intervals | p | |
Lower | Upper | |||||
AT | AT1 | 0.680** | 0.039 | 0.592 | 0.749 | 0.003 |
AT2 | 0.704** | 0.036 | 0.635 | 0.779 | 0.002 | |
AT3 | 0.824** | 0.034 | 0.748 | 0.889 | 0.003 | |
AT4 | 0.757*** | 0.036 | 0.690 | 0.828 | 0.001 | |
SN | SN1 | 0.785** | 0.043 | 0.694 | 0.866 | 0.002 |
SN2 | 0.853** | 0.046 | 0.770 | 0.954 | 0.002 | |
MN | MN1 | 0.821** | 0.038 | 0.743 | 0.899 | 0.002 |
MN2 | 0.930** | 0.040 | 0.853 | 1.009 | 0.003 | |
PBC | PBC1 | 0.853** | 0.038 | 0.773 | 0.926 | 0.003 |
PBC2 | 0.779** | 0.036 | 0.700 | 0.846 | 0.003 | |
PR | PR1 | 0.830** | 0.019 | 0.791 | 0.866 | 0.002 |
PR2 | 0.873** | 0.018 | 0.834 | 0.905 | 0.002 | |
PR3 | 0.712** | 0.030 | 0.649 | 0.768 | 0.002 | |
PR4 | 0.743*** | 0.027 | 0.692 | 0.792 | 0.001 | |
BI | BI1 | 0.836** | 0.019 | 0.797 | 0.874 | 0.002 |
BI2 | 0.901** | 0.016 | 0.864 | 0.928 | 0.004 | |
BI3 | 0.772** | 0.023 | 0.722 | 0.812 | 0.002 | |
QJ | QJ1 | 0.869** | 0.030 | 0.806 | 0.929 | 0.002 |
QJ2 | 0.888** | 0.031 | 0.828 | 0.952 | 0.002 | |
Note: p0.01; *** p0.001. β (Standardized regression weight); Std. Error (Standardized error). |
Constructs | CR | AVE | MSV | ASV | AT | SN | MN | PBC | PR | BI | QJ |
AT | 0.831 | 0.553 | 0.204 | 0.093 | 0.744 a | ||||||
SN | 0.804 | 0.672 | 0.142 | 0.087 | 0.185 b | 0.820 a | |||||
MN | 0.869 | 0.770 | 0.149 | 0.089 | 0.253 b | 0.243 b | 0.877 a | ||||
PBC | 0.800 | 0.667 | 0.169 | 0.124 | 0.366 b | 0.266 b | 0.274 b | 0.817 a | |||
PR | 0.870 | 0.628 | 0.175 | 0.112 | 0.212 b | 0.377 b | 0.274 b | 0.373 b | 0.792 a | ||
BI | 0.876 | 0.702 | 0.204 | 0.169 | 0.452 b | 0.364 b | 0.386 b | 0.411 b | 0.418 b | 0.838 a | |
QJ | 0.871 | 0.772 | 0.187 | 0.114 | 0.275 b | 0.236 b | 0.334 b | 0.394 b | 0.310 b | 0.433 b | 0.879 a |
Note: a ; b Inter-construct correlations. |
Independent Variables | t | p | VIF | |
Dependent Variable: QJ | ||||
Intercept | 0.717*** | 5.849 | 0.000 | |
PBC | 0.260*** | 5.620 | 0.000 | 1.203 |
BI | 0.326*** | 7.041 | 0.000 | 1.203 |
Dependent Variable: BI | ||||
Intercept | –0.627*** | -3.879 | 0.000 | |
AT | 0.282*** | 6.819 | 0.000 | 1.197 |
SN | 0.163*** | 3.965 | 0.000 | 1.185 |
PBC | 0.139** | 3.178 | 0.002 | 1.332 |
MN | 0.182*** | 4.455 | 0.000 | 1.171 |
PR | 0.202*** | 4.722 | 0.000 | 1.282 |
Note: β (Standardized regression coefficients); VIF (Variance inflation factor). ** p0.01; *** p0.001. |
Hypotheses | Paths | β | 95% Confidence Intervals | p | Results | |
Lower | Upper | |||||
H1 | QJBI | 0.391** | 0.265 | 0.508 | 0.002 | Supported |
H2 | QJPBC | 0.282** | 0.146 | 0.418 | 0.002 | Supported |
H3 | BIAT | 0.316** | 0.202 | 0.403 | 0.005 | Supported |
H4 | BISN | 0.175** | 0.060 | 0.282 | 0.002 | Supported |
H5 | BIPBC | 0.122* | 0.031 | 0.252 | 0.05 | Supported |
H6 | BIMN | 0.184** | 0.078 | 0.284 | 0.002 | Supported |
H7 | BIPR | 0.230*** | 0.128 | 0.349 | 0.001 | Supported |
H8 | QJPBC (Total) | 0.330** | 0.188 | 0.458 | 0.002 | Supported |
QJBIPBC (Indirect) | 0.048* | 0.001 | 0.102 | 0.037 | ||
QJPBC (Direct) | 0.282** | 0.146 | 0.418 | 0.002 | ||
Note: * p0.05; ** p0.01; *** p0.001. |
No. | Gender | Age | Education | Driving experience (years) | Driving frequency (hours per week) | Queue-jumping behavior |
1 | Male | 28 | 3 | 6 | 3 | 3 |
2 | Male | 26 | 2 | 3 | 4 | 5 |
3 | Female | 34 | 4 | 5 | 2 | 1 |
4 | Male | 55 | 1 | 12 | 5 | 4 |
5 | Male | 24 | 2 | 4 | 3 | 4 |
6 | Female | 27 | 3 | 2 | 1 | 1 |
7 | Male | 46 | 2 | 8 | 2 | 2 |
8 | Female | 57 | 4 | 13 | 3 | 2 |
9 | Male | 38 | 2 | 7 | 3 | 2 |
10 | Female | 22 | 2 | 1 | 1 | 1 |
11 | Female | 41 | 3 | 2 | 2 | 1 |
12 | Male | 62 | 1 | 15 | 2 | 3 |
13 | Male | 27 | 3 | 3 | 2 | 2 |
14 | Male | 36 | 3 | 6 | 3 | 3 |
15 | Female | 24 | 3 | 2 | 1 | 1 |
Note: Education (1 = "Below high school", 2 = "High school", 3= "Undergraduate", 4 = "Above undergraduate"); Driving frequency (1 = " < 4", 2 = "4-6", 3 = "7-10", 4 = "11-15", 5 = " > 15"/hours per week); Past queue-jumping behavior experience in the last 6 months (1 = "Never", 2 = "Occasionally", 3 = "Sometimes", 4 = "Often", 5 = "Very often"). |