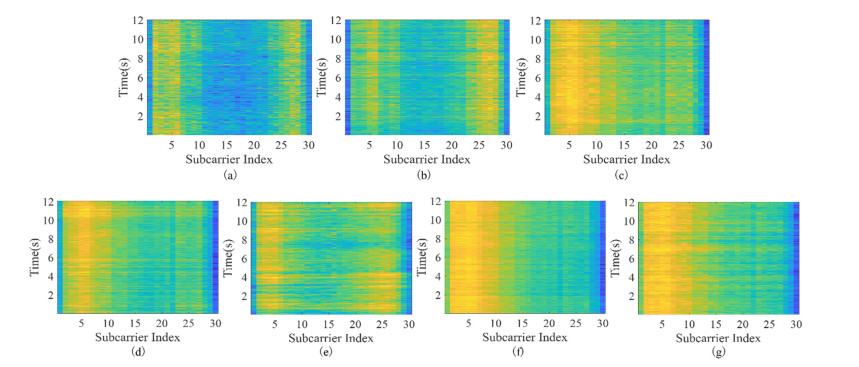
Citation: Qing Zhang, Yixiang Li, Yajun Li, Xiaodong Yang, Qammer Hussain Abbasi. Monitoring of neck activity for early warning of cervical spondylosis[J]. Mathematical Biosciences and Engineering, 2022, 19(1): 792-811. doi: 10.3934/mbe.2022036
[1] | Huihao Wang, Kuan Wang, Zhen Deng, Xiaofei Li, Yi-Xian Qin, Hongsheng Zhan, Wenxin Niu . Effects of facet joint degeneration on stress alterations in cervical spine C5–C6: A finite element analysis. Mathematical Biosciences and Engineering, 2019, 16(6): 7447-7457. doi: 10.3934/mbe.2019373 |
[2] | Rui Zhu, Yuhang Chen, Qianqian Yu, Siqing Liu, Jianjie Wang, Zhili Zeng, Liming Cheng . Effects of contusion load on cervical spinal cord: A finite element study. Mathematical Biosciences and Engineering, 2020, 17(3): 2272-2283. doi: 10.3934/mbe.2020120 |
[3] | Nengkai Wu, Dongyao Jia, Chuanwang Zhang, Ziqi Li . Cervical cell extraction network based on optimized yolo. Mathematical Biosciences and Engineering, 2023, 20(2): 2364-2381. doi: 10.3934/mbe.2023111 |
[4] | Eminugroho Ratna Sari, Fajar Adi-Kusumo, Lina Aryati . Mathematical analysis of a SIPC age-structured model of cervical cancer. Mathematical Biosciences and Engineering, 2022, 19(6): 6013-6039. doi: 10.3934/mbe.2022281 |
[5] | Caiyun Wu, Cong Ma, Jing Yuan, Pei Zhou . Exploration of potential therapeutic and prognostic value of CXC chemokines in cervical squamous cell carcinoma and endocervical adenocarcinoma based on bioinformatics analysis. Mathematical Biosciences and Engineering, 2021, 18(6): 8201-8222. doi: 10.3934/mbe.2021407 |
[6] | Suxiang Yu, Shuai Zhang, Bin Wang, Hua Dun, Long Xu, Xin Huang, Ermin Shi, Xinxing Feng . Generative adversarial network based data augmentation to improve cervical cell classification model. Mathematical Biosciences and Engineering, 2021, 18(2): 1740-1752. doi: 10.3934/mbe.2021090 |
[7] | S. M. Crook, M. Dur-e-Ahmad, S. M. Baer . A model of activity-dependent changes in dendritic spine density and spine structure. Mathematical Biosciences and Engineering, 2007, 4(4): 617-631. doi: 10.3934/mbe.2007.4.617 |
[8] | Simphiwe M. Simelane, Justin B. Munyakazi, Phumlani G. Dlamini, Oluwaseun F. Egbelowo . Projections of human papillomavirus vaccination and its impact on cervical cancer using the Caputo fractional derivative. Mathematical Biosciences and Engineering, 2023, 20(7): 11605-11626. doi: 10.3934/mbe.2023515 |
[9] | Vitalii V. Akimenko, Fajar Adi-Kusumo . Stability analysis of an age-structured model of cervical cancer cells and HPV dynamics. Mathematical Biosciences and Engineering, 2021, 18(5): 6155-6177. doi: 10.3934/mbe.2021308 |
[10] | Swati Shinde, Madhura Kalbhor, Pankaj Wajire . DeepCyto: a hybrid framework for cervical cancer classification by using deep feature fusion of cytology images. Mathematical Biosciences and Engineering, 2022, 19(7): 6415-6434. doi: 10.3934/mbe.2022301 |
Cervical spondylosis, also known as cervical syndrome, is the general name of cervical osteoarthritis, proliferative cervical spondylitis, cervical nerve root syndrome and cervical disc herniation. It is a disease based on degenerative pathological changes. It is mainly due to long-term cervical strain, hyper osteogeny, or intervertebral disc prolapse and ligament thickening, resulting in compression of cervical spinal cord, nerve root or vertebral artery, resulting in a series of clinical syndromes of dysfunction. It is characterized by instability and loosening of vertebral segments; Protrusion or prolapse of nucleus pulposus; Bone spur formation; Ligament hypertrophy and secondary spinal stenosis stimulate or compress adjacent nerve roots, spinal cord, vertebral artery and cervical sympathetic nerve, resulting in a series of symptoms and signs. Long-term poor neck posture will lead to heavy neck compression, resulting in cervical vertebral strain, which would cause increasing probability of cervical vertebral disease. In the early days, a protractor is usually used to measure the Range of Motion of Cervical Spine for different people [1]. In addition, researchers have successively used triaxial angular acceleration sensors [2,3], infrared cameras [4], CT equipment, etc., to determine the posture and range of motion of the cervical spine. In recent years, the prevalence of cervical spondylosis is increasing, and the average age of onset is decreasing year by year [5]. Loss of range of motion in the neck is the most common sign in the diagnosis of cervical spondylosis. Excluding the effect of trauma, chronic strain is one of the main pathogenesis of cervical spondylosis [6]. The poor posture of the cervical spine leads to the compression of the cervical spine under a large amount of pressure for a long time [7], which is an important cause of chronic strain. In the process of preventing cervical spondylosis, it is very necessary to recognize each abnormal posture. In the past, wearable sensor-based systems required users to carry additional devices for activity identification. In this context, the research on Contactless neck movement and posture perception based on RF signal has practical significance. High repetitive use of muscle can lead to muscle fiber injury, acute cumulative trauma injury or myogenic oral muscle injury. These injuries often occur in the neck and shoulder [8], repetitive and cumulative trauma to the neck and shoulders can lead to forward head posture (FHP) [9], this is a special musculoskeletal postural abnormality. FHP weakens the deep cervical flexor, rhomboid and inferior trapezius muscles, it also shortens the pectoralis major and neck extensors; as a result, the patient will have symptoms of muscle pain. Long term incorrect neck posture will lead to abnormal neck bending and neck muscle fatigue [9], which would cause abnormal curvature of cervical spine, resulting in early lesions of cervical spine. In clinical practice, this abnormal cervical posture can be corrected or even avoided through effective cervical posture monitoring and treatment intervention. At present, some methods have been able to obtain the bad postures of the neck, the routine measurement method in clinical evaluation is to assess them using goniometer [10]. Active neck motion tests are usually used for evaluation, includes neck flexion and extension movement, neck shoulder tilt movement and left right neck rotation movement. It is worth mentioning that this scheme requires the cooperation of professional doctors for diagnosis. Inokuchi et al. used the Vicon three-dimensional motion analysis system [11], good results have been achieved; based on this, some researchers have used triaxial angular acceleration sensors to monitor the range of motion and posture of cervical spine [3]. At present, there are two main non-contact methods: one is computer vision system [12], e.g., using infrared cameras or Kinect devices [13] to get data, the performance of these devices may be affected by low light environment; the other approach is to consider RF perception. In reference [13], researchers use Kinect to quickly and accurately obtain the three-dimensional coordinates of the subject's face and bone joints, these coordinates can be combined with bone function to judge the flexion and extension movement of the head. This measurement method can improve the measurement efficiency and reduce the measurement error caused by instrument and operation. This method based on wireless signal sensing first appeared in the research of non-contact sensing based on radio frequency signal (RF) proposed by Woyach et al. in 2006 [14]. Researchers found that human activities will lead to fluctuations and changes in the signal in the wireless channel, that is, human activities in the wireless channel environment will affect the multipath superimposed signal received by the signal receiver; further research shows that the affected multipath signal can carry the environmental characteristic information reflecting the activity [14]. Later, Radio Frequency Identification and Ultra-wideband Bandwidth radar are also used to achieve human activity recognition. The research of RFID technology appeared earlier, the research of Harry et al. In the 1950s can be regarded as the theoretical support of RFID [15]. Later, researchers proposed to use RFID to realize data exchange. They first formulated RFID tags, then tested the signal fluctuations generated by different kinds of targets approaching or passing through the tags, and calibrated different activity trajectories through these fluctuations [16]. UWB technology has a long history, which can be traced back to the era of the invention of wireless telegraph technology a hundred years ago. With the increasing progress of science and technology, this technology is gradually applied to remote communication, target location and recognition and other fields. UWB has the characteristics of short pulse, and can still have high resolution and stability in complex environment, so it can be better applied to human activity recognition. The perception radar based on UWB can track human movement and obtain human respiratory rate [17]. Katabi et al. [18] used the milliwatt radio frequency signal to complete the centimeter level through wall perception under the condition of non-line-of-sight, which can be used for human activity tracking. By using MIMO and orthogonal frequency division multiplexing (OFDM) technologies, the channel state information between each transmitting and receiving antenna is obtained at each carrier frequency. This channel state information brings a lot of environmental information, which can be applied to the field of medical monitoring. Some other studies also provide ideological support for the development of this work [19,20,21,22,23].
Electromagnetic wave propagation in wireless channel will be affected by various obstacles, so it will produce "fading", also known as shadow fading. According to different causes, it is usually divided into large-scale and small-scale fading. Considering the random characteristics of fading, that is, large-scale fading and small-scale fading are random phenomena, it is very necessary to increase a certain power reserve to ensure that the signal strength reaches the desired level, which requires link budget. When designing a wireless communication system, it is necessary to determine the fading margin so that the probability that the power of the received signal is greater than the given threshold is within the target range. For large-scale fading, free space propagation model is generally used to predict the strength of received signals in Los environment. It can be expressed by Friis formula as:
Pr(d)=PtGtGrλ2(4π)2d2L, | (1) |
where, 𝑑 represents the distance between the transmitter and the receiver, the power of the received signal is inversely proportional to the square of the distance of the transceiver, the square of transmitting power, transmitting antenna gain, receiving antenna gain and transmitting wavelength is directly proportional to the distance. 𝐿 represents the system loss coefficient, reflecting the overall loss of the system in terms of its own antenna and other hardware. In general, 𝐿 > 1. Let 𝐿 = 1; Substitute the above formula to obtain the free space path loss without space loss in the ideal hardware loss free system:
PLF(d)[dB]=10log10(PtPr)=−10log10PtGtGrλ2(4π)2d2. | (2) |
The free space path loss without antenna gain can be simplified as:
PLF(d)[dB]=10log10(PtPr)=20log104πdλ. | (3) |
It can be seen that the power of the received signal decreases as the distance decreases. In the daily practical application environment, the path loss index is often introduced to modify the model to make the model more appropriately reflect the signal propagation in the actual environment. The modified model formula is:
PLLD(d)[dB]=PLF(d0)+10nlog10(dd0). | (4) |
In the actual environment, it is also necessary to consider the placement position of the receiver, and different losses will occur when the signal propagates on different paths. Therefore, in the actual environment, lognormal shadow model is more used. The formula is as follows:
PL(d)[dB]=−PL(d)+Xσ=PLF(d0)+10nlog10(dd0)+Xσ, | (5) |
where, the last term represents a normal random variable with standard deviation 𝜎 and its value changes with the change of environment.
In the actual measurement environment, we often encounter more complex experimental scenes, especially in the indoor environment measurement, the wireless signal will be affected by a variety of obstacles in the propagation process. Affected by this, the wireless signal will have three physical phenomena: reflection, diffraction and scattering. At this time, the signal received by the receiver is the result of the superposition of multiple paths of the signal transmitted by the transmitter, that is, multipath fading, which belongs to small-scale fading. As a kind of electromagnetic wave, wireless signal is generally considered to propagate in a straight line in the free air due to the characteristics of electromagnetic wave. Therefore, the propagation characteristics of wireless signal in indoor environment can be obtained, and the theoretical basis of monitoring using wireless signal can be obtained. In a closed free environment, the transmission loss of wireless signal can be reflected by the following formula:
Lbf(dB)=32.45+20×logd+20×logf. | (6) |
The formula shows that when a wireless signal propagates in indoor free space, its loss will only be affected by two variables: propagation distance (unit: km) and electromagnetic wave frequency corresponding to wavelength (unit: MHz).
Based on the propagation principle of wireless signal in the indoor environment described above, according to the influence of indoor walls, furniture and other factors on signal propagation in the actual environment, it can be concluded that there are two main modes of wireless signal propagation in the common indoor environment: line of sight propagation and multi-path propagation. Multipath propagation in indoor environment is not only affected by fixed obstacles, but also affected by human activities. Therefore, the path fading law of wireless signal will change. In particular, human behavior will lead to multipath fading and Doppler shift, so that the received signal contains rich information of human activity environment. With the continuous development of modern wireless communication technology, people communicate and share resources more frequently. WLAN is a combined application of wireless communication technology and Internet technology. It can meet more and more interactive needs. Its general standard is IEEE802.11 standard [24]. The first version of IEEE802.11 standard was released in 1997. Its origin can be traced back to the unauthorized ISM band issued by the Federal Communications Commission in 1985. At present, several modified versions of IEEE802.11 standard are in use. IEEE802.11 hardware has backward compatibility, that is, old and new devices can be interconnected. When new devices are connected to old devices, there will only be a problem of rate reduction.
At present, most routers used by people support IEEE802.11ac network standard. The multi station throughput of this standard is 1 gigabit per second, and the single link throughput is at least 500 Mbit/s; Some old routers support 802.11n network standard, and their maximum net data rate is 600 Mbit/s. In this experiment, IEEE802.11n standard is selected. It was officially approved in 2009. New concepts such as MIMO and OFDM are added. Multiple transceiver antennas are used to achieve higher data transmission rate. At the same time, Alamouti's space-time block code proposed in 1998 is used to increase the transmission range.
The channel attribute of the communication link between the sender and the receiver in the wireless channel is the so-called channel state information. CSI belongs to the physical layer in OSI classification. In the early channel research, RSSI is usually used as an index to judge the received signal strength, which can reflect the channel quality to a certain extent. In most cases, RSSI can be brought into the propagation model of wireless signal to calculate the length of signal propagation, and RSSI can also be used to calibrate the wireless signal characteristics of specific places, so as to judge whether the wireless link is blocked by people or obstacles. The accuracy of RSSI in indoor environment will be limited by the small-scale fading caused by multipath propagation. At the same time, multipath propagation will also affect the amplitude of RSSI and make it fluctuate greatly. The research shows that in the laboratory environment, the stationary receiver will fluctuate by 5 dB per minute, which will lead to abnormal matching of wireless signal positioning. The characteristics of RSSI determine that it cannot distinguish different propagation paths of each signal, which limits the reliability of RSSI in application. Channel impulse response can accurately reflect the basic characteristics of the channel, it is usually modeled by wireless channel to describe the characteristics of multipath propagation. CIR is usually expressed as:
h(τ)=∑Ni=1aie−jθiδ(τ−τi), | (7) |
the formula is carried out under linear time invariant conditions, where 𝑁 is the total number of propagation paths, the formula also includes the amplitude attenuation, phase offset and time delay parameters of the signal. The formula can show the time-domain characteristics of amplitude, phase and time delay of any signal in the propagation path. The characteristics of CIR in frequency domain can be obtained by Fourier transform, and the channel frequency response in frequency domain can be obtained by transform:
H(f)=FT[h(τ)]=∑Ni=1aie−jθi+2πfτi. | (8) |
The CFR obtained by this formula can reflect the size of the signal with delay characteristics and power spectral density.
Use the equipment supporting IEEE802.11n standard to obtain CFR in the form of CSI, which uses OFDM technology and MIMO system in the process of adoption. The frequency domain model of a MIMO system with narrowband smooth fading can be expressed as:
Y=H×X+N, | (9) |
where, Y represents the received signal vector, X represents the signal vector of the transmitted signal, N represents the noise signal vector between the transmitted signal and the received signal, H represents the actual channel frequency response, and represents the channel state matrix in this model. From a practical point of view, a set of CSI data can be obtained from each received packet by using an IEEE802.11n compatible device. According to different subcarrier groups of IEEE802.11n, the index of subcarriers is obtained, and the amplitude and phase of each orthogonal frequency division multiplexing subcarrier are obtained by using the modulus method. In the research, the way of adjusting the hardware equipment is usually used to enable the traditional wireless propagation equipment to collect CFR sampling data on 30 OFDM subcarriers. It can be seen from the above that there will be multipath transmission phenomenon when the wireless signal propagates indoors, and the change of propagation path will lead to the change of signal amplitude and phase. In the actual environment, the sub signal will pass through different paths in the process of propagation, so there will be different delays when reaching the receiver, and the propagation attenuation in this process is also different. RSSI will change with the amplitude and phase of wireless signal, and these characteristics will change due to the change of propagation path when the signal propagates in a certain path. The RSSI data received in the study is not only related to the distance between the transmitter and the receiver, but also related to the path loss index in path propagation and environmental random variables. Considering the influence of multipath effect, the total amount of multiple signals in different phases is the received signal at the receiving end. When the phases are the same, the RSSI will be enhanced, while the opposite phase will attenuate the RSSI. CSI can obtain the channel state of multiple subcarriers at the same time, and can show the amplitude and phase of different frequency signals in multipath propagation environment. CSI can describe the special frequency response of each individual subcarrier, and CSI brings the expansion of signal information capacity; In addition, CSI can distinguish the amplitude and phase data of different subcarriers, which provides finer and more robust channel information characteristics for research.
So far, MIMO-OFDM system is mostly used for CSI extraction. Using MIMO technology can significantly improve the communication capacity, so the receiver can receive more channel information and obtain better sensing effect. The biggest advantage of OFDM technology is that it can overlap adjacent carriers. At the same time, when the number of subcarriers is large, it can have high bandwidth efficiency [25]. MIMO systems usually use two technologies: diversity technology and spatial multiplexing technology. This system can not only transmit and process the same data stream on different antennas to ensure timeliness and accuracy, but also improve the communication rate through the antenna group composed of multiple transceiver antennas. Based on these two advantages, the system significantly reduces the data packet loss caused by poor channel [26]. The multi scattering of MIMO system makes the matrix row and column uncorrelated and the channel matrix reversible. With the continuous development of communication technology, OFDM technology has been widely used in a variety of communication protocols. It overlaps the subcarrier spectrum, which solves the problem of poor spectrum utilization caused by different carriers isolated from each other and occupying a certain frequency band in the traditional frequency division multiplexing technology. Based on Nyquist criterion, OFDM technology generates orthogonal signals in the frequency domain in which adjacent carriers can be superimposed and do not interfere with each other. These orthogonal signals are processed by discrete Fourier transform and inverse discrete Fourier transform.
The concept of Fresnel zone was developed from the study of light interference and diffraction in the early 19th century [27]. Andre et al. [28] first applied this concept to the study of radio propagation in 1936. After that, Fresnel region gradually plays an important role in the research of microwave propagation, wireless base station location and antenna design [29]. In the research of wireless communication field, an ellipse is usually used to describe the Fresnel region, and the transmitting and receiving equipment of wireless signal is its focus. For objects with different motion states (including static and dynamic), their influence on the received wireless signal is different in the Fresnel region. From the above analysis, when the static object is on the boundary of odd Fresnel region, the reflected signal and LOS signal have the same phase, then the received signal is the enhanced signal; When the static object is on the boundary of even Fresnel region, the reflected signal and LOS signal have reverse phase, then the received signal is the weakened signal. According to the basic interference principle, when the object moves along the ellipse, the received signal will remain unchanged; When the object continuously passes through the boundary of Fresnel region, the peaks or troughs of the received signal appear alternately. From the above description, it can be seen that in a typical environment, the transmitted signal reaches the receiving equipment through multipath, the received signal will be obtained after multipath superposition, and the different paths of multipath process can be divided into static or dynamic paths. The fluctuation of the received signal is attributed to the interference result of the linear superposition of the human body reflected signal and the environmental multipath signal. According to the Fresnel zone perception theory, we can correctly apply CSI data and correctly analyze the principle and mechanism of CSI waveform change caused by human body in Fresnel zone. For neck monitoring, there are not only the static shape of neck posture, but also the dynamic change of neck activity. Therefore, the Fresnel region CSI perception model can better analyze neck posture, and it is an important theoretical basis for this paper.
Each data in the channel matrix is complex, and the amplitude of CSI can be easily obtained. When there is no moving object, the static wireless channel remains relatively stable. When people perform neck bending activities, the subcarrier amplitude changes significantly. Therefore, the information brought by CSI amplitude waveform transformation is large enough to ensure relative stability in the static environment and relative sensitivity in the dynamic environment. The different neck movement information contained in it is relatively easy to extract. In conclusion, the amplitude data obtained from the original CSI channel matrix can be used as an important feature of neck activity monitoring. There are three main problems with phase data: (1) the main cause of sampling frequency deviation is the asynchronous interference between receiver and transmitter, which usually refers to noise interference. In this case, the oscillator frequency shift at the transceiver will be caused, resulting in the change of sampling frequency. The number of sampling points at the transmitting end will be less than that at the receiving end, which will further lead to a time shift between the received signal and the transmitted signal, and the resulting deviation will change the phase in CSI; (2) in OFDM system, some selected training symbols are often used for symbol timing synchronization, that is, certain calculation and power detection are used to test data packets. In the test process, symbol timing deviation is introduced to make up for some hardware problems. If the deviation is not introduced, the data will be wrong, and the data closer to the end of the packet is more prone to error. The introduction of inappropriate deviation will lead to the rotation change of CSI phase; (3) there will also be deviation between the local carriers of the transceiver, resulting in the error of the carrier center frequency of the transceiver. In the wireless communication system, the frequency shift of wireless signal is very easy to occur in the process of propagation. Even if the receiver uses cyclic prefix and pilot to estimate and make up the carrier frequency offset, the firmware instability makes the signal frequency shift impossible to determine, which will have a great impact on the phase of CSI. The feature selection of CSI is to make the neck posture monitoring system simpler and more efficient. As the CSI feature selected in this paper, amplitude can not only remove unnecessary interference, but also accurately reflect the changes of the environment. In this paper, amplitude information is selected as the feature to monitor neck movement posture.
Affected by the noise in the natural environment, there are always some abnormal values in the collected CSI data, which are also called discrete values. In addition to the influence of noise, the influence of some unknown burst factors and hardware equipment factors in the actual environment should be considered. Abnormal values caused by these factors will seriously affect the collected signal data, reduce the accuracy of data, and lead to monitoring failure. Therefore, it is very necessary to deal with outliers caused by many factors before formal data processing. In this paper, Hampel [30] filtering algorithm is used to identify and deal with exceptions value. As a filter that can be used in time series, Hampel filter can not only identify outliers, it can also be replaced with more representative values. The filtering method uses a filter that can be used in time series. The window in the signal data is composed of each selected sample point and its left and right, consisting of three samples on each side. The sample is used as the median of the window, and the standard of each sample to the median is estimated difference. If a sample differs from the median by more than 3 standard deviations, the median is used to replace that sample.
In the actual environment, the signals collected are often subject to a lot of interference and produce a lot of noise. Using filtering technology to extract useful signals can significantly reduce or even eliminate noise [31]. The neck activity monitoring in this paper has environmental interference, and the CSI data is greatly affected by environmental noise, resulting in a great impact on the acquisition of useful information in neck activity monitoring. It is necessary to filter the CSI data. People use signal sources to send useful information. In the process of signal change and transmission, the noise caused by control factors, natural factors or subjective factors and the superposition of these noises make it difficult to distinguish signals from each other. The signal superposition caused by these factors must be strictly eliminated before processing the signal data, so that the correct signal information can be obtained and used accurately. The common processing method is to use filtering technology. If the noise is small, some signal smoothing methods will be used to eliminate burrs.
The traditional denoising method using filter completely separates the noise and useful signal in the frequency domain. This method can be realized only if there is no overlap between the spectrum of noise and useful signal. However, in practice, the signal spectrum and noise spectrum often overlap, while for the common Gaussian white noise, its spectrum often exists in all frequency domains. Therefore, the denoising smoothing effect of common denoising methods is often inversely proportional to the contour definition of the denoised signal; If the denoised signal is very smooth, its contour must be unclear; If the contour of the signal is clearly visible, the noise smoothing effect will be reduced. When choosing denoising, it is usually necessary to maintain a certain balance between the two in order to achieve the ideal denoising effect. Using traditional filters such as Butterworth filter to filter and remove the high-frequency noise in the signal is not applicable in this experiment, because they will blur the rising or falling edge in the received signal while eliminating the noise, but these data are important for monitoring and cannot be deleted. In conclusion, wavelet filtering is used to process the neck activity signal in this paper. This filtering method can well preserve the mutation of the neck activity signal.
Because the CSI waveform in this paper has the characteristics of short-time mutation, the signal can be processed effectively by using wavelet filtering method [32]. When wavelet filter decomposes the signal into high-frequency component and low-frequency component, its different components have opposite properties. The frequency resolution of high-frequency component is low, but the time resolution is high; The low-frequency component is in turn, so it is very suitable for analyzing abnormal signals with instantaneous changes in normal signals. Based on this feature, for low-frequency signals, it can take longer to make them more refined; For high-frequency signals, the signal information can be obtained in a short time. In the actual signal data application and processing, the signal data often contains more sudden changes, and it is often necessary to analyze the characteristics of local signals. Therefore, compared with the traditional Fourier change analysis, wavelet filtering has significantly better ability in stationary signals and is a powerful tool for non-stationary signal analysis. The discrete wavelet transforms selected in this paper is a commonly used dyadic wavelet transform, which can discretize the scale and time, and realize the decomposition and reconstruction of signals. In the decomposition process, the signal to be filtered passes through a group of decomposed low-pass filters and decomposed high-pass filters. The low-pass filter generates the low-frequency component of the corresponding signal, which is also called approximate component, and the high-frequency component generated by the corresponding high-pass filter is called detail component. In this paper, the amplitude information of CSI signal data is processed by wavelet analysis, and the reconstruction of CSI data uses discrete wavelet inverse change, because the CSI signal data is a low-frequency signal, which has certain continuous performance as a time-series signal. When the amplitude information is transferred to the wavelet domain, there may be large effective information coefficients, and the impact of environmental noise is discontinuous, so the wavelet coefficients of noise are small, so threshold denoising is needed before reconstruction. There are two ways to threshold wavelet: soft threshold and hard threshold. The difference between them lies in the different way of zeroing the wavelet coefficients. For most noises in wavelet domain, the noise coefficients are in a certain range according to the Gaussian distribution. The hard threshold directly sets all coefficients within this range to zero. This method has an obvious noise suppression effect, but it will cause certain damage to the effective part of the signal, and even make the denoised signal jitter, affecting the subsequent further analysis. In contrast, the signal after soft threshold denoising is smoother. Although the coefficient is also set to zero, it will consider different situations and select only a part for zeroing. In this paper, the detail coefficients of the collected wireless signals contain both useful information and information, it also contains noise, so the effect of soft threshold is stronger than hard threshold. Therefore, this paper selects the soft threshold method to filter data.
Firstly, the extraction of CSI amplitude data and phase data is introduced. Through further analysis, the reason for selecting amplitude as characteristic information is clarified, that is, CSI amplitude data can accurately reflect neck movement. Then, the original amplitude data of CSI can be analyzed by using two preprocessing methods: outlier processing and wavelet filtering.
The principle of this system is that when the wireless signal propagates in the indoor environment, there are a large number of multipath effects. After the CSI data carrying the indoor environment information is collected and processed by the system, it can determine the different active postures of the neck and classify various postures, so that the bad postures can be reminded in time.
Cervical spondylosis is the clinical manifestation of cervical degeneration and its secondary pathological changes and the surrounding tissue structure [5]. The incidence rate increases with age. In clinical diagnosis, the reduction of cervical range of motion is the most common sign. The commonly used diagnostic test in diagnosis is flexion and neck rotation test [5]. The common methods for measuring the range of motion of the neck are from using a protractor to gradually using X-ray examination, magnetic resonance imaging or other imaging examinations. Because the position of the cervical spine is between the head, chest and upper limbs, and the cervical spine in the spine is characterized by large weight-bearing, maximum activity frequency and flexibility, but the smallest volume, it is prone to strain and various injuries caused by load in daily activities. The basic causes of cervical spondylosis usually fall into three categories: (1) the degenerative change of cervical spondylosis, the overload use of cervical spine and the increasing age reduce the recovery ability of cervical spine, leading to the decline of cervical spine and structural decay; (2) cervical developmental spinal canal stenosis occurs mostly in the process of puberty, and the symptoms of flat vertebral arch development lead to abnormal spinal canal; (3) chronic strain, long-term activities beyond the tolerance of shoulder and neck, produces cumulative injury, which is different from obvious trauma and is often ignored. It is a common cause of cervical spondylosis, which has a direct impact on the follow-up development and treatment of cervical spondylosis. Medical studies have shown that when the head tilts forward to a deteriorating state, the pressure on the cervical spine is different gradually [7], which is necessary for cervical surgeons to diagnose the neck. The researchers created a cervical measurement model that can obtain actual values to calculate the pressure on the cervical spine. In the evaluation of the model, it shows that the pressure on the neck is different at different degrees of forward inclination. These pressures will cause heavy compression of the muscles around the cervical spine, leading to early neck wear, tear or degradation. At the same time, it will lead to the appearance of abnormal forward posture of the neck, make the neck bear a huge load for a long time, and accelerate the aging and wear of the cervical spine, which is also one of the causes of chronic strain.
Support vector machine is widely used in classification and recognition. Its basic principle is to find the best partition hyperplane in the feature space, so as to maximize the interval between positive and negative samples in the training set. Support vector machine can complete the flexible decision boundary in higher dimensions and optimize the training results [33]. In general, the following formula is usually used to indicate the way of dividing hyperplane in sample space:
ωTx+b=0. | (10) |
For the so-called "interval", it can be expressed as:
γ=2‖ω‖. | (11) |
Thus, the basic type of SVM can be described as:
min:12‖ω‖2.s.t.yi(w·xi+b)≥1,i=1,2⋯n. | (12) |
Lagrange multiplier method is applied to the formula to obtain its dual problem solution:
maxα∑mi=1αi−12∑mi=1∑mi=1αiαjyiyjxixj. | (13) |
Thus, when the KKT condition is satisfied, the solution of the original problem can be equivalent to the solution of the dual problem, that is, the solution of the above problem is transformed into the solution α, and the support vector is obtained. At the same time, kernel function is introduced to solve the problem that a suitable hyperplane cannot be found in the actual scene, so that it can accurately distinguish two kinds of different samples in the original sample space. The assumed equation is:
k(xi,xj)=〈Φ(x1),Φ(x2)〉. | (14) |
Through this formula, the inner product in high-dimensional or even infinite dimensional feature space is avoided.
K-nearest neighbor method, also known as K-nearest neighbor learning method, is a common supervised learning method and one of the simpler machine learning algorithms. K, as an important parameter, has a significant impact on the classification results. For a test sample, the distance measurement is obtained through analysis in a certain way, and then the K training samples closest to the test sample are obtained according to the distance measurement, and the information of the K samples is used for classification and prediction. In this paper, the method of weighted analysis is selected. After labeling the data according to the collected data set, the characteristic distance between the data to be classified and the training data is obtained. Select K closest data from them, and the sample corresponding to the data is the category with the most votes.
Convolutional neural network is a hierarchical model, which usually has a multi-layer structure. Convolution layer is the core layer of the model, which aims at feature extraction. The convolution kernel is used to slide, and all positions in the characteristic matrix are internally integrated with the local pixel values.
In the calculation of convolution layer, the parameter to be confirmed is the size of convolution kernel, hkernel is used here for its height and wkernel for its width. The height of the output matrix hout and width wout by the height of the input matrix hin and the width of the input matrix win and convolution kernels size to decide together, the calculation formula is as follows:
hout=(hin−hkernel+2∗padding)/stride+1, | (15) |
wout=(win−wkernel+2∗padding)/stride+1, | (16) |
where padding represents input filling, which is used to reduce the loss of corner information. Stride represents the step size, that is, the size of each sliding window movement. Pooling layer is an important layer in the model. Its functions mainly include reducing the amount of calculation, feature dimensionality reduction and over fitting. The use of the pooling layer is like the convolution layer, using the sliding window function to remove some useless information. The function of the whole junction layer is to connect all neurons in the upper and lower layers with weights, this layer is usually deployed at the end of the network model.
In this paper, a one-dimensional convolutional neural network is constructed. The different dimensions of convolutional neural network mainly differ in the data dimension and the different sliding modes of filter in the data. The wireless signal used in this paper is a one-dimensional time series signal, so a one-dimensional neural network model is proposed to analyze neck activity data. The data used in the analysis are from the data collected by the neck activity monitoring system described above. For the characteristics of amplitude information extracted in this paper, for amplitude, this paper selects "maximum amplitude", "minimum amplitude" and "average amplitude" are used to analyze the waveform of abnormal neck activity. For different experiments, other features are extracted respectively.
The first layer is the data input layer. The CSI amplitude data preprocessed above is input into the network. 1000 sampling points within 10 s are recorded in each experiment. In each different time slice, the collected wireless signal data is amplitude data, so the data format of the input layer is 1000 × 1.
The second layer is the convolution layer. The number of convolution kernels is set to 100, so 100 different features can be obtained through this layer calculation, and the length of convolution kernel is defined as 10. The data output through this layer is 991 × 100 Matrix. Relu is selected in the activation function, which can increase the sparsity of the network, make the extracted features more representative, and reduce the amount of data calculation. In addition, dropout is selected to reduce the synergy in the features, so as to better prevent over fitting. In this paper, the neuron weight in the network is randomly assigned to 0 according to 15% probability. The third layer is the pool layer. In this paper, maximum pooling is used to compress the input data, extract the main features and reduce over fitting. Considering the continuity of collection action in this paper, in order to avoid continuous data exceptions caused by over compression, the pool size selected in this paper is 3 and the pooled data format is 330 × 100. The fourth layer is the second convolution layer. The convolution kernel size of this layer is the same as that of the first convolution layer and is also set to 10 × 100, so the output data format obtained is 321 × 100. The effect of this layer is to enable the network to learn higher-level features. Similarly, the data input activation functions Relu and dropout layers are used to reduce the fitting, and the ratio of dropout random zeroing of this layer is set to 0.15. The fifth layer is the second pool layer. This layer also compresses the data of the previous layer. The pool core size is set to 3 and the output data is 107 × 100 Matrix. The sixth layer is the full connection layer. This layer is similar to an average pooling layer in setting, which pools the upper layer data evenly, that is, different feature extractors leave only one weight after passing through this layer, and the output data format is 1 × 100. The seventh layer is the output layer. This payer will compress the vector of upper layer (length: 100) into a vector with the length 4 or 7. The vector, with the length 7, represents for the classification result for 7 common neck postures; while the vector with the length 4 indicates the results of 4 different bending degrees. In this work, Softmax is used as the activation function, the sum of output is equal to 1.
After many experiments and analysis, this paper selects Adam as the optimizer, each training iteration is 300 times, and the ratio between training set and test set is 8:2. In this work, iteration is confirmed according to the experience based on the specific application. Adam is an update of RMSProp optimizer, the learning rate of each parameter is dynamically adjusted by using the first-order moment estimation and second-order moment estimation of gradient. The learning rate of each iteration has a clear range, so that the parameters change smoothly. It is the most commonly used optimization algorithm, and its effectiveness has been verified in a large number of deep neural network experiments.
In order to study the different postures corresponding to the three movements of routinely measuring the range of motion of the neck, the subjects sat at the table. In the experiment, they were asked to complete seven postures, including: normal posture, neck extension and bending posture, neck yaw, shoulder tilt posture and neck rotation posture. At the beginning of the experiment, the subjects were asked to sit straight and look forward to ensure that the head was consistent with the body in the vertical direction. After two seconds, the formal collection began. During collection, the subjects' trunk, shoulders and arms remained stationary, their legs were aligned, and their feet were flat on the ground. In the experimental parameter setting, the 5.24 GHZ frequency band is selected. The subjects sit on a chair with a height of 45 cm, the antenna is placed on the left and right sides of the subjects, the spacing is 85 cm, and the distance between the two antenna bases from the ground is 75 cm. In order to continuously obtain data, the system's packet rate is set to 100 packets per second to send data, and the receiving end continuously collects and saves data. In this experiment, one transmitting antenna and three receiving antennas are selected to obtain data, so three data streams will be obtained. The device can obtain 30 different subcarriers according to each data stream, so a total of 90 subcarriers can be obtained. Each subject completed a total of 20 acquisitions for each attitude, and a total of 126, 000 groups of data were collected, of which 18000 groups of data were collected for each attitude for processing and analysis. The following figure shows the amplitude thermodynamic diagram of CSI raw data collected under different attitudes (one subject, one group of actions). Among them, the ordinate is time, indicating that the data acquisition time of each group is 12 seconds, the number of data packets collected is 1200, and the abscissa is subcarrier information, indicating 30 different subcarriers collected.
In Figure 1, Figure 1(a)-(g) represent the amplitude data thermograms of neck rest posture, neck bending posture, neck extension posture, neck yaw motion leaning left to shoulder posture, neck yaw motion leaning right to shoulder posture, neck left rotation posture and neck right rotation posture, respectively. It can be clearly seen that there will be obvious differences in amplitude waveform when subjects are in different postures. In addition, the obvious differences between different subcarriers can be clearly seen through the thermal diagram. In further calculation, the maximum variance method is used to select the subcarrier. It is generally considered that the subcarrier with greater variance is more sensitive to small motion. After calculation, the variance of the 9th to 18th subcarriers are significantly higher than that of other subcarriers, so the data of these subcarriers are selected as the used data for analysis and better results can be obtained. In order to better identify and classify different neck postures, one-dimensional convolutional neural network model is selected to process and analyze the collected CSI data. As mentioned above, the data used in this paper is CSI amplitude information of subjects in wireless signal environment. For different waveform data, the difference between peak and trough represents the motion amplitude of different attitudes. The amplitude information is classified and processed differently, and the "maximum amplitude", "minimum amplitude" and "average amplitude" are extracted as features to analyze the waveforms of different neck postures. These features have clear physical significance and can be directly obtained by simple calculation of signal amplitude. By using 1D-CNN model, the average classification accuracy of different neck postures is 99.1%, which can reflect the availability and accuracy of amplitude information in neck activity monitoring.
In this work, wavelet analysis filtering is used to process the obtained signal amplitude information. Wavelet filtering mainly decomposes the wireless signal into high-frequency signal and low-frequency signal by using high-pass and low-pass filters, and then optimizes and reconstructs the signal for denoising. In the process of decomposition and reconstruction, the selection of wavelet basis and the confirmation of wavelet decomposition layers play an important role in the effect of wavelet filtering. Considering that the signal obtained in this paper has the characteristics of compact support, we can get good results by selecting Sym8 function as wavelet basis function, and the continuity of this function can play a good filtering effect on the signal in this paper. For the number of wavelet filter decomposition layers, considering that different people have different activity postures and different signal rates generated by neck movement, an appropriate number of layers is needed. If the selected number of layers is too low, the noise cannot be removed well. If the selected number of layers is too high, the spatial coefficient of each layer of wavelet will lose more correct signals during processing, which not only reduces the operation speed, limits the required real-time performance, but also leads to errors. These errors will eventually lead to great errors in the results. In this paper, a comparative experiment is set up to determine the most effective wavelet decomposition layers. In the experiment, the number of wavelet decomposition layers from 1 to 6 is selected to process the collected original CSI amplitude data respectively. In the classification of different neck postures, the number of wavelet filter layers will significantly affect the performance of the analysis algorithm. When the number of 4-layer wavelet filtering layers is selected, the filtered amplitude information is input into the one-dimensional convolutional neural network model constructed in this paper, which can more accurately identify different neck postures.
In order to prove the performance of the 1D-CNN model constructed, machine learning algorithms such as SVM and K-NN are used for analysis and comparison. In the experiment, taking the feature space, number of samples and the training time into account, radial basis function (RBF) is selected as the kernel function, and the value range of K in K-NN algorithm is [1,10]. The error of the experiment is reduced by traversing the value range of K.
The results show that the average accuracy of K-NN algorithm is 95.9%, while the average accuracy of SVM algorithm is 92.1%. It is seen in Figures 2 and 3 that contactless sensing also has the same detection ability as traditional sensors.
There are different pressures on the neck under different bending degrees, accurate and timely detection of abnormal cervical curvature can play a certain role in the prevention of cervical spondylosis. In the experiment, 100 packets are sent per second, there are 3 receiving antennas and 1 transmitting antenna; 90 subcarrier data are obtained in each individual experiment. In the parameter setting, the 5.24 GHz frequency band is selected, and the antenna is placed on the left and right sides of the subject with a spacing of 85 cm; the second receiving antenna and the transmitting antenna form a straight line visually, and the distance between the two antenna bases and the ground is 75cm. In this experiment, each subject collected 20 groups of data for each different activity. Each activity can collect 90 subcarrier data, so a total of 72000 groups of data and 18000 groups of each action are collected. The subjects were required to perform 15° neck bending, 30° neck bending, 45° neck bending and 60° neck bending, respectively.
Figure 4 give the collected amplitude information for 4 different postures.
For different neck bending postures, the overall trend of the figure is similar, mainly because similar postures have similar effects on space electromagnetic wave disturbance. Small differences in graphics can be used to judge different postures. The abscissa represents the number of packets, and the ordinate represents the amplitude of CSI data. With the increase of neck movement angle, the amplitude of CSI decreased from the beginning to the rise from the trough. The reason is that when the bending angle is larger and larger, the pressure on the subject's neck is larger and larger, so the subject's neck is more and more difficult to bend; therefore, it is more difficult and takes longer for subjects to bend at a larger angle in the test. The CSI amplitude information reflected on the CSI waveform needs more and more data packets, that is, the time required is longer and longer. For the amplitude waveform in this experiment, when it begins to decline, it indicates that the activity begins; the process from the waveform falling to the trough rising is the whole activity time, and the difference between the waveform falling from the initial to the trough is the activity amplitude. Therefore, the minimum amplitude, maximum amplitude, average amplitude, activity completion speed and average activity completion speed are extracted as features for processing. The classification accuracy of the four activities in the experiment reached 99.4%, 99.7%, 99.5% and 99.3%, respectively; the average accuracy was 99.5%. The decomposition basis and the number of decomposition layers of wavelet decomposition affect the final result; therefore, many different wavelet decomposition level experiments are still used to find the optimal decomposition level. When the number of wavelet layers is less than 4, the monitoring effect of neck activity in this paper is relatively stable. Both types of models have the best performance when the number of wavelet filtering layers reaches 4.
In order to improve the robustness of this paper, this paper selects four indexes, precision, accuracy, recall and F1 score, which are often used in the current research, to evaluate the model in this paper. In order to determine these data, it is necessary to determine TP, TN, FP and FN. Take the neck flexion of 15° in the neck activity monitoring used in this paper as an example, the following table gives the confusion matrix:
Correct prediction | Prediction error | |
Actually correct | TP | FN |
Actual error | FP | TN |
TP represents the number of samples whose neck activity is judged to be correct, FP represents the number of samples whose neck activity is predicted to be correct but actually wrong, FN represents the number of samples whose neck activity is predicted to be wrong, TN represents the number of samples whose neck activity is not actual and predicted to be not the neck activity.
Precision=TP+TNTP+FN+FP+TN |
Accuracy=TPTP+FP |
Recall=TPTP+FN |
F1score=2×Precision×RecallPrecision+Recall |
According to the data obtained in this paper, the final results are shown in the figure below. The results show that the three machine learning algorithms have good accuracy, for the four indexes, the accuracy of each machine learning algorithm is higher than 95%. By comparison, SVM has the lowest accuracy.
It can be seen that the performance of the 1d-cnn classification model constructed in this paper is better than other models in the comparison of four different indicators. The experimental results show the superior performance of the system in monitoring the activities of different bending angles of the neck, and can monitor the bending of the human neck to a certain extent.
It is worth mentioning that besides the amplitude information, there are some other information, e.g., phase information, could play a supporting role in movement feature extraction, and such information can also be sanitized to remove redundant information. It is important to point out that since the scale of the neck movement considered in this work are large, amplitude information is fully sufficient to characterize relevant features.
This paper verifies the feasibility of using non-contact equipment to monitor neck movement through experiments. In the experiment, various postures of neck movement and different bending activities of neck were analyzed. This paper selects the amplitude information of the collected CSI data and extracts the features to analyze different neck activities; at the same time, different algorithms are used to process, compare and analyze the experimental data. Using CSI for perception can correctly identify different neck activities and timely find abnormal posture of cervical spine, which is helpful to help users reduce the time of excessive compression of cervical spine and protect cervical health. At the same time, doctors can better analyze the causes of cervical spondylosis. In addition, this paper judges the optimal number of wavelet decomposition layers through a large number of experiments, and constructs a convolution neural network model for data analysis. The results show that the model proposed in this paper can objectively identify neck posture activities. The innovation of this paper is to monitor and analyze the symptoms in a non-contact way; In this way, problems such as self-awareness enhancement and comfort can be overcome.
All authors declare no conflicts of interest in this paper.
[1] |
J. Reynolds, D. Marsh, H. Koller, J. Zenenr, Cervical range of movement in relation to neck dimension, Eur. Spine J., 18 (2009), 863-868. doi: 10.1007/s00586-009-0894-z. doi: 10.1007/s00586-009-0894-z
![]() |
[2] | H. Tsunezuka, D. Kato, S. Okada, S. Ishihara, J. Shimada, Three-dimensional kinematic analysis of active cervical spine motion by using a multifaceted marker device, in 2013 35th Annual International Conference of the IEEE Engineering in Medicine and Biology Society (EMBC), (2013). Available from: https://ieeexplore.ieee.org/abstract/document/6610641. doi: 10.1109/EMBC.2013.6610641. |
[3] | Y. Wang, H. Zhou, Z. Yang, O. W. Samuel, W. Liu, Y. Cao, et al., An intelligent wearable device for human's cervical vertebra posture monitoring, in 40th Annual International Conference of the IEEE Engineering in Medicine and Biology Society (EMBC), (2018), 3280-3283. Available from: https: //ieeexplore.ieee.org/abstract/document/8512896. doi: 10.1109/EMBC.2018.8512896. |
[4] |
V. F. Ferrario, C. Sforza, G. Serrao, G. Grassi, E. Mossi, Active range of motion of the head and cervical spine: a three‐dimensional investigation in healthy young adults, J. Orthop. Res., 20 (2002), 122-129. doi: 10.1016/S0736-0266(01)00079-1. doi: 10.1016/S0736-0266(01)00079-1
![]() |
[5] | L. Huang, Rehabilitation Medicine, People's Medical Publishing House, Beijing, 2018. |
[6] |
A. I. Binder, Cervical spondylosis and neck pain, BMJ, 334 (2007), 527-531. doi: 10.1136/bmj.39127.608299.80. doi: 10.1136/bmj.39127.608299.80
![]() |
[7] | K. K. Hansraj, Assessment of stresses in the cervical spine caused by posture and position of the head, Surg. Technol. Int., 25 (2014), 277-279. |
[8] |
Z. Ming, S. Pietikainen, O. Hä nninen, Excessive texting in pathophysiology of first carpometacarpal joint arthritis, Pathophysiology, 13 (2006), 269-270. doi: 10.1016/j.pathophys.2006.09.001. doi: 10.1016/j.pathophys.2006.09.001
![]() |
[9] |
K. Mekhora, C. Liston, S. Nanthavanij, J. H. Cole, The effect of ergonomic intervention on discomfort in computer users with tension neck syndrome, Int. J. Ind. Ergon., 26 (2000), 367-379. doi: 10.1016/S0169-8141(00)00012-3. doi: 10.1016/S0169-8141(00)00012-3
![]() |
[10] |
W. J. De Hertogh, P. H. Vaes, V. Vijverman, A. De Cordt, W. Duquet, The clinical examination of neck pain patients: the validity of a group of tests, Man. Ther., 12 (2007), 50-55. doi: 10.1016/j.math.2006.02.007. doi: 10.1016/j.math.2006.02.007
![]() |
[11] |
H. Inokuchi, M. Tojima, H. Mano, Y. Ishikawa, N. Ogata, N. Haga, Neck range of motion measurements using a new three-dimensional motion analysis system: validity and repeatability, Eur. Spine J., 24 (2015), 2807-2815. doi: 10.1007/s00586-015-3913-2. doi: 10.1007/s00586-015-3913-2
![]() |
[12] |
Z. P. Bian, J. Hou, L. P. Chau, N. M. Thalmann, Fall detection based on body part tracking using a depth camera, IEEE J. Biomed. Health Inf., 19 (2015), 430-439. doi: 10.1109/JBHI.2014.2319372. doi: 10.1109/JBHI.2014.2319372
![]() |
[13] | X. Ma, G. Xu, M. Li, J. Xie, L. Chen, W. Pei, Measuring cervical vertebra movements using kinect sensor, in 2015 37th Annual International Conference of the IEEE Engineering in Medicine and Biology Society (EMBC), (2015), 2771-2774. Available from: https://ieeexplore.ieee.org/abstract/document/7318966. doi: 10.1109/EMBC.2015.7318966. |
[14] | K. Woyach, D. Puccinelli, M. Haenggi, Sensorless sensing in wireless networks: Implementation and measurements, in 2006 4th International Symposium on Modeling and Optimization in Mobile, Ad Hoc and Wireless Networks, (2006), 1-8. Available from: https://ieeexplore.ieee.org/abstract/document/1666495. doi: 10.1109/WIOPT.2006.1666495. |
[15] |
H. Stockman, Communication by means of reflected power, Proc. IRE, 36 (1948), 1196-1204. doi: 10.1109/JRPROC.1948.226245. doi: 10.1109/JRPROC.1948.226245
![]() |
[16] |
Y. Liu, Y. Zhao, L. Chen, J. Pei, J. Han, Mining frequent trajectory patterns for activity monitoring using radio frequency tag arrays, IEEE Trans. Parallel Distrib. Syst., 23 (2012), 2138-2149. doi: 10.1109/TPDS.2011.307. doi: 10.1109/TPDS.2011.307
![]() |
[17] |
Z. Xia, Q. Zhang, S. Ye, S. Wu, K. Tan, G. Fang, et al., Design of a handheld pseudo random coded UWB radar for human sensing, J. Radars, 4 (2015), 527-537. doi: 10.12000/JR15027. doi: 10.12000/JR15027
![]() |
[18] | F. Adib, Z. Kabelac, D. Katabi, R. C. Miller, 3D tracking via body radio reflections, in 11th USENIX Symposium on Networked Systems Design and Implementation (NSDI 14), (2014), 317-329. Available from: https://www.usenix.org/conference/nsdi14/technical-sessions/presentation/adib. |
[19] |
S. A. Shah, N. Zhao, A. Ren, Z. Zhang, X. Yang, J. Yang, et al., Posture recognition to prevent bedsores for multiple patients using leaking coaxial cable, IEEE Access, 4 (2016), 8065-8072. doi: 10.1109/ACCESS.2016.2628048. doi: 10.1109/ACCESS.2016.2628048
![]() |
[20] | D. Haider, A. Ren, D. Fan, N. Zhao, X. Yang, X. Yang, et al., Utilizing a 5G spectrum for health care to detect the tremors and breathing activity for multiple sclerosis, Trans. Emerging Tel. Tech., 29 (2018), e3454. doi: 10.1002/ett.3454. |
[21] |
X. Yang, S. A. Shah, A. Ren, D. Fan, N. Zhao, S. Zheng, et al., S-Band sensing-based motion assessment framework for cerebellar dysfunction patients, IEEE Sensors J., 19 (2019), 8460-8467. doi: 10.1109/JSEN.2018.2861906. doi: 10.1109/JSEN.2018.2861906
![]() |
[22] |
D. Haider, X. Yang, Q. H. Abbasi, Post-surgical fall detection by exploiting the 5G C-Band technology for eHealth paradigm, Appl. Soft Comput., 81 (2019), 105537. doi: 10.1016/j.asoc.2019.105537. doi: 10.1016/j.asoc.2019.105537
![]() |
[23] |
S. A. Shah, J. Ahmad, F. Masood, S. Y. Shah, H. Pervaiz, W. Taylor, et al., Privacy-preserving wandering behavior sensing in dementia patients using modified logistic and dynamic newton leipnik maps, IEEE Sensors J., 21 (2021), 3669-3679. doi: 10.1109/JSEN.2020.3022564. doi: 10.1109/JSEN.2020.3022564
![]() |
[24] |
F. Siddiqui, S. Zeadally, K. Salah, Gigabit wireless networking with IEEE 802.11ac: technical overview and challenges, J. Networks, 10 (2015), 164-171. doi: 10.4304/jnw.10.3.164-171. doi: 10.4304/jnw.10.3.164-171
![]() |
[25] | R. van Nee, R. Prasad, OFDM for Wireless Multimedia Communications, Artech House, Inc., 2000. |
[26] |
D. Halperin, W. Hu, A. Sheth, D. Wetherall, 802.11 with multiple antennas for dummies, ACM SIGCOMM Comput. Commun. Rev., 40 (2010), 19-25. doi: 10.1145/1672308.1672313. doi: 10.1145/1672308.1672313
![]() |
[27] | F. A. Jenkins, H. E. White, Fundamentals of optics, Indian J. Phys., 26 (1957), 1-23. |
[28] | C. A. Gabriel, D. R. Henri, Directional radio transmission system. US2043347A, June 9, 1936. |
[29] | H. D. Hristov, Fresnel Zones in Wireless Links, Zone Plate Lenses and Antennas, Artech House Inc., 2000. |
[30] |
L. Davies, U. Gather, The identification of multiple outliers, J. Am. Stat. Assoc., 88 (1993), 782-792. doi: 10.1080/01621459.1993.10476339. doi: 10.1080/01621459.1993.10476339
![]() |
[31] | C. Fan, L. Cao, Communication Theories, China Machine Press, 2006. |
[32] |
Q. Pan, J. Meng, L. Zhang, Y. Cheng, H. Zhang, Wavelet filtering method and its application, J. Electron. Inf. Technol., 29 (2007), 236-242. doi: 10.3724/SP.J.1146.2005.00233. doi: 10.3724/SP.J.1146.2005.00233
![]() |
[33] | W. Guan, New Support Vector Machine Formulations And Algorithms With Application To Biomedical Data Analysis, Georgia Institute of Technology, 2011. |
1. | Zhenyu Huang, Siwei Xie, Fang Liu, Ting Zhang, Yiwen Gu, Laser Needle Knife's Effects on Rabbits Cervical Spondylopathy of Vertebral Artery, Fibrinogen, and Blood Viscosity, 2022, 9, 2296-875X, 10.3389/fsurg.2022.778608 | |
2. | Vivek Sharma, Vijay Mohan K, Review on design of real-time posture monitoring system for the cervical region, 2024, 0014-0139, 1, 10.1080/00140139.2024.2334919 |
Correct prediction | Prediction error | |
Actually correct | TP | FN |
Actual error | FP | TN |