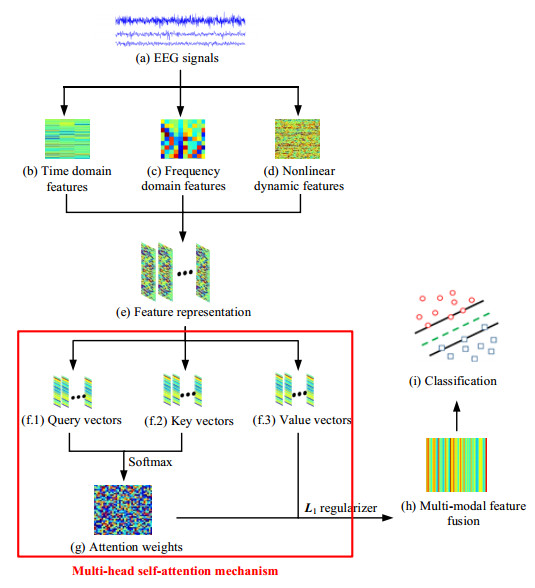
It is important to classify electroencephalography (EEG) signals automatically for the diagnosis and treatment of epilepsy. Currently, the dominant single-modal feature extraction methods cannot cover the information of different modalities, resulting in poor classification performance of existing methods, especially the multi-classification problem. We proposed a multi-modal feature fusion (MMFF) method for epileptic EEG signals. First, the time domain features were extracted by kernel principal component analysis, the frequency domain features were extracted by short-time Fourier extracted transform, and the nonlinear dynamic features were extracted by calculating sample entropy. On this basis, the features of these three modalities were interactively learned through the multi-head self-attention mechanism, and the attention weights were trained simultaneously. The fused features were obtained by combining the value vectors of feature representations, while the time, frequency, and nonlinear dynamics information were retained to screen out more representative epileptic features and improve the accuracy of feature extraction. Finally, the feature fusion method was applied to epileptic EEG signal classifications. The experimental results demonstrated that the proposed method achieves a classification accuracy of 92.76 ± 1.64% across the five-category classification task for epileptic EEG signals. The multi-head self-attention mechanism promotes the fusion of multi-modal features and offers an efficient and novel approach for diagnosing and treating epilepsy.
Citation: Ning Huang, Zhengtao Xi, Yingying Jiao, Yudong Zhang, Zhuqing Jiao, Xiaona Li. Multi-modal feature fusion with multi-head self-attention for epileptic EEG signals[J]. Mathematical Biosciences and Engineering, 2024, 21(8): 6918-6935. doi: 10.3934/mbe.2024304
[1] | Xiaowen Jia, Jingxia Chen, Kexin Liu, Qian Wang, Jialing He . Multimodal depression detection based on an attention graph convolution and transformer. Mathematical Biosciences and Engineering, 2025, 22(3): 652-676. doi: 10.3934/mbe.2025024 |
[2] | Jiacan Xu, Donglin Li, Peng Zhou, Chunsheng Li, Zinan Wang, Shenghao Tong . A multi-band centroid contrastive reconstruction fusion network for motor imagery electroencephalogram signal decoding. Mathematical Biosciences and Engineering, 2023, 20(12): 20624-20647. doi: 10.3934/mbe.2023912 |
[3] | Songlin Liu, Shouming Zhang, Zijian Diao, Zhenbin Fang, Zeyu Jiao, Zhenyu Zhong . Pedestrian re-identification based on attention mechanism and Multi-scale feature fusion. Mathematical Biosciences and Engineering, 2023, 20(9): 16913-16938. doi: 10.3934/mbe.2023754 |
[4] | Shi Liu, Kaiyang Li, Yaoying Wang, Tianyou Zhu, Jiwei Li, Zhenyu Chen . Knowledge graph embedding by fusing multimodal content via cross-modal learning. Mathematical Biosciences and Engineering, 2023, 20(8): 14180-14200. doi: 10.3934/mbe.2023634 |
[5] | Aigou Li, Chen Yang . AGMG-Net: Leveraging multiscale and fine-grained features for improved cargo recognition. Mathematical Biosciences and Engineering, 2023, 20(9): 16744-16761. doi: 10.3934/mbe.2023746 |
[6] | Xiaotong Ji, Dan Liu, Ping Xiong . Multi-model fusion short-term power load forecasting based on improved WOA optimization. Mathematical Biosciences and Engineering, 2022, 19(12): 13399-13420. doi: 10.3934/mbe.2022627 |
[7] | Jiaming Ding, Peigang Jiao, Kangning Li, Weibo Du . Road surface crack detection based on improved YOLOv5s. Mathematical Biosciences and Engineering, 2024, 21(3): 4269-4285. doi: 10.3934/mbe.2024188 |
[8] | Pingping Yang, Jiachen Ma, Yong Liu, Meng Liu . Multi-modal transformer for fake news detection. Mathematical Biosciences and Engineering, 2023, 20(8): 14699-14717. doi: 10.3934/mbe.2023657 |
[9] | Chaofan Li, Kai Ma . Entity recognition of Chinese medical text based on multi-head self-attention combined with BILSTM-CRF. Mathematical Biosciences and Engineering, 2022, 19(3): 2206-2218. doi: 10.3934/mbe.2022103 |
[10] | Chaofan Song, Tongqiang Liu, Huan Wang, Haifeng Shi, Zhuqing Jiao . Multi-modal feature selection with self-expression topological manifold for end-stage renal disease associated with mild cognitive impairment. Mathematical Biosciences and Engineering, 2023, 20(8): 14827-14845. doi: 10.3934/mbe.2023664 |
It is important to classify electroencephalography (EEG) signals automatically for the diagnosis and treatment of epilepsy. Currently, the dominant single-modal feature extraction methods cannot cover the information of different modalities, resulting in poor classification performance of existing methods, especially the multi-classification problem. We proposed a multi-modal feature fusion (MMFF) method for epileptic EEG signals. First, the time domain features were extracted by kernel principal component analysis, the frequency domain features were extracted by short-time Fourier extracted transform, and the nonlinear dynamic features were extracted by calculating sample entropy. On this basis, the features of these three modalities were interactively learned through the multi-head self-attention mechanism, and the attention weights were trained simultaneously. The fused features were obtained by combining the value vectors of feature representations, while the time, frequency, and nonlinear dynamics information were retained to screen out more representative epileptic features and improve the accuracy of feature extraction. Finally, the feature fusion method was applied to epileptic EEG signal classifications. The experimental results demonstrated that the proposed method achieves a classification accuracy of 92.76 ± 1.64% across the five-category classification task for epileptic EEG signals. The multi-head self-attention mechanism promotes the fusion of multi-modal features and offers an efficient and novel approach for diagnosing and treating epilepsy.
Epilepsy is a chronic brain dysfunction disease caused by a variety of causes, which is typically characterized by repeated and sudden excessive discharge of local neurons in the brain, resulting in central nervous system dysfunction [1]. Patients with epilepsy clinically exhibit symptoms such as muscle convulsions and loss of consciousness. Repeated seizures result in brain cell death, impairment of brain function, and life-threatening situations in severe cases. Furthermore, epilepsy imposes a significant burden on the patients' families and society. EEG plays an irreplaceable role in the diagnosis and treatment of epilepsy [2]. It has served as a crucial tool for clinical monitoring and diagnosis of epilepsy, offering a swift, reliable, cost-effective, and non-invasive technique to observe cerebral cortex brain activity [3]. Therefore, it is of great significance to study the prevention and treatment of epilepsy with EEG.
Traditional feature extraction for EEG signals was inefficient due to the differences in the subjective experience of experts. Consequently, the automatic detection of epileptic EEG signals is one of the hot issues in biomedical research [4]. Boashash et al. [5] classified and recognized neonatal epileptic EEG signals by comprehensively analyzing the statistical, image, and signal features in the time-frequency domain. On the other hand, nonlinear dynamic analysis methods mostly included signal sample entropy (SEn), approximate entropy, information entropy, and Lempel-Ziv complexity metric analysis [6], which are often combined with time-frequency domain features in classification. Sabeti et al. [7] classified epileptic EEG signals from patients and normal subjects by feature vectors comprising SEn, approximate entropy, and Lempel-Ziv complexity. The method of automatic detection of epilepsy not only helps doctors to improve the accuracy of epilepsy diagnosis but also greatly saves the diagnosis time, which was of great significance for the prevention, diagnosis, and treatment of epilepsy.
In recent years, machine learning has become popular with advances in computing and has been widely used in epilepsy recognition [8]. Researchers have tried to diagnose EEG signal features by machine learning. Extracting relevant features from the signals is the key to successfully diagnosing epilepsy. Whether it is manual extraction of a single-modal feature, manual extraction of multiple features, or automatic extraction of features by deep learning, their common purpose is to obtain more information about EEG signals [9], and the richness of such information is very important for in-depth understanding and analysis of EEG signals. Sharmila et al. [10] converted EEG signals into spectral images, but the experimental results were not satisfactory. The main reason was that a single convolutional neural network model extracts only the frequency domain and spatial domain features of EEG signals, ignoring the time domain features of EEG signals, resulting in poor classification performance. Brain activity is a time-dynamic process, and learning temporal evolution from EEG time series is important. Seal et al. [11] presented a deep mixing model with a convolutional neural network and a bidirectional short-duration memory network to extract frequency information and sequence relationships from signals. The proposed model, integrating convolutional neural networks and bidirectional short-term memory networks, efficiently extracts frequency information and sequence relationships from signals. However, when dealing with one-dimensional time series, its final classification accuracy (ACC) is unsatisfactory, and it may face challenges such as high computational complexity and overfitting. This model inputs only a one-dimensional chain time series into the classifier, and the final ACC is unsatisfactory.
The self-attention mechanism weighs input data parts, emphasizing those most influential on the output. This approach assigns higher weights to key inputs, amplifying their impact within the network [12]. Today, self-attention is a pivotal concept in deep learning and is widely applied in EEG signal classification [13]. Zheng et al. [14] introduced an attention-based bidirectional short-term memory network for visual classification. It utilizes self-attention to identify critical EEG segments, thereby enhancing visual processing accuracy. It identifies critical EEG segments via self-attention to enhance a visual ACC. However, it may require extensive training data and resources and faces risks of overfitting and longer training time. Zhang et al. [15] simplified the temporal scale by one-dimensional convolution. They combined a bidirectional long short-term memory network with self-attention for arousal-based binary classification, resulting in reduced complexity and improved performance. However, the method relies on sufficient labeled data for effective training. Kim et al. [16] combined a long short-term memory network with self-attention to analyze EEG signals and conduct binary classification based on titer and arousal, achieving a good ACC. However, the method may demand substantial labeled data and has a relatively high computational cost. Chang et al. [17] employed 3D convolutional neural networks to extract deep EEG features and leveraged dual attention to enhance them, resulting in improved performance. However, the approach comes with higher computational costs and data requirements. Traditional methods extract single-modal features without preserving the structure of EEG signals in the dimensions, time, frequency, and nonlinear kinematics and do not reach the ideal state in multiple classification tasks.
To address the aforementioned challenges, we proposed a multi-modal feature fusion (MMFF) method leveraging a multi-head self-attention mechanism to extract a time domain, frequency domain, and nonlinear dynamic features from epileptic EEG signals. Specifically, the obtained resting EEG signals were preprocessed to extract time series. Second, the time domain features of EEG were extracted by Gaussian kernel principal component analysis (GPCA), while the frequency domain features were extracted by short-time Fourier transform (STFT). Additionally, the nonlinear dynamic features were extracted by SEn. Then, the features of the three modalities were interactively learned by the multi-head self-attention mechanism, and the attention weights were trained. The fused features were derived by amalgamating the value vectors of feature representations, which transform an optimal model and introduce an L1 norm regularization term.
The major contributions of this study are summarized as follows:
(a) The multi-head self-attention mechanism fused the time domain, frequency domain, and nonlinear dynamic features derived from epileptic EEG signals. Additionally, the introduction of the L1 norm regularization term served to decrease model complexity, bolster robustness, and mitigate the risk of overfitting.
(b) We explored the variation trends of several parameters in different GPCA, including the bandwidth of the Gaussian kernel function, STFT window length and step size, SEn window length, overlap rate, threshold, and the number of multi-head attention mechanisms.
(c) We examined how these parameters, along with L1 norm regularization, affected the classification performance of epilepsy patients. Through this comprehensive investigation, an optimal parameter combination was identified, achieving an ACC of 92.76 ± 1.64%.
Figure 1 shows the framework diagram. The specific steps are as follows: (a) Preprocess resting epileptic EEG signals to extract time series data; (b) Extract the time domain features of EEG by GPCA and then calculate their self-attention scores and generate the corresponding output; (c) Extract the frequency domain features of EEG by STFT, calculate their self-attention scores, and produce the associated output; (d) Extract the nonlinear dynamic features of EEG by Sen and then calculate their self-attention scores and generate the corresponding output; (e) Derive the feature representation by fusing the self-attention outputs from the three modal features; (f) Obtain the query vector, key vector, and value vector through a linear transformation to the feature representation; (g) Determine the normalized attention weight by scaling the dot product of the query and key vectors, followed by Softmax function; (h) Generate fused features by combining the value vectors of feature representations and then transform them into optimization models with the introduction of L1 norm regularization terms; and (i) Diagnose epilepsy by the fused features, enabling assessment of classification performance.
The experimental data was sourced from a widely used epileptic EEG dataset provided by the University of Bonn, Germany (http://epileptologie-bonn.de/cms/upload/workgroup/lehnertz/eegdata.html). The dataset was divided into five subsets from Set A to Set E, with each subset containing 100 samples of the same type and each sample containing 4096 EEG time series. The data sampling frequency was 173.61 Hz, and the duration was 23.6 s. The artifacts had been removed by manual filtering. Set A and Set B were collected from 5 healthy subjects with open and closed eyes, respectively. The Set C and Set D were collected outside the focal area and in the focal area of 5 patients, respectively. Set E was collected from focal areas during the seizure. The Set C, Set D, and Set E subsets were recorded by tape electrodes implanted in the skull and affixed to the surface of the hippocampus. Among them, Set E and Set D were recorded by attaching a strip electrode to the lesion area of the hippocampus structure, and Set C was recorded by attaching a strip electrode to the hippocampus structure of the other brain hemisphere outside the lesion area. Table 1 shows the details.
Data subset | A | B | C | D | E |
Subject | Five healthy volunteers | Five patients with epilepsy | |||
Subject status | Open-eyed | Close-eyed | Interparoxysmal | Paroxysmal | |
Recording period | Normal | Epileptic interval | Epileptic phase | ||
Electrode type | Non-invasive surface | Intracranial | |||
Electrode arrangement | International 10-20 standard system | Hippocampus structure | Lesion | All seizures |
The features of EEG signals are mainly derived from time domain, frequency domain, time-frequency domain, and nonlinear dynamics [18,19,20].
The time domain features were extracted by GPCA [21]. GPCA first maps EEG signals to a high-dimensional feature space and then applies principal component analysis for dimensionality reduction. For M samples x1,x2,...,xM in the input space, each of them is a d-dimensional vector. The similarity matrix K between samples was calculated by the Gaussian kernel function. Calculate the centralized matrix H, whose expression is:
H=I−1nEET | (1) |
where I is the identity matrix and E is a n-dimensional one-all matrix.
Calculate the centralized similarity matrix K', and its expression is as follows:
K′=H−1KH | (2) |
The eigenvalue decomposition of the centralized similarity matrix K' was carried out to obtain the eigenvalue and eigenvector. The eigenvector corresponding to the first k largest eigenvalues was selected as the principal component. The projection of all the samples onto k principal components forms a new eigenmatrix Z=[z1,z2,...,zM]T, as time domain features.
The frequency domain features were extracted by STFT [22]. STFT decomposes EEG signals into components of different frequencies to obtain the amplitude spectrum and phase spectrum within each time window. These features facilitated the description of EEG signals' energy distribution and frequency features across various frequencies.
Set the original EEG signal as x(t), sampling frequency as fs, time window length as Ls, and time window move step as s. For each time window, the amplitude spectrum and phase spectrum after STFT are calculated. Frequency domain feature X∈RNt×F, where Nt is the number of time windows and F is the frequency resolution of the amplitude spectrum. The specific STFT expression is as follows:
X(m,w)=Nt−1∑n=0x(n)w(n−m)e−jwn | (3) |
where x(n) is the original EEG signal, w(n-m) is the window function, w is the frequency, m is the time shift factor, and Nt is the number of time windows.
For each time window, set its starting time as ti, and calculate its amplitude spectrum A(k, i) and phase spectrum P(k, i) at k as follows:
A(k,i)=|STFT(xti[n])|kP(k,i)=arg(STFT(xti[n]))k | (4) |
where |⋅|k represents the module of the kth frequency component of the STFT calculation result, and arg(⋅)k represents the angle of the kth frequency component of the STFT calculation result. xti[n] represents a time window of length Ls from time ti.
Finally, the amplitude spectra of all time windows are splicing together to form the frequency domain feature X, and the ith row is the amplitude spectrum of the ith time window, namely X(i,k)=A(k,i).
The nonlinear dynamic features were extracted by SEn [23]. The algorithm requires a small amount of data and takes less time [24]. The time series T is divided into m subsequences of length Le, and the overlap rate is set as R.
The frequency of occurrence is calculated for each subsequence. The similarity between two subsequences is measured according to a distance metric. Calculate the occurrence frequency p:
pi=m−1C2mm∑j=1,j≠i[d(Ti,Tj)<r] | (5) |
where Ti represents the ith sub-sequence, and C2m represents the number of 2-combinations from a set with m distinct sequences, that is, C2m=m(m−1)2. r stands for a threshold to determine whether two subsequences are similar, where [d(Ti,Tj)<r] represent 1 for d(Ti,Tj)<r and 0 otherwise.
Then, the nonlinear dynamic feature S is concretely expressed as:
A=m−1∑i=1m∑j=i+1pi[d(Ti,Tj)<r]B=m∑i=1m∑j=i+1pi[d(Ti,Tj)<r]S(Le,r)=−log(A/B) | (6) |
Nonlinear dynamic feature S∈RM×N varies with the window length Le and the threshold r. In a specific research environment, the parameter setting is certain, where M represents the number of samples and N represents the dimension of nonlinear dynamic features. In general, r ∈ 0.1·std~0.25·std, where std represents the standard deviation of a given time series.
The self-attention mechanism [25] is applicable to situations where there are complex and nonlinear dependencies between different modalities and can improve the performance and expressiveness of the fused features, which will contain cross-modal information [26].
(a) Calculate query vector QT=ZWQT, key vector KT=ZWKT and value vector VT=ZWVT, where WQT,WKT,WVT∈RT×d are the weight matrixes and d is the hidden layer dimension. Calculate query vector QF=XWQF, key vector KF=XWKF and value vector VF=XWVF, where WQF,WKF,WVF∈RF×d are the weight matrixes and d is the hidden layer dimension. Calculate query vector QN=SWQN, key vector KN=SWKN and value vector VN=SWVN, where WQN,WKN,WVN∈RN×d are the weight matrixes and d is the hidden layer dimension.
(b) Calculate the attention score AT and output OT of time domain features. Calculate attention score AF and the output OF for frequency domain features. Calculate attention score AN and output ON for nonlinear dynamic features. The attention scores A and the output O are respectively expressed as:
A=softmax(Q(K)T√d)O=AV | (7) |
where Q is the query vector, K is the key vector, and V is the value vector.
(c) Integrate self-attention output O∈RM×(T+F+N) to get the feature representation:
O=[OT,OF,ON] | (8) |
Calculate the query vector Q=OWQ, the key vector K=OWK and the value vector V=OWV, where WQ,WK,WV∈R(T+F+N)×d are the weight matrixes and d is the hidden layer dimension.
(d) Enable the model to simultaneously participate and process diverse information from various subspaces to enhance its expression and ability to handle complex tasks. Q, K, and V are split into h heads, each with dimension d/h, and an independent self-attention calculation is performed for each head. Specifically, for each head i, calculate its attention score Ai and the corresponding attention output Oi:
Qi=headi(Q),Ki=headi(K),Vi=headi(V)Ai=softmax(Qi(Ki)T√d/dhh)Oi=AiVi | (9) |
Splice together the attention output Oi of all heads to obtain the final feature fusion matrix Y through a layer of the linear transformation:
O=[O1,O2,...,Oh]Y=OWY | (10) |
where WY∈Rd×dYis the weight matrix, and dY is the dimension of the final output feature fusion.
(e) Introduce the L1 norm regular term to reduce model complexity, increase robustness, and avoid overfitting. The objective function with a square loss is expressed as:
minWQ,WK,WV,WY1MM∑i=1‖Yi−Yi‖2F+λ‖Y‖1 | (11) |
where Yi is the real feature, ˆYi is the feature predicted by the model, M is the sample size, and λ is the weight of the regularization term of the L1 norm.
Update the weight matrixes WQ,WK,WV,WY by backpropagation algorithm to minimize the objective function in the training process [27]. The objective function enabled the model to learn the feature mapping Y and control the sparsity of the features by regularization terms. We measured the square of the Euclidean distance between the predicted and real features by square loss.
We extracted the time domain, frequency domain, and nonlinear dynamic features of EEG by GPCA, STFT, and SEn, respectively. The features of these three modalities were fused by the multi-head self-attention mechanism, and L1 norm regular term was introduced. In the implementation of STFT, the attention weights were calculated by Hamming window function [28] and Softmax function [29]. Additionally, the L1 norm was resolved through the gradient descent algorithm [30]. The proposed method was verified by leave-one cross-validation due to the limited EEG samples. Ten repeated experiments were conducted to obtain the average value. The classification performance was assessed according to the ACC index [31]. The influence of different parameters on the classification performance was first discussed to verify the classification performance of fused features, and the optimal parameters were determined by a one-to-many strategy for multi-category classification and then compared with other feature extraction methods [32].
The proposed method comprises multiple parameters. The mesh search method cannot find the optimal parameters directly. The optimal parameter values were systematically determined through a sequential process. (a) Determine the bandwidth σ of the Gaussian kernel function. (b) Set the window length Ls and step size s. (c) Establish the window length Le, overlap rate R, and threshold r. (d) Finalize the number of heads h and the L1 norm regularization parameter λ. The optimal classification model was formed by the EEG training set, and the model was assessed using the test set. The average of the test results was calculated to assess the model's performance. Multiple iterations of model training were conducted to ascertain the optimal hyperparameter. Subsequently, the model employing the optimal hyperparameter underwent testing with the original sample.
GPCA has demonstrated superior performance in EEG analysis, thus justifying its selection for this task [33]. It differs from traditional principal component analysis in that it does not directly obtain a fixed number of principal components. The input data is mapped to a high-dimensional feature space by the Gaussian kernel function. Then, the eigenvector of the covariance matrix in the feature space is calculated as the kernel principal component. Since there were only 100 samples per class, we considered choosing a smaller Gaussian kernel bandwidth to avoid overfitting [34]. Accordingly, the σ was set from 1 to 10, and ACCs of different σ were compared, as shown in Figure 2.
It is observed from Figure 2 that σ has a great impact on the model performance and determines the similarity of the data in the feature space. As σ increased from 1 to 10, the accuracy of the five-category classification increased gradually, reaching a peak and gradually declining. Specifically, when σ increased from 1 to 5, ACCs increased from 65.4% to 80.2%, indicating that increasing σ helped improve ACCs in this interval. While σ increased to 6 and above, ACCs gradually declined, decreasing from 79.4% to 76.2%. With GPCA, σ controlled the "smoothness" between points mapped into a high-dimensional feature space. Smaller σ meant that the points in the high-dimensional space were more dispersed, leading the model to focus too much on details and noise in the training data, i.e., overfitting.
Conversely, larger σ caused the mapped points to be more concentrated, leading the model to fail to capture important features from epileptic EEG signals, that is, underfit. ACCs increased first and then decreased with the increase of σ, indicating an optimal σ range. In this range, the model balanced the deviation and variance well and achieved a higher ACC. When σ was 5, the model reached the highest accuracy of 80.2%. This may indicate that the model complexity at this σ setting is moderate enough to capture key features from epileptic EEG signals without being too affected by noise.
Ls is usually chosen as the power of 2 to obtain better computational efficiency when performing a fast Fourier transform.s is usually set to half Ls or 1/4 of Ls [35]. Since there were only 100 samples per category, we considered choosing a smaller Ls and s to retain more time and frequency detail. On that basis, Ls was set to 32, 64,128,256, and s was set to 4, 8, 16, and the ACCs when different Ls s were compared, as shown in Figure 3.
It is observed from Figure 3 that when Ls was short (such as 32), ACCs were greatly affected by s to some extent, and ACCs ranged from 56.6% to 73.8%. This suggests that shorter Ls may be sensitive to time resolution, but have lower frequency resolution. When Ls was medium (e.g., 64), a significant increase was observed, especially when s was 8, and the ACCs reached up to 82.5%. This suggests that a moderate Ls may provide a better time-frequency resolution balance. For longer Ls (such as 128 and 256), the ACC changes were more complex, and the overall trend showed that the maximum ACC decreased as Ls increased. This could be attributed to the fact that although a longer Ls enhances frequency resolution, it compromises time resolution, impeding the ability to capture dynamic shifts from epileptic EEG signals accurately. For a given Ls, a shorter s (e.g., 4) yielded better or relatively stable ACCs. This could be attributed to the increased overlap in time, which allows for a more detailed capture of the signal. Conversely, a longer s (e.g., 16) often leads to a lower ACC in most cases. This decline in performance might be due to the reduced time coverage, potentially resulting in the omission of crucial time-frequency information.
It is commonly recommended that Le be set to a power of 2 during EEG signal processing when extracting nonlinear dynamic features of EEG by SEn. This ensures improved computational efficiency when carrying out fast calculations. The advantage of SEn in capturing local features was reflected by setting the overlap rate R, thereby preserving a richer array of time and frequency details. R chooses a value between 0.5 and 0.9. r thresholds SEn to extract nonlinear dynamic features. Generally, r ranges from 0.1·std to 0.25·std [36], where std represents the standard deviation of a given time series. It is advisable to opt for a shorter Le and a higher overlap rate When working with a limited dataset of 100 samples per category. This approach helped to retain a greater amount of temporal and frequency detail. Then, Le was set to 32, 64,128,256, R was set to 0.6, 0.7, 0.8, 0.9, and r was set to 0.1·std, 0.15·std, 0.2·std, 0.25·std, and the ACCs of the combination of different Le, R and r were compared, as shown in Figure 4.
It is observed from Figure 4 that when Le was 64, the ACC was generally higher with the change of R and r, especially when R was 0.8 and r was 0.15·std, reaching the highest ACC of 82.6%. ACCs decreased slightly when Le increased to 128, suggesting that a shorter Le may be better suited to capturing dynamic changes in epileptic EEG signals. As Le was further increased, ACCs decreased even more, particularly when Le reached 256, leading to a significant reduction in ACCs. This decline could be explained by the fact that a longer Le decreased the temporal resolution, thereby impeding the effective capture of rapid changes in epileptic EEG signals. Under each Le setting, ACCs generally increased first and then stabilized or slightly decreased with the increase of R. This suggests that appropriately increasing R can aid in enhancing ACCs. The reason for this improvement lies in the fact that a higher R offers a more extensive data sample, enabling a more detailed capture of signal changes. When R was high (e.g., 0.8), the ACC reached its highest at shorter Le (e.g., 64). This emphasizes improving the efficiency of feature extraction by increasing R while maintaining a high temporal resolution. The influence of r variations on ACCs exhibits complexity, lacking a discernible universal trend. This complexity can be attributed to the fact that selecting an appropriate r is intricately tied to the inherent dynamic features of epileptic EEG signals. Consequently, an apt r is crucial for distinguishing signals corresponding to different states. The ACC reached its peak when r was set to 0.15 std in certain scenarios, such as when Le was set to 64, and R was 0.8. This finding suggests that, under these settings, r is optimally suited to the characteristics of the current dataset. This ensures accurate SEn calculation results by limiting the similarity between subsequences and mitigating the influence of noise or other interfering factors.
The multi-head attention mechanism can extract the feature representation of different attention weights to enhance the expression ability of the model; thus, the number of heads h was usually set to a value between 2 and 8 [37]. The introduction of L1 norm regular term makes the model learn sparse feature representation to improve the model's generalization performance. The L1 norm regularization parameter λ controls how much the regularization term affects the overall loss function, and it is usually set to a value between 0.0001 and 0.1 [38]. Since there were only 100 samples per class, h was set to 4, 5, 6, 7, 8, and the value range of λ was set to [2–5, 2–3]. They provided sufficient model complexity while avoiding excessive computational burden. We compared the values of ACCs obtained from various combinations of h and λ, as shown in Figure 5.
When h was 4, ACCs increased somewhat (from 71.4% to 85.0%). λ increased from 2–5 to 2–4 but decreased (72.4%) when it was further increased to 2–3. Smaller regularization parameters may assist the model in better retaining crucial features, whereas substantial regularization parameters have the potential to cause the model to discard an excessive amount of information. When h was 5, ACCs fluctuated in the range from 2–5 to 2–3. The highest ACC occurred when the parameter combination was 5-headers and 2–4 regularized parameters (90.8%), which may be a more desirable combination. The ACC was higher overall when h was 6, and better results were achieved under different regularization parameters. The highest ACC (92.7%) occurred when the parameter combination was 6-head and 2–4 regularized parameters, indicating that the combination positively impacts the fusion of the time domain, frequency domain, and nonlinear dynamics features. ACCs were generally low when h was 7 and 8, especially when the regularization parameter was 2–4, and ACCs decreased significantly. An excessive number of heads might result in model complexity or overfitting, explaining the observed performance decline.
To sum up, σ was set to 5, Ls was 64, s was 8, Le was 64, R was 0.8, r was 0.15·std, h was 6, and λ was 2–4.
The proposed MMFF method was compared with the out-of-state feature extraction methods for epileptic EEG signals. Ten repeated experiments were conducted to get the average value from leave-one-out cross-validation. Specific methods included fast Fourier transform (FFT) [39], wavelet transform (WT) [40], mutual correlation power spectral density (RPSD) [41], genetic process-based feature extraction system (GPF) [42], power spectral density (PSD) [43], time-frequency analysis (TFA) [44], time-frequency analysis and approximate entropy (TFAE)[45], and time-frequency domain and spatial feature fusion (TFSF) [46]. Table 2 presents the mean ACCs, specificity (SPE), sensitivity (SEN), and respective variance for the five-category classification for epileptic EEG signals.
Dataset | Feature extraction method | Classification method | ACC (%) | SPE(%) | SEN(%) |
Set A, Set B, Set C, Set D, Set E | FFT | Decision tree | 81.32 ± 3.46 | 80.02 ± 2.51 | 82.55 ± 3.04 |
WT | Artificial neural network | 82.74 ± 2.85 | 82.61 ± 2.01 | 83.69 ± 2.53 | |
RPSD | SVM | 83.17 ± 1.55 | 83.52 ± 1.50 | 83.44 ± 2.08 | |
GPF | K-nearest neighbor classifier | 78.44 ± 4.28 | 77.83 ± 3.58 | 79.48 ± 4.02 | |
PSD | Gaussian mixture model | 86.53 ± 2.62 | 86.07 ± 2.05 | 87.09 ± 2.51 | |
TFA | Artificial neural network | 88.21 ± 2.06 | 88.67 ± 1.53 | 88.57 ± 1.84 | |
TFAE | SVM | 85.64 ± 1.47 | 85.32 ± 1.01 | 86.06 ± 1.53 | |
TFSF | SVM | 89.59 ± 2.13 | 89.66 ± 1.53 | 90.83 ± 2.09 | |
MMFF | SVM | 92.76 ± 1.64 | 92.51 ± 1.73 | 93.28 ± 1.57 |
It is found in Table 2 that different feature extraction methods have a significant impact on ACCs. The ACC (89.59 ± 2.13%) was achieved by TFSF with a support vector machine (SVM) classifier, and 88.21 ± 2.06% was achieved by TFA with an artificial neural network classifier. This indicates that time-frequency domain features or their fusion positively affect epileptic EEG signal classification. The ACC of TFAE achieved 85.64 ± 1.47%, but the ACC with an artificial neural network classifier may not be optimal. The SVM classifier achieved high ACCs in multiple feature extraction methods, which showed that it had good robustness and generalization abilities for epileptic EEG signal classifications. MMFF achieved the highest ACC (92.76 ± 1.64%), indicating that the method had a good classification performance for the extracted features. There were complex interactions between feature extraction methods and classification methods. TFSF attained a high ACC, while other classifiers may not have exhibited optimal performance. This underscores the importance of considering the compatibility between feature extraction methods and the features of specific tasks when choosing them. It is crucial to identify the most suitable combination to ensure optimal performance.
Epileptic EEG signals contain a wealth of information when processing biomedical signals. However, this information is often complex and multi-modal. The time domain features were extracted by GPCA to convert the original epileptic EEG signals into a smaller feature subspace with higher differentiation, such as mean value and standard difference. The frequency domain features were extracted by STFT to convert the epileptic EEG signals into the energy distributions at different frequencies, including the power spectral density and the phase of EEG signals. The nonlinear dynamic features were extract by SEn to assesse the complexity and irregularity of epileptic EEG signals, such as self-similarity and complexity.
The MMFF method significantly increased the ACC in epileptic EEG signals classification tasks, reaching 92.76 ± 1.64%. This method verifies that the multi-head self-attention mechanism can effectively learn the time, frequency, and nonlinear dynamic features interactively to better capture the multi-modal features from epileptic EEG signals. In this method, the features of these three modallities were fused to better integrate the information of different modalities and retained the time, frequency, and nonlinear dynamic features of epileptic EEG signals. This strategy improved the robustness and accuracy of feature extraction for epileptic EEG signals.
The proposed method obtained satisfactory results, but some limitations remained. For example, experimental datasets' limited size and origin may affect the model's ability to generalize. Future considerations include expanding the dataset size and further validating the model's applicability across a broader spectrum of scenarios. The calculation time of the model was too long, and each experiment lasted 5 days. We intend to refine the model structure, enhance feature extraction techniques, and investigate more efficacious epileptic EEG signal processing methods. In addition, our model lacks interpretability and requires the integration of clinical data and expert insights from real-world medical settings. In the future, we will advance the feature extraction of epileptic EEG signals in clinical practice to improve acceptability for medical professionals and patients. With a multi-head self-attention mechanism, the result is not perfect yet, so new deep learning methods will be used in the future to improve classification performance [47,48]. The available public data was preprocessed without significant noise. In the future, we will explore the sensitivity of the model to noise in EEG data or its performance at different data qualities.
We developed an MMFF method to improve epileptic EEG signal feature classifications. Different modalities of features were extracted from a time domain, frequency domain, and nonlinear dynamics, respectively. These features were interactively learned through the multi-head self-attention mechanism, thereby acquiring attention weights among them. The fused features preserve the time, frequency, and nonlinear dynamics information of epileptic EEG signals to screen out more representative epileptic features. Experimental results show that the proposed method achieves better results for the five-category classification task. This proves the superiority and feasibility of this model in feature extraction. Introducing MMFF with a multi-head self-attention mechanism demonstrated superior performance in the five-category classification task. This study provides a compelling new method in the field of feature extraction for epileptic EEG signals and provides a reference for the diagnosis and treatment of epilepsy.
The authors declare they have not used Artificial Intelligence (AI) tools in the creation of this article.
This work was supported by National Natural Science Foundation of China (grant No. 51877013), and Jiangsu Provincial Key Research and Development Program (grant No. BE2021636). This work was also sponsored by Qing Lan Project of Jiangsu Province.
The authors declare there is no conflict of interest.
[1] |
L. Chen, W. Q. Yang, F. Yang, Y. Y. Yu, T. W. Xu, D. Wang, et al., The crosstalk between epilepsy and dementia: A systematic review and meta-analysis, Epilepsy Behav., 152 (2024), 109640. https://doi.org/10.1016/j.yebeh.2024.109640 doi: 10.1016/j.yebeh.2024.109640
![]() |
[2] |
D. R. Nordli, K. Fives, F. Galan, Portable headband electroencephalogram in the detection of absence epilepsy, Clin. EEG Neurosci., 55 (2024), 581-585. https://doi.org/10.1177/15500594241229153 doi: 10.1177/15500594241229153
![]() |
[3] |
R. Qian, Z. G. Wu, The application of video electroencephalogram in the classification and diagnosis of post-stroke epilepsy, Clin. Neurosci. Res., 1 (2023), 37-41. https://doi.org/10.26689/cnr.v1i3.5849 doi: 10.26689/cnr.v1i3.5849
![]() |
[4] |
J. Li, X. L. Kong, L. L. Sun, X. Chen, G. X. Ouyang, X. L. Li, et al., Identification of autism spectrum disorder based on electroencephalography: A systematic review, Comput. Biol. Med., 170 (2024), 108075. https://doi.org/10.1016/j.compbiomed.2024.108075 doi: 10.1016/j.compbiomed.2024.108075
![]() |
[5] |
B. Boashash, S. Ouelha, Automatic signal abnormality detection using time-frequency features and machine learning: A newborn EEG seizure case study, Knowl. Based Syst., 106 (2016), 38-50. https://doi.org/10.1016/j.knosys.2016.05.027 doi: 10.1016/j.knosys.2016.05.027
![]() |
[6] |
S. A. Irimiciuc, A. Zala, D. Dimitriu, L. M. Himiniuc, M. Agop, B. F. Toma, et al., Novel approach for EEG signal analysis in a multifractal paradigm of motions. Epileptic and eclamptic seizures as scale transitions, Symmetry, 13 (2021), 1024. https://doi.org/10.3390/sym13061024 doi: 10.3390/sym13061024
![]() |
[7] |
M. Sabeti, S. Katebi, R. Boostani, Entropy and complexity measures for EEG signal classification of schizophrenic and control participants, Artif. Intell. Med., 47 (2009), 263-274. https://doi.org/10.1016/j.artmed.2009.03.003 doi: 10.1016/j.artmed.2009.03.003
![]() |
[8] |
M. Khayretdinova, I. Zakharov, P. Pshonkovskaya, T. Adamovich, A. Kiryasov, A. Zhdanov, et al., Prediction of brain sex from EEG: using large-scale heterogeneous dataset for developing a highly accurate and interpretable ML model, NeuroImage, 285 (2024), 120495. https://doi.org/10.1016/j.neuroimage.2023.120495 doi: 10.1016/j.neuroimage.2023.120495
![]() |
[9] | K. Prantzalos, D. Upadhyaya, N. Shafiabadi, G. Fernandez-BacaVaca, N. Gurski, K. Yoshimoto, et al., MaTiLDA: An integrated machine learning and topological data analysis platform for brain network dynamics, Pac. Symp. Biocomput. 2024, (2023), 65-80. https://doi.org/10.1142/9789811286421_0006 |
[10] | S. V. J, L. J. J, P. P. R, Depression detection in working environment using 2D CSM and CNN with EEG signals, in 2022 9th International Conference on Computing for Sustainable Global Development (INDIACom), (2022), 722-726. https://doi.org/10.23919/INDIACom54597.2022.9763173 |
[11] |
A. Seal, R. Bajpai, J. Agnihotri, A. Yazidi, E. Herrera-Viedma, O. Krejcar, DeprNet: A deep convolution neural network framework for detecting depression using EEG, IEEE Trans. Instrum. Meas., 70 (2021), 1-13. https://doi.org/10.1109/TIM.2021.3053999 doi: 10.1109/TIM.2021.3053999
![]() |
[12] |
N. Zrira, A. Kamal-Idrissi, R. Farssi, H. A. Khan, Time series prediction of sea surface temperature based on BiLSTM model with attention mechanism, J. Sea Res., 198 (2024), 102472. https://doi.org/10.1016/j.seares.2024.102472 doi: 10.1016/j.seares.2024.102472
![]() |
[13] |
J. Yang, M. Awais, A. Hossain, P. L. Yee, M. Haowei, I. M. Mehedi, et al., Thoughts of brain EEG signal-to-text conversion using weighted feature fusion-based multiscale dilated adaptive densenet with attention mechanism, Biomed. Signal Process., 86 (2023), 105120. https://doi.org/10.1016/j.bspc.2023.105120 doi: 10.1016/j.bspc.2023.105120
![]() |
[14] |
X. Zheng, W. Z. Chen, An attention-based bi-LSTM method for visual object classification via EEG, Biomed. Signal Process., 63 (2021), 102174. https://doi.org/10.1016/j.bspc.2020.102174 doi: 10.1016/j.bspc.2020.102174
![]() |
[15] |
B. X. Zhang, W. K. Li, Real-time emotion classification model for few-channel EEG signals, J. Chin. Comput. Syst., 45 (2024), 271-277. https://doi.org/10.20009/j.cnki.21-1106/TP.2022-0515 doi: 10.20009/j.cnki.21-1106/TP.2022-0515
![]() |
[16] |
Y. Kim, A. Choi, EEG-based emotion classification using long short-term memory network with attention mechanism, Sensors, 20 (2020), 6727. https://doi.org/10.3390/s20236727 doi: 10.3390/s20236727
![]() |
[17] | W. Chang, L. J. Xu, Q. Yang, Y. M. Ma, EEG signal-driven human-computer interaction emotion recognition model using an attentional neural network algorithm, J. Mech. Med. Biol., 23 (2023) 2340080. https://doi.org/10.1142/S0219519423400808 |
[18] |
H. Zhang, Q. Q. Zhou, H. Chen, X. Q. Hu, W. G. Li, Y. Bai, et al., The applied principles of EEG analysis methods in neuroscience and clinical neurology, Mil. Med. Res., 10 (2023), 67. https://doi.org/10.1186/s40779-023-00502-7 doi: 10.1186/s40779-023-00502-7
![]() |
[19] |
N. M. Gregg, T. P. Attia, M. Nasseri, B. Joseph, P. Karoly, J. Cui, et al., Seizure occurrence is linked to multiday cycles in diverse physiological signals, Epilepsia, 64 (2023), 1627-1639. https://doi.org/10.1111/epi.17607 doi: 10.1111/epi.17607
![]() |
[20] |
X. Yu, W. M. Li, B. Yang, X. R. Li, J. Chen, G. H. Fu, Periodic distribution entropy: Unveiling the complexity of physiological time series through multidimensional dynamics, Inform. Fusion, 108 (2024), 102391. https://doi.org/10.1016/j.inffus.2024.102391 doi: 10.1016/j.inffus.2024.102391
![]() |
[21] |
S. X. Jin, F. Q. Si, Y. S. Dong, S. J. Ren, A Data-driven kernel principal component Analysis–Bagging–Gaussian mixture regression framework for pulverizer soft sensors using reduced dimensions and ensemble learning, Energies, 16 (2023), 6671. https://doi.org/10.3390/en16186671 doi: 10.3390/en16186671
![]() |
[22] |
Ł. Furman, W. Duch, L. Minati, K. Tołpa, Short-time Fourier transform and embedding method for recurrence quantification analysis of EEG time series, Eur. Phys. J.: Spec. Top., 232 (2023), 135-149. https://doi.org/10.1140/epjs/s11734-022-00683-7 doi: 10.1140/epjs/s11734-022-00683-7
![]() |
[23] |
J. S. Tan, Z. G. Ran, C. J. Wan, EEG signal recognition algorithm with sample entropy and pattern recognition, J. Comput. Methods Sci. Eng., 4 (2023), 1-10. https://doi.org/10.3233/JCM-226794 doi: 10.3233/JCM-226794
![]() |
[24] |
X. F. Ye, P. P. Hu, Y. Yang, X. C. Wang, D. Gao, Q. Li, et al., Application of brain functional connectivity and nonlinear dynamic analysis in brain function assessment for infants with controlled infantile spasm, Chin. J. Contemp. Pediatr., 25 (2023), 1040-1045. https://doi.org/10.7499/j.issn.1008-8830.2305030 doi: 10.7499/j.issn.1008-8830.2305030
![]() |
[25] |
T. Ye, H. R. Chen, H. B. Ren, Z. K. Zheng, Z. Y. Zhao, LPT-Net: A line-pad transformer network for efficiency coal gangue segmentation with linear multi-head self-attention mechanism, Measurement, 226 (2024), 114043. https://doi.org/10.1016/j.measurement.2023.114043 doi: 10.1016/j.measurement.2023.114043
![]() |
[26] |
X. L. Tang, Y. D. Qi, J. Zhang, K. Liu, Y. Tian, X. B. Gao, Enhancing EEG and sEMG fusion decoding using a multi-scale parallel convolutional network with attention mechanism, IEEE Trans. Neural Syst. Rehabil. Eng., 32 (2023), 212-222. https://doi.org/10.1109/TNSRE.2023.3347579 doi: 10.1109/TNSRE.2023.3347579
![]() |
[27] |
T. Y. Liu, Y. F. Lin, E. L. Zhou, Bayesian stochastic gradient descent for stochastic optimization with streaming input data, SIAM J. Optimiz., 34 (2024), 389-418. https://doi.org/10.1137/22M1478951 doi: 10.1137/22M1478951
![]() |
[28] |
S. Kumar, K. Singh, R. Saxena, Analysis of dirichlet and generalized Hamming window functions in the fractional Fourier transform domains, Signal Process., 91 (2011), 600-606. https://doi.org/10.1016/j.sigpro.2010.04.011 doi: 10.1016/j.sigpro.2010.04.011
![]() |
[29] |
A. Bhusal, A. Alsadoon, P. W. C. Prasad, N. Alsalami, T. A. Rashid, Deep learning for sleep stages classification: modified rectified linear unit activation function and modified orthogonal weight initialization, Multimed. Tools Appl., 81 (2022), 9855-9874. https://doi.org/10.1007/s11042-022-12372-7 doi: 10.1007/s11042-022-12372-7
![]() |
[30] |
T. Y. Liu, Y. F. Lin, E. L. Zhou, Bayesian stochastic gradient descent for stochastic optimization with streaming input data, SIAM J. Optimiz., 34 (2024), 389-418. https://doi.org/10.1137/22M1478951 doi: 10.1137/22M1478951
![]() |
[31] |
T. Yang, Q. Y. Yan, R. Z. Long, Z. X. Liu, X. S. Wang, PreCanCell: An ensemble learning algorithm for predicting cancer and non-cancer cells from single-cell transcriptomes, Comput. Struct. Biotechnol. J., 21 (2023), 3604-3614. https://doi.org/10.1016/j.csbj.2023.07.009 doi: 10.1016/j.csbj.2023.07.009
![]() |
[32] | O. Gaiffe, J. Mahdjoub, E. Ramasso, O. Mauvais, T. Lihoreau, L. Pazart, et al., Discrimination of vocal folds lesions by multiclass classification using autofluorescence spectroscopy, medRxiv, (2023). https://doi.org/10.1101/2023.05.11.23289778 |
[33] |
T. Zhou, Y. B. Peng, Kernel principal component analysis-based Gaussian process regression modelling for high-dimensional reliability analysis, Comput. Struct., 241 (2020), 106358. https://doi.org/10.1016/j.compstruc.2020.106358 doi: 10.1016/j.compstruc.2020.106358
![]() |
[34] | Y. Nakayama, K. Yata, M. Aoshima, Clustering by principal component analysis with Gaussian kernel in high-dimension, low-sample-size settings, J. Multivariate Anal., 185 (2021), 104779. https://doi.org/10.1016/j.jmva.2021.104779 |
[35] |
A. Allagui, O. Awadallah, B. El-Zahab, C. Wang, Short-time fourier transform analysis of current charge/discharge response of lithium-sulfur batteries, J. Electrochem. Soc., 170 (2023), 110511. https://doi.org/10.1149/1945-7111/ad07ad doi: 10.1149/1945-7111/ad07ad
![]() |
[36] |
C. W. Wang, A. K. Verma, B. Guragain, X. Xiong, C. L. Liu, Classification of bruxism based on time-frequency and nonlinear features of single channel EEG, BMC Oral Health, 24 (2024), 81. https://doi.org/10.1186/s12903-024-03865-y doi: 10.1186/s12903-024-03865-y
![]() |
[37] |
Y. L. Ma, J. X. Ren, B. Liu, Y. Y. Mao, X. Y. Wu, S. D. Chen, et al., Secure semantic optical communication scheme based on the multi-head attention mechanism, Opt. Lett., 48 (2023), 4408-4411. https://doi.org/10.1364/OL.498997 doi: 10.1364/OL.498997
![]() |
[38] |
J. Saperas-Riera, G. Mateu-Figueras, J. A. Martín-Fernández, Lasso regression method for a compositional covariate regularised by the norm L1 pairwise logratio, J. Geochem. Explor., 255 (2023), 107327. https://doi.org/10.1016/j.gexplo.2023.107327 doi: 10.1016/j.gexplo.2023.107327
![]() |
[39] |
K. Polat, S. Güneş, Classification of epileptiform EEG using a hybrid system based on decision tree classifier and fast Fourier transform, Appl. Math. Comput., 187 (2007), 1017-1026. https://doi.org/10.1016/j.amc.2006.09.022 doi: 10.1016/j.amc.2006.09.022
![]() |
[40] |
C. A. M. Lima, A. L. V. Coelho, M. Eisencraft, Tackling EEG signal classification with least squares support vector machines: a sensitivity analysis study, Comput. Biol. Med., 40 (2010), 705-714. https://doi.org/10.1016/j.compbiomed.2010.06.005 doi: 10.1016/j.compbiomed.2010.06.005
![]() |
[41] |
Z. Iscan, Z. Dokur, T. Demiralp, Classification of electroencephalogram signals with combined time and frequency features, Expert Syst. Appl., 38 (2011), 10499-10505. https://doi.org/10.1016/j.eswa.2011.02.110 doi: 10.1016/j.eswa.2011.02.110
![]() |
[42] |
L. Guo, D. Rivero, J. Dorado, C. R. Munteanu, A. Pazos, Automatic feature extraction using genetic programming: An application to epileptic EEG classification, Expert Syst. Appl., 38 (2011), 10425-10436. https://doi.org/10.1016/j.eswa.2011.02.118 doi: 10.1016/j.eswa.2011.02.118
![]() |
[43] | K. C. Chua, V. Chandran, R. Acharya, C. M. Lim, Automatic identification of epilepsy by HOS and power spectrum parameters using EEG signals: A comparative study, in 2008 30th Annual International Conference of the IEEE Engineering in Medicine and Biology Society, (2008), 3824-3827. https://doi.org/10.1109/IEMBS.2008.4650043 |
[44] |
A. T. Tzallas, M. G. Tsipouras, D. I. Fotiadis, Epileptic seizure detection in EEGs using time–frequency analysis, IEEE Trans. Inf. Technol. Biomed., 13 (2009), 703-710. https://doi.org/10.1109/TITB.2009.2017939 doi: 10.1109/TITB.2009.2017939
![]() |
[45] |
S. F. Liang, H. C. Wang, W. L. Chang, Combination of EEG complexity and spectral analysis for epilepsy diagnosis and seizure detection, EURASIP J. Adv. Signal Process., 2010 (2010), 853434. https://doi.org/10.1155/2010/853434 doi: 10.1155/2010/853434
![]() |
[46] | Y. Chen, X. X. Hu, S. Wang, Depression recognition of EEG signals based on multi domain features combined with CBAM mode, Harbin Ligong Daxue Xuebao, (2023), 1-10. http://kns.cnki.net/kcms/detail/23.1404.N.20231108.1408.012.html. |
[47] | A. Shoeibi, M. Rezaei, N. Ghassemi, Z. Namadchian, A. Zare, J. M. Gorriz, Automatic diagnosis of schizophrenia in EEG signals using functional connectivity features and CNN-LSTM model, in Artificial Intelligence in Neuroscience: Affective Analysis and Health Applications, (2022), 63-73. https://doi.org/10.1007/978-3-031-06242-1_7 |
[48] |
M. Jafari, A. Shoeibi, M. Khodatars, S. Bagherzadeh, A. Shalbaf, D. L. García, et al., Emotion recognition in EEG signals using deep learning methods: A review, Comput. Biol. Med., 165 (2023), 107450. https://doi.org/10.1016/j.compbiomed.2023.107450 doi: 10.1016/j.compbiomed.2023.107450
![]() |
Data subset | A | B | C | D | E |
Subject | Five healthy volunteers | Five patients with epilepsy | |||
Subject status | Open-eyed | Close-eyed | Interparoxysmal | Paroxysmal | |
Recording period | Normal | Epileptic interval | Epileptic phase | ||
Electrode type | Non-invasive surface | Intracranial | |||
Electrode arrangement | International 10-20 standard system | Hippocampus structure | Lesion | All seizures |
Dataset | Feature extraction method | Classification method | ACC (%) | SPE(%) | SEN(%) |
Set A, Set B, Set C, Set D, Set E | FFT | Decision tree | 81.32 ± 3.46 | 80.02 ± 2.51 | 82.55 ± 3.04 |
WT | Artificial neural network | 82.74 ± 2.85 | 82.61 ± 2.01 | 83.69 ± 2.53 | |
RPSD | SVM | 83.17 ± 1.55 | 83.52 ± 1.50 | 83.44 ± 2.08 | |
GPF | K-nearest neighbor classifier | 78.44 ± 4.28 | 77.83 ± 3.58 | 79.48 ± 4.02 | |
PSD | Gaussian mixture model | 86.53 ± 2.62 | 86.07 ± 2.05 | 87.09 ± 2.51 | |
TFA | Artificial neural network | 88.21 ± 2.06 | 88.67 ± 1.53 | 88.57 ± 1.84 | |
TFAE | SVM | 85.64 ± 1.47 | 85.32 ± 1.01 | 86.06 ± 1.53 | |
TFSF | SVM | 89.59 ± 2.13 | 89.66 ± 1.53 | 90.83 ± 2.09 | |
MMFF | SVM | 92.76 ± 1.64 | 92.51 ± 1.73 | 93.28 ± 1.57 |
Data subset | A | B | C | D | E |
Subject | Five healthy volunteers | Five patients with epilepsy | |||
Subject status | Open-eyed | Close-eyed | Interparoxysmal | Paroxysmal | |
Recording period | Normal | Epileptic interval | Epileptic phase | ||
Electrode type | Non-invasive surface | Intracranial | |||
Electrode arrangement | International 10-20 standard system | Hippocampus structure | Lesion | All seizures |
Dataset | Feature extraction method | Classification method | ACC (%) | SPE(%) | SEN(%) |
Set A, Set B, Set C, Set D, Set E | FFT | Decision tree | 81.32 ± 3.46 | 80.02 ± 2.51 | 82.55 ± 3.04 |
WT | Artificial neural network | 82.74 ± 2.85 | 82.61 ± 2.01 | 83.69 ± 2.53 | |
RPSD | SVM | 83.17 ± 1.55 | 83.52 ± 1.50 | 83.44 ± 2.08 | |
GPF | K-nearest neighbor classifier | 78.44 ± 4.28 | 77.83 ± 3.58 | 79.48 ± 4.02 | |
PSD | Gaussian mixture model | 86.53 ± 2.62 | 86.07 ± 2.05 | 87.09 ± 2.51 | |
TFA | Artificial neural network | 88.21 ± 2.06 | 88.67 ± 1.53 | 88.57 ± 1.84 | |
TFAE | SVM | 85.64 ± 1.47 | 85.32 ± 1.01 | 86.06 ± 1.53 | |
TFSF | SVM | 89.59 ± 2.13 | 89.66 ± 1.53 | 90.83 ± 2.09 | |
MMFF | SVM | 92.76 ± 1.64 | 92.51 ± 1.73 | 93.28 ± 1.57 |