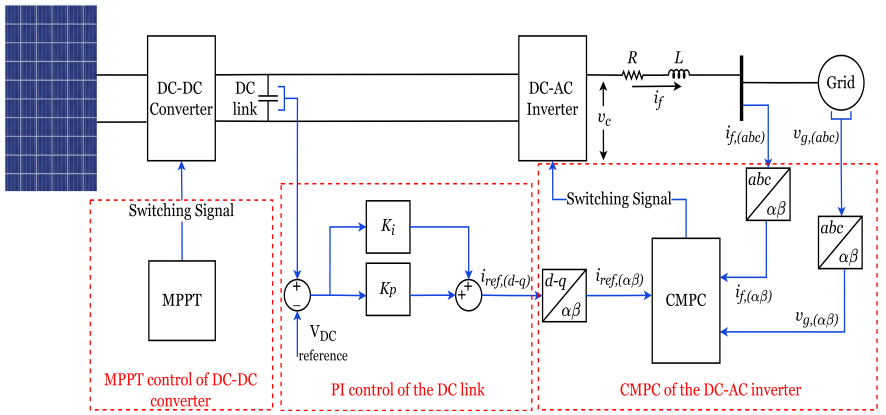
This paper investigates the performance of the current model predictive control (CMPC) for controlling a two-stage transformerless grid-connected photovoltaic (PV) system under grid fault conditions. A maximum power point tracking (MPPT) controller was used to extract the maximum power of the PV panel. To stabilize the DC link and generate the reference current values, a proportional-integral (PI) controller was used. The CMPC strategy was implemented to control the output current of the inverter that connects the PV system to the utility grid. The system and control strategy were simulated via a MATLAB/Simulink environment. The performance of the proposed control strategy was investigated under fault conditions between the three-phase two-level inverter and the grid. Moreover, to validate the capability of the CMPC, comparative case studies were conducted between CMPC, PI, and sliding mode control (SMC) under grid fault. Case studies' results showed that under grid fault, CMPC did not introduce any overshoot or undershoot in the PV output DC current and power. However, PI and SMC produced undershoots of almost 15 kW for the output power and 45 A for the output current. Under the fault conditions, the active output power and three-phase current recovery time of the inverter was 50 ms using CMPC, compared to PI and SMC with recovery times of 80 ms and 60 ms, respectively. Moreover, a voltage dip of 75 V at the DC link voltage was recorded with CMPC under faulty conditions, while the voltage dips for PI and SMC were around 180 V.
Citation: Abdulrahman J. Babqi, NasimUllah, Ahmed Althobaiti, Hend I. Alkhammash, Asier Ibeas. Current model predictive fault-tolerant control for grid-connected photovoltaic system[J]. AIMS Energy, 2022, 10(2): 273-291. doi: 10.3934/energy.2022015
[1] | Zeid Al Qaisi, Qais Alsafasfeh, Ahmad Harb . Stability impact of integrated small scale hybrid (PV/Wind) system with electric distribution network. AIMS Energy, 2018, 6(5): 832-845. doi: 10.3934/energy.2018.5.832 |
[2] | K. M. S. Y. Konara, M. L. Kolhe, Arvind Sharma . Power dispatching techniques as a finite state machine for a standalone photovoltaic system with a hybrid energy storage. AIMS Energy, 2020, 8(2): 214-230. doi: 10.3934/energy.2020.2.214 |
[3] | Amer Braik, Asaad Makhalfih, Ag Sufiyan Abd Hamid, Kamaruzzaman Sopian, Adnan Ibrahim . Impact of photovoltaic grid-tied systems on national grid power factor in Palestine. AIMS Energy, 2022, 10(2): 236-253. doi: 10.3934/energy.2022013 |
[4] | Katherin Indriawati, Bambang L. Widjiantoro, Ali Musyafa . Design of sensor and actuator fault tolerant control system on wind turbine benchmark for Region II. AIMS Energy, 2019, 7(2): 111-126. doi: 10.3934/energy.2019.2.111 |
[5] | Mohamed G Moh Almihat . An overview of AC and DC microgrid energy management systems. AIMS Energy, 2023, 11(6): 1031-1069. doi: 10.3934/energy.2023049 |
[6] | Rashid Al Badwawi, Mohammad Abusara, Tapas Mallick . Speed control of synchronous machine by changing duty cycle of DC/DC buck converter. AIMS Energy, 2015, 3(4): 728-739. doi: 10.3934/energy.2015.4.728 |
[7] | Carla Viveiros, Rui Melicio, Victor Mendes, Jose Igreja . Adaptive and predictive controllers applied to onshore wind energy conversion system. AIMS Energy, 2018, 6(4): 615-631. doi: 10.3934/energy.2018.4.615 |
[8] | Hari Charan Nannam, Atanu Banerjee . A novel control technique for a single-phase grid-tied inverter to extract peak power from PV-Based home energy systems. AIMS Energy, 2021, 9(3): 414-445. doi: 10.3934/energy.2021021 |
[9] | Nagaraj C, K Manjunatha Sharma . Fuzzy PI controller for bidirectional power flow applications with harmonic current mitigation under unbalanced scenario. AIMS Energy, 2018, 6(5): 695-709. doi: 10.3934/energy.2018.5.695 |
[10] | Arqum Shahid, Ahsan Ali, Faheem Ashiq, Umer Amir Khan, Muhammad Ilyas Menhas, Sajjad Manzoor . Comparison of resistive and inductive superconductor fault current limiters in AC and DC micro-grids. AIMS Energy, 2020, 8(6): 1199-1211. doi: 10.3934/energy.2020.6.1199 |
This paper investigates the performance of the current model predictive control (CMPC) for controlling a two-stage transformerless grid-connected photovoltaic (PV) system under grid fault conditions. A maximum power point tracking (MPPT) controller was used to extract the maximum power of the PV panel. To stabilize the DC link and generate the reference current values, a proportional-integral (PI) controller was used. The CMPC strategy was implemented to control the output current of the inverter that connects the PV system to the utility grid. The system and control strategy were simulated via a MATLAB/Simulink environment. The performance of the proposed control strategy was investigated under fault conditions between the three-phase two-level inverter and the grid. Moreover, to validate the capability of the CMPC, comparative case studies were conducted between CMPC, PI, and sliding mode control (SMC) under grid fault. Case studies' results showed that under grid fault, CMPC did not introduce any overshoot or undershoot in the PV output DC current and power. However, PI and SMC produced undershoots of almost 15 kW for the output power and 45 A for the output current. Under the fault conditions, the active output power and three-phase current recovery time of the inverter was 50 ms using CMPC, compared to PI and SMC with recovery times of 80 ms and 60 ms, respectively. Moreover, a voltage dip of 75 V at the DC link voltage was recorded with CMPC under faulty conditions, while the voltage dips for PI and SMC were around 180 V.
Due to fossil fuel environmental impacts, renewable energy sources (RESs) have been increasingly used across the globe [1]. Solar energy, especially photovoltaic (PV) energy, is considered one of the most attractive renewable energy technologies due to its abundant energy source and availability [2,3]. According to the International Energy Agency Renewable 2021 report, an annual addition of 305 GW per year of renewable energy capacity is expected to be utilized between 2021 and 2026 [4]. The solar PV annual market is expected to grow by 17% to approximately 160 GW in 2021, reaching 200 GW in 2026. Even though renewable energy sources provide better alternatives to conventional power plants, the variation of renewable energy outputs poses major technical issues in integrating these sources into the power grid [5,6]. Moreover, the output nature of these sources, such as DC, AC, and frequency level, makes these sources unsuitable to be interconnected with conventional power generation plants. Distributed generator systems (DGSs) enabled the idea of integrating RESs into the existing power grids. In general, a DGS usually consists of a power generator such as a solar PV or wind generator connected to the utility grid via a power electronic device. Depending on the nature of the systems' output (i.e., DC or AC), the power electronic devices that are utilized for the integration are chosen. These devices provide the opportunity to perform different control tasks, such as flexible power flow, voltage and frequency control, or maximum power point tracking (MPPT) [7,8].
Different topologies have been used for integrating solar PV systems into existing power grids [10,11,12,13]. One of the most commonly used topologies is the two-stages transformerless [9]. Such topology consists of a DC-DC converter and a DC-AC three-phase two-level inverter. The converter extracts the PV maximum power and boosts the output voltage of the PV generator, while the inverter transfers the DC voltage to AC voltage that is compatible with the power grid voltage level. Other topologies that reduce the leakage current of a single-phase grid connected PV system were introduced in [14,15,16]. These works implemented different power electronics devices such as H5, H6, and HERIC inverters, which show a remarkable reduction of PV solar system leakage current. An H7 three-phase inverter for integrating PV with leakage current reduction was proposed in [17]. Besides leakage current, many issues (e.g., nonlinear load and grid fault) can affect the power grid stability. In the case of grid fault, it requires the system to be capable of restoring the system operation to the normal conditions.
Various control techniques have been implemented to control the PV systems' outputs such as voltage and power. The classical proportional integral (PI) method has been widely used for integrating PV systems. A fuzzy logic MPPT combined with a PI controller for charging batteries was presented in [18]. A small-signal modeling and designing of a multi-variable PI controller for a voltage source inverter in a high-voltage DC system was introduced in [19]. In [20], an adaptive PI control method to maintain the DC link capacitor voltage balance in a solar-PV-fed grid-connected neutral point clamped inverter was proposed. PI control combined with artificial neural networks for controlling a PV system supplying a direct current water pump was presented in [21]. The authors in [22] proposed an optimal PI tuning method using a genetic algorithm for controlling a single-phase grid-connected PV system. The research work in [23] introduced a PI controller-based active filter for a grid-connected PV system's current harmonics compensation. The previous works show that PI controllers have a fast dynamic response as well as satisfactory steady-state behavior. However, the PI controller requires external signals modulation generation and excessive time and effort for tuning parameters. Moreover, severe faults may deteriorate the system's stability.
Sliding mode controllers (SMC) offer robustness to uncertainties, upper bounded disturbances and parameter variations, and the derivation of such controllers relies on the availability of system models [24]. These controllers have been frequently reported in renewable energy applications, such as super twisting SMC for grid connected PV systems [25], non integer SMC for DC micro-grid stability problems [26], adaptive non integer SMC for wind energy [27], industrial grade robust controllers [28] and fractional order control for wind energy [29]. Although SMC offer robust performance, however, such controllers compromise robustness when practically implemented using digital processors.
Another control technique method that has been implemented for controlling grid-connected PV systems is model predictive control (MPC). Compared to other types of controllers, MPC provides some advantages, including ease of implementation, the ability to control different variables at the same time, and the ability to add limitations in the control objective. All these features and others make MPC an attractive control method not only for grid-connected PV systems but also for different applications in power systems. In [30], an MPPT and leakage current reduction technique using MPC for the H5 inverter of the PV system was proposed. Voltage regulation and an MPPT method based on MPC for a two-stage grid-connected PV system were introduced in [31]. The work in [32] proposed a current sensor-less model predictive control of an MPPT algorithm. A new MPPT technique of a PV module based on MPC was presented in [33]. The authors in [34] proposed an MPC strategy and a high-efficiency two-stage topology for a single-phase PV system.
This paper investigates the performance of the current model predictive control (CMPC) for a two-stage three-phase PV system under grid fault conditions. To verify the CMPC capability, the results of the CMPC under grid fault were compared to the PI controller. Moreover, the results were compared with our previously proposed sliding mode control (SMC) [35]. The rest of the paper is organized as follows: Section 2 describes the grid connected PV system, section 3 derives the converter controller, and section 4 discusses the simulation results. Finally, the conclusion is drawn based on the presented data and results.
The PV system considered in this work is shown in Figure 1. A two-stage transformerless topology is implemented to integrate the PV system into the main grid. The system consists of a PV panel connected to a DC-DC converter and then is connected to a DC-AC three-phase two-level inverter which delivers the power to the grid through an RL filter. The DC-DC converter is controlled via an MPPT algorithm to ensure maximum output power during the PV panel operation. Then, the DC link voltage is controlled via a PI controller in the d-q reference frames to generate the reference currents for the CMPC controller. After transferring the current reference values from the d-q frames to α-β, the reference values are then sent to the CMPC, which controls the output current of the three-phase two-level inverter. Figure 1 shows the schematic diagram of the PV system control process implemented in this work.
Maximum power point tracking (MPPT) controllers are widely utilized for harvesting peak power from stochastic renewable energy sources using high-frequency power converters interface. In the existing literature, several MPPT methods have been discussed, such as perturb and observed, incremental conductance, ripple correlation, and fuzzy logic based maximum power harvesting methods. However, the incremental conductance algorithm is the most widely utilized technique and is easy to implement. In this work, the incremental conductance algorithm is used to extract maximum power from the energy source. The detailed diagram of the incremental conductance algorithm is shown in Figure 2.
In this subsection, the DC link voltage model is realized from the system block diagram of Figure 1, and then a proportional integral (PI) is utilized to stabilize the DC link voltage to a reference value. As given in Figure 1, a DC link capacitor stores input power from the PV panel in the form of energy and then delivers the stored energy to the inverter. So, the dynamics across the DC link capacitor are expressed as follows:
˙Vc=1CVc(Pin−32Vgdifd) | (3.1) |
where Vc represents DC link voltage, Pin is the PV power stored in the DC link capacitors, Vgd represents grid voltage, and ifd is the grid current.
In order to control the voltage dynamics of Eq 3.1, a PI controller is utilized and expressed as follows:
id−ref=kpeVc+ki∫eVc | (3.2) |
where eVc is the voltage error, and kp and ki are the PI proportional and integral gains, respectively.
The model predictive control strategy for controlling power electronic devices is based on the fact that there is only a finite number of switching states related to the voltage vectors of a specific power electronic device [36]. Through an optimization process, the system model is used to predict the future behavior of the control variables for each voltage vector. Then, the optimal voltage vector that results in the least error between the reference and controlled value is selected. The optimal voltage vector selection is done via a cost function that evaluates all the possible switching states and selects the optimal solution. To implement the MPC strategy, the following steps must be identified:
● Continuous-time system model,
● Discrete-time system model,
● Cost function to select the optimal voltage vector,
● Generation of switching signals related to the selected optimal voltage vector.
The block diagram in Figure 3 illustrates the MPC process. The current values that have been measured x(k) are used in the system model to forecast future values x(k+1). Then, by minimizing the error between the future and reference values, a cost function assesses all future values. Finally, the switching signals are generated by the MPC controller.
The three-phase inverter topology is shown in Figure 4. It is made up of three legs, each having two switches. Since the upper switches are complementary with the lower ones, each leg results in two control actions, which means that the three-phase two-level inverter produces eight different switching state combinations [37]. The three-phase two-level inverter's voltage state-space vector can be written as
Vx=23Vc(S1+aS2+a2S3) | (3.3) |
where Vx is the output voltage of the inverter, and a equals ej2π3. The state-space vectors of the three-phase two-level inverter are illustrated in Table 1.
Mode | S1 | S2 | S3 | Vc |
1 | 0 | 0 | 0 | 0 |
2 | 0 | 0 | 1 | 23Vdc∠0∘ |
3 | 0 | 1 | 1 | 23Vdc∠60∘ |
4 | 0 | 1 | 0 | 23Vdc∠120∘ |
5 | 1 | 1 | 0 | 23Vdc∠180∘ |
6 | 1 | 0 | 0 | 23Vdc∠240∘ |
7 | 1 | 0 | 1 | 23Vdc∠300∘ |
8 | 1 | 1 | 1 | 0 |
The mathematical model of the system AC side in Figure 1 can be derived as
Vx=VR+VL+Vg | (3.4) |
where VL is the voltage across the filter inductor, VR is the voltage across the equivalent series resistance (ESR) of the inductor, and Vg is the main grid voltage. The current load dynamics can be expressed as
Vx=Rif+Ldifdt+Vg | (3.5) |
where the current flow from the inverter to the main grid is denoted by if. Eq 3.5 can be rearranged in the following way:
Ldifdt=Vx−(Rif+Vg). | (3.6) |
To predict the future values of inverter output current, the system discrete-time must be optioned [38]. Using the approximation derivative equation
dxdt≈x(k+1)−x(k)Ts | (3.7) |
where x(k+1) is the future value, x(k) is the present value, and Ts is the sampling time, the discrete-time of Eq 3.6 is
Lif(k+1)−if(k)Ts=Vx(k)−(Rif(k)+Vg(k)). | (3.8) |
After rearrangement of Eq 3.8, the future value of the inverter output current is predicted as
if(k+1)=if(k)+TsL(Vx(k)−(Rif(k)+Vg(k))). | (3.9) |
Since there are eight voltage vectors of the three-phase two-level inverter Vs (Table 1), the CMPC predicts eight values of the current future value in each sampling time Ts.
The square error cost function (3.10) is used to evaluate each predicted value and select the optimal solution. In other words, the cost function minimizes the error for each future value of the eight predicted values in Eq 3.9. After that, the best voltage vector is chosen, and the CMPC generates the best switching states to send to the inverter. Note that the CMPC does this procedure every Ts sampling period. Figure 5 illustrates the CMPC strategy algorithm.
g=(i∗(k+1)−i(k+1))2 | (3.10) |
In order to investigate the performance of the CMPC under grid fault, the system in Figure 1 was simulated using a MATLAB/Simulink environment. A comparative analysis between CMPC and PI control was conducted. For further investigation, the results are also compared to our previously proposed SMC. The details of implementing the SMC strategy for the PV two-stage transformerless topology were presented in [29]. The parameters of the system in Figure 1, MPC and PI controller are shown in Table 2. Figure 6 illustrates the input sun irradiance waveform to the PV power generator. A short circuit fault between grids' phase A and ground is applied at t = 1 s. The fault duration is set to one cycle, which approximately equals 40 ms. The fault resistance is set to 1.31 Ω, while the ground resistance is 0.01 Ω.
Parameter | Symbol | Value |
PV Rated Power | VPV | 5 kW |
DC-link Reference Voltage | VDC | 800 V |
Filter Inductance | L | 2.8 mH |
ESR of L | R | 0.02 Ω |
Utility Grid Voltage | Vg | 380 VLL,RMS |
Grid Frequency | fgrid | 50 Hz |
Sampling Time | Ts | 10 e−6 |
Proportional Gain | Kp | 1.6 |
Integral Gain | Ki | 20 |
Figure 7 shows the MPPT reference value and the actual PV output power using CMPC, PI, and SMC controllers. As can be seen, using CMPC, the PV output power follows the MPPT reference value smoothly even under grid fault. The CMPC does not produce any overshoot or undershooting of PV output power signals. Even though the PV output powers using PI and SMC controllers follow the reference value, they both suffer from undershooting, which almost equals 15 kW in both cases of PI and SMC controlling strategies. Therefore, Figure 7 proves that CMPC has a satisfactory performance in stabilizing PV output power under grid fault compared to PI and SMC controllers.
Similar to the PV output power, the PV output current tracks the radiation value smoothly using the three controllers. Figure 8 shows the PV output current of the CMPC, PI, and SMC. Under the grid fault, CMPC maintains the PV output current value at the same level without any oscillation, overshooting, or undershooting. In contrast, a jump of 45 A is introduced using PI and SMC during the grid fault.
The DC link voltage is shown in Figure 9. As is illustrated in the figure, the recovery time for the DC link voltage is approximately 150 ms using all three controllers. However, CMPC results in less overshoot and undershoot of the DC link voltage under grid fault. A zoomed version of Figure 9 is presented in Figure 10. As can be seen, CMPC introduces a maximum of 75 V undershooting, while PI and SMC result in 180 V and 185 V, respectively. Moreover, CMPC produces less overshooting, with 20 V; however, PI and SMC result in almost 65 V overshooting. As a result, Figures 9 and 10 show the capability of the CMPC in stabilizing the DC link voltage compared to both PI and SMC during grid fault.
Figures 11 and 12 present the output active power of the inverter. It is evident that the three controllers are capable of tracking the reference values smoothly. Even though CMPC has a bit more undershoot than the PI and SMC controllers, its recovery time is considered the lowest, at 50 ms. However, the recovery times of SMC and PI controllers are 60 ms and 80 ms, respectively. The inverter's reactive powers are shown in Figures 13 and 14. Since the q axis reference current is set to zero, the three-phase two-level inverter's output reactive power should be zero. It is evident from the figures that both CMPC and SMC are capable of maintaining the reactive power at zero even under the grid fault, while the PI controllers introduce some noticeable oscillations in the reactive power. That is because of the parameters decoupling issues that the PI controller imposes.
The three-phase two-level inverters' output currents using the CMPC, PI, and SMC are shown in Figure 15. The comparative analysis shows that the recovery time of the output current under grid fault is 50 ms, while the recovery times for PI and SMC are 80 ms and 60 ms, respectively. The total harmonic distortion of the three-phase two-level inverter output current using the three control strategies is shown in Figure 16. As can be seen, CMPC results in a lower total harmonic distortion percentage and also a lower settling time compared to PI and SMC.
This research work analyzes and investigates the CMPC strategy performance for a PV two-stages transformerless topology under grid fault conditions. A comparative analysis between CMPC, PI, and SMC is conducted under grid fault. The results illustrate that CMPC has a satisfactory performance compared to PI and SMC under faulty conditions. First, CMPC does not result in any overshoot or undershoot for the PV output current and power. In contrast, PI and SMC result in undershoot, with almost 15 kW and 45 A for the PV output power and current, respectively. Second, the settling time of the three-phase inverter's output active power and current is 50 ms using CMPC. However, the settling times of the PI and SMC are 80 ms and 60 ms, respectively. Finally, CMPC results in less voltage dip (75 V) of the DC link voltage compared to a voltage dip of 180 V for both PI and SMC.
This work was supported by the Research Groups Program funded by the Deanship of Scientific Research, Taif University, Ministry of Education, Saudi Arabia, under Grant 1-441-106.
The authors declare no conflict of interest.
[1] |
Owusu PA, Asumadu-Sarkodie S (2016) A review of renewable energy sources, sustainability issues and climate change mitigation. Cogent Eng 3: 1167990. https://doi.org/10.1080/23311916.2016.1167990 doi: 10.1080/23311916.2016.1167990
![]() |
[2] |
Hoseinzadeh S, Garcia DA (2022) Numerical analysis of thermal, fluid, and electrical performance of a photovoltaic thermal collector at new micro-channels geometry. J Energy Resour Technol 144: 062105. https://doi.org/10.1115/1.4052672 doi: 10.1115/1.4052672
![]() |
[3] | Hoseinzadeh S, Sohani A, Samiezadeh S, et al. (2020) Using computational fluid dynamics for different alternatives water flow path in a thermal photovoltaic (PVT) system. Int J Numer Methods Heat Fluid Flow. https://doi.org/10.1108/HFF-02-2020-0085 |
[4] | IEA (2021) Renewables 2021, IEA, Paris. Available from : https://www.iea.org/reports/renewables-2021. |
[5] |
Rehmani MH, Reisslein M, Rachedi A, et al. (2018) Integrating renewable energy resources into the smart grid: Recent developments in information and communication technologies. IEEE Trans Industr Inf 14: 2814–2825. https://doi.org/10.1109/TII.2018.2819169 doi: 10.1109/TII.2018.2819169
![]() |
[6] |
Makkiabadi M, Hoseinzadeh S, Mohammadi M, et al. (2020) Energy feasibility of hybrid PV/wind systems with electricity generation assessment under Iran environment. Appl Solar Energy 56: 517–525. https://doi.org/10.3103/S0003701X20060079 doi: 10.3103/S0003701X20060079
![]() |
[7] |
Al-Shetwi AQ, Hannan MA, Jern KP, et al. (2020) Power quality assessment of grid-connected PV system in compliance with the recent integration requirements. Electronics 9: 366. https://doi.org/10.3390/electronics9020366 doi: 10.3390/electronics9020366
![]() |
[8] | Shahid A (2018) Smart grid integration of renewable energy systems. 2018 7th International Conference on Renewable Energy Research and Applications (ICRERA), 944–948. https://doi.org/10.1109/ICRERA.2018.8566827 |
[9] |
Zeb K, Nazir MS, Ahmad I, et al. (2021) Control of transformerless inverter-based two-stage gridconnected photovoltaic system using Adaptive-PI and adaptive sliding mode controllers. Energies 14: 2546. https://doi.org/10.3390/en14092546 doi: 10.3390/en14092546
![]() |
[10] |
Hoseinzadeh S, Garcia DA (2022) Numerical analysis of thermal, fluid, and electrical performance of a photovoltaic thermal collector at new micro-channels geometry. J Energy Resour Technol 144: 062105. https://doi.org/10.1115/1.4052672 doi: 10.1115/1.4052672
![]() |
[11] |
Hoseinzadeh S, Sohani A, Samiezadeh S, et al. (2020) Using computational fluid dynamics for different alternatives water flow path in a thermal photovoltaic (PVT) system. Int J Numer Methods Heat Fluid Flow. 31: 1618–1637. https://doi.org/10.1108/HFF-02-2020-0085 doi: 10.1108/HFF-02-2020-0085
![]() |
[12] |
Sohani A, Dehnavi A, Sayyaadi H, et al. (2022) The real-time dynamic multi-objective optimization of a building integrated photovoltaic thermal (BIPV/T) system enhanced by phase change materials. J Energy Storage 46: 103777. https://doi.org/10.1016/j.est.2021.103777 doi: 10.1016/j.est.2021.103777
![]() |
[13] |
Khodayar SH, Hoseinzadeh S, Ghadamian H, et al. (2021) Techno-economic analysis and new design of a photovoltaic power plant by a direct radiation amplification system. Sustainability 13: 11493. https://doi.org/10.3390/su132011493 doi: 10.3390/su132011493
![]() |
[14] | Rizzoli G, Mengoni M, Zarri L, et al. (2016) Comparison of single-phase H4, H5, H6 inverters for transformerless photovoltaic applications. IECON 2016—42nd Annual Conference of the IEEE Industrial Electronics Society, 3038–3045. https://doi.org/10.1109/IECON.2016.7792984 |
[15] | Islam M, Hasan M, Akter P, et al. (2014) A new transformerless inverter for grid connected photovoltaic system with low leakage current. 2013 International Conference on Electrical Information and Communication Technology (EICT), 1–6. https://doi.org/10.1109/EICT.2014.6777840 |
[16] |
Lokhande N, Phadnis G (2017) Comparison of full bridge transformerless H5, HERIC, H6 inverter topologies. Int J Innovative Res Sci Eng Technol 6: 10870–10881. https://doi.org/10.15680/IJIRSET.2017.0606136 doi: 10.15680/IJIRSET.2017.0606136
![]() |
[17] |
Freddy TKS, Rahim NA, Hew WP, et al. (2014) Modulation techniques to reduce leakage current in three-phase transformerless H7 photovoltaic inverter. IEEE Trans Ind Electron 62: 322–331. https://doi.org/10.1109/TIE.2014.2327585 doi: 10.1109/TIE.2014.2327585
![]() |
[18] |
Yilmaz U, Kircay A, Borekci S (2018) PV system fuzzy logic MPPT method and PI control as a charge controller. Renewable Sustainable Energy Rev 81: 994–1001. https://doi.org/10.1016/j.rser.2017.08.048 doi: 10.1016/j.rser.2017.08.048
![]() |
[19] |
Pradhan JK, Ghosh A, Bhende CN (2017) Small-signal modeling and multivariable PI control design of VSC-HVDC transmission link. Electr Power Syst Res 144: 115–126. https://doi.org/10.1016/j.epsr.2016.11.005 doi: 10.1016/j.epsr.2016.11.005
![]() |
[20] |
Malakondareddy B, Kumar SS, Gounden NA, et al. (2019) An adaptive PI control scheme to balance the neutral-point voltage in a solar PV fed grid connected neutral point clamped inverter. Int J Electri Power & Energy Syst 110: 318–331. https://doi.org/10.1016/j.ijepes.2019.03.012 doi: 10.1016/j.ijepes.2019.03.012
![]() |
[21] |
Attia H (2019) High performance PV system based on artificial neural network MPPT with PI controller for direct current water pump applications. Int J Power Electron Drive Syst 10: 1329–1338. http://doi.org/10.11591/ijpeds doi: 10.11591/ijpeds
![]() |
[22] |
Abo-Elyousr FK, Abdelaziz AY (2018) Optimal PI microcontroller-based realization for technical trends of single-stage single-phase grid-tied PV. Eng Sci Technol Int J 21: 945–956. https://doi.org/10.1016/j.jestch.2018.07.007 doi: 10.1016/j.jestch.2018.07.007
![]() |
[23] |
Khandelwal A, Nema P (2021) Application of PI controller based active filter for harmonic mitigation of grid-connected PV-system. Bulletin Electri Eng Inf 10: 2377–2383. https://doi.org/10.11591/eei.v10i5.2907 doi: 10.11591/eei.v10i5.2907
![]() |
[24] |
Young KD, Utkin VI, Ozguner U (1999) A control engineer's guide to sliding mode control. IEEE Trans Control Syst Technol 7: 328–342. https://doi.org/10.1109/87.761053 doi: 10.1109/87.761053
![]() |
[25] | Del Pizzo A, Di Noia LP, Meo S (2017) Super twisting sliding mode control of smart-inverters grid-connected for PV applications. 2017 IEEE 6th International Conference on Renewable Energy Research and Applications (ICRERA), 793–796. https://doi.org/10.1109/ICRERA.2017.8191168 |
[26] |
Asghar M, Khattak A, Rafiq MM (2017) Comparison of integer and fractional order robust controllers for DC/DC converter feeding constant power load in a DC microgrid. Sustainable Energy Grids Networks 12: 1–9. https://doi.org/10.1016/j.segan.2017.08.003 doi: 10.1016/j.segan.2017.08.003
![]() |
[27] |
Ullah N, Sami I, Chowdhury MS, et al. (2020) Artificial intelligence integrated fractional order control of doubly fed induction generator-based wind energy system. IEEE Access 9: 5734–5748. https://doi.org/10.1109/ACCESS.2020.3048420 doi: 10.1109/ACCESS.2020.3048420
![]() |
[28] |
Ullah N, Farooq Z, Sami I, et al. (2020) Industrial grade adaptive control scheme for a micro-grid integrated dual active bridge driven battery storage system. IEEE Access 8: 210435–210451. https://doi.org/10.1109/ACCESS.2020.3039947 doi: 10.1109/ACCESS.2020.3039947
![]() |
[29] |
Sami I, Ullah S, Ali Z, et al. (2020) A super twisting fractional order terminal sliding mode control for DFIG-based wind energy conversion system. Energies 13: 2158. https://doi.org/10.3390/en13092158 doi: 10.3390/en13092158
![]() |
[30] | Babqi AJ, Yi Z, Shi D, et al. (2018) Model predictive control of H5 inverter for transformerless PV systems with maximum power point tracking and leakage current reduction. IECON 2018—44th Annual Conference of the IEEE Industrial Electronics Society, 1860–1865. https://doi.org/10.1109/IECON.2018.8591386 |
[31] |
Ma M, Liu X, Lee KY (2020) Maximum power point tracking and voltage regulation of two-stage grid-tied PV syst based model predict control. Energies 13: 1304. https://doi.org/10.3390/en13061304 doi: 10.3390/en13061304
![]() |
[32] |
Metry M, Balog RS (2020) An adaptive model predictive controller for current sensorless MPPT in PV systems. IEEE Open J Power Electron 1: 445–455. https://doi.org/10.1109/OJPEL.2020.3026775 doi: 10.1109/OJPEL.2020.3026775
![]() |
[33] |
Zhao Y, An A, Xu Y, et al. (2021) Model predictive control of grid-connected PV power generation system considering optimal MPPT control of PV modules. Prot Control Mod Power Syst 6: 1–12. https://doi.org/10.1186/s41601-021-00210-1 doi: 10.1186/s41601-021-00210-1
![]() |
[34] |
Liu J, Cheng S, Liu Y, et al. (2019) FCS-MPC for a single-phase two-stage grid-connected PV inverter. IET Power Electron 12: 915–922. https://doi.org/10.1049/iet-pel.2018.5676 doi: 10.1049/iet-pel.2018.5676
![]() |
[35] | Ullah N, Sami I, Jamal BA, et al. (2021) Processor in the loop verification of fault tolerant control for a three phase inverter in grid connected PV system. Energy Sources 1–17. https://doi.org/10.1080/15567036.2021.2015486 |
[36] |
Xia C, Liu T, Shi T, et al. (2013) A simplified finite-control-set model-predictive control for power converters. IEEE Trans Ind Inf 10: 991–1002. https://doi.org/10.1109/TII.2013.2284558 doi: 10.1109/TII.2013.2284558
![]() |
[37] |
Kouro S, Cortés P, Vargas R, et al. (2008) Model predictive control—A simple and powerful method to control power converters. IEEE Trans Ind Electron 56: 1826–1838. https://doi.org/10.1109/TIE.2008.2008349 doi: 10.1109/TIE.2008.2008349
![]() |
[38] | Rodriguez J, Cortes P (2012) Predictive control of power converters and electrical drives. John Wiley & Sons, Ltd. https://doi.org/10.1002/9781119941446 |
1. | Waleed M. Zapar, Khalaf Gaeid , Hazli Bin Mokhlis Mokhlis, Takialddin A. Al Smadi , Review of the Most Recent Articles in Fault Tolerant Control of Power Plants 2018 – 2022, 2023, 30, 2312-7589, 103, 10.25130/tjes.30.2.11 | |
2. | Mebarek LAHBIB, Mohammed BOUSSAID, Houcine MOUNGAR, Ahmed TAHRI, Analytical assessment of the filed results on the PV system connectors performance under operating temperature, 2023, 11, 2333-8334, 555, 10.3934/energy.2023029 |
Mode | S1 | S2 | S3 | Vc |
1 | 0 | 0 | 0 | 0 |
2 | 0 | 0 | 1 | 23Vdc∠0∘ |
3 | 0 | 1 | 1 | 23Vdc∠60∘ |
4 | 0 | 1 | 0 | 23Vdc∠120∘ |
5 | 1 | 1 | 0 | 23Vdc∠180∘ |
6 | 1 | 0 | 0 | 23Vdc∠240∘ |
7 | 1 | 0 | 1 | 23Vdc∠300∘ |
8 | 1 | 1 | 1 | 0 |
Parameter | Symbol | Value |
PV Rated Power | VPV | 5 kW |
DC-link Reference Voltage | VDC | 800 V |
Filter Inductance | L | 2.8 mH |
ESR of L | R | 0.02 Ω |
Utility Grid Voltage | Vg | 380 VLL,RMS |
Grid Frequency | fgrid | 50 Hz |
Sampling Time | Ts | 10 e−6 |
Proportional Gain | Kp | 1.6 |
Integral Gain | Ki | 20 |
Mode | S1 | S2 | S3 | Vc |
1 | 0 | 0 | 0 | 0 |
2 | 0 | 0 | 1 | 23Vdc∠0∘ |
3 | 0 | 1 | 1 | 23Vdc∠60∘ |
4 | 0 | 1 | 0 | 23Vdc∠120∘ |
5 | 1 | 1 | 0 | 23Vdc∠180∘ |
6 | 1 | 0 | 0 | 23Vdc∠240∘ |
7 | 1 | 0 | 1 | 23Vdc∠300∘ |
8 | 1 | 1 | 1 | 0 |
Parameter | Symbol | Value |
PV Rated Power | VPV | 5 kW |
DC-link Reference Voltage | VDC | 800 V |
Filter Inductance | L | 2.8 mH |
ESR of L | R | 0.02 Ω |
Utility Grid Voltage | Vg | 380 VLL,RMS |
Grid Frequency | fgrid | 50 Hz |
Sampling Time | Ts | 10 e−6 |
Proportional Gain | Kp | 1.6 |
Integral Gain | Ki | 20 |