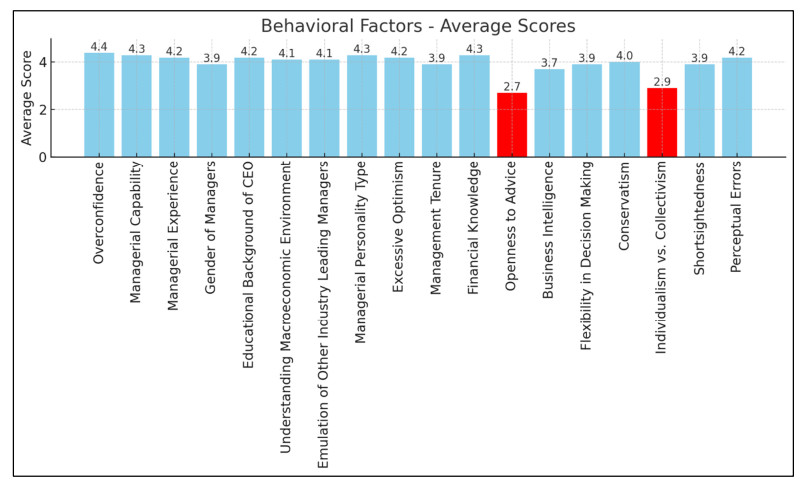
Our objective of this research was to identify and rank the factors that influence the capital structure decisions of Iranian companies, focusing on the role of behavioral elements as intermediaries. To construct the research framework, we employed a two-phase, mixed-method design that encompassed both qualitative insights and quantitative methodologies. In the qualitative phase, experts in the capital market are consulted using a snowball sampling technique, and key research factors were identified through coding analysis. Subsequently, for the quantitative phase, managers from leading Tehran Stock Exchange–listed companies were surveyed to prioritize the model components via the Analytic Hierarchy Process (AHP). Moreover, Factor Analysis with expert feedback using structural equation modeling (SEM) were performed in the model. Initially, we identified 63 concepts, later refined to 58 after expert consultation, and categorized them into behavioral, macroeconomic, political, socio-cultural, corporate characteristics, and governance factors. Quantitative analysis revealed that macroeconomic factors exhibit the highest influence (0.266), followed by behavioral factors (0.230), corporate governance (0.201), company-specific characteristics (0.141), political factors (0.103), and socio-cultural factors (0.059) in shaping financial structures. This research thus offers a more detailed examination of how behavioral biases and broader market conditions are converged to influence capital structure, underscoring the importance of integrating cognitive perspectives—particularly those of financial managers—into corporate decision-making processes. Future investigations can be benefited from integrating broader market data, such as share price movements, to further refine insights into the dynamic interplay of behavior and capital structure.
Citation: Ehsan Ahmadi, Parastoo Mohammadi, Farimah Mokhatab Rafei, Shib Sankar Sana. Behavioral factors and capital structure: identification and prioritization of influential factors[J]. Data Science in Finance and Economics, 2025, 5(1): 76-104. doi: 10.3934/DSFE.2025005
[1] | Ji Young Choi, Chae Young Lee, Man-Suk Oh . Discovering causal relationships among financial variables associated with firm value using a dynamic Bayesian network. Data Science in Finance and Economics, 2025, 5(1): 1-18. doi: 10.3934/DSFE.2025001 |
[2] | Xinying Zhang, Chuanjun Zhao, Xianwei Zhou, Xiaojun Wu, Ying Li, Meiling Wu . Capital market and public health emergencies in Chinese sports industry based on a market model. Data Science in Finance and Economics, 2023, 3(2): 112-132. doi: 10.3934/DSFE.2023007 |
[3] | Tsumbedzo Mashamba, Modisane Seitshiro, Isaac Takaidza . A comprehensive high pure momentum equity timing framework using the Kalman filter and ARIMA forecasting. Data Science in Finance and Economics, 2024, 4(4): 548-569. doi: 10.3934/DSFE.2024023 |
[4] | Changjun Zheng, Md Mohiuddin Chowdhury, Anupam Das Gupta . Investigating the influence of ownership on the relationship between bank capital and the cost of financial intermediation. Data Science in Finance and Economics, 2024, 4(3): 388-421. doi: 10.3934/DSFE.2024017 |
[5] | Yen H. Hoang, Duong T.T. Nguyen, Linh H.T. Tran, Nhung T.H. Nguyen, Ngoc B. Vu . Customers' adoption of financial services offered by banks and fintechs partnerships: evidence of a transitional economy. Data Science in Finance and Economics, 2021, 1(1): 77-95. doi: 10.3934/DSFE.2021005 |
[6] | Yong-xin Lin, Xue Chen, Heng-you Lan . Analysis and prediction of American economy under different government policy based on stepwise regression and support vector machine modelling. Data Science in Finance and Economics, 2023, 3(1): 1-13. doi: 10.3934/DSFE.2023001 |
[7] | Changjun Zheng, Md Abdul Mannan Khan, Mohammad Morshedur Rahman, Shahed Bin Sadeque, Rabiul Islam . The impact of monetary policy on banks' risk-taking behavior in an emerging economy: The role of Basel II. Data Science in Finance and Economics, 2023, 3(4): 427-451. doi: 10.3934/DSFE.2023024 |
[8] | Michael Jacobs Jr. . Validation of corporate probability of default models considering alternative use cases and the quantification of model risk. Data Science in Finance and Economics, 2022, 2(1): 17-53. doi: 10.3934/DSFE.2022002 |
[9] | Nitesha Dwarika . Asset pricing models in South Africa: A comparative of regression analysis and the Bayesian approach. Data Science in Finance and Economics, 2023, 3(1): 55-75. doi: 10.3934/DSFE.2023004 |
[10] | Yueh-Hsia Huang, Lan Sun, Tyng-Bin Ger . An analysis of enterprise resource planning systems and key determinants using the Delphi method and an analytic hierarchy process. Data Science in Finance and Economics, 2023, 3(2): 166-183. doi: 10.3934/DSFE.2023010 |
Our objective of this research was to identify and rank the factors that influence the capital structure decisions of Iranian companies, focusing on the role of behavioral elements as intermediaries. To construct the research framework, we employed a two-phase, mixed-method design that encompassed both qualitative insights and quantitative methodologies. In the qualitative phase, experts in the capital market are consulted using a snowball sampling technique, and key research factors were identified through coding analysis. Subsequently, for the quantitative phase, managers from leading Tehran Stock Exchange–listed companies were surveyed to prioritize the model components via the Analytic Hierarchy Process (AHP). Moreover, Factor Analysis with expert feedback using structural equation modeling (SEM) were performed in the model. Initially, we identified 63 concepts, later refined to 58 after expert consultation, and categorized them into behavioral, macroeconomic, political, socio-cultural, corporate characteristics, and governance factors. Quantitative analysis revealed that macroeconomic factors exhibit the highest influence (0.266), followed by behavioral factors (0.230), corporate governance (0.201), company-specific characteristics (0.141), political factors (0.103), and socio-cultural factors (0.059) in shaping financial structures. This research thus offers a more detailed examination of how behavioral biases and broader market conditions are converged to influence capital structure, underscoring the importance of integrating cognitive perspectives—particularly those of financial managers—into corporate decision-making processes. Future investigations can be benefited from integrating broader market data, such as share price movements, to further refine insights into the dynamic interplay of behavior and capital structure.
Numerous considerations come into play to determine a company's capital structure. Though corporate governance research has advanced strategies, there is much to learn about how managerial biases inform financing choices. There are traditional models at the firm, industry, and market levels like the trade-off theory (Miller, 1977), the hierarchy theory (Myers, 1984), and agency theory (Jensen and Meckling, 2019) equipped with center on external factors like taxes, market signals, and information asymmetry. These frameworks frequently assume that decisions stem from rational, utility-maximizing behavior, leaving less room for the subjective viewpoints of individual managers. Early empirical works by Bradley et al. (1984), Titman et al. (1988), and Smith et al. (1992) address certain capital structure patterns, but a portion of observed variation remains difficult to explain purely through rationalist lenses. Such gaps prompt researchers to look more closely at the possibility that internal dispositions including executive risk preferences may drive firms' funding strategies.
More recent empirical studies provide credibility to the role of managerial characteristics. In particular, Mundi and Kaur (2022) found that CEO overconfidence can significantly shape leverage decisions in emerging markets, a phenomenon echoed by Jansen et al. (2023), who document family governance structures leading to financing behaviors that is deviated from standard capital structure theory. This evidence underscores a broader shift toward recognizing the influence of behavioral finance in corporate contexts. Graham et al. (2013) pioneer the idea that heterogeneity among managers can alter financial outcomes, and later research by Zou and Bai (2022) and Wang and Zhou (2025) refine our understanding by detailing how specific corporate policies—like dividend issuance or working capital management—are filtered through managerial perceptions. Rather than presuming a one-size-fits-all bias framework, newer investigations are focused on "distinct mechanisms" by which behaviors such as overconfidence, present bias, and risk aversion directly shaped firm-level decisions (Costa et al., 2017). For instance, managers prone to overestimating their own competencies may choose debt financing more aggressively, believing they can handle any resultant risks, while highly risk-averse executives could limit leverage to avoid the possibility of distress. These decisions can translate into differences in capital costs and even short-term equity of pricing, as illustrated by Mapela and Chipeta (2023), who investigate that share price volatility can spike following certain capital structure announcements driven by strong managerial confidence.
Although substantial advances have been made in corporate governance, many aspects remain obscure. Board composition, ownership concentration, and the interplay of managerial personality with governance practices lack definitive explanations as to how they shape financing outcomes (Alves et al., 2015; Orlova et al., 2020). In some cases, management boards may mitigate excessive optimism through oversight, whereas in others, weak governance might enable managerial biases to flourish unchecked. Al-Zoubi et al. (2022) highlight how sentiment-driven corporate leaders may engage in counter cyclical market timing, amplifying volatility in less efficient markets. Collectively, these findings suggest that bridging behavioral finance with governance studies may illuminate how macro- and micro-level forces converge in capital structure determination. Within Iran's predominantly state-owned economy examining both public and private forms of ownership on company boards, it is observed and signified how these diverse governance structures influence financing strategies represents a particularly valuable research avenue. This significance arises for three major reasons. First, Iran's economy blends governmental oversight with segments of private enterprise, producing unique board compositions that differ from those in fully privatized or heavily regulated Western contexts. Second, persistent international sanctions, combined with periods of economic volatility, create conditions in which managers might rely more on heuristics or personal judgments—particularly when conventional financing channels are constrained. Third, Islamic economic principles, which shape certain debt and equity instruments, add further layers to how managers perceive and implement financing decisions. Thus, understanding how ownership configurations and these broader institutional factors interaction could reveal the unique ways in which behavioral dispositions intersect with financing outcomes. Iran's recent economic trajectory amplifies these concerns. Following the 2014 oil price downturn, the Gulf region faced a prolonged phase of public expenditure adjustments, along with heightened foreign borrowing and financial constraints. This climate introduced new complexities around liquidity and access to capital, forcing Iranian firms to navigate rapidly changing regulations and investor sentiments. In parallel, external sanctions persisted, limiting certain international transactions and intensifying uncertainty in financial planning. Under these circumstances, managerial judgments about risk, growth opportunities, and even compliance with Shariah law become pivotal. The ongoing need to balance operational realities with regulatory limitations underscores the importance of studying how biases—be they overconfidence, pessimism, or inertia—may influence the balance of debt and equity financing (Yang et al., 2021). Although many past investigations have emphasized investor-driven biases, comparatively fewer studies have spotlighted how executive-level perceptions and emotional states can steer corporate financial policy—particularly in a market shaped by partial state ownership, cross-border restrictions, and cultural-specific legal norms. Against this backdrop, we aim to determine and evaluate the behavioral factors that shape the capital structure of Iranian companies. In practical terms, these factors will be identified through a two-phase mixed-method design that combines in-depth expert interviews with quantitative assessments, including factor analysis and hierarchy-based prioritizations. By gathering qualitative insights from Iranian capital market professionals and triangulating them with firm-level data, we can isolate which biases—overconfidence, risk aversion, confirmation bias, or other behavioral traits—are most influential in the local context. We then measure their relative impact on financing decisions by using structural equation modeling (SEM), thereby linking psychological (Badheka & Pandya, 2022) predispositions to observed leverage and equity issuance patterns. This methodological approach offers a clearer window into how managerial characteristics operate alongside macro-level conditions, bridging the gap between broad theoretical constructs and specific corporate outcomes.
Moreover, a focus on Iran answers a need within the literature to investigate emerging economies wherein governance structures, political factors, and socio-religious norms all coalesce to shape corporate finance (Pérez et al., 2019; Li et al., 2021; Liu et al., 2022; Sajid et al., 2024). Studies on behavioral finance in Western settings have advanced the notion that managerial biases significantly affect debt and equity choices (Heaton, 2002; Ting et al., 2016). Yet, few have examined how these biases function in contexts that feature partial state oversight, Islamic financing rules, and prolonged sanctions. By reviewing behavioral dimensions of corporate finance in Iran—where macroeconomic imbalances and sociopolitical uncertainties set a distinct stage—this research aims to extend the applicability of existing theories and uncover any region-specific nuances. It also contributes to policy-making discussions, as regulators seeking to attract foreign investors or stabilize domestic markets might consider how managerial psychology mediates the implementation of broader governance reforms. By integrating an assessment of managerial biases with the unique economic and governance environment in Iran, this study enriches our overall understanding of capital structure. In many rational-choice frameworks, the selection between debt and equity is framed as a straightforward cost-benefit calculation, but contemporary evidence shows that executive perceptions, cultural norms, and institutional strictures play equally decisive roles. Recognizing these factors can reveal why firms deviate from theoretical optimal levels of leverage, how they respond to sudden policy shifts, or why certain sectors might exhibit persistent overconfidence in the face of global headwinds. In highlighting these aspects, we hope to offer a more nuanced perspective on corporate financing, illuminating the complexities of human behavior and institutional design in shaping capital structure decisions.
Scholars and management practitioners have long agreed that capital structure decisions significantly shape a firm's risk exposure, financing costs, and adaptive capacity. However, even as new perspectives emerge, classical theories remain fundamental. The trade-off theory (Miller, 1977) posits a balance between debt's tax benefits and potential bankruptcy woes, and the pecking order theory (Myers & Majluf, 1984) suggests that internal funds typically precede debt, with equity issuance as a last resort. After all, these early frameworks anchor decades of empirical investigations that, despite evolving critiques, continue to guide how we interpret firm-level financing behavior.
Modigliani and Miller (1958) argue capital structure may be irrelevant under perfect conditions, only to revise their stance when factoring in corporate tax considerations (Modigliani & Miller, 1963). This shift spurs much of the subsequent scholarship around leverage. Empirical tests across nations show that managers do often weigh tax advantages against the possibility of financial distress, affirming that the trade-off concept remains more than a historical curiosity (Fama et al., 1998). In parallel, market timing notions (Baker & Wurgler, 2002) resonate. Case in point: Managers in real-world contexts occasionally seize on high valuations to issue shares or buy them back if they deem their company undervalued. Put differently, even with behavioral finance challenging the assumption of perfect rationality, many features of these seminal theories remain instructive baselines for evaluating financing strategies.
One recurring critique is that studies are overwhelmingly centered on established markets, while more unpredictable settings have been comparatively overlooked (Oprean, 2014). It is striking, for instance, how a significant portion of classic research relies on data from North America or Western Europe, even though substantial economic growth occurs in Asia, Africa, and Latin America. In politically uncertain regions, managers may adopt conservative policies, perhaps retaining extra liquidity or shunning debt altogether. Alternatively, sanctions can disrupt foreign capital flows, forcing companies to revise leverage downward (Jiang et al., 2021). Likewise, cultural norms—like a deeply ingrained aversion to risk—may lead executives to limit borrowing for fear of jeopardizing stakeholder relationships (Mapela & Chipeta, 2023). By contrast, in mature economies, robust capital markets and consistent legal frameworks engender more uniform financing patterns.
In Iran, state-owned enterprises coexist with private entities and are subject to an evolving sanctions regime that complicates foreign investment. Compounding this, Iranian finance is partly guided by Shariah principles, meaning interest-based loans aren't always the default option. Such conditions differ from other Islamic markets, like Malaysia, which typically maintains broader access to global capital. The upshot is that Iranian managers may rely on short-term internal funds or specialized Islamic contracts when credit channels are narrow (Chauhan et al., 2022). Sporadic changes to sanctions can spark abrupt shifts in capital availability, prompting sudden decisions on whether to issue equity or hold back. Sometimes intangible factors, such as trust or nepotism, carry extra weight in bridging the gaps where formal institutions underperform (Xia et al., 2021).
Now, the following question arises: How do conventional capital structure models adapt, if at all, to such a constrained environment? Classic assumptions about stable market conditions are clearly falter, but not every aspect becomes irrelevant. For instance, some Iranian companies do still weigh tax shields and potential default costs, though the interplay is layered at religious restrictions and political turbulence. In essence, evaluating Iranian firms offers a unique lens to see how deeply context can reshape classical theory.
➢ Behavioral finance and key biases:
Behavioral finance began as a critique of neoclassical ideals, revealing that real people (and managers) often rely on heuristics in ways that systematically tilt decisions (Thaler, 1999). They can succumb to biases like overconfidence—where a CEO genuinely believes in superior forecasting abilities—or anchoring, where recent experiences overshadow broader, objective data. Costa et al. (2017) suggest that these tendencies can alter capital allocation, for instance, by spurring risk-taking in expansions or fueling conservative stances during downturns. Even the notion of efficient markets (Fama, 1970) is regularly challenged by evidence of herding and mispricing, driven by emotion-laden trades (Frankfurter et al., 2002). Such patterns matter because managerial biases can thwart classical predictions. Mundi and Kaur (2022) observe that CEO overconfidence, especially in emerging settings, can fuel high debt levels. Conversely, risk-averse executives may under-leverage their firms, foregoing profitable opportunities in times of uncertainty. Another subtlety arises with the availability heuristic, where managers default to easily recall the events—like a recent industry fiasco—over a comprehensive dataset (Arianpoor & Yazdanpanah, 2022). This reliance on mental short-cuts is often magnified in volatile markets where consistent information is tough to obtain.
➢ Interplay with share price movements and sanctions:
In sanctioned or politically sensitive contexts, stock price swings can be larger than usual. For example, a modest uptick in domestic equity values may embolden executives to issue shares, if they interpret the rally as a sign of investor optimism (Muhammed, 2022). However, a sudden slump, triggered by renewed geopolitical stress, can push managers to revisit internal funding or short-term borrowing to avoid an unfavorable equity dilution. As a result, share price volatility reflects not only genuine fundamentals but also managerial reactions to external signals. Sun et al. (2021) highlight how in Zimbabwe's sanctioned environment, certain intra-industry shifts in stock prices can pressure competing firms to modify capital structures as well. The Iranian scenario echoes these themes: Market sentiment can swing swiftly on rumors of diplomatic openings or conversely, the announcement of fresh sanctions.
➢ Time frames and regional focus:
Examining capital structure in emerging markets often necessitates a thoughtful selection of time frames (Aibar-Guzmán et al., 2022). For instance, the 2014 oil price collapse or the COVID-19 crisis introduces distinct macro shocks that reshape everything from currency valuations to consumer demand (Bokpin, 2010; Salam & Shourkashti, 2019). Iranian firms, in particular, find themselves squeezed by declining oil revenues and intensifying inflationary pressures, prompting them to rely more on internal networks or off-balance-sheet arrangements. Another round of sanctions may reduce access to external loans, thus intensifying reliance on local resources. Admittedly, not all managers react uniformly—some may push for debt-financed growth if they foresee a short-lived slump—but overall, these crisis intervals shed light on how psychological and structural factors meld in real-world decisions (Shahmi, 2023).
➢ Behavioral finance in Iran and comparable Islamic markets:
Finally, many Iranian firms operate under Shariah-compliant principles, restricting interest-based debt and promoting alternatives like Sukuk or profit-and-loss sharing. In secular frameworks, managers may simply ramp up traditional bonds whenever equity valuations sink. Moreover, path can conflict with religious mandates (Guizani & Ajmi, 2021). Faysal et al. (2020) note that cultural collectivism and partial state oversight add an extra layer of governance complexity by shaping managerial inclinations and investor sentiment. By the same token, other Islamic economies may share aspects of this dynamic—Malaysia's robust Sukuk market, for example—but differ in sanction exposure or in how open they are to foreign investors (Ahmed, 2024). Iran's relative isolation, therefore, heightens the importance of local trust networks, occasionally giving rise to herding-like behaviors (Ah Mand et al., 2021; Li et al., 2021; Liu et al., 2022; Lapinski et al., 2024) that defy typical finance models.
In short, foundational theories—trade-off, pecking order, and market timing—serve as core references for dissecting corporate leverage. Yet, the behavioral lens demonstrates that managers, especially in emerging or volatile contexts, do not always comply with strictly rational norms. Iran's hybrid setting of partial state control, sanctions, and Islamic finance accentuates how macro constraints intertwine with biases like overconfidence or anchoring, leading firms to craft idiosyncratic capital structures that can appear puzzling when judged solely by classic frameworks. Recognizing these overlaps underscores the need for a well-rounded analysis that merges conventional models with a deeper awareness of psychological and institutional influences.
We employed a sequential mixed-methods approach to investigate how behavioral and corporate governance variables interact with macro-level factors in shaping capital structure decisions. By combining exploratory qualitative work with confirmatory quantitative analysis, this research aims to capture the nuanced perspectives of industry specialists while ensuring generalized findings among corporate managers. Though other approaches—such as multiple regressions—were considered initially, we chose this design because it allowed us to glean in-depth insights from expert interviews before quantifying and validating key themes.
The first phase involved grounded theory coding based on semi-structured interviews with 28 experts: CEOs of large Iranian companies, faculty members from Tehran universities, and financial consultants familiar with the constraints of the Iranian market. The work uses purposive sampling, selecting individuals with proven expertise in capital structure decisions or a deep understanding of corporate governance practices (Çam & Özer, 2022; Carrizosa et al., 2020, 2023; Choi et al., 2020; Danso et al., 2021; Chua et al., 2022). Interviews proceed until no substantially new insights emerged (data saturation). During each interview, participants are asked open-ended questions about the interplay of behavioral biases, governance structures, and macroeconomic uncertainties. Additional probing questions arise spontaneously, depending on the flow of conversation. This flexibility helps us uncover hidden complexities—like managers' personal risk aversion or how state ownership intersects with Shariah-compliant financing (Rashid et al. 2023).
After transcription, interview data are coded in multiple cycles. First, open codes capture statements about critical factors and potential constructs (e.g., "overconfidence," "board composition," "political risk"). Axial coding then grouped overlapping codes into broader categories eventually forms an initial conceptual model of behavioral, governance, and macroeconomic influences. Throughout the process, peer debriefing is conducted to ensure coding consistency and to reduce subjective bias in interpreting participants' remarks.
Building on the categories identified in the qualitative phase, a quantitative examination is studied for broader validation. The proposed sample encompasses senior managers from Tehran Stock Exchange–listed firms. While the original plan is to gather purely random responses, an initial purposive screen is employed to identify companies likely to reflect the full range of governance structures (state-owned, semi-private, and private). From there, a simple random method ensures that each eligible manager has an equal chance of being included. Out of the 220 individuals contacted, Morgan's table is determined based on the population size—160, obtained from complete usable survey responses.
To address more details on variables, three major groups as classified:
1. Behavioral Factors: Overconfidence, risk aversion, short-term bias, and other individual-level predispositions are identified in the interviews.
2. Corporate Governance/Board Structure: Ownership concentration, board composition, and managerial discretion are highlighted by experts as shaping financing decisions.
3. Macroeconomic and Political Variables: Inflation volatility, sanctions, and regulatory changes are described as unique constraints in Iran.
We acknowledge that a purely market-based measure—such as share price fluctuations—can offer additional insights, as one reviewer suggests. However, due to the irregular availability of consistent daily price data across all target firms, fundamental and survey-based measures are prioritized. Future research can incorporate a "pure market data point" if these records become reliably accessible.
Before running any structural modeling, AHP is used to prioritize the categories gleaned from grounded theory. Participants perform pair-wise comparisons among rank-ordering behavioral, governance, and macroeconomic factors to obtain relative weights. This step creates a clear hierarchy of which elements managers perceive as most influential. Such prioritization is implemented via Expert Choice 11, taking advantage of its user-friendly interface and straightforward calculations. After verifying consistency ratios (all < 0.1), these weights are used to inform the next stage.
The conceptual model is tested incorporating the weighted factors using Partial Least Squares Structural Equation Modeling (PLS-SEM) via Smart-PLS software. The PLS-SEM is preferred over covariance-based SEM because the former is robust in the face of smaller sample sizes and highly interrelated constructs, both of which are anticipated in an exploratory corporate finance study. The technique enables to test how well each latent construct (behavioral bias, governance structure, macroeconomic pressure) explains variance in capital structure decisions. The reliability and validity of each measurement are also examined evaluating factor loadings, composite reliability, and average variance extracted.
One reviewer suggests that an additional dimension may be missing, hinting at a more refined approach to capturing the financial implications of Bf & CS. We recognize that integrating daily share price data or advanced market-driven indicators could further enrich our model. Nonetheless, given data availability constraints and the primary focus on expert-driven constructs, we rely on the subjective measures. Researchers are encouraged to build on these findings by incorporating real-time market indices or high-frequency trading data, which could illuminate short-term reactions to managerial biases.
Finally, to enhance the rigor of our mixed-methods design, we cross-checked preliminary quantitative findings back with a subset of interviewees, seeking their feedback on whether the results aligned with practice. This step, though informal, further bolster the credibility of our inferences.
This research is unfolded in two primary stages, beginning with a qualitative phase that pinpoints major capital structure components and shapes the preliminary model, drawing on insights from CEOs, board members, and academic specialists. Following that, a quantitative phase prioritizes these components via systematic feedback from managers of publicly traded firms. By proceeding in this sequence, we ensure that our findings will link back to the study's research questions—namely, identifying which factors (behavioral, macroeconomic, and so on) carry the greatest influence on corporate financing decisions and how they may interact within Iran's distinctive environment.
In this qualitative phase, we leverage in-depth interviews with carefully selected experts—CEOs, top managers, and faculty—to develop an initial conceptual model. Our coding process produces six core categories that may shape capital structure decisions: behavioral factors (Sunitha, 2024), macroeconomic factors, socio-cultural factors, political factors, company characteristics, and corporate governance. Within behavioral factors, respondents highlight overconfidence, managerial experience, and managerial personality traits (e.g., optimism, conservatism) as particularly relevant. Moreover, certain items—such as openness to advice or flexibility in decision-making—emerge but do not appear as dominant.
Turning to macroeconomic factors, the experts consistently emphasize that fluctuations in interest rates, inflation, tax policies, and market cycles can heavily constrain or expand corporate financing choices. Moreover, socio-cultural dynamics—like Sharia compliance, organizational culture (Mogha & Williams, 2021), or social trust—also surfaced, showing how deeply ingrain norms can dictate leverage strategies. In the political realm, governance quality and corruption levels are singled out, although political stability receives a less decisive endorsement, illustrating experts' mixed views on how directly it shapes capital structure (Singh & Kannadhasan, 2020; Xing 2020). Company characteristics (profitability, R&D, liquidity, etc.) and corporate governance (board composition, CEO duality) likewise are emerged as key considerations. This foundational mapping responds to the study's interest in locating the most influential factors—a pivotal first step before we rank and test them quantitatively.
Following the identification of six broad categories, these factors are presented to specialists for further assessment. Using a five-point scale (where 1 meant strong disagreement and 5 meant strong agreement), any factor scoring below 3 is removed. Consequently, items like Openness to Advice, Individualism vs. Collectivism, Political Stability, Research and Development Costs, and Profit Distribution do not remain in the final model. As a result, we retain a total of 58 sub-components spanning the six principal domains. This screening step aligns with our aim of ensuring that only widely supported items are progressed to the quantitative phase.
As illustrated in Figure 1, a range of behavioral factors is emerged as salient for corporate capital structure decisions. Items like Overconfidence, Managerial Capability, and Financial Knowledge stand out for exceeding a mean score of 4, signaling broad expert agreement on their importance. By contrast, Openness to Advice and Individualism vs. Collectivism fell below 3, suggests that few participants view these behaviors as strongly shaping leverage or financing strategies. Turning to macroeconomic factors in Figure 2, elements such as Economic Sanctions, Interest Rates, and Tax Rates all scored above 4, indicate a near-unanimous view that macro-level conditions substantially condition corporate financing. Notably, no macroeconomic variables are discarded, underscoring a consistent emphasis on external economic constraints in shaping capital structure.
The socio-cultural factors, presented in Figure 3, achieve mean scores between roughly 3.6 and 4.3, with Sharia compliance often highlighted as a crucial lens for financial decision-making in Iran's environment.
Political factors—including administrative corruption and non-economic sanctions—score above 4 (Figure 4), revealing that institutional and legal frameworks can heavily influence financing. By contrast, political stability achieves only 2.8, indicating that experts perceive it as less pivotal. Moreover, elements such as the rule of law and governance quality also surpasses 3, reflecting moderate to high consensus about their role in shaping capital structure decisions. These findings reinforce the notion that external constraints—like corruption and sanctions—can overshadow purely managerial considerations in emerging markets.
Figure 5 highlights the diverse impact of company attributes on capital structure. Tangible assets, the price-to-book ratio and financial leverage score above 4, reflecting strong agreement that firm-specific characteristics can drive financing decisions. However, profit distribution and R&D costs fall below the 3-point threshold, suggesting limited expert support for these variables. This disparity implies that while certain operational factors hold significant sway, others attract less consensus—an insight that connects directly to the study's aim of identifying the strongest predictors of capital structure.
All corporate governance components are shown in Figure 6—such as board composition, CEO power, and institutional ownership—score between 3.8 and 4.3, underlining how governance arrangements can shape financing strategies. This strong endorsement aligns with the broader literature that positions governance as a critical determinant of firms' risk tolerance and leverage choices, echoing our research questions about how organizational structures interact with behavioral and macro-level variables.
In this phase, the Analytic Hierarchy Process (AHP) is applied to prioritize both main and sub-components. By constructing pair-wise comparison matrices, the experts' judgments of relative importance are systematically converted into numerical weights. Specifically, each criterion's weight (wi) is derived using the following formula:
wi=∑nj=1aijn |
Here, wi denotes the weight of criterion i, aij is the comparison value between criterion i and criterion j, and n is the total number of criteria. This method enables us to clarify how the factors identified earlier might influence corporate capital structure in practice.
As shown in Table 1, Macroeconomic Factors secure the highest overall weight (0.266), with Behavioral Factors following at 0.230 and Corporate Governance at 0.201. Company Characteristics come in fourth (0.141), Political Factors fifth (0.103), and Socio-Cultural Factors last (0.059). Notably, the calculated inconsistency ratio is 0.03—well under the 0.1 benchmark—confirming that the pair-wise comparisons are logically consistent. By highlighting Macroeconomic and Behavioral domains near the top, these results reinforce the idea that both external conditions and managerial traits can significantly shape financing strategies.
Rank | Criterion | Score |
1 | Macroeconomic Factors | 0.266 |
2 | Behavioral Factors | 0.230 |
3 | Corporate Governance | 0.201 |
4 | Company Characteristics | 0.141 |
5 | Political Factors | 0.103 |
6 | Socio-Cultural Factors | 0.059 |
Incompatibility Rate=0.03 |
As detailed in Table 2, Managerial Personality Type emerges as the most influential behavioral factor (0.132), with Financial Knowledge (0.107), Overconfidence (0.089), and Business Intelligence (0.081) also scoring highly. Moreover, traits like Conservatism (0.071) and Flexibility in Decision-Making (0.067) have moderate impact, whereas Managerial Experience and CEO Educational Background garner lower scores. Nevertheless, the overall inconsistency ratio for this set of comparisons is 0.06—comfortably under 0.1—reinforcing the reliability of these rankings.
Rank | Criterion | Score |
1 | Managerial Personality Type | 0.132 |
2 | Financial Knowledge | 0.107 |
3 | Overconfidence | 0.089 |
4 | Business Intelligence | 0.081 |
5 | Conservatism | 0.071 |
6 | Flexibility in Decision Making | 0.067 |
7 | Shortsightedness | 0.064 |
8 | Excessive Optimism | 0.061 |
9 | Managerial Capability | 0.059 |
10 | Management Tenure | 0.051 |
11 | Perceptual Errors | 0.045 |
12 | Managerial Experience | 0.043 |
13 | Understanding Macroeconomic Environment | 0.038 |
14 | Educational Background of CEO | 0.036 |
15 | Gender of Managers | 0.034 |
16 | Emulation of Other Industry Leading Managers | 0.022 |
Incompatibility Rate=0.06 |
Turning to macroeconomic factors (Table 3), Information Rent is topped the list at 0.201, with Economic Sanctions (0.172) and Exchange Rate Changes (0.151) following closely. This prioritization underscores how limited information flow, external sanctions, and currency fluctuations can strongly influence capital structure decisions. By contrast, variables like Uncertainty in Monetary and Fiscal Policies and Business Cycles ranked lower, yet still play a background role. The calculated inconsistency ratio of 0.03 once again confirms a coherent set of pair-wise judgments.
Rank | Criterion | Score |
1 | Information Rent | 0.201 |
2 | Economic Sanctions | 0.172 |
3 | Exchange Rate Changes | 0.151 |
4 | Tax Rate | 0.125 |
5 | Interest Rate | 0.107 |
6 | Inflation Rate Changes | 0.083 |
7 | Economic Growth | 0.071 |
8 | Uncertainty in Monetary and Fiscal Policies | 0.049 |
9 | Business Cycles | 0.041 |
Incompatibility Rate=0.03 |
Within the socio-cultural domain (Table 4), Alignment of Financial Conditions with Sharia is registered with the highest score (0.363), followed by Religious Orientation (0.320). Organizational Culture (0.179) and Social Trust (0.138) place third and fourth, respectively. Although the latter two factors score lower, the overall inconsistency ratio stands at 0.04—still under the 0.1 cutoff. These findings highlight how religious and cultural norms can shape financing strategies, but with some experts assigning greater weight to specific doctrinal or faith-based considerations.
Rank | Criterion | Score |
1 | Alignment of Financial Conditions with Sharia | 0.363 |
2 | Religious Orientation | 0.320 |
3 | Organizational Culture | 0.179 |
4 | Social Trust | 0.138 |
Incompatibility Rate=0.04 |
Turning to political factors (Table 5), Governance Quality registers the top score (0.338), followed by the Rule of Law (0.219) and Administrative Corruption (0.153). These results imply that well-established legal and governance frameworks can significantly influence managerial confidence in financing. By contrast, Enactment of New Laws ranks lowest, indicating that experts view ongoing legal changes as less pivotal than the overall quality of governance. The inconsistency ratio of 0.04 again affirms stable pair-wise comparisons.
Rank | Criterion | Score |
1 | Governance Quality | 0.338 |
2 | Rule of Law | 0.219 |
3 | Administrative Corruption | 0.153 |
4 | Presidential Elections | 0.121 |
5 | Non-Economic Sanctions | 0.091 |
6 | Enactment of New Laws | 0.077 |
Incompatibility Rate=0.04 |
As seen in Table 6, Tangible Assets ranks highest (0.118) among company characteristics, trailed by Operational Risk (0.112) and Financial Leverage (0.107). Current Liabilities appear next (0.104), while Human Capital fall to the bottom, hinting that experts accord less weight to workforce composition in deciding capital structure. With an inconsistency ratio of 0.05, this subset also meets the standard threshold (<0.1). Overall, these company-level results suggest that firms' tangible resources and risk profiles outshine purely human or intangible elements in influencing financing.
Rank | Criterion | Score |
1 | Tangible Assets | 0.118 |
2 | Operational Risk | 0.112 |
3 | Financial Leverage | 0.107 |
4 | Current Liabilities | 0.104 |
5 | Asset Returns | 0.088 |
6 | Systematic Risk | 0.075 |
7 | Liquidity | 0.066 |
8 | Company Size | 0.062 |
9 | Audit Quality | 0.055 |
10 | Company Age | 0.051 |
11 | Sales Growth | 0.046 |
12 | Price-to-Book value | 0.042 |
13 | Non-Debt Tax Shield | 0.038 |
14 | Human Capital | 0.034 |
Incompatibility Rate=0.05 |
Within corporate governance (Table 7), CEO Power dominates at 0.191, with CEO Duality (0.164) and Institutional Ownership (0.127) following closely. Managerial Ownership (0.120) and Board Composition (0.112) also score moderately high, whereas Ownership Concentration (0.063) and Dispersed Ownership (0.057) are at the bottom. An inconsistency ratio of 0.07 remains under the 0.1 threshold, confirming logical consistency. This hierarchy reinforces the idea that top-level control and board structure can outweigh other governance nuances in financing decisions.
Rank | Criterion | Score |
1 | CEO Power | 0.191 |
2 | CEO Duality | 0.164 |
3 | Institutional Ownership | 0.127 |
4 | Managerial Ownership | 0.120 |
5 | Board Composition | 0.112 |
6 | Institutional Monitoring | 0.091 |
7 | Corporate Governance Quality | 0.074 |
8 | Ownership Concentration | 0.063 |
9 | Distributed Ownership | 0.057 |
Incompatibility Rate=0.07 |
Finally, we summarize the importance coefficients across each main criterion and its sub-criteria to produce Table 8, which lists all factors in descending order. These results confirm that behavioral factors (including CEO Personality Type, Financial Knowledge, and Overconfidence), alongside macroeconomic and governance dimensions, wield considerable influence on capital structure. Moreover, the pair-wise comparisons consistently show an inconsistency ratio below 1%, implying an overall coherent ranking. In the final scores (Table 8), an inconsistency ratio of 0.04 shows further evidence that participants largely agree with the relative significance of these variables.
Rank | Score | Rank | Score | ||
1 | Information Rent | 0.046 | 30 | Management Tenure | 0.015 |
2 | Managerial Personality Type | 0.040 | 31 | Perceptual Errors | 0.014 |
3 | Economic Sanctions | 0.039 | 32 | Liquidity | 0.014 |
4 | Exchange Rate Changes | 0.035 | 33 | Corporate Governance Quality | 0.014 |
5 | CEO Power | 0.035 | 34 | Managerial Experience | 0.013 |
6 | Financial Knowledge | 0.032 | 35 | Company Size | 0.013 |
7 | CEO Duality | 0.030 | 36 | Understanding Macroeconomic Environment | 0.012 |
8 | Tax Rate | 0.029 | 37 | Rule of Law | 0.012 |
9 | Overconfidence | 0.027 | 38 | Educational Background of CEO | 0.011 |
10 | Interest Rate | 0.025 | 39 | Uncertainty in Monetary and Fiscal Policies | 0.011 |
11 | Business Intelligence | 0.024 | 40 | Company Age | 0.011 |
12 | Tangible Assets | 0.024 | 41 | Audit Quality | 0.011 |
13 | Operational Risk | 0.023 | 42 | Ownership Concentration | 0.011 |
14 | Institutional Ownership | 0.023 | 43 | Gender of Managers | 0.010 |
15 | Financial Leverage | 0.022 | 44 | Alignment of Financial | 0.010 |
Conditions with Sharia | |||||
16 | Managerial Ownership | 0.022 | 45 | Sales Growth | 0.010 |
17 | Conservatism | 0.021 | 46 | Distributed Ownership | 0.010 |
18 | Current Liabilities | 0.021 | 47 | Business Cycles | 0.09 |
19 | Flexibility in Decision Making | 0.020 | 48 | Religious Orientation | 0.009 |
20 | Board Composition | 0.020 | 49 | Price-to-Book value | 0.009 |
21 | Shortsightedness | 0.019 | 50 | Administrative Corruption | 0.008 |
22 | Inflation Rate Changes | 0.019 | 51 | Non-Debt Tax Shield | 0.008 |
23 | Managerial Capability | 0.018 | 52 | Emulation of Other Industry Leading Managers | 0.007 |
24 | Excessive Optimism | 0.018 | 53 | Human Capital | 0.007 |
25 | Governance Quality | 0.018 | 54 | Presidential Elections | 0.006 |
26 | Asset Returns | 0.018 | 55 | Organizational Culture | 0.005 |
27 | Institutional Monitoring | 0.017 | 56 | Non-Economic Sanctions | 0.005 |
28 | Economic Growth | 0.016 | 57 | Social Trust | 0.004 |
29 | Systematic Risk | 0.016 | 58 | Enactment of New Laws | 0.004 |
Incompatibility Rate=0.04 |
In this segment, a 5-point Likert scale questionnaire identified during our earlier qualitative and AHP stages are distributed to the experts and capital market participants. Their feedback enabled us to assess each factor's relevance quantitatively, culminating in structural equation modeling (SEM). This approach ensures that the research questions (particularly those probing the synergy among behavioral, governance, and macro-level variables) are directly tested with broader input from finance professionals, though we acknowledge that market-based data (e.g., share price fluctuations) is absent and remains a possible direction for future expansions.
Structural Equation Modeling (SEM) provides a framework for examining how latent constructs—such as behavioral biases or macroeconomic forces—interact to shape corporate leverage. As shown below, we represent the connections between latent and observed variables via regression-like equations:
Y=ᴧyη+ε |
where Y denotes the observed indicators, Λy the factor loadings, η the latent constructs, and ε the measurement error. By translating theoretical categories into quantifiable constructs, we can observe how each domain (behavioral, political, etc.) contributes to capital structure, consistent with our core aims of this study.
In our Partial Least Squares (PLS-SEM) implementation, we prioritized prediction accuracy over traditional chi-square fit metrics—a logical choice given the exploratory nature of our inquiry and the multiple constructs involved. The earlier qualitative interviews and AHP rankings shape the questionnaire items administered to managers and experts, ensuring each factor are evaluated in a data-driven yet context-specific manner. Through PLS-SEM, we refine and validate how these categories—behavioral, macroeconomic, political, socio-cultural, corporate governance, and company characteristics—ultimately align with research questions about what drives capital structure in an emerging-market setting. Whereas covariance-based SEM relies on chi-square tests, PLS-SEM employs alternate measures—namely goodness of fit (GOF), redundancy, and colinearity indices—to gauge predictive accuracy. In our study, these metrics clarify how effectively each exogenous factor (e.g., managerial personality or macroeconomic constraints) helps to explain corporate leverage (the endogenous variable). As Figure 7 illustrates, we evaluate both the structural pathways and the measurement model, reporting on overall fit quality and inter-variable correlations. This emphasis on predictive strength aligns with earlier findings that political volatility and managerial biases might overshadow purely rational cost-benefit analyses in emerging markets.
In reflective measurement models, factor loadings of at least 0.70 typically demonstrate adequate homogeneity (Hair et al., 2017), though some scholars suggest removing any item below 0.40. As shown in Figure 8, most loadings in this study exceed 0.70, confirming that our observed variables strongly represent their latent constructs. One minor exception is Managerial Ownership at 0.666, which is retained because removing it will detract from the broader coverage of corporate governance. Follow-up Confirmatory Factor Analysis (CFA) further reinforces the sufficiency of these constructs, indicating that the metrics is used for each domain (behavioral, macroeconomic, etc.) consistently measure what they are intended to capture—in line with the research questions about how multiple dimension merge to shape capital structure.
The factor loading (λ) for each observed variable X is derived by:
λ=Cov(X,η)Var(η) |
Where Cov(X,η) is the covariance between the indicator and its latent construct η, and Var(η) is the construct's variance. This calculation quantifies how strongly each measured item aligns with the concept it is meant to represent—an essential step for verifying that behavioral biases or political influences are accurately captured.
Figure 8 visualizes the six latent constructs—behavioral, macroeconomic, socio-cultural, political, company characteristics, and corporate governance—showing that nearly all factor loadings surpass the 0.70 benchmark. Although Managerial Ownership again falls just short at 0.666, this minor deviation does not undercut the model's overall stability. Given that these constructs collectively address multiple RQs (including which specific managerial traits matter, and how external constraints compare), we deem it critical to preserve a comprehensive view of corporate governance in our measurement model.
Reliability of each measurement scale is assessed via Cronbach's alpha and composite reliability (CR). Cronbach's alpha (α) reflects the average covariance among items relative to their variance:
α=¯c.N¯c.(N−1)+¯υ |
Where N is the number of items, ¯c is their average covariance, and ¯υ is the average variance. CR (sometimes called Dillon-Goldstein's rho) provides a similar gauge of internal consistency. As depicted in Figure 9, all latent constructs surpass the 0.70 thresholds for both α and CR, implying robust reliability. In practical terms, these scales consistently capture the facets—such as risk aversion, institutional oversight, or economic sanctions—that our research questions identify as influential to corporate financing.
To confirm construct validity, we also compute Average Variance Extracted (AVE):
AVE=∑λ2∑λ2+∑∈i |
Where λ signifies factor loadings and ∈i the measurement error. Following Fornell et al. (1981), an AVE above 0.50 indicates that over half the variance in each set of observed indicators is captured by its latent factor. As Figure 9 shows, all AVE values exceed 0.5, verifying that each dimension—be it behavioral or macro—adequately reflects its underlying concept. This consistency is crucial for addressing our RQs about how different categories converge on a firm's capital structure choices.
A key metric in SEM is the coefficient of determination (R2), which reveals how much variance in the endogenous variable—here, capital structure—is explained by the exogenous factors. While thresholds of 0.19, 0.33, and 0.67 mark weak, moderate, and strong explanatory power, respectively, we also rely on an adjusted R2 to accommodate the number of predictors involved, ensuring we do not overstate their collective impact. The formula for R2 is
R2=1−∑(yi−ˆyi)2∑(yi−¯y)2 |
where yi are the observed values, ˆyi are the predicted values from the model, and ¯y is the mean of the observed values. Importantly, a high R2 alone does not guarantee that each variable meaningfully advances the research questions; the adjusted value helps clarify how many—and which—constructs truly drive corporate financing decisions.
The analysis reveals that the coefficient of determination for capital structure as the dependent variable is 0.963, while the adjusted coefficient of determination is 0.961. This shows that 96.3% of the variance in capital structure is explained by the independent variables, with only a minor adjustment due to the number of predictors.
The F2 effect size, developed by Cohen (1981), quantifies the impact of an independent variable on a dependent variable in structural equation modeling. Cohen defines F2 values of 0.02, 0.15, and 0.35 as small, medium, and large effects, respectively.
The F2 effect size is calculated using the formula:
f2=R2included−R2excluded1−R2included |
where R2included is the coefficient of determination with the independent variable included, and R2excluded is the coefficient of determination without the independent variable.
The results indicate that behavioral factors, company characteristics, and corporate governance have relatively weak effects, with values of 0.091, 0.131, and 0.111, respectively. Macroeconomic and socio-cultural factors exhibit moderate effects, with values of 0.162 and 0.163, respectively. Political factors exert the most significant influence on capital structure, with an F2 value of 0.967.
Figure 11 illustrates the F2 effect size for each variable, highlighting Political Factors as having the strongest influence on capital structure.
This chart displays the impact of various factors on capital structure, with political factors having the largest effect size, followed by Macroeconomic and Socio-Cultural Factors.
To evaluate the quality of the measurement model, several indices are employed, including communality (CV Com), cross-validated redundancy (CV Red), and the Goodness of Fit (GOF) index. The GOF index reflects the model's overall fit and is computed by combining the average variance extracted (AVE) with the coefficient of determination (R2):
GOF=√AVE×R2 |
A model is considered to have acceptable quality when the communality and redundancy indices are positive. GOF index values range from 0 to 1, with 0.01, 0.25, and 0.36 indicating weak, moderate, and strong model fit, respectively (Tenenhaus et al., 2011).
In this study, the communality values are positive for all variables, demonstrating the model's satisfactory quality. The GOF index for Behavioral Factors is 0.799, indicating a strong fit of the model. Redundancy (CV Red) applies only to the Capital Structure variable, and its positive value further reinforces the robustness of the model. The bar chart below visually displays the CV Com, CV Red, and GOF indices for the various variables.
In this study, we aim to identify and prioritize the factors influencing the capital structure of Iranian companies, with an emphasis on the mediating role of behavioral factors. A mixed-methods approach combining qualitative and quantitative data is used to develop and validate a comprehensive model of capital structure determinants.
The results highlight the significant influence of macroeconomic factors, behavioral aspects, corporate governance, and company-specific characteristics on capital structure decisions. Behavioral factors, particularly the personality traits and financial knowledge of CEOs, are crucial and suggest that corporate finance studies must consider psychological biases and managerial attributes.
➢ Expanding the Scope: Researchers could explore the impact of behavioral factors on capital structure decisions across industries and countries, with a focus on comparing state-owned and private enterprises. This would provide deeper insights into how cultural and institutional contexts shape financial decision-making.
➢ Longitudinal Studies: Longitudinal research could help track how behavioral factors evolve and affect long-term capital structure decisions, aiding in establishing causal relationships and better understanding the dynamics at play.
➢ Behavioral Interventions: Researchers could assess the effectiveness of behavioral interventions aimed at mitigating cognitive biases in financial decision-making. For example, training programs to enhance financial literacy and decision-making skills among managers could be evaluated for their impact on corporate outcomes.
➢ Integration with Technology: Investigating the role of technology, such as artificial intelligence and big data analytics, in shaping the influence of behavioral factors on capital structure decisions could offer valuable insights. Understanding how technology can assist in making more informed, rational decisions is a promising area for future research.
➢ Managerial Training: Companies could implement training programs to improve executives' financial knowledge and decision-making skills, reducing the impact of cognitive biases and enhancing the effectiveness of capital structure decisions.
➢ Policy Recommendations: Policymakers should consider these findings when designing regulations that promote sound corporate governance practices. Encouraging transparency and accountability in decision-making could help mitigate the negative effects of behavioral biases.
➢ Corporate Governance Reforms: Companies may benefit from revisiting their corporate governance structures, particularly regarding board composition and CEO oversight. Ensuring a diverse, balanced board can help counteract overconfidence or excessive optimism among managers.
➢ Strategic Financial Planning: Firms could incorporate the identified behavioral factors into their strategic financial planning processes. By acknowledging and addressing these factors, companies may optimize their capital structures more effectively and enhance their financial resilience.
➢ Practical and Policy-Oriented Suggestions: Organizations could implement leadership training and improved oversight to reduce biases like overconfidence, which is consistent with our findings that CEO personality traits and financial knowledge have a significant impact on firms' capital structure (Barton & Gordon, 1988; Heaton, 2002; Alves et al., 2015, Costa et al., 2017; Banerjee et al., 2018; Guenzel & Malmendier, 2020). To enhance market confidence in the face of economic uncertainty and to consider behavioral factors alongside other critical elements as key contributors to managerial decision-making, the Securities and Exchange Organization (SEO) could implement regulations requiring mandatory disclosure of board composition changes or the regular inclusion of managerial psychology assessments in annual reporting requirements.
Ehsan Ahmadi, Parastoo Mohammadi and Farimah Mokhatab Rafei: Conceptualization, methodology, data analysis, and original draft writing, Data collection, literature review; Shib Sankar Sana: Supervision and critical review of the manuscript.
The authors declare they have not used Artificial Intelligence (AI) tools in the creation of this article.
The authors declare no conflict of interest.
[1] |
Ahmad M (2024) The role of cognitive heuristic-driven biases in investment management activities and market efficiency: a research synthesis. Int J of Emer Mark 19: 273-321. https://doi.org/10.1108/IJOEM-07-2020-0749 doi: 10.1108/IJOEM-07-2020-0749
![]() |
[2] |
Ah Mand A, Janor H, Abdul RR, Sarmidi T (2023) Herding behavior and stock market conditions. PSU Res Rev 7: 105-116. https://doi.org/10.1108/PRR-10-2020-0033 doi: 10.1108/PRR-10-2020-0033
![]() |
[3] |
Aibar-Guzmán B, García-Sánchez IM, Aibar-Guzmán C, et al. (2022) Sustainable product innovation in agri-food industry: Do ownership structure and capital structure matter? J Innov Knowl 7: 100160. https://doi.org/10.1016/j.jik.2021.100160 doi: 10.1016/j.jik.2021.100160
![]() |
[4] |
Al‐Zoubi HA, O’Sullivan JA, Al‐Maghyereh AI, et al. (2022) Disentangling sentiment from cyclicality in firm capital structure. ABACUS 59: 570-605. https://doi.org/10.1111/abac.12274 doi: 10.1111/abac.12274
![]() |
[5] |
Alves P, Couto EB, Francisco PM (2015) Board of directors' composition and capital structure. Res Int Bus Financ 35: 1–32. https://doi.org/10.1016/j.ribaf.2015.03.005 doi: 10.1016/j.ribaf.2015.03.005
![]() |
[6] |
Badheka GT, Pandya K (2022) Behavioural Capital Structure: A Systematic Literature Review on the Role of Psychological Factors in Determining the Capital Structure. Int J Financ Manag 12: 1–9. doi: 10.22495/rcgv6i4c1art13 doi: 10.22495/rcgv6i4c1art13
![]() |
[7] |
Banerjee S, Humphery-Jenner M, Nanda V (2018) Does CEO bias escalate repurchase activity? J Bank Financ 93: 105–126. https://doi.org/10.1016/j.jbankfin.2018.02.003 doi: 10.1016/j.jbankfin.2018.02.003
![]() |
[8] |
Barton SL, Gordon PJ (1988) Corporate strategy and capital structure. Strategic Manage J 9: 623–632. https://doi.org/10.1002/smj.4250090608 doi: 10.1002/smj.4250090608
![]() |
[9] |
Baker M, Wurgler J (2002) Market timing and capital structure. J Financ 57: 1–32. https://doi.org/10.1111/1540-6261.00414 doi: 10.1111/1540-6261.00414
![]() |
[10] | Bokpin GA(2010) Financial market development and corporate financing: evidence from emerging market economies. J of Eco Stud 37: 96-116. http://dx.doi.org/10.1108/01443581011012270 |
[11] |
Bradley M, Jarrell GA, Kim EH (1984) On the existence of an optimal capital structure: Theory and evidence. J Financ 39: 857–878. https://doi.org/10.2307/2327950 doi: 10.2307/2327950
![]() |
[12] |
Çam İ, Özer G (2022) The influence of country governance on the capital structure and investment financing decisions of firms: An international investigation. Borsa Istanb Rev 22: 257–271. https://doi.org/10.1016/j.bir.2021.04.008 doi: 10.1016/j.bir.2021.04.008
![]() |
[13] |
Carrizosa R, Gaertner FB, Lynch D (2020) Debt and Taxes? The Effect of TCJA Interest Limitations on Capital Structure. Available at SSRN. http://dx.doi.org/10.2139/ssrn.3397285 doi: 10.2139/ssrn.3397285
![]() |
[14] |
Carrizosa RD, Gaertner FB, Lynch DP (2023) Debt and taxes? The effect of Tax Cuts & Jobs Act of 2017 interest limitations on capital structure. J Am Tax Assoc 45: 35–55. https://doi.org/10.2308/JATA-2021-010 doi: 10.2308/JATA-2021-010
![]() |
[15] |
Chauhan Y, Jaiswall M, Goyal V (2022) Does societal trust affect corporate capital structure? Emerg Mark Rev 51: 100845. https://doi.org/10.1016/j.ememar.2021.100845 doi: 10.1016/j.ememar.2021.100845
![]() |
[16] |
Choi PMS, Choi JH, Chung CY, et al. (2020) Corporate governance and capital structure: Evidence from sustainable institutional ownership. Sustainability 12: 4190. https://doi.org/10.3390/su12104190 doi: 10.3390/su12104190
![]() |
[17] |
Chua M, Ab Razak NH, Nassir AM, et al. (2022) Dynamic capital structure in Indonesia: does the education and experience of CEOs matter? Asia Pac Manag Rev 27: 58–68. https://doi.org/10.1016/j.apmrv.2021.05.003 doi: 10.1016/j.apmrv.2021.05.003
![]() |
[18] |
Costa DF, de Melo Carvalho F, de Melo Moreira BC, et al. (2017) Bibliometric analysis on the association between behavioral finance and decision making with cognitive biases such as overconfidence, anchoring effect and confirmation bias. Scientometrics 111: 1775–1799. https://doi.org/10.1007/s11192-017-2371-5 doi: 10.1007/s11192-017-2371-5
![]() |
[19] |
Danso A, Fosu S, Owusu‐Agyei S, et al. (2021) Capital structure revisited. Do crisis and competition matter in a Keiretsu corporate structure? Int J Financ Econ 26: 5073–5092. https://doi.org/10.1002/ijfe.2055 doi: 10.1002/ijfe.2055
![]() |
[20] | Faysal S, Salehi M, Moradi M (2020) Impact of corporate governance mechanisms on the cost of equity capital in emerging markets. J of Pub Affairs 21: e2166. 16 pages. https://doi.org/10.1002/pa.2166 |
[21] |
Fama EF (1970) Efficient capital markets. J Financ 25: 383–417. https://doi.org/10.7208/9780226426983-007 doi: 10.7208/9780226426983-007
![]() |
[22] |
Fama EF, French KR (1998) Taxes, financing decisions, and firm value. J Financ 53: 819–843. https://doi.org/10.1111/0022-1082.00036 doi: 10.1111/0022-1082.00036
![]() |
[23] |
Fornell C, Larcker DF (1981) Evaluating structural equation models with unobservable variables and measurement error. J Mark Res 18: 39–50. https://doi.org/10.1177/002224378101800104 doi: 10.1177/002224378101800104
![]() |
[24] |
Frank MZ, Goyal VK (2003) Testing the pecking order theory of capital structure. J Financ Econ 67: 217–248. https://doi.org/10.1016/S0304-405X(02)00252-0 doi: 10.1016/S0304-405X(02)00252-0
![]() |
[25] |
Frank MZ, Goyal VK (2009) Capital structure decisions: which factors are reliably important? Financ Manage 38: 1–37. https://doi.org/10.1111/j.1755-053X.2009.01026.x doi: 10.1111/j.1755-053X.2009.01026.x
![]() |
[26] |
Frankfurter GM, McGoun EG (2002) Resistance is futile: the assimilation of behavioral finance. J Econ Behav Organ 48: 375–389. https://doi.org/10.1016/S0167-2681(01)00241-4 doi: 10.1016/S0167-2681(01)00241-4
![]() |
[27] |
Graham JR, Harvey CR, Puri M (2013) Managerial attitudes and corporate actions. J Financ Econ 109: 103–121. https://doi.org/10.1016/j.jfineco.2013.01.010 doi: 10.1016/j.jfineco.2013.01.010
![]() |
[28] |
Guenzel M, Malmendier U (2020) Behavioral corporate finance: The life cycle of a CEO career (No. w27635). Natl Bureau Econ Res. https://doi.org/10.3386/w27635. doi: 10.3386/w27635
![]() |
[29] |
Guizani M, Ajmi AN (2021) The financial determinants of corporate cash holdings: Does sharia-compliance. Mont J of Eco 17: 157-168. http://dx.doi.org/10.14254/1800-5845/2021.17-3.13 doi: 10.14254/1800-5845/2021.17-3.13
![]() |
[30] |
Heaton JB (2002) Managerial optimism and corporate finance. FINANCIAL MANAGEMENT-TAMPA, 31: 33–46. https://doi.org/10.1515/9781400829125-022 doi: 10.1515/9781400829125-022
![]() |
[31] | Jensen MC, Meckling WH (2019) Theory of the firm: Managerial behavior, agency costs and ownership structure. In: Corporate Governance, 77–132. Gower. https://doi.org/10.1016/0304-405X(76)90026-X |
[32] |
Jansen K, Michiels A, Voordeckers W et al. (2023) Financing decisions in private family firms: a family firm pecking order. Small Bus Econ 61: 495–515 . https://doi.org/10.1007/s11187-022-00711-9 doi: 10.1007/s11187-022-00711-9
![]() |
[33] |
Jiang W, Li J, Sun G (2021) Economic policy uncertainty and stock markets: A multifractal cross-correlations analysis. Fluct and Noi Lett 22: Article no. 2150018. https://doi.org/10.1142/S0219477521500188 doi: 10.1142/S0219477521500188
![]() |
[34] |
Lapinski MK, Kerr JM, Miller Ⅲ HW, et al. (2024) Persuasive Communication, Financial Incentives, and Social Norms: Interactions and Effects on Behaviors. Curr Opin Psychol 101851. https://doi.org/10.1016/j.copsyc.2024.101851 doi: 10.1016/j.copsyc.2024.101851
![]() |
[35] |
Liu Y, Liu Y, Wei Z (2022) Property rights protection, financial constraint, and capital structure choices: Evidence from a Chinese natural experiment. J Corp Financ 73: 102167. https://doi.org/10.1016/j.jcorpfin.2022.102167 doi: 10.1016/j.jcorpfin.2022.102167
![]() |
[36] |
Li X, Chen K, Yuan GX, et al. (2021) The decision‐making of optimal equity and capital structure based on dynamical risk profiles: A Langevin system framework for SME growth. Int J Intell Syst 36: 3500–3523. https://doi.org/10.1002/int.22424 doi: 10.1002/int.22424
![]() |
[37] |
Mapela L, Chipeta C (2023) Managerial confidence and capital structure announcement effects on share prices on the Johannesburg Share Exchange. South African J Econ Manag Sci 26: 4849. https://doi.org/10.4102/sajems.v26i1.4849 doi: 10.4102/sajems.v26i1.4849
![]() |
[38] |
Miller EM (1977) Risk, uncertainty, and divergence of opinion. J Financ 32: 1151–1168. https://doi.org/10.1111/j.1540-6261.1977.tb03317.x doi: 10.1111/j.1540-6261.1977.tb03317.x
![]() |
[39] | Modigliani F, Miller M H (1958) The cost of capital, corporation finance and the theory of investment. The Amer Eco Rev 48: 261-296. https://www.jstor.org/stable/1809766 |
[40] |
Mogha V, Williams B (2021) Culture and capital structure: What else to the puzzle? Int Rev Financ Anal 73: 101614. https://doi.org/10.1016/j.irfa.2020.101614 doi: 10.1016/j.irfa.2020.101614
![]() |
[41] |
Mundi HS (2022) CEO social capital and capital structure complexity. J Behav Exp Financ 35: 100719. https://doi.org/10.1016/j.jbef.2022.100719 doi: 10.1016/j.jbef.2022.100719
![]() |
[42] |
Muhammad AUR (2022) The impact of investor sentiment on returns, cash flows, discount rates, and performance. Borsa Istanbul Revi 22: 352–362. https://doi.org/10.1016/j.bir.2021.06.005 doi: 10.1016/j.bir.2021.06.005
![]() |
[43] |
Myers SC (1984) The Capital Structure Puzzle. J Financ 39: 574–592. https://doi.org/10.1111/j.1540-6261.1984.tb03646.x doi: 10.1111/j.1540-6261.1984.tb03646.x
![]() |
[44] |
Oprean C (2014) Effects of behavioural factors on human financial decisions. Procedia Econ Financ 16: 458–463. https://doi.org/10.1016/S2212-5671(14)00825-9 doi: 10.1016/S2212-5671(14)00825-9
![]() |
[45] |
Orlova S, Harper JT, Sun L (2020) Determinants of capital structure complexity. J Econ Bus 110: 105905. https://doi.org/10.1016/j.jeconbus.2020.105905 doi: 10.1016/j.jeconbus.2020.105905
![]() |
[46] |
Pérez J, Reyna M, Vera-Martinez J (2019) Capital structure construct: a new approach to behavioral finance. Invest Manage Financ Innov 16: 86–97. https://doi.org/10.21511/imfi.16(4).2019.08 doi: 10.21511/imfi.16(4).2019.08
![]() |
[47] |
Rashid M, Hj DSNKP, Izadi S (2023) National culture and capital structure of the Shariah compliant firms: Evidence from Malaysia, Saudi Arabia and Pakistan. Int Rev Econ Financ 86: 949–964. https://doi.org/10.1016/j.iref.2020.10.006 doi: 10.1016/j.iref.2020.10.006
![]() |
[48] |
Sajid M, Mushtaq R, Murtaza G, et al. (2024) Financial literacy, confidence and well-being: The mediating role of financial behavior. J Bus Res 182: 114791. https://doi.org/10.1016/j.jbusres.2024.114791 doi: 10.1016/j.jbusres.2024.114791
![]() |
[49] |
Salam A Z, Shourkashti R (2019) Capital structure and firm performance in emerging market: An empirical analysis of Malaysian companies. Int J of Acad Res in Accou, Finan and Manag Sci 9: 70–82. http://dx.doi.org/10.6007/IJARAFMS/v9-i3/6334 doi: 10.6007/IJARAFMS/v9-i3/6334
![]() |
[50] |
Singh BP, Kannadhasan M (2020) Corruption and capital structure in emerging markets: A panel quantile regression approach. J Behav Expe Financ 28: 100417. https://doi.org/10.1016/j.jbef.2020.100417 doi: 10.1016/j.jbef.2020.100417
![]() |
[51] |
Shahmi MA (2023) Interaction of variable macroeconomic shock and monetary interventions on the profitability of Sharia commercial banking in Indonesia. Imara:J Riset Ekon Islam 7: 11-19. http://dx.doi.org/10.31958/imara.v7i1.9360 doi: 10.31958/imara.v7i1.9360
![]() |
[52] |
Sunitha K (2024) Targeting Behavior and Capital Structure Theories: An Empirical Analysis of Gulf Cooperation Council Countries. J Behav Expe Financ 100944. https://doi.org/10.1016/j.jbef.2024.100944 doi: 10.1016/j.jbef.2024.100944
![]() |
[53] |
Tenenhaus A, Tenenhaus M (2011) Regularized generalized canonical correlation analysis. Psychometrika 76: 257–284. https://doi.org/10.1007/s11336-011-9206-8 doi: 10.1007/s11336-011-9206-8
![]() |
[54] |
Thaler RH (1999) The end of behavioral finance. Financ Anal J 55: 12–17. https://doi.org/10.2469/faj.v55.n6.2310 doi: 10.2469/faj.v55.n6.2310
![]() |
[55] |
Ting IWK, Lean HH, Kweh QL, et al. (2016) Managerial overconfidence, government intervention and corporate financing decision. Int J Manag Financ 12: 4–24. https://doi.org/10.1108/IJMF-04-2014-0041 doi: 10.1108/IJMF-04-2014-0041
![]() |
[56] |
Titman S, Wessels R (1988) The determinants of capital structure choice. J Financ 43: 1–19. https://doi.org/10.1111/j.1540-6261.1988.tb02585.x doi: 10.1111/j.1540-6261.1988.tb02585.x
![]() |
[57] | Wang R, Zhou L (2025) Capital structure deviation and corporate technological innovation: Empirical evidence from the perspective of ESG responsibility fulfillment. Available at SSRN: https://ssrn.com/abstract=5163595 or http://dx.doi.org/10.2139/ssrn.5163595 |
[58] |
Xing Y (2020) The impact of behavioral bias on individual investors and corporation capital structure. Acad J Bus Manag 2: 112–121. https://doi.org/10.25236/AJBM.2020.020401. doi: 10.25236/AJBM.2020.020401
![]() |
[59] |
Xia C, Chan KC, Cao C, et al. (2021) Generalized trust, personalized trust, and dynamics of capital structure: Evidence from China. China Econ Rev 68: 101640. https://doi.org/10.1016/j.chieco.2021.101640 doi: 10.1016/j.chieco.2021.101640
![]() |
[60] |
Yang B, Gan L, Wen C (2021). Moral hazard, debt overhang and capital structure. North Am J Econ Financ 58, 101538. https://doi.org/10.1016/j.najef.2021.101538 doi: 10.1016/j.najef.2021.101538
![]() |
[61] | Zou Y, Bai Q (2022) The impact of dividend policies and financing strategies on the speed of firms’ capital structure adjustment. Discr Dyna in Nat and Soc 2022: Article ID 3209502, 12 pages. https://doi.org/10.1155/2022/3209502 |
Rank | Criterion | Score |
1 | Macroeconomic Factors | 0.266 |
2 | Behavioral Factors | 0.230 |
3 | Corporate Governance | 0.201 |
4 | Company Characteristics | 0.141 |
5 | Political Factors | 0.103 |
6 | Socio-Cultural Factors | 0.059 |
Incompatibility Rate=0.03 |
Rank | Criterion | Score |
1 | Managerial Personality Type | 0.132 |
2 | Financial Knowledge | 0.107 |
3 | Overconfidence | 0.089 |
4 | Business Intelligence | 0.081 |
5 | Conservatism | 0.071 |
6 | Flexibility in Decision Making | 0.067 |
7 | Shortsightedness | 0.064 |
8 | Excessive Optimism | 0.061 |
9 | Managerial Capability | 0.059 |
10 | Management Tenure | 0.051 |
11 | Perceptual Errors | 0.045 |
12 | Managerial Experience | 0.043 |
13 | Understanding Macroeconomic Environment | 0.038 |
14 | Educational Background of CEO | 0.036 |
15 | Gender of Managers | 0.034 |
16 | Emulation of Other Industry Leading Managers | 0.022 |
Incompatibility Rate=0.06 |
Rank | Criterion | Score |
1 | Information Rent | 0.201 |
2 | Economic Sanctions | 0.172 |
3 | Exchange Rate Changes | 0.151 |
4 | Tax Rate | 0.125 |
5 | Interest Rate | 0.107 |
6 | Inflation Rate Changes | 0.083 |
7 | Economic Growth | 0.071 |
8 | Uncertainty in Monetary and Fiscal Policies | 0.049 |
9 | Business Cycles | 0.041 |
Incompatibility Rate=0.03 |
Rank | Criterion | Score |
1 | Alignment of Financial Conditions with Sharia | 0.363 |
2 | Religious Orientation | 0.320 |
3 | Organizational Culture | 0.179 |
4 | Social Trust | 0.138 |
Incompatibility Rate=0.04 |
Rank | Criterion | Score |
1 | Governance Quality | 0.338 |
2 | Rule of Law | 0.219 |
3 | Administrative Corruption | 0.153 |
4 | Presidential Elections | 0.121 |
5 | Non-Economic Sanctions | 0.091 |
6 | Enactment of New Laws | 0.077 |
Incompatibility Rate=0.04 |
Rank | Criterion | Score |
1 | Tangible Assets | 0.118 |
2 | Operational Risk | 0.112 |
3 | Financial Leverage | 0.107 |
4 | Current Liabilities | 0.104 |
5 | Asset Returns | 0.088 |
6 | Systematic Risk | 0.075 |
7 | Liquidity | 0.066 |
8 | Company Size | 0.062 |
9 | Audit Quality | 0.055 |
10 | Company Age | 0.051 |
11 | Sales Growth | 0.046 |
12 | Price-to-Book value | 0.042 |
13 | Non-Debt Tax Shield | 0.038 |
14 | Human Capital | 0.034 |
Incompatibility Rate=0.05 |
Rank | Criterion | Score |
1 | CEO Power | 0.191 |
2 | CEO Duality | 0.164 |
3 | Institutional Ownership | 0.127 |
4 | Managerial Ownership | 0.120 |
5 | Board Composition | 0.112 |
6 | Institutional Monitoring | 0.091 |
7 | Corporate Governance Quality | 0.074 |
8 | Ownership Concentration | 0.063 |
9 | Distributed Ownership | 0.057 |
Incompatibility Rate=0.07 |
Rank | Score | Rank | Score | ||
1 | Information Rent | 0.046 | 30 | Management Tenure | 0.015 |
2 | Managerial Personality Type | 0.040 | 31 | Perceptual Errors | 0.014 |
3 | Economic Sanctions | 0.039 | 32 | Liquidity | 0.014 |
4 | Exchange Rate Changes | 0.035 | 33 | Corporate Governance Quality | 0.014 |
5 | CEO Power | 0.035 | 34 | Managerial Experience | 0.013 |
6 | Financial Knowledge | 0.032 | 35 | Company Size | 0.013 |
7 | CEO Duality | 0.030 | 36 | Understanding Macroeconomic Environment | 0.012 |
8 | Tax Rate | 0.029 | 37 | Rule of Law | 0.012 |
9 | Overconfidence | 0.027 | 38 | Educational Background of CEO | 0.011 |
10 | Interest Rate | 0.025 | 39 | Uncertainty in Monetary and Fiscal Policies | 0.011 |
11 | Business Intelligence | 0.024 | 40 | Company Age | 0.011 |
12 | Tangible Assets | 0.024 | 41 | Audit Quality | 0.011 |
13 | Operational Risk | 0.023 | 42 | Ownership Concentration | 0.011 |
14 | Institutional Ownership | 0.023 | 43 | Gender of Managers | 0.010 |
15 | Financial Leverage | 0.022 | 44 | Alignment of Financial | 0.010 |
Conditions with Sharia | |||||
16 | Managerial Ownership | 0.022 | 45 | Sales Growth | 0.010 |
17 | Conservatism | 0.021 | 46 | Distributed Ownership | 0.010 |
18 | Current Liabilities | 0.021 | 47 | Business Cycles | 0.09 |
19 | Flexibility in Decision Making | 0.020 | 48 | Religious Orientation | 0.009 |
20 | Board Composition | 0.020 | 49 | Price-to-Book value | 0.009 |
21 | Shortsightedness | 0.019 | 50 | Administrative Corruption | 0.008 |
22 | Inflation Rate Changes | 0.019 | 51 | Non-Debt Tax Shield | 0.008 |
23 | Managerial Capability | 0.018 | 52 | Emulation of Other Industry Leading Managers | 0.007 |
24 | Excessive Optimism | 0.018 | 53 | Human Capital | 0.007 |
25 | Governance Quality | 0.018 | 54 | Presidential Elections | 0.006 |
26 | Asset Returns | 0.018 | 55 | Organizational Culture | 0.005 |
27 | Institutional Monitoring | 0.017 | 56 | Non-Economic Sanctions | 0.005 |
28 | Economic Growth | 0.016 | 57 | Social Trust | 0.004 |
29 | Systematic Risk | 0.016 | 58 | Enactment of New Laws | 0.004 |
Incompatibility Rate=0.04 |
Rank | Criterion | Score |
1 | Macroeconomic Factors | 0.266 |
2 | Behavioral Factors | 0.230 |
3 | Corporate Governance | 0.201 |
4 | Company Characteristics | 0.141 |
5 | Political Factors | 0.103 |
6 | Socio-Cultural Factors | 0.059 |
Incompatibility Rate=0.03 |
Rank | Criterion | Score |
1 | Managerial Personality Type | 0.132 |
2 | Financial Knowledge | 0.107 |
3 | Overconfidence | 0.089 |
4 | Business Intelligence | 0.081 |
5 | Conservatism | 0.071 |
6 | Flexibility in Decision Making | 0.067 |
7 | Shortsightedness | 0.064 |
8 | Excessive Optimism | 0.061 |
9 | Managerial Capability | 0.059 |
10 | Management Tenure | 0.051 |
11 | Perceptual Errors | 0.045 |
12 | Managerial Experience | 0.043 |
13 | Understanding Macroeconomic Environment | 0.038 |
14 | Educational Background of CEO | 0.036 |
15 | Gender of Managers | 0.034 |
16 | Emulation of Other Industry Leading Managers | 0.022 |
Incompatibility Rate=0.06 |
Rank | Criterion | Score |
1 | Information Rent | 0.201 |
2 | Economic Sanctions | 0.172 |
3 | Exchange Rate Changes | 0.151 |
4 | Tax Rate | 0.125 |
5 | Interest Rate | 0.107 |
6 | Inflation Rate Changes | 0.083 |
7 | Economic Growth | 0.071 |
8 | Uncertainty in Monetary and Fiscal Policies | 0.049 |
9 | Business Cycles | 0.041 |
Incompatibility Rate=0.03 |
Rank | Criterion | Score |
1 | Alignment of Financial Conditions with Sharia | 0.363 |
2 | Religious Orientation | 0.320 |
3 | Organizational Culture | 0.179 |
4 | Social Trust | 0.138 |
Incompatibility Rate=0.04 |
Rank | Criterion | Score |
1 | Governance Quality | 0.338 |
2 | Rule of Law | 0.219 |
3 | Administrative Corruption | 0.153 |
4 | Presidential Elections | 0.121 |
5 | Non-Economic Sanctions | 0.091 |
6 | Enactment of New Laws | 0.077 |
Incompatibility Rate=0.04 |
Rank | Criterion | Score |
1 | Tangible Assets | 0.118 |
2 | Operational Risk | 0.112 |
3 | Financial Leverage | 0.107 |
4 | Current Liabilities | 0.104 |
5 | Asset Returns | 0.088 |
6 | Systematic Risk | 0.075 |
7 | Liquidity | 0.066 |
8 | Company Size | 0.062 |
9 | Audit Quality | 0.055 |
10 | Company Age | 0.051 |
11 | Sales Growth | 0.046 |
12 | Price-to-Book value | 0.042 |
13 | Non-Debt Tax Shield | 0.038 |
14 | Human Capital | 0.034 |
Incompatibility Rate=0.05 |
Rank | Criterion | Score |
1 | CEO Power | 0.191 |
2 | CEO Duality | 0.164 |
3 | Institutional Ownership | 0.127 |
4 | Managerial Ownership | 0.120 |
5 | Board Composition | 0.112 |
6 | Institutional Monitoring | 0.091 |
7 | Corporate Governance Quality | 0.074 |
8 | Ownership Concentration | 0.063 |
9 | Distributed Ownership | 0.057 |
Incompatibility Rate=0.07 |
Rank | Score | Rank | Score | ||
1 | Information Rent | 0.046 | 30 | Management Tenure | 0.015 |
2 | Managerial Personality Type | 0.040 | 31 | Perceptual Errors | 0.014 |
3 | Economic Sanctions | 0.039 | 32 | Liquidity | 0.014 |
4 | Exchange Rate Changes | 0.035 | 33 | Corporate Governance Quality | 0.014 |
5 | CEO Power | 0.035 | 34 | Managerial Experience | 0.013 |
6 | Financial Knowledge | 0.032 | 35 | Company Size | 0.013 |
7 | CEO Duality | 0.030 | 36 | Understanding Macroeconomic Environment | 0.012 |
8 | Tax Rate | 0.029 | 37 | Rule of Law | 0.012 |
9 | Overconfidence | 0.027 | 38 | Educational Background of CEO | 0.011 |
10 | Interest Rate | 0.025 | 39 | Uncertainty in Monetary and Fiscal Policies | 0.011 |
11 | Business Intelligence | 0.024 | 40 | Company Age | 0.011 |
12 | Tangible Assets | 0.024 | 41 | Audit Quality | 0.011 |
13 | Operational Risk | 0.023 | 42 | Ownership Concentration | 0.011 |
14 | Institutional Ownership | 0.023 | 43 | Gender of Managers | 0.010 |
15 | Financial Leverage | 0.022 | 44 | Alignment of Financial | 0.010 |
Conditions with Sharia | |||||
16 | Managerial Ownership | 0.022 | 45 | Sales Growth | 0.010 |
17 | Conservatism | 0.021 | 46 | Distributed Ownership | 0.010 |
18 | Current Liabilities | 0.021 | 47 | Business Cycles | 0.09 |
19 | Flexibility in Decision Making | 0.020 | 48 | Religious Orientation | 0.009 |
20 | Board Composition | 0.020 | 49 | Price-to-Book value | 0.009 |
21 | Shortsightedness | 0.019 | 50 | Administrative Corruption | 0.008 |
22 | Inflation Rate Changes | 0.019 | 51 | Non-Debt Tax Shield | 0.008 |
23 | Managerial Capability | 0.018 | 52 | Emulation of Other Industry Leading Managers | 0.007 |
24 | Excessive Optimism | 0.018 | 53 | Human Capital | 0.007 |
25 | Governance Quality | 0.018 | 54 | Presidential Elections | 0.006 |
26 | Asset Returns | 0.018 | 55 | Organizational Culture | 0.005 |
27 | Institutional Monitoring | 0.017 | 56 | Non-Economic Sanctions | 0.005 |
28 | Economic Growth | 0.016 | 57 | Social Trust | 0.004 |
29 | Systematic Risk | 0.016 | 58 | Enactment of New Laws | 0.004 |
Incompatibility Rate=0.04 |