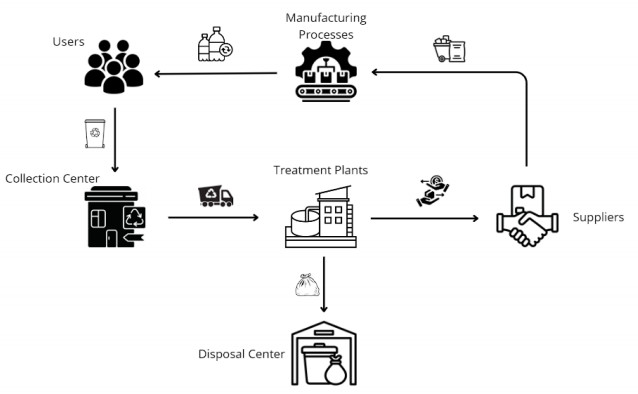
The consumption and usage of polyethylene terephthalate (PET) bottles have significantly increased in recent years, necessitating action to mitigate their environmental impact. Recycling programs offer a viable solution to address this impact within the PET industry. Therefore, adopting a circular economy approach is appropriate for incorporating recycling initiatives. Nevertheless, it is crucial not to overlook the financial aspects of the supply chain. This paper proposed a bi-level model to address both the financial and environmental concerns of a circular economy supply chain. At the upper level, a recycling company (the leader) aimd to establish PET bottle collection facilities, set prices for each kilogram of PET received, and manage the transportation of collected PET to the treatment plant. At the lower level of the bi-level problem, persons (the followers) decided whether to recycle or not. They received economic incentives based on the amount deposited at the collection facilities, but this came with associated travel costs. The leader's objective was to maximize the profit of the recycling program, assuming the sale of recycled PET. Meanwhile, followers aimed to recycle if it was economically viable for them. To solve this problem, a matheuristic algorithm was proposed, which hybridized a greedy random adaptive search procedure with an exact routing process. The matheuristic managed solutions at the leader level, while followers' responses were derived by exploiting the problem's structure. A case study derived from Mexico City was solved, and practical managerial insights were provided. Sensitivity analysis revealed crucial aspects that should not be overlooked when implementing such a recycling program.
Citation: Carlos Corpus, Ali Zahedi, Eva Selene Hernández-Gress, José-Fernando Camacho-Vallejo. A bi-level optimization model for PET bottle recycling within a circular economy supply chain[J]. AIMS Environmental Science, 2025, 12(2): 223-251. doi: 10.3934/environsci.2025010
[1] | Afshin Meraj, Tina Shoa, Fereshteh Sadeghi Naieni Fard, Hassan Mina . A comprehensive bi-objective optimization model to design circular supply chain networks for sustainable electric vehicle batteries. AIMS Environmental Science, 2024, 11(2): 279-303. doi: 10.3934/environsci.2024013 |
[2] | Pailin Srisuratsiri, Ketsarin Chantarasunthon, Wanutsanun Sudsai, Pichet Sukprasert, Laksamee Chaicharoenwimolkul Chuaitammakit, Wissawat Sakulsaknimitr . Sustainable plastic bottle recycling: employing zinc-deposited SBA-15 as a catalyst for glycolysis of polyethylene terephthalate. AIMS Environmental Science, 2024, 11(1): 90-106. doi: 10.3934/environsci.2024006 |
[3] | Filippo Sgroi . Circular economy and environmental protection. AIMS Environmental Science, 2022, 9(2): 122-127. doi: 10.3934/environsci.2022009 |
[4] | Ruchira R N Ghormare, Sana Fatima, Priya Grover, Nidhi Phutela, Vinay Kandpal, Ernesto D. R. Santibanez Gonzalez . Exploring the paradigm shift towards sustainability: A systematic literature review on circular economy and eco-innovation. AIMS Environmental Science, 2024, 11(6): 940-959. doi: 10.3934/environsci.2024047 |
[5] | Chukwuebuka Okafor, Charles Ajaero, Christian Madu, Kingsley Agomuo, Ezekiel Abu . Implementation of circular economy principles in management of end-of-life tyres in a developing country (Nigeria). AIMS Environmental Science, 2020, 7(5): 406-433. doi: 10.3934/environsci.2020027 |
[6] | Atinuke Chineme, Getachew Assefa, Irene M. Herremans, Barry Wylant, Marwa Shumo, Aliceanna Shoo, Mturi James, Frida Ngalesoni, Anthony Ndjovu, Steve Mbuligwe, Mike Yhedgo . Advancing circular economy principles through wild black soldier flies. AIMS Environmental Science, 2023, 10(6): 868-893. doi: 10.3934/environsci.2023047 |
[7] | Antonio Zanin, Ivonez Xavier de Almeida, Francieli Pacassa, Fabricia Silva da Rosa, Paulo Afonso . Maturity level of environmental management in the pulp and paper supply chain. AIMS Environmental Science, 2021, 8(6): 580-596. doi: 10.3934/environsci.2021037 |
[8] | Jana E. Archer, Ingrid E. Luffman, Arpita N. Nandi, T. Andrew Joyner . Florida’s recycled water footprint: a geospatial analysis of distribution (2009 and 2015). AIMS Environmental Science, 2019, 6(1): 41-58. doi: 10.3934/environsci.2019.1.41 |
[9] | Mehrbakhsh Nilashi, Rabab Ali Abumalloh, Hossein Ahmadi, Mesfer Alrizq, Hamad Abosaq, Abdullah Alghamdi, Murtaza Farooque, Syed Salman Mahmood . Using DEMATEL, clustering, and fuzzy logic for supply chain evaluation of electric vehicles: A SCOR model. AIMS Environmental Science, 2024, 11(2): 129-156. doi: 10.3934/environsci.2024008 |
[10] | Lemuel Clark Velasco, Mary Jane Burden, Marie Joy Satiniaman, Rachelle Bea Uy, Luchin Valrian Pueblos, Reynald Gimena . Preliminary assessment of solid waste in Philippine Fabrication Laboratories. AIMS Environmental Science, 2021, 8(3): 255-267. doi: 10.3934/environsci.2021017 |
The consumption and usage of polyethylene terephthalate (PET) bottles have significantly increased in recent years, necessitating action to mitigate their environmental impact. Recycling programs offer a viable solution to address this impact within the PET industry. Therefore, adopting a circular economy approach is appropriate for incorporating recycling initiatives. Nevertheless, it is crucial not to overlook the financial aspects of the supply chain. This paper proposed a bi-level model to address both the financial and environmental concerns of a circular economy supply chain. At the upper level, a recycling company (the leader) aimd to establish PET bottle collection facilities, set prices for each kilogram of PET received, and manage the transportation of collected PET to the treatment plant. At the lower level of the bi-level problem, persons (the followers) decided whether to recycle or not. They received economic incentives based on the amount deposited at the collection facilities, but this came with associated travel costs. The leader's objective was to maximize the profit of the recycling program, assuming the sale of recycled PET. Meanwhile, followers aimed to recycle if it was economically viable for them. To solve this problem, a matheuristic algorithm was proposed, which hybridized a greedy random adaptive search procedure with an exact routing process. The matheuristic managed solutions at the leader level, while followers' responses were derived by exploiting the problem's structure. A case study derived from Mexico City was solved, and practical managerial insights were provided. Sensitivity analysis revealed crucial aspects that should not be overlooked when implementing such a recycling program.
Polyethylene terephthalate PET has become the predominant packaging material for beverage bottles due to its properties against various contaminants such as liquids and gases [1]. However, the widespread use of PET beverage bottles in Mexico City alone results in over 123 tons of bottle waste daily [2]. According to the study by Vlad I M, et al.[3], the most effective solution to mitigate this waste generation is through PET bottle recycling. While PET bottle consumption is increasing globally [1], only 6% of PET products are currently recycled, representing approximately 10% of the daily waste generated in Mexico City [4]. The primary barrier to higher recycling rates is inadequate collection mechanisms [5], underscoring the critical need for improvements in collection systems.
In response to environmental and social pressures, the Mexico City government initiated a program in 2017 aimed at incentivizing residents to return recyclable waste in exchange for food vouchers [2]. Held every second Sunday of the month, participants bring waste ranging from 1 to 10 kg in different basket sizes to designated locations for exchange with agricultural goods. However, a governmental report* highlights the program's inefficiencies, citing a modest increase of around 20,000 participants by 2019. Challenges include limited collection points with poor accessibility, a once-a-month schedule, and insufficient financial incentives for participants. Recommendations from Olay-Romero E, et al. [6] emphasize improving infrastructure by increasing collection points, enhancing collection mechanisms with financial incentives, and maintaining the condition of collection vehicles for effective waste management in Mexico City. To address these challenges, a circular economy approach has been advocated, involving the complete life cycle management of products like used PET bottles. This approach encompasses collection, recycling, and repurposing to ensure PET materials are reintegrated into productive use cycles [7].
*Governmental report 2022: https://gobierno.cdmx.gob.mx/noticias/
Since the industrial revolution, the prevailing economic model has been the linear economy, characterized by the linear progression of extraction, production, consumption, and disposal of goods without recovery or reuse [8]. This approach, known for its inefficiency and environmental unsustainability, faces challenges due to finite resources and significant environmental impacts associated with waste disposal. The traditional "take-make-use-dispose" model is increasingly recognized as unsustainable in the long term. In contrast, the circular economy (CE) advocates for the reuse of biological and technological resources to extend their lifespan as much as possible [9]. CE aims to minimize waste generation by recycling and repurposing materials, thereby closing material and energy loops to increase resource productivity and decrease environmental impact [10]. By closing these loops, CE promotes upcycling, which generates new value from used materials [9]. Effective management of municipal waste, particularly PET bottle waste, is crucial for achieving CE goals. Optimizing waste collection vehicle routing plays a pivotal role in enhancing recycling processes, making them more economically viable and environmentally friendly [11,12]. This optimization not only improves resource recovery but also reduces the overall environmental footprint associated with waste management.
Despite the aim of the CE to address environmental concerns by conserving resources, enterprises often prioritize cost-effective measures to meet customer expectations rather than focusing on waste mitigation and environmental impact [13]. For many years, recycling post-consumer PET bottles into packaging for direct food contact was impractical due to concerns about polymer contamination during initial use and collection. Moreover, the effectiveness of recycling processes in removing contaminants was uncertain [1]. Consequently, recycled PET bottles were primarily used for producing polyester fibers [14]. However, traditional PET recycling markets, such as fiber production, struggled to handle the increasing volume of collected PET bottles, leading to the development of bottle-to-bottle recycling technologies [15]. These advancements in bottle-to-bottle recycling are crucial for integrating CE principles into the management of PET bottle waste in Mexico City, balancing economic and environmental targets through efficient collector vehicle routing.
The problem addressed in this study involves a recycling company seeking to establish PET bottle collection facilities. These facilities enable persons to drop off PET bottles and receive a financial incentive based on a price per kilogram set by the recycling company. Persons will decide whether to participate in this recycling program based on the payment offered. Once PET bottles are deposited at the collection facilities, the recycling company designs optimized routes to transport the bottles to a treatment plant. The recycled PET is then sold to suppliers for use in their respective processes. The objective of the recycling company is to maximize profit, considering both the operational logistics of collection and the willingness of persons to participate in the recycling program and utilize the collection facilities.
To model this problem, a bi-level optimization framework is employed, where the recycling company acts as the leader and persons act as the followers. This framework is appropriate since the recycling company determines the locations of the collection facilities and sets the prices paid to persons, who then decide whether to participate in the recycling program based on these decisions. Conversely, the decisions of persons directly impact the amount of PET collected by the recycling company and, consequently, its profit. By analyzing the structure of the followers' problem, the optimal prices paid by the recycling company can be identified, reducing the bi-level framework to a single-level problem. Nevertheless, additional decisions remain. The location of collection facilities is addressed using a greedy randomized adaptive search procedure (GRASP), while the routes are created using an exact routine in a general-purpose solver. An intensification phase is then applied to improve the current solution. These main phases are embedded in an iterative framework, resulting in a tailor-made matheuristic algorithm. A case study based on Mexico City is developed and solved. Interesting managerial insights are obtained from solving the case study and conducting a sensitivity analysis.
The main contributions of this research can be summarized as follows:
● A bi-level optimization model is proposed for this recycling program, which includes the location of collection facilities and the pricing decisions of the recycling company. Based on the participation of persons in the recycling program, routing decisions are subsequently made.
● The structure of the followers' problem is leveraged to reduce the bi-level problem to a single-level one.
● A matheuristic algorithm is developed to solve the problem, combining a greedy randomized adaptive search procedure (GRASP) with an exact routing routine.
● A case study set in Mexico City is conducted to perform computational experiments.
● Practical managerial insights are derived from the case study, and a sensitivity analysis reveals important aspects that must not be overlooked when implementing such a recycling program.
The remainder of this paper is organized as follows. Section 2 provides a literature review, focusing on circular economy supply chains, particularly those involving PET bottles. In Section 3, the problem definition and the proposed mathematical model are presented. Section 4 describes the solution methodology, detailing the reduction of the bi-level model to a single-level one and the constructive and intensification phases of the proposed matheuristic algorithm. Section 5 introduces a case study based in Mexico City, demonstrating the applicability of the proposed research. As well as, summarizes the results obtained from the computational experimentation and provides valuable managerial insights. This paper concludes with Section 6, which discusses the conclusions and potential directions for future research.
In recent decades, the CE has garnered attention due to growing environmental concerns and resource scarcity. CE represents a principle aimed at transitioning from linear to circular production and consumption patterns, emphasizing the importance of reducing the demand for natural resources to conserve virgin resources [16]. With the global increase in PET bottle consumption, community pressure, environmental damage from PET bottle waste generation, and natural resource consumption, supply chain actors are motivated to increase PET bottle recycling and reintroduce them into the use cycle [1]. a closed-loop supply chain network for PET bottle-to-bottle recycling in Mexico, aiming to achieve a zero waste goal, is studied [17]. The authors claim that the continuous cycle of consumption, recycling bottle-to-bottle, and reintroducing high-quality recycled PET bottles into the use cycle creates social, economic, and environmental benefits while reducing reliance on virgin production.
A lab experiment studying the feasibility of closed-loop PET bottle recycling (bottle-to-bottle) was conducted [18]. The results demonstrated that a mix of 75% recycled PET bottles and 25% virgin PET in each recycling cycle, with the processes of extrusion, solid-state polycondensation, hot washing, drying, and shaping into new bottles in each cycle, can create a circulation network of consumption and recycling for up to 11 cycles without quality degradation for food packaging bottles. Due to the challenge of the lab experiment, Lonca G, et al. [19] assessed the economic benefit of open-loop and closed-loop recycling PET bottles through three different methods: material flow analysis, material circularity indicator, and life cycle assessment. The study indicated that a single loop of recycling PET bottle-to-bottle might not be the most environmentally beneficial approach for the entire PET market. Instead, considering both open-loop and closed-loop PET bottle recycling with a high collection rate is crucial for achieving significant environmental benefits and optimizing PET bottle circularity.
Additionally, Benyathiar P, et al. [20] provided an overview of the process, advantages, and challenges of PET bottle-to-bottle recycling. The research indicated that closed-loop recycling brings environmental benefits by significantly reducing virgin PET consumption and fossil fuel reliance. The authors discussed challenges associated with PET bottle-to-bottle recycling, such as the need for improvements in sorting and collection infrastructure, potential color limitations of recycled PET, and the decontamination process.
In the study by Zapata Bravo A, et al [21], a PET bottle circular network in Colombia is examined. The core idea of their work is that recycling PET bottles into new products through a circular network, with proper collection mechanisms and sufficient recycling capacity, not only conserves virgin resource consumption but also addresses PET bottle waste in Colombia. In the study by Gall M, wt al. [22], PET bottle cap recycling is analyzed to design a circular network. The study found that PET bottle caps often contain a mix of plastic resins (like polyethylene and polypropylene) and various additives (like slip agents) that affect the recycling quality and difficulty. Therefore, they suggested designing bottle caps using more uniform plastic resins and minimizing the use of certain additives to improve recycling efficiency and quality. Moreover, Gracida-Alvarez U R, et al. [23] analyzed the sustainability of circular economy strategies for PET bottle production through life cycle assessment and material flow analysis in two scenarios: baseline and circular economy. The findings indicate that the circular economy scenario significantly reduces virgin PET consumption and solid waste generation. However, careful consideration of different approaches, such as combining recycling with a decarbonized electricity grid, is important to outperform the baseline scenario in terms of greenhouse gas emissions.
A simulation and examination of PET bottle waste recycling in China under various policy instruments, such as extended producer responsibility, deposit-refund systems, and government subsidies, have been addressed [24]. The results indicated that financial incentives can be the most effective approach to increase the collection rate of PET bottle waste, although they can also increase the collection cost. Additionally, Wang Y, et al. [25] explores the challenges of establishing a circular economy network for PET packaging in Sub-Saharan Africa, highlighting that consumer education, investment in recycling technology and capacity, support for both formal and informal collectors, and incentives can significantly promote a CE in this region. Moreover, Amirudin A, et al. [26] studied the impact of incentives on Indonesian residents' behavior toward environmentally friendly movements. The results demonstrate that designing a strategic network with incentives at the collection points can significantly increase the PET bottle collection rate.
In the study by Oliveira Neto G C, et al. [27], a PET bottle waste collection network was assessed to optimize vehicle routing. They developed a reverse logistics network considering factors such as collection locations, vehicle capacity, vehicle routes, and demand uncertainty. The study demonstrated how vehicle routing optimization can create an efficient collection network that minimizes travel distances and collection costs, even with fluctuating demand. A network addressing PET bottle collection routes in a recycling network is proposed [28]. The study suggests offering collection from various locations and applying timely monitoring to effectively reduce collection costs and enhance recycling. Similarly, Rekabi S, et al. [29] studied a recycling network addressing municipal waste generation. Their study considers the optimization of vehicle routes, waste collection, and schedules for recycling centers. The authors found that matching vehicle types with waste types not only improves waste collection efficiency but also reduces associated costs.
In summary, the increasing environmental impact of linear resource consumption has spurred interest in the CE, which advocates closed-loop systems such as PET bottle-to-bottle recycling, where resources are continually reused. Studies demonstrate the feasibility of high-quality, multi-cycle PET bottle-to-bottle recycling. However, the economic and environmental benefits hinge on factors like collection rates, collection points, and closed-loop recycling. Research also explores methods to optimize PET bottle collection networks through vehicle routing and incentive programs. A general scheme is presented in Figure 1, illustrating the structure of a PET bottle circular economy supply chain network. The diagram depicts the interconnected flow of materials and products among various stakeholders, including users, manufacturing processes, suppliers, collection centers, treatment plants, and disposal centers. It emphasizes the significance of recycling and reuse, wherein users return used products to collection centers. These products are then transported to treatment plants for recycling or processing, with reusable materials subsequently delivered to suppliers. Nonrecyclable waste is directed to disposal centers to ensure sustainable waste management. Suppliers provide the reusable materials to factories, which incorporate them into manufacturing processes to reintroduce these materials into the use cycle. The proposed network integrates reverse logistics management with circular economy principles to address the environmental impact of PET bottle waste generation. By enabling proper collection, recycling, and reuse, it strives to achieve zero-waste management and conserve virgin resources.
It is reasonable to assume that not all members of supply chains operating under a circular economy approach are coordinated. Hence, decentralized decision-makers are often present in these supply chains. When a hierarchy exists among them, bi-level optimization provides a suitable framework to model these problems. For instance, in the study by Ng T S A, et al. [30], a model is proposed to maximize total economic surpluses considering investments and individual benefits. The authors analyze waste-to-resource transformation in industries from a general perspective, where agents agree to forego a portion of their individual benefits to share additional capacity investment costs. They specifically apply their model to organic waste streams, yielding valuable insights for market design.
Regarding waste treatment within circular economy networks using a bi-level optimization framework, we found that in the study by Cao C, et al. [31], the focus is on COVID-19 medical waste management, incorporating enabling technologies like digital twins. Kokossis A, et al. [32] develops a bi-level mathematical model that considers waste producers, competitive technologies, and governmental authorities to optimize network welfare. Meanwhile, the authors propose a multi-objective bi-level model for optimizing bioenergy production using kitchen waste and rice straw disposal through a cooperative scheme involving governmental authorities and disposal plants [33]. Similarly, Safder U, et al. [34] presents a multilevel scheme for a chemical exergy resource recovery network, aiming to integrate a methodology for sustainability in the chemical industries. More recently, Alimohammadi M, et al. [35] proposes a bi-level approach to optimize the reverse logistics of pharmaceutical drugs, integrating the circular economy to recover raw materials and manage hazardous waste.
Consider C as the set of potential sites to locate a collection facility and D as the treatment plant. Define I=D∪C. Also, let J be the set of persons and L be the set of vehicles dedicated to collect the PET bottles from the located collection facilities and take them to the treatment plants.
There is an installation cost fi for locating a collection center at site i∈C, and the available budget for locating the collection centers is given by b. Additionally, there is a unitary cost t associated with the recycling process performed at the treatment plant, and a fixed unitary price π for selling recycled PET to a supplier, which generates a profit for the leader. Each person j∈J has an amount wj of PET available for recycling (in kilograms) and incurs a cost αij for delivering their PET to collection facility i∈C. Regarding the routing process, there is a cost βik for traveling between site i∈I and site k∈I, and each vehicle l∈L has a limited capacity ql.
Recall that under this approach, the recycling company acts as the leader, while the persons are considered followers. The leader determines the locations for placing collection facilities, sets the prices paid to persons for recycling PET bottles, and plans the routing for transporting PET bottles to the treatment plant. Therefore, the leader's decision variables include:
yi={1if a collection center is located in the potential site i∈C0otherwise |
pi≥0:unitary price paid for a kilogram of PET recycled in the collection facility i∈C |
ulik={1if the arc (i,k)∈I is travelled by the vehicle l∈L0otherwise |
vli≥0:amount collected from collection facility i∈C by the vehicle l∈L |
zl={1if the vehicle l∈L is used.0otherwise |
The followers decide whether to participate in the recycling program. Their decision variables encompass:
xij={1if the person j∈J recycles in the collection facility i∈C0otherwise |
To simplify the notation, let's define the decision variables as follows. For the leader's decision vectors consider y=(y1,…,y|C|), p=(p1,…,p|C|), and z=(z1,…,z|L|). Additionally, the leader has the decision array u of size |I|×|I|×|L|, where the value ulik is located at the (i,k,l)-th position. Similarly, define v as a matrix of size |I|×|L|, where vli is located in the i-th row and the l-th column of v. The followers' decision matrix is x, which contains the values xij in an analogous manner.
An illustration of the decisions in the studied problem is depicted in Figure 2. Some assumptions guide our study. For instance, it is assumed that the collection facilities possess sufficient capacity to accommodate the recycling program, thereby eliminating competition among persons for access to their preferred facility. This assumption stems from the residential nature of persons, suggesting typical quantities of PET bottles. Furthermore, persons are considered independent of each other, and since facility capacity constraints are disregarded, the bi-level problem can be simplified to a scenario featuring a single leader and multiple independent followers.
The proposed bi-level mixed-integer programming model results as follows:
maxy,u,p,v,z∑i∈Cπ(∑j∈Jwjxij)−[∑i∈Cfiyi+∑i∈Cpi(∑j∈Jwjxij)+t∑i∈C∑j∈Jwjxij+∑l∈L∑i∈I∑k∈Iβikulik] | (3.1) |
s.t∑i∈Cfiyi≤b | (3.2) |
−ulik≤yk−∀i∈I,−∀k∈C,−∀l∈L | (3.3) |
∑i∈I∑l∈Lulik≥yk,−∀k∈C | (3.4) |
∑i∈I∑l∈Luli0≥1 | (3.5) |
∑i∈Iulip−∑k∈Iulpk=0,..∀p∈I,−∀l∈L | (3.6) |
vli≤(∑j∈Jwjxij)∑k∈Iulik,∀i∈C,−∀l∈L | (3.7) |
∑l∈Lvli=(∑j∈Jwjxij),−.∀i∈C | (3.8) |
∑i∈Cvli≤Q,..∀l∈L | (3.9) |
∑i∈I∑k∈Iulik≤Mzl,∀i∈L | (3.10) |
∑k∈Cul0k=zl,.∀l∈L | (3.11) |
∑k∈Culi0=zl,..∀l∈L | (3.12) |
pi≤Myi,..∀i∈C | (3.13) |
∑i∈S∑k∈Sulik≤|S|−1..∀l∈L,S⊆C | (3.14) |
yi∈{0,1},.∀i∈C | (3.15) |
ulik∈{0,1},∀i∈I,−∀k∈I−∀l∈L | (3.16) |
zl∈{0,1},..∀l∈L | (3.17) |
pi≥0,−.∀i∈C | (3.18) |
vli≥0,−.∀i∈C,−∀l∈L | (3.19) |
where for a fixed (y, p, u, v, z), the variables x must be the optimal solution of the following problem:
maxx∑i∈C∑j∈J(piwj−αij)xij | (3.20) |
s.t.−xij≤yi,−.∀i∈C,∀j∈J | (3.21) |
∑i∈Cxij≤1,∀j∈J | (3.22) |
xij∈{0,1},.∀i∈C,j∈J. | (3.23) |
The leader's problem is given by Eqs (3.1)–(3.19). Equation (3.1) represents the leader's objective function, which aims to maximize the profit of the recycling program. The first term indicates the revenue obtained by selling the recycled PET to suppliers, the second term reflects the cost of locating collection facilities, the third term corresponds to the money paid to the persons based on their amount of PET recycled, the fourth term indicates the total recycling cost (in the treatment process), and the last term refers to the operational cost of collecting the PET. Equation (3.2) limits the location of collection facilities by a predefined budget. Equation (3.4) imposes that if a collection facility is located, then it must be visited to collect the PET. Equation (3.5) indicates that every vehicle must start their route from the treatment plant. Equation (3.6) is the classical flow conservation constraint. Equation (3.7) ensures that the collected PET by all the vehicles cannot exceed the total PET available in that collection facility, and Eq (3.8) links that the total amount of PET collected is equal to the available PET on that collection facility. Equation (3.9) imposes that the vehicle's capacity cannot be exceeded by the collected PET. Equation (3.10) links the use of an arc with the vehicle's usage. Eqs (3.11) and (3.12) impose that if a vehicle is used, then it must depart and return to the depot. Equation (3.13) guarantees that prices are only imposed to located collection facilities. Equation (3.14) is the sub-tour elimination constraint. Finally, Eqs (3.15)–(3.19) indicate the binary and real nature of the leader's decision variables.
On the other hand, the followers' problem is given by Eqs (3.20)–(3.23). The followers' objective function aims to maximize the profit of the persons willing to recycle. The profit of each follower can be summed up in a single objective function that encompasses the profit of all the persons, as demonstrated [36]. Therefore, the first term of Eq (3.20) indicates the revenue obtained by the persons participating in this recycling program, while the second term refers to the traveling costs from their residences to the collection facilities. Equation (3.21) ensures that the persons can recycle their PET only in located collection facilities. Equation (3.22) guarantees whether or not the users can participate in the recycling program, and Eq (3.23) indicates the binary nature of the followers' decision variables.
Note that the bi-level model defined by Eqs (3.1)–(3.23) can be ill-defined if alternative optimal solutions of the followers' problem exist for a fixed leader's decision [37]. To address this issue, we adopt the optimistic approach, which reflects cooperative behavior between the leader and the followers. In other words, since the recycling program benefits the entire population, persons will choose to recycle when they perceive no difference in profit between recycling and not recycling. While these persons may not gain any economic benefit, they are motivated by their commitment to environmental sustainability. Furthermore, although no financial gain is achieved, no loss is incurred from recycling either. In practice, this assumption is reasonable. Therefore, the optimistic approach is applied to the bi-level optimization model.
This section outlines the proposed solution methodology for addressing the studied problem. Upon analyzing the structure of the bi-level problem, it becomes evident that the leader's decision variables can be strategically segregated in the decision-making process. Specifically, the crucial variables for the followers' decisions are limited to location (y) and pricing (p). Each follower decides whether to recycle based on profitability; they will recycle if it proves economically beneficial. Once the followers' decisions (x) are determined, the leader can then optimize the routing plan (u, v, z).
Therefore, once the leader has determined the locations of the collection facilities, the pricing strategy—specifically, the price paid per kilogram of recycled PET at each facility—can be analytically bounded. This approach circumvents the need for solving the followers' problem using a general-purpose solver, while still ensuring their optimal response is achieved.
Next, the subsequent step involves designing the routes for collecting the recycled PET. Following this, the leader's objective function is evaluated, and an improvement procedure is implemented. These steps are integrated into an iterative algorithm that combines a metaheuristic approach with an exact procedure. Detailed descriptions of both methods are provided in the following sections.
A person j∈J will recycle if and only if the price paid by collection facility i∈C is convenient for them, that is, when the ratio piwj≥αij. In the case where pi≥αijwj, the person j∈J will recycle. When pi>αijwj, there is an evident economic benefit. On the other hand, when pi=αijwj, there is no economic benefit; however, based on the optimistic assumption introduced in Section 3, the person will still choose to recycle.
To bound the prices, first, we need to identify the benefit that a recycling person obtains from their selected collection facility. For each person, we compute the minimum ratio between their travel cost and the amount of PET bottles they recycle, that is, for all j∈J, compute mini∈C{αijwj}. This ratio allows us to allocate persons to the located collection facilities (x). If the ratio is large, it implies that recycling at that collection facility is very costly.
Once the persons' allocation is performed, prices are set as the maximum ratio among all persons allocated to each located collection facility. Specifically, for all i∈C, pi=maxi∈C{minj∈J{αijwj∣xij=1}}. For example, if some persons are allocated to a certain collection facility, we select the largest ratio among those persons as the price. Note that when the price is less than the maximum ratio, this would discourage at least one person from recycling.
The first step of our algorithm is to construct a partial leader's solution, namely a subset of located collection facilities (y). To achieve this, we implement a constructive procedure that balances between diversification and intensification. In other words, we follow a greedy randomized constructive approach.
The idea behind the constructive phase is detailed next. First, we order the installation costs in ascending order and select the collection facility with the lowest installation cost. Once the first collection facility has been located, the other ones are chosen according with their contribution to the leader's objective function. To perform this, we identify the lowest and highest allocation cost of the persons to the potential collection facilities. If the allocation cost is low, it is implied that persons are willing to go that collection facility to recycle. Therefore, we consider the sum of the allocation costs of all the persons to each potential collection facility (i∈C). This is measured in a greedy function G(i) defined as:
The idea behind the constructive phase is detailed next. First, we order the installation costs in ascending order and select the collection facility with the lowest installation cost. Once the first collection facility has been located, subsequent facilities are chosen based on their contribution to the leader's objective function. To determine this, we evaluate the range of allocation costs for persons to each potential collection facility i∈C. Lower allocation costs indicate greater willingness of persons to recycle at that facility. Therefore, we define a greedy function G(i) as follows:
G(i)=fiyi+∑j∈Jαijxij∀i∈C |
Considering the greedy function G(i) and a predefined threshold value γ, candidate collection facilities for location are identified and added to a restricted candidate list (RCL), which is created by value (see [40] for more details). Note that γ∈[0,1] regulates the degree of greediness during the constructive phase. For instance, with γ=0, a purely greedy approach is applied, while γ=1 results in a completely random constructive phase. The creation of the RCL is detailed next:
RLC={i∈C:G(i)≤G(i)min+γ(G(i)max−G(i)min)} |
Therefore, we randomly select a collection facility from the RCL. If the budget is not exceeded, then that collection center is located. Otherwise, another collection facility from the RCL is selected. Every time this procedure is successful, the greedy function must be updated. The procedure is repeated until it is not possible to include another collection facility in the current partial leader's solution. This can be due to the budget or the candidate collection facilities. A pseudo code of this constructive phase is shown in Algorithm 1.
Algorithm 1: Constructing partial leader's solutions |
![]() |
After locating a subset of potential collection facilities (y), the pricing scheme and the allocation of persons to these located facilities are determined as described in Section 4.1. Therefore, at this stage of the algorithm, p and x are also fixed, while decisions related to routing (u, v, z) remain to complete the solution to the problem. Routing decisions involve determining the sequence in which available vehicles will visit located collection facilities (routes), the amount of PET collected by each vehicle, and which vehicles are used. Recall that routing decisions are critical for optimizing the efficiency of the collection process within the problem's solution framework.
This can be achieved by solving the model described in Section 3 parameterized in variables ˉy, ˉx and ˉp. Define the set of located collection facilities as ˉC={i∈C:yi=1} and ˉI=I∪ˉC. The resulting model is presented next:
minu,v,z∑l∈L∑i∈ˉI∑k∈ˉIβikulik | (4.1) |
s.tulik≤¯yk−∀i∈ˉI,−∀k∈ˉC,−∀l∈L | (4.2) |
∑i∈ˉI∑l∈Lulik≥¯yk,−∀k∈ˉC | (4.3) |
∑i∈ˉI∑l∈Luli0≥1 | (4.4) |
∑i∈ˉIulip−∑k∈ˉIulpk=0,..∀p∈ˉI,−∀l∈L | (4.5) |
vli≤(∑j∈Jwj¯xij)∑k∈ˉIulik,∀i∈ˉC,−∀l∈L | (4.6) |
∑l∈Lvli=(∑j∈Jwj¯xij),−.∀i∈ˉC | (4.7) |
∑i∈ˉCvli≤Q,..∀l∈L | (4.8) |
ulik+ulki≤1,∀i∈ˉI,−∀k∈ˉI,−∀l∈L | (4.9) |
∑k∈ˉCul0k=zl,.∀l∈L | (4.10) |
∑k∈ˉCuli0=zl,..∀l∈L | (4.11) |
∑i∈S∑k∈Sulik≤|S|−1..∀l∈L,S⊆ˉC | (4.12) |
ulik∈{0,1},∀i∈ˉI,−∀k∈ˉI−∀l∈L | (4.13) |
zl∈{0,1},..∀l∈L | (4.14) |
vli≥0,−.∀i∈ˉC,−∀l∈L | (4.15) |
Notice that the formulation defined by (4.1)-(4.15) corresponds to the well-known vehicle routing problem (VRP). As indicated in constraint (4.12), sub-tours are prohibited in the routes. However, enforcing all sub-tour elimination constraints typically results in an exponential number of constraints, which is computationally challenging. To address this issue, these constraints are initially omitted, and a relaxed version of the VRP is solved. Subsequently, the solution is analyzed, and if any sub-tours are identified, the corresponding constraint is added to the relaxed model to tighten it. This iterative procedure is outlined in Algorithm 2.
Algorithm 2: Completing leader's solutions |
![]() |
Now, a complete leader's solution and the corresponding optimal followers' reactions are obtained. This leads us to a feasible solution for the bi-level problem being solved. Subsequently, the leader's objective function can be evaluated. However, at this stage, we cannot draw conclusions regarding its quality. Therefore, we implement an intensification phase known as local search to achieve a locally optimal solution.
Algorithm 3 shows a pseudo code of the local search designed to improve the solutions constructed by the procedure described above. It is important to note that the local search focuses on improving the locating variables (y). Once some collection facilities have been located, the remaining decisions are made as described in the previous sections.
First, we generate a neighborhood for the initial solution y using the well-known 2-Opt operator. This operator explores interchanges between one located and one non-located collection facility. If a solution in the neighborhood exceeds the budget, it is discarded. When a neighbor offers a better solution than the initial one in terms of the leader's objective function, it replaces the initial solution. Thus, the neighbor becomes the incumbent, and the neighborhood is updated accordingly. This exploration continues until no further improvement is achieved.
Algorithm 3: Local Search |
![]() |
As mentioned, feasible solutions of the bi-level problem, especially partial leader's solutions, are refined through a local search. An iterative framework is employed in the proposed matheuristic algorithm. Thus, the location of collection facilities is explored using a GRASP, while the routing plan is determined by an exact procedure. This approach resembles a matheuristic framework.
Matheuristics are heuristic algorithms that leverage the benefits of heuristics and exploit the problem structure using mathematical programming tools, as discussed in the study by Boschetti M A, et al. [38]. A recent review on metaheuristics for bi-level optimization problems highlights the advantages of employing matheuristic algorithms in such contexts [39].
In summary, once the collection facilities are located, prices and customer allocations are determined analytically. Subsequently, a general-purpose solver iteratively computes the routing. Finally, a local search is applied to enhance the solution. If improvements are found, the prices, allocations, and routing are updated accordingly. This approach is reminiscent of a GRASP framework integrated with an optimizer for route determination.
A pseudo code of the proposed matheuristic is shown in Algorithm 4. Note that the algorithm is divided into four phases. In the first phase, an initial solution y is constructed. The second phase identifies the pricing plan and allocation of persons to located collection facilities. Considering these decisions, the routing is defined by iteratively solving the relaxed but tight model in the third phase. Finally, the fourth phase aims to improve the feasible solution of the bi-level problem via a local search. This process is repeated until a predefined number of iterations without improvement is reached.
Algorithm 4: The Proposed matheuristic algorithm |
![]() |
To address the intensification and diversification required in a heuristic algorithm, the proposed matheuristic incorporates two parameters. The first parameter is utilized in the construction phase of the GRASP algorithm when creating the partial leader's initial solution. This parameter, γ, is used for the RCL and plays a crucial role. Extensive preliminary tests were conducted to determine the most suitable value of γ for our study. Following the recommendations in [40], we considered various values. The results of this preliminary experimentation indicate that a value of γ=0.5 achieves a balance between intensification and diversification, yielding the best solutions. In contrast, extreme values, such as a purely random approach (γ=1) or a greedy one (γ=0), performed the worst.
The second parameter is the number of iterations without improvement (MaxNoImprovement). After preliminary testing with different values, we set this value equal to 10 for our experimentation.
In this section, the case study under consideration is first detailed. Next, the best solution obtained is explained. Finally, a sensitivity analysis is conducted to measure the impact of different budget scenarios and how the pricing scheme affects our solutions.
To validate our proposed model, a case study using real data from Mexico City (CDMX) is conducted. CDMX is divided into 16 boroughs or territorial demarcations. For this case study, three boroughs are selected: Iztapalapa and Gustavo A. Madero because they have the highest population and can generate the largest amount of PET for recycling; and Coyoacán, because although it ranks fifth in population, it is one of the most representative areas due to its high concentration of cultural, tourist infrastructure, and green spaces. A total of 8,900 inhabitants, grouped into 1,927 concentration nodes, are considered, divided as follows: 842 in Azcapotzalco, 967 in Coyoacán, and 118 in Iztapalapa. The concentration nodes represent an apartment building or a house in which 4 or 5 people live.
The potential locations for collection facilities are in shopping centers and parks because they are traditional gathering points in CDMX and, consequently, attract a high flow of people. Specifically, we identify 15 sites in Azcapotzalco, 13 in Coyoacán, and 12 in Iztapalapa, totaling 40 sites. Additionally, we assume there is a single treatment plant. In Figure 3, the spatial distribution is shown: orange dots represent persons, green squares represent potential sites for the collection facilities, and the red triangle represents the treatment plant.
Five PET recycling companies in CDMX are chosen to be part of this recycling program: Petstar, Proambi, Instituto Nacional de Recicladores, Cambio Eco Colectivo, and PLASUR, since they are companies formally established. For our case study, we consider Petstar as the company responsible for installing the collection facilities. We assume that a coordinator will act as the leader of the program. The coordinator, in agreement with the companies, sets the prices to be paid per kilogram of recycled PET, which typically ranges from $5 to $6 Mexican pesos [41,42]; for this case study, we use the average price: $0.27 USD (or $5.5 Mexican pesos).
A truck can collect up to 10,000 kilograms of compacted PET. The estimated cost to transport this amount, including four containers and a protective roof [43], is $1515.5 USD (or $31,358.0 Mexican pesos). The cost of PET treatment ranges from $4.28 to $8.55 Mexican pesos [44]; for this case, the average cost of $0.30 USD (or $6.15 Mexican pesos).
To set the cost of the collection facilities, we use the average price of five different reverse vending PET machines, which is approximately $3,800 USD (or $65,000 Mexican pesos), including labor costs. We set an initial budget of $45,000 USD. This budget will be modified during the sensitivity analysis.
The computational experiments were conducted on a personal computer with an Intel(R) Core i5-10400F processor and 16 GB of RAM, running Windows 10 Professional. The matheuristic algorithm was implemented in Python 3.9, utilizing Gurobi 11.0 to solve the model presented in Section 4.3.
As explained, we solve the case study using the proposed matheuristic algorithm and obtain numerical results. Additionally, to graphically illustrate the obtained solution, we use the open-source software QGIS to plot the located collection facilities, the allocation of persons, and the routes designed for picking up the collected PET. Figure 4 shows the best solution found for the case study.
To interpret the obtained solution shown in Figure 4, note that the black triangle represents the treatment plant (Petstar company), the colored squares represent the located collection facilities, and the colored dots represent persons. In the best solution obtained, 11 collection facilities are located: two in Azcapotzalco (red), four in Coyoacán (blue and pink), and five in Iztapalapa (green and orange). Persons and collection facilities with the same color denote their allocation, meaning that persons of one color are allocated to their closest collection facility of the same color. Moreover, colored lines represent the routes used by the trucks to pick up PET from the located collection facilities (one route per color). Notice that some routes include multiple collection facilities, but there are single trips to specific collection facilities. This is due to the capacity of the trucks. Additionally, different routes may travel to the same collection facility, caused by the large amount of collected PET, necessitating another truck to visit that facility to pick up the remaining PET. Another important remark is that more collection facilities are needed in the area of Iztapalapa since it is more populated, while fewer collection facilities are needed in zones like Azcapotzalco since it is less crowded.
Finally, for this case study, it is important to highlight that all 1,927 persons are willing to recycle. The total profit of the recycling program, based on the best obtained solution, is $429,703 Mexican pesos (approximately $25,000 USD).
The first part of the sensitivity analysis assesses the impact of both reducing and increasing the budget for locating collection facilities on the obtained solution. Recall that the budget in the case study is $45,000 USD.
Reducing the budget: Considering a budget of $40,000 USD and solving the problem yields the solution depicted in Figure 5. In this case, 10 collection facilities are located: two in Azcapotzalco, three in Coyoacán, and five in Iztapalapa—one less than in the base case study (see pink color). Notice that Coyoacán now has only three collection facilities, and some persons in that region are allocated to Iztapalapa (in pink and orange). This adjustment results in a profit of almost half a million Mexican pesos ($484,193.13), primarily due to the savings from locating one fewer collection facility.
However, this finding should not be misleading; reducing the number of collection facilities is not always straightforward. For example, if only collection facilities are located in Azcapotzalco and you aim to serve all persons willing to recycle, the fixed price might become too high for those in Coyoacán and Iztapalapa. Consequently, persons in Azcapotzalco could receive excessive benefits due to their proximity and high prices, potentially leading to profit losses for the leader.
Increasing the budget: Now, considering a budget of $50,000 USD and applying the matheuristic algorithm, the best obtained solution is shown in Figure 6. In this case, two additional collection facilities are located -one in Azcapotzalco and another in Iztapalapa- bringing the total to 13 collection facilities. It is important to note the impact on the allocation of persons when an extra collection facility is added in Iztapalapa. Persons between Coyoacán and Iztapalapa are reallocated (see orange color).
This scenario results in a profit of $291,013 Mexican pesos, which is significantly lower than in the other two cases. This reduction is attributed to the higher costs of locating additional collection facilities. However, considering this trade-off between the number of collection facilities and the allocation of persons, a larger community participation in the recycling program is expected. Closer collection facilities, paired with appropriate pricing, may encourage more persons to recycle, leading to a greater positive impact on the environment.
The last findings prompted us to conduct the second part of this sensitivity analysis. Given the current pricing method, we decided to explore an alternative approach. Instead of setting the price based on the maximum traveling cost for persons allocated to a specific collection facility, we analyze the impact of setting the prices at 90%, 80%, and 70% of the maximum cost at each facility. It is expected that under this new pricing scheme, not all persons will participate in the recycling program. However, this approach may increase the leader's profit by reducing other related costs.
Note that the base case study is used, with $45,000 USD assumed as the available budget. The solutions obtained from this analysis are shown in Figures 7, 8 and 9.
Notice how these reduced pricing schemes lead to decreased participation. Out of the 1,927 persons considered in the case study, only 1,879, 1,807, and 1,680 persons are recycling under the 90%, 80%, and 70% of the maximum costs pricing schemes, respectively. This represents a decrease in participation of 2.5%, 6.22%, and 12.81%, respectively. In terms of uncollected PET bottles, this corresponds to 1.02, 2.6, and 5.4 tons of wasted PET, which significantly impacts the environment. Nonetheless, this reduction in participation is expected when adopting these pricing schemes.
From an economic perspective, the reduction in recycling participation negatively impacts the profit of the recycling company, despite the savings from the reduced pricing scheme. With the pricing scheme set at 90% of the maximum allocation traveling cost, the profit is $394,454 Mexican pesos. Similarly, for the 80% and 70% pricing schemes, the profits are $354,529.09 and $282,045.83 Mexican pesos, respectively. Notably, when the pricing is set at 70%, the profit decreases by nearly half compared to the base case study. In other words, excluding those 247 persons from the recycling program has a significant negative impact. We anticipated that adjusting the prices paid to recyclers would increase the leader's profit, but this was not the case.
Additionally, it is important to emphasize that the persons who are not recycling are located on the outskirts of Azcapotzalco, Coyoacán, and Iztapalapa. This indicates a clear segregation based on geographic location. Social equity should be a critical consideration when implementing recycling programs such as the one addressed in this study. Therefore, easy access to a recycling program is a crucial factor in motivating persons to return PET bottle waste to collection facilities. Improving the infrastructure to provide more convenient accessibility ensures that all segments of the population are informed and engaged. Moreover, it is essential that the pricing policy strikes a balance between the amount the recycling company is willing to pay to persons without significantly compromising its profit, while still maintaining motivation for participation in recycling. Government incentives for recycling programs and social initiatives to promote recycling culture are reasonable options to address these issues.
In this paper, we investigated the impact of incorporating recycling initiatives within a circular economy supply chain. Specifically, we focused on a problem where the managers of a treatment plant aim to locate collection facilities to collect PET from residential individuals. To encourage recycling, a price is set for each kilogram of PET deposited at the collection facility. Additionally, a routing plan must be developed to pick up the collected PET and transport it to the treatment plant. Each persons' decision to recycle is influenced by the price per kilogram offered at the collection facility and the associated traveling costs.
To address this problem, we proposed a bi-level mathematical formulation in which the leader is the manager of the treatment plant, and the followers are the persons. Leveraging the structure of the lower level, we developed an approach to avoid explicitly solving for the rational response. Instead, we used an analytical method to identify the pricing scheme and determine which persons will participate in recycling. Due to the complexity of the bi-level model, we proposed a matheuristic algorithm based on a greedy random adaptive search procedure, incorporating a general-purpose solver for determining the routing.
A case study from Mexico City was generated and solved. The results demonstrated the importance of balancing the number of collection facilities with the number of persons who will recycle. While it is important to consider the economic aspect, as the treatment plant is a private company, the environmental aspect must not be overlooked. Setting the pricing scheme appropriately to encourage persons to recycle is a crucial aspect of this research.
Some assumptions considered for the model and the case study are summarized below. Regarding the model, the waste-to-resource transformation is approached from a general perspective. In this problem, it is assumed that the decision to recycle is based purely on economic factors. Costs are considered deterministic, such as those related to locating collection facilities and the payments made to persons who recycle PET. It is also assumed that collection facilities have unlimited capacity. For the case study, only three districts of Mexico City were considered, selected because they are the most populated and, therefore, assumed to generate the highest amount of PET. Social equity aspects were not taken into account; in fact, the results indicate that recycling does not occur in the more distant areas of these districts due to the distance.
For future research, we may consider capacity constraints at the collection facilities. While our current focus was on improving the leader's profit and promoting recycling through economic incentives, this assumption led to universal participation in the case study. However, this may not always be the case. Exploring different pricing schemes could be an interesting research direction, aiming to reduce costs and increase profit. So far, only a sensitivity analysis with different payment schemes has been conducted to evaluate their impact on profits. It could also be explored to treat costs as nondeterministic and not limit them to a fixed budget. Additionally, social equity could be further advanced by analyzing participation rates in recycling programs based on differences in location, socioeconomic status, and disparities in recycling infrastructure. It may also be quite revealing to examine the role that focused education and population awareness play in promoting proper recycling.
A more systemic approach should consider multicommodity recycling to manage waste, encompassing the collection, processing, and recycling of multiple types of materials simultaneously within an integrated system. This approach should evaluate whether materials can be reused for their original purpose (e.g., PET bottles into PET bottles) or transformed into secondary products. Also, other disposable items like glass, paper, and cardboard may involve different assumptions and constraints. For example, organic waste cannot remain uncollected for many days due to decomposition, odor, and the risk of spreading diseases. Addressing these variations will provide a more comprehensive understanding of recycling initiatives within a circular economy supply chain.
The authors declare they have not used Artificial Intelligence (AI) tools in the creation of this article.
The corresponding author acknowledges financial support by grant RED2022-134149-T funded by MICIU/AEI/10.13039/501100011033 (Thematic Network on Location Science and Related Problems).
The authors have no relevant financial or non-financial interests to disclose.
[1] |
Welle F (2011) Twenty years of pet bottle to bottle recycling—an overview. Resour Conserv Recycl 55: 865–875. https://doi.org/10.1016/j.resconrec.2011.04.009 doi: 10.1016/j.resconrec.2011.04.009
![]() |
[2] |
Kutralam-Muniasamy G, Shruti V C, Pérez-Guevara F (2023) Citizen involvement in reducing end-of-life product waste in mexico city. Sustain Prod Consump 41: 167–178. https://doi.org/10.1016/j.spc.2023.08.010 doi: 10.1016/j.spc.2023.08.010
![]() |
[3] | Vlad I M, Toma E (2022) Overview on the key figures with impact on the circular economy through the life cycle of plastics. Mater Plast 59. https://doi.org/10.37358/MP.22.2.5594 |
[4] | Hotspot H C (2021) Waste management in the latam region: business opportunities for the netherlands in waste/circular economy sector in eight countries of latin america. The Netherlands Enterprise Agency (RVO), The Hague. |
[5] |
Gutierrez Galicia F, Coria Paez A L, Tejeida Padilla R (2019) A study and factor identification of municipal solid waste management in mexico city. Sustainability 11: 6305. https://doi.org/10.3390/su11226305 doi: 10.3390/su11226305
![]() |
[6] |
Olay-Romero E, Turcott-Cervantes D E, del Consuelo Hernández-Berriel M, et al. (2020) Technical indicators to improve municipal solid waste management in developing countries: A case in mexico. Waste Manage 107: 201–210. https://doi.org/10.1016/j.wasman.2020.03.039 doi: 10.1016/j.wasman.2020.03.039
![]() |
[7] | Esposito M, Tse T, Soufani K (2017) Is the circular economy a new fast-expanding market? Thunderbird Int Bus Rev 59: 9–14. https://doi.org/10.1002/tie.21764 |
[8] |
Abad-Segura E, Fuente A B, González-Zamar M D, et al. (2020) Effects of circular economy policies on the environment and sustainable growth: Worldwide research. Sustainability 12: 5792. https://doi.org/10.3390/su12145792 doi: 10.3390/su12145792
![]() |
[9] |
Gallego-Schmid A, Chen H M, Sharmina M, et al. (2020) Links between circular economy and climate change mitigation in the built environment. J Clean Prod 260: 121115. https://doi.org/10.1016/j.jclepro.2020.121115 doi: 10.1016/j.jclepro.2020.121115
![]() |
[10] |
Georgescu I, Kinnunen J, Androniceanu A M (2022) Empirical evidence on circular economy and economic development in europe: a panel approach. J Bus Econ Manag 23: 199–217. https://doi.org/10.3846/jbem.2022.16050 doi: 10.3846/jbem.2022.16050
![]() |
[11] |
Bing X, de Keizer M, Bloemhof-Ruwaard J M, et al.(2014) Vehicle routing for the eco-efficient collection of household plastic waste. Waste manage 34: 719–729. https://doi.org/10.1016/j.wasman.2014.01.018 doi: 10.1016/j.wasman.2014.01.018
![]() |
[12] |
Marampoutis I, Vinot M, Trilling L (2022) Multi-objective vehicle routing problem with flexible scheduling for the collection of refillable glass bottles: A case study. EURO J Decis Process 10: 100011. https://doi.org/10.1016/j.ejdp.2021.100011 doi: 10.1016/j.ejdp.2021.100011
![]() |
[13] | McDonough W, Braungart M (2010) Cradle to cradle: Remaking the way we make things. North point press. |
[14] |
Shen L, Worrell E, Patel M K (2010) Open-loop recycling: A lca case study of pet bottle-to-fibre recycling. Resour Conserv Recycl 55: 34–52. https://doi.org/10.1016/j.resconrec.2010.06.014 doi: 10.1016/j.resconrec.2010.06.014
![]() |
[15] |
Stallkamp C, Hennig M, Volk R, et al. (2024) Pyrolysis of mixed engineering plastics: Economic challenges for automotive plastic waste. Waste Manage 176: 105–116. https://doi.org/10.1016/j.wasman.2024.01.035 doi: 10.1016/j.wasman.2024.01.035
![]() |
[16] |
van Langen S K, Passaro R (2021) The dutch green deals policy and its applicability to circular economy policies. Sustainability 13: 11683. https://doi.org/10.3390/su132111683 doi: 10.3390/su132111683
![]() |
[17] | Cámara-Creixell J, Scheel-Mayenberger C (2019) Petstar pet bottle-to-bottle recycling system, a zero-waste circular economy business model. Towards Zero Waste Circular Economy Boost Waste Resour 191–213. |
[18] |
Pinter E, Welle F, Mayrhofer E, et al. (2021) Circularity study on pet bottle-to-bottle recycling. Sustainability 13: 7370. https://doi.org/10.3390/su13137370 doi: 10.3390/su13137370
![]() |
[19] |
Lonca G, Lesage P, Majeau-Bettez G, et al. (2020) Assessing scaling effects of circular economy strategies: A case study on plastic bottle closed-loop recycling in the usa pet market. Resour Conserv Recycl 162: 105013. https://doi.org/10.1016/j.resconrec.2020.105013 doi: 10.1016/j.resconrec.2020.105013
![]() |
[20] |
Benyathiar P, Kumar P, Carpenter G, et al. (2022) Polyethylene terephthalate (pet) bottle-to-bottle recycling for the beverage industry: a review. Polymers 14: 2366. https://doi.org/10.3390/polym14122366 doi: 10.3390/polym14122366
![]() |
[21] | Zapata Bravo Á, Vieira Escobar V, Zapata-Domínguez Á, et al. (2021) The circular economy of pet bottles in colombia. Cuadernos de Administración (Universidad del Valle) 37. https://doi.org/10.25100/cdea.v37i70.10912 |
[22] |
Gall M, Schweighuber A, Buchberger W, et al. (2020) Plastic bottle cap recycling—characterization of recyclate composition and opportunities for design for circularity. Sustainability 12: 10378. https://doi.org/10.3390/su122410378 doi: 10.3390/su122410378
![]() |
[23] |
Gracida-Alvarez U R, Xu H, Benavides P T, et al. (2023) Circular economy sustainability analysis framework for plastics: application for poly (ethylene terephthalate)(pet). ACS Sustainable Chemistry & Engineering 11: 514–524. https://doi.org/10.1021/acssuschemeng.2c04626 doi: 10.1021/acssuschemeng.2c04626
![]() |
[24] |
Wang Y, Gu Y, Wu Y, et al. (2020) Performance simulation and policy optimization of waste polyethylene terephthalate bottle recycling system in china. Resour Conserv Recycl 162: 105014. https://doi.org/10.1016/j.resconrec.2020.105014 doi: 10.1016/j.resconrec.2020.105014
![]() |
[25] |
Ayeleru O O, Dlova S, Akinribide O J, et al. (2020) Challenges of plastic waste generation and management in sub-saharan africa: A review. Waste Manage 110: 24–42. https://doi.org/10.1016/j.wasman.2020.04.017 doi: 10.1016/j.wasman.2020.04.017
![]() |
[26] |
Amirudin A, Inoue C, Grause G (2023) Assessment of factors influencing indonesian residents’ intention to use a deposit–refund scheme for pet bottle waste. Circular Economy 2: 100061. https://doi.org/10.1016/j.cec.2023.100061 doi: 10.1016/j.cec.2023.100061
![]() |
[27] |
Oliveira Neto G C, de Araujo S A, Gomes R A, et al. (2023) Simulation of electronic waste reverse chains for the sao paulo circular economy: An artificial intelligence-based approach for economic and environmental optimizations. Sensors 23: 9046. https://doi.org/10.3390/s23229046 doi: 10.3390/s23229046
![]() |
[28] |
Rahmanifar G, Mohammadi M, Sherafat A, et al. (2023) Heuristic approaches to address vehicle routing problem in the iot-based waste management system. Expert Syst Appl 220: 119708. https://doi.org/10.1016/j.eswa.2023.119708 doi: 10.1016/j.eswa.2023.119708
![]() |
[29] | Rekabi S, Sazvar Z, Tavakkoli-Moghaddam R (2022) A green vehicle routing problem in the solid waste network design with vehicle and technology compatibility. Comput Sci Eng 2: 299–309. |
[30] |
Ng T S A, Mah A X Y, Zhao K (2024) Towards a circular economy with waste-to-resource system optimization. Nav Res Logist 71: 553–574. https://doi.org/10.1002/nav.22163 doi: 10.1002/nav.22163
![]() |
[31] |
Cao C, Liu J, Liu Y, et al. (2023) Digital twin-driven robust bi-level optimisation model for covid-19 medical waste location-transport under circular economy. Comput Ind Eng 186: 109107. https://doi.org/10.1016/j.cie.2023.109107 doi: 10.1016/j.cie.2023.109107
![]() |
[32] | Kokossis A, Melampianakis E (2022) A game-theoretical approach for the analysis of waste treatment and circular economy networks. In Comput Aided Chem Eng 51: 1657–1662. https://doi.org/10.1016/B978-0-323-95879-0.50277-0 |
[33] |
Huang Y, Xu J (2022) Bi-level multi-objective programming approach for bioenergy production optimization towards co-digestion of kitchen waste and rice straw. Fuel 316: 123117. https://doi.org/10.1016/j.fuel.2021.123117 doi: 10.1016/j.fuel.2021.123117
![]() |
[34] |
Safder U, Tariq S, Yoo C K (2022) Multilevel optimization framework to support self-sustainability of industrial processes for energy/material recovery using circular integration concept. Appl Energy 324: 119685. https://doi.org/10.1016/j.apenergy.2022.119685 doi: 10.1016/j.apenergy.2022.119685
![]() |
[35] | Alimohammadi M, Behnamian J (2024) Supporting circular economy through using digital transformation in sustainable pharmaceutical reverse logistics: Multi-objective bi-level modeling. Process Integr Optim Sustain 1–26. https://doi.org/10.1007/s41660-024-00460-0 |
[36] |
Calvete H I, Gal C (2007) Linear bilevel multi-follower programming with independent followers. J Glob Optim 39: 409–417. https://doi.org/10.1007/s10898-007-9144-2 doi: 10.1007/s10898-007-9144-2
![]() |
[37] | Dempe S, Zemkoho A (2020) Bilevel optimization. In Springer optimization and its applications 161. Springer. https://doi.org/10.1007/978-3-030-52119-6 |
[38] |
Boschetti M A, Maniezzo V (2022) Matheuristics: using mathematics for heuristic design. 4OR 20: 173–208. https://doi.org/10.1007/s10288-022-00510-8 doi: 10.1007/s10288-022-00510-8
![]() |
[39] | Camacho-Vallejo J F, Corpus C, Villegas J G (2023) Metaheuristics for bilevel optimization: A comprehensive review. Comput Oper Res 106410. https://doi.org/10.1016/j.cor.2023.106410 |
[40] | Resende M G C, Ribeiro C C (2016) Optimization by GRASP. Springer. https://doi.org/10.1007/978-1-4939-6530-4 |
[41] | Zaleta J (2024) ¿cuánto dinero puedes ganar reciclando? esto es lo que vale el kilo de pet en méxico? https://heraldodemexico.com.mx/estilo-de-vida/2024/2/5/cuanto-dinero-puedes-ganar-reciclando-esto-es-lo-que-vale-el-kilo-de-pet-en-mexico-575356.html, 2024. Accessed: 2024-07-15. |
[42] | Álvarez D (2024) Costo de materiales reciclables a la baja. https://www.diariodequeretaro.com.mx/finanzas/costo-de-materiales-reciclables-a-la-baja-10186587.html#:~:text=El%20precio%20com%C3%BAn%20para%20el,y%20de%20segunda%20en%20100., 2024. Accessed: 2024-07-15. |
[43] | ULINE. Contenedor plegable para carga a granel - 32 x 30 x 34", capacidad de 1,500 lb. https://es.uline.mx/Product/Detail/H-4051/Bulk-Containers/Collapsible-Bulk-Container-32-x-30-x-34-1500-Capacity?pricode=WB7091&gadtype=pla&id=H-4051&gad_source=1&gclid=Cj0KCQjw7ZO0BhDYARIsAFttkCinP4LOAdO0G_KuTh4Xn_gXz5KsloY0fRvemZJlN0svbQeK8OorZQIaAoAZEALw_wcB, 2024. Accessed: 2024-07-15. |
[44] | Educa verde. https://educa-verde.ecoce.mx, 2024. Accessed: 2024-07-15. |