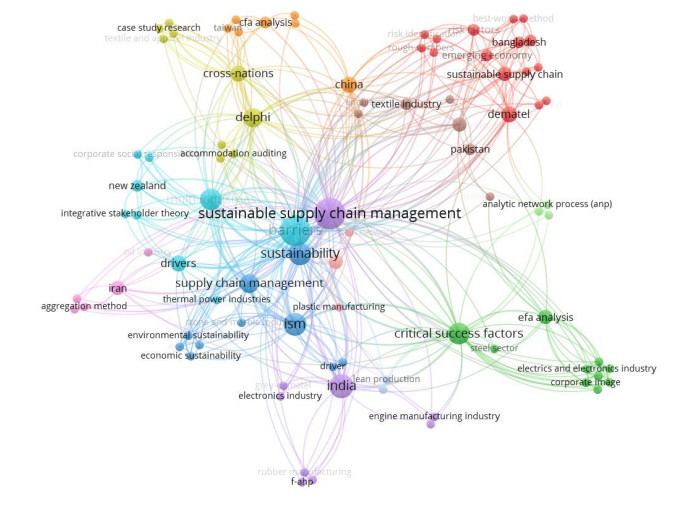
Zero-shot learning aims to transfer the model of labeled seen classes in the source domain to the disjoint unseen classes without annotations in the target domain. Most existing approaches generally consider directly adopting the visual-semantic projection function learned in the source domain to the target domain without adaptation. However, due to the distribution discrepancy between the two domains, it remains challenging in dealing with the projection domain shift problem. In this work, we formulate a novel bi-shifting semantic auto-encoder to learn the semantic representations of the target instances and reinforce the generalization ability of the projection function. The encoder aims at mapping the visual features into the semantic space by leveraging the visual features of target instances and is guided by the semantic prototypes of seen classes. While two decoders manage to respectively reconstruct the original visual features in the source and target domains. Thus, our model can capture the generalized semantic characteristics related with the seen and unseen classes to alleviate the projection function problem. Furthermore, we develop an efficient algorithm by the advantage of the linear projection functions. Extensive experiments on the five benchmark datasets demonstrate the competitive performance of our proposed model.
Citation: Yu Wang. Bi-shifting semantic auto-encoder for zero-shot learning[J]. Electronic Research Archive, 2022, 30(1): 140-167. doi: 10.3934/era.2022008
[1] | G. Nandini, M. Venkatachalam, Raúl M. Falcón . On the r-dynamic coloring of subdivision-edge coronas of a path. AIMS Mathematics, 2020, 5(5): 4546-4562. doi: 10.3934/math.2020292 |
[2] | Meiqin Jin, Ping Chen, Shuangliang Tian . Interval edge colorings of the generalized lexicographic product of some graphs. AIMS Mathematics, 2024, 9(11): 30597-30611. doi: 10.3934/math.20241477 |
[3] | T. Deepa, M. Venkatachalam, Raúl M. Falcón . On the r-dynamic coloring of the direct product of a path with either a path or a cycle. AIMS Mathematics, 2020, 5(6): 6496-6520. doi: 10.3934/math.2020419 |
[4] | Shuangliang Tian, Ping Chen . Edge-coloring of generalized lexicographic product of graphs. AIMS Mathematics, 2024, 9(6): 15988-15995. doi: 10.3934/math.2024774 |
[5] | Syed Ahtsham Ul Haq Bokhary, Zill-e-Shams, Abdul Ghaffar, Kottakkaran Sooppy Nisar . On the metric basis in wheels with consecutive missing spokes. AIMS Mathematics, 2020, 5(6): 6221-6232. doi: 10.3934/math.2020400 |
[6] | Gaixiang Cai, Fengru Xiao, Guidong Yu . The identification numbers of lollipop graphs. AIMS Mathematics, 2025, 10(4): 7813-7827. doi: 10.3934/math.2025358 |
[7] | Kiki A. Sugeng, Fery Firmansah, Wildan, Bevina D. Handari, Nora Hariadi, Muhammad Imran . Several properties of antiadjacency matrices of directed graphs. AIMS Mathematics, 2024, 9(10): 27834-27847. doi: 10.3934/math.20241351 |
[8] | Yanyi Li, Lily Chen . Injective edge coloring of generalized Petersen graphs. AIMS Mathematics, 2021, 6(8): 7929-7943. doi: 10.3934/math.2021460 |
[9] | Shahbaz Ali, Muhammad Khalid Mahmmod, Raúl M. Falcón . A paradigmatic approach to investigate restricted hyper totient graphs. AIMS Mathematics, 2021, 6(4): 3761-3771. doi: 10.3934/math.2021223 |
[10] | Hongyu Chen, Li Zhang . A smaller upper bound for the list injective chromatic number of planar graphs. AIMS Mathematics, 2025, 10(1): 289-310. doi: 10.3934/math.2025014 |
Zero-shot learning aims to transfer the model of labeled seen classes in the source domain to the disjoint unseen classes without annotations in the target domain. Most existing approaches generally consider directly adopting the visual-semantic projection function learned in the source domain to the target domain without adaptation. However, due to the distribution discrepancy between the two domains, it remains challenging in dealing with the projection domain shift problem. In this work, we formulate a novel bi-shifting semantic auto-encoder to learn the semantic representations of the target instances and reinforce the generalization ability of the projection function. The encoder aims at mapping the visual features into the semantic space by leveraging the visual features of target instances and is guided by the semantic prototypes of seen classes. While two decoders manage to respectively reconstruct the original visual features in the source and target domains. Thus, our model can capture the generalized semantic characteristics related with the seen and unseen classes to alleviate the projection function problem. Furthermore, we develop an efficient algorithm by the advantage of the linear projection functions. Extensive experiments on the five benchmark datasets demonstrate the competitive performance of our proposed model.
The number of studies and discussions in terms of sustainable supply chain management (SSCM) has been increasing rapidly in recent years. As a result, emerged concepts related to sustainability, such as sustainable production and sustainable development, have gradually become more popular globally under various industries [1,2]. Incredibly, although it is easier to focus on the manufacturers and assess their efforts towards sustainability by eco-friendly products, conducted sustainability standards [1,3]. It is nevertheless clear that the SSCM can only be obtained by the contributions of all stakeholders in the supply chain consist of suppliers or customers [4]. In recent years, managers' cognition has been changed in this regard. In general, all issues related to the natural environment, community, or financial benefits are carefully considered under global competitive conditions [5,6]. Consequently, several scholars believed that the top management should integrate sustainability philosophy into business strategies to solve the pressures from stakeholders [3,7]. The evolution of the sustainability philosophy has influenced the organizations' development strategy. Some recent studies documented that under different research contexts would identify various parameters as well as barriers [1,8]. Such as, Zimon et al. (2020) have highlighted various drivers and barriers for the SSCM through the conditions of seventeen Sustainable Development Goals (SDGs) of the United Nations [2]. Combination employing ISM and AHP approach to pointed out twenty most essential factors of the SSCM by the Indian engine sector [9]. In the Iranian mining industries, forty-one SSCM barriers have been identified [10]. Besides, the different research methods of the scholars will also present inconsistent findings related to the parameters' importance, such as Delphi technique [7,11]; ISM approach [3,12]; or conducting simultaneously two techniques to address research objectives [8,9]. Thus, it is clear that these diversities have suggested many promising future research gaps for other scholars to be concerned with the SSCM practice topic in identifying the prevailing parameters.
In Taiwan, numerous sustainability initiatives have been discussed and widely adopted in the various supply chains [13]. However, most studies only focus on Taiwan's vital industries, such as semiconductor manufacturing or electronics assembly [13,14]. Lack of research about the SSCM of agriculture sectors; meanwhile, the agricultural industries have great significance to the households of indigenous people, especially in rural areas [15]. Besides, several sustainable development policies have been published to improve the livelihood of that community group [16]. Recently, Taiwan's fresh fruit export industry is seriously affected by import bans from the Chinese market, and the total expected export value will be reduced by twenty percent in 2021 [17]. Thereby, this problem has a significant impact on Taiwan's fruit supply chain, particularly affecting farmers' livelihoods. Besides, excessive dependence on one market has seriously affected the sustainability of this supply chain. Thus, forcing Taiwan's fruit supply chain to change to ensure sustainability. According to the existing literature on the sustainable supply chain management topic, the authors aim to identify which essential parameters are given the attention and effect on the SSCM of the industry. Therefore, the Taiwanese fresh-fruit sector has been chosen for practical research to examine which parameters must be considered. This article aims to solve research objectives as follows: RO1. Identification of critical parameters for SSCM under the Taiwanese fresh-fruit sector; RO2. Evaluation to identify the most significant SSCM parameters in this context.
Concentrating on the discussion in parameters contribute to the Taiwanese sustainable fresh-fruit supply chain context as a unique research and promising. The Technique for Order of Preference by Similarity to Ideal Solution (TOPSIS) is one of the multi-criteria decision-making methods and is an outstanding technique to widely conducted [4]. Its ability has an excellent quality to solve complex problems and evaluate the importance of parameters [4,18]. Thereby, to solve two research objectives that this manuscript would conduct the TOPSIS tool to present the most significant SSCM parameters by the empirical research in Taiwan. Hence, this study would reveal some new methodological insights that bridge academia and practice for SSCM regard. Importantly, the research findings will contribute several theoretical implications for the SSCM literature. Besides, it would suggest some suggestions for managers several practical implications in this regard.
The existing literature recently revealed that the SSCM implementation topic had gained significant discussion in academics and practices thanks to the positive impact of sustainability in order to enhance business performance [2,19]. Although there are still controversial discussions about the definition of SSCM; however, most scholars agree that the SSCM implementation is in line with the economic, social, and environmental considerations to making business strategies [2,8]. Several recent studies indicated that under different research contexts would identify various parameters and barriers [1]. The examples can be Narimissa et al. (2020) suggested ten drivers and barrier to implement SSCM in the Iranian oil sector [7]. Consistent with that, Zimon et al. (2020) proposed the model for conducting SDGs in the SSCM [2]. After that, Pandey et al. (2021) employed the hybrid method to pointed out twenty most essential factors of the SSCM by the Indian engine sector [9]. Hence, these studies suggested promising future research gaps for other scholars to be concerned with the SSCM practice topic in identifying the prevailing parameters.
Furthermore, to determine the research gaps and identify the critical variables for SSCM; hence, the authors collect all papers related to SSCM practices and relevant impact elements based on the WoS and SCOPUS database. Several keywords for searching include "sustainable"; "supply chain management"; "parameter"; "barriers"; "elements"; "critical factors". As a result, a total of thirty-one journal papers have been assembled. They are discussed in various contexts, such as nations, industries, and approaches. A systematic analysis of all previous studies on the SSCM implementation topic has been conducted (presented Figure 1). The research gaps are identified in the following points relying on the existing SSCM implementation studies.
Firstly, the SSCM literature has witnessed the lack of empirical research in Taiwan, especially agriculture sector [13,14]; meanwhile, developing nations are the most preferred research context, for instance, India (n = 10) and China (n = 4). This is the first empirical research in Taiwanese food industry for the SSCM practices regard.
Secondly, sustainability studies regarding supply chain management have focused mainly on manufacturing, while the agricultural sector has experienced no specific research. Moreover, Taiwan's economy was well-known by the semiconductor manufacturing industry [14]; thus, conducting the study for the fresh-fruit supply chain has a significant impact on Taiwan's sustainable development, notably would broaden the SSCM practices literature in various sectors.
Thirdly, in terms of research method conducted, the majority of documents prioritize employed qualitative methods, such as ISM (n = 9) and Delphi (n = 6). However, the existing literature does not record any studies that have conducted the TOPSIS approach to consider this issue. Besides, some researchers proposed the TOPSIS as an outstanding technique, its ability is deemed good-quality to solve complex problems and enough to evaluate the importance of elements [4,18]. Hence, conducting the TOPSIS technique in this study would offer some new methodological insights that bridge academia and practice for SSCM regard.
According to the existing sustainability literature about supply chain management, twelve critical parameters reported to contribute to SSCM practices are demonstrated in Table 1.
Coding | Parameters | Brief Description | Sources |
P1 | Planning & Implementation | The optimal design of the fresh fruit supply chain is inevitable, and it requires proper planning and implementation. | [4,7,20,21,22] |
P2 | Knowledge & Training of fruit preservation | The preservation of post-harvest products needs to apply the latest knowledge and disseminate it to the stakeholders of the chain, particularly in farms and micro corporates. | [4,23,24,25] |
P3 | Farming area/ farmer | As the first stage of the food supply chain, the essential role of farms to provide fresh products to the wholesalers, factories, and end customers. | [5,15,26,27,28] |
P4 | Processing & Packaging | Fresh products need to maintain optimum quality for the longest time. Therefore, standardization and synchronization of processing and packaging must be attention for all the stages. | [4,20,29,30,31] |
P5 | Warehouse/Storage | Warehouse acts as a transit station in the middle of the food supply chain, the quality of products will be significantly affected by storage operation in warehouses. | [18,24,30,32] |
P6 | Distribution & Logistics service | The smooth flow of goods in the food supply chain will depend heavily on distribution channels and logistics services. | [5,18,33,34] |
P7 | Product quality & Safety | Fresh goods have stringent requirements for standard product quality and safety. It is the participation of the whole chain and must be guaranteed from the stage of planting and harvesting to the end stage. | [4,35,36] |
P8 | Contracting | Offering a variety of contracts for choose to ensure the benefits of each counterpart in the food supply chain. Thereby, it helps to reduce risks and conflicts among members. | [12,18,37,38] |
P9 | Technology adoption | Adopting the latest technology to the supply chain is part of the stakeholders' efforts to obtain sustainability, such as minimizing the impact on the natural environment, reducing costs. | [39,40,41] |
P10 | Customer | As the end-stage in the supply chain and consume products, the perceptions and reactions of this group is the driver and will significantly impact the sustainability initiatives of other stakeholders. | [20,34,42,43] |
P11 | Organizational social responsibilities | Conducting social programs for the local communities has been launched in the sustainable development goals. Forcing the various organization to perform. | [1,21,44,45] |
P12 | Collaboration | Collaboration among supply chain members would reinforce sustainability and obtain better performance by reducing financial constraints and production scale. | [5,6,31,46,47]. |
Pointing out optimal solutions according to various parameter selections, these results can be a resource to refer to before decision-making in many different practice situations. The authors assemble data through surveying with ten professionals in the SSCM expertise. They would play the role of decision-makers relying on their experience and knowledge. Collecting data has been done based on twelve clarified SSCM parameters. Employing the TOPSIS approach analyzes the expert's response to solving the second research objective.
The authors can rank all SSCM parameters to determine the most significant variables for the current Taiwanese fresh-fruit supply chain. In this study, ten experts in the Taiwanese fresh-fruit supply chain were asked to rate all criteria. The language terms will be defined from 1 to 10; in detail, "1" means "Extremely unimportant", and "10" means "Extremely important". Consequently, the TOPSIS algorithm could be conducted by the following process:
Step one: Relying on m alternative and n criteria having a rating of ith decision-makers and jth criteria. A matrix format as [xij]m∗n can be expressed for a MCDM issues as below.
{x11x12......x1nx21x22......x2n..xm1xm2......xmn}i=1,2,...,m;j=1,2,...,n |
Step two: The normalize decision matrix has been calculated in Eq 1, where i = 1, 2, ..., n; and j = 1, 2, ..., m. And then, obtaining the normalized decision matrix by calculate the value rij.
rij=xij√n∑i=1x2ij | (1) |
{r11r12......r1nr21r22......r2n..rm1rm2......rmn} |
Step three: The authors then construct the weighted normalized matrix by calculate vij.
vij=wj∗rij | (2) |
{v11v12......v1nv21v22......v2n..vm1vm2......vmn} |
In details, wj = weights of different attributes; (i = 1, 2, …, n; j = 1, 2, …, m)
Step four: Identifying the ideal solution consist of positive ideal solution (A+) and negative ideal solution (A-), the distance of these ideal solutions has been revealed by maximum and minimize value as below.
A+=(v+1,v+2,...,v+n)withvj+={maxxi.vij,mini.vij} | (3) |
A−=(v−1,v−2,...,v−n)withvj−={mini.vij,maxxi.vij} | (4) |
Step five: Determining each alternative's distance by calculating the Euclidean distance from the positive and negative ideal solutions. Those solutions are calculated by two values as s+i and s−i, and can be shown in Eqs 5 and 6, respectively.
s+i=√∑j(v+ij−v+j)2 | (5) |
s−i=√∑j(v−ij−v−j)2 | (6) |
with i = 1, 2, ..., m and j = 1, 2, ..., n.
Step six: The authors calculate the closeness coefficient values (CCi) of each alternative by the Eq 7:
CCi=s−is−i+s+iwithi=1,2,...,m | (7) |
Step seven: Determining the optimal solutions according to the CCi values range from 0 to 1 scale, the great solution according to the alternative value closest to 1 cutoff. Further, the authors also can determine exactly the rank of these alternatives thanks to these CCi values. These values will provide the key findings for the study to discuss.
Integration sustainability initiatives into business strategies are widely conducted in almost all Taiwanese sectors, most of them concentrated on essential industries like semiconductor manufacturing or electronics assembly compared to agriculture industries [13,14,48]. However, the agriculture sector has a significant influence on the indigenous people's livelihood are struggling with different risks to obtain sustainable development goals [16]. For instance, in recent years observed that Taiwan's fresh fruit supply chain has been easily vulnerable to customer pressuring [17]. Thereby, this problem has a significant impact on social performance, particularly affecting farmers' livelihoods. Thus, forcing Taiwan's fruit supply chain to change to attain sustainability through identifying SSCM prevailing parameters.
The authors assemble data for this study by interviewing ten experts regarding the Taiwanese fresh fruit supply chain. These respondents have a long experience along with their expertise in SSCM practices. Besides, they offer responses about twelve SSCM parameters. The respondents' personal information was also described in Table 2.
Category | Frequency | Percentage | |
Gender | Male | 7 | 70.0% |
Female | 3 | 30.0% | |
Age group | 31-40 | 3 | 30.0% |
41-50 | 5 | 50.0% | |
51-60 | 1 | 10.0% | |
over 60 | 1 | 10.0% | |
Degree | Bachelor degree | 4 | 40.0% |
Master degree | 4 | 40.0% | |
Ph.D. degree | 2 | 20.0% | |
Organizational | Process Manufacturing | 6 | 60.0% |
Service | 4 | 40.0% | |
Working Position | Top Managers | 3 | 30.0% |
Sales Department | 3 | 30.0% | |
Financial Management | 1 | 10.0% | |
Human Resource Management | 2 | 20.0% | |
Production Management | 1 | 10.0% |
The second research objective requires determining the importance level of SSCM parameters. The Taiwanese fresh-fruit supply chain has been chosen for this empirical research to address this objective. The study assembles data for analysis relying on the excellent knowledge and experiences of ten experts (Es) in this regard. Following the sequence of seven steps in TOPSIS analysis, the findings would report the significance of all twelve SSCM parameters. Further, they are considered good suggestions for the top managers in the Taiwanese fresh-fruit supply chain toward sustainability.
According to the years of experience of each respondent, that decides the weightage for each decision-maker (presented in Table 3). The normalized matrix of the respondents' answers has been conducted (follow Eq 1) to determine (xij) values.
Parameters | E1 | E2 | E3 | E4 | E5 | E6 | E7 | E8 | E9 | E10 |
Weight | 0.13 | 0.10 | 0.18 | 0.12 | 0.11 | 0.11 | 0.06 | 0.05 | 0.05 | 0.09 |
P1 | 0.0414 | 0.0319 | 0.0574 | 0.0489 | 0.0315 | 0.0160 | 0.0142 | 0.0177 | 0.0052 | 0.0276 |
P2 | 0.0322 | 0.0283 | 0.0574 | 0.0381 | 0.0315 | 0.0279 | 0.0142 | 0.0132 | 0.0173 | 0.0221 |
P3 | 0.0460 | 0.0212 | 0.0574 | 0.0272 | 0.0276 | 0.0359 | 0.0142 | 0.0132 | 0.0138 | 0.0166 |
P4 | 0.0184 | 0.0319 | 0.0319 | 0.0272 | 0.0394 | 0.0279 | 0.0170 | 0.0155 | 0.0173 | 0.0276 |
P5 | 0.0322 | 0.0177 | 0.0447 | 0.0326 | 0.0355 | 0.0319 | 0.0227 | 0.0132 | 0.0173 | 0.0276 |
P6 | 0.0414 | 0.0319 | 0.0574 | 0.0272 | 0.0315 | 0.0359 | 0.0198 | 0.0110 | 0.0173 | 0.0276 |
P7 | 0.0414 | 0.0354 | 0.0319 | 0.0326 | 0.0315 | 0.0359 | 0.0142 | 0.0110 | 0.0173 | 0.0276 |
P8 | 0.0414 | 0.0354 | 0.0574 | 0.0272 | 0.0355 | 0.0319 | 0.0113 | 0.0132 | 0.0138 | 0.0276 |
P9 | 0.0368 | 0.0248 | 0.0447 | 0.0326 | 0.0276 | 0.0359 | 0.0198 | 0.0177 | 0.0173 | 0.0276 |
P10 | 0.0414 | 0.0319 | 0.0574 | 0.0381 | 0.0276 | 0.0319 | 0.0198 | 0.0132 | 0.0017 | 0.0221 |
P11 | 0.0276 | 0.0212 | 0.0574 | 0.0326 | 0.0276 | 0.0279 | 0.0170 | 0.0199 | 0.0104 | 0.0276 |
P12 | 0.0414 | 0.0283 | 0.0574 | 0.0435 | 0.0315 | 0.0359 | 0.0198 | 0.0110 | 0.0138 | 0.0276 |
Then, conducting two Eqs 2 and 3 to explore (rij) and (vij) values, respectively. Table 3 demonstrate the weighted normalized decision matrix for this research.
Thereby, the next step can be shown in Table 4, in detail, two essential values are pointed out includes positive (A+) and negative (A−) ideal solutions.
Ideal Solutions | E1 | E2 | E3 | E4 | E5 | E6 | E7 | E8 | E9 | E10 |
Positive (A+) | 0.0460 | 0.0354 | 0.0574 | 0.0489 | 0.0394 | 0.0359 | 0.0227 | 0.0199 | 0.0173 | 0.0276 |
Negative (A−) | 0.0184 | 0.0177 | 0.0319 | 0.0272 | 0.0276 | 0.0160 | 0.0113 | 0.0110 | 0.0017 | 0.0166 |
The Euclidean distance would be considered in step five by conducting two Eqs as 5 and 6 for each SSCM parameter. As a result, Table 5 and Table 6 are illustrate the positive and negative ideal solution values, respectively.
Parameters | E1 | E2 | E3 | E4 | E5 | E6 | E7 | E8 | E9 | E10 |
P1 | 0.0046 | 0.0035 | 0 | 0 | 0.0079 | 0.0200 | 0.0085 | 0.0022 | 0.0121 | 0 |
P2 | 0.0138 | 0.0071 | 0 | 0.0109 | 0.0079 | 0.0080 | 0.0085 | 0.0066 | 0 | 0.0055 |
P3 | 0 | 0.0142 | 0 | 0.0218 | 0.0118 | 0 | 0.0085 | 0.0066 | 0.0035 | 0.0110 |
P4 | 0.0276 | 0.0035 | 0.0255 | 0.0218 | 0 | 0.0080 | 0.0057 | 0.0044 | 0 | 0 |
P5 | 0.0138 | 0.0177 | 0.0128 | 0.0163 | 0.0039 | 0.0040 | 0 | 0.0066 | 0 | 0 |
P6 | 0.0046 | 0.0035 | 0 | 0.0218 | 0.0079 | 0 | 0.0028 | 0.0088 | 0 | 0 |
P7 | 0.0046 | 0 | 0.0255 | 0.0163 | 0.0079 | 0 | 0.0085 | 0.0088 | 0 | 0 |
P8 | 0.0046 | 0 | 0 | 0.0218 | 0.0039 | 0.0040 | 0.0113 | 0.0066 | 0.0035 | 0 |
P9 | 0.0092 | 0.0106 | 0.0128 | 0.0163 | 0.0118 | 0 | 0.0028 | 0.0022 | 0 | 0 |
P10 | 0.0046 | 0.0035 | 0 | 0.0109 | 0.0118 | 0.0040 | 0.0028 | 0.0066 | 0.0155 | 0.0055 |
P11 | 0.0184 | 0.0142 | 0 | 0.0163 | 0.0118 | 0.0080 | 0.0057 | 0 | 0.0069 | 0 |
P12 | 0.0046 | 0.0071 | 0 | 0.0054 | 0.0079 | 0 | 0.0028 | 0.0088 | 0.0035 | 0 |
Parameters | E1 | E2 | E3 | E4 | E5 | E6 | E7 | E8 | E9 | E10 |
P1 | 0.0230 | 0.0142 | 0.0255 | 0.0218 | 0.0039 | 0 | 0.0028 | 0.0066 | 0.0035 | 0.0110 |
P2 | 0.0138 | 0.0106 | 0.0255 | 0.0109 | 0.0039 | 0.0120 | 0.0028 | 0.0022 | 0.0155 | 0.0055 |
P3 | 0.0276 | 0.0035 | 0.0255 | 0 | 0 | 0.0200 | 0.0028 | 0.0022 | 0.0121 | 0 |
P4 | 0 | 0.0142 | 0 | 0 | 0.0118 | 0.0120 | 0.0057 | 0.0044 | 0.0155 | 0.0110 |
P5 | 0.0138 | 0 | 0.0128 | 0.0054 | 0.0079 | 0.0160 | 0.0113 | 0.0022 | 0.0155 | 0.0110 |
P6 | 0.0230 | 0.0142 | 0.0255 | 0 | 0.0039 | 0.0200 | 0.0085 | 0 | 0.0155 | 0.0110 |
P7 | 0.0230 | 0.0177 | 0 | 0.0054 | 0.0039 | 0.0200 | 0.0028 | 0 | 0.0155 | 0.0110 |
P8 | 0.0230 | 0.0177 | 0.0255 | 0 | 0.0079 | 0.0160 | 0 | 0.0022 | 0.0121 | 0.0110 |
P9 | 0.0184 | 0.0071 | 0.0128 | 0.0054 | 0 | 0.0200 | 0.0085 | 0.0066 | 0.0155 | 0.0110 |
P10 | 0.0230 | 0.0142 | 0.0255 | 0.0109 | 0 | 0.0160 | 0.0085 | 0.0022 | 0 | 0.0055 |
P11 | 0.0092 | 0.0035 | 0.0255 | 0.0054 | 0 | 0.0120 | 0.0057 | 0.0088 | 0.0086 | 0.0110 |
P12 | 0.0230 | 0.0106 | 0.0255 | 0.0163 | 0.0039 | 0.0200 | 0.0085 | 0 | 0.0121 | 0.0110 |
Finally, the authors calculate the closeness ratio (CCi) values for all essential SSCM parameters to determine the importance level by Eq 7. Table 7 reports the ranking of twelve SSCM parameters for the Taiwanese fresh fruit sector based on their CCi values. Consequently, the final rank of the SSCM parameters in terms of the Taiwanese fresh-fruit industry is P12 > P6 > P10 > P8 > P1 > P2 > P9 > P3 > P7 > P5 > P11 > P4. Additionally, Figure 2 is expressed the results of these findings analysis related to the comparison among the ranked key parameters of SSCM implementation on the basis of CCi values.
Parameters | s+i | s−i | CCi | Ranking |
Collaboration (P12) | 0.0162 | 0.0481 | 0.7485 | 1 |
Distribution & Logistic service (P6) | 0.0256 | 0.0472 | 0.6485 | 2 |
Customer (P10) | 0.0251 | 0.0431 | 0.6318 | 3 |
Contracting (P8) | 0.0266 | 0.0456 | 0.6313 | 4 |
Planning & Implementation (P1) | 0.0268 | 0.0453 | 0.6286 | 5 |
Knowledge & Training of fruit preservation (P2) | 0.0251 | 0.0389 | 0.6079 | 6 |
Technology adoption (P9) | 0.0279 | 0.0382 | 0.5777 | 7 |
Farming area/ farmer (P3) | 0.0326 | 0.0445 | 0.5772 | 8 |
Product quality & Safety (P7) | 0.0339 | 0.0407 | 0.5453 | 9 |
Warehouse/Storage (P5) | 0.0317 | 0.0346 | 0.5214 | 10 |
Organizational social responsibilities (P11) | 0.0330 | 0.0350 | 0.5151 | 11 |
Processing & Packaging (P4) | 0.0449 | 0.0300 | 0.4006 | 12 |
The experts' responses have documented that "Collaboration" has obtained the highest priority (CCi = 0.7485) among the SSCM parameters in the Taiwanese fresh-fruit sector. Meanwhile, the second importance level belongs to "Distribution & Logistics service" (CCi = 0.6485), and followed by "Customer" issues (CCi = 0.6318). On the contrary, the TOPSIS analysis reported that three parameters consist of "Warehouse/Storage" (CCi = 0.5214), "Organizational social responsibilities" (CCi = 0.5151), and "Processing & Packaging" (CCi = 0.4006) are located at the bottom of the ranking table. In the middle of the rankings are other variables; in detail, "Contracting" (CCi = 0.6313) and "Planning & Implementation" (CCi = 0.6286) have ranked fourth and fifth place, respectively. Meanwhile, other parameters consist of "Knowledge & Training of fruit preservation" (CCi = 0.6079), "Technology adoption" (CCi = 0.5777), "Farming area/ farmer" (CCi = 0.5772) and "Product quality and safety" (CCi = 0.5453) are the less critical level consecutively. These criteria have been recognized as critical parameters for the sustainability of the Taiwanese fresh-fruit supply chain, their importance level as the reference sources for this industry to consider and carry out. Particularly in the current competitive period, when even the most minor efforts are attention and could improve performance.
In Taiwan's context, the agricultural industry has great significance to the livelihood of indigenous people, especially in remote areas [15]. Meanwhile, several sustainable development policies have been launched to enhance living conditions and improve the livelihood of the indigenous people [16]. Further, connecting sustainable agricultural development to other fields and therefore make a meaningful contribution towards sustainability [15]. Regarding the food supply chain, particularly in the fresh-fruit supply chain, which should face various risks from the production process to the end customers [1,34]. Thus, the Taiwanese fresh-fruit sector can refer to these twelve identified SSCM parameters as a reference resource in making decisions through these research findings. To contribute some managerial implications, based on ten experts' opinions, the data has been collected to analyze and discuss twelve identified parameters. The results analysis report that these variables have a different ranking from highest-priority to lowest. These findings indicate the importance level of various parameters for the SSCM of the Taiwanese fresh-fruit sector.
In terms of the food supply chain, there is a presence and participation of many different stakeholders [5]. Thus, several solutions regarding collaboration have been discussed to promote and improve intimate cooperation among members in the food supply chain, besides targeting further obtaining supply chain sustainability [47]. Importantly, collaborating among suppliers will achieve better performance due to they could remove financial constraints and production scale, particularly suitable in the context of small and medium enterprises of agriculture industries [46]. Hence, in line with previous studies regarding the "Collaboration" variable [6,46,47], ten experts in this study believed that collaboration among members of the Taiwanese fresh-fruit supply chain is the most significance to obtain sustainability. In this research, "Distribution & Logistics service" variable obtained second place in the preoccupation of experts about SSCM practices. As the risk sector, the agriculture industry must determine the optimal time from the stage in collecting fresh products in various farms, distribution to the warehouses, then sending to the end customers [5]. Besides, avoiding damage to fresh fruit during transportation is very essential [33,34]. Dhaoui et al. (2020) explored the close relationship between the consumers' and distribution channels of these fresh products in Greece, such as fruit and vegetables. "Customer" parameter is rated higher than "Contracting" variable, two criteria are statistically significant at third and fourth positions, respectively. An interesting fact in the supply chain is that manufacturers that act as suppliers can also be customers of other suppliers [18]. The critical role of the customers variable in the food supply chain has been proven in other prior studies [34,42]. Thus, customers' expectations have become the targets of various suppliers [20,43]. To avoid risks from customers parameter, expanding the market scope to find more different customers has become a solution for suppliers in this area [31].
Notably, considering "Contracting" as the supply chain parameters, Shen et al. [37] have divided into nine contracting clusters based on information. Besides, contracting with information updating among counterparts has been suggested to attain a sustainable supply chain. Surprisingly, these findings are consistent with the exploring of Chaudhuri et al. [12] and Xiao et al. [38], they have identified and particularly interested in contracting to reduce the risk for the food supply chain in India and China, respectively. Further, the study findings have contributed to the existing literature some promising ideas for the top managers when considering various proposed SSCM parameters. Although the remaining variables have lower CCi values; however, their significance for the food supply chain has been determined and verified once again by the fresh-fruit supply chain in Taiwan. Consequently, unfortunately, the analysis results witnessed the lowest values of some parameters, for instance, "Warehouse/Storage" [24,30,32], "Organizational social responsibilities" [1,44,45], and "Packaging & Processing" [4,20,30]. But the role of these criteria should not be underestimated or ignored. Ideally, to attain SSCM needs to conduct simultaneously different blueprints by the best efforts of all members.
Sustainability target is an inevitable trend worldwide [23]. It forces all various industries and nations to adopt different solutions to obtain [1]. In fact, fresh agriculture products have great significance on the livelihoods of the Taiwanese indigenous communities [15,16]. Therefore, determining critical parameters for the fruits chain in Taiwan is the main contribution insight; as a result, twelve essential variables have been identified. Moreover, as a different approach insight, the authors employed the TOPSIS technique for this study to explore the importance level of all parameters. It is considered an excellent tool to address research objectives through comparison among closeness coefficients. Consequently, based on the experts' responses, this study successfully determined the role of these essential variables in the Taiwanese fresh-fruit chain. Analysis outcomes revealed that "Collaboration" at the peak of the list, "Distribution & Logistics service" obtained second place, and followed by "Customer" get the third position with their CCi values as 0.7485, 0.6485, 0.6318, respectively. On the contrary, at the bottom of the prioritized list is three essential parameters consisting of "Warehouse/Storage", "Organizational social responsibilities", and "Processing & Packaging" with their CCi values as 0.5214, 0.5151, 0.4006, respectively. Unsurprisingly, collaboration with the right partner toward obtain sustainability together is very emerging attention; for instance, Song et al. [49] reported that it is the most considerable risk factor in the telecommunications sector. Meanwhile, Pandey et al. [9] considered the collaboration parameter less essential in an engine manufacturing industry. The contradictory assessments compared to the findings of this study have presented in some previous studies, such as, Prasad et al. [50] believed that customer's influence ranks bottom among the twenty SSCM critical factors of the Indian steel industry. Hence, differences in the importance ranking of SSCM components in practices have been observed depending on various industries and countries' contexts, consistent with the suggestions of Panigrahi et al. [1] and Zimon et al. [2].
As part of agricultural sustainability, the whole fruit supply chain members must carry out simultaneously these twelve identified critical parameters. Hence, these research results are a positive signal for senior managers to refer to making business decisions. This research is the first empirical research concentrating on the fresh-fruit supply chain in Taiwan to determine essential SSCM parameters, and provide the new theoretical insights to existing supply chain literature; unfortunately, this study cannot avoid certain limitations. Nevertheless, it can become promising suggestions to consider for others scholars to develop further. Firstly, this manuscript adopted the TOPSIS technique to analyze only ten professionals' responses. Therefore, the survey on a broader scale can be conducted to examine the impact of these variables on the SSCM performance in practices. Secondly, to broaden the concern of SSCM literature, particularly in the food supply chain, other scholars can suggest more parameters contribute to SSCM implementation. Thirdly, the Taiwanese fresh-fruit supply chain is considered as the case to examine the appropriateness of these SSCM parameters. Hence, the authors believe that these proposed SSCM criteria need to explore in another context, such as the national context, different supply chains of the agriculture sectors.
The authors acknowledge the chief editor and the reviewers for their valuable comments to improve the manuscript.
All authors declare no conflicts of interest in this paper.
[1] |
M. Everingham, S. M. Eslami, L. Gool, C. K. Williams, J. Winn, A. Zisserman, The pascal visual object classes challenge: a retrospective, Int. J. Comput. Vis., 111 (2015), 98–136. https://doi.org/10.1007/s11263-014-0733-5 doi: 10.1007/s11263-014-0733-5
![]() |
[2] | S. J. Dickinson, A. Leonardis, B. Schiele, M. J. Tarr, Object categorization: computer and human vision perspectives, Cambridge University Press, Cambridge, 2009. https://doi.org/10.1017/cbo9780511635465 |
[3] |
X. Zhang, Y. H. Yang, Z. Han, H. Wang, C. Gao, Object class detection: a survey, ACM Comput. Surv., 46 (2013), 1–53. https://doi.org/10.1145/2522968.2522978 doi: 10.1145/2522968.2522978
![]() |
[4] |
Y. Li, S. Wang, Q. Tian, X. Ding, Feature representation for statistical-learning-based object detection: a review, Pattern Recognit., 48 (2015), 3542–3559. https://doi.org/10.1016/j.patcog.2015.04.018 doi: 10.1016/j.patcog.2015.04.018
![]() |
[5] |
Z. Zhao, P. Zheng, S. Xu, X. Wu, Object detection with deep learning: a review, IEEE Trans. Neural Netw. Learn. Syst., 30 (2019), 3212–3232. https://doi.org/10.1109/tnnls.2018.2876865 doi: 10.1109/tnnls.2018.2876865
![]() |
[6] |
K. Oksuz, B. C. Cam, S. Kalkan, E. Akbas, Imbalance problems in object detection: a review, IEEE Trans. Pattern Anal. Mach. Intell., 43 (2021), 3388–3415. https://doi.org/10.1109/tpami.2020.2981890 doi: 10.1109/tpami.2020.2981890
![]() |
[7] |
L. Liu, W. Ouyang, X. Wang, P. Fieguth, J. Chen, X. Liu, et al. Deep learning for generic object detection: a survey, Int. J. Comput. Vis., 128 (2020), 261–318. https://doi.org/10.1007/s11263-019-01247-4 doi: 10.1007/s11263-019-01247-4
![]() |
[8] |
S. Ghosh, N. Das, I. Das, U. Maulik, Understanding deep learning techniques for image segmentation, ACM Comput. Surv., 52 (2019), 1–35. https://doi.org/10.1145/3329784 doi: 10.1145/3329784
![]() |
[9] |
S. Minaee, Y. Y. Boykov, F. Porikli, A. J. Plaza, N. Kehtarnavaz, D. Terzopoulos, Image segmentation using deep learning: a survey, IEEE Trans. Pattern Anal. Mach. Intell., (2021). https://doi.org/10.1109/tpami.2021.3059968 doi: 10.1109/tpami.2021.3059968
![]() |
[10] |
R. Datta, D. Joshi, J. Li, J. Z. Wang, Image retrieval: ideas, influences, and trends of the new age, ACM Comput. Surv., 40 (2008), 1–60. https://doi.org/10.1145/1348246.1348248 doi: 10.1145/1348246.1348248
![]() |
[11] |
D. Zhang, M. M. Islam, G. Lu, A review on automatic image annotation techniques, Pattern Recognit., 45 (2012), 346–362. https://doi.org/10.1016/j.patcog.2011.05.013 doi: 10.1016/j.patcog.2011.05.013
![]() |
[12] |
X. Li, T. Uricchio, L. Ballan, M. Bertini, C. G. Snoek, A. D. Bimbo, Socializing the semantic gap: a comparative survey on image tag assignment, refinement, and retrieval, ACM Comput. Surv., 49 (2016), 1–39. https://doi.org/10.1145/2906152 doi: 10.1145/2906152
![]() |
[13] |
P. Wiriyathammabhum, D. Summers-Stay, C. Fermüller, Y. Aloimonos, Computer vision and natural language processing: recent approaches in multimedia and robotics, ACM Comput. Surv., 49 (2020), 1–44. https://doi.org/10.1145/3009906 doi: 10.1145/3009906
![]() |
[14] |
Y. Belinkov, J. Glass, Analysis methods in neural language processing: a survey, Trans. Assoc. Comput. Linguist., 7 (2019), 49–72. https://doi.org/10.1162/tacl_a_00254 doi: 10.1162/tacl_a_00254
![]() |
[15] |
K. Gauman, B. Leibe, Visual object recognition, Synth. Lect. Artif. Intell. Mach. Learn., 5 (2011), 1–181. https://doi.org/10.2200/S00332ED1V01Y201103AIM011 doi: 10.2200/S00332ED1V01Y201103AIM011
![]() |
[16] |
Y. Bengio, A. Courville, P. Vincent, Representation learning: a review and new perspectives, IEEE Trans. Pattern Anal. Mach. Intell., 35 (2013), 1798–1828. https://doi.org/10.1109/tpami.2013.50 doi: 10.1109/tpami.2013.50
![]() |
[17] | I. Goodfellow, Y. Bengio, A. Courville, Deep learning, MIT Press, Cambridge, 2016. |
[18] |
G. Litjens, T. Kooi, B. E. Bejnordi, A. A. A. Setio, F. Ciompi, M. Ghafoorian, et al., A survey on deep learning in medical image analysis, Med. Image Anal., 42 (2017), 60–88. https://doi.org/10.1016/j.media.2017.07.005 doi: 10.1016/j.media.2017.07.005
![]() |
[19] |
J. Gu, Z. Wang, J. Kuen, L. Ma, A. Shahroudy, B. Shuai, et al., Recent advances in convolutional neural networks, Pattern Recognit., 77 (2018), 354–377. https://doi.org/10.1016/j.patcog.2017.10.013 doi: 10.1016/j.patcog.2017.10.013
![]() |
[20] | M. G. Kendall, A. Stuart, J. K. Ord, Kendall's advanced theory of statistics, 5th edition, Oxford University Press, Oxford, 1987. |
[21] | J. Deng, W. Dong, R. Socher, L. Li, K. Li, F. Li, Imagenet: a large-scale hierarchical image database, in IEEE Conf. Comput. Vis. Pattern Recognit., IEEE, (2009), 248–255. https://doi.org/10.1109/cvpr.2009.5206848 |
[22] | California Institute of Technology, The caltech-ucsd birds-200-2011 dataset, Computation & Neural Systems Technical Report of California Institute of Technology, 2011. Available from: http://www.vision.caltech.edu/visipedia/CUB-200-2011.html. |
[23] |
Y. Wang, Q. Yao, J. T. Kwok, L. M. Ni, Generalizing from a few examples: a survey on few-shot learning, ACM Comput. Surv., 53 (2020), 1–34. https://doi.org/10.1145/3386252 doi: 10.1145/3386252
![]() |
[24] |
W. J. Scheirer, A. de Rezende Rocha, A. Sapkota, T. E. Boult, Toward open set recognition, IEEE Trans. Pattern Anal. Mach. Intell., 35 (2012), 1757–1772. https://doi.org/10.1109/tpami.2012.256 doi: 10.1109/tpami.2012.256
![]() |
[25] |
C. Geng, S. Huang, S. Chen, Recent advances in open set recognition: a survey, IEEE Trans. Pattern Anal. Mach. Intell., 43 (2021), 3614–3631. https://doi.org/10.1109/tpami.2020.2981604 doi: 10.1109/tpami.2020.2981604
![]() |
[26] |
I. Biederman, Recognition-by-components: a theory of human image understanding, Psychol. Rev., 94 (1987), 115–147. https://doi.org/10.1037/0033-295x.94.2.115 doi: 10.1037/0033-295x.94.2.115
![]() |
[27] |
C. H. Lampert, H. Nickisch, S. Harmeling, Attribute-based classification for zero-shot visual object categorization, IEEE Trans. Pattern Anal. Mach. Intell., 36 (2013), 453–465. https://doi.org/10.1109/tpami.2013.140 doi: 10.1109/tpami.2013.140
![]() |
[28] | W. Xu, Y. Xian, J. Wang, B. Schiele, Z. Akata, Attribute prototype network for zero-shot learning, preprint, arXiv: 2008.08290. |
[29] |
S. Changpinyo, W. L. Chao, B. Gong, F. Sha, Classifier and exemplar synthesis for zero-shot learning, Int. J. Comput. Vis., 128 (2020), 166–201. https://doi.org/10.1007/s11263-019-01193-1 doi: 10.1007/s11263-019-01193-1
![]() |
[30] |
Z. Ji, H. Wang, Y. Pang, L. Shao, Dual triplet network for image zero-shot learning, Neurocomputing, 373 (2020), 90–97. https://doi.org/10.1016/j.neucom.2019.09.062 doi: 10.1016/j.neucom.2019.09.062
![]() |
[31] |
Y. Ma, X. Xu, F. Shen, H. Shen, Similarity preserving feature generating networks for zero-shot learning, Neurocomputing, 406 (2020), 333–342. https://doi.org/10.1016/j.neucom.2019.08.111 doi: 10.1016/j.neucom.2019.08.111
![]() |
[32] | M. M. Palatucci, D. A. Pomerleau, G. E. Hinton, T. Mitchell, Zero-shot learning with semantic output codes, in Ann. Conf. Neural Inf. Process. Syst., MIT Press, 22 (2009), 1410–1418. |
[33] |
M. Johnson, M. Schuster, Q. V. Le, M. Krikun, Y. Wu, Z. Chen, et al., Google' s multilingual neural machine translation system: enabling zero-shot translation, Trans. Assoc. Comput. Linguist., 5 (2017), 339–351. https://doi.org/10.1162/tacl_a_00065 doi: 10.1162/tacl_a_00065
![]() |
[34] | N. Nakashole, R. Flauger, Knowledge distillation for bilingual dictionary induction, in Conf. Empirical Methods Nat. Language Process., ACL, (2017), 2497–2506. https://doi.org/10.18653/v1/d17-1264 |
[35] | H. Larochelle, D. Erhan, Y. Bengio, Zero-data learning of new tasks, in AAAI Conf. Artif. Intell., AAAI, 1 (2008), 646–651. |
[36] | C. H. Lampert, H. Nickisch, S. Harmeling, Learning to detect unseen object classes by between-class attribute transfer, in IEEE Conf. Comput. Vis. Pattern Recognit., IEEE, (2009), 951–958. https://doi.org/10.1109/cvpr.2009.5206594 |
[37] | N. Mohammad, M. Tomas, B. Samy, S. Yoram, S. Jonathon, F. Andrea, et al., Zero-shot learning by convex combination of semantic embeddings, preprint, arXiv: 1312.5650. |
[38] | A. Frome, G. S. Corrado, J. Shlens, S. Bengio, J. Dean, M. Ranzat, et al., Devise: a deep visual-semantic embedding model, in Ann. Conf. Neural Inform. Process. Syst., MIT Press, 2 (2013), 2121–2129. |
[39] | R. Socher, M. Ganjoo, H. Sridhar, O. Bastani, C. D. Manning, A. Y. Ng, Zero-shot learning through cross-modal transfer, in Ann. Conf. Neural Inform. Process. Syst., MIT Press, 26 (2013), 935–943. |
[40] | S. Reed, Z. Akata, H. Lee, B. Schiele, Learning deep representations of fine-grained visual descriptions, in IEEE Conf. Comput. Vis. Pattern Recognit., IEEE, (2016), 49–58. https://doi.org/10.1109/cvpr.2016.13 |
[41] | Z. Akata, S. Reed, D. Walter, H. Lee, B. Schiele, Evaluation of output embeddings for fine-grained image classification, in IEEE Conf. Comput. Vis. Pattern Recognit., IEEE, (2015), 2927–2936. https://doi.org/10.1109/cvpr.2015.7298911 |
[42] | Y. Xian, Z. Akata, G. Sharma, Q. Nguyen, M. Hein, B. Schiele, Latent embeddings for zero-shot classification, in IEEE Conf. Comput. Vis. Pattern Recognit., IEEE, (2016), 69–77. https://doi.org/10.1109/cvpr.2016.15 |
[43] |
Y. Fu, T. M. Hospedales, T. Xiang, S. Gong, Transductive multi-view zero-shot learning, IEEE Trans. Pattern Anal. Mach. Intell., 37 (2015), 2332–2345. https://doi.org/10.1109/tpami.2015.2408354 doi: 10.1109/tpami.2015.2408354
![]() |
[44] |
S. Rahman, S. Khan, F. Porikli, A unified approach for conventional zero-shot, generalized zero-shot, and few-shot learning, IEEE Trans. Image Process., 27 (2018), 5652–5667. https://doi.org/10.1109/tip.2018.2861573 doi: 10.1109/tip.2018.2861573
![]() |
[45] | S. Daghaghi, T. Medini, A. Shrivastava, Sdm-net: a simple and effective model for generalized zero-shot learning, preprint, arXiv: 1909.04790. |
[46] |
Z. Jia, Z. Zhang, L. Wang, C. Shan, T. Tan, Deep unbiased embedding transfer for zero-shot learning, IEEE Trans. Image Process., 29 (2019), 1958–1971. https://doi.org/10.1109/tip.2019.2947780 doi: 10.1109/tip.2019.2947780
![]() |
[47] | B. Romera-Paredes, P. H. S. Torr, An embarrassingly simple approach to zero-shot learning, in Int. Conf. Machine Learn., ACM, 37 (2015), 2152–2161. https://doi.org/10.1007/978-3-319-50077-5_2 |
[48] | E. Kodirov, T. Xiang, S. Gong, Semantic autoencoder for zero-shot learning, in IEEE Conf. Comput. Vis. Pattern Recognit., IEEE, (2017), 3174–3183. https://doi.org/10.1109/cvpr.2017.473 |
[49] | S. Changpinyo, W. L. Chao, B. Gong, F. Sha, Synthesized classifiers for zero-shot learning, in IEEE Conf. Comput. Vis. Pattern Recognit., IEEE, (2016), 5327–5336. https://doi.org/10.1109/cvpr.2016.575 |
[50] | Y. Xian, T. Lorenz, B. Schiele, Z. Akata, Feature generating networks for zero-shot learning, in IEEE Conf. Comput. Vis. Pattern Recognit., IEEE, (2018), 5542–5551. https://doi.org/10.1109/cvpr.2018.00581 |
[51] | H. Huang, C. Wang, P. S. Yu, C. Wang, Generative dual adversarial network for generalized zero-shot learning, in IEEE Conf. Comput. Vis. Pattern Recognit., IEEE, (2019), 801–810. https://doi.org/10.1109/cvpr.2019.00089 |
[52] | S. Xu, Z. Gao, G. Xie, Bi-semantic reconstructing generative network for zero-shot learning, preprint, arXiv: 1912.03877. |
[53] | Z. Ye, F. Lyu, L. Li, Q. Fu, J. Ren, F. Hu, Sr-gan: semantic rectifying generative adversarial network for zero-shot learning, in IEEE Int. Conf. Multimedia & Expo, IEEE, (2019), 85–90. https://doi.org/10.1109/icme.2019.00023 |
[54] | Y. Zhu, M. Elhoseiny, B. Liu, X. Peng, A. Elgammal, A generative adversarial approach for zero-shot learning from noisy texts, in IEEE Conf. Comput. Vis. Pattern Recognit., IEEE, (2018), 1004–1013. https://doi.org/10.1109/cvpr.2018.00111 |
[55] | A. Mishra, S. Krishna Reddy, A. Mittal, H. A. Murthy, A generative model for zero shot learning using conditional variational autoencoders, in IEEE Conf. Comput. Vis. Pattern Recognit., IEEE, (2018), 2188–2196. https://doi.org/10.1109/cvprw.2018.00294 |
[56] | Z. Wan, D. Chen, Y. Li, X. Yan, J. Zhang, Y. Yu, et al., Transductive zero-shot learning with visual structure constraint, preprint, arXiv: 1901.01570. |
[57] | M. Ye, Y. Guo, Zero-shot classification with discriminative semantic representation learning, in IEEE Conf. Comput. Vis. Pattern Recognit., IEEE, (2017), 7140–7148. https://doi.org/10.1109/cvpr.2017.542 |
[58] |
Z. Akata, F. Perronnin, Z. Harchaoui, C. Schmid, Label-embedding for image classification, IEEE Trans. Pattern Anal. Mach. Intell., 38 (2016), 1425–1438. https://doi.org/10.1109/tpami.2015.2487986 doi: 10.1109/tpami.2015.2487986
![]() |
[59] |
Y. Yu, Z. Ji, X. Li, J. Guo, Z. Zhang, H. Ling, et al., Transductive zero-shot learning with a self-training dictionary approach, IEEE T. Cybern., 48 (2018), 2908–2919. https://doi.org/10.1109/tcyb.2017.2751741 doi: 10.1109/tcyb.2017.2751741
![]() |
[60] |
Y. Shi, W. Wei, Discriminative embedding autoencoder with a regressor feedback for zero-shot learning, IEEE Access, 8 (2020), 11019–11030. https://doi.org/10.1109/access.2020.2964613 doi: 10.1109/access.2020.2964613
![]() |
[61] | J. Song, C. Shen, Y. Yang, Y. Liu, M. Song, Transductive unbiased embedding for zero-shot learning, in IEEE Conf. Comput. Vis. Pattern Recognit., IEEE, (2018), 1024–1033. https://doi.org/10.1109/cvpr.2018.00113 |
[62] | V. K. Verma, P. Rai, A simple exponential family framework for zero-shot learning, in Joint European Conference on Machine Learning and Knowledge Discovery in Databases, Springer, (2017), 792–808. https://doi.org/10.1007/978-3-319-71246-8_48 |
[63] | M. Kan, S. Shan, X. Chen, Bi-shifting auto-encoder for unsupervised domain adaptation, in Int. Conf. Comput. Vis., IEEE, (2015), 3846–3854. https://doi.org/10.1109/cvpr.2017.547 |
[64] | J. Zhang, W. Li, P. Ogunbona, Joint geometrical and statistical alignment for visual domain adaptation, in IEEE Conf. Comput. Vis. Pattern Recognit., IEEE, (2017), 1859–1867. https://doi.org/10.1109/cvpr.2017.547 |
[65] | E. Kodirov, T. Xiang, Z. Fu, S. Gong, Unsupervised domain adaptation for zero-shot learning, in Int. Conf. Comput. Vis., IEEE, (2015), 2452–2460. https://doi.org/10.1109/iccv.2015.282 |
[66] | Y. Liu, Q. Gao, J. Li, J. Han, L. Shao, Zero shot learning via low-rank embedded semantic autoencoder, in International Joint Conference on Artificial Intelligence, Morgan Kaufmann, (2018), 2490–2496. https://doi.org/10.24963/ijcai.2018/345 |
[67] | Z. Zhang, V. Saligrama, Zero-shot learning via semantic similarity embedding, in Int. Conf. Comput. Vis., IEEE, (2015), 4166–4174. https://doi.org/10.1109/iccv.2015.474 |
[68] | J. Li, M. Jin, K. Lu, Z. Ding, Z. Huang, Leveraging the invariant side of generative zero-shot learning, in IEEE Conf. Comput. Vis. Pattern Recognit., IEEE, (2019), 7394–7403. https://doi.org/10.1109/cvpr.2019.00758 |
[69] | M. Radovanović, A. Nanopoulos, M. Ivanović, Hubs in space: popular nearest neighbors in high-dimensional data, J. Mach. Learn. Res., 11 (2010), 2487–2531. |
[70] | L. Zhang, T. Xiang, S. Gong, Learning a deep embedding model for zero-shot learning, in IEEE Conf. Comput. Vis. Pattern Recognit., IEEE, (2017), 2021–2030. https://doi.org/10.1109/cvpr.2017.321 |
[71] | Y. Shigeto, I. Suzuki, K. Hara, M. Shimbo, Y. Matsumoto, Ridge regression, hubness, and zero-shot learning, in Joint European Conference on Machine Learning and Knowledge Discovery in Databases, Springer, (2015), 135–151. https://doi.org/10.1007/978-3-319-23528-8_9 |
[72] | Y. Wu, W. Cao, Y. Liu, Z. Ming, J. Li, B. Lu, Semantic auto-encoder with l2-norm constraint for zero-shot learning, in Int. Conf. Mach. Learn. Comput., ACM, (2021), 101–105. https://doi.org/10.1145/3457682.3457699 |
[73] | Y. Li, D. Wang, H. Hu, Y. Lin, Y. Zhuang, Zero-shot recognition using dual visual-semantic mapping paths, in IEEE Conf. Comput. Vis. Pattern Recognit., IEEE, (2017), 3279–3287. https://doi.org/10.1109/cvpr.2017.553 |
[74] | P. Lancaster, M. Tismenetsky, The theory of matrices: with applications, 2nd edition, Academic Press, Amsterdam, 1985. |
[75] |
Y. Xian, C. H. Lampert, B. Schiele, Z. Akata, Zero-shot learning – a comprehensive evaluation of the good, the bad and the ugly, IEEE Trans. Pattern Anal. Mach. Intell., 41 (2018), 2251–2265. https://doi.org/10.1109/tpami.2018.2857768 doi: 10.1109/tpami.2018.2857768
![]() |
[76] | A. Farhadi, I. Endres, D. Hoiem, D. Forsyth, Describing objects by their attributes, in IEEE Conf. Comput. Vis. Pattern Recognit., IEEE, (2009), 1778–1785. https://doi.org/10.1109/cvpr.2009.5206772 |
[77] |
G. Patterson, C. Xu, H. Su, J. Hays, The sun attribute database: beyond categories for deeper scene understanding, Int. J. Comput. Vis., 108 (2014), 59–81. https://doi.org/10.1007/s11263-013-0695-z doi: 10.1007/s11263-013-0695-z
![]() |
[78] | C. Szegedy, W. Liu, Y. Jia, P. Sermanet, S. Reed, D. Anguelov, et al., Going deeper with convolutions, in IEEE Conf. Comput. Vis. Pattern Recognit., IEEE, (2015), 1–9. https://doi.org/10.1109/cvpr.2015.7298594 |
[79] | S. M. Shojaee, M. S. Baghshah, Semi-supervised zero-shot learning by a clustering-based approach, preprint, arXiv: 1605.09016. |
[80] |
Y. Guo, G. Ding, J. Han, Y. Gao, Zero-shot learning with transferred samples, IEEE Trans. Image Process., 26 (2017), 3277–3290. https://doi.org/10.1109/tip.2017.2696747 doi: 10.1109/tip.2017.2696747
![]() |
[81] | Y. Annadani, S. Biswas, Preserving semantic relations for zero-shot learning, in IEEE Conf. Comput. Vis. Pattern Recognition, IEEE, (2018), 7603–7612. https://doi.org/10.1109/cvpr.2018.00793 |
[82] |
H. Zhang, Y. Long, Y. Guan, L. Shao, Triple verification network for generalized zero-shot learning, IEEE Trans. Image Process., 28 (2019), 506–517. https://doi.org/10.1109/tip.2018.2869696 doi: 10.1109/tip.2018.2869696
![]() |
[83] | L. Van der Maaten, G. Hinton, Visualizing data using t-sne, J. Mach. Learn. Res., 9 (2008), 2579–2605. |
[84] |
Z. Zhang, Y. Li, J. Yang, Y. Li, M. Gao, Cross-layer autoencoder for zero-shot learning, IEEE Access, 7 (2019), 167584–167592. https://doi.org/10.1109/access.2019.2953454 doi: 10.1109/access.2019.2953454
![]() |
1. | Abbott Po Shun Chen, Yung-Fu Huang, Manh-Hoang Do, Exploring the Challenges to Adopt Green Initiatives to Supply Chain Management for Manufacturing Industries, 2022, 14, 2071-1050, 13516, 10.3390/su142013516 | |
2. | Nahiyah Jaidi, Jane Liu, Zahrotush Sholikhah, Mega Murti Andhini, Ambidexterity Behavior of Creative SMEs for Disruptive Flows of Innovation: A Comparative Study of Indonesia and Taiwan, 2022, 8, 2199-8531, 141, 10.3390/joitmc8030141 | |
3. | Vu-Dung-Van Phan, Yung-Fu Huang, Thi-Them Hoang, Manh-Hoang Do, Evaluating Barriers to Supply Chain Resilience in Vietnamese SMEs: The Fuzzy VIKOR Approach, 2023, 11, 2079-8954, 121, 10.3390/systems11030121 | |
4. | Yung-Fu Huang, Abbott Po-Shun Chen, Manh-Hoang Do, Jen-Chieh Chung, Assessing the Barriers of Green Innovation Implementation: Evidence from the Vietnamese Manufacturing Sector, 2022, 14, 2071-1050, 4662, 10.3390/su14084662 | |
5. | Manh-Hoang Do, Yung-Fu Huang, Barriers to university social responsibility implementation in the Vietnamese higher education, 2023, 0951-354X, 10.1108/IJEM-11-2021-0432 | |
6. | Manh-Hoang Do, Yung-Fu Huang, Vu-Dung-Van Phan, Analyzing the barriers to green supply chain management implementation: a case study of the Vietnamese agriculture sector, 2023, 1741-0398, 10.1108/JEIM-10-2021-0459 | |
7. | Muhamad Rusliyadi, Yu Hua Chen, 2025, chapter 8, 9798369353509, 133, 10.4018/979-8-3693-5350-9.ch008 | |
8. | Imadeddine Oubrahim, 2025, Chapter 18, 978-3-031-81377-1, 204, 10.1007/978-3-031-81378-8_18 | |
9. | Damian Dubisz, Arkadiusz Kawa, Correlation Between Key Parameters of Wood Biomass Reverse Supply Chains and Their Impact on Total Biomass Acquisition Levels, 2023, 66, 2956-9141, 1, 10.12841/wood.1644-3985.449.06 |
Coding | Parameters | Brief Description | Sources |
P1 | Planning & Implementation | The optimal design of the fresh fruit supply chain is inevitable, and it requires proper planning and implementation. | [4,7,20,21,22] |
P2 | Knowledge & Training of fruit preservation | The preservation of post-harvest products needs to apply the latest knowledge and disseminate it to the stakeholders of the chain, particularly in farms and micro corporates. | [4,23,24,25] |
P3 | Farming area/ farmer | As the first stage of the food supply chain, the essential role of farms to provide fresh products to the wholesalers, factories, and end customers. | [5,15,26,27,28] |
P4 | Processing & Packaging | Fresh products need to maintain optimum quality for the longest time. Therefore, standardization and synchronization of processing and packaging must be attention for all the stages. | [4,20,29,30,31] |
P5 | Warehouse/Storage | Warehouse acts as a transit station in the middle of the food supply chain, the quality of products will be significantly affected by storage operation in warehouses. | [18,24,30,32] |
P6 | Distribution & Logistics service | The smooth flow of goods in the food supply chain will depend heavily on distribution channels and logistics services. | [5,18,33,34] |
P7 | Product quality & Safety | Fresh goods have stringent requirements for standard product quality and safety. It is the participation of the whole chain and must be guaranteed from the stage of planting and harvesting to the end stage. | [4,35,36] |
P8 | Contracting | Offering a variety of contracts for choose to ensure the benefits of each counterpart in the food supply chain. Thereby, it helps to reduce risks and conflicts among members. | [12,18,37,38] |
P9 | Technology adoption | Adopting the latest technology to the supply chain is part of the stakeholders' efforts to obtain sustainability, such as minimizing the impact on the natural environment, reducing costs. | [39,40,41] |
P10 | Customer | As the end-stage in the supply chain and consume products, the perceptions and reactions of this group is the driver and will significantly impact the sustainability initiatives of other stakeholders. | [20,34,42,43] |
P11 | Organizational social responsibilities | Conducting social programs for the local communities has been launched in the sustainable development goals. Forcing the various organization to perform. | [1,21,44,45] |
P12 | Collaboration | Collaboration among supply chain members would reinforce sustainability and obtain better performance by reducing financial constraints and production scale. | [5,6,31,46,47]. |
Category | Frequency | Percentage | |
Gender | Male | 7 | 70.0% |
Female | 3 | 30.0% | |
Age group | 31-40 | 3 | 30.0% |
41-50 | 5 | 50.0% | |
51-60 | 1 | 10.0% | |
over 60 | 1 | 10.0% | |
Degree | Bachelor degree | 4 | 40.0% |
Master degree | 4 | 40.0% | |
Ph.D. degree | 2 | 20.0% | |
Organizational | Process Manufacturing | 6 | 60.0% |
Service | 4 | 40.0% | |
Working Position | Top Managers | 3 | 30.0% |
Sales Department | 3 | 30.0% | |
Financial Management | 1 | 10.0% | |
Human Resource Management | 2 | 20.0% | |
Production Management | 1 | 10.0% |
Parameters | E1 | E2 | E3 | E4 | E5 | E6 | E7 | E8 | E9 | E10 |
Weight | 0.13 | 0.10 | 0.18 | 0.12 | 0.11 | 0.11 | 0.06 | 0.05 | 0.05 | 0.09 |
P1 | 0.0414 | 0.0319 | 0.0574 | 0.0489 | 0.0315 | 0.0160 | 0.0142 | 0.0177 | 0.0052 | 0.0276 |
P2 | 0.0322 | 0.0283 | 0.0574 | 0.0381 | 0.0315 | 0.0279 | 0.0142 | 0.0132 | 0.0173 | 0.0221 |
P3 | 0.0460 | 0.0212 | 0.0574 | 0.0272 | 0.0276 | 0.0359 | 0.0142 | 0.0132 | 0.0138 | 0.0166 |
P4 | 0.0184 | 0.0319 | 0.0319 | 0.0272 | 0.0394 | 0.0279 | 0.0170 | 0.0155 | 0.0173 | 0.0276 |
P5 | 0.0322 | 0.0177 | 0.0447 | 0.0326 | 0.0355 | 0.0319 | 0.0227 | 0.0132 | 0.0173 | 0.0276 |
P6 | 0.0414 | 0.0319 | 0.0574 | 0.0272 | 0.0315 | 0.0359 | 0.0198 | 0.0110 | 0.0173 | 0.0276 |
P7 | 0.0414 | 0.0354 | 0.0319 | 0.0326 | 0.0315 | 0.0359 | 0.0142 | 0.0110 | 0.0173 | 0.0276 |
P8 | 0.0414 | 0.0354 | 0.0574 | 0.0272 | 0.0355 | 0.0319 | 0.0113 | 0.0132 | 0.0138 | 0.0276 |
P9 | 0.0368 | 0.0248 | 0.0447 | 0.0326 | 0.0276 | 0.0359 | 0.0198 | 0.0177 | 0.0173 | 0.0276 |
P10 | 0.0414 | 0.0319 | 0.0574 | 0.0381 | 0.0276 | 0.0319 | 0.0198 | 0.0132 | 0.0017 | 0.0221 |
P11 | 0.0276 | 0.0212 | 0.0574 | 0.0326 | 0.0276 | 0.0279 | 0.0170 | 0.0199 | 0.0104 | 0.0276 |
P12 | 0.0414 | 0.0283 | 0.0574 | 0.0435 | 0.0315 | 0.0359 | 0.0198 | 0.0110 | 0.0138 | 0.0276 |
Ideal Solutions | E1 | E2 | E3 | E4 | E5 | E6 | E7 | E8 | E9 | E10 |
Positive (A+) | 0.0460 | 0.0354 | 0.0574 | 0.0489 | 0.0394 | 0.0359 | 0.0227 | 0.0199 | 0.0173 | 0.0276 |
Negative (A−) | 0.0184 | 0.0177 | 0.0319 | 0.0272 | 0.0276 | 0.0160 | 0.0113 | 0.0110 | 0.0017 | 0.0166 |
Parameters | E1 | E2 | E3 | E4 | E5 | E6 | E7 | E8 | E9 | E10 |
P1 | 0.0046 | 0.0035 | 0 | 0 | 0.0079 | 0.0200 | 0.0085 | 0.0022 | 0.0121 | 0 |
P2 | 0.0138 | 0.0071 | 0 | 0.0109 | 0.0079 | 0.0080 | 0.0085 | 0.0066 | 0 | 0.0055 |
P3 | 0 | 0.0142 | 0 | 0.0218 | 0.0118 | 0 | 0.0085 | 0.0066 | 0.0035 | 0.0110 |
P4 | 0.0276 | 0.0035 | 0.0255 | 0.0218 | 0 | 0.0080 | 0.0057 | 0.0044 | 0 | 0 |
P5 | 0.0138 | 0.0177 | 0.0128 | 0.0163 | 0.0039 | 0.0040 | 0 | 0.0066 | 0 | 0 |
P6 | 0.0046 | 0.0035 | 0 | 0.0218 | 0.0079 | 0 | 0.0028 | 0.0088 | 0 | 0 |
P7 | 0.0046 | 0 | 0.0255 | 0.0163 | 0.0079 | 0 | 0.0085 | 0.0088 | 0 | 0 |
P8 | 0.0046 | 0 | 0 | 0.0218 | 0.0039 | 0.0040 | 0.0113 | 0.0066 | 0.0035 | 0 |
P9 | 0.0092 | 0.0106 | 0.0128 | 0.0163 | 0.0118 | 0 | 0.0028 | 0.0022 | 0 | 0 |
P10 | 0.0046 | 0.0035 | 0 | 0.0109 | 0.0118 | 0.0040 | 0.0028 | 0.0066 | 0.0155 | 0.0055 |
P11 | 0.0184 | 0.0142 | 0 | 0.0163 | 0.0118 | 0.0080 | 0.0057 | 0 | 0.0069 | 0 |
P12 | 0.0046 | 0.0071 | 0 | 0.0054 | 0.0079 | 0 | 0.0028 | 0.0088 | 0.0035 | 0 |
Parameters | E1 | E2 | E3 | E4 | E5 | E6 | E7 | E8 | E9 | E10 |
P1 | 0.0230 | 0.0142 | 0.0255 | 0.0218 | 0.0039 | 0 | 0.0028 | 0.0066 | 0.0035 | 0.0110 |
P2 | 0.0138 | 0.0106 | 0.0255 | 0.0109 | 0.0039 | 0.0120 | 0.0028 | 0.0022 | 0.0155 | 0.0055 |
P3 | 0.0276 | 0.0035 | 0.0255 | 0 | 0 | 0.0200 | 0.0028 | 0.0022 | 0.0121 | 0 |
P4 | 0 | 0.0142 | 0 | 0 | 0.0118 | 0.0120 | 0.0057 | 0.0044 | 0.0155 | 0.0110 |
P5 | 0.0138 | 0 | 0.0128 | 0.0054 | 0.0079 | 0.0160 | 0.0113 | 0.0022 | 0.0155 | 0.0110 |
P6 | 0.0230 | 0.0142 | 0.0255 | 0 | 0.0039 | 0.0200 | 0.0085 | 0 | 0.0155 | 0.0110 |
P7 | 0.0230 | 0.0177 | 0 | 0.0054 | 0.0039 | 0.0200 | 0.0028 | 0 | 0.0155 | 0.0110 |
P8 | 0.0230 | 0.0177 | 0.0255 | 0 | 0.0079 | 0.0160 | 0 | 0.0022 | 0.0121 | 0.0110 |
P9 | 0.0184 | 0.0071 | 0.0128 | 0.0054 | 0 | 0.0200 | 0.0085 | 0.0066 | 0.0155 | 0.0110 |
P10 | 0.0230 | 0.0142 | 0.0255 | 0.0109 | 0 | 0.0160 | 0.0085 | 0.0022 | 0 | 0.0055 |
P11 | 0.0092 | 0.0035 | 0.0255 | 0.0054 | 0 | 0.0120 | 0.0057 | 0.0088 | 0.0086 | 0.0110 |
P12 | 0.0230 | 0.0106 | 0.0255 | 0.0163 | 0.0039 | 0.0200 | 0.0085 | 0 | 0.0121 | 0.0110 |
Parameters | s+i | s−i | CCi | Ranking |
Collaboration (P12) | 0.0162 | 0.0481 | 0.7485 | 1 |
Distribution & Logistic service (P6) | 0.0256 | 0.0472 | 0.6485 | 2 |
Customer (P10) | 0.0251 | 0.0431 | 0.6318 | 3 |
Contracting (P8) | 0.0266 | 0.0456 | 0.6313 | 4 |
Planning & Implementation (P1) | 0.0268 | 0.0453 | 0.6286 | 5 |
Knowledge & Training of fruit preservation (P2) | 0.0251 | 0.0389 | 0.6079 | 6 |
Technology adoption (P9) | 0.0279 | 0.0382 | 0.5777 | 7 |
Farming area/ farmer (P3) | 0.0326 | 0.0445 | 0.5772 | 8 |
Product quality & Safety (P7) | 0.0339 | 0.0407 | 0.5453 | 9 |
Warehouse/Storage (P5) | 0.0317 | 0.0346 | 0.5214 | 10 |
Organizational social responsibilities (P11) | 0.0330 | 0.0350 | 0.5151 | 11 |
Processing & Packaging (P4) | 0.0449 | 0.0300 | 0.4006 | 12 |
Coding | Parameters | Brief Description | Sources |
P1 | Planning & Implementation | The optimal design of the fresh fruit supply chain is inevitable, and it requires proper planning and implementation. | [4,7,20,21,22] |
P2 | Knowledge & Training of fruit preservation | The preservation of post-harvest products needs to apply the latest knowledge and disseminate it to the stakeholders of the chain, particularly in farms and micro corporates. | [4,23,24,25] |
P3 | Farming area/ farmer | As the first stage of the food supply chain, the essential role of farms to provide fresh products to the wholesalers, factories, and end customers. | [5,15,26,27,28] |
P4 | Processing & Packaging | Fresh products need to maintain optimum quality for the longest time. Therefore, standardization and synchronization of processing and packaging must be attention for all the stages. | [4,20,29,30,31] |
P5 | Warehouse/Storage | Warehouse acts as a transit station in the middle of the food supply chain, the quality of products will be significantly affected by storage operation in warehouses. | [18,24,30,32] |
P6 | Distribution & Logistics service | The smooth flow of goods in the food supply chain will depend heavily on distribution channels and logistics services. | [5,18,33,34] |
P7 | Product quality & Safety | Fresh goods have stringent requirements for standard product quality and safety. It is the participation of the whole chain and must be guaranteed from the stage of planting and harvesting to the end stage. | [4,35,36] |
P8 | Contracting | Offering a variety of contracts for choose to ensure the benefits of each counterpart in the food supply chain. Thereby, it helps to reduce risks and conflicts among members. | [12,18,37,38] |
P9 | Technology adoption | Adopting the latest technology to the supply chain is part of the stakeholders' efforts to obtain sustainability, such as minimizing the impact on the natural environment, reducing costs. | [39,40,41] |
P10 | Customer | As the end-stage in the supply chain and consume products, the perceptions and reactions of this group is the driver and will significantly impact the sustainability initiatives of other stakeholders. | [20,34,42,43] |
P11 | Organizational social responsibilities | Conducting social programs for the local communities has been launched in the sustainable development goals. Forcing the various organization to perform. | [1,21,44,45] |
P12 | Collaboration | Collaboration among supply chain members would reinforce sustainability and obtain better performance by reducing financial constraints and production scale. | [5,6,31,46,47]. |
Category | Frequency | Percentage | |
Gender | Male | 7 | 70.0% |
Female | 3 | 30.0% | |
Age group | 31-40 | 3 | 30.0% |
41-50 | 5 | 50.0% | |
51-60 | 1 | 10.0% | |
over 60 | 1 | 10.0% | |
Degree | Bachelor degree | 4 | 40.0% |
Master degree | 4 | 40.0% | |
Ph.D. degree | 2 | 20.0% | |
Organizational | Process Manufacturing | 6 | 60.0% |
Service | 4 | 40.0% | |
Working Position | Top Managers | 3 | 30.0% |
Sales Department | 3 | 30.0% | |
Financial Management | 1 | 10.0% | |
Human Resource Management | 2 | 20.0% | |
Production Management | 1 | 10.0% |
Parameters | E1 | E2 | E3 | E4 | E5 | E6 | E7 | E8 | E9 | E10 |
Weight | 0.13 | 0.10 | 0.18 | 0.12 | 0.11 | 0.11 | 0.06 | 0.05 | 0.05 | 0.09 |
P1 | 0.0414 | 0.0319 | 0.0574 | 0.0489 | 0.0315 | 0.0160 | 0.0142 | 0.0177 | 0.0052 | 0.0276 |
P2 | 0.0322 | 0.0283 | 0.0574 | 0.0381 | 0.0315 | 0.0279 | 0.0142 | 0.0132 | 0.0173 | 0.0221 |
P3 | 0.0460 | 0.0212 | 0.0574 | 0.0272 | 0.0276 | 0.0359 | 0.0142 | 0.0132 | 0.0138 | 0.0166 |
P4 | 0.0184 | 0.0319 | 0.0319 | 0.0272 | 0.0394 | 0.0279 | 0.0170 | 0.0155 | 0.0173 | 0.0276 |
P5 | 0.0322 | 0.0177 | 0.0447 | 0.0326 | 0.0355 | 0.0319 | 0.0227 | 0.0132 | 0.0173 | 0.0276 |
P6 | 0.0414 | 0.0319 | 0.0574 | 0.0272 | 0.0315 | 0.0359 | 0.0198 | 0.0110 | 0.0173 | 0.0276 |
P7 | 0.0414 | 0.0354 | 0.0319 | 0.0326 | 0.0315 | 0.0359 | 0.0142 | 0.0110 | 0.0173 | 0.0276 |
P8 | 0.0414 | 0.0354 | 0.0574 | 0.0272 | 0.0355 | 0.0319 | 0.0113 | 0.0132 | 0.0138 | 0.0276 |
P9 | 0.0368 | 0.0248 | 0.0447 | 0.0326 | 0.0276 | 0.0359 | 0.0198 | 0.0177 | 0.0173 | 0.0276 |
P10 | 0.0414 | 0.0319 | 0.0574 | 0.0381 | 0.0276 | 0.0319 | 0.0198 | 0.0132 | 0.0017 | 0.0221 |
P11 | 0.0276 | 0.0212 | 0.0574 | 0.0326 | 0.0276 | 0.0279 | 0.0170 | 0.0199 | 0.0104 | 0.0276 |
P12 | 0.0414 | 0.0283 | 0.0574 | 0.0435 | 0.0315 | 0.0359 | 0.0198 | 0.0110 | 0.0138 | 0.0276 |
Ideal Solutions | E1 | E2 | E3 | E4 | E5 | E6 | E7 | E8 | E9 | E10 |
Positive (A+) | 0.0460 | 0.0354 | 0.0574 | 0.0489 | 0.0394 | 0.0359 | 0.0227 | 0.0199 | 0.0173 | 0.0276 |
Negative (A−) | 0.0184 | 0.0177 | 0.0319 | 0.0272 | 0.0276 | 0.0160 | 0.0113 | 0.0110 | 0.0017 | 0.0166 |
Parameters | E1 | E2 | E3 | E4 | E5 | E6 | E7 | E8 | E9 | E10 |
P1 | 0.0046 | 0.0035 | 0 | 0 | 0.0079 | 0.0200 | 0.0085 | 0.0022 | 0.0121 | 0 |
P2 | 0.0138 | 0.0071 | 0 | 0.0109 | 0.0079 | 0.0080 | 0.0085 | 0.0066 | 0 | 0.0055 |
P3 | 0 | 0.0142 | 0 | 0.0218 | 0.0118 | 0 | 0.0085 | 0.0066 | 0.0035 | 0.0110 |
P4 | 0.0276 | 0.0035 | 0.0255 | 0.0218 | 0 | 0.0080 | 0.0057 | 0.0044 | 0 | 0 |
P5 | 0.0138 | 0.0177 | 0.0128 | 0.0163 | 0.0039 | 0.0040 | 0 | 0.0066 | 0 | 0 |
P6 | 0.0046 | 0.0035 | 0 | 0.0218 | 0.0079 | 0 | 0.0028 | 0.0088 | 0 | 0 |
P7 | 0.0046 | 0 | 0.0255 | 0.0163 | 0.0079 | 0 | 0.0085 | 0.0088 | 0 | 0 |
P8 | 0.0046 | 0 | 0 | 0.0218 | 0.0039 | 0.0040 | 0.0113 | 0.0066 | 0.0035 | 0 |
P9 | 0.0092 | 0.0106 | 0.0128 | 0.0163 | 0.0118 | 0 | 0.0028 | 0.0022 | 0 | 0 |
P10 | 0.0046 | 0.0035 | 0 | 0.0109 | 0.0118 | 0.0040 | 0.0028 | 0.0066 | 0.0155 | 0.0055 |
P11 | 0.0184 | 0.0142 | 0 | 0.0163 | 0.0118 | 0.0080 | 0.0057 | 0 | 0.0069 | 0 |
P12 | 0.0046 | 0.0071 | 0 | 0.0054 | 0.0079 | 0 | 0.0028 | 0.0088 | 0.0035 | 0 |
Parameters | E1 | E2 | E3 | E4 | E5 | E6 | E7 | E8 | E9 | E10 |
P1 | 0.0230 | 0.0142 | 0.0255 | 0.0218 | 0.0039 | 0 | 0.0028 | 0.0066 | 0.0035 | 0.0110 |
P2 | 0.0138 | 0.0106 | 0.0255 | 0.0109 | 0.0039 | 0.0120 | 0.0028 | 0.0022 | 0.0155 | 0.0055 |
P3 | 0.0276 | 0.0035 | 0.0255 | 0 | 0 | 0.0200 | 0.0028 | 0.0022 | 0.0121 | 0 |
P4 | 0 | 0.0142 | 0 | 0 | 0.0118 | 0.0120 | 0.0057 | 0.0044 | 0.0155 | 0.0110 |
P5 | 0.0138 | 0 | 0.0128 | 0.0054 | 0.0079 | 0.0160 | 0.0113 | 0.0022 | 0.0155 | 0.0110 |
P6 | 0.0230 | 0.0142 | 0.0255 | 0 | 0.0039 | 0.0200 | 0.0085 | 0 | 0.0155 | 0.0110 |
P7 | 0.0230 | 0.0177 | 0 | 0.0054 | 0.0039 | 0.0200 | 0.0028 | 0 | 0.0155 | 0.0110 |
P8 | 0.0230 | 0.0177 | 0.0255 | 0 | 0.0079 | 0.0160 | 0 | 0.0022 | 0.0121 | 0.0110 |
P9 | 0.0184 | 0.0071 | 0.0128 | 0.0054 | 0 | 0.0200 | 0.0085 | 0.0066 | 0.0155 | 0.0110 |
P10 | 0.0230 | 0.0142 | 0.0255 | 0.0109 | 0 | 0.0160 | 0.0085 | 0.0022 | 0 | 0.0055 |
P11 | 0.0092 | 0.0035 | 0.0255 | 0.0054 | 0 | 0.0120 | 0.0057 | 0.0088 | 0.0086 | 0.0110 |
P12 | 0.0230 | 0.0106 | 0.0255 | 0.0163 | 0.0039 | 0.0200 | 0.0085 | 0 | 0.0121 | 0.0110 |
Parameters | s+i | s−i | CCi | Ranking |
Collaboration (P12) | 0.0162 | 0.0481 | 0.7485 | 1 |
Distribution & Logistic service (P6) | 0.0256 | 0.0472 | 0.6485 | 2 |
Customer (P10) | 0.0251 | 0.0431 | 0.6318 | 3 |
Contracting (P8) | 0.0266 | 0.0456 | 0.6313 | 4 |
Planning & Implementation (P1) | 0.0268 | 0.0453 | 0.6286 | 5 |
Knowledge & Training of fruit preservation (P2) | 0.0251 | 0.0389 | 0.6079 | 6 |
Technology adoption (P9) | 0.0279 | 0.0382 | 0.5777 | 7 |
Farming area/ farmer (P3) | 0.0326 | 0.0445 | 0.5772 | 8 |
Product quality & Safety (P7) | 0.0339 | 0.0407 | 0.5453 | 9 |
Warehouse/Storage (P5) | 0.0317 | 0.0346 | 0.5214 | 10 |
Organizational social responsibilities (P11) | 0.0330 | 0.0350 | 0.5151 | 11 |
Processing & Packaging (P4) | 0.0449 | 0.0300 | 0.4006 | 12 |