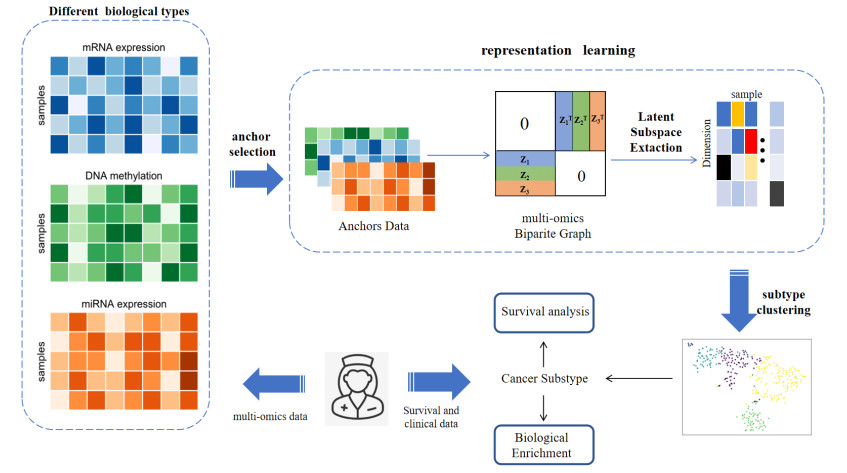
Referring tothe study of epidemic mathematical models, this manuscript presents a noveldiscrete-time COVID-19 model that includes the number of vaccinated individuals as an additional state variable in the system equations. The paper shows that the proposed compartment model, described by difference equations, has two fixed points, i.e., a disease-free fixed point and an epidemic fixed point. By considering both the forward difference system and the backward difference system, some stability analyses of the disease-free fixed point are carried out.In particular, for the backward difference system a novel theorem is proved, which gives a condition for the disappearance of the pandemic when an inequality involving some epidemic parameters is satisfied. Finally, simulation results of the conceived discrete model are carried out, along with comparisons regarding the performances of both the forward difference system and the backward difference system.
Citation: A Othman Almatroud, Noureddine Djenina, Adel Ouannas, Giuseppe Grassi, M Mossa Al-sawalha. A novel discrete-time COVID-19 epidemic model including the compartment of vaccinated individuals[J]. Mathematical Biosciences and Engineering, 2022, 19(12): 12387-12404. doi: 10.3934/mbe.2022578
[1] | Jacob Guinot, Zina Barghouti, Inmaculada Beltrán-Martín, Ricardo Chiva . Corporate social responsibility toward employees and green innovation: Exploring the link in the tourism sector. Green Finance, 2023, 5(2): 298-320. doi: 10.3934/GF.2023012 |
[2] | Joseph F. Hair, Juan José García-Machado, Minerva Martínez-Avila . The impact of organizational compliance culture and green culture on environmental behavior: The moderating effect of environmental commitment. Green Finance, 2023, 5(4): 624-657. doi: 10.3934/GF.2023024 |
[3] | Rizwan Ullah Khan, Hina Arif, Noor E Sahar, Arif Ali, Munir A. Abbasi . The role of financial resources in SMEs' financial and environmental performance; the mediating role of green innovation. Green Finance, 2022, 4(1): 36-53. doi: 10.3934/GF.2022002 |
[4] | Leo-Paul DANA, Mohammad Mahdi Rounaghi, Gholamreza Enayati . Increasing productivity and sustainability of corporate performance by using management control systems and intellectual capital accounting approach. Green Finance, 2021, 3(1): 1-14. doi: 10.3934/GF.2021001 |
[5] | Abdul Samad Kakar, Mrestyal Khan . Exploring the impact of green HRM practices on pro-environmental behavior via interplay of organization citizenship behavior. Green Finance, 2022, 4(3): 274-294. doi: 10.3934/GF.2022013 |
[6] | Ana Bellostas, Cristina Del Río, Karen González-Álvarez, Francisco J López-Arceiz . Cultural context, organizational performance and Sustainable Development Goals: A pending task. Green Finance, 2023, 5(2): 211-239. doi: 10.3934/GF.2023009 |
[7] | Sabuj Saha, Ahmed Rizvan Hasan, Kazi Rezwanul Islam, Md Asraful Islam Priom . Sustainable Development Goals (SDGs) practices and firms' financial performance: Moderating role of country governance. Green Finance, 2024, 6(1): 162-198. doi: 10.3934/GF.2024007 |
[8] | Fu-Hsaun Chen . Green finance and gender equality: Keys to achieving sustainable development. Green Finance, 2024, 6(4): 585-611. doi: 10.3934/GF.2024022 |
[9] | Óscar Suárez-Fernández, José Manuel Maside-Sanfiz, Mª Celia López-Penabad, Mohammad Omar Alzghoul . Do diversity & inclusion of human capital affect ecoefficiency? Evidence for the energy sector. Green Finance, 2024, 6(3): 430-456. doi: 10.3934/GF.2024017 |
[10] | Jeremy Gorelick, Neil Walmsley . The greening of municipal infrastructure investments: technical assistance, instruments, and city champions. Green Finance, 2020, 2(2): 114-134. doi: 10.3934/GF.2020007 |
Referring tothe study of epidemic mathematical models, this manuscript presents a noveldiscrete-time COVID-19 model that includes the number of vaccinated individuals as an additional state variable in the system equations. The paper shows that the proposed compartment model, described by difference equations, has two fixed points, i.e., a disease-free fixed point and an epidemic fixed point. By considering both the forward difference system and the backward difference system, some stability analyses of the disease-free fixed point are carried out.In particular, for the backward difference system a novel theorem is proved, which gives a condition for the disappearance of the pandemic when an inequality involving some epidemic parameters is satisfied. Finally, simulation results of the conceived discrete model are carried out, along with comparisons regarding the performances of both the forward difference system and the backward difference system.
Cancer is an extremely complex and highly heterogeneous disease. According to the World Health Organization, one in six deaths worldwide is due to cancer[1]. As the molecular complexity of cancer etiology is reflected at different levels with different pathogenesis and clinical features, morphologically similar tumors can have different pathogenesis and belong to other subtypes, which are clusters of tumors with standard features among cancer types[2]. Prognostic responses and treatment outcomes vary widely across cancer subtypes, and uncovering the underlying characteristics of cancer subtypes is a long-standing and unresolved issue[3]. Determining cancer subtypes is, therefore, crucial for cancer diagnosis, prognosis, and treatment.
Integrating multi-omics data related to cancer onset and progression can lead to a better understanding of cancer pathogenesis, cancer subtypes, and personalized therapeutic regimens, so it is critical to efficiently capture the interactions between different omics. The rapid developments of high-throughput technologies have made it possible to collect data from various types of multi-omics[4]. The Cancer Genome Atlas (TCGA)[5] is a landmark program in cancer genomics, which molecularly characterized over 20,000 primary cancers and matched standard samples from over 30 cancer types. Also, the International Cancer Genome Consortium (ICGC)[6] collects multilevel, multi-omics data on cancer patients, allowing access to genome-wide data on the molecular processes of different samples. Different high-throughput sequencing technologies can only focus on the specificity of a single genomic. At the same time, multi-omics data provides a more comprehensive view of gene regulation than a single data type[7]. Early studies of cancer subtype determination focused on single-omics data (e.g., gene expression). However, integrating multi-omics data related to cancer occurrence and progression can lead to a better understanding of cancer pathogenesis, cancer subtypes, and personalized therapeutic regimens, none of which can be achieved by utilizing only single-omics data[8]. Most multi-omics data samples in cancer multi-omics research are not labeled with specific cancer subtypes. Therefore, unsupervised methods (e.g., clustering) are commonly used to be able to identify cancer subtypes[9,10,11,12].
Clustering is essential in exploring embedded patterns and structures in multidimensional datasets[13,14,15]. Clustering multiple omics, datasets make it possible to obtain more accurate clusters to identify cancer subtypes, which helps identify biomarkers, pathways, and therapeutic targets specific to each subtype[16]. Meanwhile, researchers have been able to draw different conclusions and give various interpretations of the criteria for defining subtypes by utilizing various methods to extract hidden information from genomic. For example, previous studies have classified glioblastoma multiforme (GBM) into two[17], three[18], and six[19] subtypes based on different criteria. As a result, the definition of subtypes evolves as research progresses. Typically, researchers use dimensionality reduction algorithms to solve high-dimensional problems in genomics and then use various clustering methods to divide the data into groups. This is done to discover features inherent in the data and provide an intuitive grouping that allows healthcare professionals to define subtypes more accurately[20]. Due to the high-dimensional, sparse, and high-noise-rate nature of the multi-omics data, it is still challenging for traditional methods to accurately identify cancer subtypes by capturing potential relationships between multiple omics and accurately identify cancer subtypes. Therefore, how to effectively integrate multi-omics data to identify cancer subtypes accurately is a critical point in current cancer research. To deal with such challenges, we propose an efficient multi-omics bipartite graph subspace learning anchor-based clustering (MBSLC) method with the innovations listed as follows:
1) In order to solve the problems of high computational complexity and unstable clustering results in biological multi-omics clustering, we propose a novel and effective method called MBSLC, which integrates anchor selection, cross-view methods, and spectral clustering into a unified framework. Extensive experiments on real cancer datasets demonstrate the efficiency and accuracy of the proposed model, which generally outperforms other existing methods.
2) Unlike generating anchor sets with traditional random selection and k-means, selecting higher-quality anchors by a particular anchor selection strategy can mine the hidden structures underneath the data further. As a result, the effective use of anchor graphs can significantly improve the clustering efficiency.
3) An end-to-end model is proposed, which is more user-friendly as it does not require manual feature extraction and step-by-step task execution.
In recent years, the research of multi-omics clustering has gained much attention, and various algorithms and techniques have been developed for integrating and analyzing multi-omics data[10]. Regarding the main strategies for integrating multi-omics data, these approaches can be broadly categorized into four types[11].
1) Graph-based methods: they use graphs or similarity matrices to describe sample relationships.
2) Dimensionality-based methods: the joint dimensionality reduction of dimensions between various omics are conducted.
3) Statistic-based methods: they use a statistical model, such as the Bayesian model, to analyze multi-omics data.
4) Neural network-based methods: deep neural network-based techniques and intense learning methods are adopted for integrating multi-omics data.
Usually, each algorithm does not strictly belong to one category and may be designed simultaneously considering two or more ideas. The most direct approach, such as the low-rank approximation based multi-omics data clustering (LRAcluster) method[12], is a statistic approach that applies single-omics clustering on a cascade matrix consisting of all the genomic matrices. However, this method may ignore distributional differences across omics, which degrades clustering performance for cancer subtype identification. To capitalize on the complementary nature of multi-omics data from patients, similarity network fusion (SNF)[18] uses a graphical approach to construct a similarity network for each genomic data individually and uses iterative message passing to update and fuse them into a unified network. In the final similarity network, cancer subtypes are clustered using spectral clustering. The NEighborhood based Multi-Omics clustering (NEMO) method [21] incorporates multi-omics data to calculate the average similarity matrix for each genomic. However, in high-dimensional spaces where the samples are more widely spaced, the Euclidean distance may have corrupted meaningful signals within the dataset. DeFusion[22] employs a deep neural network approach to capture noise and data patterns at the error boundary, which is used to reveal consistent latent representations between multi-genomic data. The Multi‐Omics Factor Analysis (MOFA) method [23] is used to obtain a joint latent variable model by means of dimensionality reduction to infer interpretable low-dimensional data representations based on neural networks, and these learned joint latent variables capture the main sources of variation in different data patterns. The Deep Subspace Mutual Learning (DSML) method [24] uses a deep neural network to learn the subspace structure of individual genomic data and the overall multi-genomic data. However, employing a deep subspace clustering network for each omics could result in heavy computational burden. The multi-omics clustering method based on latent sub-space learning (MCLS) method [25] uses the principal component analysis (PCA) method for feature extraction and singular value decomposition (SVD) methods to construct latent subspaces by dimensionality reduction techniques and then performs spectral clustering in the hidden subspace.
Multi-omics clustering can be regarded as a special kind of multi-view clustering problem, which aims to automatically group data points with similar intrinsic properties into the same cluster[26], which is similar to the clustering purpose. Spectral clustering (SC) is a well-known unsupervised learning method for exploring the graphical structure of unlabeled data. It is widely used in multivariate clustering[27,28,29] due to its precise mathematical formulation and ability to capture intricate structures and relationships between almost any shape data points. The anchor graph-based approach is inspired by SC. The difference is that SC constructs a complete sample graph between every two samples with a scale of n×n (where n is the number of samples), while anchor graph-based methods generate an anchor graph between the samples and their anchor points (where m is the number of anchor points) with a scale of m. In general, m≪n results in a significant reduction of the data scale and a substantial increase in clustering efficiency.
Anchor-based strategy for generating bipartite graph integrated clustering has already been a colorful approach in the multi-view field. However, the bipartite graph fusion clustering method has not yet to be proposed for bio-multi-omics clustering. The well-researched anchor-based bipartite graph method in the multi-view field has already proved its high efficiency and excellent accuracy. It is reasonable to believe that this method can also be effective in the problem of cancer subtype prediction in bioinformatics, and the experimental results also confirm our conjecture. To evaluate the predictive performance of MBSLC, we compared its performance with 10 state-of-the-art multi-omics data clustering methods on seven TCGA datasets. In addition, we performed a series of survival and clinical analyses to demonstrate the effectiveness of MBSLC.
Assume that X=[X1,X2.....Xv] is a multi-omics dataset where V is the number of omics. Xv∈Rn∗dv denotes the vth multi-omics dataset, where n is the number of samples and dv is the feature dimension. Our algorithm consists of three main parts, i.e., cluster-specific subspace learning of samples and anchors, latent representation extraction, and label prediction by spectral clustering. The algorithmic framework is shown in Figure 1.
To start, the samples are concatenated to obtain the anchor points according to the discretization-based edge anchors selection algorithm (DEA). The similarity matrix between anchors and samples is then generated by cross-omics subspace learning between anchors and samples W∈R(n+m×v)×(n+m×v). Then, the potential subspace of the sample is constructed by selecting the eigenvalues vector corresponding to the smallest q eigenvalues from the learned cross-omics subspace among the anchor points and the sample. Finally, the clustering labels of each sample are obtained by spectral clustering of the potential subspaces, and the experimental results are analyzed.
Traditional multi-omics clustering methods usually perform the construction of potential subspaces by constructing an n×n similarity matrix, given the entire dataset X=[X1,X2....Xv], assuming that a sample point can be written as a linear transformation or combination of mappings of all other sample points as follows[30]:
Xv=XvZTv+Ev | (3.1) |
where Zv∈Rn×n is the self-representation matrix of the vth multi-omics, with the constraint zii=0, which forces each point not to be represented by itself. Ev∈Rd×n is the error term, which aims to minimize the error and find a better self-representation matrix. The objective function can be formulated as[31]:
minZv||Xv−XvZTv||2F+α||Zv||2F | (3.2) |
where ||Z||2F is the regulation expression that restricts the model from performing normal solutions, and α>0 is a hyperparameter used to control the effect of the regularization expression. Under the condition Z≥0,Z1=1, the Z term (0≤zij≤1) can represent the similarity between the i-th and the j-th sample. Therefore, Z can also be considered a similarity graph.
In practice, the similarity graph is usually constructed using the K-Nearest Neighbor (KNN) [32] graph, where a point represents a vertex on the affinity graph, and each edge denotes the affinity of a pair of vertices. If at least one of the given measurements (usually Euclidean distances) is among the k nearest neighbors of the other, xi and xj are connected, and the weights of the edges between xi and xj are defined as:
WVij={exp(||xVi−xVj||2σ2),if xi and xj are connected0,otherwise | (3.3) |
where σ is the equilibrium parameter.
It is worth noting that we use the Gaussian kernel as an example, and other types of kernels are also applicable in this paper. However, the high-dimensional nature of biological omics means constructing an N∗N similarity matrix usually requires an extensive time expenditure, and most clustering algorithms are susceptible to noise when constructing the similarity graph and also face the problem of information loss during the clustering process, which reduces the accuracy of the clustering results. On the one hand, the similarity matrix can be constructed by anchors to reduce the number of similarity relations, the dimension of which can be reduced from n to m (m is the number of anchors). On the other hand, by selecting high-quality anchors it is possible to better cover the data categories and have better data characterization ability to fully explore the potential hidden information of multi-homology, and to eliminate the noise generated by different problems of noise generated by different omics to improve the accuracy of clustering results. The objective function to learn the potential subspace can be represented as follows[33]:
minZv||Xv−AvZTv||2F+α||Zv||2F | (3.4) |
where Av represents the data of the vth genomic that has gone through the anchor selection strategy, while the similarity matrix consisting of anchors-samples is constructed as follows, and the similarity map matrix of genomic XV is denoted as:
WVij={exp(||xVi−aVj||2σ2),if xi and aj are connected, 0,otherwise. | (3.5) |
In order to achieve scalability in our approach and improve our algorithm's performance, we include potential features derived from cross-omics anchor graphs in our framework. Specifically, we construct a similarity matrix for each genome {Zk}vk=1 by performing bipartite graph learning on individual genome data, where Zk∈Rm∗n denotes the similarity matrix between data points and anchor points in the genome kth, where m denotes the number of anchor points. The anchor rate determines the number of anchor points, such as m=n∗rate, and we use a specific anchor point selection strategy to select more representative sample points, which is more applicable to multiple biological genomics. Finally, the similarity matrix of the anchor point map between different omics W∈R(n+v∗m)∗(n+v∗m) is presented as follows[34]:
W=[0ZT1⋯ZTvZ10⋯0⋮⋮⋱⋮Zv0⋯0] | (3.6) |
where v is the number of omics.
Next, the standard Laplace matrix for the cross-omics graph W is computed by L=I−Δ−12WΔ−12, which denotes a diagonal matrix, such that Δi,i=∑jwij. Then, the potential subspace of the cross-omics graph denoted by ˜Z can be solved by the following problem:
min˜ZTr(˜ZL˜ZT) | (3.7) |
˜Z∈Rq∗n is a low-dimensional matrix derived from the cross-omics matrices, which can be considered a potential subspace for multi-omics data. The potential subspace ˜Z consists of the eigenvectors corresponding to the minimum eigenvalues of q, where q is the user-defined dimension. Once a potential subspace is generated, we can perform a spectral clustering algorithm directly on ˜Z to generate the final labeling matrix.
To speed up graph-based clustering, anchor graphs that approximate complete sample graphs are constructed to compress the data scale [35]. Selecting anchor points is crucial for high-quality anchor graphs. Two common strategies for anchor point selection are random sampling and k-mean[36] clustering. A random sampling technique randomly selects M data points as anchor points from all samples. However simple and practical, the results of the selected anchor points are dependent, leading to unstable and unsatisfactory clustering results. In contrast, k-means is often applied in practice because it provides more significant anchor points than random sampling. The standard k-means, however, suffers from the following drawbacks: it is not as efficient as it should be when dealing with large-scale data, the performance of clustering is overly dependent on the initialization of the center of mass, and k-means[36] is sensitive to the initial center of mass and must be run independently multiple times to eliminate the randomness of the random sampling results[37]. This work uses an efficient DEA method to select anchor points.
PCA [38] is a representative technique for the dimension reduction of data. It characterizes the original data space and all clusters well by selecting the projected directions representing the most significant variations in the projected dimensions. Inspired by this, we use PCA to explore the most representative samples by considering the sample space and dimension space as dimension and sample space, respectively. Therefore, a simple and efficient anchor selection scheme is proposed - i.e., standard deviation-based edge anchor selection. It considers the features in the ith sample as the feature subspace of the ith projection direction. Then, it selects representative sample points based on their standard deviations in dimension space. It is a straightforward idea to select the first r sample points with the most significant standard deviation. However, this approach does not ensure good coverage of the entire data cluster and characterize the intrinsic structure of the data fundamentally, since samples with relatively large standard deviations among the patient samples are likely to belong to the same clusters.
In this study, we connect all omics to get a large matrix X=[X1,X2....Xv], where Xi denotes the ith genomic dataset. The essence of constructing the bipartite graph is to extract m(m≪n) representative data points, i.e., anchor points, from all sampling points. The standard deviation of the d functions for the ith sample can be computed using the following:
θi=Std(xi) | (3.8) |
where Std(⋅) denotes the standard deviation of the computed samples. Also, after obtaining each θi via Eq (3.8), the standard deviation vector Θ=[θ1,θ2.θn]∈Rn is generated. Thereafter, the sample point with the highest standard deviation is selected as the initial anchor point by:
Index=argmaxiθi | (3.9) |
Since the same clusters of samples can have the same standard deviation, we iterate the set of standard deviations for the following anchor selection from the samples in the last election to avoid double selection of samples between the same clusters; first we calculate the correlation of all samples with the last selection of anchors, where the correlation of θIndex with θi, δi can be computed as:
δi=11+||θIndex−θi||2 | (3.10) |
where δi=[δ1,δ2...δn]∈Rn is the vector of correlation coefficients, and then we can compute the standard deviation score for each sample processed with edge selection:
θi=θi×(1−δi) | (3.11) |
After the above processing step, we can ensure that each anchor point comes from each different sample cluster as much as possible, and the resulting anchor set can cover the whole data category well and characterize the data's inherent structure. We select the most significant sample in θi as an anchor point and update it using Eq (3.11) until the selected anchor point reaches the specified m. Algorithm 1 presents the details of the DEA-based anchor point selection process.
Algorithm 1 Anchor selection method |
Input: Dataset{Xi}vi=1={X1,X2,...,Xv},Xi∈Rdi×n, anchor selection number m.
Output: Anchor graph set{A1,A2,...,Av,Ai∈Rdi×m}. 1: Connect all views togetherX∈R(d1+d2+...+dv)×n. 2: Compute the variance Θ=[θ1,θ2,...,θn]of d-dimensional attributes for each sample by Eq (3.8) 3: Repeat 4: Select the maximum point in θ as the anchor point and record its subscript. 5: Update θ based on Eqs (3.10) and (3.11) 6: Until select m anchors. 7: return Outputs |
By incorporating cross-omics subspace learning and DEA anchor selection methods into a unified framework, latent representations and clustering structures can be simultaneously optimized and mutually enhanced, resulting in high-quality clustering results. Also, in the ablation experiment section, we can demonstrate that each module can improve the algorithm's performance. The overall pseudo-code of the algorithm is shown in Algorithm 2.
Algorithm 2 MBSLC |
Input: Dataset{Xi}vi=1={X1,X2,...,Xv},Xi∈Rdi×n, number of anchors m, subspace dimensions q.
Output: Clustering label Y. 1: Select anchors {Ai}vi=1∈Rdi×n by Algorithm 1. 2: Calculate the matrix of similar graphs Wv for each view by Eq (3.5). 3: Construct bipartite graphs{Bv}Vv=1∈R(n+r×v)×(n+r×v) by Eq (3.6). 4: Calculate subspace information ˇZ∈Rn×q by Eq (3.7). 5: Subspace clustering yields cluster labels. 6: return Outputs |
Time complexity: selecting anchor points requires calculating the standard deviation of different sample points and the correlation between sample points, with a time complexity of O(n2). Similarity graph construction is a necessary step in the graph-based multi-omics clustering algorithm. The similarity graph fusion of this paper's algorithm only needs to be calculated once, which has a greater efficiency advantage compared with the algorithms that need to iteratively update the optimal similarity graph as well as the deep neural network, and when constructing the similarity graph, it is necessary to compute the similarity between the anchor points and the sample points, and the time complexity of the similarity computation is O(mn/2). It is necessary to construct the corresponding similarity matrix ∑Vv=1Wv for each genomic, so the time complexity is O(V×mn/2), and the first q feature vectors are then computed to obtain the potential subspace with a time complexity of O(q(n+m×v)2). Finally, perform spectral clustering on the potential subspace with time complexity O(qn2). Therefore, the time complexity of MBSLC is O(mn+v×mn+q(n+m×v)2), where m,v,q≪n, so the time complexity of the algorithm can be expressed as O(n2).
Space complexity: the main space overhead of the algorithm is the relevance score graph δ for anchor selection, the similarity graph Wv for each genomic, the similarity graph W after concatenation, and the potential subspace ˜Z. Their space complexity is O(mn), O(vmn), O(n+m×v)2, O(qn), respectively. Since m,v,q≪n, the space complexity of the algorithm can be expressed as O(n2).
The proposed method, MBSLC, is compared with other integrative methods, tested on both artificial and natural datasets. The experiments were performed on a computer with a 2.40 GHz Intel(R) Core (TM) i7-13700U CPU and 16 GB of RAM.
To validate the effectiveness of MBSLC, seven typical cancer datasets were selected for subsequent analysis, including breast invasive carcinoma (BIC), colorectal adenocarcinoma (COAD), GBM, lung squamous cell carcinoma (LSCC), renal clear cell carcinoma (KRCCC), bladder uroepithelial carcinoma (BLCA), and low-grade glioma of the brain (LGG). The multi-omics data and patient clinical information were downloaded from the National Cancer Institute (https://portal.gdc.cancer.gov/). Each cancer dataset includes messenger RNA (mRNA) expression data, microRNA (miRNA) expression, DNA methylation or protein expression, and clinical information for each patient. Details of the multi-omics datasets used in this paper are described as mRNA expression, miRNA expression, DNA methylation or protein expression, and the number of patients in each dataset. According to [25], we have performed mean centering on each feature to ensure that the mean of each features is zero. The properties of these datasets are provided in Table 1.
Dataset | Sample | Three omics (features) |
BIC | 621 | Methylation (2000), mRNA (2000), miRNA (885) |
COAD | 220 | Methylation (2000), mRNA (2000), miRNA (613) |
GBM | 274 | Methylation (2000), mRNA (2000), miRNA (534) |
KRCCC | 184 | Methylation (2000), mRNA (2000), miRNA (791) |
LSCC | 341 | Methylation (2000), mRNA (2000), miRNA (881) |
BLCA | 338 | Protein (49), mRNA (5133), miRNA (262) |
LGG | 425 | Protein (45), mRNA (5133), miRNA (262) |
In this section, our MBSLC model is compared with 10 multi-omics clustering methods, including SNF[18], LRAcluster[12], Spectrum[39], DeFusion[22], MOFA[23], NEMO[21], DSML[24], MCLS[25], as well as consensus clustering (CC) and SNF.CC [40]. Note that CC and SNF.CC is the cancer subtype realized by the R package[40]. For all comparative methods, we used their open-source source code, and the parameters of each algorithm were set according to their original papers.
We used two assessment methods to evaluate the performance of MBSLC. First, we used the log-rank (log-rank) test to calculate the -log10P value for the overall survival cycle analysis to compare whether significant differences existed between the cancer subtypes identified by MBSLC. Second, to avoid bias in the enrichment analysis, we selected the same set of clinical labels for all cancers. We also tested the enrichment of these labels—i.e., age at initial diagnosis, gender, and four discrete clinicopathological parameters: measurement of tumor progression (pathologic T), lymph node cancer (pathologic N), metastasis (pathologic M), and total progression (pathologic stage). Note that, pathologic T is a manifestation of the primary tumor to assess the size of the tumor, depth of infiltration, and whether or not it invades the surrounding tissues or organs. For pathologic N, the number and location of lymph node involvement are critical for assessing the spread of cancer. Also, the greater the number of lymph nodes involved, the more severe the disease usually is. For pathologic M, metastasis is the spread of cancer cells to organs or tissues outside the primary site. M0 indicates no distant metastasis, and M1 indicates distant metastasis. In the clinical labeling enrichment analysis, we used the chi-square test for discrete clinical parameters and the Kruskal-Wallis test for numerical parameters. In addition, only some of these six clinical indicators were complete in the dataset, and there were missing cases, as shown in Table 2.
Dataset | age at initial diagnosis | gender | pathologic T | pathologic M | pathologic N | pathologic stage |
BIC | ✓ | ✓ | ✓ | ✓ | ✓ | ✓ |
COAD | ✓ | ✓ | ✓ | ✓ | ✓ | ✓ |
GBM | ✓ | ✓ | × | × | × | × |
KRCCC | ✓ | ✓ | ✓ | ✓ | ✓ | ✓ |
LSCC | ✓ | ✓ | × | × | × | × |
BLCA | ✓ | ✓ | ✓ | ✓ | ✓ | ✓ |
LGG | ✓ | ✓ | × | × | × | × |
We have compared our method with 10 other comparative methods based on the -log10P value of the survival analysis, the number of enriched clinical parameters, and the average runtime of the runs in the seven cancer datasets. In addition, we referred to previous articles on the setting of the number of each cancer subtype and chose three cluster numbers: 4, 5, and 7. It is worth mentioning that the number of cancer subtypes in the BIC dataset is usually considered to be definitive (BIC-sur: 5)[41]. Table 2 shows the comparison of clustering performance of empirical survival in terms of P-values on 7 cancer datasets. For better illustration, all values were negative log-transformed, and values greater than or equal to 1.30 (≈ -log10 (0.05)) were considered statistically significant. As shown in Table 3, the proposed MBSLC method obtains higher-quality clustering results for clinical parameter enrichment analysis on six datasets (BIC, COAD, GBM, KRCCC, LGG, and LSCC).
Dataset | SNF | CC | SNF.CC | LRAcluster | Spectrum | Defusion | MOFA | NEMO | DSML | MCLS | MBSLC |
BIC | 1.7 | 2.2 | 3.8 | 0.2 | 5.4 | 5.3 | 5.7 | 1.9 | 3.9 | 8.1 | 11.2 |
COAD | 0.3 | 0.1 | 0.4 | 0.3 | 0.3 | 0.3 | 0.1 | 0.1 | 1.0 | 2.1 | 3.9 |
GBM | 3.2 | 1.6 | 2.9 | 1.6 | 4.2 | 4.5 | 6.7 | 3.9 | 2.3 | 6.8 | 7.7 |
KRCCC | 1.0 | 2.3 | 0.9 | 3.8 | 1.1 | 1.0 | 0.3 | 1.1 | 1.9 | 9.9 | 4.3 |
LSCC | 1.3 | 0.8 | 1.8 | 1.1 | 2.4 | 1.7 | 1.9 | 1.2 | 1.9 | 3.2 | 7.3 |
BLCA | 3.3 | 3.5 | 4.2 | 3.2 | 3.4 | 2.8 | 3.1 | 3.0 | 2.0 | 4.0 | 4.5 |
LGG | 14.5 | 10.4 | 14.8 | 10.9 | 12.7 | 16.0 | 15.1 | 14.6 | 12.3 | 16.0 | 28.3 |
Mean | 3.6 | 2.9 | 4.1 | 3.0 | 4.2 | 4.6 | 4.7 | 3.7 | 3.6 | 7.2 | 9.6 |
Moreover, the number of enriched clinical labels obtained by each method is shown in Table 4. We can see that our proposed method has higher mean survival analysis -log10P values than the other 10 methods in the seven datasets, demonstrating that our method identifies more significant differences in cancer subtypes. These results indicate that MBSLC outperforms ten comparative clustering methods. This means that MBSLC effectively captures and integrates a significant portion of each multi-omics dataset. Although the -log10P value of our method on the KRCCC dataset is lower than that of MCLS in Table 3, our method enriches clinical parameters more than the MCLS method. As presented in Table 4, our MBSLC method outperformed all other methods in terms of the number of enriched clinical labels, since it achieves the highest mean number as 3.2. The average performance of the methods on the 7 cancer datasets is shown in Figure 2. The X-axis shows the average number of enriched clinical parameters in the clusters, and the dashed line shows the performance of the MBSLC. The Y-axis shows the average difference in survival between the clusters significance (-log10 log-rank test p-value). The Figure 2 shows that MBSLC is superior to all other methods regarding the number of enriched clinical labels.
Dataset | SNF | CC | SNF.CC | LRAcluster | Spectrum | Defusion | MOFA | NEMO | DSML | MCLS | MBSLC |
BIC(6) | 2 | 2 | 2 | 3 | 4 | 3 | 3 | 2 | 3 | 4 | 4 |
COAD(6) | 1 | 1 | 1 | 1 | 2 | 2 | 1 | 1 | 1 | 2 | 3 |
GBM(2) | 1 | 1 | 1 | 1 | 1 | 1 | 2 | 1 | 2 | 2 | 2 |
KRCCC(6) | 2 | 4 | 3 | 2 | 5 | 4 | 4 | 2 | 3 | 4 | 5 |
LSCC(2) | 1 | 1 | 1 | 1 | 0 | 0 | 2 | 1 | 1 | 1 | 2 |
BLCA(6) | 5 | 4 | 5 | 5 | 5 | 5 | 4 | 4 | 2 | 6 | 5 |
LGG(2) | 1 | 1 | 2 | 1 | 1 | 1 | 1 | 2 | 2 | 2 | 2 |
Mean | 1.9 | 2.0 | 2.1 | 2.0 | 2.6 | 2.3 | 2.4 | 1.9 | 2.0 | 3.0 | 3.2 |
In addition, Figure 3 illustrates the Kaplan-Meier overall survival curves for the MBSLC method for identifying subtypes on the 7 cancer datasets to show the method's effectiveness in distinguishing survival rates for different subtypes. Different colored curves indicate different cancer subtypes. The degree of separation between the curves indicates the significance of the difference in survival between patients with different subtypes. The greater the degree of separation, the more significant the difference in survival outcomes. Survival curves for cancer subtypes identified by MBSLC on the seven datasets are well separated.
We have also compared the prognostic performance of our MBSLC method with other standard methods using multi-omics data using latent subspace. As shown in Table 5, MBSLC has the highest C-index[42] value with an average of 0.737. On average, MBSLC improves the Harrel C-index value by 13% compared to other methods.
Dataset | MCLS | LRAcluster | DSML | MBCLS |
BIC | 0.612 | 0.715 | 0.662 | 0.561 |
COAD | 0.577 | 0.628 | 0.597 | 0.671 |
GBM | 0.575 | 0.636 | 0.622 | 0.962 |
KRCCC | 0.531 | 0.591 | 0.563 | 0.595 |
LSCC | 0.649 | 0.735 | 0.644 | 0.883 |
BLCA | 0.812 | 0.801 | 0.823 | 0.784 |
LGG | 0.586 | 0.635 | 0.646 | 0.701 |
Average | 0.620 | 0.677 | 0.651 | 0.737 |
Finally, the average running times of the ten methods on the seven datasets are summarized in Table 6. It is clear that our MBSLC algorithm is the fastest on each dataset, while MCLS and Spectrum have the second and third highest average runtimes, respectively.
Dataset | SNF | CC | SNF.CC | LRAcluster | Spectrum | Defusion | MOFA | NEMO | DSML | MCLS | MBSLC |
BIC | 42.0 | 12.2 | 1178.2 | 140.1 | 11.2 | 1140.8 | 46.2 | 19.0 | 2110.0 | 10.2 | 1.15 |
COAD | 7.1 | 3.1 | 71.0 | 22.0 | 1.3 | 605.2 | 28.2 | 5.8 | 602. 5 | 1.8 | 0.87 |
GBM | 6.9 | 4.2 | 124.1 | 45.2 | 2.3 | 838.8 | 32.1 | 6.1 | 807.3 | 2.4 | 0.42 |
KRCCC | 6.2 | 2.0 | 49.2 | 21.0 | 1.0 | 522.0 | 26.3 | 5.5 | 595.2 | 1.2 | 0.50 |
LSCC | 12.8 | 5.1 | 212.3 | 46.1 | 2.7 | 616.2 | 34.2 | 10.7 | 937.4 | 3.6 | 0.54 |
BLCA | 11.1 | 3.2 | 215.1 | 37.8 | 4.2 | 586.8 | 33.4 | 10.5 | 1007.2 | 2.9 | 0.95 |
LGG | 15.9 | 6.1 | 404.0 | 88.3 | 6.2 | 735.6 | 38.2 | 13.2 | 1376.4 | 4.3 | 0.47 |
In order to examine the impact of the different anchor selection algorithms on the clustering results, we selected two standard anchor selection algorithms to compare with the one proposed in this paper (i.e., the algorithm 1), which include the random sampling strategy and the K-means. We run the three anchor selection algorithms on a multi-clustered and bimonthly dataset to investigate the differences between these two anchor selection algorithms and the proposed algorithm.
Figure 4 illustrates comparing the visualization results of different anchor selection methods on two toy datasets. Meanwhile, Figure 5 shows the visualization results on the two unbalanced toy datasets, where a different color represents each cluster. One hundred anchors were selected for each method on the unbalanced toys dataset (see red circles). Compared to the results of anchor selection with random sampling and K-Means, we can see that the proposed DEA can select appropriate anchor points on the toy dataset, covering the edge part of each cluster. This makes the distance between different clusters more substantial. Also, the DEA method can select a more appropriate number of anchor points and does not ignore the overall characteristics of a cluster due to the small number of samples in that cluster. The K-means method also performs well in some cases. However, the Euclidean distance between all samples and anchor features must be calculated several times to obtain stable results. As shown in Figure 6, this anchor selection scheme will be very time-consuming when the dimensionality of the input data is high. In addition, we also compare the clustering performance of the proposed method under different anchor selection schemes on all datasets. The clustering results are shown in Figure 7. It can be seen that the clustering results of the DEA scheme consistently outperform the random selection and K-means anchor selection strategies. These results validate that DEA provides more favorable and effective anchor selection results for the sample imbalance problem that may occur in multi-omics clustering.
In order to further illustrate the effectiveness of the DEA anchor selection method for multi-omics data, we also show the results of anchor selection and the generated potential subspaces on the multi-omics dataset, as shown in Figure 8. The visualization of the two-dimensional space is performed using the t-Stochastic Neighbor Embedding (t-SNE)[43] method to downscale the original data into the potential subspaces, and different colors represent different clusters. The clustering effect of differentiation is visible in the potential subspace, which shows that the DEA algorithm selects high-quality anchors to generate higher-quality latent subspaces. Because of the excellent separation of these clusters, the Figure 8 explains how potential features learned from such high-quality anchors are robust to the downstream clustering algorithms, resulting in highly similar subtypes.
As shown in Tables 3 and 4, MBSLC performed excellently on the KRCCC dataset, detecting more enriched clinical parameter counts than all methods. In addition, as shown in Figure 3, the Kaplan-Meier survival curves for different subtypes are separated, indicating that MBSLC can distinguish the survival results of patients with different subtypes. To further clarify the biological significance of MBSLC, we analyzed the five cancer subtypes identified by MBSLC on the KRCCC dataset. First, we screened for genes with significant differences in mRNA expression between cancer subtypes using a t-test (P-adjust ≤ 0.05, FoldChange = 1).
Figure 9 shows a volcano plot of Differential Expression Genes (DEGs) among different subtypes, where the red dots, blue dots, and gray dots indicate up-regulated genes, down-regulated genes, and non-significantly differentially expressed genes, respectively. A total of 1173 DEGs were characterized, including DEGs widely reported to be associated with cancer, such as Mitogen-Activated Protein Kinase Kinase Kinase 1 (MAP3K1). MAP3K1 is a type of protein kinase that modulates the activity of Jun kinase [44], Erk MAP kinase, and the p38 signaling pathway, which is implicated in controlling cell proliferation and death. A somatic mutation of MAP3K1 has been identified in breast cancer, mainly in ER+ cases, the majority of which are protein-truncated. MAP3K1 phrases and activates proteins encoded by MAP2K4, a known recessive oncogene with significant inactivating mutations in breast and other cancers. MAP3K1 is a protein kinase that regulates Jun kinase and p38 signaling pathways that control cell proliferation and death[45]. Meanwhile, to get the differences of DEGs among different subtypes further, we plotted the heatmap of mRNA distribution of different subtypes in Figure 10. It can be seen that there are intergroup variations in the mRNA expression of the differentially expressed genes in different cancer subtypes, which further proves that the cancer subtypes identified by MBSLC have good interpretability and biological significance.
In order to reveal the molecular pathways and biological functions of these genes and provide a better understanding of their biological significance, we analyzed the identified DEGs by Gene Ontology and Kyoto Encyclopedia of Genes and Genomes (GO/KEGG) enrichment using the HiPlot online tool (https://hiplot.com.cn). Enrichment results for the top 7 pathways with the highest enrichment counts were displayed using a bubble plot, as shown in Figure 11. The X-axis represents the gene proportion, i.e., the proportion of differentially expressed proteins annotated by the pathway in the species, and the Y-axis represents the pathway name. In the bubble plot, the size and color of the bubbles represent the number of enriched genes and the P-value level, respectively. In Figure 11, the highly enriched GO pathways–biological process (BP), molecular function (MF) and cellular component (CC), regulate cell-cell adhesion, channel activity, and the apical part of the cell, respectively.
We estimated the impact of adding and combining different modules on our approach. We also developed three other versions. Some details of each version are outlined below:
1) MBSLC-NA (NA indicates no anchor): There is no module in this method. Specifically, no anchor selection is used, and the entire sample is used to construct the similarity graph; in other words, we can be seen as setting the anchor rate r to 1 in this method.
2) MBSLC-K: Anchor points are generated using the traditional K-means clustering method, where the center of mass of each cluster is used as each anchor point and the anchor point rate r is used as a parameter of the algorithm.
3) MBSLC: Both modules are added to the MBSLC-NA method as the overall MBCSLC method.
Figure 12(a) indicates the comparison of clustering performance in terms of -log10P value, by combining three different methods with SNF–i.e., MBSLC-NA, MBSLC-K, and MBSLC. Also, SNF is used as a baseline method. The X-axis represents different cancer datasets, and the Y-axis represents different cancer datasets with survival analysis -log10P values of the corresponding methods. The results of Figure 12(a) indicate that the performance of MBSLC continues to improve with the addition of different modules compared to the baseline method (SNF[18]). Methods that added all two modules outperformed other methods that lacked some modules.
In addition, more detailed information like significant clinical parameters, is shown in Figure 12(b). SNF is still used as a baseline method, and the highest standard is the MBSLC performance. The X-axis denotes different cancer datasets, and the Y-axis denotes different cancer datasets with the corresponding method clinical enrichment parameters.
In this study, a novel multi-omics bipartite graph clustering algorithm MBSLC, has been proposed to address the cancer subtype prediction problem. The MBSLC algorithm integrates the bipartite graph, latent subspace extraction, and spectral clustering techniques into a unified framework, which can improve both the effectiveness and efficiency of multi-omics clustering. To be specific, it first selects the anchor points that are representative of the original dataset based on the standard deviation in the tandem omics. Anchor points and raw data similarity map metric operations are then used to obtain overall bipartite maps. These bipartite maps were fused after feature vector extraction to form the latent subspace, thereafter spectral clustering is applied to the potential subspace. The anchor selection algorithm and potential subspace extraction reduce the algorithm's complexity. The experimental results show that MBSLC can outperform the state-of-the-art multi-omics clustering methods. Moreover, it reduces the time complexity without degrading the clustering performance.
In the future, we will attempt to improve the performance of our framework from two perspectives: (1) more effective anchor extraction methods can be investigated to enhance the quality of the anchors, and hence, to produce better clustering results; (2) recently, a few of the standard multi-omics fusion methods assign weights to each cluster according to the importance of the group, which is explainable in mathematical theory. However, the actual situation of real multi-omics data in applications should be more complicated. Therefore, we can explore alternative methods to obtain new multi-omics fusion strategies that reflect real scenarios better.
The authors declare they have not used Artificial Intelligence (AI) tools in the creation of this article.
This work was supported by the Natural Science Foundation of China (No. 62206113), the Natural Science Foundation of Jiangsu Province, China (No. BK20221067 and No. BK20230923), and the High-End Foreign Expert Recruitment Plan of China (No. G2023144007L). The cancer dataset used in this article was provided from the National Cancer Institute.
The authors declare that there is no conflict of interest.
[1] |
H. W. Hethcote, The mathematics of infectious diseases, SIAM Rev., 42 (2000), 599–653. https://doi.org/10.1137/S0036144500371907 doi: 10.1137/S0036144500371907
![]() |
[2] |
T. T. Marinov, R. S. Marinova, Dynamics of COVID-19 using inverse problem for coefficient identification in SIR epidemic models, Chaos Solit. Fract., 5 (2020). https://doi.org/10.1016/j.csfx.2020.100041 doi: 10.1016/j.csfx.2020.100041
![]() |
[3] |
J. T. Wu, K. Leung, M. Bushman, N. Kishore, R. Niehus, P. M. de Salazar, et al., Estimating clinical severity of COVID-19 from the transmission dynamics in Wuhan, China, Nat. Med., 26 (2020), 506–510. https://doi.org/10.1038/s41591-020-0822-7 doi: 10.1038/s41591-020-0822-7
![]() |
[4] |
S. Mangiarotti, M. Peyre, Y. Zhang, M. Huc, F. Roger, Y. Kerr, Chaos theory applied to the outbreak of COVID-19: An ancillary approach to decision making in pandemic context, Epidem. Infect, 148 (2020), 1–29. https://doi.org/10.1017/S0950268820000990 doi: 10.1017/S0950268820000990
![]() |
[5] |
S. Gounane, Y. Barkouch, A. Atlas, M. Bendahmane, F. Karami, D. Meskine, An adaptive social distancing SIR model for COVID-19 disease spreading and forecasting, Epidem. Methods, 10 (2021), 20200044. https://doi.org/10.1515/em-2020-0044 doi: 10.1515/em-2020-0044
![]() |
[6] |
G. Giordano, F. Blanchini, R. Bruno, P. Colaneri, A. Di Filippo, A. Di Matteo, et al., Modelling the COVID-19 epidemic and implementation of population-wide interventions in Italy, Nat. Med., 26 (2020), 855–860. https://doi.org/10.1038/s41591-020-0883-7 doi: 10.1038/s41591-020-0883-7
![]() |
[7] |
A. Ajbar, R. T. Alqahtani, M. Boumaza1, Dynamics of an SIR-Based COVID-19 Model With Linear Incidence Rate, Nonlinear Removal Rate, and Public Awareness, Front. Phys., (2021). https://doi.org/10.3389/fphy.2021.634251 doi: 10.3389/fphy.2021.634251
![]() |
[8] |
P. Kumara, V. S. Erturk, M. Murillo-Arcila, A new fractional mathematical modelling of COVID-19 with the availability of vaccine, Results Phys., 24 (2021), 2211–3797. https://doi.org/10.1016/j.rinp.2021.104213 doi: 10.1016/j.rinp.2021.104213
![]() |
[9] |
N. Gozalpour, E. Badfar, A. Nikoofard, Transmission dynamics of novel coronavirus SARS-CoV-2 among healthcare workers, a case study in Iran, Nonlinear Dynam., 105 (2021), 3749–-3761. https://doi.org/10.1007/s11071-021-06778-5 doi: 10.1007/s11071-021-06778-5
![]() |
[10] |
E. Badfar, E. J. Zaferani, A. Nikoofard, Design a robust sliding mode controller based on the state and parameter estimation for the nonlinear epidemiological model of Covid-19, Nonlinear Dynam., (2021), 5–-18. https://doi.org/10.1007/s11071-021-07036-4 doi: 10.1007/s11071-021-07036-4
![]() |
[11] |
A. Rajaei, M. Raeiszadeh, V. Azimi, M. Sharifi, State estimation-based control of COVID-19 epidemic before and after vaccine development, J. Pro. Control, 102 (2021), 1–14. https://doi.org/10.1016/j.jprocont.2021.03.008 doi: 10.1016/j.jprocont.2021.03.008
![]() |
[12] |
M. De la Sen, A. Ibeas, R. Nistal, About partial reachability issues in an SEIR epidemic model and related infectious disease tracking in finite time under vaccination and treatment controls, Discrete Dynam. Nat. Soc., (2021). https://doi.org/10.1155/2021/5556897 doi: 10.1155/2021/5556897
![]() |
[13] |
M. De la Sen, A. Ibeas, On an SE(Is)(Ih)AR epidemic model with combined vaccination and antiviral controls for COVID-19 pandemic, Adv. Difference Equ., 92 (2021). https://doi.org/10.1186/s13662-021-03248-5 doi: 10.1186/s13662-021-03248-5
![]() |
[14] |
S. Zhai, G. Luo, T. Huang, X. Wang, J. Tao, P. Zhou, Vaccination control of an epidemic model with time delay and its application to COVID-19, Nonlinear Dynam., 106 (2021), 1279–1292. https://doi.org/10.1007/s11071-021-06533-w doi: 10.1007/s11071-021-06533-w
![]() |
[15] |
E. Hwang, Prediction intervals of the COVID-19 cases by HAR models with growth rates and vaccination rates in top eight affected countries: Bootstrap improvement, Chaos Solit. Fract., 155 (2022). https://doi.org/10.1016/j.chaos.2021.111789 doi: 10.1016/j.chaos.2021.111789
![]() |
[16] |
P. Mahmood, M. Saeed, Stability of the equilibria in a discrete-time sivs epidemic model with standard incidence, Filomat, 33 (2019), 2393–2408. https://doi.org/10.1016/j.chaos.2021.111789 doi: 10.1016/j.chaos.2021.111789
![]() |
[17] |
M. De la Sen, S. Alonso-Quesada, A. Ibeas, On a Discrete SEIR Epidemic Model with Exposed Infectivity, Feedback Vaccination and Partial Delayed Re-Susceptibility, Mathematics, 9 (2021), 5–9. https://doi.org/10.3390/math9050520 doi: 10.3390/math9050520
![]() |
[18] |
M. De la Sen, S. Alonso-Quesada, A. Ibeas, R. Nistal, On a Discrete SEIR Epidemic Model with Two-Doses Delayed Feedback Vaccination Control on the Susceptible, Vaccines, 9 (2021). https://doi.org/10.3390/vaccines9040398 doi: 10.3390/vaccines9040398
![]() |
[19] |
Y. Omae, Y. Kakimoto, M. Sasaki, J. Toyotani, K. Hara, Y. Gon, et al., SIRVVD model-based verification of the effect of first and second doses of COVID-19/SARS-CoV-2 vaccination in Japan, Math. Biosci. Eng., 19 (2021), 1026–1040. https://doi.org/10.3934/mbe.2022047 doi: 10.3934/mbe.2022047
![]() |
[20] | N. Djenina, I. Rezzoug, A. Ouannas, T-E. Oussaeif, Giuseppe Grassi, A new COVID-19 pandemic model including the compartment of vaccinated individuals: Global stability of the disease-free fixed point, Submitted to CMMM, 2022 (2022). |
[21] |
P. van den Driesschea, J. Watmough, Reproduction numbers and sub-threshold endemic equilibria for compartmental models of disease transmission, Math. Biosci., 180 (2002). https://doi.org/10.1016/S0025-5564(02)00108-6 doi: 10.1016/S0025-5564(02)00108-6
![]() |
[22] |
S. Elaydi, An introduction to difference equations, Springer SBM, 3 (2005). https://doi.org/10.1007/0-387-27602-5 doi: 10.1007/0-387-27602-5
![]() |
Dataset | Sample | Three omics (features) |
BIC | 621 | Methylation (2000), mRNA (2000), miRNA (885) |
COAD | 220 | Methylation (2000), mRNA (2000), miRNA (613) |
GBM | 274 | Methylation (2000), mRNA (2000), miRNA (534) |
KRCCC | 184 | Methylation (2000), mRNA (2000), miRNA (791) |
LSCC | 341 | Methylation (2000), mRNA (2000), miRNA (881) |
BLCA | 338 | Protein (49), mRNA (5133), miRNA (262) |
LGG | 425 | Protein (45), mRNA (5133), miRNA (262) |
Dataset | age at initial diagnosis | gender | pathologic T | pathologic M | pathologic N | pathologic stage |
BIC | ✓ | ✓ | ✓ | ✓ | ✓ | ✓ |
COAD | ✓ | ✓ | ✓ | ✓ | ✓ | ✓ |
GBM | ✓ | ✓ | × | × | × | × |
KRCCC | ✓ | ✓ | ✓ | ✓ | ✓ | ✓ |
LSCC | ✓ | ✓ | × | × | × | × |
BLCA | ✓ | ✓ | ✓ | ✓ | ✓ | ✓ |
LGG | ✓ | ✓ | × | × | × | × |
Dataset | SNF | CC | SNF.CC | LRAcluster | Spectrum | Defusion | MOFA | NEMO | DSML | MCLS | MBSLC |
BIC | 1.7 | 2.2 | 3.8 | 0.2 | 5.4 | 5.3 | 5.7 | 1.9 | 3.9 | 8.1 | 11.2 |
COAD | 0.3 | 0.1 | 0.4 | 0.3 | 0.3 | 0.3 | 0.1 | 0.1 | 1.0 | 2.1 | 3.9 |
GBM | 3.2 | 1.6 | 2.9 | 1.6 | 4.2 | 4.5 | 6.7 | 3.9 | 2.3 | 6.8 | 7.7 |
KRCCC | 1.0 | 2.3 | 0.9 | 3.8 | 1.1 | 1.0 | 0.3 | 1.1 | 1.9 | 9.9 | 4.3 |
LSCC | 1.3 | 0.8 | 1.8 | 1.1 | 2.4 | 1.7 | 1.9 | 1.2 | 1.9 | 3.2 | 7.3 |
BLCA | 3.3 | 3.5 | 4.2 | 3.2 | 3.4 | 2.8 | 3.1 | 3.0 | 2.0 | 4.0 | 4.5 |
LGG | 14.5 | 10.4 | 14.8 | 10.9 | 12.7 | 16.0 | 15.1 | 14.6 | 12.3 | 16.0 | 28.3 |
Mean | 3.6 | 2.9 | 4.1 | 3.0 | 4.2 | 4.6 | 4.7 | 3.7 | 3.6 | 7.2 | 9.6 |
Dataset | SNF | CC | SNF.CC | LRAcluster | Spectrum | Defusion | MOFA | NEMO | DSML | MCLS | MBSLC |
BIC(6) | 2 | 2 | 2 | 3 | 4 | 3 | 3 | 2 | 3 | 4 | 4 |
COAD(6) | 1 | 1 | 1 | 1 | 2 | 2 | 1 | 1 | 1 | 2 | 3 |
GBM(2) | 1 | 1 | 1 | 1 | 1 | 1 | 2 | 1 | 2 | 2 | 2 |
KRCCC(6) | 2 | 4 | 3 | 2 | 5 | 4 | 4 | 2 | 3 | 4 | 5 |
LSCC(2) | 1 | 1 | 1 | 1 | 0 | 0 | 2 | 1 | 1 | 1 | 2 |
BLCA(6) | 5 | 4 | 5 | 5 | 5 | 5 | 4 | 4 | 2 | 6 | 5 |
LGG(2) | 1 | 1 | 2 | 1 | 1 | 1 | 1 | 2 | 2 | 2 | 2 |
Mean | 1.9 | 2.0 | 2.1 | 2.0 | 2.6 | 2.3 | 2.4 | 1.9 | 2.0 | 3.0 | 3.2 |
Dataset | MCLS | LRAcluster | DSML | MBCLS |
BIC | 0.612 | 0.715 | 0.662 | 0.561 |
COAD | 0.577 | 0.628 | 0.597 | 0.671 |
GBM | 0.575 | 0.636 | 0.622 | 0.962 |
KRCCC | 0.531 | 0.591 | 0.563 | 0.595 |
LSCC | 0.649 | 0.735 | 0.644 | 0.883 |
BLCA | 0.812 | 0.801 | 0.823 | 0.784 |
LGG | 0.586 | 0.635 | 0.646 | 0.701 |
Average | 0.620 | 0.677 | 0.651 | 0.737 |
Dataset | SNF | CC | SNF.CC | LRAcluster | Spectrum | Defusion | MOFA | NEMO | DSML | MCLS | MBSLC |
BIC | 42.0 | 12.2 | 1178.2 | 140.1 | 11.2 | 1140.8 | 46.2 | 19.0 | 2110.0 | 10.2 | 1.15 |
COAD | 7.1 | 3.1 | 71.0 | 22.0 | 1.3 | 605.2 | 28.2 | 5.8 | 602. 5 | 1.8 | 0.87 |
GBM | 6.9 | 4.2 | 124.1 | 45.2 | 2.3 | 838.8 | 32.1 | 6.1 | 807.3 | 2.4 | 0.42 |
KRCCC | 6.2 | 2.0 | 49.2 | 21.0 | 1.0 | 522.0 | 26.3 | 5.5 | 595.2 | 1.2 | 0.50 |
LSCC | 12.8 | 5.1 | 212.3 | 46.1 | 2.7 | 616.2 | 34.2 | 10.7 | 937.4 | 3.6 | 0.54 |
BLCA | 11.1 | 3.2 | 215.1 | 37.8 | 4.2 | 586.8 | 33.4 | 10.5 | 1007.2 | 2.9 | 0.95 |
LGG | 15.9 | 6.1 | 404.0 | 88.3 | 6.2 | 735.6 | 38.2 | 13.2 | 1376.4 | 4.3 | 0.47 |
Dataset | Sample | Three omics (features) |
BIC | 621 | Methylation (2000), mRNA (2000), miRNA (885) |
COAD | 220 | Methylation (2000), mRNA (2000), miRNA (613) |
GBM | 274 | Methylation (2000), mRNA (2000), miRNA (534) |
KRCCC | 184 | Methylation (2000), mRNA (2000), miRNA (791) |
LSCC | 341 | Methylation (2000), mRNA (2000), miRNA (881) |
BLCA | 338 | Protein (49), mRNA (5133), miRNA (262) |
LGG | 425 | Protein (45), mRNA (5133), miRNA (262) |
Dataset | age at initial diagnosis | gender | pathologic T | pathologic M | pathologic N | pathologic stage |
BIC | ✓ | ✓ | ✓ | ✓ | ✓ | ✓ |
COAD | ✓ | ✓ | ✓ | ✓ | ✓ | ✓ |
GBM | ✓ | ✓ | × | × | × | × |
KRCCC | ✓ | ✓ | ✓ | ✓ | ✓ | ✓ |
LSCC | ✓ | ✓ | × | × | × | × |
BLCA | ✓ | ✓ | ✓ | ✓ | ✓ | ✓ |
LGG | ✓ | ✓ | × | × | × | × |
Dataset | SNF | CC | SNF.CC | LRAcluster | Spectrum | Defusion | MOFA | NEMO | DSML | MCLS | MBSLC |
BIC | 1.7 | 2.2 | 3.8 | 0.2 | 5.4 | 5.3 | 5.7 | 1.9 | 3.9 | 8.1 | 11.2 |
COAD | 0.3 | 0.1 | 0.4 | 0.3 | 0.3 | 0.3 | 0.1 | 0.1 | 1.0 | 2.1 | 3.9 |
GBM | 3.2 | 1.6 | 2.9 | 1.6 | 4.2 | 4.5 | 6.7 | 3.9 | 2.3 | 6.8 | 7.7 |
KRCCC | 1.0 | 2.3 | 0.9 | 3.8 | 1.1 | 1.0 | 0.3 | 1.1 | 1.9 | 9.9 | 4.3 |
LSCC | 1.3 | 0.8 | 1.8 | 1.1 | 2.4 | 1.7 | 1.9 | 1.2 | 1.9 | 3.2 | 7.3 |
BLCA | 3.3 | 3.5 | 4.2 | 3.2 | 3.4 | 2.8 | 3.1 | 3.0 | 2.0 | 4.0 | 4.5 |
LGG | 14.5 | 10.4 | 14.8 | 10.9 | 12.7 | 16.0 | 15.1 | 14.6 | 12.3 | 16.0 | 28.3 |
Mean | 3.6 | 2.9 | 4.1 | 3.0 | 4.2 | 4.6 | 4.7 | 3.7 | 3.6 | 7.2 | 9.6 |
Dataset | SNF | CC | SNF.CC | LRAcluster | Spectrum | Defusion | MOFA | NEMO | DSML | MCLS | MBSLC |
BIC(6) | 2 | 2 | 2 | 3 | 4 | 3 | 3 | 2 | 3 | 4 | 4 |
COAD(6) | 1 | 1 | 1 | 1 | 2 | 2 | 1 | 1 | 1 | 2 | 3 |
GBM(2) | 1 | 1 | 1 | 1 | 1 | 1 | 2 | 1 | 2 | 2 | 2 |
KRCCC(6) | 2 | 4 | 3 | 2 | 5 | 4 | 4 | 2 | 3 | 4 | 5 |
LSCC(2) | 1 | 1 | 1 | 1 | 0 | 0 | 2 | 1 | 1 | 1 | 2 |
BLCA(6) | 5 | 4 | 5 | 5 | 5 | 5 | 4 | 4 | 2 | 6 | 5 |
LGG(2) | 1 | 1 | 2 | 1 | 1 | 1 | 1 | 2 | 2 | 2 | 2 |
Mean | 1.9 | 2.0 | 2.1 | 2.0 | 2.6 | 2.3 | 2.4 | 1.9 | 2.0 | 3.0 | 3.2 |
Dataset | MCLS | LRAcluster | DSML | MBCLS |
BIC | 0.612 | 0.715 | 0.662 | 0.561 |
COAD | 0.577 | 0.628 | 0.597 | 0.671 |
GBM | 0.575 | 0.636 | 0.622 | 0.962 |
KRCCC | 0.531 | 0.591 | 0.563 | 0.595 |
LSCC | 0.649 | 0.735 | 0.644 | 0.883 |
BLCA | 0.812 | 0.801 | 0.823 | 0.784 |
LGG | 0.586 | 0.635 | 0.646 | 0.701 |
Average | 0.620 | 0.677 | 0.651 | 0.737 |
Dataset | SNF | CC | SNF.CC | LRAcluster | Spectrum | Defusion | MOFA | NEMO | DSML | MCLS | MBSLC |
BIC | 42.0 | 12.2 | 1178.2 | 140.1 | 11.2 | 1140.8 | 46.2 | 19.0 | 2110.0 | 10.2 | 1.15 |
COAD | 7.1 | 3.1 | 71.0 | 22.0 | 1.3 | 605.2 | 28.2 | 5.8 | 602. 5 | 1.8 | 0.87 |
GBM | 6.9 | 4.2 | 124.1 | 45.2 | 2.3 | 838.8 | 32.1 | 6.1 | 807.3 | 2.4 | 0.42 |
KRCCC | 6.2 | 2.0 | 49.2 | 21.0 | 1.0 | 522.0 | 26.3 | 5.5 | 595.2 | 1.2 | 0.50 |
LSCC | 12.8 | 5.1 | 212.3 | 46.1 | 2.7 | 616.2 | 34.2 | 10.7 | 937.4 | 3.6 | 0.54 |
BLCA | 11.1 | 3.2 | 215.1 | 37.8 | 4.2 | 586.8 | 33.4 | 10.5 | 1007.2 | 2.9 | 0.95 |
LGG | 15.9 | 6.1 | 404.0 | 88.3 | 6.2 | 735.6 | 38.2 | 13.2 | 1376.4 | 4.3 | 0.47 |