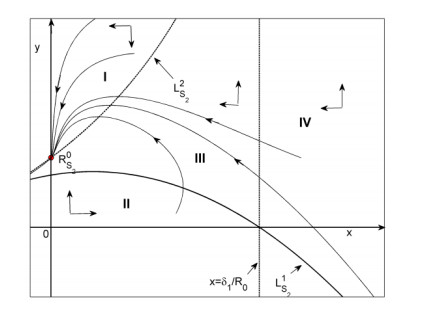
Proton exchange membrane fuel cell (PEMFC) is an alternate energy source that produces electricity without any adverse effects on the environment. The drawbacks of PEMFC are its short life and its non-linear voltage with loading current. Also, PEMFC is prone to ambient conditions, and its performance varies with different ambient conditions. In this work, the semi-empirical modeling approach has been used to predict the PEMFC voltage accurately. However, when the ambient condition varies, the voltage of PEMFC varies accordingly and consequently the previous parameters of the EMI-empirical model don't produce good results. Previously the voltage variation due to changes in ambient has been predicted with the help of ambient conditions and load resistance, but this model isn't sui for all PEMFCs. In this work, a new method has been proposed where fast and accurate optimization technique such as Transient search optimization (TSO) has been used to optimize parameters when ambient condition varies and predicts the PEMFC voltage accurately and doesn't consume a lot of time. The proposed method will be very helpful in future research for predicting the PEMFC voltage for various PEMFC systems at different ambient conditions. The proposed method has been validated experimentally by performing experiments on n single-cell PEMFC system at normal and high ambient temperature.
Citation: Amine Abbou, Abdennebi El Hassnaoui. A novel approach for predicting PEMFC in varying ambient conditions by using a transient search optimization algorithm based on a semi-empirical model[J]. AIMS Energy, 2022, 10(2): 254-272. doi: 10.3934/energy.2022014
[1] | Baolin Kang, Xiang Hou, Bing Liu . Threshold control strategy for a Filippov model with group defense of pests and a constant-rate release of natural enemies. Mathematical Biosciences and Engineering, 2023, 20(7): 12076-12092. doi: 10.3934/mbe.2023537 |
[2] | Yi Yang, Lirong Liu, Changcheng Xiang, Wenjie Qin . Switching dynamics analysis of forest-pest model describing effects of external periodic disturbance. Mathematical Biosciences and Engineering, 2020, 17(4): 4328-4347. doi: 10.3934/mbe.2020239 |
[3] | Yuan Tian, Sanyi Tang . Dynamics of a density-dependent predator-prey biological system with nonlinear impulsive control. Mathematical Biosciences and Engineering, 2021, 18(6): 7318-7343. doi: 10.3934/mbe.2021362 |
[4] | Bing Liu, Gang Hu, Baolin Kang, Xin Huang . Analysis of a hybrid pest management model incorporating pest resistance and different control strategies. Mathematical Biosciences and Engineering, 2020, 17(5): 4364-4383. doi: 10.3934/mbe.2020241 |
[5] | Liping Wu, Zhongyi Xiang . A study of integrated pest management models with instantaneous and non-instantaneous impulse effects. Mathematical Biosciences and Engineering, 2024, 21(2): 3063-3094. doi: 10.3934/mbe.2024136 |
[6] | Bruno Buonomo , Francesco Giannino , Stéphanie Saussure , Ezio Venturino . Effects of limited volatiles release by plants in tritrophic interactions. Mathematical Biosciences and Engineering, 2019, 16(5): 3331-3344. doi: 10.3934/mbe.2019166 |
[7] | Wenjie Qin, Yue Xia, Yi Yang . An eco-epidemic model for assessing the application of integrated pest management strategies. Mathematical Biosciences and Engineering, 2023, 20(9): 16506-16527. doi: 10.3934/mbe.2023736 |
[8] | Luis F. Gordillo . Optimal sterile insect release for area-wide integrated pest management in a density regulated pest population. Mathematical Biosciences and Engineering, 2014, 11(3): 511-521. doi: 10.3934/mbe.2014.11.511 |
[9] | Aili Wang, Yanni Xiao, Huaiping Zhu . Dynamics of a Filippov epidemic model with limited hospital beds. Mathematical Biosciences and Engineering, 2018, 15(3): 739-764. doi: 10.3934/mbe.2018033 |
[10] | Guodong Li, Wenjie Li, Ying Zhang, Yajuan Guan . Sliding dynamics and bifurcations of a human influenza system under logistic source and broken line control strategy. Mathematical Biosciences and Engineering, 2023, 20(4): 6800-6837. doi: 10.3934/mbe.2023293 |
Proton exchange membrane fuel cell (PEMFC) is an alternate energy source that produces electricity without any adverse effects on the environment. The drawbacks of PEMFC are its short life and its non-linear voltage with loading current. Also, PEMFC is prone to ambient conditions, and its performance varies with different ambient conditions. In this work, the semi-empirical modeling approach has been used to predict the PEMFC voltage accurately. However, when the ambient condition varies, the voltage of PEMFC varies accordingly and consequently the previous parameters of the EMI-empirical model don't produce good results. Previously the voltage variation due to changes in ambient has been predicted with the help of ambient conditions and load resistance, but this model isn't sui for all PEMFCs. In this work, a new method has been proposed where fast and accurate optimization technique such as Transient search optimization (TSO) has been used to optimize parameters when ambient condition varies and predicts the PEMFC voltage accurately and doesn't consume a lot of time. The proposed method will be very helpful in future research for predicting the PEMFC voltage for various PEMFC systems at different ambient conditions. The proposed method has been validated experimentally by performing experiments on n single-cell PEMFC system at normal and high ambient temperature.
Various modelling and analytical techniques have been widely employed to address the effectiveness of integrated pest management (IPM), which is a threshold control strategy [1,2,3] and it can be defined as follows: if the number of pests is below the economic threshold (ET), then the integrated control strategy is not applied at all; above the ET then the integrated control measures including biological control and chemical control are applied with the aim of maintaining the number of pests below the economic injury level (EIL). In order to reveal the effectiveness of IPM, various mathematical models have been developed and studied based on the action mechanism of pesticides and the implementation of control strategies [4,5,6,7,8,9,10]. In particular, Filippov systems which are widely employed to depict intermittent control strategies and to describe many practical problems [7,11,12,13,14,15,16,17,18], have been received much attention and investigated [7,16,17,18]. However, most of the previous models strategy were designed to simplify the dynamic behavior of two subsystems without considering the constant releasing rate commonly used in IPM [17,18]. In practical biological control methods, a constant releasing strategy is often used to facilitate operation and avoid monitoring the number of natural enemies, which has been proved to play an important role in the pest control [19,20,21,22].
In the present paper, a planar Filippov system formulated by two subsystems is proposed to reveal the effect of the constant releasing rate of natural enemy on the pest control, in which the discontinuity boundary or switching line is determined by the ET [23,24,25,26,27]. Therefore, the IPM strategy including spraying pesticide which the killing rate assumes to be proportional to the number of pests and releasing a constant number of natural enemies is applied once the number of the pest population exceeds the ET, otherwise the pest-natural enemy system is free from any control effects.
Based on the above facts, the pest-natural enemy interaction system without control measurements can be modelled by the classical Holling-Ⅱ predator-prey model [28,29] as follows:
{dx(t)dt=rx−rKx2−βxy1+ωx,dy(t)dt=ηβxy1+ωx−δy, | (1.1) |
where x(t) and y(t) represent the number of prey (pest) and predator (natural enemy), r is the intrinsic growth rate of the pest population and K represents its carrying capacity, βx1+ωx denotes the Holling-Ⅱ functional response function, which is a saturating function of the numbers of pests present, δ denotes the death rate of the natural enemy population, and η (η∈(0,1]) denotes the conversion rate of the pest. The dynamics of this subsystem (also called an untreated subsystem) is well known and has been well studied [28], and will be summarized in the coming section.
The other subsystem (also called a treated subsystem) must be employed to model the artificial control strategies (spraying pesticides and releasing natural enemies), which can be represented as follows:
{dx(t)dt=rx−rKx2−βxy1+ωx−αx,dy(t)dt=ηβxy1+ωx−δ1y+τ, | (1.2) |
where τ represents the releasing constant of natural enemies, α denotes the pest killing rate due to the spraying of pesticides, and δ1≥δ implies that the pesticide has side effects on the natural enemy. From a mathematical point of view, the essential difference between model (1.1) and model (1.2) is the constant release rate τ, which will result in rich dynamical behaviors and will be investigated in more detail in Section 3.
Combining the above two subsystems, we get the following non-smooth Filippov pest-natural enemy system with constant releasing rate as follows:
{dx(t)dt=rx−rKx2−βxy1+ωx−ϵαx,dy(t)dt=ηβxy1+ωx−(δ+ϵh)y+ϵτ | (1.3) |
with
{ϵ=0,x(t)<ET,ϵ=1,x(t)>ET, |
where for convenience we denote δ1=δ+h with h≥0. Note that if τ=0, then two subsystems (i.e., models (1.1) and (1.2) of Filippov system (1.3) have exactly the same dynamics, which have been widely used and investigated [24].
The objective of this paper is to systematically study the dynamical properties of non-smooth Filippov pest-natural enemy system with constant releasing rate and discuss bifurcations of model (1.3) depending on important parameters. This paper is organized as follows. In Section 2, we introduce the main definitions and terminologies of the generic second-order Filippov system. In Section 3, we systematically study the dynamical behavior of system (1.2) (i.e., subsystem S2) and address how the constant releasing strategy affects the qualitative behaviors, which is crucial for analyzing Filippov system (1.3). The results show that system (1.2) exists rich dynamical behaviors including transcritical, saddle-node, Hopf and Bogdanov-Takens bifurcations [30,31]. In Section 4, we study the existence and stability of the regular/virtual equilibria, pseudo-equilibria, sliding segments and sliding bifurcations of system (1.3) by employing the methods for non-smooth dynamical systems including the Filippov convex method and sliding bifurcation techniques [23,26,27,32]. Particular attention is paid to the effect of the threshold level ET and releasing constant τ on the dynamical behavior of Filippov system (1.3) and successful pest control. The paper ends with brief conclusions and discussions.
The generic second-order (i.e., X(t)∈R2+) Filippov system can be defined as follows [12,17,23]:
˙X(t)={FS1(X),X∈S1,FS2(X),X∈S2, | (2.1) |
where X(t)={x(t),y(t)}T and R2+={(x,y)|x≥0,y≥0}, and the regions S1 and S2 are separated by the discontinuity boundary Σ described by H(X)=0, where H(X) is a smooth scalar function with non-vanishing gradient HX(X) on Σ. For convenience, we call Filippov system (2.1) defined in region S1 as subsystem S1 and defined in region S2 as subsystem S2. Denote
σ(X)=⟨HX(X),FS1(X)⟩⋅⟨HX(X),FS2(X)⟩, |
where ⟨,⟩ is a Cartesian product in R2 and HX(X) pointing to the region S2 is called the positive direction. Based on the above concepts, we provide the necessary definitions of Filippov system (2.1) as follows:
Definition 1. If all these points (x,y)∈Σ satisfy the condition σ(X)≤0, then we say that (x,y) belongs to the sliding segment, denoted by Σs. While the complement to Σs in Σ is called a crossing set, denoted by Σc. For the stability of the sliding segment, if
⟨HX(X),FS1(X)⟩>0,⟨HX(X),FS2(X)⟩<0, |
then the sliding segment is stable. If
⟨HX(X),FS1(X)⟩<0,⟨HX(X),FS2(X)⟩>0, |
then it is unstable.
Definition 2. If the point R(x∗,y∗) of Filippov system (2.1) satisfies the following conditions
FS1(x∗,y∗)=0 for (x∗,y∗)∈S1orFS2(x∗,y∗)=0 for (x∗,y∗)∈S2, |
then it is called a regular equilibrium of Filippov system (2.1). Similarly, if it satisfies
FS1(x∗,y∗)=0 for (x∗,y∗)∈S2orFS2(x∗,y∗)=0 for (x∗,y∗)∈S1, |
then it is called a virtual equilibrium of Filippov system (2.1).
Definition 3. If the point T(x∗,y∗) of Filippov system (2.1) satisfies
⟨HX(x∗,y∗),FS1(x∗,y∗)⟩=0 for (x∗,y∗)∈Σsor⟨HX(x∗,y∗),FS2(x∗,y∗)⟩=0 for (x∗,y∗)∈Σs, |
then it is called a tangent point of Filippov system (2.1).
Definition 4. If the point P(x∗,y∗) of Filippov system (2.1) satisfies
FS1(x∗,y∗)=0 for H(x∗,y∗)=0orFS2(x∗,y∗)=0 for H(x∗,y∗)=0, |
then it is called a boundary equilibrium of Filippov system (2.1).
Note that the three points P(x∗,y∗), T(x∗,y∗) and R(x∗,y∗) may collide together for some critical cases, and for more definitions and properties of Filippov system (2.1) please see [12,17,23]. Now let us turn to non-smooth Filippov system (1.3), which can be represented as:
˙X(t)={f1(x,y),(x,y)∈S1,f2(x,y),(x,y)∈S2, | (2.2) |
where the discontinuity boundary is Σ={(x,y)∈R2+|H(x,y)=0} and H(x,y)=x−ET, which separates the two regions S1={(x,y)∈R2+|H(x,y)<0} and S2={(x,y)∈R2+|H(x,y)>0}. The vector field for subsystem S1 is
f1(x,y)={rx−rKx2−βxy1+ωx,ηβxy1+ωx−δy}T |
and the vector field for subsystem S2 is
f2(x,y)={rx−rKx2−βxy1+ωx−αx,ηβxy1+ωx−δ1y+τ}T. |
The dynamics of two subsystems S1 and S2 are crucial for analyzing the global dynamics of Filippov system (2.2), and the complete dynamical behavior of subsystem S1 has been discussed in more detail in the literature [28]. That is to say, subsystem S1 always exists with a trivial equilibrium R0S1(0,0) which is unstable, and a boundary equilibrium R1S1(K,0) which is globally stable if δηβ−δω≥K or δηβ−δω<0holds true. Meanwhile, subsystem S1 exists with a positive equilibrium R2S1(δηβ−δω,rη(Kηβ−Kδω−δ)K(ηβ−δω)2) if and only if 0<δηβ−δω<K, which is a globally stable node or focus provided that ωK≤ηβ+δωηβ−δω. Otherwise, it is an unstable node or focus and there exists a unique and stable limit cycle.
However, the dynamical behavior of subsystem S2 has not been addressed so far because the constant releasing rate will bring some challenges for qualitative analysis. Although a similar model was investigated in reference [31], we realize that the sign of the constant τ (minus τ was used in [31]) could result in different dynamics including the existence and stability of equilibria and various bifurcations. Thus, we first carry out qualitative analyses for subsystem S2 in the following.
In this section, we will investigate the dynamical behavior of subsystem S2 and focus on the effect of parameter τ on the dynamics, i.e., the effect of the control strategy (releasing natural enemies) in the pest control. For convenience, we rewrite the subsystem S2 as follows:
{dx(t)dt=rx−rKx2−βxy1+ωx−αx≐P1(x,y),dy(t)dt=ηβxy1+ωx−δ1y+τ≐Q1(x,y), | (3.1) |
here we assume that r>α.
Firstly, let us begin to determine the location and number of the equilibria of system (3.1) in R2+. It is easy to check that the point R0S2(0,τδ1) is always the boundary equilibrium of system (3.1). In the following, we mainly focus on the existence of the positive equilibria of system (3.1).
It is clear that equations
{P1(x,y)=0,Q1(x,y)=0, | (3.2) |
have at most two positive real solutions:
x1≐KR2−K√Δ2rR0, y1≐(r1−rKx1)1+ωx1β |
and
x2≐KR2+K√Δ2rR0, y2≐(r1−rKx2)1+ωx2β, |
where
r1≐r−α, R0≐ηβ−δ1ω, R1≐βτ−r1δ1, R2≐r1(ηβ−δ1ω)+rδ1K and Δ≐R22+4rR0R1K. |
That is to say, system (3.1) has at most two positive equilibria in R2+, denoted by R1S2(x1,y1) and R2S2(x2,y2). And we have the following simple theorem which describes the number and location of equilibria of system (3.1). The proof is omitted.
Lemma 3.1. For system (3.1), with R0, R1, R2 and Δ defined as above, we have:
(ⅰ) when R0>0, system (3.1) has at most two equilibria in R2+ and it has the following possibilities:
(a) if R1>0 holds, then system (3.1) has a unique equilibrium R0S2;
(b) if R1<0 holds, then system (3.1) has two equilibria R0S2 and R1S2;
(c) if R1=0 holds, then R0S2 and R1S2 coalesce at a boundary equilibrium of multiplicity 2.
(ⅱ) when R0<0, system (3.1) has at most three equilibria in R2+ and it has the following possibilities:
(a) if R1>0, R2<0 and Δ>0 hold, then system (3.1) has three equilibria R0S2, R1S2 and R2S2;
(b) if R1>0, R2<0 and Δ=0 hold, then R1S2 and R2S2 coalesce at a positive equilibrium of multiplicity 2, which coexists with R0S2;
(c) if R1>0, R2<0 and Δ<0 hold, then system (3.1) has a unique equilibrium R0S2;
(d) if R1<0 holds, then system (3.1) has two equilibria R0S2 and R1S2;
(e) if R1=0 and R2<0 hold, then R0S2 and R2S2 coalesce at a boundary equilibrium of multiplicity 2, which coexists with R1S2;
(f) if R1=0 and R2>0 hold, then R0S2 and R1S2 coalesce at a boundary equilibrium of multiplicity 2, and R2S2 disappears in this case;
(g) if R1=0 and R2=0 hold, then R0S2, R1S2 and R2S2 coalesce at a boundary equilibrium of multiplicity 3.
Next we discuss the possible phase portraits of system (3.1) and analyze the stability of equilibria according to Lemma 3.1.
In this case, system (3.1) has at most two equilibria R1S2(x1,y1) and R0S2(0,τδ1) in R2+, and we can get the following results.
Theorem 3.1. If R0>0 and R1>0, then system (3.1) exists with a boundary equilibrium R0S2 in R2+, which is a globally asymptotically stable node.
Proof. In this case, system (3.1) only has a boundary equilibrium R0S2(0,τδ1) in R2+. And the Jacobian matrix at R0S2 is given by
AR0S2=(r1−2rxK−βy(1+ωx)2−βx1+ωxηβy(1+ωx)2ηβx1+ωx−δ1)|R0S2=(r1−βτδ10ηβτδ1−δ1). |
The corresponding characteristic equation is
|AR0S2−λE|=λ2+pR0S2λ+qR0S2=0, |
where qR0S2≐βτ−r1δ1=R1>0,pR0S2≐δ1+βτδ1−r1>0 and p2R0S2−4qR0S2=(δ1−βτδ1+r1)2>0. This means that R0S2 is a locally asymptotically stable node. Especially, when τ=0, we have qR0S2=−r1δ1<0 which indicates that R0S2 is an unstable saddle.
Now we begin to discuss the global stability of R0S2. In the first quadrant, the two non-zero isoclines L1S2 and L2S2 of system (3.1) divide R2+ into four regions:
I={(x,y)|P1(x,y)<0,Q1(x,y)<0}; II={(x,y)|P1(x,y)>0,Q1(x,y)>0}; |
III={(x,y)|P1(x,y)<0,Q1(x,y)>0,x≤δ1R0}; |
IV={(x,y)|P1(x,y)<0,Q1(x,y)>0,x>δ1R0}, |
at which the signs of two functions P1(x,y) and Q1(x,y) are clear.
It is known that system (3.1) does not exist with any other equilibria in R2+ except R0S2. From Figure 1, we can see that the trajectory {x(t,x0,y0),y(t,x0,y0)} of system (3.1) will approach the boundary equilibrium R0S2 directly, or intersects with the non-zero isoclines L1S2 or L2S2 firstly, and then tends to the boundary equilibrium R0S2 as t→+∞ for any initial value (x0,y0)∈I⋃II⋃III.
By a simple calculation, we have
|dydx|=|Q1(x,y)P1(x,y)|=|ηβ−δ1ωβ|⋅|1−δ1ηβ−δ1ωx+δ1ηβ−δ1ω(τβ+τωβx)xy1+rωβKx2−r1ω−rKβ−r1βxy|<R0β |
for all (x,y)∈IV and y≫1. That is to say, the trajectories of system (3.1) can not always remain to the right of the line x=δ1R0, which indicates that the trajectories starting from the region IV must cross the line x=δ1R0 and enter into the region III, and then approach the boundary equilibrium R0S2 as t→+∞. Thus, the boundary equilibrium R0S2 is a globally stable node. This completes the proof.
Theorem 3.2. If R0>0 and R1=0, then system (3.1) does not exist with a positive equilibrium, and the boundary equilibrium R0S2 is a saddle-node of codimension 1.
Proof. Note that if R1=0, then the boundary equilibrium R0S2 collides with the positive equilibrium R1S2 in R2+, which is a high order singular point. Translating the boundary equilibrium R0S2(0,τδ1) to the origin by making the change of variables u=x and v=y−τδ1, renaming (u,v) as (x,y) and expanding the right-hand side of system (3.1) in a Taylor series about the origin, then we can obtain
{dxdt=(r1ω−rK)x2−βxy−r1ω2x3+βωx2y+M1(x,y),dydt=r1ηx−δ1y−r1ηωx2+ηβxy+r1ηω2x3−ηβωx2y+M2(x,y), | (3.3) |
where M1(x,y) and M2(x,y) are C∞ functions in (x,y) at least of the fourth order.
Notice that δ1>0, making the following change of variables
u=x, v=r1ηx−δ1y |
and renaming (u,v) as (x,y), then system (3.3) becomes
{dxdt=−R2δ1x2+βδ1xy+r1ωR0δ1x3−βωδ1x2y+M3(x,y)≐P2(x,y),dydt=−δ1y−r1ηδ1(R2+δ1R0)x2+(1+r1δ1)ηβxy+r1ηωR0(1+r1δ1)x3−ηβω(1+r1δ1)x2y+M4(x,y)≐Q2(x,y)−δ1y, | (3.4) |
where R2>0, and M3(x,y), M4(x,y) are C∞ functions in (x,y) at least of the fourth order. Solving the equation −δ1y+Q2(x,y)=0, we can obtain the implicit solution
ϕ(x)≐−r1ηδ21(R2+δ1R0)x2+O(x3). | (3.5) |
where R2+δ1R0>0. And substituting ϕ(x) into P2(x,y), which can be written in the form
ψ(x)≐P2(x,ϕ(x))=−R2δ1x2+O(x3). | (3.6) |
According to Theorems 7.1–7.3 in [30], it is easy to obtain that R0S2 is a saddle-node of codimension 1 if R0>0 and R1=0. The proof is completed.
In the following, we discuss the stability of the positive equilibria of system (3.1). For convenience, we first denote
R3≐K(ηβ−δ1ω−rK+r1ω)x1Kδ1+2rωx21 and R4≐(ηβ−δ1ω−rK+r1ω)√2rδ1ωK4rδ1ω. |
Moreover, we have the following main results.
Theorem 3.3. If R0>0 and R1<0, then system (3.1) has an unstable boundary equilibrium R0S2 and a positive equilibrium R1S2 in R2+. Further, if R3<1, then R1S2 is a locally stable node (or a focus), and it is globally stable provided that R4<1.
Proof. For the boundary equilibrium R0S2(0,τδ1), we have
|AR0S2−λE|=λ2+pR0S2λ+qR0S2=0 |
with qR0S2=βτ−r1δ1=R1<0, which indicates that R0S2 is an unstable saddle.
For the positive equilibrium R1S2(x1,y1), the characteristic equation is as follows:
|AR1S2−λE|=λ2+pR1S2λ+qR1S2=0, | (3.7) |
where
pR1S2=11+ωx1[2rωKx21−(ηβ−δ1ω−rK+r1ω)x1+δ1] | (3.8) |
and
qR1S2=2rR0x1K(1+ωx1)[KR22rR0−x1]. | (3.9) |
Obviously, qR1S2>0. It follows from (3.8) that if
ηβ−δ1ω−rK+r1ω<Kδ1+2rωx21Kx1 |
holds true, which is equivalent to the following inequality
K(ηβ−δ1ω−rK+r1ω)x1Kδ1+2rωx21<1, i.e., R3<1, |
then pR1S2>0 and consequently R1S2 is a locally asymptotically stable node (or focus).
In order to show the global stability of R1S2, for simplifying the calculations, we consider a polynomial system, which has the same equilibria R0S2, R1S2, R2S2 and dynamics as system (3.1) for x>−1ω. To do this, multiplying both sides of system (3.1) by the function 1+ωxβ and introducing a new time variable τ by dt=1+ωxβdτ yield the following polynomial system:
{dx(t)dτ=x(a1+a2x−a3x2−y)≐P2(x,y),dy(t)dτ=b1xy−b2y+b3+b4x≐Q2(x,y), | (3.10) |
where a1=r1β,a2=r1ω−rKβ,a3=rωβK,b1=ηβ−δ1ωβ,b2=δ1β,b3=τβ,b4=τωβ, and a1,a3,b1,b2,b3,b4 are all positive constants, the signs of a2 could vary.
Let B(x,y)=x−1 be the Dulac function [30], by a simple calculation, we can obtain that
∂BP2(x,y)∂x=∂(a1+a2x−a3x2−y)∂x=a2−2a3x, |
∂BQ2(x,y)∂y=∂(b1y−b2x−1y+b3x−1+b4)∂y=b1−b2x−1, |
D≐∂BP2∂x+∂BQ2∂y=−x−1[2a3x2−(a2+b1)x+b2]≐−x−1g(x). | (3.11) |
It follows from (3.11) that if
Δ2≐(a2+b1)2−8a3b2=(ηβ−δ1ω−rK+r1ωβ)2−8rδ1ωβ2K<0, |
i.e.,
−2√2rδ1ωK<ηβ−δ1ω−rK+r1ω<2√2rδ1ωK, |
then the function g(x)=2a3x2−(a2+b1)x+b2>0 for all x∈R. That is to say, D<0 for all (x,y)∈R2+. Note that for the function g(x), it is easy to see that when a2+b1<0, i.e., ηβ−δ1ω−rK+r1ω<0, we have g(x)>0 for all x≥0 and consequently D<0 for all (x,y)∈R2+.
Therefore, according to the above analyses, we can conclude that if
ηβ−δ1ω−rK+r1ω<2√2rδ1ωK, |
which is equivalent to the inequality
(ηβ−δ1ω−rK+r1ω)√2rδ1ωK4rδ1ω<1, i.e., R4<1, |
then D<0, and consequently system (3.10) does not exist with any periodic solutions lying in the interior of R2+, which indicates that R1S2 is globally stable, as shown in Figure 2A. This completes the proof.
Remark It is easy to see that the condition R4<1 is stronger than the inequality R3<1, which reveals that the local stability of the equilibrium R1S2 does not indicate the global stability. However, extensive numerical investigations show that R1S2 is a globally stale equilibrium provided that R3<1, but unfortunately we have no way to prove it at present.
Theorem 3.4. If R3>1 holds true, then system (3.10) has at least one limit cycle in the interior of R2+.
Proof. Based on the analyses in Theorem 3.3, we know that if R3>1, then the positive equilibrium R1S2 is an unstable focus (or node). To show the existence of a limit cycle, we define the following four lines:
L1:x−r1Kr=0; L2:x=0; L3:y=0 and L4:x+1b1y+P=0, |
which form a closed region G, where P is a constant and the positive equilibrium R1S2 belongs to G. For all x∈[0,r1Kr] and y>0, we have
dL1dt|x=r1Kr=−r1Kry<0, |
which indicates that if the trajectories of system (3.10) intersect with the line L1, it will pass from the right side of the line L1 to the left, entering into the region G. Moreover, it follows from
dL3dt|y=0=b3+b4x>0 |
that if the trajectories of system (3.10) intersect with the line L3, it will pass from the below of the line L3 to the top, entering into the region G. Further, by a simple calculation, we have
dL4dt|y=−b1(x+P)=x(a1+a2x−a3x2+b4b1)+(1+b2)P+b3b1. |
Thus, if we choose the constant P such that P<−max{11+b2[x(a1+a2x−a3x2+b4b1)+b3b1]}, then dL4dt|y=−b1(x+P)<0. That is to say, if the trajectories of system (3.10) intersect with the line L4, it will pass from the top of the line L4 to below it, entering into the region G.
Note that L2 is one of the trajectories of system (3.10), which approaches the boundary equilibrium R0S2 and the trajectories initiating from region G will remain in it, i.e., L1, L2, L3 and L4 form a Bendixson curve. Thus, according to the Poincaré-Bendixson Theorem [30], system (3.10) has at least one limit cycle around the positive equilibrium R1S2, as shown in Figure 2B. This completes the proof.
Unfortunately, we can not employ the main results shown in literature [30] to address the uniqueness of the limit cycle. Therefore, in the following, we further consider the uniqueness and stability of the limit cycle through bifurcation analyses in subsection 3.2.
In this case, system (3.1) has at most three equilibria in R2+ including two positive equilibria R1S2(x1,y1) and R2S2(x2,y2), and a boundary equilibrium R0S2(0,τδ1) which is a stable node if R1>0 and it is an unstable saddle if R1<0. Based on the characteristic equation (3.7), for R1S2 and R2S2, we have
pR1S2=11+ωx1[2rwKx21−(ηβ−δ1ω−rK+r1ω)x1+δ1],qR1S2=x1√Δ1+ωx1 |
and
pR1S2=11+ωx2[2rwKx22−(ηβ−δ1ω−rK+r1ω)x2+δ1],qR1S2=−x2√Δ1+ωx2. |
Obviously, qR1S2>0 and qR2S2<0. That is to say, R2S2 is an unstable saddle and R1S2 is an elementary and not saddle-type equilibrium. Further, we have the following results:
Theorem 3.5. If R0<0, R1>0, R2<0 and Δ>0, then system (3.1) exists with three equilibria: R0S2, R1S2 and R2S2. Further, R0S2 is a locally stable node, R2S2 is an unstable saddle and R1S2 is a node (or a focus) which is locally stable provided R3<1.
Theorem 3.6. If R0<0, R1>0, R2<0 and Δ=0, then system (3.1) exists with a locally stable boundary equilibrium R0S2. Moreover, R1S2 collides with R2S2, which is a saddle-node of codimension 1 when R3≠1.
Theorem 3.7. If R0<0, R1>0, R2<0 and Δ<0, then system (3.1) exists with a boundary equilibrium R0S2, which is a globally asymptotically stable node.
Theorem 3.8. If R0<0 and R1<0, then system (3.1) has an unstable boundary equilibrium R0S2 and a positive equilibrium R1S2. Further, if R3<1, then R1S2 is a locally stable node (or a focus), and it is globally stable provided that R4<1.
Theorem 3.9. If R0<0 and R1=0, then the boundary equilibrium R0S2 is a high order singular point. More precisely,
(ⅰ) R0S2 is a saddle-node of codimension 1 if R2≠0;
(ⅱ) R0S2 is an unstable saddle of codimension 2 if R2=0.
Proof. The proof of the conclusion (ⅰ) is similar to that for Theorem 2, we discuss the conclusion (ⅱ) in the following.
For system (3.4), when R2=0, then it can be represented as
{dxdt=βδ1xy+r1ωR0δ1x3−βωδ1x2y+M3(x,y)≐P2(x,y),dydt=−δ1y−r1ηR0x2+(1+r1δ1)ηβxy+r1ηωR0(1+r1δ1)x3−ηβω(1+r1δ1)x2y+M4(x,y)≐Q2(x,y)−δ1y, | (3.12) |
Solving the equation −δ1y+Q2(x,y)=0, we obtain the implicit solution
ϕ(x)≐−r1ηR0δ1x2+O(x3). | (3.13) |
Then substituting ϕ(x) into P2(x,y), which can be written in the form
ψ(x)≐P2(x,ϕ(x))=−r1R20δ21x3+O(x4). | (3.14) |
According to Theorems 7.1–7.3 in [30], it is easy to obtain that R0S2 is an unstable saddle of codimension 2 if R0<0, R1=0 and R2=0. The proof is completed.
In this section, we will discuss various possible bifurcations of system (3.1) including transcritical, saddle-node, Hopf and Bogdanov-Takens bifurcations.
It follows from Lemma 3.1 and Theorems 3.1–3.3, that the positive equilibrium R1S2 collides with the boundary equilibrium R0S2 when R1=0, and solving R1=0 with respect to τ, we have τ∗0=r1δ1β. Moreover, if τ<τ∗0, then system (3.1) has two equilibria: R1S2 which is a stable node (or focus) provided that R3<1, and R0S2 which is an unstable saddle. If τ>τ∗0, then R1S2 becomes negative which is unstable, and R0S2 becomes a stable node, which indicates that the transcritical bifurcation occur at τ∗0, as shown in Figure 3. Further, we can obtain that
TB={(r,α,β,η,ω,τ,δ1)| R0>0,τ=τ∗0} |
is a transcritical bifurcation surface. This indicates that there exists a critical releasing rate τ∗0 such that the pests and natural enemies coexist in the form of a positive equilibrium or a periodic orbit with a finite period when the releasing rate τ<τ∗0, and the pest population goes extinct when the releasing rate τ>τ∗0.
From Lemma 3.1 and Theorems 3.5–3.7, we can obtain that
SN={(r,α,β,η,ω,τ,δ1,K)| R0<0,R1>0,R2<0 and Δ=0} |
is a saddle-node bifurcation surface. When the parameters pass from the one side of the surface to the other, the number of positive equilibria of system (3.1) changes from zero to two, and the two positive equilibria are saddle and node, as shown in Figure 4. Especially, if we choose τ as bifurcation parameter and solving Δ=0 with respect to τ, we have τ∗1=K[rδ1K−r1R0]24βrR0 and the above saddle-node bifurcation surface can be represented as
SN={(r,α,β,η,ω,τ,δ1,K)| R0<0,R1>0,R2<0 and τ=τ∗1}. |
The saddle-node bifurcation reveals that if the releasing rate τ<τ∗1, then there exist some parameter spaces such that the pests and natural enemies may coexist in the form of a positive equilibrium or a periodic orbit with a finite period for different initial values, and if the releasing rate τ>τ∗1, then the pest population will be driven to extinction, as shown in Figure 4B, C.
For the characteristic equation related to the positive equilibrium R1S2(x1,y1) of system (3.1), we have
λ2+pR1S2λ+qR1S2=0, | (3.15) |
where
qR1S2=2rR0x1K(1+ωx1)[KR22rR0−x1]>0. | (3.16) |
and
pR1S2=11+ωx1[2rωKx21−(ηβ−δ1ω−rK+r1ω)x1+δ1]. | (3.17) |
Obviously, when pR1S2=0, solving the characteristic equation (3.15), we can get two complex eigenvalues λ1,2=±ib0 with b0=√qR1S2. That is to say, the positive equilibrium R1S2 is a center-type equilibrium of the linear system of system (3.1), which indicates that system (3.1) may undergo a Hopf bifurcation if the bifurcation parameters are chosen suitably.
We choose the releasing constant τ as the bifurcation parameter and fix all other parameters. To determine the critical bifurcation value τ∗, we consider the equation pR1S2(τ∗)=0, i.e.,
m2(τ∗)2+m1τ∗+m0=0, | (3.18) |
where m2=16r2β2a24R20K2, m1=4rβR0(2a4a6−a25−2r1R0+2rδ1K)K, m0=a24[rδ1K−r1R0]4+(2a4a6−a25)[rδ1K−r1R0]2, a4=Kω2rβR20, a5=K2rR0(r1ω−rK+ηβ−δ1ωβ−r1Kr−δ1R0) and a6=rω2βK(r1Kr+δ1R0)2+[δ1β−r1ω−rK+ηβ−δ1ωβ(r1K2r+δ12R0)]. Denote Δ3≐m21−4m0m2, then for the existence of critical value τ∗ we have the following results:
Lemma 3.2. The existence of parameter τ∗ can be described as follows:
(ⅰ) if Δ3<0, then τ∗ does not exist.
(ⅱ) if Δ3=0 and m1<0, then there exists a unique τ∗.
(ⅲ) if Δ3>0, m0=0 and m1<0, then there exists a unique τ∗.
(Ⅴ) if Δ3>0, m0<0, then there exists a unique τ∗.
(Ⅳ) if Δ3>0, m0>0 and m1<0, then there exist two critical values of τ∗.
Based on Lemma 3.2, we assume that there exists at least a critical value τ∗ such that pR1S2(τ∗)=0, and then address the Hopf bifurcation, i.e., we have the following main results:
Theorem 3.10. For system (3.1), assume that there exists a critical value τ∗>0 such that pR1S2(τ∗)=0, then system (3.1) undergoes a Hopf bifurcation and there is a unique limit cycle in the neighborhood of R1S2 provided R4>1.
Proof. Let λ=a(τ)+ib(τ) be a complex root of the characteristic equation (3.15), where a(τ∗)=0 and b(τ∗)=b0. Substituting it into the characteristic equation (3.15), it becomes
[a(τ)+ib(τ)]2+pR1S2[a(τ)+ib(τ)]+qR1S2=0, |
which can be represented as
{a(τ)2−b(τ)2+pR1S2(τ)a(τ)+qR1S2(τ)=0,2a(τ)b(τ)+pR1S2(τ)b(τ)=0. | (3.19) |
Taking the partial derivative of (3.19) with respect to τ, we can obtain
{2[a(τ)a′(τ)−b(τ)b′(τ)]+pR1S2(τ)a′(τ)+p′R1S2(τ)a(τ)+q′R1S2(τ)=0,2[a′(τ)b(τ)+a(τ)b′(τ)]+p′R1S2(τ)b(τ)+pR1S2(τ)b′(τ)=0. |
It follows from a(τ∗)=0, pR1S2(τ∗)=0 and b(τ∗)=b0 that we have
a′(τ)|τ=τ∗=−12p′R1S2(τ)|τ=τ∗, | (3.20) |
which is called the transversality condition [33,34].
If a′(τ)|τ=τ∗≠0, then the transversality condition (3.20) is satisfied, which indicates that the Hopf bifurcation takes place and there exists a unique limit cycle in the neighborhood of the positive equilibrium R1S2. By a simple calculation, we can obtain that
a′(τ)|τ=τ∗=−12(1+ωx1)[4rwKx1−(ηβ−δ1ω−rK+r1ω)]dx1dτ|τ=τ∗=−2rωK(1+ωx1)[(x1−K(ηβ−δ1ω−rK+r1ω)4rω]dx1dτ|τ=τ∗, | (3.21) |
where dx1dτ|τ=τ∗=−β√R22+4rR0R1K<0.
It follows from pR1S2=11+ωx1[2rωKx21−(ηβ−δ1ω−rK+r1ω)x1+δ1] that x1 is a positive real root of the following equation:
2rωKx21−(ηβ−δ1ω−rK+r1ω)x1+δ1=0 | (3.22) |
at τ=τ∗, which indicates that
Δ4≐(ηβ−δ1ω−rK+r1ω)2−8rωδ1K≥0 and ηβ−δ1ω−rK+r1ω>0 |
in the above equation. Solving equation (3.22) yields two roots, denoted by
x11=K(ηβ−δ1ω−rK+r1ω)4rω+K√Δ44rω and x12=K(ηβ−δ1ω−rK+r1ω)4rω−K√Δ44rω. |
If x1=x11, then we have
a′(τ)|τ=τ∗=−K√Δ44rωdx1dτ|τ=τ∗. | (3.23) |
If x1=x12, then we have
a′(τ)|τ=τ∗=K√Δ44rωdx1dτ|τ=τ∗. | (3.24) |
Therefore, it follows from (3.23) and (3.24) that if Δ4>0, which is equivalent to the inequality
(ηβ−δ1ω−rK+r1ω)√2rδ1ωK4rδ1ω>1, i.e., R4>1, |
then a′(τ)|τ=τ∗≠0, and consequently the Hopf bifurcation takes place at τ=τ∗.
To determine the stability of the limit cycle and the direction of the Hopf bifurcation in this case, we need to compute the first Liapunov coefficient l1(τ∗) [34,35] related to the positive equilibrium R1S2. To do this, we translate the origin of the coordinates to the positive equilibrium R1S2 of system (3.1) by the change of variables
ˉx=x−x1,ˉy=y−y1, |
rewrite (ˉx,ˉy) as (x, y) and expand the right-hand side of system (3.1) in a Taylor series about the origin, then we can obtain
{dxdt=ax+by+a20x2+2a11xy+a30x3+a21x2y+R1(x,y),dydτ=cx+dy+b20x2+2b11xy+b30x3+b21x2y+R2(x,y), | (3.25) |
where a≐r1−2rKx1−βy1(1+ωx1)2, b≐−βx11+ωx1, c≐ηβy1(1+ωx1)2, d≐−δ1+ηβx11+ωx1, a20≐−rK+βωy1(1+ωx1)3, a11≐−β2(1+ωx1)2, b20≐−ηβωy1(1+ωx1)3, b11≐ηβ2(1+ωx1)2, a30≐−βω2y1(1+ωx1)4, a21≐βω(1+ωx1)3, b30≐ηβω2y1(1+ωx1)4, b21≐−ηβω(1+ωx1)3, and R1(x,y) and R2(x,y) are C∞ functions in (x,y) at least of the fourth order.
Therefore, by employing the formula of the first Liapunov number l1(τ∗) at the origin of (3.25) in [34,35], we have
l1(τ∗)=−rωx21Kβb30[(r1δ1β2−τβ)+rω(ηβ−δ1ω)β2Kx31+βK2rω(δ1β−ηβ−δ1ωβx1)(δ1(ηβ−δ1ω)β2x1+rδ1ωβ2K)]=−rωx21Kβ3b30[−R1+rωR0Kx31+Kδ1R02rω(δ1R0−x1)(R0x1+rωK)]. |
Moreover, if l1(τ∗)<0, then system (3.1) undergoes a supercritical Hopf bifurcation at τ=τ∗ and there is a unique and stable limit cycle [35]. If l1(τ∗)>0, then system (3.1) undergoes a subcritical Hopf bifurcation at τ=τ∗ and there is a unique and unstable limit cycle [35]. This completes the proof.
To confirm the main results obtained here, we choose τ as the bifurcation parameter and fix the others as those shown in Figure 5. Bifurcation diagrams shown in Figure 5A, B indicate there exist Hopf bifurcation points τ∗i (i=2,3,4) marked as HB. Moreover, by a simple calculation, we have τ∗4=0.27, pR1S2(τ∗4)=0, a′R1S2(τ)|τ=τ∗4≠0 and l1(τ∗4)<0, i.e., system (3.1) undergoes a supercritical Hopf bifurcation at τ∗4 and it has a stable limit cycle in the neighborhood of R1S2, as shown in Figure 5B, C.
From Lemma 3.1 and Theorem 3.6, we can obtain that if R0<0, R1>0, R2<0 and Δ=0, then R1S2 collides with R2S2 as shown in Figure 4-A, and for convenience we still denote it by R1S2. According to the characteristic equation (3.7), by a simple calculation, we have
{pR1S2=11+ωx1[2rwKx21−(ηβ−δ1ω−rK+r1ω)x1+δ1],qR1S2=2rR0x1K(1+ωx1)[KR22rR0−x1] | (3.26) |
with x1=r1K2r+δ12(ηβ−δ1ω)=KR22rR0. Note that we have qR1S2=0 in this case. And if pR1S2=0 (i.e., R3=1), then the characteristic equation (3.7) related to the positive equilibrium R1S2 has two zero eigenvalues. This suggests that system (3.1) may admit a Bogdanov-Takens bifurcation [30,31,34] under a small parameter perturbation if the bifurcation parameters are chosen suitably and we confirm this by giving the following theorem.
Theorem 3.11. Suppose pR1S2=0 and qR1S2=0, then the positive equilibrium R1S2(x1,y1) of system (3.1) is a cusp of codimension 2 if ef≠0, where e=2b11+2a20−2aa11b and f=aa20−2a2a11b+bb20−2ab11.
Proof. Assume that pR1S2=0 and qR1S2=0, translating the positive equilibrium R1S2(x1,y1) to the origin by the change of variables u=x−x1 and v=y−y1, renaming (u,v) as (x,y) and expanding the right-hand side of system (3.1) in a Taylor series about the origin, then we can obtain
{dxdt=ax+by+a20x2+2a11xy+R3(x,y),dydt=cx+dy+b20x2+2b11xy+R4(x,y), | (3.27) |
where R3(x,y) and R4(x,y) are C∞ functions in (x,y) at least of the third order.
Notice that b≠0, making the following change of variables
u=x,v=ax+by |
and renaming (u,v) as (x,y), then system (3.27) becomes
{dxdt=y+(a20−2aa11b)x2+2a11bxy+R5(x,y),dydt=(aa20−2a2a11b+bb20−2ab11)x2+(2aa11b+2b11)xy+R6(x,y), | (3.28) |
where R5(x,y) and R6(x,y) are C∞ functions in (x,y) at least of the third order. Let
u=x−a11bx2,v=y+(a20−2aa11b)x2 |
and rename (u,v) as (x,y), then system (3.28) can be written as
{dxdt=y+R7(x,y),dydt=(aa20−2a2a11b+bb20−2ab11)x2+(2b11+2a20−2aa11b)xy+R8(x,y), | (3.29) |
which can be represented as
{dxdt=y+R7(x,y),dydt=exy+fx2+R8(x,y), |
where e=2b11+2a20−2aa11b and f=aa20−2a2a11b+bb20−2ab11, and R7(x,y) and R8(x,y) are C∞ functions in (x,y) at least of the third order.
Therefore, if ef≠0, then the positive equilibrium R1S2 of system (3.1) is a cusp of codimension 2 by the qualitative theory of ordinary differential equations and the theory of differential manifolds [30,34]. This completes the proof.
Further, we choose τ and δ1 as bifurcation parameters, and suppose that there exist parameters τ∗>0 and δ∗1>0 such that pR1S2=0 and qR1S2=0, i.e.,
{2rωK[rδ∗1+Kr1(ηβ−δ∗1ω)2r(ηβ−δ∗1ω)]2−(ηβ−δ∗1ω−rK+r1ω)[rδ∗1+Kr1(ηβ−δ∗1ω)2r(ηβ−δ∗1ω)]+δ∗1=0,4rK(βτ∗−r1δ∗1)(ηβ−δ∗1ω)+[r1(ηβ−δ∗1ω)+rδ∗1K]2=0. |
Note that the existence of (τ∗,δ∗1) can be easily confirmed by numerical investigations. We study the dynamical behavior of system (3.1) when parameters τ and δ1 vary in a small neighborhood of (τ∗,δ∗1), and analyze the local representations of the bifurcation curves in a small neighborhood of the positive equilibrium R1S2.
Theorem 3.12. Suppose ef≠0 at the positive equilibrium R1S2, then system (3.1) undergoes a Bogdanov-Takens bifurcation in a small neighborhood of R1S2 as (τ,δ1) varies near (τ∗,δ∗1) provided that ae+2f≠0, and system (3.1) has the following bifurcation behaviors in a small neighborhood of R1S2:
(ⅰ) the saddle-node bifurcation curve: SN={(ε1,ε2)| ge4f31=0};
(ⅱ) the Hopf bifurcation curve: HB={(ε1,ε2)| ge4f31+h2e4f41=0,he2f21>0};
(ⅲ) the homoclinic bifurcation curve: HL={(ε1,ε2)| ge4f31+4925h2e4f41=0,he2f21>0}.
Proof. First of all, let δ1=δ∗1−ε1 and τ=τ∗−ε2, then system (3.1) can be represented as
{dxdt=r1x−rKx2−βxy1+ωx,dydt=ηβxy1+ωx−(δ∗1−ε1)y+(τ∗−ε2). | (3.30) |
Translating the positive equilibrium R1S2(x1,y1) to the origin by the change of variables ˉx=x−x1 and ˉy=y−y1, renaming (ˉx,ˉy) as (x,y) and expanding the right-hand side of system (3.30) in a Taylor series about the origin, then we can obtain
{dxdt=ax+by+a20x2+2a11xy+R9(x,y,ε1,ε2),dydt=(ε1y1−ε2)+cx+d1y+b20x2+2b11xy+R10(x,y,ε1,ε2), | (3.31) |
where d1=(−δ∗1+ε1)+ηβx11+ωx1, and R9(x,y,ε1,ε2) and R10(x,y,ε1,ε2) are C∞ functions in (x,y) at least of the third order, whose coefficients depend smoothly on ε1 and ε2. Let
u=x,v=ax+by |
and rename (u,v) as (x,y), then system (3.31) can be written as
{dxdt=y+(a20−2aa11b)x2+2a11bxy+R11(x,y,ε1,ε2),dydt=b(ε1y1−ε2)−aε1x+ε1y+(aa20−2a2a11b+bb20−2ab11)x2+(2aa11b+2b11)xy+R12(x,y,ε1,ε2), | (3.32) |
where R11(x,y,ε1,ε2) and R12(x,y,ε1,ε2) are C∞ functions in (x,y) at least of the third order, whose coefficients depend smoothly on ε1 and ε2. Making the following change of variables
u=x−a11bx2,v=y+(a20−2aa11b)x2 |
and renaming (u,v) as (x,y), then system (3.32) becomes
{dxdt=y+R13(x,y,ε1,ε2),dydt=b(ε1y1−ε2)−aε1x+ε1y+exy+f1x2+R14(x,y,ε1,ε2), | (3.33) |
where f1=aa20−2a2a11b+bb20−2ab11−aa11bε1−ε1(a20−2aa11b), and R13(x,y,ε1,ε2) and R14(x,y,ε1,ε2) are C∞ functions in (x,y) at least of the third order, whose coefficients depend smoothly on ε1 and ε2.
Next, we make the following change of variables
u=x,y=y+R13(x,y,ε1,ε2) |
and rename (u,v) as (x,y), then system (3.33) becomes
{dxdt=y,dydt=b(ε1y1−ε2)−aε1x+ε1y+exy+f1x2+R15(x,y,ε1,ε2), | (3.34) |
where R15(x,y,ε1,ε2) is a C∞ function in (x,y) at least of the third order, whose coefficients depend smoothly on ε1 and ε2. By setting u=x+ε1e and v=y, renaming (u,v) as (x,y), then system (3.34) becomes
{dxdt=y,dydt=g+hx+exy+f1x2+R16(x,y,ε1,ε2), | (3.35) |
where g=b(ε1y1−ε2)+f1ε21e2+aε21e and h=−aε1−2f1ε1e, and R14(x,y,ε1,ε2) is a C∞ function in (x,y) at least of the third order, whose coefficients depend smoothly on ε1 and ε2. Moreover,
limεj→0f1=aa20−2a2a11b+bb20−2ab11=f, |
where j=1,2.
Notice that f1≠0 when εj are small. Making the change of variables one more time by setting
u=e2f1x,v=e3f21y,τ=ef1t |
when εj are small, and renaming (u,v,τ) as (x,y,t), then system (3.35) can be represented as
{dxdt=y,dydt=μ1(ε1,ε2)+μ2(ε1,ε2)x+xy+x2+R17(x,y,ε1,ε2), | (3.36) |
where μ1(ε1,ε2)=ge4f31 and μ2(ε1,ε2)=he2f21, and R15(x,y,ε1,ε2) is a C∞ function in (x,y) at least of the third order, whose coefficients depend smoothly on ε1 and ε2. Moreover, by a simple calculation, we can obtain that
|∂(μ1(ε1,ε2),μ2(ε1,ε2))∂(ε1,ε2)|(0,0)=(−1)be5f5(ae+2f), |
when ae+2f≠0, the above parameter transformation is a homeomorphism in a small neighborhood of the origin [36], and μ1 and μ2 are independent parameters.
Based on the above analyses, according to the theorems in Bogdanov and Takens [30,31], we can obtain the local representations of the bifurcation curves of system (3.36) in a small neighborhood of the origin if ae+2f≠0, and it is equivalent to the bifurcation behavior of system (3.1) in a small neighborhood of R1S2, as shown in Theorem 3.12. This completes the proof.
In this section, we mainly analyze the complete behavior of non-smooth Filippov system (2.2) including the existence, stability of the regular/virtual equilibria, pseudo-equilibria, sliding segments, sliding bifurcations and sliding periodic solutions.
In order to analyze the dynamical behavior of non-smooth Filippov system (2.2) in R2+, we need to analyze the existence and stability of the sliding segments and tangent points firstly.
The sliding segment: by a simple calculation, we can obtain
σ(x,y)=⟨HX(x,y),f1(x,y)⟩⟨HX(x,y),f2(x,y)⟩=⟨(1,0),(rx−rKx2−βxy1+ωx,ηβxy1+ωx−δy)⟩ ⟨(1,0),(rx−rKx2−βxy1+ωx−αx,ηβxy1+ωx−δ1y+τ)⟩=x2(r−rKx−βy1+ωx)(r1−rKx−βy1+ωx), |
where r1=r−α and 0<ET<K. It is easy to obtain that if x=ET, then σ(x,y)≤0 is equivalent to the inequalities
r1β+(r1ωβ−rβK)ET−rωβKET2≤y≤rβ+(rωβ−rβK)ET−rωβKET2. |
For convenience, denote
ymin≐r1β+(r1ωβ−rβK)ET−rωβKET2, |
ymax≐rβ+(rωβ−rβK)ET−rωβKET2, |
where ymin≥0 for all ET∈(0,r1Kr]; ymax>0 for all ET∈(0,K). And if one of the following conditions
y<ymin or y>ymax |
holds true, then σ(x,y)>0.
Therefore, the sliding segment of non-smooth Filippov system (2.2) is defined as
Σs={(x,y)|x=ET,max{0,ymin}≤y≤ymax}, |
and the crossing set is given as
Σc={(x,y)|x=ET,0≤y<max{0,ymin} or y>ymax}. |
Moreover, we have
⟨HX(x,y),f1(x,y)⟩=⟨(1,0),(rx−rKx2−βxy1+ωx,ηβxy1+ωx−δy)⟩=rx−rKx2−βxy1+ωx>0 |
and
⟨HX(x,y),f2(x,y)⟩=⟨(1,0),(rx−rKx2−βxy1+ωx−αx,ηβxy1+ωx−δ1y+τ)⟩=r1x−rKx2−βxy1+ωx<0 |
for all points (x,y)∈Σs, which indicates that the sliding segment Σs is stable.
According to the Filippov convex method [32], we can obtain the following sliding dynamical equation defined in (x,y)∈Σs
dydt=λ(ηβx1+ωxy−δy)+(1−λ)(ηβx1+ωxy−δ1y+τ)≐F(y), x(t)=ET, | (4.1) |
where
λ=⟨(1,0),((r1−rxK)x−βx1+ωxy,ηβx1+ωxy−δ1y+τ)⟩⟨(1,0),(−αx,(δ−δ1)y+τ)⟩=(1−rα)+rETαK+βyα(1+ωET). |
The equilibrium R0(x∗,y∗)∈Σs of system (4.1) is called the pseudo-equilibrium of non-smooth Filippov system (2.2).
Tangent point: The tangent points Ti(xi,yi)(i=1,2) of non-smooth Filippov system (2.2) satisfy the following equations
{H(xi,yi)=0,⟨HX(xi,yi),fi(xi,yi)⟩=0. |
By a simple calculation, we have
{x1=ET,y1=ymax;x2=ET,y2=ymin. |
That is to say, the sliding segment Σs is delimited by tangent points T1(x1,y1) and T2(x2,y2), which lie between the horizontal non-zero isocline L1S1 of subsystem S1 and L1S2 of subsystem S2, as shown in Figure 6A. Note that the tangent point T2 becomes an end point of sliding segment once ymin is less than zero. Therefore, we call T2(ET,ymin)∈R2+ as a tangent point if ET∈(0,r1Kr]. Otherwise, if ET∈(r1Kr,K), we call T2 as an end point of the sliding segment Σs.
For the equilibria of non-smooth Filippov system (2.2), there are four types: regular equilibrium, virtual equilibrium, pseudo-equilibrium and boundary equilibrium, which are associated with the discontinuity boundary Σ and have been defined in Section 2. For convenience, the equilibria of subsystem S1 are denoted by RiS1(xiS1,yiS1) and the equilibria of subsystem S2 are denoted by RiS2(xiS2,yiS2), i=0,1,2.
For the existence and stability of the pseudo-equilibrium R0(x∗,y∗) of non-smooth Filippov system (2.2), we only need to consider the existence and stability of equilibrium of system (4.1). Thus, we consider the equation F(y)=0, i.e.,
λ(ηβET1+ωETy−δy)+(1−λ)(ηβET1+ωETy−δ1y+τ)=0, | (4.2) |
which can be represented as
β(δ1−δ)α(1+ωET)y2+[−δ−(rα−rETαK)(δ1−δ)+ηβET1+ωET−βτα(1+ωET)]y+rτα(1−ETK)=0. | (4.3) |
If δ1=δ (i.e., the pesticide does not affect the predator), solving the above equation with respect to y, we can obtain
y∗=τrα(ETK−1)ηβET1+ωET−βτα(1+ωET)−δ, |
where y∗∈[max{0,ymin},ymax] if and only if ET∈[x1S2,x2S1]. Moreover, it is easy to obtain that
F′(y∗)=−βτα(1+ωET)+ηβET1+ωET−δ=−τrαy∗(1−ETK)<0, |
which indicates that the pseudo-equilibrium R0(x∗,y∗)=R0(ET,y∗)∈Σs is locally stable. Further, we have the following result.
Lemma 4.1. Suppose δ1=δ, then non-smooth Filippov system (2.2) has a unique and stable pseudo-equilibrium R0(ET,y∗) if and only if ET∈[x1S2,x2S1].
If δ1>δ, it is clear that Eq (4.3) have at most two positive real roots:
y∗1=(r−rETK)(1+ωET)2β+αδ+βτ−α(ηβ−δω)ET2β(δ1−δ)+α(1+ωET)√Δ52β(δ1−δ) |
and
y∗2=(r−rETK)(1+ωET)2β+αδ+βτ−α(ηβ−δω)ET2β(δ1−δ)−α(1+ωET)√Δ52β(δ1−δ), |
where
Δ5≐[−δ−(rα−rETαK)(δ1−δ)+ηβET1+ωET−βτα(1+ωET)]2−4βrτ(δ1−δ)(1−ETK)α2(1+ωET)>0. | (4.4) |
This indicates that there may exist two pseudo-equilibria, denoted by R10(ET,y∗1) and R20(ET,y∗2), as shown in Figure 7. Further, by a simple calculation, we can obtain that if max{0,ymin}≤y∗2<y∗1≤ymax, system (2.2) can exactly exist two pseudo-equilibria, and
F′(y∗1)=√Δ5andF′(y∗2)=−√Δ5, |
which indicate that R10(ET,y∗1) is unstable provided that it lies in the Σs, and R20(ET,y∗2) is locally stable provided that it lies in the Σs.
Note that to show how the threshold value ET affects the existence of pseudo-equilibria of system (2.2), we first consider the function F(y) defined in (4.1) as a function of x, i.e., the curve L1 or L2 shown in Figure 7, which can intersect with isoclines L1S1 and L1S2 at two points R1S2 and R2S1. Therefore, the horizontal components of both points may confirm the ranges of ET, within them system (2.2) could exist one or two pseudo-equilibria, as shown in Figure 7. In particular, if δ1=δ, then there exists a pseudo-equilibrium R0(ET,y∗)∈Σs with ET∈[x1S2,x2S1]. If δ1>δ, then for ET∈[x1S2,x2S1), there exists a unique pseudo-equilibrium R10; for ET∈[x2S1,ET1) with Δ5(ET1)=0 (here Δ5 is considered as a function of ET defined by (4.4)), there exist two pseudo-equilibria R10 and R20, shown in Figure 7B. Especially, if ET=ET1, then R10 collides with R20.
Based on the above analyses, we can now clarify the types of equilibria of non-smooth Filippov system (2.2). It follows from the conditions of existence and stability of equilibria of subsystem S1, as shown in Section 2, then we consider the following three cases:
(C1):0<δηβ−δω<K; (C2):δηβ−δω<0; (C3):δηβ−δω≥K. |
For Case (C1), according to the properties of subsystem S2, we obtain that if R0>0 and R1<0, then there exist two equilibria: a boundary equilibrium R0S2(0,τδ1) which is a unstable saddle, and a positive equilibrium R1S2(x1S2,y1S2) with
x1S2=KR2−K√R22+4rR0R1K2rR0, y1S2=(r1−rKx1S2)1+ωx1S2β. |
While subsystem S1 has a unique positive equilibrium R2S1(δηβ−δω,rη(Kηβ−Kδω−δ)K(ηβ−δω)2).
If δ1=δ, by a simple calculation, we obtain that x1S2<δηβ−δω, which indicates that R1S2 is on the left of R2S1, as shown in Figure 6B and Figure 7A. And from Figure 7A, it is easy to see that R0S1 is always a regular equilibrium for subsystem S1, R1S1 is always a virtual equilibrium for subsystem S1 and R0S2 is always a virtual equilibrium for subsystem S2. Further, the existence of all types of equilibria will be discussed briefly in the following:
(ⅰ) If 0<ET<x1S2, we conclude that R2S1 is a virtual equilibrium for subsystem S1, R1S2 is a regular equilibrium for subsystem S2, and T1 is an invisible tangent point and T2 is a visible tangent point [23,24].
(ⅱ) If x1S2<ET<x2S1, we have that R2S1 is a virtual equilibrium for subsystem S1, R1S2 becomes a virtual equilibrium for subsystem S2, and T1 is an invisible tangent point and T2 becomes an invisible tangent point. Moreover, there exists a pseudo-equilibrium R0.
(ⅲ) If x2S1<ET<K, then R0 disappears, R2S1 becomes a regular equilibrium for subsystem S1, R1S2 is a virtual equilibrium for subsystem S2, T1 becomes a visible tangent point and T2 is an invisible tangent point. Especially, when ET=x1S2 (or ET=x2S1), R1S2 (or R2S1) collides with Σ, which is a boundary equilibrium. Moreover, three points T2, R1S2 and R0 collide together in this case.
If δ1>δ, the position relations of all possible equilibria of two subsystems are hard to determine analytically. However, similar results can be obtained numerically as those shown above, so we omit them here. For Cases (C2) and (C3), subsystem S1 only has steady state R0S1(0,0) which is unstable, and exists a boundary equilibrium R1S1(K,0) which is globally stable related to region S1. The positive equilibria of subsystem S2 lie between R0S1(0,0) and R1S1(K,0). Therefore, we can discuss the types of equilibria of system (2.2) similarly.
In this subsection, we address the bifurcations related to equilibria and sliding cycles concerning sliding segment Σs.
Note that one of the main purposes of the present paper is to address the integrated pest management strategies for pest control and propose the non-smooth Filippov system (2.2), which indicates that how the key parameters related to control effectiveness affect the dynamics of system (2.2) are quite important. Thus, in this section we choose the releasing constant τ as a bifurcation parameter and fix all others to discuss the variations of the trajectories and equilibria of system (2.2).
For Case (C1), if δ1=δ, there may exist a stable pseudo-equilibrium R0(x∗,y∗). Moreover, the bifurcation diagrams with respect to τ shown in Figure 8A reveals that the existence interval of pseudo-equilibrium is an increasing function of τ. In this case, the pseudo-equilibrium R0 is stable with respect to the sliding segment Σs. All these results confirm that the larger τ, the more easily does the system stabilize at the pseudo-equilibrium R0, i.e., the number of pests finally stabilizes at the ET, as shown in Figures 8D and 8E. Note that for the untreated subsystem S1, the solution may exceed the EIL resulting in a pest outbreak. However, if we choose the appropriate ET such as ETi(i=1,2,3,4) shown in Figures 8D and 8E such that there exists the pseudo-equilibrium R0∈Σs, we can see that the number of pests is not only less than EIL, but also can be stabilized at the pseudo-equilibrium R0. Moreover, the lower is ET, the lower is the number of pests.
If δ1>δ, i.e., spraying pesticides could kill natural enemies, then the effect of releasing constant τ on the existence interval of the pseudo-equilibrium is complex and there may exist two pseudo-equilibria, as shown in the bifurcation diagrams of Figure 9A, B, C. From these we can see that the existence interval of the pseudo-equilibrium is a non-monotonic function of τ, which indicates that if pesticides can kill natural enemies, then the dynamics (particular sliding dynamics) of system (2.2) could be significantly affected, such as the stabilization level as shown in Figures 9D and 9E. It is interesting to note that although the number of pests in untreated subsystem S1 could increase and exceed the EIL, as shown in Figure 9D, the final size is less than the treated Filippov system (2.2) due to side effects of the pesticide on natural enemies. And it is easy to obtain that the side effects can be effectively avoided by increasing the releasing constant τ, as shown in Figure 9E, which can maintain the number of pests always below the EIL and realizes the control purpose.
For Case (C2), it is easy to obtain that R0S1 and R0S2 always keep in region S1, and R1S1 always lies in region S2. Hence, R0S1 is a regular equilibrium for subsystem S1, R0S2 is a virtual equilibrium for subsystem S2 and R1S1 is a virtual equilibrium for subsystem S1. Moreover, in this case, R2S1 disappears. If we choose τ as the bifurcation parameter, then system (2.2) may have multiple positive equilibria such as R1S2 and R2S2, as parameter τ varies. And the boundary equilibrium (BE) and pseudo-equilibrium could also appear. For example, if R1S2 collides with the switching line Σ as parameter τ increases, then a BE appears, as shown in Figure 10. In particular, we have:
(ⅰ) if τ∗5<τ<τ∗6, then two virtual equilibria R1S2 and R2S2 coexist, and R1S2 collides with R2S2 at τ=τ∗6.
(ⅱ) if τ>τ∗6, R1S2 and R2S2 disappear simultaneously, and system (2.2) does not exist with any positive equilibrium.
Note that the existence and types of equilibria for non-smooth system (2.2) can be discussed by using bifurcation analyses of two subsystems with respect to key parameters (Fig. 10), which bring convenience to the analysis of local bifurcations of the Filippov system, especially those bifurcations related to equilibria. Thus, the local equilibrium bifurcations for other cases can be similarly addressed by employing similar techniques.
In this subsection, we choose ET as the bifurcation parameter to investigate the codimension-1 local and global bifurcations to analyze the effect of ET on the dynamics of system (2.2). We realize that there may exist similar local and global sliding bifurcations for Cases (C1), (C2) and (C3), so we will take (C1) as an example to discuss the possible local and global sliding bifurcations in more detail in the following.
For Case (C1), if R1<0, then system (2.2) could have five equilibria R0S1(0,0), R1S1(K,0), R2S1(x2S1,y2S1), R0S2(0,τδ1), R1S2(x1S2,y1S2). In particular, if δ1=δ, then x1S2<x2S1, i.e., R1S2 is on the left of R2S1, as shown in Figure 7A, which indicates that R1S2 and R2S1 can not be the regular equilibria at the same time and it is impossible to have limit cycles on both sides of the Σ. If δ1≠δ, there may exist rich bifurcations which will be addressed numerically, as shown in Figure 11. It reveals how the ET affects the dynamics of system (2.2), it is easy to see that as parameter ET varies, system (2.2) exists with boundary equilibrium bifurcations and periodic solutions which lie in region S1 (or S2). Moreover, there exist other types of periodic solutions including sliding periodic solutions, which include a piece of the sliding segment or the entire sliding segment in Σ; crossing periodic solutions, which only include a point of the sliding segment or without any points of the sliding segment in Σ [17,23].
In the following part, we begin to discuss boundary equilibrium bifurcations and other types of periodic solutions though the global sliding bifurcations as parameter ET varies, as shown in Figure 11.
Touching bifurcation: A standard piece of the cycle can collide with the discontinuity boundary as parameter ET varies, this bifurcation is called a touching bifurcation (or grazing or even a sliding-grazing bifurcation) [17,23]. From Figure 11A, B and C, it is easy to see that if 8.45<ET<K, there exists a unique and stable cycle (i.e., periodic solution) in region S1. As parameter ET decreases, the cycle closes to Σ. Especially, when ET≈8.45, a touching bifurcation occurs, as shown in Figure 11B, where the cycle is tangent to Σs at the visible tangent point T1, denoted by X0(x(t),y(t)). As parameter ET continues to decrease, X0(x(t),y(t)) will contain a piece of the sliding segment in Σ, becoming a sliding cycle (i.e., sliding periodic solution), as shown in Figure 11C with ET=8. The computation of the critical cycle X0(x(t),y(t)), which ends at the visible tangent point T1, is equivalent to solving the following boundary-value problem, which can be solved by XPPAUT software [37].
{˙X0(x(t),y(t))=f1(x(t),y(t)),X0(T1)=0,x(T01)=x(0)=ET,y(T01)=y(0)=rβ+(rωβ−rβK)ET−rωβKET2,⟨HX(T1),f1(T1)⟩=0, |
where T01 represents the period of the cycle X0(x(t),y(t)).
Buckling bifurcation: From Figure 11C, D, we can obtain that there exists a stable sliding cycle, which passes the visible tangent point T1 for 7.25<ET<8.45. As parameter ET decreases, especially ET≈7.25, the sliding cycle passes the invisible tangent point T2, denoted by X1(x(t),y(t)). Moreover, it contains the entire sliding segment Σs, which means that a buckling bifurcation occurs. As parameter ET continues to decrease, X1(x(t),y(t)) remains but enters into region S2 before returning back to the sliding segment for ET>4.9, as shown in Figure 11E.
Crossing bifurcation: From Figure 11E, F and G, we can see that a stable sliding cycle becomes a stable crossing cycle as parameter ET decreases. Especially, when ET reaches around 4.9, the sliding cycle only passes one point of the sliding segment Σs (i.e., the visible tangent point T1), denoted by X2(x(t),y(t)). As parameter ET continues to decrease, X2(x(t),y(t)) becomes a stable crossing cycle without any points of the sliding segment Σs, as shown in Figure 11G. For the above processes, we say that a crossing bifurcation (or sliding-crossing) has occurred.
Pseudo-homoclinic bifurcation: As parameter ET decreases from 4.3 to 1.9128, the crossing bifurcation and buckling bifurcation occur again, as shown in Figure 11H, I and J. If parameter ET continues to decrease, we can see that when ET=1.8998 (i.e., ET=x1S2), a stable sliding cycle surrounds the unstable focus R2S1 and the tangent point T2 collides with R1S2 simultaneously. As parameter ET reaches around 1.899, the stable sliding cycle passes the pseudo-equilibrium R0, which is a pseudo-saddle, forming a homoclinic orbit which contains a piece of sliding segment Σs. In this case, we say that a pseudo-homoclinic bifurcation [17,23] occurs and there are limit cycles on both sides of the Σ, as shown in Figure 11L. In fact, when ET=x1S2, three points T2, R1S2 and pseudo-equilibrium R0 can collide together. As parameter ET continues to decrease, the three points T2, R1S2 and R0 coexist, and there exists a stable sliding cycle surrounding the unstable equilibrium R1S2, as shown in Figure 11M. It follows from Figure 11(J-M) that a boundary equilibrium bifurcation (or the pseudo-equilibrium bifurcation) occurs at ET=1.8998 (i.e., ET=x1S2). This bifurcation entails the catastrophic disappearance of a stable sliding cycle and a unstable pseudo-equilibrium R0.
Based on the above discussion, we can conclude that when we choose ET as the bifurcation parameter and fix all other parameters of system (2.2), there exist rich local and global sliding bifurcations as follows: touching → buckling → crossing → crossing → buckling → pseudo-homoclinic → boundary equilibrium bifurcations. It is interesting to note that it will cause the change of the regular/virtual equilibria, pseudo-equilibria and trajectories of system (2.2) as ET varies, as shown in Figures 11 and 12. In particular, the results shown in Figure 12 reveal the importance of choosing the threshold level ET. Comparing with the two trajectories of treated and untreated systems shown in Figure 12, we conclude that for a given ET, the pest population can be successfully controlled and maintained below the EIL if the IPM is properly designed.
Based on the implementation of biological control in real life, we propose a new non-smooth Filippov system (2.2) with constant releasing rate in this paper. The detailed qualitative analyses for subsystem S2 were carried out first, which will be crucial for analyzing Filippov system (2.2). Our main results reveal that the releasing constant τ plays an important role in determining the dynamics and bifurcations of subsystem S2. For example, the threshold conditions for the existence and stability of equilibria and the type of bifurcations including Hopf bifurcation, transcritical bifurcation, saddle-node bifurcation and Bogdanov-Takens bifurcation can be significantly affected by the releasing constant. Moreover, from a pest control point of view there exists a critical releasing rate such that the pest population will be driven to extinction when the releasing rate is greater than the critical value. Meanwhile, compared with the main results obtained in literature [31], we conclude that the sign of the constant τ can significantly affect the dynamical behaviour.
Combining the dynamics of two subsystems S1 and S2, and employing the techniques for a non-smooth Filippov system, we focus on some typical cases on system (2.2) with aims to address how the threshold level ET affects the sliding dynamics and pest control [17,24]. The existence and stability of the sliding mode and pseudo-equilibria have been discussed first, and our results indicate that the releasing constant and side effects of the pesticide on natural enemies could result in multiple pseudo-equilibria. Especially, the existence interval of pseudo-equilibria can be greatly influenced by the threshold level ET and releasing constant τ, as shown in Figures 8 and 9. It is interesting to note that although the number of pests in the untreated subsystem could increase and exceed the EIL, the stabilization level could be less than ET and stabilizes at a lower level than the treated subsystem (i.e., one of stable states of Filippov system (2.2)) due to side effects of the pesticide on natural enemies. In this case, the paradoxical effects of the Volterra principle occur, i.e., spraying pesticide does not reduce the number of pests, but increases them. However, the side effects can be effectively avoided by increasing the releasing constant, which can maintain the number of pests always below the EIL and realizes the control purpose. Furthermore, by numerical bifurcation analyses, the sliding bifurcations including boundary equilibrium bifurcation, touching bifurcation, buckling bifurcation, crossing bifurcation, pseudo-homoclinic bifurcation have been discussed as the threshold level ET varies, which indicates that the pest population can be successfully controlled and maintained below the EIL if the IPM strategy is properly designed, as shown in Figure 12.
In summary, the new model presented in this paper not only has new dynamical behaviour, but also adopts and develops new qualitative techniques. Moreover, the new dynamical behaviour presented in the model has clear practical significance which can help to guide pest control. Some new non-smooth systems involving the development of pest resistance [38,39] and adaptive control strategy [40,41] will be developed in later research and will be studied in detail.
The authors thank Prof Robert A. Cheke for his kind help and comments, thank the anonymous referees and the editor for their helpful comments and valuable suggestions. This work is supported by the National Natural Science Foundation of China (NSFCs, 61772017, 11631012, 11601301), and by the Fundamental Research Funds For the Central Universities (2018CBLZ001, GK201901008, GK201803006).
The authors declare there is no conflict of interest.
[1] |
Amphett JC, Baumert RM, Mann RF, et al. (1995) Performance modeling of the Ballard Mark IV solid polymer electrolyte fuel cell. J Electrochem Soc 142: 1–8. https://doi.org/10.1149/1.2043866 doi: 10.1149/1.2043866
![]() |
[2] |
Mann RF, Amphlett JC, Hooper MAI, et al. (2000) Development, and application of a generalized steady-state electrochemical model for a PEM fuel cell. J Power Sources 86: 173–180. https://doi.org/10.1016/S0378-7753(99)00484-X doi: 10.1016/S0378-7753(99)00484-X
![]() |
[3] |
Akimoto Y, Suzuki S (2018) Overpotential evaluation of PEMFC using semi-empirical equation and SEM. Conf E3S Web 67: 01015. https://doi.org/10.1051/e3sconf/20186701015 doi: 10.1051/e3sconf/20186701015
![]() |
[4] |
Kandidayeni M, Macias A, Khalatbarisoltani A, et al. (2019) Benchmark of proton exchange membrane fuel cell parameters extraction with metaheuristic optimization algorithms. Energy 183: 912–925. https://doi.org/10.1016/j.energy.2019.06.152 doi: 10.1016/j.energy.2019.06.152
![]() |
[5] |
Xu S, Wang Y, Wang Z (2019) Parameter estimation of proton exchange membrane fuel cells using Eagle strategy based on JAYA algorithm and Nelder-Mead simplex method. Energy 173: 457–467.https://doi.org/10.1016/j.energy.2019.02.106 doi: 10.1016/j.energy.2019.02.106
![]() |
[6] |
Murugesan K, Subramaniam U (2020) Characterization and experimental validation of a semi-empirical fuel-cell model for investigating the water dynamics on the electrical behavior of a 5 kW Ballard stack system using Nafion 117 polymer membrane. J Renewable Sustainable Energy 12: 024301. https://doi.org/10.1063/1.5121609 doi: 10.1063/1.5121609
![]() |
[7] |
Selem S, Hasanien H, El-Fergany A (2020) Parameters extraction of PEMFC's model using manta rays foraging optimizer. Energy Res 44: 4629–4640. https://doi.org/10.1002/er.5244 doi: 10.1002/er.5244
![]() |
[8] |
Ariza HE, Correcher A, Sánchez C, et al. (2018) Thermal and electrical parameter identification of a proton exchange membrane fuel cell using genetic algorithm. Energies 11: 2099. https://doi.org/10.3390/en11082099 doi: 10.3390/en11082099
![]() |
[9] |
Werner C, Gores F, Busemeyer L, et al. (2015) Characteristics of PEMFC operation in ambient- and low-pressure environment considering the fuel cell humidification. CEAS Aeronaut J 6: 229–243. https://doi.org/10.1007/s13272-014-0142-z doi: 10.1007/s13272-014-0142-z
![]() |
[10] |
Pratt JW, Brouwer J, Samuelsen GS (2007) Performance of proton exchange membrane fuel cell at High-Altitude conditions. J Propuls Power 23: 437–444. https://doi.org/10.2514/1.20535 doi: 10.2514/1.20535
![]() |
[11] |
Khan SS, Hussain S, Bouhaddioui C, et al. (2020) Membrane-hydration-state detection in proton exchange membrane fuel cells using improved ambient-condition-based dynamic model. Energy Res 44: 869–889. https://doi.org/10.1002/er.4927 doi: 10.1002/er.4927
![]() |
[12] |
Khan S, Shareef H, Khan I, et al. (2019) Effect of ambient conditions on water management and faults in PEMFC systems: A Review. Conf IEEE Electr Comput Eng. https://doi.org/10.1109/CCECE.2019.8861579 doi: 10.1109/CCECE.2019.8861579
![]() |
[13] |
Khan SS, Shareef H, Wahyudie A, et al. (2019) Influences of ambient conditions on the performance of proton exchange membrane fuel cell using various models. Energy Environ 30: 1087–1110. https://doi.org/10.1177/0958305X18802775 doi: 10.1177/0958305X18802775
![]() |
[14] |
Ye M, Wang X, Xu Y, et al. (2009) Parameter identification for proton exchange membrane fuel cell model using particle swarm optimization. Int J Hydrogen Energy 34: 981–989. https://doi.org/10.1016/j.ijhydene.2008.11.026 doi: 10.1016/j.ijhydene.2008.11.026
![]() |
[15] |
Menesy AS, Sultan HM, Korashy A, et al. (2020) Effective parameter extraction of different polymer electrolyte membrane fuel cell stack models using a modified artificial ecosystem optimization algorithm. IEEE Access 8: 31892–31909. https://doi.org/10.1109/ACCESS.2020.2973351 doi: 10.1109/ACCESS.2020.2973351
![]() |
[16] |
Sultan HM, Menesy AS, Kamel S, et al. (2020) Parameter identification of proton exchange membrane fuel cell stacks using Bonobo optimizer. Conference IEEE Environment and Electrical Engineering, Madrid. https://doi.org/10.1109/EEEIC/ICPSEurope49358.2020.9160597 doi: 10.1109/EEEIC/ICPSEurope49358.2020.9160597
![]() |
[17] |
Abedinpourshotorban H, Shamsuddin SM, Beheshti Z, et al. (2016) Electromagnetic field optimization: a physics-inspired metaheuristic optimization algorithm. Swarm Evol Comput 26: 8–22. https://doi.org/10.1016/j.swevo.2015.07.002 doi: 10.1016/j.swevo.2015.07.002
![]() |
[18] |
Kaveh A, Dadras A (2017) A novel meta-heuristic optimization algorithm: thermal exchange optimization. Adv Eng Softw 110: 69–84. https://doi.org/10.1016/j.advengsoft.2017.03.014 doi: 10.1016/j.advengsoft.2017.03.014
![]() |
[19] |
Javidy B, Hatamlou A, Mirjalili S (2015) Ions motion algorithm for solving optimization problems. Appl Soft Comput 32: 72–79. https://doi.org/10.1016/j.asoc.2015.03.035 doi: 10.1016/j.asoc.2015.03.035
![]() |
[20] |
Kaveh A, Bakhshpoori B (2016) Water evaporation optimization: a novel physically inspired optimization algorithm. Comput Struct 167: 69–85. https://doi.org/10.1016/j.compstruc.2016.01.008 doi: 10.1016/j.compstruc.2016.01.008
![]() |
[21] |
Eskandar H, Sadollah A, Bahreinineja A, et al. (2012) Water cycle algorithm—a novel metaheuristic optimization method for solving constrained engineering optimization problems. Comput Struct 110–111: 151–166. https://doi.org/10.1016/j.compstruc.2012.07.010 doi: 10.1016/j.compstruc.2012.07.010
![]() |
[22] | Menesy AS, Sultan H, Kamel S (2020) Extracting model parameters of proton exchange membrane fuel cell using equilibrium optimizer algorithm. Conference IEEE Radio Electronics, Electrical and Power Engineering (REEPE) 2020, 1–7. https: //doi.org/10.1109/REEPE49198.2020.9059219 |
[23] |
Sultan H, Menesy AS, Kamel S, et al. (2020) Tree growth algorithm for parameter identification of proton exchange membrane fuel cell models. J Interact Multimed Artif Intell 3: 1–44. https://doi.org/10.9781/ijimai.2020.03.003 doi: 10.9781/ijimai.2020.03.003
![]() |
[24] |
Tian P, Liu X, Luo K, et al. (2021) Deep learning from three-dimensional multiphysics simulation in operational optimization and control of polymer electrolyte membrane fuel cell for maximum power. Appl Energy 288: 116632. https://doi.org/10.1016/j.apenergy.2021.116632 doi: 10.1016/j.apenergy.2021.116632
![]() |
[25] |
Ma R, Dang H, Xie R, et al. (2021) Online fault diagnosis for Open-cathode PEMFC systems based on output voltage measurements and data-driven method. IEEE Trans Transp Electrif 8: 2050–2061. https://doi.org/10.1109/TTE.2021.3114194 doi: 10.1109/TTE.2021.3114194
![]() |
[26] |
Wang Y, Feng X (2009) Analysis of the reaction rates in the cathode electrode of polymer electrolyte fuel cells: II. Dual-Layer electrodes. J Electrochem Society 156: 403–409. https://doi.org/10.1149/1.3056057 doi: 10.1149/1.3056057
![]() |
[27] |
Nguyen D, Pham T, Tanveer M, et al. (2022) Deep learning-based optimization of a microfluidic membraneless fuel cell for maximum power density via data-driven three-dimensional multiphysics simulation. Bioresour Technol 348: 126794. https://doi.org/10.1016/j.biortech.2022.126794 doi: 10.1016/j.biortech.2022.126794
![]() |
[28] |
Zhao J, Li X, Shum C, et al. (2021) A Review of physics-based and data-driven models for real-time control of polymer electrolyte membrane fuel cells. Energy AI 6: 100114. https://doi.org/10.1016/j.egyai.2021.100114 doi: 10.1016/j.egyai.2021.100114
![]() |
[29] |
Qais MH, Hasanien HM, Alghuwainem S (2020) Transient search optimization: a new meta-heuristic optimization algorithm. Appl Intell 50: 3926–3941. https://doi.org/10.1007/s10489-020-01727-y doi: 10.1007/s10489-020-01727-y
![]() |
[30] |
Yuan Z, Wang W, Wang H (2020) Optimal parameter estimation for PEMFC using modified monarch butterfly optimization. Energy Res 44: 8427–8441. https://doi.org/10.1002/er.5527 doi: 10.1002/er.5527
![]() |
[31] |
Wishart J, Dong Z, Secanell M (2006) Optimization of a PEM fuel cell system based on empirical data and a generalized electrochemical semi-empirical model. J Power Sources 161: 1041–1055. https://doi.org/10.1016/j.jpowsour.2006.05.056 doi: 10.1016/j.jpowsour.2006.05.056
![]() |
[32] |
Salim R, Nabag M, Noura H, et al. (2015) The parameter identification of the Nexa 1.2 kW PEMFC's model using particle swarm optimization. Renewable Energy 82: 26–34. https://doi.org/10.1016/j.renene.2014.10.012 doi: 10.1016/j.renene.2014.10.012
![]() |
[33] |
El-fergany A, Hasanien H, Agwa A (2019) Semi-empirical PEM fuel cells model using whale optimization algorithm. Energy Convers Manag 201: 112197. https://doi.org/10.1016/j.enconman.2019.112197 doi: 10.1016/j.enconman.2019.112197
![]() |
[34] |
Ali M, Elhameed MA, Farahat MA (2017) Effective parameters identification for polymer electrolyte membrane fuel cell models using grey wolf optimizer. Renewable Energy 111: 455–462. https://doi.org/10.1016/j.renene.2017.04.036 doi: 10.1016/j.renene.2017.04.036
![]() |
[35] |
Kaveh A, Bakhshpoori T (2016) Water evaporation optimization: a novel physically inspired optimization algorithm. Comput Struct 167: 69–85. https://doi.org/10.1016/j.compstruc.2016.01.008 doi: 10.1016/j.compstruc.2016.01.008
![]() |
[36] |
Menesy AS, Sultan HM, Korashy A, et al. (2021) A modified farmland fertility optimizer for parameters estimation of fuel cell models. Neural Comput Appl 33: 12169–12190. https://doi.org/10.1007/s00521-021-05821-1 doi: 10.1007/s00521-021-05821-1
![]() |
[37] |
Al-zeyoudi H, Sasmito AP, Shamim T (2020) Performance evaluation of an open-cathode PEM fuel cell stack under ambient conditions: Case study of United Arab Emirates. Energy Convers Manag 105: 798–809. https://doi.org/10.1016/j.enconman.2015.07.082 doi: 10.1016/j.enconman.2015.07.082
![]() |
[38] | Ozcelep Y, Gurkan K, Kuntman A (2013) Maximum load behaviour of a fuel cell stack under different ambient temperatures. Conference Electrical and Electronics Engineering. https://doi.org/10.1109/ELECO.2013.6713808 |
1. | Ayman A. Arafa, Soliman A.A. Hamdallah, Sanyi Tang, Yong Xu, Gamal M. Mahmoud, Dynamics Analysis of a Filippov Pest Control Model with Time Delay, 2021, 10075704, 105865, 10.1016/j.cnsns.2021.105865 | |
2. | Hao Zhou, Biao Tang, Huaiping Zhu, Sanyi Tang, Bifurcation and Dynamic Analyses of Non-monotonic Predator–Prey System with Constant Releasing Rate of Predators, 2022, 21, 1575-5460, 10.1007/s12346-021-00539-w | |
3. | Christian Cortés García, Bifurcations in discontinuous mathematical models with control strategy for a species, 2021, 19, 1551-0018, 1536, 10.3934/mbe.2022071 | |
4. | Xiang Hou, Bing Liu, Yilin Wang, Zhong Zhao, Complex dynamics in a Filippov pest control model with group defense, 2022, 15, 1793-5245, 10.1142/S179352452250053X | |
5. | Yunxiang Lu, Min Xiao, Jinling Liang, Jie Ding, Ying Zhou, Youhong Wan, Chunxia Fan, Hybrid Control Synthesis for Turing Instability and Hopf Bifurcation of Marine Planktonic Ecosystems With Diffusion, 2021, 9, 2169-3536, 111326, 10.1109/ACCESS.2021.3103446 | |
6. | Christian Cortés García, Bifurcations on a discontinuous Leslie–Grower model with harvesting and alternative food for predators and Holling II functional response, 2023, 116, 10075704, 106800, 10.1016/j.cnsns.2022.106800 | |
7. | Igor Belykh, Rachel Kuske, Maurizio Porfiri, David J. W. Simpson, Beyond the Bristol book: Advances and perspectives in non-smooth dynamics and applications, 2023, 33, 1054-1500, 010402, 10.1063/5.0138169 | |
8. | Hui Wang, Youping Yang, Dynamics analysis of a non-smooth Filippov pest-natural enemy system with time delay, 2023, 0924-090X, 10.1007/s11071-023-08332-x | |
9. | Yi Yang, Lirong Liu, Changcheng Xiang, Wenjie Qin, Switching dynamics analysis of forest-pest model describing effects of external periodic disturbance, 2020, 17, 1551-0018, 4328, 10.3934/mbe.2020239 | |
10. | Hao Zhou, Sanyi Tang, Bifurcation dynamics on the sliding vector field of a Filippov ecological system, 2022, 424, 00963003, 127052, 10.1016/j.amc.2022.127052 | |
11. | Jingna Liu, Qi Qi, Bing Liu, Shujing Gao, Pest control switching models with instantaneous and non-instantaneous impulsive effects, 2023, 205, 03784754, 926, 10.1016/j.matcom.2022.10.027 | |
12. | Soliman A. A. Hamdallah, Ayman A. Arafa, Stability analysis of Filippov prey–predator model with fear effect and prey refuge, 2024, 70, 1598-5865, 73, 10.1007/s12190-023-01934-z | |
13. | Wenxiu Li, Bifurcation analysis of a Filippov predator–prey model with two thresholds, 2024, 112, 0924-090X, 9639, 10.1007/s11071-024-09527-6 |