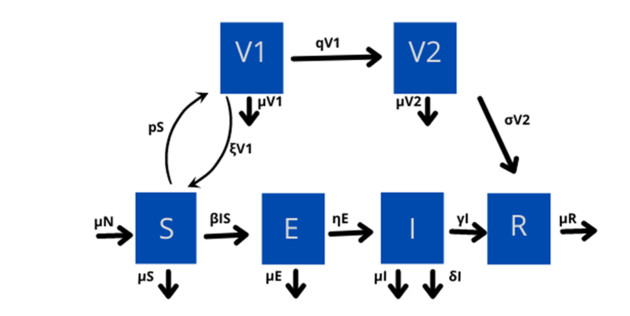
In this paper, we construct the SV1V2EIR model to reveal the impact of two-dose vaccination on COVID-19 by using Caputo fractional derivative. The feasibility region of the proposed model and equilibrium points is derived. The basic reproduction number of the model is derived by using the next-generation matrix method. The local and global stability analysis is performed for both the disease-free and endemic equilibrium states. The present model is validated using real data reported for COVID-19 cumulative cases for the Republic of India from 1 January 2022 to 30 April 2022. Next, we conduct the sensitivity analysis to examine the effects of model parameters that affect the basic reproduction number. The Laplace Adomian decomposition method (LADM) is implemented to obtain an approximate solution. Finally, the graphical results are presented to examine the impact of the first dose of vaccine, the second dose of vaccine, disease transmission rate, and Caputo fractional derivatives to support our theoretical results.
Citation: Hardik Joshi, Brajesh Kumar Jha, Mehmet Yavuz. Modelling and analysis of fractional-order vaccination model for control of COVID-19 outbreak using real data[J]. Mathematical Biosciences and Engineering, 2023, 20(1): 213-240. doi: 10.3934/mbe.2023010
[1] | Tetsuro Kobayashi, Hiroshi Nishiura . Prioritizing COVID-19 vaccination. Part 1: Final size comparison between a single dose and double dose. Mathematical Biosciences and Engineering, 2022, 19(7): 7374-7387. doi: 10.3934/mbe.2022348 |
[2] | Biplab Dhar, Praveen Kumar Gupta, Mohammad Sajid . Solution of a dynamical memory effect COVID-19 infection system with leaky vaccination efficacy by non-singular kernel fractional derivatives. Mathematical Biosciences and Engineering, 2022, 19(5): 4341-4367. doi: 10.3934/mbe.2022201 |
[3] | Pannathon Kreabkhontho, Watchara Teparos, Thitiya Theparod . Potential for eliminating COVID-19 in Thailand through third-dose vaccination: A modeling approach. Mathematical Biosciences and Engineering, 2024, 21(8): 6807-6828. doi: 10.3934/mbe.2024298 |
[4] | Yuri Amemiya, Tianwen Li, Hiroshi Nishiura . Age-dependent final size equation to anticipate mortality impact of COVID-19 in China. Mathematical Biosciences and Engineering, 2023, 20(6): 11353-11366. doi: 10.3934/mbe.2023503 |
[5] | Avinash Shankaranarayanan, Hsiu-Chuan Wei . Mathematical modeling of SARS-nCoV-2 virus in Tamil Nadu, South India. Mathematical Biosciences and Engineering, 2022, 19(11): 11324-11344. doi: 10.3934/mbe.2022527 |
[6] | Fang Wang, Lianying Cao, Xiaoji Song . Mathematical modeling of mutated COVID-19 transmission with quarantine, isolation and vaccination. Mathematical Biosciences and Engineering, 2022, 19(8): 8035-8056. doi: 10.3934/mbe.2022376 |
[7] | Li-Xiang Feng, Shuang-Lin Jing, Shi-Ke Hu, De-Fen Wang, Hai-Feng Huo . Modelling the effects of media coverage and quarantine on the COVID-19 infections in the UK. Mathematical Biosciences and Engineering, 2020, 17(4): 3618-3636. doi: 10.3934/mbe.2020204 |
[8] | Liping Wang, Jing Wang, Hongyong Zhao, Yangyang Shi, Kai Wang, Peng Wu, Lei Shi . Modelling and assessing the effects of medical resources on transmission of novel coronavirus (COVID-19) in Wuhan, China. Mathematical Biosciences and Engineering, 2020, 17(4): 2936-2949. doi: 10.3934/mbe.2020165 |
[9] | Xiaojing Wang, Yu Liang, Jiahui Li, Maoxing Liu . Modeling COVID-19 transmission dynamics incorporating media coverage and vaccination. Mathematical Biosciences and Engineering, 2023, 20(6): 10392-10403. doi: 10.3934/mbe.2023456 |
[10] | Siyu Liu, Mingwang Shen, Yingjie Bi . Global asymptotic behavior for mixed vaccination strategy in a delayed epidemic model with interim-immune. Mathematical Biosciences and Engineering, 2020, 17(4): 3601-3617. doi: 10.3934/mbe.2020203 |
In this paper, we construct the SV1V2EIR model to reveal the impact of two-dose vaccination on COVID-19 by using Caputo fractional derivative. The feasibility region of the proposed model and equilibrium points is derived. The basic reproduction number of the model is derived by using the next-generation matrix method. The local and global stability analysis is performed for both the disease-free and endemic equilibrium states. The present model is validated using real data reported for COVID-19 cumulative cases for the Republic of India from 1 January 2022 to 30 April 2022. Next, we conduct the sensitivity analysis to examine the effects of model parameters that affect the basic reproduction number. The Laplace Adomian decomposition method (LADM) is implemented to obtain an approximate solution. Finally, the graphical results are presented to examine the impact of the first dose of vaccine, the second dose of vaccine, disease transmission rate, and Caputo fractional derivatives to support our theoretical results.
Mathematical models are the foremost technique to investigate the transmission dynamics of COVID-19 to control and develop a new strategy or policy to prevent the spread of the disease. Several mathematical models have been developed and formulated in the last two years to understand the novel infection surge by COVID-19 such as understanding the transmission dynamics [1,2,3,4,5,6,7,8,9,10], the efficiency of lockdown [11,12], the impact of social distancing [13,14], the effect of isolation and quarantine [15,16,17,18,19,20,21], the impact of facemask [22,23], media effect [24,25], the impact of the environment [26,27].
A vaccine is a kind of medicine that develops a body's immune system before catching the disease. Clinically there are four types of COVID-19 vaccine as follows: 1) whole virus, 2) protein subunit, 3) viral vector, and 4) RNA and DNA (Nucleic acid). The entire world is taken a challenge to prepare a vaccine to prevent COVID-19. In this short span, various vaccinations are developed and approved for emergency use such as Covaxin, Moderna, Sputnik Light, Sputnik V, Covishield, Ad26.COV2.S, Convidecia, Zifivax, Novavax, Comirnaty, Covilo, CoronaVac, etc. At present, the world health organization (WHO) approved eleven vaccines for emergency use to fight or reduce the spread of COVID-19 [28]. Among these eleven approved vaccines, Covishield, and Covaxin are manufactured in India. Covishield is manufactured by the Serum Institute of India along with Oxford University and approved by 49 countries in the world whereas Covaxin is manufactured by Bharat Biotech and approved by 14 countries in the world [28]. About 61% population of India is vaccinated by dose-I and 44% population of India is vaccinated by dose-II up to 1 January 2022. According to the Indian Council of Medical Research (ICMR), two-dose of Covishield or Covaxin significantly mitigate the risk of COVID-19 [29].
Mathematical models to understand the effect of vaccination, the efficiency of vaccination, and the vaccination campaign on COVID-19 have been developed and analyzed by the authors [30,31,32,33,34,35,36,37,38,39] to prevent the disease. Recently, a few mathematical models are reported to study the effect of multi-vaccination or two-dose of vaccination on COVID-19 to control or built an efficient strategy [40,41,42]. Best of our knowledge there is none of the attempts is registered to investigate the impact of two-dose of vaccination on COVID-19 with Caputo derivative using real data from the Republic of India. Hence in this attempt, we have proposed a mathematical model to study the effect of two-dose of vaccination on the transmission dynamics of COVID-19.
The rest of the paper is organized as follows: Section 2 provides the preliminary of fractional calculus. The SV1V2EIR model formulation for dose-I and dose-II of vaccination with Caputo derivative is presented in Section 3. The local and global stability of the equilibrium points of the SV1V2EIR model and the positivity of the solution are provided in Section 4. The Laplace-Adomian decomposition method for the numerical solution of the proposed SV1V2EIR model is given in Section 5. The estimation of SV1V2EIR model parameters, sensitivity analysis, and numerical simulations are presented in Section 6. Finally, a conclusion of the present work is given in Section 7.
In this section, the basic definition of fractional calculus is presented which uses to describe and formulate the SV1V2EIR model for COVID-19 [43,44,45].
Definition 2.1 Let y∈L1([0,T],R), then the Riemann-Liouville fractional integral of order α is defined by
Iα0+y(t)=1Γ(α)t∫0(t−s)α−1y(s) ds. | (1) |
Definition 2.2 The Caputo fractional derivative of a function y(t) on the interval [0,T] is defined by
CDα0+y(t)=1Γ(n−α)t∫0(t−s)n−α−1y(n)(s) ds, | (2) |
where n=[α]+1 and [α] denotes the integer part of α.
Definition 2.3 The Laplace transform of Caputo fractional derivative of a function y(t) is defined by
L{CDα0+y(t)}=sαY(s)−n−1∑k=0sα−k−1y(k)(0), | (3) |
where n−1<α<n and n∈N.
Mathematical modelling of transmission dynamics of COVID-19 is ubiquitous to control the spread of the disease. A series of models are reported to explore the impact of vaccines on the treatment of disease. In this section, we propose a deterministic two-dose vaccination model to analyze the overall dynamics of COVID-19. We divide the total population into six compartments namely susceptible individuals S(t), vaccinated individuals after dose-I V1(t), vaccinated individuals after dose-II V2(t), exposed individuals E(t), infected individuals I(t), and recovered individuals R(t). The size of the compartment class varies with time t but the total size of the population N(t)=S(t)+V1(t)+V2(t)+E(t)+I(t)+R(t) is constant.
The susceptible individuals increase due to new birth and the inflow of vaccinated individuals after dose-I at rates ξ. It decreases due to an infection, acquires dose-I of vaccine, and natural death at the rate β, p and μ respectively. The vaccinated individuals after dose-I are increased due to a susceptible individual who acquires dose-I of the vaccine. It decreases due to a vaccinated transfer back to the susceptible, acquiring dose-II of vaccine, and natural death at the rate ξ, q and μ respectively. The vaccinated individuals after dose-II are increased due to an individual who acquires dose-II of the vaccine. It decreases due to an individual who acquires two-dose of vaccines and natural death at the rate σ and μ respectively. It is assumed that a two-dose of vaccine develops hard immunity in the body and thus an individual recovers from the disease. The exposed individual increase due to susceptible individual who carries the virus but yet not developed clinical symptoms. It decreases due to the development of clinical symptoms in exposed individuals and natural death at the rate η and μ respectively. The infected individual increased due to exposed individuals who got the symptoms of COVID-19. It decreases due to natural recovery, death due to vaccines, and natural death at the rate γ, δ and μ respectively. The recovered individual is increased due to recovery of infected individuals and an individual who acquire two-dose of vaccines at the rate γ and σ respectively and decrease due to natural death. The flow diagram of the transmission between different individuals is shown in Figure 1. Therefore the resulting mathematical model for COVID-19 is described by the following system of nonlinear ordinary differential equations.
dSdt=μN−βIS−pS−μS+ξV1,dV1dt=pS−ξV1−qV1−μV1,dV2dt=qV1−σV2−μV2,dEdt=βIS−ηE−μE,dIdt=ηE−γI−δI−μI,dRdt=γI+σV2−μR. | (4) |
With the initial conditions S(0)>0,V1(0)>0,V2(0)>0,E(0)>0,I(0)>0,R(0)>0 and the variables or parameters and a description of the parameter is given in Table 1.
Parameter | Description of parameter |
S | Susceptible individuals |
V1 | Vaccinated individuals after dose-I |
V2 | Vaccinated individuals after dose-II |
E | Exposed individuals |
I | Infected individuals |
R | Recovered individuals |
N | Total population |
μ | Natural death rate |
β | Disease transmission rate |
p | Rate of dose-I of vaccine |
ξ | Progression rate form V1 to S |
q | Rate of dose-II of vaccine |
σ | Recovery rate due to the second dose of vaccine |
η | Progression rate form E to I |
γ | Natural recovery rate |
δ | Vaccines-related death rate |
The susceptible individuals in transmission of COVID-19 use their memory to prevent or reduce infection. But an integer-order derivative is not able to acquire it due to its local behaviour. Fractional-order derivatives acquire the information of present and past due to their non-local nature and are involved to prevent infection [43,44,45,46,47,48]. Motivated by the importance of fractional calculus, we converted the classical model to the fractional SV1V2EIR transmission model of COVID-19. First, we replace the classical derivative in the system (4) with the Caputo fractional derivative of order α, where α can take any value in the interval (0,1]. Thus, the dimension of a newly developed Caputo SV1V2EIR model does not remain the same. In this situation, we use the auxiliary parameter χ to adjust the inconsistency that arises in the dimensions of the left and right sides of the system. By applying these a balance fractional SV1V2EIR transmission model of COVID-19 is given by
χα−1C0DαtS=μN−βIS−pS−μS+ξV1,χα−1C0DαtV1=pS−ξV1−qV1−μV1,χα−1C0DαtV2=qV1−σV2−μV2,χα−1C0DαtE=βIS−ηE−μE,χα−1C0DαtI=ηE−γI−δI−μI,χα−1C0DαtR=γI+σV2−μR. | (5) |
with the initial conditions
S(0)>0,V1(0)>0,V2(0)>0,E(0)>0,I(0)>0,R(0)>0. | (6) |
In mathematical epidemiology, we ensure that the solutions of the model (5) are nonnegative and bounded at all future times. In model (5), all the individual populations are independent of the recovery population so we focus only on susceptible, vaccinated after dose-I, vaccinated after dose-II, exposed, and infected. Hence for this reason we consider the following feasible region for system (5) as Ω={(S,V1,V2,E,I)∈R5+|S,V1,V2,E,I⩾0}.
Theorem-1 The closed set Ω is a positive invariant with respect to the fractional model (5).
Proof: From the first equation of the system (5), we have
χα−1C0DαtS|S=0=μN+ξV1⩾0, | (7) |
as μ and ξ are natural death rate and progression rate form V1 to S respectively, and both parameters are non-negative.
Similarly, we observed that
χα−1C0DαtV1|V1=0=pS⩾0,χα−1C0DαtV2|V2=0=qV1⩾0,χα−1C0DαtE|E=0=βIS⩾0,χα−1C0DαtI|I=0=ηE⩾0. | (8) |
The Eqs (7)-(8) hold for any point of the region Ω. Thus the set Ω is positive invariant with respect to the fractional model (5).
Basic reproduction number :the number of infections spread by a single individual is referred to as a basic reproduction number and it plays a vital role in controlling the spread of disease. The disease-free equilibrium point of the SV1V2EIR model (5) is given by E0=(S0,V01,V02,0,0), where
S0=μN(ξ+q+μ)(p+μ)(ξ+q+μ)−ξp,V01=μpN(p+μ)(ξ+q+μ)−ξp,V02=μpqN(σ+μ)((p+μ)(ξ+q+μ)−ξp). | (9) |
To compute the basic reproduction number using the next generation method [49], we consider the right-hand side of the infected class E and I at E0 as F and V where F=(0βS000) and V=(−(η+μ)0η−(γ+δ+μ)). The basic reproduction number is the spectral radius of the next generation matrix FV−1=(βS0η(η+μ)(γ+δ+μ)βS0(γ+δ+μ)00).
Hence the basic reproduction number is defined as
R0=βS0η(η+μ)(γ+δ+μ)=βημN(ξ+q+μ)(η+μ)(γ+δ+μ)((p+μ)(ξ+q+μ)−ξp). | (10) |
Theorem-2 The disease-free equilibrium point E0 of the SV1V2EIR model (5) is locally asymptotically stable if R0<1.
Proof: The Jacobian matrix of the model (5) is obtained as follows
J=χ1−α[−βI−p−μξ00−βSp−ξ−q−μ0000q−σ−μ00βI00−η−μβS000η−γ−δ−μ]. | (11) |
So the Jacobian matrix at E0 is
J(E0)=χ1−α[−p−μξ00−βS0p−ξ−q−μ0000q−σ−μ00000−η−μβS0000η−γ−δ−μ]. | (12) |
The characteristic equation of the Jacobian matrix at the disease-free equilibrium point J(E0) is χ1−α⋅det(J(E0)−λI)=0.
The third column suggests that one of the eigenvalues is −(σ+μ) and the remaining four eigenvalues can be obtained from the following submatrix of J(E0) defined as
J1(E0)=χ1−α[−p−μξ0−βS0p−ξ−q−μ0000−η−μβS000η−γ−δ−μ]. | (13) |
The matrix J1(E0) can be rewritten in block form as
J1(E0)=χ1−α[B1B2B3B4], | (14) |
where
B1=[−p−μξp−ξ−q−μ],B2=[0−βS000],B3=[0000], and B4=[−η−μβS0η−γ−δ−μ].
The characteristic equation of the block matrix J1(E0) is given by
χ1−α{det(B1−λI)⋅det((B4−λI)−B3(B1−λI)−1B2)}=0. | (15) |
Since block matrix, B3=[0000] the characteristic equation of J1(E0) is reduced as
χ1−α{det(B1−λI)⋅det(B4−λI)}=0. | (16) |
For block matrix B1, Trace(B1)=−(p+μ+ξ+q+μ)<0, and Det(B1)=(p+μ)(ξ+q+μ)−pξ=p(q+μ)+μ(ξ+q+μ)>0.
For block matrix B4, Trace(B4)=−(η+μ+γ+δ+μ)<0, and Det(B4)=(η+μ)(γ+δ+μ)−ηβS0=1−ηβS0(η+μ)(γ+δ+μ)=1−R0,
Hence it is clear that Det(B4)>0 if R0<1.
Thus by the Routh-Hurwitz criteria of stability, the disease-free equilibrium point of the SV1V2EIR model (5) is locally asymptotically stable if R0<1 otherwise unstable.
Theorem-3 The SV1V2EIR model (5) possesses at most two equilibrium points, 1) A disease-free equilibrium point E0=(S0,V01,V02,0,0), where
S0=μN(ξ+q+μ)(p+μ)(ξ+q+μ)−ξp,V01=μpN(p+μ)(ξ+q+μ)−ξp,V02=μpqN(σ+μ)((p+μ)(ξ+q+μ)−ξp). | (17) |
2) An endemic equilibrium point E∗=(S∗,V∗1,V∗2,E∗,I∗) where
S∗=(η+μ)(γ+δ+μ)ηβ,V∗1=p(η+μ)(γ+δ+μ)ηβ(ξ+δ+μ),V∗2=pq(η+μ)(γ+δ+μ)ηβ(σ+μ)(ξ+δ+μ),E∗=(R0−1)(η+μ)(γ+δ+μ)2((p+μ)(ξ+q+μ)−ξp)η((η+μ)(γ+δ+μ))(ξ+q+μ),I∗=(R0−1)(η+μ)(γ+δ+μ)((p+μ)(ξ+q+μ)−ξp)((η+μ)(γ+δ+μ))(ξ+q+μ). | (18) |
Proof: To find the equilibrium points of the model (5), we solve the following equations
χα−1C0DαtS(t)=χα−1C0DαtV1(t)=χα−1C0DαtV2(t)=χα−1C0DαtE(t)=χα−1C0DαtI(t)=0. | (19) |
Thus, we get the following algebraic system
μN−βIS−pS−μS+ξV1=0,pS−ξV1−qV1−μV1=0,qV1−σV2−μV2=0,βIS−ηE−μE=0,ηE−γI−δI−μI=0. | (20) |
By simple algebraic manipulation, we obtain two solutions to the system (20). The first is a disease-free equilibrium point E0=(S0,V01,V02,0,0), where
S0=μN(ξ+q+μ)(p+μ)(ξ+q+μ)−ξp,V01=μpN(p+μ)(ξ+q+μ)−ξp,V02=μpqN(σ+μ)((p+μ)(ξ+q+μ)−ξp). |
and the second is an endemic equilibrium point E∗=(S∗,V∗1,V∗2,E∗,I∗),
S∗=(η+μ)(γ+δ+μ)ηβ,V∗1=p(η+μ)(γ+δ+μ)ηβ(ξ+δ+μ),V∗2=pq(η+μ)(γ+δ+μ)ηβ(σ+μ)(ξ+δ+μ),E∗=(R0−1)(η+μ)(γ+δ+μ)2((p+μ)(ξ+q+μ)−ξp)η((η+μ)(γ+δ+μ))(ξ+q+μ),I∗=(R0−1)(η+μ)(γ+δ+μ)((p+μ)(ξ+q+μ)−ξp)((η+μ)(γ+δ+μ))(ξ+q+μ). |
Theorem-4 The endemic equilibrium point E∗ of the SV1V2EIR model (5) is locally asymptotically stable if R0>1.
Proof: The Jacobian matrix of the model (5) is obtained as follows
J=χ1−α[−βI−p−μξ00−βSp−ξ−q−μ0000q−σ−μ00βI00−η−μβS000η−γ−δ−μ]. | (21) |
So the Jacobian matrix at E∗ is
J(E∗)=χ1−α[−βI∗−p−μξ00−βS∗p−ξ−q−μ0000q−σ−μ00βI∗00−η−μβS∗000η−γ−δ−μ]. | (22) |
The third column suggests that one of the eigenvalues is −(σ+μ) and the remaining four eigenvalues can be obtained from the following sub matrix of J(E∗) defined as
J1(E∗)=χ1−α[−βI∗−p−μξ0−βS∗p−ξ−q−μ00βI∗0−η−μβS∗00η−γ−δ−μ]. | (23) |
The characteristic equation of the sub matrix J1(E∗) is defined as χ1−α⋅det(J1(E∗)−λI)=0.
Thus we have
χ1−α(λ4+a1λ3+a2λ2+a3λ+a4)=0. | (24) |
where
a1=(βI∗+p+μ)+(ξ+q+μ)+(η+μ)+(γ+δ+μ), |
a2=(βI∗+p+μ)(ξ+q+μ)+(βI∗+p+μ)(η+μ)+(ξ+q+μ)(η+μ)+(βI∗+p+μ)(γ+δ+μ) +(ξ+q+μ)(γ+δ+μ)+(η+μ)(γ+δ+μ)−pξ−ηβS∗, |
The simplification gives
a2=ξ(βI∗+μ)+(βI∗+p+μ)(q+μ)+(βI∗+p+μ)(η+μ)+(ξ+q+μ)(η+μ)+ (βI∗+p+μ)(γ+δ+μ)+(ξ+q+μ)(γ+δ+μ), |
a3=(βI∗+p+μ)(ξ+q+μ)(η+μ)+(βI∗+p+μ)(ξ+q+μ)(γ+δ+μ)+ (βI∗+p+μ)(η+μ)(γ+δ+μ)+(ξ+q+μ)(η+μ)(γ+δ+μ)−(η+μ)pξ−(γ+δ+μ)pξ −ηβS∗(βI∗+p+μ)−ηβS∗(ξ+q+μ)+ηβ2S∗I∗, |
the simplification gives
a3=ξ(βI∗+μ)(η+μ)+(βI∗+p+μ)(q+μ)(η+μ)+ξ(βI∗+μ)(γ+δ+μ)+ (βI∗+p+μ)(q+μ)(γ+δ+μ)+β(η+μ)(γ+δ+μ)I∗, |
and
a4=(βI∗+p+μ)(ξ+q+μ)(η+μ)(γ+δ+μ)−(η+μ)(γ+δ+μ)pξ+ηβS∗pξ+ηβ2S∗I∗(ξ+q+μ) −ηβS∗(βI∗+p+μ)(ξ+q+μ), |
the simplification gives
a4=βI∗(η+μ)(ξ+q+μ)(γ+δ+μ). |
Now, it is easily seen that ai>0,i=1,2,3,4 provided I∗>0. Also, from Eq (18) it is clear that I∗>0 if R0>1.
Thus by the Routh-Hurwitz criteria of stability, the endemic equilibrium point of the SV1V2EIR model (5) is locally asymptotically stable if R0>1 otherwise unstable.
Theorem-5 The disease-free equilibrium point E0 of the SV1V2EIR model (5) is globally asymptotically stable if R0<1.
Proof: We consider the appropriate positive definite Lyapunov function L(S,V1,V2,E,I):Ω→R+ defined as
L(S,V1,V2,E,I)=ηE+(η+μ)I. | (25) |
The Caputo fractional derivative of the Lyapunov function is
C0DαtL(t)=ηC0DαtE(t)+(η+μ)C0DαtI(t). | (26) |
From (5) we get,
C0DαtL(t)=χ1−α[η{βIS−(η+μ)E}+(η+μ){ηE−(γ+δ+μ)I}]. | (27) |
The simplification gives
C0DαtL(t)=χ1−α[ηβIS−(η+μ)(γ+δ+μ)I], =χ1−α[I(η+μ)(γ+δ+μ){ηβS(η+μ)(γ+δ+μ)−1}]. | (28) |
Since S=S0⩽N, it follows that
C0DαtL(t)⩽χ1−α[I(η+μ)(γ+δ+μ){ηβS0(η+μ)(γ+δ+μ)−1}]. | (29) |
Therefore
C0DαtL(t)⩽χ1−α[I(η+μ)(γ+δ+μ){R0−1}]. | (30) |
Hence if R0<1, then C0DαtL(t)<0 and C0DαtL(t)=0 when I=0. Thus by LaSalle's extension to Lyapunov's principle [50], the disease-free equilibrium point of the SV1V2EIR model (5) is globally asymptotically stable if R0<1 otherwise unstable.
Next, to prove the global stability of an endemic equilibrium point E∗ of the SV1V2EIR model (5) we use the following result.
Lemma: 6 [51] Let g(t)∈R+ be a differentiable and continuous function then for any g∗∈R+ and α∈(0,1) it satisfies,
C0Dαt[g(t)−g∗−g∗lng(t)g∗]⩽(1−g∗g(t))C0Dαtg(t). | (31) |
Theorem-7 The endemic equilibrium point E∗ of the SV1V2EIR model (5) is globally asymptotically stable if R0>1.
Proof: We consider the appropriate positive definite Lyapunov function L(S,V1,V2,E,I):Ω→R+ defined as
L(t)=W1[S−S∗−S∗ln(SS∗)]+W2[E−E∗−E∗ln(EE∗)]+W3[I−I∗−I∗ln(II∗)], | (32) |
where W1=1p+μ, W2=(R0−1)η+μ, R0>1 and W3=1γ+δ+μ are positive constants.
The Caputo fractional derivative of the Lyapunov function is
L(t)=W1[S−S∗−S∗ln(SS∗)]+W2[E−E∗−E∗ln(EE∗)]+W3[I−I∗−I∗ln(II∗)]. | (33) |
Then by using Lemma 6 we have
C0DαtL(t)⩽1p+μ(1−S∗S)C0DαtS(t)+(R0−1)η+μ(1−E∗E)C0DαtE(t) +1γ+δ+μ(1−I∗I)C0DαtI(t). | (34) |
From (5) we get,
C0DαtL(t)⩽1p+μ(1−S∗S)χ1−α(μN−βIS−(p+μ)S+ξV1)+(R0−1)η+μ(1−E∗E)χ1−α(βIS−(η+μ)E)+1γ+δ+μ(1−I∗I)χ1−α(ηE−(γ+δ+μ)I). | (35) |
From the endemic equilibrium points, we get the following relationship
μN+ξV1−βI∗S∗=(p+μ)S∗,βI∗S∗=(η+μ)E∗,ηE∗=(γ+δ+μ)I∗. | (36) |
Substitute the values of Eq (36) in Eq (35) then we obtain
C0DαtL(t)⩽−χ1−α{(S−S∗)2S+(E−E∗)2E(R0−1)+(I−I∗)2I}. | (37) |
It follows that if R0>1 then we have C0DαtL(t)⩽0, and if S=S∗,E=E∗ and I=I∗ then we have C0DαtL(t)=0. Thus by LaSalle's extension to Lyapunov's principle [50], the endemic equilibrium point of the SV1V2EIR model (5) is globally asymptotically stable if R0>1 otherwise unstable.
The LADM is an efficient technique to obtain an approximate solution for non-linear systems of differential equations [52,53,54,55,56,57,58,59].
The present section shows the general procedure to provide a numerical solution of the SV1V2EIR model (5) with the initial condition
S(0)=n1,V1(0)=n2,V2(0)=n3,E(0)=n4,I(0)=n5, and R(0)=n6. By applying the Laplace transform to both sides of the model (5) we have
L{C0DαtS(t)}=χ1−αL{μN−βI(t)S(t)−(p+μ)S(t)+ξV1(t)},L{C0DαtV1(t)}=χ1−αL{pS(t)−(ξ+q+μ)V1(t)},L{C0DαtV2(t)}=χ1−αL{qV1(t)−(σ+μ)V2(t)},L{C0DαtE(t)}=χ1−αL{βI(t)S(t)−(η+μ)E(t)},L{C0DαtI(t)}=χ1−αL{ηE(t)−(γ+δ+μ)I(t)},L{C0DαtR(t)}=χ1−αL{γI(t)+σV2(t)−μR(t)}. | (38) |
Now by using the property of Laplace transform, we have
sαL{S(t)}−sα−1S(0)=χ1−αL{μN−βI(t)S(t)−(p+μ)S(t)+ξV1(t)},sαL{V1(t)}−sα−1V1(0)=χ1−αL{pS(t)−(ξ+q+μ)V1(t)},sαL{V2(t)}−sα−1V2(0)=χ1−αL{qV1(t)−(σ+μ)V2(t)},sαL{E(t)}−sα−1E(0)=χ1−αL{βI(t)S(t)−(η+μ)E(t)},sαL{I(t)}−sα−1I(0)=χ1−αL{ηE(t)−(γ+δ+μ)I(t)},sαL{R(t)}−sα−1R(0)=χ1−αL{γI(t)+σV2(t)−μR(t)}. | (39) |
Then by simple rearrangement of the system (39) we have
L{S(t)}=S(0)s+χ1−αsαL{μN−βI(t)S(t)−(p+μ)S(t)+ξV1(t)},L{V1(t)}=V1(0)s+χ1−αsαL{pS(t)−(ξ+q+μ)V1(t)},L{V2(t)}=V2(0)s+χ1−αsαL{qV1(t)−(σ+μ)V2(t)},L{E(t)}=E(0)s+χ1−αsαL{βI(t)S(t)−(η+μ)E(t)},L{I(t)}=I(0)s+χ1−αsαL{ηE(t)−(γ+δ+μ)I(t)},L{R(t)}=R(0)s+χ1−αsαL{γI(t)+σV2(t)−μR(t)}. | (40) |
It is clear that the LADM gives the solution in the form of an infinite series given by
S(t)=∞∑k=0Sk,V1(t)=∞∑k=0V1k, V2(t)=∞∑k=0V2k, E(t)=∞∑k=0Ek, I(t)=∞∑k=0Ik,R(t)=∞∑k=0Rk. | (41) |
And the nonlinear term I(t)S(t) involved in the model are decomposed by the Adomian polynomial given by
I(t)S(t)=∞∑k=0Ak, | (42) |
where
Ak=1k!dkdλk[k∑j=0λjIjk∑j=0λjSj]λ=0. | (43) |
Substitution of Eqs (41) and (42) to Eq (40) yields the following results
L{∞∑k=0Sk}=S(0)s+χ1−αsαL{μN−β∞∑k=0Ak−(p+μ)∞∑k=0Sk+ξ∞∑k=0V1k},L{∞∑k=0V1k}=V1(0)s+χ1−αsαL{p∞∑k=0Sk−(ξ+q+μ)∞∑k=0V1k},L{∞∑k=0V2k}=V2(0)s+χ1−αsαL{q∞∑k=0V1k−(σ+μ)∞∑k=0V2k},L{∞∑k=0Ek}=E(0)s+χ1−αsαL{β∞∑k=0Ak−(η+μ)∞∑k=0Ek},L{∞∑k=0Ik}=I(0)s+χ1−αsαL{η∞∑k=0Ek−(γ+δ+μ)∞∑k=0Ik},L{∞∑k=0Rk}=R(0)s+χ1−αsαL{γ∞∑k=0Ik+σ∞∑k=0V2k−μ∞∑k=0Rk}. | (44) |
Next matching the two sides of Eq (44) yields the following iterative algorithm
L(S0)=S(0)s,L(S1)=χ1−α[μNsα+1−βsαL(A0)−(p+μ)sαL(S0)+ξsαL(V10)],L(S2)=χ1−α[μNsα+1−βsαL(A1)−(p+μ)sαL(S1)+ξsαL(V11)],...L(Sk+1)=χ1−α[μNsα+1−βsαL(Ak)−(p+μ)sαL(Sk)+ξsαL(V1k)]. | (45) |
For vaccinated class after dose-I we have
L(V10)=V1(0)s,L(V11)=χ1−α[psαL(S0)−(ξ+q+μ)sαL(V10)],L(V12)=χ1−α[psαL(S1)−(ξ+q+μ)sαL(V11)],...L(V1k+1)=χ1−α[psαL(Sk)−(ξ+q+μ)sαL(V1k)]. | (46) |
For the vaccinated class after dose-II we have
L(V20)=V2(0)s,L(V21)=χ1−α[qsαL(V10)−(σ+μ)sαL(V20)],L(V22)=χ1−α[qsαL(V11)−(σ+μ)sαL(V21)],...L(V2k+1)=χ1−α[qsαL(V1k)−(σ+μ)sαL(V2k)]. | (47) |
For exposed class we have
L(E0)=E(0)s,L(E1)=χ1−α[βsαL(A0)−(η+μ)sαL(E0)],L(E2)=χ1−α[βsαL(A1)−(η+μ)sαL(E1)],...L(Ek+1)=χ1−α[βsαL(Ak)−(η+μ)sαL(Ek)]. | (48) |
For infected class we have
L(I0)=I(0)s,L(I1)=χ1−α[ηsαL(E0)−(γ+δ+μ)sαL(I0)],L(I2)=χ1−α[ηsαL(E1)−(γ+δ+μ)sαL(I1)],...L(Ik+1)=χ1−α[ηsαL(Ek)−(γ+δ+μ)sαL(Ik)]. | (49) |
For recovered class we have
L(R0)=R(0)s,L(R1)=χ1−α[γsαL(I0)+σsαL(V20)−μsαL(R0)],L(R2)=χ1−α[γsαL(I1)+σsαL(V21)−μsαL(R1)],...L(Rk+1)=χ1−α[γsαL(Ik)+σsαL(V2k)−μsαL(Rk)]. | (50) |
Then by inverting the Laplace transform of Eqs (45)-(50) along with the initial condition we have an iterative formula as
Sk+1=χ1−αL−1[μNsα+1−βsαL(Ak)−(p+μ)sαL(Sk)+ξsαL(V1k)],V1k+1=χ1−αL−1[psαL(Sk)−(ξ+q+μ)sαL(V1k)],V2k+1=χ1−αL−1[qsαL(V1k)−(σ+μ)sαL(V2k)],Ek+1=χ1−αL−1[βsαL(Ak)−(η+μ)sαL(Ek)],Ik+1=χ1−αL−1[ηsαL(Ek)−(γ+δ+μ)sαL(Ik)],Rk+1=χ1−αL−1[γsαL(Ik)+σsαL(V2k)−μsαL(Rk)], k=0,1,2,3,... | (51) |
Therefore the approximate solution of the SV1V2EIR model (5) is
S(t)≈m∑k=0Sk, V1(t)≈m∑k=0V1k, V2(t)≈m∑k=0V2k,E(t)≈m∑k=0Ek,I(t)≈m∑k=0Ik, R(t)≈m∑k=0Rk. |
where
limm→∞m∑k=0Sk=S(t), limm→∞m∑k=0V1k=V1(t), limm→∞m∑k=0V2k=V2(t), limm→∞m∑k=0Ek=E(t), limm→∞m∑k=0Ik=I(t), limm→∞m∑k=0Rk=R(t). | (52) |
The solution of the SV1V2EIR model (5) is obtained in infinite series (52), which rapidly and uniformly converge to the exact solution. To check the convergence of the series solution we use the classical technique [60,61]. For this purpose, the obtained solution (52) can be recast as
xn=Fxn−1, xn−1=n∑i=1xi, n=1,2,3,... | (53) |
Next, we present Theorem 8 to prove the convergence of LADM.
Theorem 8 Let X be a Banach space and F:X→X a contractive map with 0<k<1 then F has a unique point x such that F(x)=x, where x=(S,V1,V2,E,I,R). Let x0∈Br(x), where Br(x)={x′∈X:||x′−x||<r} then we have xn∈Br(x) and limn→∞ xn=x.
Proof: We used mathematical induction to prove the result. For n=1, we have
||x0−x||=||F(x0)−F(x)||⩽k||x0−x||. | (54) |
Let's assume the result is true for n−1, then
||xn−1−x||⩽kn−1||x0−x||. | (55) |
We get
||xn−x||=||F(xn−1)−F(x)||⩽k||xn−1−x||⩽kn||x0−x||. | (56) |
That is
||xn−x||⩽kn||x0−x||⩽knr<r. | (57) |
It means that xn∈Br(x).
Moreover, ||xn−x||⩽kn||x0−x|| and limn→∞||xn−x||=0 as limn→∞ kn=0, therefore we have limn→∞ xn=x.
This completes the proof.
To investigate a numerical simulation of the model (5) for India in the Caputo sense we have considered a few parametric values from the literature and the rest are estimated or fitted by the least-squares curve fitting method. We have to use the total population of India N = 1, 408, 044, 253 as of 1 January 2022 [63]. The birth rate in India for the year 2022 is 17.163 births per 1000 people [64], so we estimate a new recruitment rate Birth rate∗N365. The mortality rate for India in 2022 is 27.695 deaths per 1000 live births [65], so we estimate a natural death rate 27.6951000=0.0277. The goal is to estimate the remaining parameters of the SV1V2EIR model to approximate our numerical results to fit real data. For this purpose, we have considered real data of cumulative covid-19 cases in India from 1 January 2022 to 30 April 2022 provided by worldometer [62] and grouped them as weekly in Table 2.
Month | Cumulative confirmed cases of COVID-19 in India | Month | Cumulative confirmed cases of COVID-19 in India |
01 January 2022 | 34, 889, 132 | 05 March 2022 | 42, 967, 315 |
08 January 2022 | 35, 528, 004 | 12 March 2022 | 42, 993, 494 |
15 January 2022 | 37, 122, 164 | 19 March 2022 | 43, 007, 841 |
22 January 2022 | 39, 237, 264 | 26 March 2022 | 43, 019, 453 |
29 January 2022 | 41, 092, 522 | 02 April 2022 | 43, 028, 131 |
05 February 2022 | 42, 188, 138 | 09 April 2022 | 43, 035, 271 |
12 February 2022 | 42, 631, 421 | 16 April 2022 | 43, 042, 097 |
19 February 2022 | 42, 822, 473 | 23 April 2022 | 43, 057, 545 |
26 February 2022 | 42, 924, 130 | 30 April 2022 | 43, 079, 188 |
The model (5) was fitted to the daily cumulative covid-19 cases for India to minimize the summation of square error given by the model solution over a considered period and reported real data. We have taken t = 1 day as of 1 January 2022 and t = 120 days as of 30 April 2022. Figure 2 shows the model fitting of daily cumulative confirmed cases of COVID-19 in India.
We have performed the sensitivity analysis of the reproduction number R0 of the SV1V2EIR model (5) to provide a good strategy and prevent the spread of the disease. A sensitivity index measure provides the proportion that relative changes that may occur in a parameter lead to the relative change in a variable. The normalized forward sensitivity index of R0 with a parameter x is defined as follows [66]
ΠR0x=∂R0∂x×xR0. | (58) |
For the basic reproduction number R0, we compute the following sensitivity index as
ΠR0β=∂R0∂β×βR0=1. | (59) |
From the Eq (59), we note that as there is an increase or decrease in transmission rate β by a certain percentage say k then the reproduction number R0 also increases or decreases by the same percentage k. The sensitivity indices of R0 with parameters of the SV1V2EIR model were evaluated at the parameter values listed in Table 3 and reported in Table 4.
Parameter | Values | Source |
μ | 0.0277 | [65] |
β | 8.25*10-8 | Fitted |
p | 0.94 | Fitted |
ξ | 0.6 | Fitted |
q | 0.62 | Fitted |
σ | 0.84 | Fitted |
η | 0.012 | Fitted |
δ | 0.125 | Fitted |
γ | 0.61 | Fitted |
Parameters | β | μ | η | N | ξ | q | γ | δ | p |
Values | 1 | 0.1928 | 0.6977 | 1 | 0.4551 | −0.4356 | −0.7998 | −0.1639 | −0.9463 |
Figure 3 shows the graphical analysis of the sensitivity indices of R0 with respect to the model parameters. This analysis suggests that the transmission rate of disease, mortality rate, progression rate form E to I, the total population of India, and progression rate form V1 to S are in positive correlation with R0 whereas the rate of dose-I of vaccine, rate of dose-II of vaccine, vaccines related death rate, and the natural recovery rate are in negative correlation with R0. Also, the sensitivity analysis suggests that the most influential parameter are disease transmission rate and rate of dose-I of vaccine, and the least influential parameter is vaccines related death rate. Hence based on this analysis we can develop a suitable strategy to control and prevent the spread of disease.
In this subsection, we have employed the LADM scheme to obtain a numerical simulation of the SV1V2EIR model (5). To analyze the model (5) we simulate it for various biological parameters mentioned in Table 3.
First, we performed a numerical simulation for various values of the rate of dose-I of the vaccine (p) to explore its impact on the infected population. In Figure 4, the values of p taken into consideration are p=0,0.30,0.60 and 0.94. In the absence of dose-I of vaccine, there is a giant peak in a number of an infected population near to 20, 190, 000. As values of rate of dose-I of vaccine increase then there is dramatically declined in a number of an infected population. The estimated value of p=0.94 and the number of an infected population is around 7, 570, 000. The model is also simulated for α=0.9 and α=0.8 to analyze the impact of rate of dose-I of the vaccine on the infected population. For α=0.9 and p=0 the number of an infected population is around 19, 410, 000 whereas it is dramatically decreased for p=0.94 around 7, 378, 000 For α=0.8 and p=0 the number of an infected population is around 18, 730, 000 whereas it is dramatically decreased for p=0.94 around 7, 365, 000. Thus there is a strong negative correlation between the rate of dose-I of vaccine and infected population.
Figure 5 shows the impact of the rate of dose-II of the vaccine (q) on the infected population. The values of q taken into consideration are q=0,0.30,0.62 and 0.90. In the absence of dose-II of vaccine, there is a giant peak in a number of an infected population near to 18, 640, 000. As values of rate of dose-II of vaccine increase then there is dramatically declined in a number of an infected population. The estimated value of q=0.62 and the number of an infected population is around 7, 597, 000. The model is also simulated for α=0.9 and α=0.8 to analyze the impact of the rate of dose-II of the vaccine on the infected population. For α=0.9 and q=0 the number of an infected population is around 18, 000, 000 whereas it is dramatically decreased for q=0.62 around 7, 412, 000. For α=0.8 and q=0 the number of an infected population is around 17, 270, 000 whereas it is dramatically decreased for q=0.62 around 7, 407, 000. Thus there is a strong negative correlation between the rate of dose-II of vaccine and infected population.
Figure 6 shows the impact of disease transmission rate (β) on the infected population for various transmission rates such as 9.25 × 10-8, 8.25 × 10-8, 7.25 × 10-8, and 6.25 × 10-8. As the disease transmission rate decrease the number of an infected population is decreased. Thus there is a strong positive correlation between the disease transmission rate and the infected population. The impact of β on the infected population is also evaluated for fractional-order α=0.9 and 0.8. The same strong positive correlation is observed between the disease transmission rate and the infected population.
Figure 7 shows the impact of the rate of dose-I and dose-II of the vaccine on the infected population. Figure 7 is simulated for the estimated value of p and q for fractional-order α=1,0.9 and 0.8. For α=1, the infected population after getting dose-I of vaccine is around 485, 100, 000 whereas after getting dose-II of vaccine is around 263, 300, 000. For α=0.9, the infected population after getting dose-I of vaccine is around 454, 500, 000 whereas after getting dose-II of vaccine is around 240, 400, 000. For α=0.8, the infected population after getting dose-I of vaccine is around 432, 600, 000 whereas after getting dose-II of vaccine is around 219, 600, 000. Thus there is a dramatically declined in a number of an infected population after getting dose-II of vaccine as compare to dose-I of the vaccine. Also, we observed that as the fractional order decreased the number of the infected population is also gradually decreased due to a non-local property of fractional derivative.
In this paper, we have investigated the SV1V2EIR model to reveal the impact of dose-I, and dose-II vaccination on COVID-19 by using the Caputo fractional derivative. The basic reproduction number of the model is derived by using the next-generation matrix method. The local and global stability analysis is investigated for both the disease-free and endemic equilibrium points. Next, we have to consider real data of cumulative COVID-19 cases in India from 1 January 2022 to 30 April 2022 then the model (5) was fitted to the daily cumulative COVID-19 cases for India to minimize summation of square error by least-squares curve fitting method to estimate model parameters. Then we performed a sensitivity analysis to examine the effects of model parameters that affect the basic reproduction number. From a sensitivity index, we analyze that the most influential parameters are disease transmission rate and rate of dose-I of vaccine, and the least influential parameter is the vaccine-related death rate. Finally, the LADM is implemented to obtain a numerical result of an SV1V2EIR model. The numerical results suggest that there is a strong negative correlation between the rate of dose-I of the vaccine and the infected population. Thus dose-I of the vaccine is necessary to control the spread of COVID-19. According to numerical results, the dose-II of the vaccine is the most efficient to restrict the wide spread of disease. Furthermore, the numerical results suggest that there is a strong positive correlation between the disease transmission rate and the infected population. Thus by following the proper guideline declared by the WHO and the government we decrease the disease transmission rate and ultimately restrict the spread of the pandemic. The fractional-order model provides accurate results due to a non-local property and reveals the precise number of infected population and hence in advanced intimate to develop a most efficient strategy to prevent an outbreak.
The authors declare there is no conflict of interest.
[1] |
P. A. Naik, K. M. Owolabi, J. Zu, M. U. D. Naik, Modeling the transmission dynamics of COVID-19 pandemic in caputo type fractional derivative, J. Multiscale Model., 12 (2021). https://doi.org/10.1142/S1756973721500062 doi: 10.1142/S1756973721500062
![]() |
[2] |
K. M. Safare, V. S. Betageri, D. G. Prakasha, P. Veeresha, S. Kumar, A mathematical analysis of ongoing outbreak COVID-19 in India through nonsingular derivative, Numer. Meth. Part. Differ. Equations., 37 (2021), 1282–1298. https://doi.org/10.1002/num.22579 doi: 10.1002/num.22579
![]() |
[3] |
K. S. Nisar, S. Ahmad, A. Ullah, K. Shah, H. Alrabaiah, M. Arfan, Mathematical analysis of SIRD model of COVID-19 with Caputo fractional derivative based on real data, Results Phys., 21 (2021), 103772. https://doi.org/10.1016/j.rinp.2020.103772 doi: 10.1016/j.rinp.2020.103772
![]() |
[4] |
P. A. Naik, M. Yavuz, S. Qureshi, J. Zu, S. Townley, Modeling and analysis of COVID-19 epidemics with treatment in fractional derivatives using real data from Pakistan, Eur. Phys. J. Plus, 135 (2020), 795. https://doi.org/10.1140/epjp/s13360-020-00819-5 doi: 10.1140/epjp/s13360-020-00819-5
![]() |
[5] |
F. Özköse, M. Yavuz, Investigation of interactions between COVID-19 and diabetes with hereditary traits using real data: A case study in Turkey, Comput. Biol. Med., 141 (2022), 105044. https://doi.org/10.1016/j.compbiomed.2021.105044 doi: 10.1016/j.compbiomed.2021.105044
![]() |
[6] |
P. Pandey, J. F. Gómez-Aguilar, M. K. A. Kaabar, Z. Siri, A. A. A. Mousa, Mathematical modeling of COVID-19 pandemic in India using Caputo-Fabrizio fractional derivative, Comput. Biol. Med., 145 (2022), 105518. https://doi.org/10.1016/j.compbiomed.2022.105518 doi: 10.1016/j.compbiomed.2022.105518
![]() |
[7] |
N. Sene, Analysis of the stochastic model for predicting the novel coronavirus disease, Adv. Differ. Equations, 568 (2020), 1–19. https://doi.org/10.1186/s13662-020-03025-w doi: 10.1186/s13662-020-03025-w
![]() |
[8] |
T. Sitthiwirattham, A. Zeb, S. Chasreechai, Z. Eskandari, M. Tilioua, S. Djilali, Analysis of a discrete mathematical COVID-19 model, Results Phys., 28 (2021), 104668. https://doi.org/10.1016/j.rinp.2021.104668 doi: 10.1016/j.rinp.2021.104668
![]() |
[9] |
S. Kumar, R. P. Chauhan, S. Momani, S. Hadid, Numerical investigations on COVID-19 model through singular and non-singular fractional operators, Numer. Methods Partial Differ. Equations, (2020), 1–27. https://doi.org/10.1002/num.22707 doi: 10.1002/num.22707
![]() |
[10] |
F. Özköse, M. Yavuz, M. T. Şenel, R. Habbireeh, Fractional order modelling of omicron SARS-CoV-2 variant containing heart attack effect using real data from the United Kingdom, Chaos Solitons Fractals, 157 (2022), 111954. https://doi.org/10.1016/j.chaos.2022.111954 doi: 10.1016/j.chaos.2022.111954
![]() |
[11] |
A. Atangana, Modelling the spread of COVID-19 with new fractal-fractional operators: Can the lockdown save mankind before vaccination?, Chaos Solitons Fractals, 136 (2020), 109860. https://doi.org/10.1016/j.chaos.2020.109860 doi: 10.1016/j.chaos.2020.109860
![]() |
[12] |
T. Sardar, S. S. Nadim, S. Rana, J. Chattopadhyay, Assessment of lockdown effect in some states and overall India: A predictive mathematical study on COVID-19 outbreak, Chaos Solitons Fractals, 139 (2020), 110078. https://doi.org/10.1016/j.chaos.2020.110078 doi: 10.1016/j.chaos.2020.110078
![]() |
[13] | S. Choi, M. Ki, Analyzing the effects of social distancing on the COVID-19 pandemic in Korea using mathematical modeling, Epidemiol. Health, 42 (2020). https://doi.org/10.4178/epih.e2020064 |
[14] |
D. Aldila, S. H. A. Khoshnaw, E. Safitri, Y. R. Anwar, A. R. Q. Bakry, B. M. Samiadji, et al., A mathematical study on the spread of COVID-19 considering social distancing and rapid assessment: The case of Jakarta, Indonesia, Chaos Solitons Fractals, 139 (2020), 110042. https://doi.org/10.1016/j.chaos.2020.110042 doi: 10.1016/j.chaos.2020.110042
![]() |
[15] |
D. Baleanu, M. Hassan Abadi, A. Jajarmi, K. Zarghami Vahid, J. J. Nieto, A new comparative study on the general fractional model of COVID-19 with isolation and quarantine effects, Alex. Eng. J., 61 (2022), 4779–4791. https://doi.org/10.1016/j.aej.2021.10.030 doi: 10.1016/j.aej.2021.10.030
![]() |
[16] |
Z. Memon, S. Qureshi, B. R. Memon, Assessing the role of quarantine and isolation as control strategies for COVID-19 outbreak: A case study, Chaos Solitons Fractals, 144 (2021), 110655. https://doi.org/10.1016/j.chaos.2021.110655 doi: 10.1016/j.chaos.2021.110655
![]() |
[17] |
A. M. Mishra, S. D. Purohit, K. M. Owolabi, Y. D. Sharma, A nonlinear epidemiological model considering asymptotic and quarantine classes for SARS CoV-2 virus, Chaos Solitons Fractals, 138 (2020), 109953. https://doi.org/10.1016/j.chaos.2020.109953 doi: 10.1016/j.chaos.2020.109953
![]() |
[18] |
A. Din, A. Khan, D. Baleanu, Stationary distribution and extinction of stochastic coronavirus (COVID-19) epidemic model, Chaos Solitons Fractals, 139 (2020), 110036. https://doi.org/10.1016/j.chaos.2020.110036 doi: 10.1016/j.chaos.2020.110036
![]() |
[19] |
P. Pandey, Y. M. Chu, J. F. Gómez-Aguilar, H. Jahanshahi, A. A. Aly, A novel fractional mathematical model of COVID-19 epidemic considering quarantine and latent time, Results Phys., 26 (2021), 104286. https://doi.org/10.1016/j.rinp.2021.104286 doi: 10.1016/j.rinp.2021.104286
![]() |
[20] |
Y. Gu, S. Ullah, M. A. Khan, M. Y. Alshahrani, M. Abohassan, M. B. Riaz, Mathematical modeling and stability analysis of the COVID-19 with quarantine and isolation, Results Phys., 34 (2022), 105284. https://doi.org/10.1016/j.rinp.2022.105284 doi: 10.1016/j.rinp.2022.105284
![]() |
[21] |
K. N. Nabi, P. Kumar, V. S. Erturk, Projections and fractional dynamics of COVID-19 with optimal control strategies, Chaos Solitons Fractals, 145 (2021), 110689. https://doi.org/10.1016/j.chaos.2021.110689 doi: 10.1016/j.chaos.2021.110689
![]() |
[22] |
A. K. Srivastav, P. K. Tiwari, P. K. Srivastava, M. Ghosh, Y. Kang, A mathematical model for the impacts of face mask, hospitalization and quarantine on the dynamics of COVID-19 in India: Deterministic vs. stochastic, Math. Biosci. Eng., 18 (2020), 182–213. https://doi.org/10.3934/mbe.2021010 doi: 10.3934/mbe.2021010
![]() |
[23] |
P. Riyapan, S. E. Shuaib, A. Intarasit, A mathematical model of COVID-19 pandemic: A case study of Bangkok, Thailand, Comput. Math. Methods Med., 2021 (2021). https://doi.org/10.1155/2021/6664483 doi: 10.1155/2021/6664483
![]() |
[24] |
F. Karim, S. Chauhan, J. Dhar, Analysing an epidemic–economic model in the presence of novel corona virus infection: capital stabilization, media effect, and the role of vaccine, Eur. Phys. J.: Spec. Top., (2022), 1–18. https://doi.org/10.1140/epjs/s11734-022-00539-0 doi: 10.1140/epjs/s11734-022-00539-0
![]() |
[25] |
B. B. Fatima, M. A. Alqudah, G. Zaman, F. Jarad, T. Abdeljawad, Modeling the transmission dynamics of middle eastern respiratory syndrome coronavirus with the impact of media coverage, Results Phys., 24 (2021), 104053. https://doi.org/10.1016/j.rinp.2021.104053 doi: 10.1016/j.rinp.2021.104053
![]() |
[26] |
J. K. K. Asamoah, M. A. Owusu, Z. Jin, F. T. Oduro, A. Abidemi, E. O. Gyasi, Global stability and cost-effectiveness analysis of COVID-19 considering the impact of the environment: using data from Ghana, Chaos Solitons Fractals, 140 (2020), 110103. https://doi.org/10.1016/j.chaos.2020.110103 doi: 10.1016/j.chaos.2020.110103
![]() |
[27] |
P. A. Naik, J. Zu, M. B. Ghori, M. Naik, Modeling the effects of the contaminated environments on COVID-19 transmission in India, Results Phys., 29 (2021), 104774. https://doi.org/10.1016/j.rinp.2021.104774 doi: 10.1016/j.rinp.2021.104774
![]() |
[28] | World Health Organization, COVID19 Vaccine Tracker, Report of World Health Organization, https://covid19.trackvaccines.org/agency/who/ (13-Jun-2022). |
[29] | Indian Council of Medical Research, Vaccine information, https://vaccine.icmr.org.in/ (13-Jun-2022). |
[30] |
M. Yavuz, F. Ö. Coşar, F. Günay, F. N. Özdemir, A New Mathematical Modeling of the COVID-19 Pandemic Including the Vaccination Campaign, Open J. Modell. Simul., 9 (2021), 299–321. https://doi.org/10.4236/ojmsi.2021.93020 doi: 10.4236/ojmsi.2021.93020
![]() |
[31] |
B. H. Foy, B. Wahl, K. Mehta, A. Shet, G. I. Menon, C. Britto, Comparing COVID-19 vaccine allocation strategies in India: A mathematical modelling study, Int. J. Infect. Dis., 103 (2021), 431–438. https://doi.org/10.1016/j.ijid.2020.12.075 doi: 10.1016/j.ijid.2020.12.075
![]() |
[32] |
R. Ikram, A. Khan, M. Zahri, A. Saeed, M. Yavuz, P. Kumam, Extinction and stationary distribution of a stochastic COVID-19 epidemic model with time-delay, Comput. Biol. Med., 141 (2022), 105115. https://doi.org/10.1016/j.compbiomed.2021.105115 doi: 10.1016/j.compbiomed.2021.105115
![]() |
[33] |
K. Liu, Y. Lou, Optimizing COVID-19 vaccination programs during vaccine shortages, Infect. Dis. Modell., 7 (2022), 286–298. https://doi.org/10.1016/j.idm.2022.02.002 doi: 10.1016/j.idm.2022.02.002
![]() |
[34] |
P. Kumar, V. S. Erturk, M. Murillo-Arcila, A new fractional mathematical modelling of COVID-19 with the availability of vaccine, Results Phys., 24 (2021), 104213. https://doi.org/10.1016/j.rinp.2021.104213 doi: 10.1016/j.rinp.2021.104213
![]() |
[35] |
O. Akman, S. Chauhan, A. Ghosh, S. Liesman, E. Michael, A. Mubayi, et al., The Hard Lessons and Shifting Modeling Trends of COVID-19 Dynamics: Multiresolution Modeling Approach, Bull. Math. Biol., 3 (2022), 1–30. https://doi.org/10.1007/s11538-021-00959-4 doi: 10.1007/s11538-021-00959-4
![]() |
[36] |
M. Amin, M. Farman, A. Akgül, R. T. Alqahtani, Effect of vaccination to control COVID-19 with fractal fractional operator, Alex. Eng. J., 61 (2022), 3551–3557. https://doi.org/10.1016/j.aej.2021.09.006 doi: 10.1016/j.aej.2021.09.006
![]() |
[37] |
A. Beigi, A. Yousefpour, A. Yasami, J. F. Gómez-Aguilar, S. Bekiros, H. Jahanshahi, Application of reinforcement learning for effective vaccination strategies of coronavirus disease 2019 (COVID-19), Eur. Phys. J. Plus, 609 (2021), 1–22. https://doi.org/10.1140/epjp/s13360-021-01620-8 doi: 10.1140/epjp/s13360-021-01620-8
![]() |
[38] |
M. L. Diagne, H. Rwezaura, S. Y. Tchoumi, J. M. Tchuenche, A Mathematical Model of COVID-19 with Vaccination and Treatment, Comput. Math. Methods Med., 2021 (2021). https://doi.org/10.1155/2021/1250129 doi: 10.1155/2021/1250129
![]() |
[39] |
I. M. Bulai, R. Marino, M. A. Menandro, K. Parisi, S. Allegretti, Vaccination effect conjoint to fraction of avoided contacts for a Sars-Cov-2 mathematical model, Math. Modell. Numer. Simul. with Appl., 1 (2021), 56–66. https://doi.org/10.53391/mmnsa.2021.01.006 doi: 10.53391/mmnsa.2021.01.006
![]() |
[40] |
A. Omame, D. Okuonghae, U. K. Nwajeri, C. P. Onyenegecha, A fractional-order multi-vaccination model for COVID-19 with non-singular kernel, Alex. Eng. J., 61 (2022), 6089–6104. https://doi.org/10.1016/j.aej.2021.11.037 doi: 10.1016/j.aej.2021.11.037
![]() |
[41] |
O. A. M. Omar, R. A. Elbarkouky, H. M. Ahmed, Fractional stochastic modelling of COVID-19 under wide spread of vaccinations: Egyptian case study, Alex. Eng. J., 61 (2022), 8595–8609. https://doi.org/10.1016/j.aej.2022.02.002 doi: 10.1016/j.aej.2022.02.002
![]() |
[42] |
A. K. Paul, M. A. Kuddus, Mathematical analysis of a COVID-19 model with double dose vaccination in Bangladesh, Results Phys., 35 (2022), 105392. https://doi.org/10.1016/j.rinp.2022.105392 doi: 10.1016/j.rinp.2022.105392
![]() |
[43] | I. Podlubny, Fractional differential equations: an introduction to fractional derivatives, fractional differential equations, to methods of their solution and some of their, 1st Edition. Academic Press, San Diego, 1998. |
[44] |
R. L. Magin, Fractional Calculus in Bioengineering, Crit. Rev. Biomed. Eng., 32 (2004), 1-104. http://dx.doi.org/10.1615/critrevbiomedeng.v32.i1.10 doi: 10.1615/critrevbiomedeng.v32.i1.10
![]() |
[45] | D. Baleanu, K. Diethelm, E. Scalas, J. J. Trujillo, Fractional Calculus: Models and Numerical Methods, World Scientific, New Jersey, 2012. |
[46] |
H. Joshi, B. K. Jha, Fractional-order mathematical model for calcium distribution in nerve cells, Comput. Appl. Math., 56 (2020), 1–22. https://doi.org/10.1007/s40314-020-1082-3 doi: 10.1007/s40314-020-1082-3
![]() |
[47] |
E. Hanert, E. Schumacher, E. Deleersnijder, Front dynamics in fractional-order epidemic models, J. Theor. Biol., 279 (2011), 9–16. https://doi.org/10.1016/j.jtbi.2011.03.012 doi: 10.1016/j.jtbi.2011.03.012
![]() |
[48] |
H. Joshi, B. K. Jha, Chaos of calcium diffusion in Parkinson's infectious disease model and treatment mechanism via Hilfer fractional derivative, Math. Modell. Numer. Simul. with Appl., 1 (2021), 84–94. https://doi.org/10.53391/mmnsa.2021.01.008 doi: 10.53391/mmnsa.2021.01.008
![]() |
[49] |
O. Diekmann, J. A. P. Heesterbeek, M. G. Roberts, The construction of next-generation matrices for compartmental epidemic models, J. R. Soc. Interface, 7 (2020), 873–885. https://doi.org/10.1098/rsif.2009.0386 doi: 10.1098/rsif.2009.0386
![]() |
[50] |
M. Y. Li, H. L. Smith, L. Wang, Global dynamics of an seir epidemic model with vertical transmission, SIAM J. Appl. Math., 62 (2001), 58–69. https://doi.org/10.1137/S0036139999359860 doi: 10.1137/S0036139999359860
![]() |
[51] |
C. Vargas-De-León, Volterra-type Lyapunov functions for fractional-order epidemic systems, Commun. Nonlinear Sci. Numer. Simul., 24 (2015), 75–85. https://doi.org/10.1016/j.cnsns.2014.12.013 doi: 10.1016/j.cnsns.2014.12.013
![]() |
[52] |
A. Omame, M. Abbas, A. Abdel-Aty, Assessing the impact of SARS-CoV-2 infection on the dynamics of dengue and HIV via fractional derivatives, Chaos Solitons Fractals, 162 (2022), 112427. https://doi.org/10.1016/j.chaos.2022.112427 doi: 10.1016/j.chaos.2022.112427
![]() |
[53] |
A. Omame, M. E. Isah, M. Abbas, A. Abdel-Aty, C. P. Onyenegecha, A fractional order model for Dual Variants of COVID-19 and HIV co-infection via Atangana-Baleanu derivative, Alex. Eng. J., 61 (2022), 9715–9731. https://doi.org/10.1016/j.aej.2022.03.013 doi: 10.1016/j.aej.2022.03.013
![]() |
[54] |
O. H. Mohammed, H. A. Salim, Computational methods based laplace decomposition for solving nonlinear system of fractional order differential equations, Alex. Eng. J., 57 (2018), 3549–3557. https://doi.org/10.1016/j.aej.2017.11.020 doi: 10.1016/j.aej.2017.11.020
![]() |
[55] |
M. Y. Ongun, The Laplace Adomian Decomposition Method for solving a model for HIV infection of CD4+T cells, Math. Comput. Modell., 53 (2011), 597–603. https://doi.org/10.1016/j.mcm.2010.09.009 doi: 10.1016/j.mcm.2010.09.009
![]() |
[56] |
F. Haq, K. Shah, G. Ur Rahman, M. Shahzad, Numerical solution of fractional order smoking model via laplace Adomian decomposition method, Alex. Eng. J., 57 (2018), 1061–1069. https://doi.org/10.1016/j.aej.2017.02.015 doi: 10.1016/j.aej.2017.02.015
![]() |
[57] |
D. Baleanu, S. M. Aydogn, H. Mohammadi, S. Rezapour, On modelling of epidemic childhood diseases with the Caputo-Fabrizio derivative by using the Laplace Adomian decomposition method, Alex. Eng. J., 59 (2020), 3029–3039. https://doi.org/10.1016/j.aej.2020.05.007 doi: 10.1016/j.aej.2020.05.007
![]() |
[58] |
M. Ur Rahman, S. Ahmad, R. T. Matoog, N. A. Alshehri, T. Khan, Study on the mathematical modelling of COVID-19 with Caputo-Fabrizio operator, Chaos Solitons Fractals, 150 (2021), 111121. https://doi.org/10.1016/j.chaos.2021.111121 doi: 10.1016/j.chaos.2021.111121
![]() |
[59] |
O. Nave, U. Shemesh, I. HarTuv, Applying Laplace Adomian decomposition method (LADM) for solving a model of Covid-19, Comput. Methods Biomech. Biomed. Eng., 24 (2021), 1618–1628. https://doi.org/10.1080/10255842.2021.1904399 doi: 10.1080/10255842.2021.1904399
![]() |
[60] |
A. Abdelrazec, D. Pelinovsky, Convergence of the Adomian decomposition method for initial-value problems, Numer. Methods Partial Differ. Equations, 27 (2011), 749–766. https://doi.org/10.1002/num.20549 doi: 10.1002/num.20549
![]() |
[61] | A. A. Kilbas, H. M. Srivastava, J. J. Trujillo, Theory and applications of fractional differential equations. Elsevier, New York. |
[62] | Worldometer, India COVID - Coronavirus Statistics, https://www.worldometers.info/coronavirus/country/india/ (11-Jun-2022). |
[63] | Countrymeters, India population (2022) live, https://countrymeters.info/en/India. (11-Jun-2022). |
[64] | MacroTrends, India Birth Rate 1950-2019, https://www.macrotrends.net/countries/IND/india/birth-rate (11-Jun-2022). |
[65] | MacroTrends, India Infant Mortality Rate 1950-2022, https://www.macrotrends.net/countries/IND/india/infant-mortality-rate (12-Jun-2022). |
[66] |
N. Chitnis, J. M. Hyman, J. M. Cushing, Determining Important Parameters in the Spread of Malaria Through the Sensitivity Analysis of a Mathematical Model, Bull. Math. Biol. 1272 (2008), 1272–1296. https://doi.org/10.1007/s11538-008-9299-0 doi: 10.1007/s11538-008-9299-0
![]() |
1. | Hem Raj Pandey, Ganga Ram Phaijoo, Dil Bahadur Gurung, Vaccination effect on the dynamics of dengue disease transmission models in Nepal: A fractional derivative approach, 2023, 7, 26668181, 100476, 10.1016/j.padiff.2022.100476 | |
2. | Yogita Mahatekar, Pallavi S Scindia, Pushpendra Kumar, A new numerical method to solve fractional differential equations in terms of Caputo-Fabrizio derivatives, 2023, 98, 0031-8949, 024001, 10.1088/1402-4896/acaf1a | |
3. | Md Abdul Kuddus, Anip Kumar Paul, Global Dynamics of a Two-Strain Disease Model with Amplification, Nonlinear Incidence and Treatment, 2023, 47, 2731-8095, 259, 10.1007/s40995-023-01412-y | |
4. | Hardik Joshi, Mehmet Yavuz, Stuart Townley, Brajesh Kumar Jha, Stability analysis of a non-singular fractional-order covid-19 model with nonlinear incidence and treatment rate, 2023, 98, 0031-8949, 045216, 10.1088/1402-4896/acbe7a | |
5. | Xuemeng Fan, Cong Wu, Data-driven discovery of Caputo fractional order systems, 2023, 98, 0031-8949, 045225, 10.1088/1402-4896/acc3cb | |
6. | Bahatdin Daşbaşı, Fractional order bacterial infection model with effects of anti-virulence drug and antibiotic, 2023, 170, 09600779, 113331, 10.1016/j.chaos.2023.113331 | |
7. | Olumuyiwa James Peter, Afeez Abidemi, Fatmawati Fatmawati, Mayowa M. Ojo, Festus Abiodun Oguntolu, Optimizing tuberculosis control: a comprehensive simulation of integrated interventions using a mathematical model, 2024, 4, 2791-8564, 238, 10.53391/mmnsa.1461011 | |
8. | Saba Jamil, Parvaiz Ahmad Naik, Muhammad Farman, Muhammad Umer Saleem, Abdul Hamid Ganie, Stability and complex dynamical analysis of COVID-19 epidemic model with non-singular kernel of Mittag-Leffler law, 2024, 70, 1598-5865, 3441, 10.1007/s12190-024-02105-4 | |
9. | Xinghua Hu, Yingyue Liu, Stability analysis of a fractional-order SIV1V2S epidemic model for the COVID-19 pandemic, 2024, 138, 10075704, 108183, 10.1016/j.cnsns.2024.108183 | |
10. | Amr Elsonbaty, Mohammed Alharbi, A. El-Mesady, Waleed Adel, Dynamical analysis of a novel discrete fractional lumpy skin disease model, 2024, 9, 26668181, 100604, 10.1016/j.padiff.2023.100604 | |
11. | Mehmet KOCABIYIK, Mevlüde YAKIT ONGUN, Discretization and Stability Analysis for a Generalized Type Nonlinear Pharmacokinetic Models, 2023, 36, 2147-1762, 1675, 10.35378/gujs.1027381 | |
12. | Ramziya Rifhat, Kai Wang, Lei Wang, Ting Zeng, Zhidong Teng, Global stability of multi-group SEIQR epidemic models with stochastic perturbation in computer network, 2023, 31, 2688-1594, 4155, 10.3934/era.2023212 | |
13. | Martins Onyekwelu Onuorah, Nandadulal Bairagi, An optimal control vaccine model of COVID-19 with cost-effective analysis, 2024, 0020-7179, 1, 10.1080/00207179.2024.2367596 | |
14. | Parvaiz Ahmad Naik, Muhammad Farman, Anum Zehra, Kottakkaran Sooppy Nisar, Evren Hincal, Analysis and modeling with fractal-fractional operator for an epidemic model with reference to COVID-19 modeling, 2024, 10, 26668181, 100663, 10.1016/j.padiff.2024.100663 | |
15. | Parvaiz Ahmad Naik, Zohreh Eskandari, Nonlinear dynamics of a three-dimensional discrete-time delay neural network, 2024, 17, 1793-5245, 10.1142/S1793524523500572 | |
16. | Abeer Alshareef, Quantitative analysis of a fractional order of the $ SEI_{c}\, I_{\eta} VR $ epidemic model with vaccination strategy, 2024, 9, 2473-6988, 6878, 10.3934/math.2024335 | |
17. | Derya Avcı, Mine Yurtoğlu, 2023, Chapter 6, 978-3-031-33182-4, 93, 10.1007/978-3-031-33183-1_6 | |
18. | Hardik Joshi, Mechanistic insights of COVID-19 dynamics by considering the influence of neurodegeneration and memory trace, 2024, 99, 0031-8949, 035254, 10.1088/1402-4896/ad2ad0 | |
19. | Parvaiz Ahmad Naik, Muhammad Owais Kulachi, Aqeel Ahmad, Muhammad Farman, Faiza Iqbal, Muhammad Taimoor, Zhengxin Huang, Modeling different strategies towards control of lung cancer: leveraging early detection and anti-cancer cell measures, 2024, 1025-5842, 1, 10.1080/10255842.2024.2404540 | |
20. | Vora Hardagna Vatsal, Brajesh Kumar Jha, Tajinder Pal Singh, To study the effect of ER flux with buffer on the neuronal calcium, 2023, 138, 2190-5444, 10.1140/epjp/s13360-023-04077-z | |
21. | Brajesh Kumar Jha, Vora Hardagna Vatsal, Hardik Joshi, 2023, A Fractional Approach to Study of Calcium Advection Distribution and VGCC in Astrocyte, 979-8-3503-2168-5, 1, 10.1109/ICFDA58234.2023.10153226 | |
22. | Abdelalim A. Elsadany, Yassine Sabbar, Waleed Adel, Ahmed El-Mesady, Dynamics of a novel discrete fractional model for maize streak epidemics with linear control, 2025, 13, 2195-268X, 1, 10.1007/s40435-024-01509-1 | |
23. | Anum Zehra, Parvaiz Ahmad Naik, Ali Hasan, Muhammad Farman, Kottakkaran Sooppy Nisar, Faryal Chaudhry, Zhengxin Huang, Physiological and chaos effect on dynamics of neurological disorder with memory effect of fractional operator: A mathematical study, 2024, 250, 01692607, 108190, 10.1016/j.cmpb.2024.108190 | |
24. | Dumitru Baleanu, Mojtaba Hajipour, Amin Jajarmi, An accurate finite difference formula for the numerical solution of delay-dependent fractional optimal control problems, 2024, 14, 2146-5703, 183, 10.11121/ijocta.1478 | |
25. | Ibad ullah, Nigar Ali, Ihtisham Ul Haq, Mohammed Daher Albalwi, Shah Muhammad, Mohammad Shuaib, Comprehensive analysis of COVID-19 transmission dynamics: mathematical modeling, stability analysis, and optimal control strategies, 2024, 99, 0031-8949, 075035, 10.1088/1402-4896/ad562c | |
26. | A El-Mesady, Waleed Adel, A A Elsadany, Amr Elsonbaty, Stability analysis and optimal control strategies of a fractional-order monkeypox virus infection model, 2023, 98, 0031-8949, 095256, 10.1088/1402-4896/acf16f | |
27. | Neslihan İyit, Ferhat Sevim, A novel statistical modeling of air pollution and the COVID-19 pandemic mortality data by Poisson, geometric, and negative binomial regression models with fixed and random effects, 2023, 21, 2391-5420, 10.1515/chem-2023-0364 | |
28. | Kunal Shah, Hardik Joshi, EOQ model for deteriorating items with fuzzy demand and finite horizon under inflation effects, 2024, 0, 1937-1632, 0, 10.3934/dcdss.2024160 | |
29. | Moulay Rchid Sidi Ammi, Achraf Zinihi, Aeshah A. Raezah, Yassine Sabbar, Optimal control of a spatiotemporal SIR model with reaction–diffusion involving p-Laplacian operator, 2023, 52, 22113797, 106895, 10.1016/j.rinp.2023.106895 | |
30. | Esra KARAOĞLU, On the stability analysis of a fractional order epidemic model including the most general forms of nonlinear incidence and treatment function, 2023, 73, 1303-5991, 285, 10.31801/cfsuasmas.1258454 | |
31. | Olegario Marín-Machuca, Chinchay-Barragán Carlos Enrique , José Francisco Moro-Pisco , Jessica Blanca Vargas-Ayala , José Ambrosio Machuca-Mines , Rojas-Rueda María del Pilar , Abel Walter Zambrano-Cabanillas , Statistical Mathematical Analysis of COVID-19 at World Level, 2024, 7, 27662748, 040, 10.29328/journal.ijpra.1001082 | |
32. | Shuo Li, Imam Bukhsh, Ihsan Ullah Khan, Muhammad Imran Asjad, Sayed M. Eldin, Magda Abd El-Rahman, Dumitru Baleanu, The impact of standard and nonstandard finite difference schemes on HIV nonlinear dynamical model, 2023, 173, 09600779, 113755, 10.1016/j.chaos.2023.113755 | |
33. | Hayman Thabet, Subhash Kendre, Conformable mathematical modeling of the COVID‐19 transmission dynamics: A more general study, 2023, 46, 0170-4214, 18126, 10.1002/mma.9549 | |
34. | Jinbin Wang, Jiankang Liu, Rui Zhang, Stability and bifurcation analysis for a fractional-order cancer model with two delays, 2023, 173, 09600779, 113732, 10.1016/j.chaos.2023.113732 | |
35. | Bibi Fatima, Mati ur Rahman, Saad Althobaiti, Ali Althobaiti, Muhammad Arfan, Analysis of age wise fractional order problems for the Covid-19 under non-singular kernel of Mittag-Leffler law, 2024, 27, 1025-5842, 1303, 10.1080/10255842.2023.2239976 | |
36. | Hardik Joshi, Mehmet Yavuz, Transition dynamics between a novel coinfection model of fractional-order for COVID-19 and tuberculosis via a treatment mechanism, 2023, 138, 2190-5444, 10.1140/epjp/s13360-023-04095-x | |
37. | Beyza Billur İskender Eroğlu, Dilara Yapışkan, 2023, Chapter 3, 978-3-031-33182-4, 39, 10.1007/978-3-031-33183-1_3 | |
38. | Neeru Adlakha, Non-linear dynamics and control of COVID-19 in india revisited : evidence of synergistic, antagonistic and threshold effects, 2024, 99, 0031-8949, 115248, 10.1088/1402-4896/ad8271 | |
39. | S. Sweatha, S. Sindu Devi, Prediction and decision making in corona virus using fuzzy mathematical model, 2024, 46, 10641246, 2447, 10.3233/JIFS-231945 | |
40. | Parvaiz Ahmad Naik, Bijal M. Yeolekar, Sania Qureshi, Mahesh Yeolekar, Anotida Madzvamuse, Modeling and analysis of the fractional-order epidemic model to investigate mutual influence in HIV/HCV co-infection, 2024, 112, 0924-090X, 11679, 10.1007/s11071-024-09653-1 | |
41. | Garima Agarwal, Man Mohan Singh, D. L. Suthar, S. D. Purohit, Analysis and estimation of the COVID-19 pandemic by modified homotopy perturbation method, 2023, 31, 2769-0911, 10.1080/27690911.2023.2279170 | |
42. | Parvaiz Ahmad Naik, Zohreh Eskandari, Mehmet Yavuz, Zhengxin Huang, Bifurcation results and chaos in a two-dimensional predator-prey model incorporating Holling-type response function on the predator, 2024, 0, 1937-1632, 0, 10.3934/dcdss.2024045 | |
43. | Xiaoxuan Chu, Kon Max Wong, Jun Chen, Jiankang Zhang, Systems of Linear Equations with Non-Negativity Constraints: Hyper-Rectangle Cover Theory and Its Applications, 2023, 11, 2227-7390, 2338, 10.3390/math11102338 | |
44. | Ihtisham ul Haq, Nigar Ali, Hijaz Ahmad, Ramadan Sabra, M. Daher Albalwi, Imtiaz Ahmad, Mathematical analysis of a coronavirus model with Caputo, Caputo–Fabrizio–Caputo fractional and Atangana–Baleanu–Caputo differential operators, 2025, 18, 1793-5245, 10.1142/S1793524523500857 | |
45. | Sonu Kurmi, Usha Chouhan, 2025, Chapter 20, 978-981-97-6793-9, 311, 10.1007/978-981-97-6794-6_20 | |
46. | Muhammad Farman, Rabia Sarwar, Kottakkaran Sooppy Nisar, Parvaiz Ahmad Naik, Sundus Shahzeen, Aceng Sambas, Global wave analysis of a fractional-order cholera disease model in society, 2025, 14, 2192-6670, 10.1007/s13721-025-00505-5 | |
47. | Parvaiz Ahmad Naik, Muhammad Farman, Muhammad Umer Saleem, Zhengxin Huang, Hijaz Ahmad, Muhammad Sultan, 2025, Chapter 11, 978-981-97-6793-9, 163, 10.1007/978-981-97-6794-6_11 |
Parameter | Description of parameter |
S | Susceptible individuals |
V1 | Vaccinated individuals after dose-I |
V2 | Vaccinated individuals after dose-II |
E | Exposed individuals |
I | Infected individuals |
R | Recovered individuals |
N | Total population |
μ | Natural death rate |
β | Disease transmission rate |
p | Rate of dose-I of vaccine |
ξ | Progression rate form V1 to S |
q | Rate of dose-II of vaccine |
σ | Recovery rate due to the second dose of vaccine |
η | Progression rate form E to I |
γ | Natural recovery rate |
δ | Vaccines-related death rate |
Month | Cumulative confirmed cases of COVID-19 in India | Month | Cumulative confirmed cases of COVID-19 in India |
01 January 2022 | 34, 889, 132 | 05 March 2022 | 42, 967, 315 |
08 January 2022 | 35, 528, 004 | 12 March 2022 | 42, 993, 494 |
15 January 2022 | 37, 122, 164 | 19 March 2022 | 43, 007, 841 |
22 January 2022 | 39, 237, 264 | 26 March 2022 | 43, 019, 453 |
29 January 2022 | 41, 092, 522 | 02 April 2022 | 43, 028, 131 |
05 February 2022 | 42, 188, 138 | 09 April 2022 | 43, 035, 271 |
12 February 2022 | 42, 631, 421 | 16 April 2022 | 43, 042, 097 |
19 February 2022 | 42, 822, 473 | 23 April 2022 | 43, 057, 545 |
26 February 2022 | 42, 924, 130 | 30 April 2022 | 43, 079, 188 |
Parameter | Values | Source |
μ | 0.0277 | [65] |
β | 8.25*10-8 | Fitted |
p | 0.94 | Fitted |
ξ | 0.6 | Fitted |
q | 0.62 | Fitted |
σ | 0.84 | Fitted |
η | 0.012 | Fitted |
δ | 0.125 | Fitted |
γ | 0.61 | Fitted |
Parameters | β | μ | η | N | ξ | q | γ | δ | p |
Values | 1 | 0.1928 | 0.6977 | 1 | 0.4551 | −0.4356 | −0.7998 | −0.1639 | −0.9463 |
Parameter | Description of parameter |
S | Susceptible individuals |
V1 | Vaccinated individuals after dose-I |
V2 | Vaccinated individuals after dose-II |
E | Exposed individuals |
I | Infected individuals |
R | Recovered individuals |
N | Total population |
μ | Natural death rate |
β | Disease transmission rate |
p | Rate of dose-I of vaccine |
ξ | Progression rate form V1 to S |
q | Rate of dose-II of vaccine |
σ | Recovery rate due to the second dose of vaccine |
η | Progression rate form E to I |
γ | Natural recovery rate |
δ | Vaccines-related death rate |
Month | Cumulative confirmed cases of COVID-19 in India | Month | Cumulative confirmed cases of COVID-19 in India |
01 January 2022 | 34, 889, 132 | 05 March 2022 | 42, 967, 315 |
08 January 2022 | 35, 528, 004 | 12 March 2022 | 42, 993, 494 |
15 January 2022 | 37, 122, 164 | 19 March 2022 | 43, 007, 841 |
22 January 2022 | 39, 237, 264 | 26 March 2022 | 43, 019, 453 |
29 January 2022 | 41, 092, 522 | 02 April 2022 | 43, 028, 131 |
05 February 2022 | 42, 188, 138 | 09 April 2022 | 43, 035, 271 |
12 February 2022 | 42, 631, 421 | 16 April 2022 | 43, 042, 097 |
19 February 2022 | 42, 822, 473 | 23 April 2022 | 43, 057, 545 |
26 February 2022 | 42, 924, 130 | 30 April 2022 | 43, 079, 188 |
Parameter | Values | Source |
μ | 0.0277 | [65] |
β | 8.25*10-8 | Fitted |
p | 0.94 | Fitted |
ξ | 0.6 | Fitted |
q | 0.62 | Fitted |
σ | 0.84 | Fitted |
η | 0.012 | Fitted |
δ | 0.125 | Fitted |
γ | 0.61 | Fitted |
Parameters | β | μ | η | N | ξ | q | γ | δ | p |
Values | 1 | 0.1928 | 0.6977 | 1 | 0.4551 | −0.4356 | −0.7998 | −0.1639 | −0.9463 |