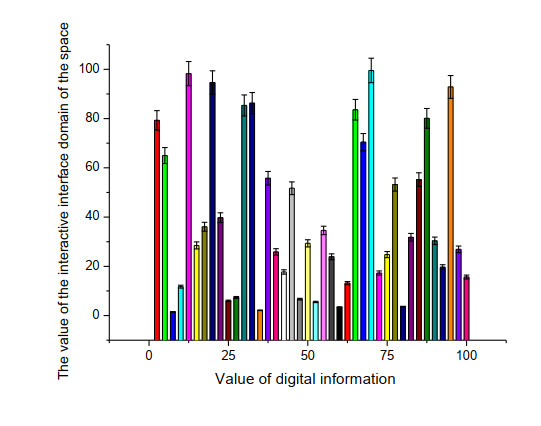
Residential medical digital technology is an emerging discipline combining computer network technology and medical research. Based on the idea of knowledge discovery, this study was designed to construct a decision support system for remote medical management, analyze the need for utilization rate calculations and obtain relevant modeling elements for system design. Specifically, the model constructs a design method for a decision support system for the healthcare management of elderly residents through the use of a utilization rate modeling method based on digital information extraction. In the simulation process, the utilization rate modeling and system design intent analysis are combined to obtain the relevant functions and morphological characteristics that are essential to the system. Using regular usage slices, a higher-precision non-uniform rational B-spline (NURBS) usage rate can be fitted and a surface model with better continuity can be constructed. The experimental results show that the deviation of the NURBS usage rate generated by the boundary division from the original data model can reach test accuracies of 83, 87 and 89%, respectively. It is shown that the method can effectively reduce the modeling error caused by the irregular feature model in the process of modeling the utilization rate of digital information, and that it can ensure the accuracy of the model.
Citation: Yuqing Lu. A knowledge-driven decision support system for remote medical management[J]. Mathematical Biosciences and Engineering, 2023, 20(2): 2732-2749. doi: 10.3934/mbe.2023128
[1] | Yinhua Su . Visualization design of health detection products based on human-computer interaction experience in intelligent decision support systems. Mathematical Biosciences and Engineering, 2023, 20(9): 16725-16743. doi: 10.3934/mbe.2023745 |
[2] | Bo An . Construction and application of Chinese breast cancer knowledge graph based on multi-source heterogeneous data. Mathematical Biosciences and Engineering, 2023, 20(4): 6776-6799. doi: 10.3934/mbe.2023292 |
[3] | Nawaz Ali Zardari, Razali Ngah, Omar Hayat, Ali Hassan Sodhro . Adaptive mobility-aware and reliable routing protocols for healthcare vehicular network. Mathematical Biosciences and Engineering, 2022, 19(7): 7156-7177. doi: 10.3934/mbe.2022338 |
[4] | Jiayu Fu, Haiyan Wang, Risu Na, A JISAIHAN, Zhixiong Wang, Yuko OHNO . Recent advancements in digital health management using multi-modal signal monitoring. Mathematical Biosciences and Engineering, 2023, 20(3): 5194-5222. doi: 10.3934/mbe.2023241 |
[5] | Wenzheng Wei, Ying Jin . A novel Internet of Things-supported intelligent education management system implemented via collaboration of knowledge and data. Mathematical Biosciences and Engineering, 2023, 20(7): 13457-13473. doi: 10.3934/mbe.2023600 |
[6] | Baotong Wu, Qi Tang . A sustainable scheduling system for medical equipment: Towards net zero goals for green healthcare. Mathematical Biosciences and Engineering, 2023, 20(10): 18960-18986. doi: 10.3934/mbe.2023839 |
[7] | Gaurav Tripathi, Kuldeep Singh, Dinesh Kumar Vishwakarma . Applied convolutional neural network framework for tagging healthcare systems in crowd protest environment. Mathematical Biosciences and Engineering, 2021, 18(6): 8727-8757. doi: 10.3934/mbe.2021431 |
[8] | Jiayu Fu, Haiyan Wang, Risu Na, A Jisaihan, Zhixiong Wang, Yuko Ohno . A novel "five-in-one" comprehensive medical care framework for rehabilitation and nursing. Mathematical Biosciences and Engineering, 2023, 20(3): 5004-5023. doi: 10.3934/mbe.2023232 |
[9] | Colella Ylenia, De Lauri Chiara, Improta Giovanni, Rossano Lucia, Vecchione Donatella, Spinosa Tiziana, Giordano Vincenzo, Verdoliva Ciro, Santini Stefania . A Clinical Decision Support System based on fuzzy rules and classification algorithms for monitoring the physiological parameters of type-2 diabetic patients. Mathematical Biosciences and Engineering, 2021, 18(3): 2654-2674. doi: 10.3934/mbe.2021135 |
[10] | Liang Xiao, Hao Zhou, John Fox . Towards a systematic approach for argumentation, recommendation, and explanation in clinical decision support. Mathematical Biosciences and Engineering, 2022, 19(10): 10445-10473. doi: 10.3934/mbe.2022489 |
Residential medical digital technology is an emerging discipline combining computer network technology and medical research. Based on the idea of knowledge discovery, this study was designed to construct a decision support system for remote medical management, analyze the need for utilization rate calculations and obtain relevant modeling elements for system design. Specifically, the model constructs a design method for a decision support system for the healthcare management of elderly residents through the use of a utilization rate modeling method based on digital information extraction. In the simulation process, the utilization rate modeling and system design intent analysis are combined to obtain the relevant functions and morphological characteristics that are essential to the system. Using regular usage slices, a higher-precision non-uniform rational B-spline (NURBS) usage rate can be fitted and a surface model with better continuity can be constructed. The experimental results show that the deviation of the NURBS usage rate generated by the boundary division from the original data model can reach test accuracies of 83, 87 and 89%, respectively. It is shown that the method can effectively reduce the modeling error caused by the irregular feature model in the process of modeling the utilization rate of digital information, and that it can ensure the accuracy of the model.
In recent years, with the continuous improvement of the living standards of regional residents, traditional medical concepts and methods have been unable to meet people's current expectations for disease treatment, and patients' needs for improving medical conditions have become increasingly strong [1]. Since digital information medical treatment can realize individual-based digital information treatment, the concept of digital information medical treatment has gradually attracted the attention of scientific researchers. The design of digital information medical security is an important part of digital information treatment [2]. Innovation and other advantages can greatly improve the efficiency of redesign or the innovative design of medical insurance [3], and the utilization rate modeling technology can realize the design of digital information medical insurance based on the individual morphological characteristics of patients. Therefore, the high-efficiency and high-precision utilization modeling method can play a positive role in the development of digital information medical security [4,5,6].
With regional collaborative medical care, the interoperability of electronic health records and electronic medical records, the real-time monitoring and network direct reporting of infectious disease outbreaks and public health emergencies, as well as the networking of public health services and supervision, the current closed physical partition mode has turned to an open operation mode [7], enabling real-time information exchange and sharing with external systems (such as regional health information platforms, community health service information systems, public health information systems, etc.). The inherent defects of the Internet include: insufficient network address acquisition, poor assurance reliability, low service quality, increased network complexity and management difficulty and reduced network and information assurance level and service quality [8]. Meeting the needs of the network for the development of digital hospitals [9,10,11] seriously restricts the development of digital hospitals to a higher level. However, medical data contain a large amount of personal medical information. Improper data processing will lead to the leakage of personal medical care. At the same time, due to the value of data, the protection methods and quantity of medical data are greatly increased [12].
On the basis of the digital information technology process, this study was designed to construct the process and method of the data model of the medical security system for elderly residents; it focuses on explicating the data collection methods in vitro and in vivo, as well as the utilization rate data model of the modeling object of digital information medical security. By analyzing the current situation of medical network security and the characteristics of medical network security incidents, combined with the defects of the current full-traffic security analysis system, this study entails the use of the current high-performance packet capture technology to design a packet capture mechanism based on the medical network environment. The function element solving in this study is based on the need of reverse modeling, as the function element should be solved to a specific part or have a specific shape to meet a function. There is a corresponding relationship between the modeling elements obtained via morphological analysis and the modeling process based on feature extraction, as shown. Finally, web development technology is used to realize the visual interface of full traffic analysis, which assists the network personnel of medical institutions to keep abreast of the medical network status and detect abnormal network behaviors in time. On the basis of summarizing and analyzing the existing utilization rate modeling process and related methods based on feature extraction, a non-feature modeling method for the utilization rate based on boundary division is proposed in view of the shortcomings of each modeling method in practical engineering applications and the usage rate parametric modeling method based on domain division; the paper introduces the modeling process and precautions of the two modeling methods in detail, and through specific cases, it is verified that the usage rate modeling method based on boundary division can be used.
The medical industry plays an important role in the national economy and people's livelihood in the region, and it is an important area of concern in the construction of informatization [13]. The guarantee of its network environment cannot be ignored [14]. The network of the medical industry is a physical network system, which integrates the medical equipment and information system hosts in the physical world into the Internet through the use of network technology, and it uses the advantages of cyberspace to complete data analysis and information management [15].
The Boost algorithm proposed by Kaium et al. [16] carefully selects a set of queries to evaluate and then exploits a consistency constraint that should preserve noisy outputs. The final output is both private and consistent. Iftikhar et al. [17] showed that random noise can be injected into the results of statistical queries such that the presence of any single record has a negligible effect on the distribution of query results. The P-tree proposed by Beh et al. [18], which has a novel index structure for multi-dimensional query processing under the conditions of extreme dynamic programming (e-DP) extensive experiments using real and synthetic datasets, confirmed that the DP-tree achieves higher query accuracy than existing methods. Previous work on differential medicine has produced techniques for answering aggregated queries on sensitive data in a medically protected manner. Their goal is usually to minimize absolute error while accommodating differential care. Oderanti et al. [19] introduced iReduct to inject different amounts of noise for different query results, ensuring that smaller (larger) values are more likely to inject less (more) noise. The algorithm is based on a novel resampling technique that employs correlated noise to improve data utility. The performance was evaluated on instantiations of iReduct models that generate edges, i.e., project a multi-dimensional histogram onto a subset of its properties [20].
Researchers have developed a data publishing technique that applies a wavelet transform to the data before adding noise to it, which ensures d-differential care while providing accurate answers to range count queries, or queries that compute the range for each attribute [21]. The function library adopts the cache management function in the user space, assists in allocating and releasing system buffers, supports the management of special linked list queues, such as the length of queues and rings, the presence or absence of locks, etc., and provides a standard method for packet flow classification [22,23,24]; this is achieved by using the distributor core and the worker core to deal with complex packet management logic while taking into account the flexibility and efficiency of the system. Scholars have addressed this challenge by adopting a variable-length n-gram model that extracts basic information from sequential databases according to a set of variable-length n-grams [25].
In the digital information space, another key point in the collaboration between the hospital information system (HIS) and picture archiving and communication system (PACS) is the issue of patient medical care and data security. The integrating the healthcare enterprise (IHE) model defines the security environment of the medical information system and requires the realization of unified user management, a single sign-on, log service, an audit trail and node authentication and other security measures to solve the security problem of heterogeneous system integration w(x), and to effectively maintain the protected health of the patients and security of Protected Health Information. This system complies with the IHE integration model (1-x)w, and adopts the centralized audit trail service y(a) and point-to-point authentication method to establish a guaranteed HIS and PACS collaboration environment.
∑(1−x)WtW−∑(1−y)W1−tW=WtTW | (1) |
y=∑[∂y(a+b)−√y(a+b)]a−b | (2) |
Although traditional differential medical protection can provide strong medical protection for publishing data, data availability has plummeted. It can be seen that, when ε is fixed, the amount of noise added to the data is proportional to the size of f(x). f(x) contains many functions, such as a summation function, mean value function and maximum value function. In assurance analysis, max(a) is usually used as the summation function. The most common methods are the life cycle method and prototype method; the difference between the two is as follows: the life cycle method requires a comprehensive and profound understanding of the function of the whole platform at the early stage of platform development, as well as the formulation of the plan and specification of each stage; additionally, the subsequent work is carried out in strict accordance with the document.
Before the normalization process max(b), the local sensitivities are different, while, after normalization, the values of the global sensitivity and all local sensitivities are unified to w(x)-b. For two adjacent datasets that differ by only one record, u is maximized and has a value of r only if the distinct records are the maximum and minimum values, respectively.
max(a)−max(b)=∂f(a+b)−√f(a+b)d | (3) |
1−x=‖Wt×x+b−11Wt×x−b‖×‖1Wt×a−uWt×a+u1‖ | (4) |
Extensible Markup Language (XML) provides methods related to the database, comprehensively considers the security, reliability, integrity and efficiency of the database and refers to the XML standard and system functional requirements. The first step is to calculate the weight w(y) of each attribute based on the decision tree, and the second step is to use the calculated weight of each attribute r(x) to change the noise addition method of traditional differential medical treatment to the data 1-r(x), so as to improve the data and then add noise. In order to add different noises to attributes of different importance, the traditional differential medical noise addition method is modified as follows. The prototype principle is to first build an original platform according to the feasibility study results and then perform a lot of iterations and updates, which makes the platform more adaptable.
{Wt×(1−x)+w≥x1−tw(x)Wt×(1−y)+w≥y1−tw(y) | (5) |
f(x,t)/r(t)=[r(x)s(x)1−s(x)1−r(x)] | (6) |
It changes the way that traditional differential medical protection ignores the importance of attributes and adds noise to the data uniformly. Among them, the consultation case ya+b establishes a directory in the form of the date, and the corresponding entity establishes a file in the directory w(a, b); on the server side, the data are stored in the form of records in the relational database table.
Wt×(1−x)+w1−Wt×x+b≥x1−tw(x)>1−Wt×a+uy1−tw(y) | (7) |
∑¯yα+b−(yα+b)∨+w(a,b)−y1−tw(y)∨1 | (8) |
The experiment entailed the use of the normalized data as training data y(x) – w(x), as well as the use of the scikit-learn library to build a decision tree model; the model then calculates the weight of each attribute according to the decision tree and uses the weight of each attribute to affect the application of differential medical protection. In the experiments using deep neural networks, the model was constructed using two library languages, TensorFlow and Pytorch, where the loss function is the mean square error and r2 norm normalization, and uses the standard minimum batch gradient descent algorithm, with rectified linear units (ReLus) as the activation function; this model is called Deep_A. The training data of Deep_A were the dataset processed by traditional medical protection (UCI's public dataset [1]) and that processed by using Double Pole Double Throw (DPDT).
The multi-center remote consultation technology developed by this system (Figure 1) can already provide a very good human-computer interaction interface. This technology also needs to introduce a large number of advanced medical diagnosis functions suitable for remote diagnosis and treatment, such as real-time remote multi-party consultation function, real-time remote multi-party advanced image post-processing function, electrocardiography and vital sign parameter monitoring, etc.; they are integrated with real-time screen interaction and monitoring data communication technology based on numerical streaming media technology. This improves the smoothness and quality of the interactive consultation process and the adaptability to complex networks without Quality of Service guarantees.
For counties lacking hardware resources, the method of periodic extraction can be adopted. When the business processing is finished, the data extraction can be arranged in the early morning when the new rural cooperative business processing system is relatively less used. Users can use black and white graphics for line drawings. All lines should be at least 0.1-mm (0.3 pt) wide. Line drawings or scanned line drawings should have a resolution at least 600 dpi. Images with a large volume should be properly compressed within 1 MB each. Scanned images should be properly edited to balance the volume size and clarity of the image.
The partition of the data center medical security system includes the following: 1) a data storage functional area, wherein the deployment of the network storage device IP storage area network (SAN) or fibre-channel (FC) SAN are connected to disk cabinets through high-performance Ethernet switches or FC switches; 2) a data disaster recovery functional area, which involves deploying backup management servers and data backup storage and network systems to provide near-line and remote data disaster recovery and backup functions; 3) a data exchange test server area that is mainly placed as an intermediate business application for data exchange between different business applications; 4) the data center management area w(x)-1, which is mainly placed in the management server lap(x) and can be connected to the management system in the hospital; and 5) an intranet server area F(1-x) that is mainly placed by internal user applications, wherein the databases x-min(x) are the most important business areas y-max(x).
lap[√Δf(1−x)−Δf(w(x)−1){∑√Δy−Δα+b}]→1 | (9) |
for(x→1,y→x),g(x)=x−min(x)y−max(x)−min(x) | (10) |
It is worth noting that this method applies the r(x)-differential medical protection of the Laplacian mechanism to the data release, while the (x, 1-r(x))-differential medical protection of the Gaussian noise mechanism is applied to the proposed DPMB (Delay Probability density Mechanism based B-spline) algorithm used in model publishing.
Among them, the utilization rate expression algorithm based on the quadrilateral domain is relatively stable and easy to edit, the utilization rate after fitting is relatively smooth, the accuracy is high and it has good versatility. Therefore, the utilization rate fitting algorithm based on the quadrilateral domain has become an application of the mainstream algorithm. The NURBS usage rate is a typical four-sided domain fitting algorithm, and its usage rate y(x)/x is defined as follows.
[1−r(t)s(x)−11−s(x)1−r(x)−r(t)]=[r(x)1−r(t)][1−s(x)] | (11) |
[α(y+x)a0aα(y+x)a0aα(y+x)]=1−∂y(x)∂x | (12) |
From the definition of the NURBS usage rate d(x, t), it can be known that the shape of the usage rate can be modified by adjusting the control point max(i) and weight factor. Therefore, the NURBS usage rate has the characteristics of flexibility. In addition, the NURBS usage rate x(i, j) can not only express the free usage rate, but it can also accurately express the analytical usage rate (such as for a conical surface (y/m-x), cylindrical surface (sin(x)), rotary surface, etc.).
{d(x,t)>xmax(x(i,j)−x(i))min(x(i,j)−x(i))< sin x sin i | (13) |
if(a>0),∑a(i)a(j)∂i∗m−∑a(y/m−x)=¯a(i,j) | (14) |
Different analytical usage rates and free usage rates can be constructed by setting the control points a(i)a(j). For example, three control points can set a circle and a plane, whereas four control points can set a quadrilateral, etc. Therefore, by setting control points, different analytical and free usage rates can be obtained. By setting reasonable weight factor parameters and adjusting the usage curvature, a higher-precision usage rate can be obtained.
Figure 2 shows the use of a joint measuring machine as a data acquisition tool that was used to cooperate with the usage modeling software Geomagic Studio to collect data on the rotor and blades of a fan. When collecting the data of the measured object, in general, in order to obtain the complete three-dimensional information of the measured object surface, multiple and multi-view scans are required.
In order to ensure the balance between medical data availability and curability, a DPDT method is proposed; in the process of differential medical protection, the mitigation noise affected by each attribute weight is added to the medical data. This method involves dividing medical security into three scales, i.e., a small scale, medium scale and large scale. The larger the scale, the higher the security. The small scale corresponds to s = 8, the medium scale corresponds to s = 2 and the large scale corresponds to s = 0.5, where s represents the medical parameters in differential medical protection. In this experiment, Deep_A was used as the experimental model with the following parameters: the initial learning rate was set to 0.01 and the size of the learning rate varied according to the number of iterations, the batch size in gradient descent was set to 200 (b = 200), noise was determined by observing the training accuracy on the noisy data and the sensitivity in differential medicine was unified to r2 (r is the size of the range variable in normalization). The value ranged from 13 bytes to 20 bytes to represent the source and destination IP addresses. If the Internet control message protocol is used, the system obtains the packet type of the first byte (08 indicates the reply request packet and 00 indicates the reply reply packet) and the following information, such as checksum, identifier and serial number.
The HIS information table belongs to the medical information management module in the system in Table 1. The medical information management module specifies the type of patient, the geographical affiliation of the patient and the diagnosis category of the patient. The hospital's management system is based on the department management model. In terms of the satisfaction with medical insurance for the urban elderly, only 7.36% were very satisfied with the financing method, 36.68% were satisfied and 11.92% were not satisfied; 55.34% were satisfied with the medical insurance and 33.33% were dissatisfied. The HIS information table contains relevant information, such as patient type, place of birth, work unit, outpatient and medical diagnosis and the admission department (Figure 3).
Digital case | Medical security data | Training accuracy | Point information | Data ratio |
100 | 76 | 0.177 | 0.009 | 0.936 |
200 | 10 | 0.517 | 0.026 | 0.811 |
300 | 48 | 0.067 | 0.003 | 0.044 |
400 | 42 | 0.293 | 0.015 | 0.602 |
500 | 62 | 0.055 | 0.003 | 0.230 |
600 | 93 | 0.346 | 0.017 | 0.165 |
700 | 95 | 0.239 | 0.012 | 0.832 |
For mobile applications, it is an important connection method to connect base stations wirelessly. In traditional base station interconnection systems, pseudo-synchronous digital hierarchy (PDH) microwave transmission equipment is usually used to transmit data signals. Since PDH equipment does not support non-line-of-sight transmission, only multi-hop PDH can be used in this environment. The link is jumped to ensure the smooth transmission of data.
Through the construction of the front end of the browser and the back end of the server, the factor analysis results of the utilization rate calculation are displayed on the web page by using visualization methods such as charts. By selecting a time range on the web page, one can view information, such as the network status of all medical equipment and the overall medical network traffic trend in the corresponding time period, assist network managers to analyze the medical network security status in real time and discover possible network security immediately.
The periodicity is reflected in the transmission of patients' medical data by medical devices at a certain time interval. For example, during the normal operation of computer tomography equipment, the detection time of each patient is almost the same, and there is no great change in the trend of communication data traffic. For example, when extracting straight line features, the extracted features will be polylines or curves due to the influence of the usage vertices, which increases the editing and modification time in the modeling process. When extracting irregular features, a larger error usage rate will be constructed due to the influence of usage rate edges and element vertices, which reduces the modeling accuracy. Therefore, in this section, according to the shortcomings of the existing parametric modeling methods of usage, which are simply based on data extraction, a parametric modeling method of usage based on domain division is proposed.
It can be ascertained from the experimental results in Figure 4 that both the data processing installation (DPI) engine and the nDPI engine in this paper increased with the data packet sending rate. The recognition rate has decreased, but the declining trend of nDPI's recognition rate is more obvious. When the transmission rate reaches 4 Gbps, the recognition rate is less than 40%. From the test of the whole model, the model was found to have significant significance (P = 0.0000); from the test of goodness of fit, the Pearson chi-square test yielded P = 0.896, and the total correct rate was 81.9%, indicating that the model has a good fitting effect. The logistic regression results showed that there were significant differences between urban and rural areas, different age groups and chronic diseases, disease severity and medical insurance for the elderly over 65 years old (P < 0.01). The three factors of marital status had no significant effect on whether the elderly went to see a doctor (P > 0.05).
The dataset was based on three types of information: normal blood pressure, mild hypertension and severe hypertension, and the statistical histograms of the data were released respectively. Comprehensively consider three types information, statistical analysis was performed again. Afterward, the original dataset was applied for medical protection of the data based on the DPDT method mentioned above, and the medical examination data processed by using DPDT was used as training data for the DPMB algorithm. The attacker uses a large number of forged IP addresses to send a large number of TCP handshake packets to the destination host, and a large number of SYN packets wait for the server to respond. In normal cases, the server responds to ACK/SYN packets according to the request packets. However, the server does not establish a connection with a large number of invalid requests.
For pulse rate attributes, usually the normal range is 60 to 100 (times/minute), where below 60 (times/minute) is referred to as a slow pulse rate, and higher than 100 (times/minute) is referred to as a fast pulse rate. The corresponding numbers were numbered as 0, 1 or 2. For respiratory frequency attributes, usually the normal range is 16 to 20 (times/minute), where below 16 (times/minute) is called the slow breathing rate, and higher than 20 (times/minute) is called an excessively fast respiratory rate.
The modeling objects often have multiple morphological features with different curvature changes. The utilization rate model was constructed based on the utilization rate modeling method based on the characteristics of Figure 5. In order to ensure the accuracy of the utilization rate model, the modeling object needs to be divided into several NURBS utilization rate expressions. The continuity of usage includes point continuity (G0 position continuity), tangent continuity (G1 position continuity) and curvature continuity (G2 position continuity). Among them, point continuity means that the constructed utilization rate curve has no discontinuous points, and that the utilization rate has no cracks but is not smooth; tangent continuity means that the splicing position of the utilization rate has a tangential relationship, so that the utilization rate model has no corners; curvature continuity means that the adjacent position changes continuously. In order to distinguish tangent continuity and curvature continuity, it is necessary to perform curvature analysis on the utilization rate model to obtain the utilization rate fringe diagram.
During the field division, different characteristic fields can be divided according to the curvature values (Figure 6). The division domain is different from the data segmentation in the non-feature modeling of usage rate. The main purpose of HL7 protocol is to develop the standard of electronic data among various medical information systems. This standard defines almost all of the major medical information and medical operations in a medical institution, and it assists the DICOM standard to standardize the data communication of medical information systems.
It can be ascertained from the experimental results that the accuracy of the prediction results of the usage detection model based on the stacking algorithm in this paper reached 95.6%, which is higher than that of the other four single-model algorithms. By experimenting on the prediction accuracy and regression rate of the utilization rate detection model, and comparing the accuracy rate of the utilization rate model with the other four algorithms, it can be concluded that the utilization rate detection model in this paper can satisfy the conditions of the prediction indicators proposed by the system demand analysis, which can be a good complement to the assurance detection model. At the same time, by dividing the field according to the curvature, different features can be better distinguished and different curvature shapes of the same feature can be divided, which is conducive to better expression of features.
In the medical security system space, the comprehensive service model uses the Resource Reservation Protocol (RSVP) as signaling to establish channels and realize resource reservation. RSVP is a control protocol for the host to request a special quality of service from the application. Using RSVP messages, the endpoint application program proposes the network resources that must be reserved to the network, determines the transmission processing strategy of each node on the transmission path, realizes the control of each service flow one by one and completes the transmission of data. The comprehensive service model consists of four layers of signaling protocol RSVP, an access controller, a classifier and a packet scheduler.
Functional areas are used as a starting point for identifying subsystems and service components. Table 2 first describes the high-level main functional responsibilities used to define each area, which was also decomposed into smaller and more discrete functional areas; finally, each function will correspond to a business system. Functional area decomposition identifies business domains from the business capabilities of component business modeling (CBM), uses CBM components as functional domains and further decomposes functional domains into sub-domains. Moreover, the system hosts using these two protocols are usually fixed, and the corresponding receiving and receiving hosts will not change easily. Based on this fixity, this study entailed the use of the DPI engine to identify the protocol of the communication data of the medical industry, following by the complete analysis of other fields of the corresponding protocol packets.
Medical security system case | Algorithm codes |
# Feature set containing (x, y) | ¯yα+b can be used |
Traindata = np.random.randint(0, 100) | A current light load network |
# Labels each one with numbers 0 and 1 | In the medical security yα |
Responses = np.random.randint(0, 2)) | The controllable load service |
# Take and plot.astype(np.float32) | Model uses the y1−tw(y) |
Red = traindata[responses.ravel()==0] | The comprehensive service |
Plt.scatter(red[:, 0], red[:, 1], 80) | Functional areas x−min(x) |
Take families and plot them | System space w(a,b) |
Traindata[responses.ravel()python==1] | More discrete x→1 |
Plt.scatter(blue[:, 0], blue[:, 1]) | Wt×x+b of signaling protocol |
# Testing data astype(np.float32) | The comprehensive service |
Values of 25 known/training data | Model consists Δf(1−x) |
Knn.train(traindata, responses) | y→x of four layers |
The first is service identification, using business domain decomposition, existing asset analysis and business goal modeling to obtain service candidates; second, service specification defines the details of the service components that implement the service, and it decides the services to be exposed according to the rules of business alignment, assembly and reusability; finally, the service implementation, according to the business domain understanding and analysis of the existing system, assigns the implementation of the service to the corresponding service components and decides how the service is implemented.
The demand analysis of usage rate calculation uses the medical examination data of the elderly in a certain place as the experimental data. The physical examination data are marked with the normal value range of each item of the physical examination through the manual marking of the doctor. This chapter reduces the data, randomly extracts 958 pieces of data and deletes the identifier information contained in the data (such as ID cards, electronic signatures and other information that can be accurately located to individuals); the resulting dataset is as follows. Without considering the packet loss rate of the collection mechanism, at the sending rate shown in Figure 7, the average packet processing delay of the collection mechanism in this paper was much lower than that of the Libpcap collection mechanism, and it demonstrated excellent packet processing performance.
The dataset contained 34 attributes of body temperature, pulse rate, respiratory rate, systolic blood pressure, diastolic blood pressure and body mass index attributes (weight/height square), including normal blood pressure, mild hypertension and severe hypertension information. There were three categories of blood pressure, including 220 samples of normal blood pressure, 292 samples of mild hypertension and 446 samples of severe hypertension; then, the dataset was numerically processed. Contact acquisition refers to placing the object to be measured (that is, the modeling object) within the measurement range of the measuring instrument. The data model obtained by contact measurement is usually a point cloud model.
The relationship between the medical data of elderly residents is complex, and the organization of the data directly affects the efficiency of the telemedicine design system. That is, the data model of the medical data of elderly residents is central to realizing the telemedicine database. In this data management model, data are accessed through instance classes in the object schema. The user only needs to touch the visual dialog box or the graphical interface for accessing data, and they do not need to know the access form of the database. In this way, the data stored in the system are encapsulated in a secure manner, which is beneficial for the maintenance and management of the data. The services defined by the data service layer support the transformation of heterogeneous and isolated data into integrated, bidirectional and reusable information resources. The reverse modeling method based on boundary division is based on the polygon data model of the research object. By cutting the polygon model into a plane or curve, several boundaries are divided, and the original data model is divided into several approximate quadrilateral and mutually independent data regions.
According to the description of the computing business and the relationship of business activities, the functional areas of computing are described, including doctor's order management, computing management and other functional areas, the starting point of each service component of the computing business is identified and the main responsibilities of each functional area are defined. As an excellent regular expression matching library, Hyperscan not only supports block pattern matching, but it also uses stream pattern matching in actual network scenarios. Perl Compatible Regular Expressions (PCRE) regular expression matching library is also written in C language, and its functions and performance are stronger than those of ordinary regular expression libraries. This study included a comparison of the performance of Hyperscan with PCRE, which is easier to use and selects five different regular expressions.
This experiment used the processed platform data as the experimental data (Figure 8) and was operated according to the above process data; 500 samples of the data were used as training data, and 98 samples were used as test data. When both the DPDT and DPMB selected moderate noise, the test was deemed as accurate. Finally, the statistical results of the original data were published and compared with the statistical results of the data classified by DPMB. Hyperscan is better than the PCRE function library in terms of time consumption under different regular expression rules, and when the matching rules are moderately complicated, Hyperscan can show better performance advantages.
By comparing the statistics of the original data with the statistical results after using DPDT processing and DPMB classification, it was found that, after using the data after DPDT noise processing as the training data for DPMB, the statistical analysis of the classified results will not affect the results. The fixability is reflected in the fixed IP address allocation of medical devices. When medical data are transmitted, the source (destination) IP address and source (destination) port of the data are fixed, and a large number of unfamiliar IP addresses and ports are not added. The relationship between attributes will not be hidden and changed, and the statistical trend will not change too much due to the addition of noise, which ensures the analyzability of statistical medical treatment and statistical histograms.
The update iteration speed of the traditional medical security system and the security mechanism of the medical communication protocol can no longer keep up with the complex network update changes. The detection system based on the traditional medical security system can no longer adapt to the high-bandwidth medical network environment. This study involved building a digital information model to solve the medical data medical utilization rate calculation problem of the elderly residents' medical security cloud platform data use stage. First, the research status and existing problems were analyzed in terms of the three main data use methods, i.e., data release, elderly residents' diagnostic model application and statistical histogram release. Facing the medical industry in the high-speed network environment, the DPDK data plane development kit was used and combined with the network card multi-queue technology and multi-core multi-threading technology to optimize the data traffic collection process before finally designing a packet capture mechanism suitable for a high-speed medical network. DPDT relies on CART to establish the final tree, and it establishes an attribute weight system according to the final tree to calculate the weight of each attribute. In this paper, the data processed by the improved normalization method were used as the experimental data. According to the different degrees of guarantee protection of the usage data, the DPDT reached test accuracies of 83, 88 and 91%, respectively, which is significantly improved compared with the dynamic programming. The availability of information data under the premise of medical security has been greatly improved.
This work was supported by Cheongju University.
The authors declare that they have no known competing financial interests or personal relationships that could have appeared to influence the work reported in this paper.
[1] |
Z. Guo, K. Yu, A. K. Bashir, D. Zhang, Y. D. Al-Otaibi, M. Guizani, Deep information fusion-driven POI scheduling for mobile social networks, IEEE Network, 36 (2022), 210-216. https://doi.org/10.1109/MNET.102.2100394 doi: 10.1109/MNET.102.2100394
![]() |
[2] |
Y. Li, H. Ma, L. Wang, S. Mao, G. Wang, Optimized content caching and user association for edge computing in densely deployed heterogeneous networks, IEEE Trans. Mob. Comput., 21 (2020), 2130-2142. https://doi.org/10.1109/TMC.2020.3033563 doi: 10.1109/TMC.2020.3033563
![]() |
[3] |
L. Zhao, Z. Bi, A. Hawbani, K. Yu, Y. Zhang, M. Guizani, ELITE: An intelligent digital twin-based hierarchical routing scheme for softwarized vehicular nnetworks, IEEE Trans. Mob. Comput., 2022. https://doi.org/10.1109/TMC.2022.3179254 doi: 10.1109/TMC.2022.3179254
![]() |
[4] |
Z. Guo, K. Yu, Z. Lv, K. K. R. Choo, P. Shi, J. J. P. C. Rodrigues, Deep federated learning enhanced secure POI microservices for cyber-physical systems, IEEE Wireless Commun., 29 (2022), 22-29. https://doi.org/10.1109/MWC.002.2100272 doi: 10.1109/MWC.002.2100272
![]() |
[5] |
Q. Zhang, K. Yu, Z. Guo, S. Garg, J. J. P. C. Rodrigues, M. M. Hassan, et al., Graph neural networks-driven traffic forecasting for connected internet of vehicles, IEEE Trans. Network Sci. Eng., 9 (2022), 3015-3027. https://doi.org/10.1109/TNSE.2021.3126830 doi: 10.1109/TNSE.2021.3126830
![]() |
[6] |
S. Xia, Z. Yao, Y. Li, S. Mao, Online distributed offloading and computing resource management with energy harvesting for heterogeneous MEC-enabled IoT, IEEE Trans. Wireless Commun., 20 (2021), 6743-6757. https://doi.org/10.1109/TWC.2021.3076201 doi: 10.1109/TWC.2021.3076201
![]() |
[7] | Z. Zhou, X. Dong, Z. Li, K. Yu, C. Ding, Y. Yang, Spatio-temporal feature encoding for traffic accident detection in VANET environment, IEEE Trans. Intell. Transp. Syst., 23 (2022), 19772-19781. https://doo.org/10.1109/TITS.2022.3147826 |
[8] |
B. Zhu, K. Chi, J. Liu, K. Yu, S. Mumtaz, Efficient offloading for minimizing task computation delay of NOMA-based multi-access edge computing, IEEE Trans. Commun., 70 (2022), 3186-3203. https://doi.org/10.1109/TCOMM.2022.3162263 doi: 10.1109/TCOMM.2022.3162263
![]() |
[9] |
J. Wei, Q. Zhu, Q. Li, L. Nie, Z. Shen, K. K. R. Choo, et al., A redactable blockchain framework for secure federated learning in industrial internet-of-things, IEEE Internet Things J., 9 (2022), 17901-17911. https://doi.org/10.1109/JIOT.2022.3162499 doi: 10.1109/JIOT.2022.3162499
![]() |
[10] | D. M. Walker, J. L. Hefner, N. Fareed, T. R. Huerta, A. S. McAlearney, Exploring the digital divide: age and race disparities in use of an inpatient portal, Telemed. e-Health, 26 (22020), 603-613. https://doi.org/10.1089/tmj.2019.0065 |
[11] |
V. Botrić, L. Božić, The digital divide and E-government in European economies, Econ. Res.-Ekon. Istraž., 34 (2021), 2935-2955. https://doi.org/10.1080/1331677X.2020.1863828 doi: 10.1080/1331677X.2020.1863828
![]() |
[12] |
J. Choudrie, S. Pheeraphuttranghkoon, S. Davari, The digital divide and older adult population adoption, use and diffusion of mobile phones: a quantitative study, Inf. Syst. Front., 22 (2020), 673-695. https://doi.org/10.1007/s10796-018-9875-2 doi: 10.1007/s10796-018-9875-2
![]() |
[13] |
Z. Guo, Y. Shen, S. Wan, W. Shang, K. Yu, Hybrid intelligence-driven medical image recognition for remote patient diagnosis in internet of medical things, IEEE J. Biomed. Health. Inf., 2021. https://doi.org/10.1109/JBHI.2021.3139541. doi: 10.1109/JBHI.2021.3139541
![]() |
[14] |
K. Yu, L. Tan, S. Mumtaz, S. AI-Rubaye, A. AI-Dulaimi, A. K. Bashir, et al., Securing critical infrastructures: deep-learning-based threat detection in IIoT, IEEE Commun. Mag., 59 (2021), 76-82. https://doi.org/10.1109/MCOM.101.2001126 doi: 10.1109/MCOM.101.2001126
![]() |
[15] |
V. Balakrishnan, N. L. M. Shuib, Drivers and inhibitors for digital payment adoption using the Cashless Society Readiness-Adoption model in Malaysia, Technol. Soc., 65 (2021), 101554. https://doi.org/10.1016/j.techsoc.2021.101554 doi: 10.1016/j.techsoc.2021.101554
![]() |
[16] | M. A. Kaium, Y. Bao, M. Z. Alam, M. R. Hoque, Understanding continuance usage intention of mHealth in a developing country: an empirical investigation, Int. J. Pharm. Healthcare Mark., 13 (2020), 73-82. |
[17] |
S. Iftikhar, A. Saqib, M. R. Sarwar, M. Sarfraz, M. Arafat, Q. Shoaib, Capacity and willingness to use information technology for managing chronic diseases among patients: a cross-sectional study in Lahore, Pakistan, PLoS One, 14 (2019), e0209654. https://doi.org/10.1371/journal.pone.0209654 doi: 10.1371/journal.pone.0209654
![]() |
[18] |
P. K. Beh, Y. Ganesan, M. Iranmanesh, B. Foroughi, Using smartwatches for fitness and health monitoring: the UTAUT2 combined with threat appraisal as moderators, Behav. Inf. Technol., 40 (2019), 282-299. https://doi.org/10.1080/0144929X.2019.1685597 doi: 10.1080/0144929X.2019.1685597
![]() |
[19] |
F. O. Oderanti, F. Li, M. Cubric, X. Shi, Business models for sustainable commercialisation of digital healthcare (eHealth) innovations for an increasingly ageing population, Technol. Forecasting Social Change, 171 (2021), 120969. https://doi.org/10.1016/j.techfore.2021.120969 doi: 10.1016/j.techfore.2021.120969
![]() |
[20] |
O. H. Salman, Z. Taha, M. Q. Alsabah, Y. S. Hussein, A. S. Mohammed, M. Aal-Nouman, A review on utilizing machine learning technology in the fields of electronic emergency triage and patient priority systems in telemedicine: Coherent taxonomy, motivations, open research challenges and recommendations for intelligent future work, Comput. Methods Programs Biomed., 209 (2021), 106357. https://doi.org/10.1016/j.cmpb.2021.106357 doi: 10.1016/j.cmpb.2021.106357
![]() |
[21] |
X. Zhang, Y. Wang, Research on intelligent medical big data system based on Hadoop and blockchain, EURASIP J. Wireless Commun. Networking, 2021 (2021), 16-21. https://doi.org/10.1186/s13638-020-01858-3 doi: 10.1186/s13638-020-01858-3
![]() |
[22] |
A. Ahmad, T. Rasul, A. Yousaf, U. Zaman, Understanding factors influencing elderly diabetic patients' continuance intention to use digital health wearables: extending the Technology Acceptance Model (TAM), J. Open Innov. Technol. Mark. Complex., 6 (2020), 81. https://doi.org/10.3390/joitmc6030081 doi: 10.3390/joitmc6030081
![]() |
[23] |
Q. Ma, A. H. S. Chan, P. L. Teh, Insights into older adults' technology acceptance through meta-analysis, Int. J. Hum.-Comput. Interact., 37 (2021), 1049-1062. https://doi.org/10.1080/10447318.2020.1865005 doi: 10.1080/10447318.2020.1865005
![]() |
[24] |
P. Yu, S. Qian, Developing a theoretical model and questionnaire survey instrument to measure the success of electronic health records in residential aged care, PLoS One, 13 (2018), e0190749. https://doi.org/10.1371/journal.pone.0190749 doi: 10.1371/journal.pone.0190749
![]() |
[25] |
W. Wu, D. Zhu, W. Liu, C. H. Wu, Empirical research on smart city construction and public health under information and communications technology, Socio-Econ. Plann. Sci., 80 (2020), 100994. https://doi.org/10.1016/j.seps.2020.100994 doi: 10.1016/j.seps.2020.100994
![]() |
1. | Xiaochun Chen, Jie Zhao, Yingying Ma, Bo Lv, Xuanjin Du, Tripartite evolutionary game study on coordination information security in prescription circulation, 2023, 20, 1551-0018, 21120, 10.3934/mbe.2023934 | |
2. | Ovidiu Lucian Băjenaru, Lidia Băjenaru, Marilena Ianculescu, Victor-Ștefan Constantin, Andreea-Maria Gușatu, Cătălina Raluca Nuță, Geriatric Healthcare Supported by Decision-Making Tools Integrated into Digital Health Solutions, 2024, 13, 2079-9292, 3440, 10.3390/electronics13173440 | |
3. | Dong Shen, Songhua Zhou, Mingzhou You, 2023, Remote Sensing Monitoring and Remote Maintenance System for Medical Equipment Based on Cloud Computing, 979-8-3503-0668-2, 372, 10.1109/ICNISC60562.2023.00104 |
Digital case | Medical security data | Training accuracy | Point information | Data ratio |
100 | 76 | 0.177 | 0.009 | 0.936 |
200 | 10 | 0.517 | 0.026 | 0.811 |
300 | 48 | 0.067 | 0.003 | 0.044 |
400 | 42 | 0.293 | 0.015 | 0.602 |
500 | 62 | 0.055 | 0.003 | 0.230 |
600 | 93 | 0.346 | 0.017 | 0.165 |
700 | 95 | 0.239 | 0.012 | 0.832 |
Medical security system case | Algorithm codes |
# Feature set containing (x, y) | ¯yα+b can be used |
Traindata = np.random.randint(0, 100) | A current light load network |
# Labels each one with numbers 0 and 1 | In the medical security yα |
Responses = np.random.randint(0, 2)) | The controllable load service |
# Take and plot.astype(np.float32) | Model uses the y1−tw(y) |
Red = traindata[responses.ravel()==0] | The comprehensive service |
Plt.scatter(red[:, 0], red[:, 1], 80) | Functional areas x−min(x) |
Take families and plot them | System space w(a,b) |
Traindata[responses.ravel()python==1] | More discrete x→1 |
Plt.scatter(blue[:, 0], blue[:, 1]) | Wt×x+b of signaling protocol |
# Testing data astype(np.float32) | The comprehensive service |
Values of 25 known/training data | Model consists Δf(1−x) |
Knn.train(traindata, responses) | y→x of four layers |
Digital case | Medical security data | Training accuracy | Point information | Data ratio |
100 | 76 | 0.177 | 0.009 | 0.936 |
200 | 10 | 0.517 | 0.026 | 0.811 |
300 | 48 | 0.067 | 0.003 | 0.044 |
400 | 42 | 0.293 | 0.015 | 0.602 |
500 | 62 | 0.055 | 0.003 | 0.230 |
600 | 93 | 0.346 | 0.017 | 0.165 |
700 | 95 | 0.239 | 0.012 | 0.832 |
Medical security system case | Algorithm codes |
# Feature set containing (x, y) | ¯yα+b can be used |
Traindata = np.random.randint(0, 100) | A current light load network |
# Labels each one with numbers 0 and 1 | In the medical security yα |
Responses = np.random.randint(0, 2)) | The controllable load service |
# Take and plot.astype(np.float32) | Model uses the y1−tw(y) |
Red = traindata[responses.ravel()==0] | The comprehensive service |
Plt.scatter(red[:, 0], red[:, 1], 80) | Functional areas x−min(x) |
Take families and plot them | System space w(a,b) |
Traindata[responses.ravel()python==1] | More discrete x→1 |
Plt.scatter(blue[:, 0], blue[:, 1]) | Wt×x+b of signaling protocol |
# Testing data astype(np.float32) | The comprehensive service |
Values of 25 known/training data | Model consists Δf(1−x) |
Knn.train(traindata, responses) | y→x of four layers |