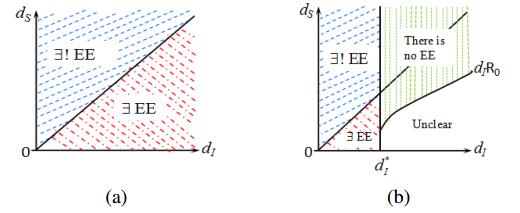
Environmental degradation, including air quality deterioration, has been mainly attributed to anthropogenic activities. Air pollution has become a pressing issue in industrialised and highly populated areas due to the combustion of fossil fuels and industrial operations. Recently, the COVID-19 pandemic led to a nationwide lockdown to control the spread of the coronavirus. This imposed restrictions on many economic activities, thus providing the environment with an opportunity to heal. The COVID-19 response measures adopted by most countries, including lockdown, restricted movement, and other containment measures, led to a significant decrease in energy use in the transport sector. Due to low electricity access levels in developing countries, traditional energy sources make up the bulk of energy used for most domestic energy services. Biomass combustion emits carbon monoxide (CO), while the transport sector is a major contributor of nitrogen dioxide (NO2). This study was purposed to investigate the short-term effects of COVID-19 on CO and NO2 concentration levels in Nairobi, Vihiga and Tana River counties. The study utilised data on CO surface concentration, NO2 column concentration and reported COVID-19 cases. Time series, correlation analysis and spatial and temporal map analysis were carried out to investigate the changes and relationships among the study parameters. The three counties were selected based on the urbanisation and population. Nairobi county represented an urban setting, while the Vihiga and Tana River counties represented rural areas with high and low population densities, respectively. The CO surface concentrations in Nairobi and Vihiga county significantly correlated with the COVID-19 cases, with both counties portraying negative correlations, i.e., −0.59 (P-value: 0.008) and −0.45 (P-value: 0.05), respectively. NO2 column concentration also exhibited a significant negative relationship with reported COVID-19 cases in the Vihiga (−0.018, P-value = 0.05) and Tana River (0.17, P-value = 0.00) counties. These findings highlight the need for demographic and economic considerations in CO and NO2 assessments, and allude to a decreased health risk due to CO and NO2 emissions during the COVID-19 pandemic.
Citation: Cohen Ang'u, Nzioka John Muthama, Mwanthi Alexander Mutuku, Mutembei Henry M'IKiugu. Carbon monoxide and nitrogen dioxide patterns associated with changes in energy use during the COVID-19 pandemic in Kenya[J]. AIMS Environmental Science, 2022, 9(3): 244-259. doi: 10.3934/environsci.2022017
[1] | Yoichi Enatsu, Yukihiko Nakata . Stability and bifurcation analysis of epidemic models with saturated incidence rates: An application to a nonmonotone incidence rate. Mathematical Biosciences and Engineering, 2014, 11(4): 785-805. doi: 10.3934/mbe.2014.11.785 |
[2] | Jinzhe Suo, Bo Li . Analysis on a diffusive SIS epidemic system with linear source and frequency-dependent incidence function in a heterogeneous environment. Mathematical Biosciences and Engineering, 2020, 17(1): 418-441. doi: 10.3934/mbe.2020023 |
[3] | Wenzhang Huang, Maoan Han, Kaiyu Liu . Dynamics of an SIS reaction-diffusion epidemic model for disease transmission. Mathematical Biosciences and Engineering, 2010, 7(1): 51-66. doi: 10.3934/mbe.2010.7.51 |
[4] | Baoxiang Zhang, Yongli Cai, Bingxian Wang, Weiming Wang . Dynamics and asymptotic profiles of steady states of an SIRS epidemic model in spatially heterogenous environment. Mathematical Biosciences and Engineering, 2020, 17(1): 893-909. doi: 10.3934/mbe.2020047 |
[5] | Yanyan Du, Ting Kang, Qimin Zhang . Asymptotic behavior of a stochastic delayed avian influenza model with saturated incidence rate. Mathematical Biosciences and Engineering, 2020, 17(5): 5341-5368. doi: 10.3934/mbe.2020289 |
[6] | James M. Hyman, Jia Li . Epidemic models with differential susceptibility and staged progression and their dynamics. Mathematical Biosciences and Engineering, 2009, 6(2): 321-332. doi: 10.3934/mbe.2009.6.321 |
[7] | Ruixia Zhang, Shuping Li . Analysis of a two-patch SIS model with saturating contact rate and one- directing population dispersal. Mathematical Biosciences and Engineering, 2022, 19(11): 11217-11231. doi: 10.3934/mbe.2022523 |
[8] | Abdennasser Chekroun, Mohammed Nor Frioui, Toshikazu Kuniya, Tarik Mohammed Touaoula . Global stability of an age-structured epidemic model with general Lyapunov functional. Mathematical Biosciences and Engineering, 2019, 16(3): 1525-1553. doi: 10.3934/mbe.2019073 |
[9] | Fang Wang, Juping Zhang, Maoxing Liu . Dynamical analysis of a network-based SIR model with saturated incidence rate and nonlinear recovery rate: an edge-compartmental approach. Mathematical Biosciences and Engineering, 2024, 21(4): 5430-5445. doi: 10.3934/mbe.2024239 |
[10] | Peter Rashkov, Ezio Venturino, Maira Aguiar, Nico Stollenwerk, Bob W. Kooi . On the role of vector modeling in a minimalistic epidemic model. Mathematical Biosciences and Engineering, 2019, 16(5): 4314-4338. doi: 10.3934/mbe.2019215 |
Environmental degradation, including air quality deterioration, has been mainly attributed to anthropogenic activities. Air pollution has become a pressing issue in industrialised and highly populated areas due to the combustion of fossil fuels and industrial operations. Recently, the COVID-19 pandemic led to a nationwide lockdown to control the spread of the coronavirus. This imposed restrictions on many economic activities, thus providing the environment with an opportunity to heal. The COVID-19 response measures adopted by most countries, including lockdown, restricted movement, and other containment measures, led to a significant decrease in energy use in the transport sector. Due to low electricity access levels in developing countries, traditional energy sources make up the bulk of energy used for most domestic energy services. Biomass combustion emits carbon monoxide (CO), while the transport sector is a major contributor of nitrogen dioxide (NO2). This study was purposed to investigate the short-term effects of COVID-19 on CO and NO2 concentration levels in Nairobi, Vihiga and Tana River counties. The study utilised data on CO surface concentration, NO2 column concentration and reported COVID-19 cases. Time series, correlation analysis and spatial and temporal map analysis were carried out to investigate the changes and relationships among the study parameters. The three counties were selected based on the urbanisation and population. Nairobi county represented an urban setting, while the Vihiga and Tana River counties represented rural areas with high and low population densities, respectively. The CO surface concentrations in Nairobi and Vihiga county significantly correlated with the COVID-19 cases, with both counties portraying negative correlations, i.e., −0.59 (P-value: 0.008) and −0.45 (P-value: 0.05), respectively. NO2 column concentration also exhibited a significant negative relationship with reported COVID-19 cases in the Vihiga (−0.018, P-value = 0.05) and Tana River (0.17, P-value = 0.00) counties. These findings highlight the need for demographic and economic considerations in CO and NO2 assessments, and allude to a decreased health risk due to CO and NO2 emissions during the COVID-19 pandemic.
To understand the dynamics of disease transmission in a spatially heterogeneous environment, an SIS epidemic reaction-diffusion model was proposed in [1], satisfying the parabolic system
{ˉSt=dSΔˉS−β(x)ˉSˉIˉS+ˉI+γ(x)ˉI,x∈Ω, t>0,ˉIt=dIΔˉI+β(x)ˉSˉIˉS+ˉI−γ(x)ˉI,x∈Ω, t>0,∂ˉS∂ν=∂ˉI∂ν=0,x∈∂Ω, t>0, | (1.1) |
where ˉS(x,t) and ˉI(x,t) denote the densities of susceptible and infected individuals at position x and time t, respectively; the positive constants dS and dI are the diffusion rates of the susceptible and infected individuals; the habitat Ω is assumed to be a bounded domain in Rn(n≥1) with smooth boundary ∂Ω; the positive Hölder-continuous functions β(x) and γ(x) on ˉΩ represent the rates of disease transmission and disease recovery at x, respectively; the homogeneous Neumann boundary condition means that there is no flux across the boundary ∂Ω, and ∂/∂ν is the outward normal derivative to ∂Ω.
In [1], under the assumption that the total population keep constant, the existence and uniqueness of the endemic equilibrium (EE) were achieved in terms of the basic reproduction number R0. Furthermore, the asymptotic profile of EE was obtained for small diffusion rate of susceptible individuals. To further understand the impact of large and small diffusion rates on the persistence and extinction of the disease, the global stability and asymptotic behavior of EE for system (1.1) were investigated in [20,21,22]. In [23], Peng and Zhao considered the diffusive SIS model with spatially heterogeneous and temporally periodic disease transmission and recovery rates. The authors in [4,5,11] studied the effects of diffusion and advection for a spatial SIS model in heterogeneous environments. Their results suggest that advection can help speed up the elimination of disease. Other related works on (1.1) can be found in [8,9,13,14,15].
The aforementioned studies adopt the standard incidence rate βˉSˉI/(ˉS+ˉI). Another most frequently used incidence rate is the bilinear incidence rate βˉSˉI (see [2,10]), which gives rise to the dependence of the basic reproduction number on the total population. For the diffusive SIS epidemic model with the bilinear incidence rate, Deng and Wu discussed the existence and the global attractivity of the EE in [7]. In the continued work [25], Wu and Zou explored the asymptotic profile of EE for large and small diffusion rates of the susceptible and infected individuals. They observed some new interesting profiles for such model. In contrast, Capasso and Serio in [6] pointed out that the number of effective contacts between infective individuals and susceptible individuals cannot always increase linearly with I; the bilinear incidence rate might be true for a small number of infectives, but unrealistic for large I. They introduced a saturated incidence rate g(ˉI)ˉS into epidemic models based on the study of the cholera epidemic spread in Bari of Italy, where
g(ˉI)=βˉI1+mˉI. |
Such an incident rate seems more realistic in certain situations because the number of effective contacts between infective individuals and susceptible individuals may saturate at high infective levels due to crowding of the infective individuals or due to the protection measures by the susceptible individuals. Here βˉI measures the infection force of the disease, 1/(1+mˉI) measures the inhibition effect from the behavioral change of the susceptible individuals when their number increases or from the crowding effect of the infective individuals, m>0 is the saturation coefficient. This type of incidence rate has been adopted by many authors [12,19,26,27].
However, to our best knowledge, little work has been devoted to the study of the diffusive epidemic model with saturated incidence rate. Inspired by the above research, we here consider an SIS epidemic reaction-diffusion model with saturated incidence rate. We are interested in the existence of the EE and particularly the effects of the diffusion rates and the saturated coefficient on asymptotic profile of EE. In contrast to [1] and [25], for some special case, such as the rate of disease transmission β being a constant and Ω being a high-risk domain, our results indicate that it is not enough to just restrict the movement of the susceptible individuals to completely eradicate the disease in the whole habitat; however, if the inhibition effect is large, the infectious disease will extinct eventually (see Theorem 1.3 and Corollary 1.5). In general, we conclude that the infective individuals cannot persist if the saturated coefficient is large with fixed diffusion rates of the susceptible and infected individuals (see Theorem 1.9).
In this paper, we are concerned with the following SIS epidemic reaction-diffusion model with saturated incidence rate:
{ˉSt=dSΔˉS−β(x)ˉSˉI1+mˉI+γ(x)ˉI,x∈Ω, t>0,ˉIt=dIΔˉI+β(x)ˉSˉI1+mˉI−γ(x)ˉI,x∈Ω, t>0,∂ˉS∂ν=∂ˉI∂ν=0,x∈∂Ω, t>0, | (1.2) |
where the parameters are described as before. We assume that the initial data satisfies the following hypothesis.
(H) ˉS(x,0), ˉI(x,0)≥0 are nonnegative continuous functions in ˉΩ, and the number of initial infectious individuals in the region is positive, i.e, ∫ΩˉI(x,0)dx>0.
By a similar argument as in [1], it is easy to show that system (1.2) admits a unique global classical solution (ˉS(x,t),ˉI(x,t)). Let
N:=∫Ω(ˉS(x,0)+ˉI(x,0))dx>0 |
be the total number of individuals in Ω at t=0. Adding the two equations in (1.2) and integrating over the domain Ω, we get
∂∂t∫Ω(ˉS+ˉI)dx=0, t>0. |
Hence, the total population size is a constant, i.e.,
∫Ω(ˉS(x,t)+ˉI(x,t))dx=N, t≥0. | (1.3) |
In the current paper, we mainly focus on the nonnegative equilibrium of problem (1.2), which is the nonnegative solution of the following semilinear elliptic system:
{dSΔS−β(x)SI1+mI+γ(x)I=0,x∈Ω,dIΔI+β(x)SI1+mI−γ(x)I=0,x∈Ω,∂S∂ν=∂I∂ν=0,x∈∂Ω. | (1.4) |
Here S(x) and I(x) denote the densities of susceptible and infected individuals at x∈ˉΩ, respectively. In view of (1.3), we have to impose the additional hypothesis:
∫Ω(S(x)+I(x))dx=N. | (1.5) |
Obviously, system (1.4)–(1.5) always has a solution E0=(N/|Ω|,0), which is the uniquedisease-free equilibrium (DFE). On the other hand, a nonnegative solution E1=(S,I) of (1.4)–(1.5) with I(x)≥0,≢0 is called an endemic equilibrium (EE) of (1.4)–(1.5).
Similar to [1,7], let us define the basic reproduction number R0 for (1.2) as follows:
R0:=sup{N∫Ωβφ2dx|Ω|∫Ω(dI|∇φ|2+γφ2)dx:φ∈H1(Ω) and φ≠0}, |
where H1(Ω)={u:u∈L2(Ω),Du∈L2(Ω)}. Denote the high-risk set and low-risk set respectively by
Ω+:={x∈Ω:N|Ω|β(x)>γ(x)} |
and
Ω−:={x∈Ω:N|Ω|β(x)<γ(x)}. |
We say the domain Ω is a high-risk domain if N|Ω|∫Ωβ(x)dx≥∫Ωγ(x)dx and it is a low-risk domain if N|Ω|∫Ωβ(x)dx<∫Ωγ(x)dx.
We begin with some properties of R0 which is similar to Lemmas 2.2 and 2.3 in [1].
Proposition 1.1. The basic reproduction number R0 has the following properties.
(ⅰ) R0 is positive decreasing function of dI>0;
(ⅱ) R0→N|Ω|maxx∈ˉΩβ(x)γ(x) as dI→0+, and R0→N|Ω|∫Ωβ(x)dx∫Ωγ(x)dx as dI→∞;
(ⅲ) if Ω is a high-risk domain, then R0>1 for dI>0;
(ⅳ) if Ω is a low-risk domain with nonempty Ω+, then there exists d∗I>0 such that R0=1 when dI=d∗I, R0>1 when dI<d∗I, and R0<1 when dI>d∗I;
(ⅴ) R0>1 implies N|Ω|>minx∈ˉΩγ(x)β(x).
The first goal of this paper is to establish the existence of EE.
Theorem 1.2. The following statements hold.
(ⅰ) If dS≥dI, there exists a unique EE when R0>1 and EE does not exist when R0≤1;
(ⅱ) if dS<dI, there exists an EE when R0>1 and EE does not exist when R0≤dS/dI.
Theorem 1.2(ⅰ) indicates that R0=1 is the critical value for the existence of EE when dS≥dI. However, if dS<dI, we do not know whether an EE exists or not in the case of R0∈(dS/dI,1).
A combination of Proposition 1.1 and Theorem 1.2 implies that the EE always exists when Ω is a high-risk domain (see Figure 1(a)) or Ω is a low-risk domain with nonempty Ω+ and 0<dI<d∗I (see Figure 1(b)), where d∗I>0 is uniquely determined in Proposition 1.1(ⅳ).
The second goal of this paper is to investigate the effects of diffusion rates and saturation coefficient on asymptotic profiles of the EE when it exists. Here we consider the following three cases: (ⅰ) small diffusion, (ⅱ) large diffusion, (ⅲ) large saturation.
The following theorem presents the asymptotic profile of EE when dS is sufficiently small or large.
Theorem 1.3. Let dI and m be fixed. Assume R0>1 and (S(x),I(x)) is an EE of (1.2). Then the following statements hold.
(ⅰ) (S,I)→(S∗,I∗) in C2(ˉΩ) when dS→∞, where I∗ is the unique positive solution of the following problem
{−dIΔI=I[β1+mI(N|Ω|−1|Ω|∫ΩIdx)−γ],x∈Ω,∂I∂ν=0,x∈∂Ω | (1.6) |
and
S∗=N|Ω|−1|Ω|∫ΩI∗dx. |
(ⅱ) (S,I)→(S∗,I∗) in C(ˉΩ) when dS→0+, where S∗ and I∗ satisfy
(S∗,I∗)=(γβ|Ω|+mN|Ω|+m∫Ωγ/βdx,N−∫Ωγ/βdx|Ω|+m∫Ωγ/βdx), | (1.7) |
or I∗=0 and
S∗=N|Ω|+dI(1|Ω|∫ΩˇIdx−ˇI), | (1.8) |
where ˇI>0 satisfies the following problem
{dIΔI+I[β(N|Ω|+dI|Ω|∫ΩIdx−dII)−γ]=0,x∈Ω,∂I∂ν=0,x∈∂Ω. | (1.9) |
Corollary 1.4. Suppose that R0>1 and N<∫Ωγ/βdx. Then, for any fixed dI>0 and m>0, the EE (S,I)→(S∗,0) in C(ˉΩ) as dS→0+, where S∗ satisfies (1.8).
Corollary 1.5. Fixed dI>0 and m>0. Suppose R0>1 and N>∫Ωγ/βdx. Then the EE (S,I)→(S∗,I∗) in C(ˉΩ) as dS→0+, where (S∗,I∗) satisfies (1.7) if one of the following conditions holds:
(ⅰ) β is a positive constant;
(ⅱ) N|Ω|>maxx∈ˉΩγ(x)β(x);
(ⅲ) γ(x)β(x)=r for any x∈ˉΩ, where r is some positive constant.
Corollary 1.5 implies that the infectious disease may persist even if the movement of the susceptible population is controlled to be very small, which is in sharp contrast to the epidemic model in [1].
Next, we are going to explore the asymptotic profile of EE as dI→0+ and dI/dS→d>0.
Theorem 1.6. Let m be fixed. Assume that Ω+ is nonempty. Then the following statements hold:
(ⅰ) If dI→0+ and dI/dS→d∈(0,∞), then (S,I)→(S∗,I∗) in C(ˉΩ), where I∗ is the unique positive solution of
(dβ(x)+mγ(x))I∗=[β(x)(N|Ω|−1−d|Ω|∫ΩI∗dx)−γ(x)]+ | (1.10) |
and S∗ is given by
S∗=N|Ω|−1−d|Ω|∫ΩI∗dx−dI∗. |
(ⅱ) If d∈(0,1), then {x∈Ω:I∗>0}⫋Ω+; if d∈(1,∞), then {x∈Ω:I∗>0}⫆Ω+ and this inclusion is strict if Ω− is nonempty.
Remark 1.7. If d=1 in Theorem 1.6, then
S∗=N|Ω|−I∗, I∗=1β+mγ(βN|Ω|−γ)+, |
which implies that {x∈Ω:I∗>0}=Ω+. It follows from Theorem 1.6(ii) that, in this situation, the ratio dI/dS plays a critical role in determining the existing region of the infected population. If d=1, the infected individuals survive exactly in the high-risk set; if d∈(0,1), the habitat of infected individual is confined within some subset of the high-risk set; if d>1, the infected individuals only die out at part of the low-risk sites.
We now establish the asymptotic profile when the diffusion rate dI is large.
Theorem 1.8. Let m be fixed. Suppose that Ω is a high-risk domain. Then the following statements hold.
(ⅰ) If dI→∞ and dS→∞, then (S,I)→(S∗,I∗) in C2(ˉΩ), where S∗ and I∗ are positive constants satisfying
S∗=∫Ωγdx∫Ωβdx(1+mN∫Ωβdx−|Ω|∫Ωγdx|Ω|(∫Ωβdx+m∫Ωγdx)), I∗=N∫Ωβdx−|Ω|∫Ωγdx|Ω|(∫Ωβdx+m∫Ωγdx). |
(ⅱ) If dS is fixed, then there exists a sequence {dIn} with dIn→∞ as n→∞ such that the corresponding EE (Sn,In)→(S∗,I∗) in C2(ˉΩ), where I∗ is a positive constant and S∗ is the positive solution of the following problem
{−dSΔS=−βI∗1+mI∗S+γI∗,x∈Ω,∂S∂ν=0,x∈∂Ω,∫ΩSdx=N−|Ω|I∗. | (1.11) |
Furthermore, if dS→0+ in (1.11), then (S∗,I∗)→(ˆS∗,ˆI∗) in C1(ˉΩ), where ˆS∗ and ˆI∗ satisfy (1.7) or ˆI∗=0 and ˆS∗ satisfies
{−ΔS=1K1(−βS+γ),x∈Ω,∂S∂ν=0,x∈∂Ω,∫ΩSdx=N | (1.12) |
with K1 being a positive constant.
Finally, we describe the asymptotic profile when the saturated coefficient m is large.
Theorem 1.9. Suppose that R0>1. Then for any fixed dI>0 and dS>0, the corresponding EE (S,I) of (1.2) satisfies (S,I)→(N/|Ω|,0) in C(ˉΩ) as m→∞. Furthermore, either ‖mI‖∞→∞ or mI→w∗ as m→∞, where w∗ is the unique positive solution of the following problem
{−dIΔw=w(βN|Ω|(1+w)−γ),x∈Ω,∂w∂ν=0,x∈∂Ω. | (1.13) |
Theorem 1.9 implies that large saturated coefficient can help to eliminate the disease. That is, if the susceptible individuals change the behavior when their number increases or the infective individuals produce crowding effect, the infectious disease may extinct eventually.
The rest of this paper is arranged as follows. In Section 2, we focus on the existence, uniqueness and nonexistence of the EE, and give the proof of Theorem 1.2. In Section 3, the impacts of diffusion rates and saturated coefficient on the persistence and extinction of the infectious disease are studied. Then, we illustrate the influences of the population diffusion and the saturation coefficient on system (1.2) numerically in Section 4. In Section 5, we conclude the paper with some discussion of the epidemiological implication of our theoretical results. Finally, some well-known facts, which are frequently used in the proofs of our main results, are collected in the appendix.
Since the existence of the EE is related to the stability of the DFE, we first investigate the stability of the DFE. To this end, we linearize (1.2) around the DFE to obtain
{ηt=dSΔη−(N|Ω|β−γ)ξ,x∈Ω,t>0,ξt=dIΔξ+(N|Ω|β−γ)ξ,x∈Ω,t>0. |
Here η(x,t)=¯S(x,t)−N/|Ω| and ξ(x,t)=¯I(x,t). Let (η(x,t),ξ(x,t))=(e−λtϕ(x),e−λtψ(x)) be the solution of the linear system. Then, we derive an eigenvalue problem
{dSΔϕ−(N|Ω|β−γ)ψ+λϕ=0,x∈Ω,dIΔψ+(N|Ω|β−γ)ψ+λψ=0,x∈Ω | (2.1) |
with boundary conditions
∂ϕ∂ν=∂ψ∂ν=0, x∈∂Ω. | (2.2) |
In view of (1.3), we impose an additional condition
∫Ω(ϕ+ψ)dx=0. | (2.3) |
Indeed, it suffices to consider the eigenvalue problem
{dIΔψ+(N|Ω|β−γ)ψ+λψ=0,x∈Ω,∂ψ∂ν=0,x∈∂Ω. | (2.4) |
It is well known that all eigenvalues of (2.4) are real, and the principal eigenvalue, denoted by λ∗, is simple, and its corresponding eigenfunction ψ∗ can be chosen positive on Ω. Furthermore, the eigenvalue λ∗ is given by the variational characterization
λ∗=inf{∫Ω[dI|∇φ|2+(γ−N|Ω|β)φ2]dx:φ∈H1(Ω) and ∫Ωφ2dx=1}. |
It has been shown in [7] that the basic reproduction number R0 and the principal eigenvalue λ∗ has the following relationship.
Lemma 2.1. 1−R0 and λ∗ have the same sign.
As discussed in Lemma 2.4 of [1], the stability of the DFE depends on the value of R0.
Lemma 2.2. The DFE is linearly stable if R0<1 and unstable if R0>1.
To study the existence of the EE, we first convert problem (1.4)-(1.5) to an equivalent but more accessible problem.
Lemma 2.3. The pair (S,I) is a nonnegative solution of problem (1.4)-(1.5) if and only if I is a nonnegative solution of the following problem
{dIΔI+I[β1+mI(N|Ω|−(1−dIdS)1|Ω|∫ΩIdx−dIdSI)−γ]=0,x∈Ω,∂I∂ν=0,x∈∂Ω | (2.5) |
and
S=N|Ω|−(1−dIdS)1|Ω|∫ΩIdx−dIdSI, x∈Ω. | (2.6) |
Proof. By standard calculations, one can easily check that (S,I) is a nonnegative solution of problem (1.4)-(1.5) if and only if it solves the following problem:
dSS+dII=κ, x∈Ω, | (2.7) |
dIΔI+β(x)SI1+mI−γ(x)I=0, x∈Ω, | (2.8) |
∂S∂ν=∂I∂ν=0, x∈∂Ω, | (2.9) |
∫Ω(S+I)dx=N, | (2.10) |
where κ is some positive constant independent of x∈Ω.
Now we show the equivalence between problems (2.7)-(2.10) and (2.5)-(2.6).
Suppose that (S,I) is a nonnegative solution of (2.7)-(2.10). By (2.7), we have dS(S+I)=κ+(dS−dI)I. Integrating it over Ω and using (2.10), we get dSN=κ|Ω|+(dS−dI)∫ΩIdx. Substituting (2.7) into the equation gives
S=N|Ω|−(1−dIdS)1|Ω|∫ΩIdx−dIdSI, x∈Ω. |
That is, (2.6) holds. Substituting such S into (2.8), we get (2.5).
Suppose that (S,I) is a nonnegative solution of problem (2.5)-(2.6). Substituting (2.6) into (2.5) yields (2.8). Clearly, ∂S/∂ν=0, i.e., (2.9) holds. Integrating both sides of (2.6) over Ω, we get (2.10). Applying the Laplace operator to both sides of (2.6), we find that dSΔS=−dIΔI which means Δ(dSS+dII)=0. Since ∂∂ν(dSS+dII)=0, the maximum principle implies that dSS+dII is a constant. In view of (2.10), this constant must be positive, which yields (2.7).
The nonlocal elliptic problem (2.5) has the following estimate.
Lemma 2.4. If I∈C2(Ω)∩C1(ˉΩ) is a nonnegative solution of the nonlocal elliptic problem (2.5), then we have
(1−dIdS)1|Ω|∫ΩIdx+dIdSI≤N|Ω| for all x∈ˉΩ. | (2.11) |
Proof. It is easy to see that (2.11) holds if I≡0 on ˉΩ. Suppose I≥0,≢0. Then there exists some x0∈ˉΩ such that I(x0)=maxx∈ˉΩI(x)>0. By Lemma A.3, we have
β(x0)1+mI(x0)[N|Ω|−(1−dIdS)1|Ω|∫ΩIdx−dIdSI(x0)]−γ(x0)≥0, |
which implies that
N|Ω|−(1−dIdS)1|Ω|∫ΩIdx≥dIdSI(x0)+γ(x0)β(x0)≥dIdSI. | (2.12) |
The conclusion holds.
Set
S:=N|Ω|−(1−dIdS)1|Ω|∫ΩIdx−dIdSI, x∈ˉΩ. |
If follows from Lemma 2.4 that S is nonnegative. Hence the pair (S,I) solves problem (2.5)–(2.6) as well as (1.4)–(1.5). Next, we focus on the existence of positive solution to the nonlocal elliptic problem (2.5) that only involves I.
Let Γ={τ∈[0,∞):N−(1−dI/dS)τ≥0} and Y={z∈C2+α(ˉΩ):∂z/∂ν=0 on ∂Ω}. Define a mapping F:Γ×Y→Cα(ˉΩ) by
F(τ,I)=dIΔI+If(τ,I) |
with
f(τ,I)=β|Ω|(1+mI)[N−(1−dIdS)τ−dI|Ω|dSI]−γ. |
Then I is a nonnegative solution of (2.5) if and only if F(τ,I)=0 and τ=∫ΩIdx.
Now we consider the following problem:
{dIΔI+If(τ,I)=0,x∈Ω,∂I∂ν=0,x∈∂Ω. | (2.13) |
It is easy to check that (2.13) meets all the requirements of Lemma A.2. Thus the existence of positive solution of (2.13) is tightly related to an eigenvalue λ1(dI,f(τ,0)), which is defined by (8.1). For simplicity, we denote λτ=λ1(dI,f(τ,0)), and hence λ0=λ∗, where λ∗ is the principal eigenvalue of (2.4).
Lemma 2.5. Suppose that τ≥0.
(ⅰ) If λτ≥0, the only nonnegative solution of (2.13) is I=0;
(ⅱ) if λτ<0, there is a unique positive solution I∈Y of (2.13).
We are now ready to prove Theorem 1.2. To this end, we need to prove several lemmas as follows.
Lemma 2.6. Suppose λ∗<0.
(ⅰ) If dS>dI, then there exists a smooth curve (τ,Iτ(x)) in Γ×Y such that F(τ,Iτ)=0. Moreover, there is a Λ>0 such that IΛ=0 and Iτ(x)>0 for all x∈ˉΩ, τ∈[0,Λ). Furthermore, Iτ is decreasing and continuously differentiable with respect to τ in (0,Λ);
(ⅱ) if dS<dI, then there exists a smooth curve (τ,Iτ(x)) in [0,∞)×Y such that F(τ,Iτ)=0 with Iτ>0 for x∈ˉΩ and τ∈[0,∞). Moreover, Iτ(x) is increasing and continuously differentiable in τ on (0,∞), and it satisfies the following estimate:
∫ΩIτ(x)dx≤dSdIN+(1−dSdI)τ. | (2.14) |
Proof. (ⅰ) Suppose that (τ0,Iτ0(x))∈Γ×Y satisfies F(τ0,Iτ0)=0 and Iτ0(x)>0 on ˉΩ. The Freˊchet derivative of F is given by
F_I(\tau_0, I_{\tau_0})w = d_I\Delta w + \left[f(\tau_0, I_{\tau_0})+f_I(\tau_0, I_{\tau_0})I_{\tau_0}\right]w |
for all w\in Y , where
f_I(\tau_0, I_{\tau_0}) = -\frac{\beta}{|\Omega|(1+mI_{\tau_0})^2} \left\{\frac{d_I|\Omega|}{d_S}+m\left[N-\left(1-\frac{d_I}{d_S}\right)\tau_0\right]\right\} \lt 0. |
We claim that F_I(\tau_0, I_{\tau_0}) is invertible. To this end, we need to show the unique solvability of the following problem for any h\in C^\alpha(\bar{\Omega}) ,
\begin{equation} \begin{cases} d_I\Delta w + \left[f(\tau_0, I_{\tau_0})+f_I(\tau_0, I_{\tau_0})I_{\tau_0} \right]w = h, &x\in\Omega, \\ \frac{\partial w}{\partial \nu} = 0, & x\in\partial\Omega. \end{cases} \end{equation} | (2.15) |
Since F(\tau_0, I_{\tau_0}) = 0 , i.e. d_I\Delta I_{\tau_0} + I_{\tau_0}f(\tau_0, I_{\tau_0}) = 0 , we see that \lambda_1(d_I, f(\tau_0, I_{\tau_0})) = 0 and I_{\tau_0} is a corresponding eigenvector by (8.1). It follows from Lemma A.1 and f_I(\tau_0, I_{\tau_0}) < 0 that \lambda_1\left(d_I, f(\tau_0, I_{\tau_0})+f_I(\tau_0, I_{\tau_0})I_{\tau_0} \right) > \lambda_1(d_I, f(\tau_0, I_{\tau_0})) = 0 . So all eigenvalues of the problem
\begin{equation} \begin{cases} d_I\Delta \varphi +\left[f(\tau_0, I_{\tau_0})+f_I(\tau_0, I_{\tau_0})I_{\tau_0}\right]\varphi+\lambda\varphi = 0, &x\in\Omega, \\ \frac{\partial \varphi}{\partial \nu} = 0, & x\in\partial\Omega \end{cases} \end{equation} | (2.16) |
are positive. By the Fredholm alternative, (2.15) has a unique solution for every h\in C^\alpha(\bar{\Omega}) . The continuity of the unique solution follows from the classical Schauder estimate. Since \lambda_0 = \lambda^* < 0 , there exists a unique positive I_0\in Y such that F(0, I_0) = 0 by Lemma 2.5. Hence, by the implicit function theorem, there is a unique I_\tau \in Y such that F(\tau, I_\tau) = 0 for \tau \in [0, \hat{\tau}) with \hat{\tau} > 0 , and I_\tau is continuously differentiable with respect to \tau .
Now we show that I_\tau is decreasing with respect to \tau . Suppose that 0 < \tau_1 < \tau_2 < \hat{\tau} . Since d_S > d_I , we have that F(\tau_1, I_{\tau_2}) > F(\tau_2, I_{\tau_2}) = 0 . Hence, I_{\tau_2} is a lower solution of the equation F(\tau_1, I) = 0 . On the other hand, we choose a sufficiently large number as an upper solution of the equation F(\tau_1, I) = 0 . Then, by the method of upper/lower solutions and the uniqueness of the positive solution of F(\tau_1, I) = 0 , we obtain that I_{\tau_1} > I_{\tau_2} .
The curve (\tau, I_\tau) satisfying F(\tau, I_\tau) = 0 will continue as long as I_\tau > 0 , i.e., \lambda_\tau < 0 , due to Lemma 2.5. By the variational formula, \lambda_\tau is increasing with respect to \tau and \lambda_\tau > 0 for large \tau . It follows from Lemma 2.5 again that, there is no positive solution of F(\tau, I) = 0 if \tau is large, i.e., I_\tau = 0 for large \tau . Let [0, \Lambda) be the maximal interval of existence of \tau such that I_\tau > 0 . Then I_\Lambda = 0 .
(ⅱ) The existence and continuous differentiability of the curve (\tau, I_\tau) can be obtained by a similar argument as in the proof of (ⅰ). And one can check that I_\tau is increasing with respect to \tau since d_S < d_I . Thus the curve is continuous with respect to \tau on [0, \infty) .
To show (2.14), let I_\tau(y_0) = \max\limits_{\bar{\Omega}}I_\tau(x) . Applying Lemma A.3 to the first equation of (2.13), we obtain that
\begin{equation*} f(\tau, I_\tau(y_0)) = \frac{\beta(y_0)} {(1+mI_\tau(y_0))}\left[\frac{N}{|\Omega|}-\left(1-\frac{d_I}{d_S}\right)\frac{\tau}{|\Omega|}-\frac{d_I}{d_S}I_\tau(y_0)\right]-\gamma(y_0)\geq0, \end{equation*} |
which implies
\begin{equation*} \frac{\beta(y_0)}{(1+mI_\tau(y_0))}\left[\frac{N}{|\Omega|}-\left(1-\frac{d_I}{d_S}\right)\frac{\tau}{|\Omega|}-\frac{d_I}{d_S}I_\tau(y_0)\right]\geq0, \end{equation*} |
and hence
\begin{equation*} \label{estimate-I-ds \lt dI} I_\tau(x) \leq I_\tau(y_0)\leq\frac{d_SN}{d_I|\Omega|}+\left(1-\frac{d_S}{d_I}\right)\frac{\tau}{|\Omega|} \end{equation*} |
for any x\in \bar{\Omega} . It follows that (2.14) holds by integrating the above inequality over \Omega .
Lemma 2.7. Suppose \mathcal {R}_0 > 1 . Then there exists a unique EE if d_S\geq d_I , and there exists at least one EE if d_S < d_I .
Proof. If d_S = d_I , then \lambda_\tau = \lambda^* < 0 based on \mathcal {R}_0 > 1 . The result follows directly from Lemma 2.5.
For d_S > d_I , by Lemma 2.6 (ⅰ), there is a smooth curve (\tau, I_\tau) satisfying F(\tau, I_\tau) = 0 . By the definition of F , I_\tau is a solution of problem (2.5) if \tau = \int_\Omega I_\tau dx . Let H(\tau) = \int_\Omega I_\tau dx-\tau . Then H(\tau) is continuous and strictly decreasing with respect to \tau in [0, \Lambda) because of the continuity and monotonicity of I_\tau . Since \int_\Omega I_0dx > 0\ \text{and} \ 0 = \int_\Omega I_\Lambda dx < \Lambda , we have H(0) > 0, \ H(\Lambda) < 0 . Then, there exists a unique \tau_0 \in (0, \Lambda) such that H(\tau_0) = 0 , i.e. \tau_0 = \int_\Omega I_{\tau_0}dx . Hence problem (2.5) has a unique positive solution.
For d_S < d_I , by Lemma 2.6 (ⅱ), there exists a smooth curve (\tau, I_\tau) satisfying F(\tau, I_\tau) = 0 . We also take H(\tau) = \int_\Omega I_\tau dx-\tau . Then it is continuous with respect to \tau . The estimate (2.14) implies that H(\tau)\leq \frac{d_S}{d_I}(N-\tau) . Since H(0) > 0 and H(\tau) < 0 with \tau > N , there exists a \tau_0 > 0 such that H(\tau_0) = 0 , i.e. \tau_0 = \int_\Omega I_{\tau_0}dx . Hence, problem (2.5) has at least one positive solution.
Lemma 2.8. The EE does not exist if one of the following conditions holds:
(i) d_S\geq d_I and \mathcal {R}_0\leq1 ;
(ii) d_S < d_I and \mathcal {R}_0\leq d_S/d_I .
Proof. (ⅰ) The case d_S = d_I follows directly from Lemma 2.5. We analyze the case d_S > d_I indirectly. Assume that an EE (S^*, I^*) exists if \mathcal {R}_0 \leq1 . Then there is a \tau^* > 0 such that \tau^* = \int_\Omega I^* dx and F(\tau^*, I^*) = 0 . By Lemma 2.5, we know \lambda_{\tau^*} < 0 , which leads to \lambda^* = \lambda_0\leq\lambda_{\tau^*} < 0 since f(\tau, 0) is decreasing in \tau when d_S > d_I . Then \mathcal {R}_0 > 1 by Lemma 2.1, which is a contradiction.
(ⅱ) The case d_S < d_I . Assume to the contrary that an EE (S^*, I^*) exists when \mathcal {R}_0 \leq d_s/d_I . Let \tau^* = \int_\Omega I^* dx . Then I^* is also the positive solution of F(\tau^*, I^*) = 0 , and it satisfies (2.11) for all x\in\bar{\Omega} , i.e.
\left(1-\frac{d_I}{d_S}\right)\frac{ 1}{|\Omega|}\int_\Omega I^* dx+\frac{d_I}{d_S}I^*\leq \frac{ N}{|\Omega|}. |
Integrating this inequality over \Omega , we get \tau^* = \int_\Omega I^* dx\leq N . Noting that \lambda_{\tau^*} < 0 by Lemma 2.5 and f(\tau, 0) is increasing in \tau provided d_S < d_I , Lemma A.1 implies that \lambda_N\leq\lambda_{\tau^*} < 0 , where \lambda_N is the principal eigenvalue of the following problem
\begin{equation*} \begin{cases} d_I \Delta \varphi + \left(\frac{d_IN}{d_S|\Omega|}\beta-\gamma\right)\varphi = \lambda\varphi, &x\in\Omega, \\ \frac{\partial \varphi}{\partial \nu} = 0, &x\in\partial\Omega. \end{cases} \end{equation*} |
Define
\mathcal {R}'_0: = \sup\left\{ \frac{d_IN\int_\Omega\beta\varphi^2dx}{d_S|\Omega|\int_\Omega(d_I |\nabla\varphi|^2+\gamma\varphi^2)dx}: \varphi\in H^1(\Omega) \ \text{and}\ \varphi\not = 0\right\}. |
Then \mathcal {R}'_0 > 1 if and only if \lambda_N < 0 , which can be obtained as the properties of \mathcal {R}_0 . Since \mathcal {R}'_0 = d_I\mathcal {R}_0/d_S and \lambda_N < 0 , we have \mathcal {R}_0 > d_S/d_I , which is a contradiction.
Proof of Theorem 1.2. Theorem 1.2 follows from Lemmas 2.6, 2.7 and 2.8.
The goal of this section is to investigate the asymptotic profile of EE. To this end, we always assume \mathcal {R}_0 > 1 , so that (1.2) has an EE. As a first step, we establish the priori estimates of any EE.
Lemma 3.1. Assume that (S, I) is a nonnegative solution of (1.4)-(1.5). Then
\begin{equation} I\leq\left(1+\frac{d_S}{d_I}\right)\frac{ N}{|\Omega|}, \end{equation} | (3.1) |
\begin{equation} \min\limits_{x\in\bar{\Omega}}\left\{\frac{ \gamma }{\beta}(1+mI)\right\}\leq S\leq \max\limits_{x\in\bar{\Omega}}\left\{\frac{ \gamma }{\beta}(1+mI)\right\}. \end{equation} | (3.2) |
Proof. By (1.5), we have \int_\Omega Idx\leq N . Applying the inequality (2.11), we get
\begin{equation*} I\leq\frac{d_S}{d_I}\left(\frac{ N}{|\Omega|}+\frac{d_I}{d_S}\frac{ 1}{|\Omega|}\int_\Omega Idx\right)\leq\left(1+\frac{d_S}{d_I}\right)\frac{ N}{|\Omega|}. \end{equation*} |
Let S(x_0) = \max\limits_{x\in\bar{\Omega}}S(x) , S (y_0) = \min\limits_{x\in\bar{\Omega}}S (x) . We apply Lemma A.3 to the first equation of (1.4) to obtain that
\begin{equation*} -\frac{ \beta(x_0) S (x_0)}{1+mI (x_0)}+\gamma(x_0)\geq0, \ \ -\frac{ \beta(y_0) S (y_0)}{1+mI (y_0)}+\gamma(y_0)\leq0, \end{equation*} |
which imply S (x_0)\leq\frac{ \gamma(x_0) }{\beta(x_0)}(1+mI (x_0)) and S (y_0)\geq\frac{ \gamma(y_0) }{\beta(y_0)}(1+mI (y_0)) . Hence (3.2) holds.
Lemma 3.2. Assume that (S, I) is an EE of (1.4)-(1.5). Then I and S are uniformly bounded in L^\infty(\bar{\Omega}) if d_S/d_I\rightarrow\infty .
Proof. Note that (S, I) satisfies (1.4)-(1.5) (or (2.5)-(2.6)). By (2.6), we have S\leq N/|\Omega| provided d_S/d_I\rightarrow\infty . Then, we are going to derive a priori estimate of I when d_S/d_I\rightarrow\infty by the Harnack inequality. Applying Lemma A.4 to the second equation of (1.4), we obtain that there is a positive constant C_0 such that \max\limits_{\overline{\Omega}} I\leq C_0\min\limits_{\overline{\Omega}} I . In view of N\geq \int_\Omega Idx\geq |\Omega|\min\limits_{\overline{\Omega}} I\geq|\Omega|\max\limits_{\overline{\Omega}} I/C_0 , we conclude that \|I\|_\infty\leq C_0N/|\Omega| .
Now, we are ready to investigate the asymptotic profiles of the EE when d_S is sufficiently small or large. To this end, we show the existence and uniqueness of the solution (1.6).
Lemma 3.3. Suppose \mathcal {R}_0 > 1 . Then (1.6) has a unique positive solution.
Proof. Since \mathcal {R}_0 > 1 is equivalent to \lambda_1(d_I, N\beta/|\Omega|-\gamma) = \lambda^* < 0 by Lemma 2.1. Taking
\begin{equation*} f(\tau, I) = \frac{\beta} {|\Omega|(1+mI)}(N-\tau)-\gamma \end{equation*} |
and by similar arguments as in Lemma 2.7, we get that (1.6) has a unique positive solution.
Proof of Theorem 1.3.. It follows from Theorem 1.2 that an EE (S, I) exists provided \mathcal {R}_0 > 1 for any d_S > 0 .
(ⅰ) We consider the asymptotic profile when d_S\rightarrow\infty . By Lemma 3.2, I and S are uniformly bounded in C(\bar{\Omega}) for fixed d_I > 0 and d_S\rightarrow\infty . Then using the elliptic estimate and the Sobolev embedding theorem for (1.4), there exists a sequence \{d_{S_n}\} with {d_{S_n}}\rightarrow\infty as n\rightarrow\infty such that the corresponding EE (S_n, I_n)\rightarrow(S^*, I^*) in C^2(\bar{\Omega}) . Letting n\rightarrow\infty in (2.5), we get that I^* satisfies (1.6) which has a unique positive solution by Lemma 3.3. Thus, the strong maximum principle implies that there are two possibilities: I^* > 0 or I^*{\equiv}0 . By (2.5) and the positivity of I_n , we have
\begin{equation} \lambda_1\left(d_I, \frac{\beta} {1+mI_n}\left(\frac{ N}{|\Omega|} -\left(1-\frac{d_I}{d_{S_n}}\right)\frac{ 1}{|\Omega|}\int_\Omega I_n dx-\frac{d_I}{d_{S_n}}I_n\right)-\gamma\right) = 0. \end{equation} | (3.3) |
If I^*{\equiv}0 , letting n\rightarrow\infty in (3.3), we have \lambda_1(d_I, N\beta/|\Omega|-\gamma) = 0 , i.e. \lambda^* = 0 , which contradicts \mathcal {R}_0 > 1 by Lemma 1. Hence I^* is the positive solution of (1.6). By (1.4), S^* satisfies
\begin{equation} \begin{cases} \Delta S^* = 0, & x\in\Omega, \\ \frac{\partial S^*}{\partial \nu} = 0, & x\in\partial\Omega. \end{cases} \end{equation} | (3.4) |
The strong maximum principle implies that S^* is a constant. Furthermore, it follows from (1.5) that
S^* = \frac{ N}{|\Omega|}-\frac{ 1}{|\Omega|}\int_\Omega I^* dx. |
(ⅱ) We analyze the asymptotic profile when d_S\rightarrow0^+ . It follows from (3.1) that I is uniformly bounded for fixed d_I and small d_S . Hence there exists a sequence \{d_{S_n}\} with d_{S_n}\rightarrow0^+ as n \rightarrow\infty such that the corresponding EE (S_n, I_n) satisfies
\int_\Omega I_n dx\rightarrow K \ \text{for some}\ K\geq0. |
It then follows that
F_n: = \beta\left(\frac{N}{|\Omega|}d_{S_n}-(d_{S_n}-d_I)\frac{1}{|\Omega|}\int_\Omega I_n dx\right)\rightarrow \frac{d_I\beta K}{|\Omega|}\ \text{as}\ n \rightarrow\infty. |
Hence, for any \epsilon > 0 , there exists n_1 > 0 such that for n\geq n_1 ,
\begin{equation} \frac{d_I\beta}{|\Omega|}(K-\epsilon)\leq F_n \leq \frac{d_I\beta}{|\Omega|}(K+\epsilon)\ \text{and}\ 0 \lt d_{S_n}\leq\min\left\{\frac{\min_{x\in \overline{\Omega}}\beta(x)}{\max_{x\in \overline{\Omega}}\gamma(x)}\epsilon, d_I\right\}. \end{equation} | (3.5) |
We claim that
\begin{equation} I_n\rightarrow \frac{K}{|\Omega|}\ \text{uniformly on}\ \bar{\Omega}\ \text{as}\ n \rightarrow\infty. \end{equation} | (3.6) |
Noting that I_n satisfies (2.5), we rewrite it as
\begin{equation} \begin{cases} d_{S_n}d_I \Delta I_n + I_n\left(\frac{F_n-d_I\beta I_n}{1+mI_n}-d_{S_n}\gamma(x)\right) = 0, &x\in\Omega, \\ \frac{\partial I_n}{\partial \nu} = 0, &x\in\partial\Omega. \end{cases} \end{equation} | (3.7) |
It follows from (3.5) that I_n is a lower solution of the problem
\begin{equation} \begin{cases} d_{S_n}d_I \Delta I + I\left[\frac{d_I\beta}{|\Omega|}(K+\epsilon)-d_I\beta I\right] = 0, &x\in\Omega, \\ \frac{\partial I}{\partial \nu} = 0, &x\in\partial\Omega. \end{cases} \end{equation} | (3.8) |
On the other hand, (3.1) and (3.5) imply that
\begin{equation} I_n\leq \left(1+\frac{d_{S_n}}{d_I}\right)\frac{ N}{|\Omega|}\leq 2\frac{ N}{|\Omega|} \end{equation} | (3.9) |
for n\geq n_1 . Meanwhile, I_n is an upper solution of the problem
\begin{equation} \begin{cases} d_{S_n}d_I \Delta I + I\left(\frac{ d_I\beta(K-\epsilon)/|\Omega|-d_I\beta I}{1+2mN/|\Omega|}-\beta\epsilon\right) = 0, &x\in\Omega, \\ \frac{\partial I}{\partial \nu} = 0, &x\in\partial\Omega. \end{cases} \end{equation} | (3.10) |
Observing that I = \frac{K+\epsilon}{|\Omega|} is the unique positive solution of (3.8) and I = \frac{K-\epsilon}{|\Omega|}-\frac{\epsilon}{d_I}\left(1+\frac{2mN}{|\Omega|}\right) is the unique positive solution of (3.10), we have
\begin{equation} \frac{K-\epsilon}{|\Omega|}-\frac{\epsilon}{d_I}(1+2mN/|\Omega|)\leq I_n\leq\frac{K+\epsilon}{|\Omega|} \ \text{for all}\ n\geq n_1. \end{equation} | (3.11) |
Since \epsilon > 0 is arbitrary, (3.11) indeed implies that I_n\rightarrow \frac{K}{|\Omega|} uniformly on \bar{\Omega} as n \rightarrow\infty .
Now, we have two possibilities K > 0 or K = 0 . First, we consider the case K > 0 . Obviously, S_n satisfies
\begin{equation} \begin{cases} d_{S_n} \Delta S_n + \left(-\frac{ \beta S_n}{1+mI_n}+\gamma\right)I_n = 0, &x\in\Omega, \\ \frac{\partial S_n}{\partial \nu} = 0, &x\in\partial\Omega. \end{cases} \end{equation} | (3.12) |
By the fact that I_n\rightarrow \frac{K}{|\Omega|} and Lemma A.2, we can prove that
\begin{equation} S_n\rightarrow \frac{\gamma}{\beta}(1+mK/|\Omega|) \end{equation} | (3.13) |
uniformly on \bar{\Omega} as n \rightarrow\infty . Since (S_n, I_n) satisfies (1.5), letting n\rightarrow \infty, we have
\left(1+\frac{mK}{|\Omega|}\right)\int_\Omega \frac{\gamma}{\beta}dx+K = N, |
which implies that K = \frac{|\Omega|(N-\int_\Omega \gamma/\beta dx)}{|\Omega|+m\int_\Omega \gamma/\beta dx} when N > \int_\Omega \gamma/\beta dx . By (3.6) and (3.13), we know that (1.7) holds provided N > \int_\Omega \gamma/\beta dx .
For the case K = 0 , we have I_n\rightarrow 0 uniformly on \bar{\Omega} as n \rightarrow\infty . Passing to a subsequence if necessary, we then have either case (1) \|I_n\|_\infty/d_{S_n}\leq C with C\geq0 , or case (2) \|I_n\|_\infty/d_{S_n}\rightarrow\infty as n \rightarrow\infty .
If the case (1) occurs, then \int_\Omega I_n dx/d_{S_n}\leq \|I_n\|_\infty/d_{S_n}\leq C . Let \check{I}_n: = I_n/d_{S_n}. Then \|\check{I}_n\|_\infty\leq C and \check{I}_n satisfies
\begin{equation*} \begin{cases} -d_I \Delta \check{I}_n = \check{I}_n\left[\frac{\beta}{1+mI_n}\left(\frac{ N}{|\Omega|}-(d_{S_n}-d_I)\frac{ 1}{|\Omega|} \int_\Omega \check{I}_n dx-d_I\check{I}_n\right)-\gamma\right], &x\in\Omega, \\ \frac{\partial \check{I}_n}{\partial \nu} = 0, &x\in\partial\Omega. \end{cases} \end{equation*} |
Since the right hand of this equation is uniformly bounded in L^\infty(\Omega) , by standard elliptic regularity we know that \{\check{I}_n\} is precompact in C^1(\bar\Omega) . Hence by passing to a subsequence we may assume that \check{I}_n\rightarrow\check{I}\geq0 in C(\bar \Omega) . Moreover, \check{I} satisfies (1.9), i.e.
\begin{equation} \begin{cases} -d_I \Delta \check{I} = \check{I}\left[\beta\left(\frac{ N}{|\Omega|}+\frac{d_I}{|\Omega|}\int_\Omega \check{I} dx-d_I\check{I}\right)-\gamma\right], &x\in\Omega, \\ \frac{\partial \check{I}}{\partial \nu} = 0, &x\in\partial\Omega. \end{cases} \end{equation} | (3.14) |
We claim that \check{I} > 0 . To this end, we assume \check{I}\equiv0 on \bar{\Omega} , i.e, \check{I}_n\rightarrow 0 in C(\bar\Omega) . Let \tilde{I}_n = \check{I}_n/\|\check{I}_n\|_\infty. Then \|\tilde{I}_n\|_\infty = 1 and \tilde{I}_n satisfies
\begin{equation*} \begin{cases} -d_I \Delta \tilde{I}_n = \tilde{I}_n\left[\frac{\beta}{1+mI_n}\left( \frac{ N}{|\Omega|}-(d_{S_n}-d_I)\frac{ 1}{|\Omega|} \int_\Omega \check{I}_n dx-d_I\check{I}_n\right)-\gamma\right], &x\in\Omega, \\ \frac{\partial \tilde{I}_n}{\partial \nu} = 0, &x\in\partial\Omega. \end{cases} \end{equation*} |
Similarly, by passing to a subsequence we may assume that \tilde{I}_n\rightarrow\tilde{I} in C(\bar\Omega) . Furthermore, \|\tilde{I}_n\|_\infty = 1 and \tilde{I} satisfies
\begin{equation} \begin{cases} -d_I \Delta \tilde{I} = \tilde{I}(\beta\frac{ N}{|\Omega|}-\gamma), &x\in\Omega, \\ \frac{\partial \tilde{I}}{\partial \nu} = 0, &x\in\partial\Omega. \end{cases} \end{equation} | (3.15) |
It follows from the strong maximum principle that \tilde{I} > 0 on \bar{\Omega} . Hence, the definition of \mathcal {R}_0 and (3.15) implies that \mathcal {R}_0 = 1, which is a contradiction. Therefore, \check{I}\geq0, \not\equiv0 . By the strong maximum principle again, we get \check{I} > 0 on \bar{\Omega} . Hence, \int_\Omega I_n/d_{S_n}dx\rightarrow \int_\Omega\check{I}dx > 0 in C(\bar\Omega) as n\rightarrow\infty . However, if \|I_n\|_\infty/d_{S_n}\rightarrow0 , then \int_\Omega I_n/d_{S_n}dx\rightarrow 0 . It's a contradiction. Therefore, it remains \|I_n\|_\infty/d_{S_n}\rightarrow C_1 with some C_1 > 0 . In this case, I_n\rightarrow 0 in C(\bar\Omega) and by passing to a subsequence
S_n = \frac{ N}{|\Omega|}-(d_S-d_I)\frac{ 1}{|\Omega|}\int_\Omega \check{I}_n dx-d_I\check{I}_n\rightarrow \frac{ N}{|\Omega|}+\frac{ d_I}{|\Omega|}\int_\Omega \check{I} dx-d_I\check{I} \;\;\text{in} \;\; C^1 |
as n\rightarrow\infty , i.e. (1.8) holds.
If case (2) occurs, i.e., \|I_n\|_\infty/d_{S_n}\rightarrow\infty . Recalling I_n\rightarrow0 uniformly on \bar{\Omega} as n\rightarrow\infty , we have \|I_n\|_\infty\rightarrow 0 . By Lemma 3.1, we know that S_n is uniformly bounded. Let \tilde{I}_n = I_n/\|I_n\|_\infty. Then \|\tilde{I}_n\|_\infty = 1 and \tilde{I}_n satisfies
\begin{equation} \begin{cases} -d_I \Delta \tilde{I}_n = \tilde{I}_n\left(\frac{\beta S_n}{1+mI_n}-\gamma\right), &x\in\Omega, \\ \frac{\partial \tilde{I}_n}{\partial \nu} = 0, &x\in\partial\Omega. \end{cases} \end{equation} | (3.16) |
By the standard elliptic estimates, \tilde{I}_n is uniformly bounded in C^1(\bar{\Omega}) for fixed d_I > 0 . So passing to a subsequence if necessary, we have \tilde{I}_n\rightarrow\tilde{I} in C(\bar\Omega) with \|\tilde{I}\|_\infty = 1 . By the Harnack inequality, there is a positive constant K_* independent of n such that
1 = \max\limits_{x\in\bar{\Omega}}\tilde{I}_n(x)\leq K_*\min\limits_{x\in\bar{\Omega}}\tilde{I}_n(x). |
Hence {\min _{x\in\bar{\Omega}}\tilde{I}}\geq 1/K_* > 0 , i.e. \tilde{I} is strictly positive. We now turn to consider the equation (3.12) for S_n . Dividing (3.12) by \|I_n\|_\infty , we have
\begin{equation*} \begin{cases} -\frac{d_{S_n}}{\|I_n\|_\infty} \Delta S_n = \left(-\frac{ \beta S_n}{1+mI_n}+\gamma\right)\frac{I_n}{\|I_n\|_\infty}, &x\in\Omega, \\ \frac{\partial S_n}{\partial \nu} = 0, &x\in\partial\Omega. \end{cases} \end{equation*} |
Since d_{S_n}/\|I_n\|_\infty \rightarrow 0^+ , I_n/\|I_n\|_\infty \rightarrow \tilde{I} and I_n\rightarrow0 , it follows from Lemma A.2 that
S_n \rightarrow \frac{\gamma}{\beta}\ \text{uniformly on}\ \bar{\Omega}\ \text{as}\ n \rightarrow\infty. |
Moreover by (1.5) and I_n\rightarrow0 , we get
\int_\Omega\frac{\gamma}{\beta}dx = N, |
which is a contradiction if \int_\Omega\gamma/\beta dx\not = N. But if \int_\Omega\gamma/\beta dx = N , (1.7) holds.
Proof of Corollary 1.4. From the proof of Theorem 1.3, we know that if \mathcal {R}_0 > 1 and N < \int_\Omega \gamma/\beta dx , then I_n\rightarrow 0 uniformly on \bar{\Omega} as n \rightarrow\infty and \|I_n\|_\infty/d_{S_n}\leq C with C\geq0 . In this case, S^* satisfies (1.8).
Proof of Corollary 1.5. From the proof of Theorem 1.3, we only need to rule out I^* = 0 .
(ⅰ) Suppose that \beta is a positive constant. Then N > \int_\Omega \gamma/\beta dx implies that \frac{N}{|\Omega|}\int_\Omega \beta dx > \int_\Omega \gamma dx , i.e., \Omega is a high-risk domain. By Proposition 1.1(ⅲ) and Theorem 1.2, we know that the EE (S, I) exists for all d_I > 0 and m > 0 . Multiplying both sides of the first equation of (2.5) by (1+mI)/I and integrating it over \Omega , we get
\begin{equation*} d_I\int_\Omega \frac{|\nabla I|^2}{I^2}dx+\int_\Omega \left\{\beta\left[\frac{ N}{|\Omega|}-\left(1-\frac{d_I}{d_S}\right)\frac{ 1}{|\Omega|}\int_\Omega Idx-\frac{d_I}{d_S}I\right]-\gamma(1+mI)\right\}dx = 0, \end{equation*} |
which implies that
\begin{equation*} \int_\Omega \left\{\beta\left[\frac{ N}{|\Omega|}-\left(1-\frac{d_I}{d_S}\right)\frac{ 1}{|\Omega|}\int_\Omega Idx-\frac{d_I}{d_S}I\right]-\gamma(1+mI)\right\}dx\leq 0. \end{equation*} |
Since \beta is a constant, it follows from the above inequality that
\begin{equation} N- \int_\Omega Idx- \int_\Omega \frac{\gamma}{\beta}(1+mI)dx\leq0. \end{equation} | (3.17) |
On the other hand, by Theorem 1.3, we know that (S, I)\rightarrow (S^*, I^*) in C(\bar{\Omega}) as d_{S}\rightarrow0 , where I^* is a nonnegative constant. Letting d_S\rightarrow 0^+ , the inequality (3.17) implies that
\begin{equation*} \left(|\Omega|+m\int_\Omega \frac{\gamma}{\beta}dx\right)I^* \geq N- \int_\Omega \frac{\gamma}{\beta}dx \gt 0. \end{equation*} |
Hence I^* > 0 , which indicates that (S^*, I^*) satisfies (1.7).
(ⅱ)-(ⅲ). From (3.6), we know I^* = K/|\Omega| . We need to prove K > 0 .
If K = 0 , there are three cases to consider: (1) \|I_n\|_\infty/d_{S_n}\rightarrow C_1 > 0 , (2) \|I_n\|_\infty/d_{S_n}\rightarrow 0 , and (3) \|I_n\|_\infty/d_{S_n}\rightarrow\infty . If N > \int_\Omega \gamma/\beta dx , from the proof of Theorem 1.3, the case (2) and (3) are excluded directly. It remains to consider case (1). In this case, I_n/d_{S_n}\rightarrow \check{I} > 0 , where \check{I} satisfies (1.9). Next, we show that (1.9) has no positive solution under the conditions (ⅱ) or (ⅲ), which will deduce a contradiction. Take
\begin{equation*} f_1(\tau, I) = \frac{\beta} {|\Omega|}(N+d_I\tau-d_I|\Omega|I)-\gamma, \ \ F_1(\tau, I) = d_I\Delta I + If_1(\tau, I), \end{equation*} |
and consider the problem
\begin{equation} \begin{cases} d_I \Delta I + If_1(\tau, I) = 0, &x\in\Omega, \\ \frac{\partial I}{\partial \nu} = 0, & x\in\partial\Omega. \end{cases} \end{equation} | (3.18) |
Repeating the arguments as in the proof of Theorem 1.2, we can prove that there exists a smooth curve (\tau, \tilde{I}_\tau(x)) in [0, \infty)\times Y such that F_1(\tau, \tilde{I}_\tau) = 0 with \tilde{I}_\tau > 0 for all x\in \bar{\Omega} and \tau\in [0, \infty) if \mathcal {R}_0 > 1 . Moreover, \tilde{I}_\tau(x) is strictly increasing and continuously differentiable with respect to \tau in (0, \infty) . It is easy to see that \tilde{I}_\tau is a positive solution of (1.9) if and only if \tau = \int_\Omega \tilde{I}_\tau dx .
Let x_\tau, y_\tau \in \bar\Omega satisfy \tilde{I}_\tau(x_\tau) = \min\limits_{x\in\bar\Omega}\tilde{I}_\tau(x) and \tilde{I}_\tau(y_\tau) = \max\limits_{x\in\bar\Omega}\tilde{I}_\tau(x) . Using Lemma A.3 to (3.18), it is easy to check that, for every \tau\in [0, \infty)
\begin{equation*} \frac{1}{d_I}\left[\frac{N}{|\Omega|}-\frac{\gamma(x_\tau)}{\beta(x_\tau)}\right]+\frac{\tau}{|\Omega|}\leq \tilde{I}_\tau\leq \frac{1}{d_I}\left[\frac{N}{|\Omega|}-\frac{\gamma(y_\tau)}{\beta(y_\tau)}\right]+\frac{\tau}{|\Omega|}. \end{equation*} |
Then for every \tau\in [0, \infty) , we have
\begin{equation} \frac{N}{|\Omega|}-\frac{\gamma(x_\tau)}{\beta(x_\tau)}\leq\frac{d_I}{|\Omega|}(\int_\Omega \tilde{I}_\tau dx-\tau)\leq \frac{N}{|\Omega|}-\frac{\gamma(y_\tau)}{\beta(y_\tau)}, \end{equation} | (3.19) |
which implies that
\begin{equation} \frac{N}{|\Omega|}-\max\limits_{x\in\bar\Omega}\frac{\gamma(x)}{\beta(x)}\leq\frac{d_I}{|\Omega|}(\int_\Omega \tilde{I}_\tau dx-\tau)\leq \frac{N}{|\Omega|}-\min\limits_{x\in\bar\Omega}\frac{\gamma(x)}{\beta(x)}. \end{equation} | (3.20) |
Note that \mathcal {R}_0 > 1 implies N/|\Omega| > \min\limits_{\bar\Omega}\gamma(x)/\beta(x) by Proposition 1.1(v). It follows from (3.20) that \tau\not = \int_\Omega I_\tau dx for every \tau\in [0, \infty) provided N/|\Omega| > \max\limits_{\bar\Omega}\gamma(x)/\beta(x) or \gamma(x)/\beta(x) = r . Hence (1.9) has no positive solution under the conditions (ⅱ) or (ⅲ). K = 0 is impossible and we complete the proof of Corollary 1.5.
Next, we will prove Theorem 1.6. To this end, we first give the following result.
Lemma 3.4. Assume that \Omega^+ is nonempty and d is a positive constant. Then the following equation
\begin{equation} (d\beta(x)+m\gamma(x))I = \left[\beta(x)\left(\frac{N}{|\Omega|}-\frac{1-d}{|\Omega|}\int_\Omega Idx\right)-\gamma(x)\right]^+ \end{equation} | (3.21) |
has a unique nonnegative solution.
Proof. It is easy to see that (3.21) has a unique nonnegative solution I = \frac{[\beta(x)N/|\Omega| -\gamma(x)]^+}{\beta+m\gamma} if d = 1 . Hence, we only consider d\in (0, 1)\cup(1, +\infty) below. Let
G_\tau: = \left[\beta(x)\left(\frac{N}{|\Omega|}-\frac{1-d}{|\Omega|}\tau\right)-\gamma(x)\right]^+\left/(d\beta(x)+m\gamma(x))\right.. |
If d\in(0, 1) , then \int_\Omega G_\tau dx is non-increasing in \tau for \tau\geq 0 and \int_\Omega G_\tau dx = 0 for sufficiently large \tau . Define
\tau_0: = \min\{\tau\geq0: \int_\Omega G_\tau dx = 0\}. |
Since \Omega^+ is nonempty, we conclude that \int_\Omega G_0 dx = \int_\Omega \frac{[\beta(x)N/|\Omega|-\gamma(x)]^+}{d\beta(x)+m\gamma(x)}dx > 0 and \int_\Omega G_\tau dx is decreasing with respect to \tau\in [0, \tau_0] . Hence, there exists a unique \tau^*\in (0, \tau_0) , such that \int_\Omega G_{\tau^*} dx = \tau^* , i.e., G_{\tau^*} is the unique nonnegative solution of (3.21).
If d > 1 , then \int_\Omega G_\tau dx is non-decreasing in \tau for \tau\geq 0 and \int_\Omega G_\tau dx\rightarrow\infty as \tau\rightarrow\infty . We notice that
\int_\Omega G_\tau dx\leq \frac{1}{d}\int_\Omega \left(\frac{N}{|\Omega|}-\frac{\gamma}{\beta}\right)^+dx+\left(1-\frac{1}{d}\right)\tau. |
Since the right hand side of the above inequality is linear in \tau with slope less than 1, there exists \tau^* > 0 such that \int_\Omega G_{\tau^*} dx = \tau^* , which implies that G_{\tau^*} is a solution of (3.21). On the other hand, since \int_\Omega G_\tau dx is concave up in \tau , \tau^* is the unique solution of \int_\Omega G_\tau dx = \tau . Hence, (3.21) has a unique nonnegative solution. The proof is complete.
Proof of Theorem 1.6. Since \Omega^+ is nonempty, the EE (S, I) exists if d_I is small by Proposition 1.1 and Theorem 1.2.
First, we prove the conclusion for the case d < 1 . We claim that \int_\Omega Idx\rightarrow \int_\Omega I^*dx as d_I\rightarrow0^+ and d_I/d_S\rightarrow d , where I^* is the unique solution of (3.21). Since \int_\Omega Idx \leq N , there exist two sequences \{d_{I_n}\} and \{d_{I_n}/d_{S_n}\} with d_{I_n}\rightarrow0^+ and d_{I_n}/d_{S_n} \rightarrow d as n\rightarrow\infty such that the corresponding EE (S_n, I_n) satisfies \int_\Omega I_ndx\rightarrow K_0\in[0, N] . Then, for any \epsilon > 0 , there exists n^* > 0 such that K_0-\epsilon < \int_\Omega I_ndx < K_0+\epsilon and d-\epsilon < d_{I_n}/d_{S_n} < d+\epsilon when n > n^* . Therefore, I_n is a lower solution of the problem
\begin{equation} \begin{cases} d_{I_n} \Delta I + I\left\{\frac{\beta}{1+mI}\left[ \frac{N}{|\Omega|}-\frac{1}{|\Omega|}(1-d-\epsilon)(K_0-\epsilon)-(d-\epsilon)I\right]-\gamma\right\} = 0, &x\in\Omega, \\ \frac{\partial I}{\partial \nu} = 0, &x\in\partial\Omega, \end{cases} \end{equation} | (3.22) |
and is an upper solution of the problem
\begin{equation} \begin{cases} d_{I_n} \Delta I + I\left\{\frac{\beta}{1+mI}\left[ \frac{N}{|\Omega|}-\frac{1}{|\Omega|}(1-d+\epsilon)(K_0+\epsilon)-(d+\epsilon)I\right]-\gamma\right\} = 0, &x\in\Omega, \\ \frac{\partial I}{\partial \nu} = 0, &x\in\partial\Omega \end{cases} \end{equation} | (3.23) |
for n > n^* . Denote the unique positive solutions of (3.22) and (3.23) by I_{n, -\epsilon} and I_{n, +\epsilon} respectively if they exist; otherwise, let I_{n, -\epsilon} = 0 ( I_{n, +\epsilon} = 0 ). Then, it follows from an upper-lower solution argument that I_{n, +\epsilon}\leq I_n\leq I_{n, -\epsilon} for n > n^* . Furthermore, by Lemma A.2, we have
\lim\limits_{n\rightarrow\infty}I_{n, \pm \epsilon} = I_{\pm \epsilon} |
in C(\bar{\Omega}), where
\begin{equation} I_{\pm \epsilon} = \left[\frac{1}{ \beta(d\pm\epsilon) +m\gamma}\left(\frac{\beta N}{|\Omega|}-\beta( 1-d\pm\epsilon)\frac{K_0\pm\epsilon}{|\Omega|}-\gamma\right)\right]^+. \end{equation} | (3.24) |
Since \epsilon > 0 is arbitrary, by Lemma 3.4 we obtain
K_0 = \lim\limits_{n\rightarrow\infty}\int_\Omega I_ndx = \int_\Omega\left[\frac{1}{\beta d +m\gamma}\left(\frac{\beta N}{|\Omega|}-\beta( 1-d)\frac{K_0}{|\Omega|}-\gamma\right)\right]^+dx = \int_\Omega I^*dx. |
Now, we show I\rightarrow I^* as d_I\rightarrow0^+ and d_I/d_S\rightarrow d . Substituting K_0 = \int_\Omega I^*dx into (3.24), we get that
I\rightarrow\left[\frac{1}{ \beta d +m\gamma}\left(\frac{\beta N}{|\Omega|}-\frac{\beta( 1-d)}{|\Omega|}\int_\Omega I^*dx-\gamma\right)\right]^+ = I^*, |
and hence
S = \frac{ N}{|\Omega|}-\left(1-\frac{d_I}{d_S}\right)\frac{ 1}{|\Omega|}\int_\Omega Idx-\frac{d_I}{d_S}I\rightarrow\frac{N}{|\Omega|}-\frac{1-d}{|\Omega|}\int_\Omega I^* dx-dI^* = S^* |
as d_I\rightarrow0^+ and d_I/d_S\rightarrow d .
The proof of the case d\geq1 is similar, so we omit it here. The conclusion in (ⅱ) is easily obtained from equation (3.21).
Proof of Theorem 1.8. Since \Omega is a high-risk domain, the EE (S, I) always exists for any d_S > 0 and d_I > 0 . By Lemmas 3.1 and 3.2, we know that I and S are uniformly bounded in L^\infty (\Omega) for any positive d_I and d_S .
(ⅰ) We consider the asymptotic profile of the EE when d_I\rightarrow \infty and d_S\rightarrow \infty . Note that \beta SI/(1+mI)-\gamma I is uniformly bounded in L^\infty (\Omega) . Applying the standard elliptic estimate arguments, we obtain that S and I are uniformly bounded in C^{2+\alpha}(\bar{\Omega}) for all \alpha\in (0, 1) , d_S, d_I\geq 1 . It then follows from the compactness of the embedding C^{2+\alpha}(\bar{\Omega})\hookrightarrow C^{2}(\bar{\Omega}) that there exist sequences \{d_{S_n}\}, \{d_{I_n}\} with {d_{S_n}}\rightarrow\infty, {d_{I_n}}\rightarrow\infty as n\rightarrow\infty such that the corresponding EE (S_n, I_n)\rightarrow(S^*, I^*) in C^{2}(\bar{\Omega}) , where (S^*, I^*) satisfies
\begin{equation*} \begin{cases} \Delta S^* = \Delta I^* = 0, &x\in\Omega, \\ \frac{\partial S^*}{\partial \nu} = \frac{\partial I^*}{\partial \nu} = 0, &x\in\partial\Omega. \end{cases} \end{equation*} |
By the strong maximum principle, S^* and I^* are constants. Let \tilde{I}_n = I_n/\|I_n\|_\infty . By (1.4), we have
\begin{equation} \begin{cases} -d_{I_n}\Delta \tilde{I}_n = \left(\frac{\beta S_n}{1+mI_n}-\gamma\right) \tilde{I}_n, &x\in\Omega, \\ \frac{\partial \tilde{I}_n}{\partial \nu} = 0, &x\in\partial\Omega. \end{cases} \end{equation} | (3.25) |
Since \|\tilde{I}_n\|_\infty = 1 and I_n, S_n are uniformly bounded, it follows from the elliptic estimate and the Sobolev embedding theorem that \tilde{I}_n is uniformly bounded in C^{2+\alpha}(\bar{\Omega}) . Passing to a subsequence if necessary, we have \tilde{I}_n\rightarrow \tilde{I} in C^{2}(\bar{\Omega}) , where \tilde{I} satisfies
\begin{equation*} \begin{cases} \Delta \tilde{I} = 0, &x\in\Omega, \\ \frac{\partial \tilde{I}}{\partial \nu} = 0, &x\in\partial\Omega. \end{cases} \end{equation*} |
Using the strong maximum principle again, \tilde{I} is a constant. And then \tilde{I}\equiv1 since \|\tilde{I}_n\|_\infty = 1 . Integrating both sides of the first equation in (3.25) over \Omega , we find
\int_\Omega\left(\frac{\beta S_n}{1+mI_n}-\gamma\right) \tilde{I}_ndx = 0. |
Letting n \rightarrow\infty , we have
\int_\Omega\left(\frac{\beta S^*}{1+mI^*}-\gamma\right)dx = 0. |
Noticing that \int_\Omega(S^*+I^*)dx = N and S^*, I^*, m are constants, we have
I^* = \frac{N\int_\Omega\beta dx-|\Omega|\int_\Omega\gamma dx}{|\Omega|\left(\int_\Omega\beta dx+m\int_\Omega\gamma dx\right)}, \ S^* = \frac{\int_\Omega\gamma dx}{\int_\Omega\beta dx}\left[1+m\frac{N\int_\Omega\beta dx-|\Omega|\int_\Omega\gamma dx}{|\Omega|\left(\int_\Omega\beta dx+m\int_\Omega\gamma dx\right)}\right]. |
(ⅱ) Now we consider the asymptotic profile of the EE when d_I\rightarrow\infty . The proof is similar to (ⅰ) and Theorem 1.3, so we sketch it in the following.
Suppose d_S is fixed. By the elliptic estimate, the Sobolev embedding theorem and the maximum principle, there exists a sequence \{d_{I_n}\} with {d_{I_n}}\rightarrow\infty as n\rightarrow\infty such that the corresponding EE (S_n, I_n)\rightarrow(S^*, I^*) in C^2(\bar{\Omega}) , where I^*\geq0 is a constant. If I^* = 0 , then S^* satisfies (3.4), which indicates that S^* is also a constant. As in the proof of (ⅰ), we also introduce \tilde{I}_n = I_n/\|I_n\|_\infty. Then we can prove \tilde{I}_n\rightarrow1 in C^2(\bar{\Omega}) as n\rightarrow\infty , which leads to
\int_\Omega(\beta S^*-\gamma)dx = 0, |
and so S^* = \int_\Omega\gamma dx /\int_\Omega\beta dx . On the other hand, one can see from (1.5) that S^* = N/|\Omega| when I^* = 0 . Hence, \frac{N}{|\Omega|}\int_\Omega\beta dx = \int_\Omega\gamma dx , which contradicts the assumption that \Omega is a high-risk domain. Therefore I^* is a positive constant, and S^* > 0 satisfies (1.11).
Furthermore, let d_{S}\rightarrow0^+ . There exists a sequence \{d_{S_n}\} with {d_{S_n}}\rightarrow0^+ as n\rightarrow\infty such that the corresponding solution (S_n^*, I_n^*) of (1.11) satisfies d_{S_n}/I^*_n\rightarrow0 , or d_{S_n}/I^*_n\rightarrow \infty , or d_{S_n}/I^*_n\rightarrow K_1 for some positive constant K_1 . If d_{S_n}/I^*_n\rightarrow0 , we have (S_n^*, I_n^*)\rightarrow(\tilde{S}^*, \tilde{I}^*) in C(\bar{\Omega}) . Rewrite the equation of { S_n^* } as
\begin{equation*} \begin{cases} \frac{d_{S_n} }{I^*_n}\Delta S_n^* + \left(-\frac{ \beta S_n^*}{1+mI^*_n}+\gamma\right) = 0, &x\in\Omega, \\ \frac{\partial S_n^*}{\partial \nu} = 0, &x\in\partial\Omega. \end{cases} \end{equation*} |
Letting n\rightarrow\infty , we obtain
\tilde{S}^* = \frac{\gamma}{\beta}(1+m\tilde{I}^*), \ \tilde{I}^* = \frac{N}{|\Omega|}-\frac{1}{|\Omega|}\int_\Omega \tilde{S}^*dx, |
which implies that (\tilde{S}^*, \tilde{I}^*) satisfies (1.7).
If d_{S_n}/I^*_n\rightarrow K_1 , by passing to a subsequence, we have (S_n^*, I_n^*)\rightarrow(\tilde{S}^*, 0) in C(\bar{\Omega}) as d_{S_n}\rightarrow 0^+ . Rewrite the equation of S_n^* as follow
\begin{equation} \begin{cases} -\Delta S_n^* = \left(-\frac{ \beta S_n^*}{1+mI^*_n}+\gamma\right)\frac{ I^*_n}{d_{S_n}}, &x\in\Omega, \\ \frac{\partial S_n^*}{\partial \nu} = 0, &x\in\partial\Omega. \end{cases} \end{equation} | (3.26) |
Letting n\rightarrow\infty , we obtain \tilde{S}^* satisfies (1.12).
If d_{S_n}/I^*_n\rightarrow\infty as d_{S_n}\rightarrow0^+ , by passing to a subsequence, we have (S_n^*, I_n^*)\rightarrow(\tilde{S}^*, 0) in C(\bar{\Omega}) and S_n^* satisfies (3.26). Letting n\rightarrow\infty , we know that \tilde{S}^* is a constant. By the last equation of (1.11), we actually have \tilde{S}^* = \frac{ N}{|\Omega|} . Integrating both sides of the first equation in (1.11), we get \int_\Omega\left[-\beta S_n^*/(1+mI_n^*)+\gamma\right]dx = 0 . Letting n\rightarrow\infty , we find \frac{N}{|\Omega|}\int_\Omega\beta dx = \int_\Omega\gamma dx , which contradicts the assumption that \Omega is a high-risk domain. Hence d_{S_n}/I^*_n\rightarrow\infty is impossible. The proof is complete.
Proof of Theorem 1.9. Since \mathcal {R}_0 is independent of m , the EE (S, I) exists for any m > 0 provided \mathcal {R}_0 > 1 by Theorem 1.2. It follows from (3.1) that I is uniformly bounded for fixed d_I > 0, d_S > 0 . Hence there exists a sequence \{m_n\} with m_n\rightarrow\infty as n \rightarrow\infty such that the corresponding EE (S_n, I_n) satisfies
\begin{equation} \begin{cases} -d_I \Delta I_n = I_n\left\{\frac{\beta} {1+m_nI_n}\left[\frac{ N}{|\Omega|}-\left(1-\frac{d_I}{d_S}\right)\frac{ 1}{|\Omega|}\int_\Omega I_ndx-\frac{d_I}{d_S}I_n\right]-\gamma\right\}, & x\in\Omega, \\ \frac{\partial I_n}{\partial \nu} = 0, &x\in\partial\Omega. \end{cases} \end{equation} | (3.27) |
Since the right hand of the above equation is uniformly bounded in L^\infty(\Omega) , by standard elliptic regularity we know that \{I_n\} is precompact in C^1(\Omega) . Hence by passing to a subsequence we may assume that I_n \rightarrow I_*\geq 0 in C(\bar\Omega) . There are two possibilities (a) \|m_nI_n\|_\infty\rightarrow\infty as n\rightarrow\infty or (b) \|m_nI_n\|_\infty\leq C , where C is a positive constant.
If (a) occurs, then I_* satisfies
\begin{equation} \begin{cases} -d_I \Delta I+\gamma I = 0, & x\in\Omega, \\ \frac{\partial I}{\partial \nu} = 0, &x\in\partial\Omega. \end{cases} \end{equation} | (3.28) |
The Fredholm alternative implies that I_* = 0 .
If (b) occurs, then I_n\rightarrow 0 uniformly on \bar{\Omega} as n\rightarrow\infty . Denote m_nI_n = w_n . Then w_n is uniformly bounded in L^\infty(\Omega) and w_n\rightarrow w_*\geq 0 in C(\bar\Omega) , where w_* satisfies
\begin{equation} \begin{cases} d_I \Delta w_*+w_*\left(\frac{\beta N}{|\Omega|(1+w_*)}-\gamma\right) = 0, & x\in\Omega, \\ \frac{\partial w_*}{\partial \nu} = 0, &x\in\partial\Omega. \end{cases} \end{equation} | (3.29) |
If w_*\equiv 0 , set \hat{w}_n = \frac{w_n}{\|w_n\|_\infty} . Then \hat{w}_n satisfies
\begin{equation} \begin{cases} -d_I \Delta \hat{w}_n = \hat{w}_n\left\{\frac{\beta} {1+w_n}\left[\frac{ N}{|\Omega|}-\left(1-\frac{d_I}{d_S}\right)\frac{ 1}{|\Omega|}\int_\Omega I_ndx-\frac{d_I}{d_S}I_n\right]-\gamma\right\}, & x\in\Omega, \\ \frac{\partial \hat{w}_n}{\partial \nu} = 0, &x\in\partial\Omega. \end{cases} \end{equation} | (3.30) |
By standard elliptic regularity we may assume that \hat{w}_n\rightarrow \hat{w} in C(\bar\Omega) . Then \hat{w}\geq0, \not\equiv 0 satisfies
\begin{equation} \begin{cases} d_I \Delta \hat{w}+\hat{w}\left(\frac{\beta N}{|\Omega|}-\gamma\right) = 0, & x\in\Omega, \\ \frac{\partial \hat{w}}{\partial \nu} = 0, &x\in\partial\Omega. \end{cases} \end{equation} | (3.31) |
It follows from the strong maximum principle that \hat{w} > 0 on \bar{\Omega} . So \lambda_1(d_I, \beta N/|\Omega|-\gamma) = \lambda^* = 0 , which implies \mathcal {R}_0 = 1 . This is a contradiction to the assumption \mathcal {R}_0 > 1 . Hence w_*\geq0, \not\equiv 0 in \bar{\Omega} . Using the strong maximum principle again, we know that w_* > 0 on \bar{\Omega} . It follows from Lemma A.2 that (3.29) has a unique positive solution w_* as \lambda_1(d_I, \beta N/|\Omega|-\gamma) = \lambda^* < 0 .
To illustrate the influences of the population diffusion and the saturation coefficient on system (1.2), we suppose the spatial domain \Omega = [0, 1] . Let \beta(x) = \sin(2\pi x)+a, \gamma(x) = \cos(2\pi x)+b , where a > 1, b > 1. Take S(x, 0) = \sin(\pi x)+1, I(x, 0) = \sin(\pi x)+1 , a = 1.5 , b = 4.5 . Then N = \int_\Omega(S(x, 0)+I(x, 0))dx = 3.2731 satisfies
\begin{equation} 3 = \frac{\int_\Omega \gamma(x)dx}{\int_\Omega \beta(x)dx} \lt \frac{N}{|\Omega|} \lt \frac{1}{|\Omega|}\int_\Omega \frac{\gamma(x)}{\beta(x)}dx \lt \max\limits_\Omega \frac{\gamma(x)}{\beta(x)} = 9.1094, \end{equation} | (4.1) |
where \frac{1}{|\Omega|}\int_\Omega \frac{\gamma(x)}{\beta(x)}dx = 4.0249 . Hence \Omega^+ , \Omega^- are nonempty, and \Omega is a high-risk domain which implies that \mathcal {R}_0 > 1 by Proposition 1.1 (ⅲ).
(ⅰ) Firstly, we explore the influence of d_S on system (1.2). Fix d_I = 0.5 and m = 2 . A numerical positive equilibrium solution (S(x), I(x)) to (1.2) was computed in Figure 2(1) with d_S = 0.1 . When d_S is large, we find that S(x) tends to a constant and I(x) closely approximates the unique positive solution of (1.6) (see Figure 2(2) with d_S = 10^3 ), which is consistent with Theorem 1.3 (ⅰ). As d_S decreases, the value of I(x) decreases too. In Figure 2(3), I(x) closely approximates zero with d_S = 10^{-6} , which coincides with the results of Theorem 1.3(ⅱ) and Corollary 1.4.
If we take a = 1.5 and b = 1.2 , then 3.2731 = \frac{N}{|\Omega|} > \max\limits_{x\in\bar\Omega}\frac{\gamma(x)}{\beta(x)} = 2.7514 , which implies that \mathcal {R}_0 > 1 by Proposition 1.1(ⅰ) and (ⅱ). By taking d_I = 0.5 and d_S = 10^{-6} , we find that I(x) closely approximates a positive constant (see Figure 2(4)), which coincides with the results of Theorem 1.3 (ⅱ) and Corollary 1.5.
(ⅱ) Secondly, we analyze the asymptotic profile of the equilibrium solution (S(x), I(x)) to system (1.2) when d_S and d_I are small. Fix a = 1.5 , b = 4.5 and m = 2 . Then \Omega^+ and \Omega^- are nonempty from (4.1). By taking d_S = 10^{-4} and d_I = 0.2\times 10^{-4} , we find that (0.0470, 0.5590)\approx\{x\in \Omega: I^* > 0\}\subsetneqq\Omega^+ = (0.0281, 0.5663), which coincides with the result of Theorem 1.6 with d < 1 (see Figure 3(1)–(2)). By taking {d_S} = 10^{-4} and d_I = 2\times 10^{-4} , we find that [0, 0.6954) = \{x\in \Omega: I^* > 0\}\supseteqq\Omega^+ = (0.0281, 0.5663), which coincides with the result of Theorem 1.6 with d > 1 (see Figure 3(1), (3)).
(ⅲ) Thirdly, we explore the asymptotic profile of the equilibrium solution (S(x), I(x)) to system (1.2) when d_I is large. Fix a = 1.5 , b = 4.5 and m = 2 . Then \Omega is a high-risk domain from (4.1). A numerical positive equilibrium solution (S(x), I(x)) to (1.2) was computed (see Figure 4). In Figure 4(1), by taking d_S = d_I = 10^4 , we find that (S(x), I(x)) closely approximates a constant equilibrium solution, which coincides with the result of Theorem 1.8(ⅰ). In Figure 4(2), by taking d_S = 0.1 and d_I = 10^{4} , we find that I(x) closely approximates a positive constant, which coincides with the result of Theorem 1.8(ⅱ).
(ⅳ) Finally, we show the influence of saturation coefficient m on system (1.2). Fix a = 1.5 , b = 4.5 , d_S = 0.1 and d_I = 0.5 . Then \mathcal {R}_0 > 1 from (4.1). A numerical positive equilibrium solution (S(x), I(x)) to (1.2) was computed (see Figure 5). In Figure 5(1), by taking m = 2 , there is a positive equilibrium solution (S(x), I(x)) to (1.2). In Figure 5(2), by taking m = 10^3 , we find that (S(x), I(x)) closely approximates the DFE (N/|\Omega|, 0) , which coincides with the result of Theorem 1.9.
In this paper, we investigate an SIS reaction-diffusion population model (1.2) with saturated incidence rate \beta\bar{I}\bar{S}/(1+m\bar{I}) . We focus on the existence of EE and particularly the effects of the diffusion rates and the saturated coefficient on asymptotic profiles of EE. Similar questions are addressed for the model with the standard incidence rate \beta \bar{S}\bar{I}/(\bar{S}+\bar{I}) in [1,20] or the bilinear rate \beta\bar{S}\bar{I} in [7,16,25].
Firstly, by introducing the basic reproduction number \mathcal {R}_0 as in [1,7], we obtain for (1.2) that there is at least one EE if \mathcal {R}_0 > 1 , especially, EE exists uniquely when d_S\geq d_I . In contrast to [1], the definition of \mathcal {R}_0 , \Omega^+ and \Omega^- for our model (1.2) depends on the average population density, i.e. N/|\Omega| . From the epidemiology point of view, the more crowded the population is, the easier endemic a disease becomes.
Secondly, we analyse the asymptotic profile of EE when it exists. Regarding this, it is worthwhile to mention the following three results.
(ⅰ) The diffusion rate of the susceptible individuals d_S is small. Theorem 4 in [1] indicates that the asymptotic profile is some spatially inhomogeneous DFE. However, our results for (1.2) show that EE (if it exists) may approach a coexistence limiting equilibrium where the susceptible individuals spatially heterogeneously exist and the infected population is a spatially homogeneous state (see Theorem 1.3(ⅱ) and Corollary 1.5). Furthermore, we observe that the coexistence limiting equilibrium tends to a spatially inhomogeneous DFE when the saturated coefficient m is large. From a disease control point of view, if a disease is governed by system (1.2), it is not enough to just restrict the movement of the susceptible individuals to completely eradicate the disease in the whole habitat in certain situations, especially if the rate of disease transmission \beta is a constant and \Omega is a high-risk domain; see Corollary 1.5 (ⅰ).
(ⅱ) d_I\rightarrow0 and d_I/d_S\rightarrow d\in(0, \infty). Theorem 1.1(2) and Corollary 1.1(ⅰ) of [20] show that the existence habitat of the infective individuals is exactly the high-risk set. However, in our results, the ratio d plays a key role (see Theorem 1.6 and Remark 1.7). If d = 1 , the infected individuals survive exactly in the high-risk set; if d\in(0, 1) , the habitat of infected individual is confined within some subset of the high-risk set; if d > 1 , the infected individuals only die out at part of the low-risk sites. This information suggests that reducing the radio d to less than 1 will help to control disease; in other words, the more isolated the patients become, the better disease control is.
(ⅲ) The saturated coefficient m is large. For model (1.2), Theorem 1.9 indicates that the EE tends to the DFE, i.e., the infective individuals cannot persist. This result seems to coincide with the realistic intuition: the more inhibition effect from the behavioral change of the susceptible individuals when their number increases or from the crowding effect of the infective individuals, the better for disease control.
Finally, we would like to mention some open problems left for future study: (1) the existence of EE when d_S < d_I and \mathcal {R}_0\in (d_S/d_I, 1) ; (2) the asymptotic profile of EE when \mathcal {R}_0 > 1 and \frac{1}{|\Omega|}\int_\Omega \frac{\gamma(x)}{\beta(x)} dx < \frac{N}{|\Omega|}\leq\max\limits_{x\in\bar\Omega}\frac{\gamma(x)}{\beta(x)} as d_S\rightarrow0^+ ; (3) the uniqueness of EE if it exists when d_S < d_I.
The work of Y. Wang and Z. Wang was supported by the Natural Science Foundation of China (11671243, 61672021, 11771262, 11571274, 11501496), the Fundamental Research Funds for the Central Universities (GK201903088), the Natural Science Basic Research Plan in Shaanxi Province of China (2018JM1020, 2018JQ1021). The work of C. Lei was supported by the Natural Science Foundation of China (11801232), the Priority Academic Program Development of Jiangsu Higher Education Institution, the Natural Science Foundation of the Jiangsu Province(BK20180999), the Foundation of Jiangsu Normal University (17XLR008).
The authors declare there is no conflict of interest.
We recall the following well-known facts without proof.
Let \lambda_1(d, g) be the principal eigenvalue of
\begin{equation} \begin{cases} d \Delta \varphi+g(x)\varphi+\lambda\varphi = 0, &x\in\Omega, \\ \frac{\partial \varphi}{\partial \nu} = 0, &x\in\partial\Omega, \end{cases} \end{equation} | (A.1) |
where g(x)\in L^\infty (\Omega) and d > 0 . It is folklore that \lambda_1(d, g) is given by
\begin{equation} \lambda_1(d, g) = \inf\left\{\int_\Omega \left(d |\nabla\varphi|^2-g(x) \varphi^2\right)dx: \varphi\in H^1(\Omega) \ \text{and}\ \int_\Omega\varphi^2dx = 1\right\}. \end{equation} | (A.2) |
The properties of \lambda_1(d, g) are stated as follows; they can be found in [1,3].
Lemma A.1.
(ⅰ) If g_1(x)\leq g_2(x) in \Omega with g_i\in L^\infty (\Omega) for i = 1, 2, then \lambda_1(d, g_1)\geq\lambda_1(d, g_2) with equality holds if and only if g_1 = g_2 a.e. in \Omega ;
(ⅱ) if g\in L^\infty (\Omega) is non-constant, then \lambda_1(d_1, g) < \lambda_1(d_2, g) when d_1 < d_2 ;
(ⅲ) \lambda_1(d, g) depends continuously on g and d , and it satisfies
\begin{equation} \lim\limits_{d\rightarrow0^+}\lambda_1(d, g) = \min\limits_{x\in\bar{\Omega}}\{-g(x)\} \ \text{ and }\ \lim\limits_{d\rightarrow \infty}\lambda_1(d, g) = -\frac{1}{|\Omega|}\int_\Omega g(x)dx. \end{equation} | (A.3) |
The following lemma is about the existence of an elliptic problem and its asymptotic profile (as d\rightarrow0^+ ), which can be found in [3] or can be directly proved by an upper/lower solution argument. Let us denote h^+(x): = \max\{h(x), 0\} for any function h defined on \bar{\Omega} .
Lemma A.2. Suppose that positive functions a(x), b(x), d(x)\in C^\alpha(\bar{\Omega}) . Then the following statements hold for the problem
\begin{equation} \begin{cases} d\Delta u+ \left(\frac{a(x)}{1+b(x)u}-c(x)\right)u = 0, &x\in\Omega, \\ \frac{\partial u}{\partial \nu} = 0, & x\in\partial\Omega.\\ \end{cases} \end{equation} | (A.4) |
(ⅰ) If \lambda_1(d, a-c)\geq 0 , then u = 0 is the only non-negative solution of (A.4);
(ⅱ) if \lambda_1(d, a-c) < 0 , then (A.4) has a unique positive solution u\in C^{2+\alpha}(\bar{\Omega}) . Furthermore, u\rightarrow \left(\frac{a-c}{bc}\right)^+ as d\rightarrow0^+ provided a(x_0)-c(x_0) > 0 for some x_0\in \bar{\Omega} .
To obtain the priori estimates for solutions, the following maximum principle (due to Lou and Ni [18]) and the Harnack's inequality (see, e.g., [17]) are useful.
Lemma A.3. Suppose that g\in C(\bar \Omega\times\mathbb{R}) .
(ⅰ) Assume that \omega\in C^2(\Omega)\cap C^1(\bar\Omega) and satisfies
\begin{equation*} \begin{cases} \Delta\omega(x)+g(x, \omega(x))\geq0, &x\in\Omega, \\ \frac{\partial\omega}{\partial \nu} = 0, & x\in\partial\Omega. \end{cases} \end{equation*} |
If \omega(x_0) = \max\limits_{x\in\bar\Omega}\omega(x) , then g(x_0, \omega(x_0))\geq0.
(ⅱ) Assume that \omega\in C^2(\Omega)\cap C^1(\bar\Omega) and satisfies
\begin{equation*} \begin{cases} \Delta\omega(x)+g(x, \omega(x))\leq 0, &x\in\Omega, \\ \frac{\partial\omega}{\partial \nu} = 0, & x\in\partial\Omega. \end{cases} \end{equation*} |
If \omega(x_0) = \min\limits_{x\in\bar\Omega}\omega(x) , then g(x_0, \omega(x_0))\leq0.
Lemma A.4. Let w\in C^2(\Omega)\cap C^1(\bar{\Omega}) be a positive solution of
\begin{equation*} \begin{cases} \Delta\omega(x)+c(x)\omega(x) = 0, &x\in\Omega, \\ \frac{\partial\omega}{\partial \nu} = 0, & x\in\partial\Omega, \end{cases} \end{equation*} |
where c(x)\in C(\bar{\Omega}) . Then there exists a positive constant C = C(n, \Omega, \|c\|_\infty) such that
\max\limits_{\overline{\Omega}} w\leq C\min\limits_{\overline{\Omega}} w. |
[1] |
Piedrahita R, Coffey E R, Hagar Y, et al. (2019) Exposures to carbon monoxide in a cookstove intervention in Northern Ghana. Atmosphere 10: 402. doi: 10.3390/atmos10070402. doi: 10.3390/atmos10070402
![]() |
[2] | IPCC, Climate Change: The IPCC Scientific Assessment, World meteorological organization / united nations environmental program, Melbourne, Sydney, 1990. |
[3] | Eipa S J, Nganga J K, Muthama N J (2019) Utilization of livestock manure based biogas for climate change mitigation in Nakuru county, Kenya. J Sustain Environ Peace 1: 39–44. |
[4] |
Dubnov J, Portnov B A, Barchana M (2011) Air pollution and development of children's pulmonary function. Enc Environ Health 2011: 17–25. doi: 10.1016/B978-0-444-52272-6.00132-X. doi: 10.1016/B978-0-444-52272-6.00132-X
![]() |
[5] |
Shi Y, Xia Y, Lu B, et al. (2014) Emission inventory and trends of NO x for China, 2000–2020. J Zhejiang Univ Sci A 15: 454–464. doi: 10.1631/jzus.A1300379. doi: 10.1631/jzus.A1300379
![]() |
[6] |
Wilkins C K, Clausen P A, Wolkoff P, et al. (2011) Formation of strong airway irritants in mixtures of isoprene/ozone and isoprene/ozone/nitrogen dioxide. Environ Health Perspect 109: 937–941. doi: 10.1289/ehp.01109937. doi: 10.1289/ehp.01109937
![]() |
[7] |
Wilkins C K, Clausen P A, Wolkoff P, et al. (2016) Formation of strong airway irritants in mixtures of isoprene/ozone and isoprene/ozone/nitrogen dioxide. Adv Biosci Biotechnol 7: 278–288. doi: 10.4236/abb.2016.76026. doi: 10.4236/abb.2016.76026
![]() |
[8] |
Hamra G B, Laden F, Cohen A J, et al. (2015) Lung cancer and exposure to nitrogen dioxide and traffic: a systematic review and meta-analysis. Environ Health Perspect 123: 1107–1112. doi: 10.1289/ehp.1408882. doi: 10.1289/ehp.1408882
![]() |
[9] |
Rohde R A, Muller R A (2015) Air pollution in China: mapping of concentrations and sources. PLOS ONE 10: e0135749. doi: 10.1371/journal.pone.0135749. doi: 10.1371/journal.pone.0135749
![]() |
[10] |
Wang C, Horby P W, Hayden F G, et al. (2020) A novel coronavirus outbreak of global health concern. The Lancet 395: 470–473. doi: 10.1016/S0140-6736(20)30185-9. doi: 10.1016/S0140-6736(20)30185-9
![]() |
[11] |
Zhu N, Zhang D, Wang W, et al. (2020) A novel coronavirus from patients with pneumonia in China, 2019. N Engl J Med 382: 727–733.doi: 10.1056/NEJMoa2001017. doi: 10.1056/NEJMoa2001017
![]() |
[12] |
Shereen M A, Khan S, Kazmi A, et al. (2020) COVID-19 infection: Origin, transmission, and characteristics of human coronaviruses J Adv Res 24: 91–98. doi: 10.1016/j.jare.2020.03.005. doi: 10.1016/j.jare.2020.03.005
![]() |
[13] |
He G, Pan Y, Tanaka T (2020) The short-term impacts of COVID-19 lockdown on urban air pollution in China. Nat Sustain 3: 1005–1011. doi: 10.1038/s41893-020-0581-y. doi: 10.1038/s41893-020-0581-y
![]() |
[14] |
Aluga M A (2020) Coronavirus Disease 2019 (COVID-19) in Kenya: Preparedness, response and transmissibility. J Microbiol Immunol Infect doi: 10.1016/j.jmii.2020.04.011. doi: 10.1016/j.jmii.2020.04.011
![]() |
[15] |
Quaife M, Van Zandvoort K, Gimma A, et al. (2020) The impact of COVID-19 control measures on social contacts and transmission in Kenyan informal settlements. BMC medicine 18: 1–11. doi: 10.1101/2020.06.06.20122689. doi: 10.1101/2020.06.06.20122689
![]() |
[16] | Daniel A Vallero (2015) Air pollution. In Kirk-Othmer Encyclopedia of Chemical Technology, John Wiley & Sons, Inc (Ed.). doi: 10.1002/0471238961.01091823151206.a01.pub3. |
[17] | Acker J G, Leptoukh G (2007) Online analysis enhances use of NASA earth science data. Eos Trans AGU 88: 14–17. |
[18] | Efegbidiki L O (2015) Carbon monoxide: Its impacts on human health in Abuja, Nigeria. DOI: 10.13140/RG.2.1.2067.3441 |
[19] | Zaid M A (2015) Correlation and Regression Analysis. Oran, Ankara – Turkey: The statistical, economic and social research and training centre for islamic countries. |
[20] | Cohen J (1988) Statistical Power Analysis for the Behavioral Sciences, 2nd ed. Hillsdale, NJ: Erlbaum. |
[21] |
Comunian S, Dongo D, Milani C, et al. (2020) Air pollution and COVID-19: the role of particulate matter in the spread and increase of COVID-19's morbidity and mortality. Int J Environ Res Public Health 17: 4487. doi: 10.3390/ijerph17124487. doi: 10.3390/ijerph17124487
![]() |
[22] |
Ciencewicki J, Jaspers I (2007) Air pollution and respiratory viral infection. Inhal Toxicol 19: 1135–1146. doi: 10.1080/08958370701665434. doi: 10.1080/08958370701665434
![]() |
[23] |
Li S, Xu J, Jiang Z, et al. (2019) Correlation between indoor air pollution and adult respiratory health in Zunyi City in Southwest China: situation in two different seasons. BMC Public Health 19: 1–14. doi: 10.1186/s12889-019-7063-z. doi: 10.1186/s12889-019-7063-z
![]() |
[24] |
Sanità di Toppi L, Sanità di Toppi L, Bellini E (2020) Novel coronavirus: how atmospheric particulate affects our environment and health. Challenges 11: 6. doi: 10.3390/challe11010006. doi: 10.3390/challe11010006
![]() |
[25] |
Chen K, Wang M, Huang C, et al. (2020) Air pollution reduction and mortality benefit during the COVID-19 outbreak in China. Lancet Planet Health 4: e210–e212. doi: 10.1016/S2542-5196(20)30107-8. doi: 10.1016/S2542-5196(20)30107-8
![]() |
[26] |
Albayati N, Waisi B, Al-Furaiji M, et al. (2021) Effect of COVID-19 on air quality and pollution in different countries. J Transp Health 21: 101061. doi: 10.1016/j.jth.2021.101061. doi: 10.1016/j.jth.2021.101061
![]() |
[27] |
Nigam R, Pandya K, Luis A J, et al. (2021) Positive effects of COVID-19 lockdown on air quality of industrial cities (Ankleshwar and Vapi) of Western India. Sci Rep 11: 1–12. doi: 10.1038/s41598-021-83393-9. doi: 10.1038/s41598-021-83393-9
![]() |
[28] |
Morales-Solís K, Ahumada H, Rojas J P, et al. (2021) The effect of COVID-19 lockdowns on the air pollution of urban areas of central and southern Chile. Aerosol Air Qual Res 21: 200677. doi: 10.4209/aaqr.200677. doi: 10.4209/aaqr.200677
![]() |
[29] | Nriagu J O (2011) Encyclopedia of environmental health. Amsterdam: Elsevier, 2011. Accessed on: Jul. 23, 2020. Available: http://www.sciencedirect.com/science/referenceworks/9780444522726 |
[30] |
Yoo J M, Jeong M J, Kim D, et al. (2015) Spatiotemporal variations of air pollutants (O 3, NO 2, SO 2, CO, PM 10, and VOCs) with land-use types. Atmospheric Chem Phys 15: 10857–10885. doi: 10.5194/acp-15-10857-2015. doi: 10.5194/acp-15-10857-2015
![]() |
[31] |
Kumie A, Emmelin A, Wahlberg S, et al. (2009) Sources of variation for indoor nitrogen dioxide in rural residences of Ethiopia. Environ Health 8: 1–11. doi: 10.1186/1476-069X-8-51. doi: 10.1186/1476-069X-8-51
![]() |
[32] |
Levy J I, Lee K, Yanagisawa Y, et al. (1998) Determinants of nitrogen dioxide concentrations in indoor ice skating rinks. Am J Public Health 88: 1781–1786. doi: 10.2105/AJPH.88.12.1781. doi: 10.2105/AJPH.88.12.1781
![]() |
[33] |
Andersen I B (1972) Relationships between outdoor and indoor air pollution. Atmospheric Environ. 1967. 6: 275–278. doi: 10.1016/0004-6981(72)90086-8. doi: 10.1016/0004-6981(72)90086-8
![]() |
[34] |
El-Hougeiri N, El Fadel M (2004) Correlation of indoor-outdoor air quality in urban areas. Indoor Built Environ. 13: 421–431. doi: 10.1177/1420326X04049344. doi: 10.1177/1420326X04049344
![]() |
[35] |
Leung D Y C (2015) Outdoor-indoor air pollution in urban environment: challenges and opportunity. Front Environ Sci 2: 69. doi: 10.3389/fenvs.2014.00069. doi: 10.3389/fenvs.2014.00069
![]() |
[36] |
López-Aparicio S, Smolík J, Mašková L, et al. (2011) Relationship of indoor and outdoor air pollutants in a naturally ventilated historical building envelope. Build Environ 46: 1460–1468. doi: 10.1016/j.buildenv.2011.01.013. doi: 10.1016/j.buildenv.2011.01.013
![]() |
[37] |
Ribeiro H V, Rybski D, Kropp J P (2019) Effects of changing population or density on urban carbon dioxide emissions. Nat Commun 10: 1–9. doi: 10.1038/s41467-019-11184-y. doi: 10.1038/s41467-019-11184-y
![]() |
[38] | Neto T G S, Dias F F, Saito V O, et al. (2012) Emission factors for CO2, CO and main hydrocarbon gases, and biomass consumption in an Amazonian forest clearing fire. USEPA |
[39] |
Zak M, Melaniuk-Wolny E, Widziewicz K (2017) The exposure of pedestrians, drivers and road transport passengers to nitrogen dioxide. Atmospheric Pollut Res 8: 781–790. doi: 10.1016/j.apr.2016.10.011. doi: 10.1016/j.apr.2016.10.011
![]() |
[40] | European Environmental Agency.2020 Air pollution goes down as Europe takes hard measures to combat coronavirus. Accessed on: Mar. 25, 2020. https://www.eea.europa.eu/highlights/air-pollution-goes-down-as |
[41] | McMahon J (2020) Coronavirus lockdown likely saved 77,000 lives in China just by reducing pollution. Forbes 20: 2020. https://go.nature.com/2BiWBt3. |
[42] | NASA Earth Observatory, "2020 Airborne nitrogen dioxide plummets over China, ", Accessed on: Sep. 09, 2020. https://go.nature.com/2Vxj3oQ |
[43] |
Venter Z S, Aunan K, Chowdhury S, et al. (2020) COVID-19 lockdowns cause global air pollution declines. Proc Natl Acad Sci 117: 18984–18990. doi: 10.1073/pnas.2006853117. doi: 10.1073/pnas.2006853117
![]() |
[44] | Suresh A, Chauhan D, Othmani A, et al. (2020) Diagnostic Comparison of Changes in Air Quality over China before and during the COVID-19 Pandemic. doi: 10.21203/rs.3.rs-30482/v1. |
[45] |
Timmons D, Zirogiannis N, Lutz M (2016) Location matters: population density and carbon emissions from residential building energy use in the United States. Energy Res Soc Sci 22: 137–146. doi: 10.1016/j.erss.2016.08.011. doi: 10.1016/j.erss.2016.08.011
![]() |
1. | Linhe Zhu, Xiaoyuan Huang, Ying Liu, Zhengdi Zhang, Spatiotemporal dynamics analysis and optimal control method for an SI reaction-diffusion propagation model, 2021, 493, 0022247X, 124539, 10.1016/j.jmaa.2020.124539 | |
2. | Shuyu Han, Chengxia Lei, Xiaoyan Zhang, Qualitative analysis on a diffusive SIRS epidemic model with standard incidence infection mechanism, 2020, 71, 0044-2275, 10.1007/s00033-020-01418-1 | |
3. | Jialiang Zhang, Renhao Cui, Asymptotic behavior of an SIS reaction–diffusion–advection model with saturation and spontaneous infection mechanism, 2020, 71, 0044-2275, 10.1007/s00033-020-01375-9 | |
4. | Xin Huo, Renhao Cui, A reaction–diffusion SIS epidemic model with saturated incidence rate and logistic source, 2020, 0003-6811, 1, 10.1080/00036811.2020.1859495 | |
5. | Xueying Sun, Renhao Cui, Analysis on a diffusive SIS epidemic model with saturated incidence rate and linear source in a heterogeneous environment, 2020, 490, 0022247X, 124212, 10.1016/j.jmaa.2020.124212 | |
6. | Jialiang Zhang, Renhao Cui, Asymptotic profiles of the endemic equilibrium of a diffusive SIS epidemic system with saturated incidence rate and spontaneous infection, 2021, 44, 0170-4214, 517, 10.1002/mma.6754 | |
7. | Renhao Cui, Asymptotic profiles of the endemic equilibrium of a reaction-diffusion-advection SIS epidemic model with saturated incidence rate, 2021, 26, 1553-524X, 2997, 10.3934/dcdsb.2020217 | |
8. | Oyoon Abdul Razzaq, Najeeb Alam Khan, Muhammad Faizan, Asmat Ara, Saif Ullah, Behavioral response of population on transmissibility and saturation incidence of deadly pandemic through fractional order dynamical system, 2021, 26, 22113797, 104438, 10.1016/j.rinp.2021.104438 | |
9. | Xueying Sun, Renhao Cui, Existence and asymptotic profiles of the steady state for a diffusive epidemic model with saturated incidence and spontaneous infection mechanism, 2021, 14, 1937-1632, 4503, 10.3934/dcdss.2021120 | |
10. | Qi Cao, Yuying Liu, Wensheng Yang, Global dynamics of a diffusive SIR epidemic model with saturated incidence rate and discontinuous treatments, 2022, 10, 2195-268X, 1770, 10.1007/s40435-022-00935-3 | |
11. | Lingmin Dong, Bo Li, Guanghui Zhang, Analysis on a Diffusive SI Epidemic Model with Logistic Source and Saturation Infection Mechanism, 2022, 45, 0126-6705, 1111, 10.1007/s40840-022-01255-7 | |
12. | Yutong Guo, Jinliang Wang, Desheng Ji, Asymptotic profiles of a diffusive SIS epidemic model with vector-mediated infection and logistic source, 2022, 73, 0044-2275, 10.1007/s00033-022-01888-5 | |
13. | Xuan Tian, Shangjiang Guo, Zhisu Liu, Qualitative analysis of a diffusive SEIR epidemic model with linear external source and asymptomatic infection in heterogeneous environment, 2022, 27, 1531-3492, 3053, 10.3934/dcdsb.2021173 | |
14. | Chuanxin Liu, Renhao Cui, Qualitative analysis on an SIRS reaction–diffusion epidemic model with saturation infection mechanism, 2021, 62, 14681218, 103364, 10.1016/j.nonrwa.2021.103364 | |
15. | Huicong Li, Tian Xiang, On an SIS epidemic model with power‐like nonlinear incidence and with/without cross‐diffusion, 2024, 153, 0022-2526, 10.1111/sapm.12683 | |
16. | Daozhou Gao, Chengxia Lei, Rui Peng, Benben Zhang, A diffusive SIS epidemic model with saturated incidence function in a heterogeneous environment * , 2024, 37, 0951-7715, 025002, 10.1088/1361-6544/ad1495 | |
17. | Anqi Qu, Jinfeng Wang, Asymptotic profiles of positive steady states in a reaction–diffusion benthic–drift model, 2024, 153, 0022-2526, 10.1111/sapm.12752 | |
18. | Soufiane Bentout, Salih Djilali, Asymptotic profiles of a generalized reaction-diffusion SIS epidemic model with spatial heterogeneity, 2024, 75, 0044-2275, 10.1007/s00033-024-02373-x | |
19. | Han Lu, Yan’e Wang, Jianhua Wu, An Efficient Competitive Control Mechanism for Negative Information Spread in Online Social Networks, 2024, 34, 0218-1274, 10.1142/S0218127424501724 | |
20. | Renhao Cui, Huicong Li, Zikun Wang, Saturated incidence tends to ease disease persistence: Analysis of an SIS epidemic patch model, 2025, 0, 1078-0947, 0, 10.3934/dcds.2025010 | |
21. | Hongmin Zhang, Jian Zhang, Xin Huo, Theoretical Analysis on a Diffusive SIS Epidemic Model with Logistic Source, Saturated Incidence Rate and Spontaneous Infection Mechanism, 2025, 13, 2227-7390, 1244, 10.3390/math13081244 |