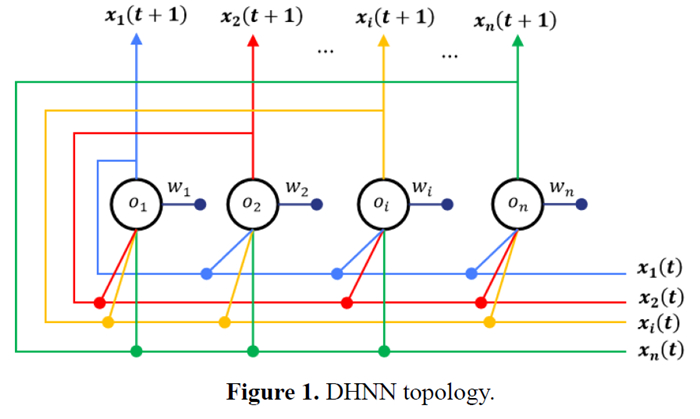
Neuroendocrine-immune homeostasis in health and disease is a tightly regulated bidirectional network that influences predisposition, onset and progression of age-associated disorders. The complexity of interactions among the nervous, endocrine and immune systems necessitates a complete review of all the likely mechanisms by which each individual system can alter neuroendocrine-immune homeostasis and influence the outcome in age and disease. Dysfunctions in this network with age or external/internal stimuli are implicated in the development of several disorders including autoimmunity and cancer. The existence of sympathetic noradrenergic innervations on lymphoid organs in synaptic association with immune cells that express receptors for endocrine mediators such as hormones, neural mediators such as neurotransmitters and immune effector molecules such as cytokines explains the complicated nature of the regulatory pathways that must always maintain homeostatic equilibrium within and among the nervous, endocrine and immune systems. The incidence, development and progression of cancer, affects each of the three systems by disrupting regulatory pathways and tipping the scales away from homeostasis to favour pathways that enable it to evade, override and thrive by using the network to its advantage. In this review, we have explained how the neuroendocrine-immune network is altered in female reproductive aging and cancer, and how these modulations contribute to incidence and progression of cancer and hence prove to be valuable targets from a therapeutic standpoint. Reproductive aging, stress-associated central pathways, sympathetic immunomodulation in the periphery, inflammatory and immunomodulatory changes in central, peripheral and tumor-microenvironment, and neuro-neoplastic associations are all likely candidates that influence the onset, incidence and progression of cancer.
Citation: Hannah P. Priyanka, Rahul S. Nair, Sanjana Kumaraguru, Kirtikesav Saravanaraj, Vasantharekha Ramasamy. Insights on neuroendocrine regulation of immune mediators in female reproductive aging and cancer[J]. AIMS Molecular Science, 2021, 8(2): 127-148. doi: 10.3934/molsci.2021010
[1] | Caicai Feng, Saratha Sathasivam, Nurshazneem Roslan, Muraly Velavan . 2-SAT discrete Hopfield neural networks optimization via Crow search and fuzzy dynamical clustering approach. AIMS Mathematics, 2024, 9(4): 9232-9266. doi: 10.3934/math.2024450 |
[2] | Farah Liyana Azizan, Saratha Sathasivam, Nurshazneem Roslan, Ahmad Deedat Ibrahim . Logic mining with hybridized 3-satisfiability fuzzy logic and harmony search algorithm in Hopfield neural network for Covid-19 death cases. AIMS Mathematics, 2024, 9(2): 3150-3173. doi: 10.3934/math.2024153 |
[3] | Muhammad Aqmar Fiqhi Roslan, Nur Ezlin Zamri, Mohd. Asyraf Mansor, Mohd Shareduwan Mohd Kasihmuddin . Major 3 Satisfiability logic in Discrete Hopfield Neural Network integrated with multi-objective Election Algorithm. AIMS Mathematics, 2023, 8(9): 22447-22482. doi: 10.3934/math.20231145 |
[4] | Gaeithry Manoharam, Azleena Mohd Kassim, Suad Abdeen, Mohd Shareduwan Mohd Kasihmuddin, Nur 'Afifah Rusdi, Nurul Atiqah Romli, Nur Ezlin Zamri, Mohd. Asyraf Mansor . Special major 1, 3 satisfiability logic in discrete Hopfield neural networks. AIMS Mathematics, 2024, 9(5): 12090-12127. doi: 10.3934/math.2024591 |
[5] | Xiaoyan Liu, Mohd Shareduwan Mohd Kasihmuddin, Nur Ezlin Zamri, Yunjie Chang, Suad Abdeen, Yuan Gao . Higher order Weighted Random k Satisfiability (k=1,3) in Discrete Hopfield Neural Network. AIMS Mathematics, 2025, 10(1): 159-194. doi: 10.3934/math.2025009 |
[6] | Nur 'Afifah Rusdi, Nur Ezlin Zamri, Mohd Shareduwan Mohd Kasihmuddin, Nurul Atiqah Romli, Gaeithry Manoharam, Suad Abdeen, Mohd. Asyraf Mansor . Synergizing intelligence and knowledge discovery: Hybrid black hole algorithm for optimizing discrete Hopfield neural network with negative based systematic satisfiability. AIMS Mathematics, 2024, 9(11): 29820-29882. doi: 10.3934/math.20241444 |
[7] | Nurshazneem Roslan, Saratha Sathasivam, Farah Liyana Azizan . Conditional random k satisfiability modeling for k = 1, 2 (CRAN2SAT) with non-monotonic Smish activation function in discrete Hopfield neural network. AIMS Mathematics, 2024, 9(2): 3911-3956. doi: 10.3934/math.2024193 |
[8] | Jin Gao, Lihua Dai . Anti-periodic synchronization of quaternion-valued high-order Hopfield neural networks with delays. AIMS Mathematics, 2022, 7(8): 14051-14075. doi: 10.3934/math.2022775 |
[9] | S. Neelakandan, Sathishkumar Veerappampalayam Easwaramoorthy, A. Chinnasamy, Jaehyuk Cho . Fuzzy adaptive learning control network (FALCN) for image clustering and content-based image retrieval on noisy dataset. AIMS Mathematics, 2023, 8(8): 18314-18338. doi: 10.3934/math.2023931 |
[10] | Mohammed D. Kassim . Controlling stability through the rate of decay of the delay feedback kernels. AIMS Mathematics, 2023, 8(11): 26343-26356. doi: 10.3934/math.20231344 |
Neuroendocrine-immune homeostasis in health and disease is a tightly regulated bidirectional network that influences predisposition, onset and progression of age-associated disorders. The complexity of interactions among the nervous, endocrine and immune systems necessitates a complete review of all the likely mechanisms by which each individual system can alter neuroendocrine-immune homeostasis and influence the outcome in age and disease. Dysfunctions in this network with age or external/internal stimuli are implicated in the development of several disorders including autoimmunity and cancer. The existence of sympathetic noradrenergic innervations on lymphoid organs in synaptic association with immune cells that express receptors for endocrine mediators such as hormones, neural mediators such as neurotransmitters and immune effector molecules such as cytokines explains the complicated nature of the regulatory pathways that must always maintain homeostatic equilibrium within and among the nervous, endocrine and immune systems. The incidence, development and progression of cancer, affects each of the three systems by disrupting regulatory pathways and tipping the scales away from homeostasis to favour pathways that enable it to evade, override and thrive by using the network to its advantage. In this review, we have explained how the neuroendocrine-immune network is altered in female reproductive aging and cancer, and how these modulations contribute to incidence and progression of cancer and hence prove to be valuable targets from a therapeutic standpoint. Reproductive aging, stress-associated central pathways, sympathetic immunomodulation in the periphery, inflammatory and immunomodulatory changes in central, peripheral and tumor-microenvironment, and neuro-neoplastic associations are all likely candidates that influence the onset, incidence and progression of cancer.
The Boolean satisfiability (SAT) problem is a classical issue in computational complexity theory and has been a significant research subject in computer science and artificial intelligence since the 1970s [1]. In 1971, S. A. Cook [2] proved that the SAT problem is the world's first NP-complete problem, meaning any NP problem can be reduced to the SAT problem for a polynomial-time solution. The SAT problem serves as a benchmark for the difficulty of a category of problems known as the core of NP-complete problems. It plays a crucial role in various areas of computer science, including theoretical computer science, complexity theory, cryptosystems, and artificial intelligence [3,4,5,6]. With the advancements in computer hardware performance and algorithm design, traditional SAT solvers have become effective in many practical applications [7,8,9,10]. However, as problems grow in size and complexity, traditional methods often face challenges such as inefficiency and high consumption of computational resources. This has prompted researchers to explore new solution methods and techniques. Among these, the discrete Hopfield neural network (DHNN) [11], a classical neural network model, has shown significant potential and effectiveness in solving combinatorial optimization problems since its inception. Hopfield [11] demonstrated the stability of network dynamics, highlighting that the evolution of network states is essentially a process of energy minimization. When the association weights are symmetric, the system reaches a stable state. This stable equilibrium point aligns with the correct storage state, providing a clear physical explanation for associative memory. The network, by emphasizing the collective function of neurons from a systems perspective, offers preliminary insights into the nature of associative memory. Due to its robust memory capabilities and parallel processing power, the DHNN is particularly effective in addressing combinatorial optimization problems such as SAT [12,13,14].
In the study of SAT problems, the 3-satisfiability (3SAT) logic has received significant attention from researchers because higher-order Boolean SAT can be converted or reduced to the 3SAT form [15]. In the 3SAT problem, each clause contains three literals, making it more complex and closer to practical logic constraint problems. To address the 3SAT problem, researchers have mapped the variables and clauses of a Boolean formula into the neurons and energy functions of a discrete Hopfield network. In this network, each variable and clause is encoded as a neuron's state and connection weights [16,17,18]. A satisfying solution to the Boolean formula is then found by adjusting the neuron states to minimize the energy function. This method of solving the 3-SAT problem implemented in a DHNN is referred to as the DHNN-3SAT model. The DHNN-3SAT model has garnered extensive attention and research interest due to its significant improvement in solving ability and effectiveness on 3SAT problems [19,20]. Early research efforts focused on basic discrete Hopfield network structures, utilizing simple connection weights and update rules. As research progressed, scholars proposed various improvement and optimization strategies to enhance the network's performance and efficiency. In 1992, Wan Abdullah successfully integrated special logic programming as symbolic rules into a DHNN [21], and in 2011, Sathasivam and Abdullah extended this approach and formally named it the Wan Abdullah method (WA method) [22]. In 2014, Sathasivam et al. embedded higher-order SAT into DHNN [23]. Kasihmuddin et al. [24] applied k-satisfiability planning in DHNN. In 2017, Mansor et al. [25] demonstrated the hybrid use of the DHNN artificial immune system for the 3-SAT problem. Subsequently, Kasihmuddin et al. [26] proposed a genetic algorithm for k-satisfiability logic programming based on DHNN. In 2021, Mansor and Sathasivam [12] proposed a DHNN-3SAT optimal performance index. In 2023, Azizan and Sathasivam [27] proposed a DHNN model with a 3SAT fuzzy logic model of DHNN. However, as researchers delved deeper into the DHNN-SAT model, they found that its computational efficiency is not optimal for large-scale problems due to the inherent limitations of the DHNN, with a tendency to fall into local minima. To address these issues, researchers have been working to integrate heuristic algorithms into the optimization process [28,29,30,31] to enhance the accuracy of the DHNN-SAT model. Currently, these research methods are achieving high global minimum ratios in DHNN-SAT models with fewer neurons. By adjusting the structure and parameters of the neural network, researchers [32] have been exploring various model variations and optimization strategies to further enhance the performance and generalizability of the model. These efforts not only offer a new perspective and approach to understanding and solving SAT problems but also make significant contributions and provide inspiration for the application of DHNNs in combinatorial optimization and discrete problem-solving.
Although the DHNN-3SAT model has been successful in addressing certain problems, it still has some challenges and limitations. First, the model's computational complexity and storage requirements may increase significantly with the problem size, leading to performance issues when dealing with large-scale SAT problems. Second, the model's training and optimization process may be sensitive to parameter tuning and initialization, necessitating more experimental validation and tuning. Third, it may take a longer time to reach a stable solution when dealing with complex problems, which can impact its practical application in engineering and other fields. Lastly, in real-life scenarios, the constraints of SAT problems often change over time, leading to the need for network redesign and the generation of a large number of redundant computations with the increase, decrease, and update of large-scale constraints, ultimately limiting the traditional DHNN-3SAT model's performance.
To address the changing constraints of the SAT problem and the increasing size and complexity of the network, this paper proposes a WA method based on basic logical clauses. This method utilizes information about the synaptic weights of the original SAT problem in the DHNN, leading to significant savings in repetitive calculations. In addition, to tackle the issue of increasing Boolean variables and logical clauses leading to a rapidly expanding solution space and the traditional DHNN-WA model being prone to oscillations and local minima, this paper introduces a DHNN 3SAT model, based on a genetic algorithm-optimized K-modes clustering. This approach uses the genetic optimization K-modes clustering algorithm to cluster the initial space, reducing the retrieval space and avoiding repeated searches, thus improving retrieval efficiency.
The paper is organized as follows: Section 2 introduces the knowledge related to the research, including 3SAT and DHNN. Section 3 details the implementation and workflow for determining the synaptic weights of the DHNN 3SAT model using the WA method. To address the issue of a large number of redundant computations caused by the changing constraints of the 3SAT problem, the basic logic clause-based WA (BLC-WA) method is proposed. Section 4 introduces the K-modes clustering algorithm optimized by a genetic algorithm. Section 5 details the implementation steps and development process of the DHNN 3SAT model based on genetic algorithm-optimized K-modes clustering. Section 6 presents an experimental comparative analysis of the DHNN-3SAT model based on the genetic optimization K-modes clustering algorithm (DHNN-3SAT-GAKM) model proposed in this paper, and the three models DHNN-3SAT-WA, the DHNN-3SAT-WA model by using the Genetic Algorithm (DHNN-3SAT-GA), and the DHNN-3SAT-WA model by using Competition Algorithm (DHNN-3SAT-ICA), which are comprehensively evaluated using four evaluation metrics. Finally, Section 7 summarizes the work presented in this paper.
Definition 2.1. 3SAT is a satisfiability problem for a set of logical clauses consisting strictly of 3 literal variables. 3SAT problems can be expressed in 3 conjunctive normal forms (CNFs). Let the set of Boolean variables be {S1,S2,⋯,Sn} and the set of logical clauses be {C1,C2,⋯,Cm}, then the general form of a CNF 3SAT formula P containing n Boolean variables and m logical clauses is defined as:
P=⋀mk=1Ck, | (1) |
where the clause Ck consists of 3 literals connected by the classical operator or (∨): Ck=Z(k,1)∨Z(k,2)∨Z(k,3), and the state of the literals can be either a positive variable or the negation of a positive variable, i.e., Z(k,i)=Sj or Z(k,i)=¬Sj, 1≤k≤m, 1≤i≤3, 1≤j≤n. Each literal variable takes on the binary discrete value {1,−1}, where 1 denotes true and −1 denotes false. Each clause in 3SAT contains unique variables, meaning there is no repetition of the same variable (variable or negation of a variable) in clause Ck. Additionally, there are no repeated logical clauses within logical rules.
The problem denoted by 3SAT can be formally described as follows: Given a 3SAT formula, the task is to determine if there is an assignment of Boolean variables that makes the entire formula true. In particular, each clause in the formula must have at least one true literal for the whole formula to be true.
Instance. Suppose that for given a 3SAT problem, the conversion to the CNF 3SAT formula is:
P=(S1∨S2∨S3)∧(¬S1∨S2∨S3)∧(S1∨¬S2∨S3)∧(S1∨S2∨¬S3)∧(¬S1∨¬S2∨S3)∧(¬S1∨S2∨¬S3)∧(S1∨¬S2∨¬S3)∧(S1∨S2∨S4). | (2) |
In Eq (2), P is satisfiable if there exists a set of values for the variable S1,S2,S3,S4 such that P=1; otherwise, P is unsatisfiable.
The problem regarding 3SAT is a fundamental issue in computational complexity theory. Its NP-completeness and wide range of applications make it a crucial subject of research in both theoretical and practical contexts. Through a thorough examination of the 3SAT problem, a better understanding of computational complexity theory can be achieved, and effective tools and methods for solving practical problems can be provided. This study contributes to the advancement of computer science by exploring solution methods for 3SAT problems.
Neural networks can be divided into two types based on the flow of information: Feed-forward and feedback neural networks. The output of a feedforward neural network depends only on the current input vector and weight matrix, independent of the network's previous input state. An example of this is the commonly used back propagation (BP) neural network. In 1982, physicist professor J. J. Hopfield proposed [11] a single-layer feedback neural network, later called the Hopfield neural network. This network is of two types: Continuous Hopfield neural network (CHNN) and discrete Hopfield neural network (DHNN) [33,34]. DHNN has garnered significant attention due to its concise network structure and powerful memory function. It holds potential practical value in image recovery and optimization problems [35,36,37]. Figure 1 depicts the topology of a DHNN network with n neurons. Each neuron is functionally identical and interconnected in pairs. The neurons are represented by the set O={o1,o2,⋯,on}, and their corresponding states are denoted by the vector X=(x1,x2,⋯,xn), and the value of xi takes binary discrete values, typically {−1,1} or {0,1}. The state of the network is described as X(t)=(x1(t),x1(t),⋯,xn(t)) at time t, and the DHNN is stimulated by an external input to start its evolution. The outputs of localized lots are generated before the final state. The output of the local lot of the double link is:
hi(t)=∑jwijxi(t)−wi, | (3) |
where wi denotes a predefined threshold. The output of higher-order linked local lots is represented by Eq (4) as proposed by Mansor et al [22].
hi(t)=⋯+∑j∑kwijkxi(t)xj(t)+∑nj=1wijxi(t)−wi. | (4) |
The output state of the neuron oi at the time t+1 is denoted as:
xi(t+1)=sgn(hi(t))={1,hi(t)≥0,−1,hi(t)<0, | (5) |
where "sgn" denotes the sign function and wij denotes the connection weights of neuron oi and neuron oj, with the weights specified as follows.
wij={wji,i≠j,0,i=j. | (6) |
In the network training phase, the Hebbian rule is usually used to calculate the weights wij as:
wij=∑ms=1(2xsi−1)(2xsj−1), | (7) |
where m denotes the number of samples to be memorized.
The DHNN is essentially a nonlinear dynamical system. The network starts evolving from an initial state, and the DHNN is considered stable when its state no longer changes after a finite number of iterations. In DHNN, stability is determined by introducing the Lyapunov function as the energy function, which serves as an indicator of stability [38]. The system reaches stability when the energy function reaches a minimum point of invariance. The energy function in DHNN is defined as:
E(X)=⋯−13∑i∑j∑kwijkxixjxk−12∑i∑jwijxixj−∑iwixi. | (8) |
In 1983, Cohen and S. Grossberg showed that DHNNs evolve with a decreasing energy function and that a stable state of the network corresponds to a minimal value of the energy function. Consequently, for each stable state, we can check whether this state represents a global minimum by determining whether the energy function has reached a minimum [28]. If Eq (9) is satisfied, the stable state is considered a global minimum; otherwise, it is a local minimum.
|E(X)−Emin|<δ, | (9) |
where Emin denotes the minimum value of the energy function and δ is the user-defined tolerance value.
In this section, we will start by determining the synaptic weights of the DHNN 3SAT model using the WA method [21]. This method is a computational approach for deriving the synaptic weights of a network by aligning the cost function with the DHNN energy function. Our study acknowledges some challenges in this comparative method of deriving network synaptic weights, particularly as the number of variables and logical clauses increase. Additionally, the addition, deletion, and updating of logical clauses result in a large number of redundant computations. To tackle these issues, this section will outline the cost function of the basic logical clauses and compute the network synaptic weights by establishing the basic logical clauses of the CNF 3SAT formulae. This approach will allow for a more adaptable implementation in computing the network synaptic weights of the 3SAT when incorporated in a DHNN. The method is termed the BLC-WA method. Furthermore, the detailed calculation process using the BLC-WA method will be demonstrated with specific examples as logical clauses are added, deleted, and updated.
The WA method introduces a cost function based on propositional logic rules for the first time. It derives the synaptic weights of the network by comparing the cost function with the DHNN energy function, presenting a novel approach to using DHNN for solving the SAT problem. In this study, the WA method is used to incorporate the 3SAT problem into the DHNN for computing the network synaptic weights. The flowchart illustrating the implementation of the WA method is shown in Figure 2. The specific steps are as follows:
Step 1. Given any 3SAT problem, transform it into a CNF 3SAT formula P. Suppose the formula P contains n Boolean variables and m logical clauses.
Step 2. The 3SAT formula P is embedded into the DHNN, and for each Boolean variable, a unique neuron is specified. At moment t, the state of these neurons is denoted by {St1,St2,⋯,Stn}.
Step 3. Applying De. Morgan's law to obtain ¬P. When ¬P=0, correspond to the consistency interpretation of P; when ¬P= 1, correspond to the fact that at least one clause of P is not satisfied.
Step 4. Deriving the cost function EP. When the literal variable in ¬P is represented by 12(1−Si) when it is ¬Si and 12(1+Si) when it is Si, the logical clauses are internally connected by the multiplication operation and between logical clauses by addition. This creates the cost function EP. The magnitude of EP corresponds to the degree to which all logical clauses are satisfied. When EP=0 it represents a consistent interpretation of P. A larger value of EP represents a larger number of unsatisfied logical clauses.
Step 5. Comparing the cost function EP with the energy function E(X), the DHNN synaptic weight matrix W corresponding to the 3SAT formula P is obtained.
In this section, we use the problem of Eq (2) in Section 2.1 as an example to illustrate the process of computing synaptic weights in the DHNN using the WA method of embedding logical clauses into the DHNN.
To determine whether Eq (2) is satisfiable, the negation of Eq (2) is applied to De Morgan's law, which results in:
¬P=(¬S1∧¬S2∧¬S3)∨(S1∧¬S2∧¬S3)∨(¬S1∧S2∧¬S3)∨(¬S1∧¬S2∧S3)∨(S1∧S2∧¬S3)∨(S1∧¬S2∧S3)∨(¬S1∧S2∧S3)∨(¬S1∧¬S2∧¬S4). | (10) |
Since seeking a consistent interpretation of the terms of Eq (2) is the same as finding the smallest combination of inconsistent interpretations of Eq (10), the cost function can be defined as follows:
EP=12(1−S1)12(1−S2)12(1−S3)+12(1+S1)12(1−S2)12(1−S3)+12(1−S1)12(1+S2)12(1−S3)+12(1−S1)12(1−S2)12(1+S3)+12(1+S1)12(1+S2)12(1−S3)+12(1+S1)12(1−S2)12(1+S3)+12(1−S1)12(1+S2)12(1+S3)+12(1−S1)12(1−S2)12(1−S4)=−18S1S2S3−18S1S2S4−18S1S3+18S1S4−18S2S3+18S2S4−14S1−14S2−18S3−18S4+1 | (11) |
When the formula of Eq (2) provided The 3SAT formula is satisfied, the cost function EP reaches the minimum value of 0. At this point, the energy function for the corresponding DHNN converges to the global minimum, causing both the cost function and energy function to reach their minimum values. The network's synaptic weight matrix WP, embedded in the DHNN by Eq (2), is derived by comparing the cost function (11) with the energy function (8) using the WA method, and the results are shown in Table 1.
Weights | w123 | w124 | w134 | w234 | w12 | w13 | w14 | w23 | w24 | w34 | w1 | w2 | w3 | w4 |
P | 116 | 116 | 0 | 0 | 0 | 18 | −18 | 18 | −18 | 0 | 14 | 14 | 18 | 18 |
Definition 3.1. For any CNF formula containing n Boolean variables and m logical clauses, it can be viewed as consisting of the following eight basic logical clauses:
Cl1=(Si∨Sj∨Sk),Cl2=(¬Si∨Sj∨Sk), |
Cl3=(Si∨¬Sj∨Sk),Cl4=(Si∨Sj∨¬Sk), |
Cl5=(¬Si∨¬Sj∨Sk),Cl6=(¬Si∨Sj∨¬Sk), |
Cl7=(Si∨¬Sj∨¬Sk),Cl8=(¬Si∨¬Sj∨¬Sk), | (12) |
where l denotes the index at which the basic logical clause is looked up.
Applying De Morgan's law to the eight basic logical clauses in Eq (12) yields the corresponding negative basic logical clauses:
¬Cl1=(¬Si∧¬Sj∧¬Sk),¬Cl2=(Si∧¬Sj∧¬Sk), |
¬Cl3=(¬Si∧Sj∧¬Sk),Cl4=(¬Si∧¬Sj∧Sk), |
¬Cl5=(Si∧Sj∧¬Sk),¬Cl6=(Si∧¬Sj∧Sk), |
¬Cl7=(¬Si∧Sj∧Sk),¬Cl8=(Si∧Sj∧Sk). | (13) |
The consistency clause of each basic logic clause in seeking pair Eq (12) is equal to the minimum of the inconsistency clause of the negated basic logic clause in seeking pair Eq (13). The corresponding cost function for each basic logic clause is defined as follows:
ECl1=12(1−Si)12(1−Sj)12(1−Sk),ECl2=12(1+Si)12(1−Sj)12(1−Sk), |
ECl3=12(1−Si)12(1+Sj)12(1−Sk),ECl4=12(1−Si)12(1−Sj)12(1+Sk), |
ECl5=12(1+Si)12(1+Sj)12(1−Sk),ECl6=12(1+Si)12(1−Sj)12(1+Sk), |
ECl7=12(1−Si)12(1+Sj)12(1+Sk),ECl8=12(1+Si)12(1+Sj)12(1+Sk). | (14) |
Each basic logic clause is embedded into a DHNN separately. When each basic logic clause is satisfiable, the corresponding DHNN converges to the global minimum. At this point, the cost function and the corresponding energy function of the basic logic clause reach their minimum values. By comparing the cost function (14) of the basic logic clauses with the energy function (8), the basic logic clause weight matrix of the 3SAT formula can be derived. This weight matrix is abbreviated as 3SAT-BLCWM, and the results are shown in Table 2.
Weights | Cl1 | Cl2 | Cl3 | Cl4 | Cl5 | Cl6 | Cl7 | Cl8 |
wi | 1/8 | −1/8 | 1/8 | 1/8 | −1/8 | −1/8 | 1/8 | −1/8 |
wj | 1/8 | 1/8 | −1/8 | 1/8 | −1/8 | 1/8 | −1/8 | −1/8 |
wk | 1/8 | 1/8 | 1/8 | −1/8 | 1/8 | −1/8 | −1/8 | −1/8 |
wij | −1/8 | 1/8 | 1/8 | −1/8 | −1/8 | 1/8 | 1/8 | −1/8 |
wik | −1/8 | −1/8 | 1/8 | 1/8 | 1/8 | −1/8 | 1/8 | −1/8 |
wjk | −1/8 | 1/8 | −1/8 | 1/8 | 1/8 | −1/8 | 1/8 | −1/8 |
wijk | 1/16 | −1/16 | −1/16 | −1/16 | 1/16 | 1/16 | 1/6 | −1/16 |
Any 3SAT formula can be seen as made up of the basic logical clauses in Eq (12). Each logical clause in the 3SAT formula corresponds to a basic logical clause. So, when the DHNN learns a new logical clause, it only needs to identify the corresponding basic logical clauses, then refer to Table 2, and combine and calculate the network weight values to derive the network synaptic weights after adding a new logical clause. Figure 3 illustrates the flowchart of calculating network synaptic weights using the BLC-WA method. The specific steps for calculating network synaptic weights using the BLC-WA method are as follows:
Step 1. Given any 3SAT problem, transform it into CNF 3SAT formula P, which is assumed to contain N Boolean variables and M logical clauses;
Step 2. The 3SAT-BLCWM was established using the WA method (Table 2);
Step 3. Analyze CNF 3SAT formula P to map each logical clause to the basic logical clause;
Step 4. Based on Table 2 (3SAT-BLCWM), the weights corresponding to each logical clause of the 3SAT formula P are found. These weights are then spelled out by columns into the indexed result weight matrix W';
Step 5 The weight matrix W of formula P for 3SAT is obtained by combining and summing the result weight matrices W' by columns.
Next, we compute the 3SAT instances of Section 2.1 using the BLC-WA method based on Eq (2) which can be written in correspondence with the basic logical clause as:
P=C11∧C12∧C13∧C14∧C15∧C16∧C17∧C21. | (15) |
According to Table 2 (3SAT-BLCWM), the network synaptic weights corresponding to each logical clause of formula P can be found by indexing the results of the weight matrix W' using the columns. The results are shown in Table 3. The network synaptic weight matrix WP for the 3SAT formula P can be obtained by merging and adding the indexing results according to the columns, which are also shown in Table 3.
Weights | C11 | C12 | C13 | C14 | C15 | C16 | C17 | C21 | P |
w1 | 1/8 | −1/8 | 1/8 | 1/8 | −1/8 | −1/8 | 1/8 | 1/8 | 1/4 |
w2 | 1/8 | 1/8 | −1/8 | 1/8 | −1/8 | 1/8 | −1/8 | 1/8 | 1/4 |
w3 | 1/8 | 1/8 | 1/8 | −1/8 | 1/8 | −1/8 | −1/8 | 0 | 1/8 |
w4 | 0 | 0 | 0 | 0 | 0 | 0 | 0 | 1/8 | 1/8 |
w12 | −1/8 | 1/8 | 1/8 | −1/8 | −1/8 | 1/8 | 1/8 | −1/8 | 0 |
w13 | −1/8 | −1/8 | 1/8 | 1/8 | 1/8 | −1/8 | 1/8 | 0 | 1/8 |
w14 | 0 | 0 | 0 | 0 | 0 | 0 | 0 | −1/8 | −1/8 |
w23 | −1/8 | 1/8 | −1/8 | 1/8 | 1/8 | −1/8 | 1/8 | 0 | 1/8 |
w24 | 0 | 0 | 0 | 0 | 0 | 0 | 0 | −1/8 | −1/8 |
w34 | 0 | 0 | 0 | 0 | 0 | 0 | 0 | 0 | 0 |
w123 | 1/16 | −1/16 | −1/16 | −1/16 | 1/16 | 1/16 | 1/16 | 0 | 1/16 |
w124 | 0 | 0 | 0 | 0 | 0 | 0 | 0 | 1/16 | 1/16 |
w234 | 0 | 0 | 0 | 0 | 0 | 0 | 0 | 0 | 0 |
In SAT problems, the constraints often change, with constraints increasing, decreasing, and updating. The traditional DHNN-SAT method requires redesigning the weights of the SAT problem and constructing a new DHNN when facing this type of problem, which does not utilize the original SAT problem's information. As the original SAT problem's constraints increase, the corresponding original CNF formulation also increases the logical clauses, leading to a large number of redundant computations when dealing with large-scale logical clauses. This severely limits the effectiveness of the traditional DHNN-SAT method in solving problems with large-scale increasing constraints. To address this issue, this study proposes the BLC-WA method, a new design method for the SAT problem with changing constraints. This method utilizes the synaptic weight information of the original SAT problem in DHNN, saving a significant amount of repeated calculations. In the following section, the network synaptic weight design method for SAT problems with increasing, decreasing, and updating constraints will be introduced, providing a new approach for solving SAT problems with constantly changing constraints.
The addition of constraints to the original SAT problem is equivalent to adding logical clauses to the CNF SAT formula.
There is a 3SAT problem that translates into the CNF 3SAT formula:
P=Cl11∧Cl22∧⋯∧Clmm. | (16) |
When r logical clauses are added, the original CNF 3SAT formula becomes:
Padd=Cl11∧Cl22⋯∧Clmm∧Clm+1m+1∧Clm+2m+2∧⋯∧Clm+2m+r. | (17) |
Figure 4 depicts the flowchart of the BLC-WA method for solving the original 3SAT problem with additional constraints. This method is implemented as follows:
Step 1. Let the original CNF 3SAT formula P become Padd by adding r logical clauses (Eq 14);
Step 2. The basic logical clauses were mapped to the additional logical clauses, and the synaptic weights of the additional logical clauses were determined based on Table 2 (3SAT-BLCWM);
Step 3. The synaptic weights of the CNF 3SAT formula Padd after adding the r logical clauses were calculated using the following Eq (18).
Wadd=WP+Wadd(1)+Wadd(2)+⋯+Wadd(r). | (18) |
The following is a concrete demonstration of the implementation process using the 3SAT instance from Section 2.1.
Assuming that Eq (2) combines the logical clauses C22=¬S1∨S2∨S4 and C23=S1∨¬S2∨S4, the new CNF formula at this point is notated as Padd, specifically, as follows:
Padd=C11∧C12∧C13∧C14∧C15∧C16∧C17∧C21∧C22∧C23. | (19) |
In Section 3.3, the synaptic weights (WP) of the formula P in the network have been obtained using the BLC-WA method. Then, the synaptic weights of the newly added logical clauses (C22 and C23) are obtained by searching for Table 2 (3SAT-BLCWM) and then combined and summed with the synaptic weights (WP) of the formula P to obtain the new synaptic weights (Wadd) of the CNF formula 3SAT Padd. The calculation results are shown in Table 4.
Weights | P | C22 | C23 | Padd | C12 | C13 | Pdec | C17 | C21 | C18 | C31 | Pupd |
w1 | 1/4 | −1/8 | 1/8 | 1/4 | −1/8 | 1/8 | 1/4 | 1/8 | 1/8 | −1/8 | 0 | −1/8 |
w2 | 1/4 | 1/8 | −1/8 | 1/4 | 1/8 | −1/8 | 1/4 | −1/8 | 1/8 | −1/8 | 1/8 | 1/4 |
w3 | 1/8 | 0 | 0 | 1/8 | 1/8 | 1/8 | −1/8 | −1/8 | 0 | −1/8 | 1/8 | 1/4 |
w4 | 1/8 | 1/8 | 1/8 | 3/8 | 0 | 0 | 1/8 | 0 | 1/8 | 0 | 1/8 | 1/8 |
w12 | 0 | 1/8 | 1/8 | 1/4 | 1/8 | 1/8 | −1/4 | 1/8 | −1/8 | −1/8 | 0 | −1/8 |
w13 | 1/8 | 0 | 0 | 1/8 | −1/8 | 1/8 | 1/8 | 1/8 | 0 | −1/8 | 0 | −1/8 |
w14 | −1/8 | −1/8 | 1/8 | −1/8 | 0 | 0 | −1/8 | 0 | −1/8 | 0 | 0 | 0 |
w23 | 1/8 | 0 | 0 | 1/8 | 1/8 | −1/8 | 1/8 | 1/8 | 0 | −1/8 | −1/8 | −1/4 |
w24 | −1/8 | 1/8 | −1/8 | −1/8 | 0 | 0 | −1/8 | 0 | −1/8 | 0 | −1/8 | −1/8 |
w34 | 0 | 0 | 0 | 0 | 0 | 0 | 0 | 0 | 0 | 0 | −1/8 | −1/8 |
w123 | 1/16 | 0 | 0 | 1/16 | −1/16 | −1/16 | 3/16 | 1/16 | 0 | −1/16 | 0 | −1/16 |
w124 | 1/16 | −1/16 | −1/16 | −1/16 | 0 | 0 | 1/16 | 0 | 1/16 | 0 | 0 | 0 |
w234 | 0 | 0 | 0 | 0 | 0 | 0 | 0 | 0 | 0 | 0 | 1/16 | 1/16 |
Setting the original CNF 3SAT formula (16) reduces d logical clauses, and the original CNF 3SAT formula becomes:
Pdec=Cl11∧Cl22∧⋯∧Clm−dm−d. | (20) |
The flowchart for solving the original 3SAT problem with reduced constraints based on the BLC-WA method is also shown in Figure 4. It is implemented as follows:
Step 1. The original CNF 3SAT formula P is reduced by d logical clauses to Pdec;
Step 2. The basic logical clauses were mapped to the reduced logical clauses, and the synaptic weights of the reduced logical clauses were determined based on Table 2 (3SAT-BLCWM);
Step 3. The synaptic weights of the CNF 3SAT formula Pdec after declining the d logical clauses were calculated using the following Eq (21).
Wdec=WP−Wdec(1)−Wdec(2)−⋯−Wdec(d). | (21) |
The following is a concrete demonstration of the implementation process using the 3SAT instance from Section 2.1.
Assuming that Eq (2) reduces the logical clauses C12=¬S1∨S2∨S3 and C13=S1∨¬S2∨S3, the new CNF formula at this point is notated as Pdec, specifically, as follows:
Pdec=C11∧C14∧C15∧C16∧C17∧C21. | (22) |
To start, the synaptic weights for the reduced logical clauses C12 and C13 are determined by searching for Table 2 (3SAT-BLCWM). Next, the synaptic weights WP of the original 3SAT formula P are subtracted from the synaptic weights of the reduced logical clauses. This process yields the synaptic weights Wdec for the new CNF 3SAT formula Pdec. The computational results are also displayed in Table 4.
When the original CNF formula (16) is updated with u logical clauses, it can be regarded as a reduction of u logical clauses from the original formula and the addition of u new logical clauses. The updated CNF formula is:
Pupd=Cl11∧Cl22∧⋯∧Clm−um−u∧Cl'm−u+1m−u+1∧Cl'm−u+2m+2∧⋯∧Cl'mm | (23) |
The flowchart when updating the constraints based on the BLC-WA method is also shown in Figure 4, which is implemented as follows:
Step 1. The original CNF 3SAT formula P is updated by u logical clauses to Pupd;
Step 2. The basic logical clauses were mapped to the updated logical clauses, and the synaptic weights of the updated logical clauses were determined based on Table 2 (3SAT-BLCWM);
Step 3. The synaptic weights of the CNF 3SAT formula Pupd after updating the u logical clauses were calculated using the following Eq (24).
Wupd=WP−Wdec(1)−Wdec(2)−⋯−Wdec(u)+Wadd(1)+Wadd(2)+⋯+Wadd(u). | (24) |
The following is a concrete demonstration of the implementation process using the 3SAT instance from Section 2.1.
Suppose the logical clauses C17=S1∨¬S2∨¬S3 and C21=S1∨S2∨S4 in the original CNF 3SAT formula P are updated to C18=¬S1∨¬S2∨¬S3 and C31=S2∨S3∨S4, and the updated CNF 3SAT formula is now denoted as Pupd, specifically for:
Pupd=C11∧C12∧C13∧C14∧C15∧C16∧C18∧C31. | (25) |
To begin, find the synaptic weights of logical clauses C17, C21, C18 and C31 by searching for Table 2 (3SAT-BLCWM). Then, subtract the synaptic weights of logical clause C17, C21 from the original SAT formula P. Finally, the synaptic weights of logical clause C18, C31 are added to obtain the network synaptic weights Wupd of the updated 3SAT formula Pupd. The results of the computation are also displayed in Table 4.
The K-modes clustering algorithm is a method specifically designed for handling discrete data [39,40,41,42]. It extends the traditional K-means algorithm, which is mainly used for datasets with continuous attributes. The K-modes algorithm uses the Hamming distance as a metric [43], where this distance measures the number of differing attribute values between two sample points. In this algorithm, the Hamming distance is computed by adding the number of different attribute values between two samples, representing the degree of difference for a given sample compared to a clustering center. Finally, the samples are classified into the category that belongs to the clustering center with the smallest degree of difference. We can see the clustering process of the K-modes algorithm in Figure 5.
Let X={X1,X2,⋯,Xm} represent the set of samples to be clustered, and Xi=(x1,x2,⋯,xn) represent the n -dimensional vector with each component taking discrete values. Z={Z1,Z2,⋯,Zk} represents the clustering center and Zj=(z1,z2,⋯,zn),j=1,2,⋯,k. The objective function of the K-modes clustering algorithm is defined as:
F(Φ,Z)∑kj=1∑mi=1φijD(Xi,Zj), | (26) |
where φij∈{0,1} , ∑kj=1φij=1,1≤i≤n,Φ is the matrix of one, k denotes the number of clusters, φij=1 if the i th object is classified in the j -th class, otherwise φij=0. Zj is the center of the j -th class. D(Xi,Zj) denotes the computation of the Hamming distance between Xi and Zj :
D(Xi,Zj)=∑ni=1d(xi,zi), | (27) |
where d(xi,zi)={0,xi=zi1,xi≠zi.
The classification process must meet the following conditions: (1) every family must contain at least one sample; (2) each sample must belong to one and only one class. The fundamental steps of the K-modes clustering algorithm are as follows:
Step 1. Randomly identifying k clustering centers Z1,Z2,⋯,Zk.
Step 2. For each sample Xi(i=1,2,⋯,m) in the dataset, its Hamming distance from the k clustering centers is calculated separately using Eq (27), and the sample Xi is classified into the category closest to the centroid.
Step 3. After dividing all the samples into clusters, the cluster center " Zj " is recalculated, and each center component is updated to its plural.
Step 4. Repeat the process of Steps 2 and 3 above until the objective function F no longer changes.
To address the limitations of the K-modes clustering algorithm, which make it difficult to determine the optimal number of clusters and easy to get stuck at a local optimum, researchers have incorporated a genetic algorithm with adaptive global optimization search capabilities into the K-modes clustering algorithm [44,45]. This involves using a fitness function to carry out genetic operations, primarily mutation, to automatically learn the cluster centroids for the K-modes algorithm. Figure 6. shows the workflow diagram of the K-modes clustering algorithm for genetic optimization, which was developed in the following steps:
Step 1. Parameter initialization. Set relevant parameters: Initial cluster number k, population size m, crossover probability pc, variation probability pm, maximum number of iterations t.
Step 2. Randomly generate the initial population. Randomly generate k initial clustering centers Z1,Z2,⋯,Zk as initial population individuals.
Step 3. Take the population individual Z1,Z2,⋯,Zk as the clustering center and use K-modes clustering algorithm for clustering.
Step 4. Calculate the fitness value of individuals in the population. Here the fitness function is defined as follows:
f=Dmin¯D(X), | (28) |
where Dmin is the minimum class spacing and ¯D(X) is the average class spacing which is defined as follows:
Dmin=mini,j=1D(Zi,Zj). | (29) |
¯D(X)=1k∑kj=1∑mji=1D(Xi,Zj)mj. | (30) |
This fitness function is based on the idea that class separation should be maximized while intra-class spacing should be minimized. In other words, the goal is to maximize the distance between classes (Dmin) and minimize the variability within classes (¯D(X)). Throughout the evolutionary process, the individual population size is represented by the k value. If the k value is less than the optimal number of clusters, increasing k leads to a decrease in Dmin and ¯D(X), but the clustering division is not optimal. The decrease in ¯D(X) is more significant than Dmin, resulting in an increase in the fitness function value. Conversely, if the k value exceeds the optimal number of clusters, the change in ¯D(X) is not significant, and the intra-class spacing becomes very small due to secondary clustering. As a result, Dmin becomes very small, leading to a decrease in the overall fitness function value. Therefore, this fitness function can guide the k value toward the optimal number of clusters when the initial clustering center is optimized.
Step 5. Perform selection, crossover, and mutation operations to generate a new generation population.
Step 6. Repeat Step 3 to Step 5 until the maximum number of iterations is reached.
Step 7. Calculate the fitness value for each individual in the population and select the output with the highest fitness value.
The conventional DHNN-3SAT-WA model uses an exhaustive search (ES) during the retrieval phase [46], aiming to conduct a random search among individual candidate solutions to find a consistent interpretation that satisfies the 3SAT terms. Some researchers have proposed optimizing the traditional DHNN-3SAT-WA model by using heuristic algorithms such as the GA and ICA, denoted as DHNN-3SAT-GA [26] and DHNN-3SAT-ICA [38]. These methods can expedite the search for global or feasible solutions. However, unguided random initial assignment of candidate solutions leads to numerous repeated invalid solutions and fails to converge, often falling into a local optimum after DHNN evolution. Furthermore, as the number of Boolean variables and logical clauses increases, the size and logical complexity of the network expands, resulting in a rapid growth of the solution space. This makes the model susceptible to oscillations and more likely to land in local minima. Therefore, reducing the DHNN-3SAT-WA model's search time and preventing it from falling into local minima is a significant challenge in this field. The implementation process of the traditional DHNN-3SAT-WA, DHNN-3SAT-GA, and DHNN-3SAT-ICA models is depicted in Figure 7.
This study proposes a new solution to address these challenges: the DHNN-3SAT model based on the genetic optimization K-modes clustering algorithm referred to as DHNN-3SAT-GAKM. In this model, candidate solutions in the allocation space are clustered using the K-modes clustering algorithm, leading to initial allocation through a random search from each class. By reducing repeated initial candidate solutions and avoiding local optima to some extent, this process accelerates the search for the global minimum, improving the efficiency of global minimum retrieval. To determine the optimal number of clusters for the K-modes clustering algorithm, the genetic algorithm with adaptive global optimization search capability is introduced. The number of clusters is determined by calculating the value of the constructed fitness function, further enhancing global search capability.
The DHNN-3SAT-GAKM model aims to find a consistent set of Boolean variable values for the 3SAT problem. During the model's initialization phase, each neuron in the DHNN is connected to a specific Boolean variable in the CNF, and the connection weights represent the relationship between the variable and the clause. A WA method using basic logical clauses will be employed to determine the cost during the learning phase. In the retrieval phase, the DHNN is utilized to evolve, update, and iterate until the network reaches a stable equilibrium state, signified by a minimal energy function value. The energy function's primary purpose is to indicate whether this stable state corresponds to a global minimum of the 3SAT problem, which in turn represents a consistent interpretation of the CNF. Please see Figure 8 for the flowchart of the DHNN-3SAT-GAKM model development, and the implementation steps are summarized as follows:
Step 1. Model Preparation. For a given 3SAT problem, transform it into the corresponding CNF formulation, denoted as P. Assume it contains n Boolean variables and m logical clauses. Initialize the optimization algorithm parameters.
Step 2. Each Boolean variable of the 3SAT formula is uniquely assigned a Hopfield neuron in the DHNN design, which consists of n neurons O={o1,o2,⋯,on}, with the state at moment t denoted X(t)=(x1(t),x1(t),⋯,xn(t)).
Step 3. The BLC-WA method was used to calculate the 3SAT formula P synaptic weights and derive its cost function Ep. When P=1, Ep=0, at which time the energy function reaches its minimum value, giving Emin=−m8.
Step 4. Generate an initial candidate solution space by randomly creating m initial candidate solutions {X1(t),X2(t),⋯,Xm(t)}.
Step 5. The initial candidate solution {X1(t),X2(t),⋯,Xm(t)} is clustered using the K-modes clustering algorithm based on genetic optimization to obtain the optimal number of clusters k.
Step 6. Determine the candidate subset for retrieval. Candidate subset denoted as {Y1(t),Y2(t),⋯,Yc(t)}, where c=m/k , Yl(t)=(y1(t),x、y2(t),⋯,yn(t)),l=1,2,⋯,c. yi(t) corresponds to the state of t at the moment of the neuron oi.
Step 7. DHNN Evolution. For Yl(t)=(y1(t),y1(t),⋯,yn(t)), l=0,t=0, state updates are performed using Eq (5) until a stable state is reached. If Yl(t+1)≠Yl(t), then t=t+1, and if Yl(t+1)=Yl(t), the network reaches a steady state. Proceed to the next step.
Step 8. Retrieval Phase. Check if the energy of the steady state satisfies |E−Emin|<δ. If it does, store the steady-state Yl(t) as a global minimum. If it doesn't, l=l+1 , consider it as a local minimum and go back to Step 6.
Step 9. Model Evaluation. The model is assessed using the metrics of global minimum ratio, Hamming distance, CPU time, steady-state retrieval rate, and global minimum retrieval rate.
To thoroughly evaluate the performance of the DHNN-3SAT-GAKM model and its ability to solve real-world application problems, this section examines its performance alongside the conventional DHNN-3SAT-WA, DHNN-3SAT-GA, and DHNN-3SAT-ICA models on a benchmark dataset. Experimental analyses were conducted to compare their performance and demonstrate the superiority of the DHNN-3SAT-GAKM model proposed in this study. The experiments were carried out using MATLAB R2023b on a laptop computer running the Windows 10 operating system, equipped with an AMD Razor R5-3500U processor and 8 GB of RAM.
This study utilizes the DIMACS Benchmark Instances AIM dataset from SATLIB, provided by Kazuo Iwama et al. (https://www.cs.ubc.ca/~hoos/SATLIB/benchm.html). The AIM dataset comprises of 48 instances, with 24 being satisfiable and 24 unsatisfiable. To create a representative set of instances, 12 of these satisfiable instances are chosen for this study. Each instance contains three clauses, and you can find specific descriptions of the instances in Table 5.
No. | Instance | variables | Clauses | No. | Instance | variables | Clauses |
1 | aim-50-1_6-yes1-1 | 50 | 80 | 7 | aim-100-3_4-yes1-1 | 100 | 340 |
2 | aim-50-2_0-yes1-1 | 50 | 100 | 8 | aim-100-6_0-yes1-1 | 100 | 600 |
3 | aim-50-3_4-yes1-1 | 50 | 170 | 9 | aim-200-1_6-yes1-1 | 200 | 320 |
4 | aim-50-6_0-yes1-1 | 50 | 300 | 10 | aim-200-2_0-yes1-1 | 200 | 400 |
5 | aim-100-1_6-yes1-1 | 100 | 160 | 11 | aim-200-3_4-yes1-1 | 200 | 680 |
6 | aim-100-2_0-yes1-1 | 100 | 200 | 12 | aim-200-6_0-yes1-1 | 200 | 1200 |
In the search phase, the traditional DHNN-3SAT-WA model directly examines 10, 000 different combinations of initial neuron assignments [46]. DHNN-3SAT-GA and DHNN-3SAT-ICA guide the search among these 10, 000 combinations using a genetic algorithm and an imperialistic competition algorithm, respectively. This paper introduces the DHNN-3SAT-GAKM model, which utilizes genetic optimization K-modes clustering to preprocess these 10000 neuron initial allocation combinations. It then selects a candidate subset for search. This approach reduces the actual search space and minimizes repeated local searches to avoid getting stuck in local minima, thereby improving the efficiency of retrieving the global minimum. The tolerance values for the conventional DHNN-3SAT-WA model align with Sathasivam's work [16]. The CPU time thresholds are based on Zamri's settings [47]. The parameter settings can be found in Table 6. The parameter settings for the DHNN-3SAT-GA model are in line with Kasihmuddin's work [26] and are listed in Table 7. The parameter settings for the DHNN-3SAT-ICA model remain consistent with Shazli's work [38], as shown in Table 8. Table 9 details the parameter settings of the model in this paper, with optimization of the relevant parameters through iterative tuning.
parametric | parameter value | parametric | parameter value |
Initial assigned amount | 10000 | tolerance value δ | 0.001 |
CPU time threshold | 24 hours | - | - |
parametric | parameter value | parametric | parameter value |
Initial assigned amount | 10000 | probability of mutation pm | 0.05 |
population size | 50 | Maximum Iterations t | 100 |
crossover probability pc | 0.6 | - | - |
parametric | parameter value | parametric | parameter value |
Initial assigned amount | 10000 | revolutionary rate α | 0.3 |
population size | 50 | Maximum Iterations t | 100 |
parametric | parameter value | parametric | parameter value |
Initial assigned amount | 10000 | crossover probability pc | 0.6 |
population size | 50 | probability of mutation pm | 0.05 |
Initial number of clusters | 3 | Maximum Iterations t | 100 |
We use the global minimum ratio (GMR) [16] and the mean CPU time (MCT) to assess the model's performance in this paper. To provide a more comprehensive evaluation of the model's ability to find the global minimum, we introduce 2 new evaluation metrics: the mean minimum Hamming distance (MMHD) and the mean logical satisfiability ratio (MLSR). This study will utilize a total of 4 evaluation metrics, as detailed in Table 10. The calculations are based on the average of 100 repeated experiment runs for each instance, and the results are displayed in Tables 11 and 12. Figures 9 to 12 compare our model, DHNN-3SAT-GAKM, with the models DHNN-3SAT-WA, DHNN-3SAT-GA, and DHNN-3SAT-ICA across the 4 evaluation metrics.
Indicators | calculation formula | instructions |
GMR | GMR=NGMT | GMR represents the ratio of the global minimum solution to the total number of runs [16]. GMR is an effective metric for assessing the efficiency of an algorithm. A model is considered robust when its GMR value is close to 1 [23]. Here, NGM represents the number of times the global minimum is converged, and T represents the total number of runs. |
MCT | MCT=1NGM∑TGMiNTi | The MCT refers to the average time needed for each model to reach the global minimum. A smaller MCT indicates that the model is more efficient in finding the global minimum. NTi represents the CPU time needed to find the global minimum at the i th retrieval result, and NGM represents the number of times the global minimum converged. |
MMHD | MMHD=1T∑TiminjD(Xi,Zj) | The MMHD value represents the mean minimum Hamming distance, which is the average of the smallest bit difference between the retrieval result of each run and the global minimum. When the MMHD value is closer to 0, it indicates that the model retrieves a result closer to the global minimum. In this context, D(Xi,Zj) represents the Hamming distance between the retrieval result of the i th run and the global minimum, and T represents the total number of runs. |
MLSR | MLSR=1T∑TiNsat(i)m | The MLSR value indicates the average proportion of the total number of clauses that can be satisfied by the retrieval results. The closer the MLSR value is to 1, the closer the model retrieval results are to the global minimum. Here, Nsat(i) denotes the number of satisfying clauses for the i th retrieval result, and m denotes the total number of clauses. |
No. |
GMR | MCT | MMHD | MLSR | ||||
DHNN-3SAT-WA | DHNN-3SAT-GA | DHNN-3SAT-WA | DHNN-3SAT-GA | DHNN-3SAT-WA | DHNN-3SAT-GA | DHNN-3SAT-WA | DHNN-3SAT-GA | |
1 | 1.0000 | 1.0000 | 4.83 | 2.51 | 0.0000 | 0.0000 | 1 | 1 |
2 | 0.9123 | 0.9323 | 16.67 | 11.70 | 1.1000 | 1.0900 | 0.9744 | 0.9745 |
3 | 0.8234 | 0.8456 | 26.67 | 22.83 | 3.6000 | 3.4900 | 0.9355 | 0.9578 |
4 | 0.5802 | 0.6204 | 48.82 | 45.80 | 3.7200 | 3.7100 | 0.8923 | 0.8966 |
5 | 1.0000 | 1.0000 | 11.72 | 6.31 | 0.0000 | 0.0000 | 1 | 1 |
6 | 0.8812 | 0.9011 | 46.51 | 16.01 | 2.5000 | 2.4000 | 0.9433 | 0.9533 |
7 | 0.5467 | 0.6041 | 113.28 | 35.50 | 3.6200 | 3.6100 | 0.9288 | 0.9363 |
8 | 0.2018 | 0.2188 | 440.39 | 171.23 | 4.7400 | 4.2300 | 0.9139 | 0.9231 |
9 | 0.4114 | 0.4222 | 537.92 | 431.04 | 4.8600 | 4.4500 | 0.9835 | 0.9844 |
10 | 0.2261 | 0.2352 | 978.78 | 773.75 | 5.9800 | 5.6700 | 0.9312 | 0.9474 |
11 | 0.1616 | 0.1653 | 1369.44 | 1100.94 | 7.1000 | 6.8900 | 0.8537 | 0.8775 |
12 | 0.1413 | 0.1518 | 1566.18 | 1198.85 | 8.2200 | 8.1100 | 0.8234 | 0.8641 |
No. |
GMR | MCT | MMHD | MLSR | ||||
DHNN-3SAT-ICA | DHNN-3SAT-GAKM | DHNN-3SAT-ICA | DHNN-3SAT-GAKM | DHNN-3SAT-ICA | DHNN-3SAT-GAKM | DHNN-3SAT-ICA | DHNN-3SAT-GAKM | |
1 | 1.0000 | 1.0000 | 2.49 | 2.21 | 0.0000 | 0.0000 | 1 | 1 |
2 | 0.9441 | 0.9658 | 10.64 | 8.29 | 1.0700 | 1.0400 | 0.9761 | 0.9783 |
3 | 0.8542 | 0.9126 | 20.45 | 15.08 | 3.2700 | 1.1400 | 0.9662 | 0.9751 |
4 | 0.6356 | 0.7229 | 40.18 | 26.87 | 3.3700 | 2.9700 | 0.8978 | 0.9354 |
5 | 1.0000 | 1.0000 | 5.49 | 4.68 | 0.0000 | 0.0000 | 1 | 1 |
6 | 0.9124 | 0.9256 | 14.02 | 11.06 | 2.2000 | 2.1000 | 0.9644 | 0.9881 |
7 | 0.6205 | 0.6898 | 30.59 | 22.03 | 3.2000 | 2.9300 | 0.9375 | 0.9523 |
8 | 0.2291 | 0.3211 | 141.54 | 74.60 | 3.9800 | 3.7600 | 0.9251 | 0.9336 |
9 | 0.4301 | 0.4503 | 435.12 | 396.82 | 4.0800 | 3.8900 | 0.9851 | 0.9928 |
10 | 0.2488 | 0.2632 | 752.20 | 678.91 | 5.0800 | 4.7200 | 0.9488 | 0.9557 |
11 | 0.1689 | 0.2203 | 1108.03 | 935.02 | 6.0800 | 5.5500 | 0.8809 | 0.9028 |
12 | 0.1612 | 0.2097 | 1160.96 | 982.28 | 7.0800 | 6.3800 | 0.8732 | 0.8822 |
Tables 11 and 12 show the computational results of the DHNN-3SAT-GAKM model in this paper, as well as the DHNN-3SAT-WA, DHNN-3SAT-GA, and DHNN-3SAT-ICA models. The results are presented in terms of GMR, MCT, MMHD, and MLSR. These calculations are based on the metrics formulas provided in Table 10. Figures 9 to 12 illustrate the performance differences between this paper's DHNN-3SAT-GAKM model and the DHNN-3SAT-WA, DHNN-3SAT-GA, and DHNN-3SAT-ICA models. These differences are shown in the four evaluation metrics through radargram-based visualization. The DHNN-3SAT-GAKM model outperforms the DHNN-3SAT-WA, DHNN-3SAT-GA, and DHNN-3SAT-ICA models.
Figure 9 shows the GMR of each model in solving 3-SAT instances with varying levels of complexity. In this paper, the DHNN-3SAT-GAKM model achieved the highest GMR value, indicating its superior global retrieval ability and ability to avoid falling into local minima to some extent. Additionally, the DHNN-3SAT-GA and DHNN-3SAT-ICA models also demonstrated an improved ability over the traditional DHNN-3SAT-WA model to retrieve the global minimum to some extent. As the complexity of SAT problems, Boolean variables, and logical clauses increases, the GMRs of each model decrease rapidly. Hence, further optimization and improvement of the algorithms and architectures of the DHNN-3SAT models are needed to enhance their performance and efficiency when dealing with large-scale and complex SAT problems in the future.
In Figure 10, we can see the average time taken by each model to reach the global minimum. The MTC value of the DHNN-3SAT-GAKM model in this paper is the smallest, indicating that this model is more efficient in finding the global minimum. This is because the model initially clusters the allocation space using the K-modes clustering algorithm, enabling it to escape local minima more quickly and avoid repetitive retrieval of local minima. As a result, a large number of redundant calculations are reduced, leading to improved efficiency in converging to the global minimum. On the other hand, the traditional DHNN-3SAT-WA is more prone to getting stuck in local minima, especially as the number of local minimum solutions increases, resulting in a substantial number of repetitive evolutions and computations, ultimately affecting the efficiency of converging to the global minimum. While the DHNN-3SAT-GA and DHNN-3SAT-ICA models also use heuristic algorithms for guided search to some extent, helping to reduce the search space and speed up retrieval of the global minimum, the rapidly expanding search space due to the increasing complexity of the SAT problem can lead to longer retrieval times or even search failure. Consequently, for large-scale SAT problems, further improving the efficiency of searching for the global minimum is a future priority.
In dealing with large-scale SAT problems, the increasing logic complexity results in a progressively smaller solution space, making it very challenging to find a global minimum solution within a limited timeframe. Most attempts only end up finding the local minimum solution. The goal of model optimization at this stage is to make each retrieval result as close as possible to the global minimum solution. To evaluate the proximity of the model retrieval results to the global minimum solution, two new evaluation criteria are introduced in this study: the MMHD and the MLSR. These criteria reflect the proximity of the model retrieval results to the global optimum. A lower MMHD value and a higher MLSR value indicate that the model retrieval results are closer to the global minimum solution. Figures 11 and 12 illustrate the relationship between the MMHD and MLSR values of each model. These figures show that the DHNN-3SAT-GAKM model in this paper has the smallest MMHD value and the largest MLSR value, indicating that its retrieval results are closer to the global minimum than those of the DHNN-3SAT-WA, DHNN-3SAT-GA, and DHNN-3SAT-ICA models. This suggests that the retrieval results of the DHNN-3SAT-GAKM model are closer to the global minimum solution overall. Conversely, the DHNN-3SAT-GA and DHNN-3SAT-ICA models are closer to the global minimum than the overall retrieval results of the conventional DHNN-3SAT-WA.
Based on the combined analyses above, it can be observed that the DHNN-3SAT-GAKM model, introduced in this paper, exhibits significant improvements when compared to the traditional DHNN-3SAT-WA model, as well as the DHNN-3SAT-GA and DHNN-3SAT-ICA models that directly utilize heuristic algorithms for bootstrap retrieval. This demonstrates the superior performance of the DHNN-3SAT-GAKM model in retrieving the global minima in the SAT problem, while also highlighting its potential for practical applications.
This paper introduces a method for designing network synaptic weights based on basic logical clauses to handle dynamic changes in constraints in the SAT problem. This method aims to utilize synaptic weight information efficiently, reducing the need for repetitive calculations in the DHNN network. Additionally, it proposes a DHNN-3SAT model based on genetic algorithm optimized K-modes clustering to address the limitations of the traditional DHNN-3SAT-WA, which tends to get stuck in local minima. The new model uses genetic algorithms to cluster the initial space, effectively reducing the retrieval space and improving retrieval efficiency. Experimental results show that the DHNN-3SAT-GAKM model outperforms DHNN-3SAT-WA, DHNN-3SAT-GA, and DHNN-3SAT-ICA in terms of various evaluation metrics, including GMR, MCT, MMHD, and MLSR. This study not only expands the application of DHNN in solving the SAT problem but also offers valuable insights for future research.
The DHNN-3SAT model is an innovative approach to using deep learning technology to solve SAT problems, offering insights and potential for future research and applications. There are several areas for future work: first, optimizing and enhancing the algorithm and architecture of the DHNN-3SAT model to improve its performance on large-scale and complex SAT problems; second, exploring the model's extension to other NP-complete problems to demonstrate its versatility and applicability; and finally, conducting thorough research on the model in specific domains and practical applications to further promote the use of deep learning techniques in combinatorial optimization and decision-making problems. In summary, the proposal and study of the DHNN-3SAT model not only enhances methods in the field of SAT problem-solving but also provides new ideas and tools for solving complex problems using deep learning techniques. With ongoing technological and theoretical progress, the application of deep learning in combinatorial optimization problem-solving is expected to bring about broader development and deliver effective solutions for real-life complex problems.
Xiaojun Xie: Writing-review & editing, Writing-original draft, Methodology, Formal analysis, Conceptualization. Saratha Sathasivam: Writing-review & editing, Methodology, Funding acquisition, Conceptualization, Validation, Supervision. Hong Ma: Writing-review & editing, Writing-original draft, Methodology, Investigation, Formal analysis, Conceptualization. All authors have read and approved the final version of the manuscript for publication.
This research was supported by the Ministry of Higher Education Malaysia (MOHE) through the Fundamental Research Grant Scheme (FRGS), FRGS/1/2022/STG06/USM/02/11, and University Sains Malaysia.
All authors declare no conflicts of interest in this paper.
[1] | Ader R, Felten DL, Cohen N (2001) Psychoneuroimmunology New York: Academic Press. |
[2] |
Seifert P, Spitznas M (2001) Tumours may be innervated. Virchows Arch 438: 228-231. doi: 10.1007/s004280000306
![]() |
[3] |
Seifert P, Benedic M, Effert P (2002) Nerve fibers in tumors of the human urinary bladder. Virchows Arch 440: 291-297. doi: 10.1007/s004280100496
![]() |
[4] |
Lu SH, Zhou Y, Que HP, et al. (2003) Peptidergic innervation of human esophageal and cardiac carcinoma. World J Gastroenterol 9: 399-403. doi: 10.3748/wjg.v9.i3.399
![]() |
[5] | Liang YJ, Zhou P, Wongba W, et al. (2010) Pulmonary innervation, inflammation and carcinogenesis. Sheng Li Xue Bao 62: 191-195. |
[6] |
Priyanka HP, ThyagaRajan S (2013) Selective modulation of lymphoproliferation and cytokine production via intracellular signaling targets by α1- and α2-adrenoceptors and estrogen in splenocytes. Int Immunopharmacol 17: 774-784. doi: 10.1016/j.intimp.2013.08.020
![]() |
[7] | Meites J, Quadri SK (1987) Neuroendocrine theories of aging. The Encyclopedia of Aging New York: Springer, 474-478. |
[8] |
ThyagaRajan S, MohanKumar PS, Quadri SK (1995) Cyclic changes in the release of norepinephrine and dopamine in the medial basal hypothalamus: effects of aging. Brain Res 689: 122-128. doi: 10.1016/0006-8993(95)00551-Z
![]() |
[9] | ThyagaRajan S, Priyanka HP (2012) Bidirectional communication between the neuroendocrine system and the immune system: relevance to health and diseases. Ann Neurosci 19: 40-46. |
[10] |
Mravec B, Gidron Y, Hulin I (2008) Neurobiology of cancer: interactions between nervous, endocrine and immune systems as a base for monitoring and modulating the tumorigenesis by the brain. Semin Cancer Biol 18: 150-163. doi: 10.1016/j.semcancer.2007.12.002
![]() |
[11] |
Mukhtar RA, Nseyo O, Campbell MJ, et al. (2011) Tumor-associated mac-rophages in breast cancer as potential biomarkers for new treatments and diagnostics. Expert Rev Mol Diagn 11: 91-100. doi: 10.1586/erm.10.97
![]() |
[12] |
Müller A, Homey B, Soto H, et al. (2001) Involvement of chemokine receptors in breast cancer metastasis. Nature 410: 50-56. doi: 10.1038/35065016
![]() |
[13] |
Hanahan D, Coussens LM (2012) Accessories to the crime: functions of cells recruited to the tumor microenvironment. Cancer Cell 21: 309-322. doi: 10.1016/j.ccr.2012.02.022
![]() |
[14] |
Hanahan D, Weinberg RA (2011) Hallmarks of cancer: the next generation. Cell 144: 646-674. doi: 10.1016/j.cell.2011.02.013
![]() |
[15] |
Cole SW, Nagaraja AS, Lutgendorf SK, et al. (2015) Sympathetic nervous system regulation of the tumour microenvironment. Nat Rev Cancer 15: 563-572. doi: 10.1038/nrc3978
![]() |
[16] |
Segerstrom SC, Miller GE (2004) Psychological stress and the human immune system: a meta-analytic study of 30 years of inquiry. Psychol Bull 130: 601-630. doi: 10.1037/0033-2909.130.4.601
![]() |
[17] |
Greten FR, Grivennikov SI (2019) Inflammation and cancer: triggers, mechanisms, and consequences. Immunity 51: 27-41. doi: 10.1016/j.immuni.2019.06.025
![]() |
[18] |
Yang H, Xia L, Chen J, et al. (2019) Stress-glucocorticoid-TSC22D3 axis compromises therapy-induced antitumor immunity. Nat Med 25: 1428-1441. doi: 10.1038/s41591-019-0566-4
![]() |
[19] |
Curtin NM, Boyle NT, Mills KH, et al. (2009) Psychological stress suppresses innate IFN-gamma production via glucocorticoid receptor activation: reversal by the anxiolytic chlordiazepoxide. Brain Behav Immun 23: 535-547. doi: 10.1016/j.bbi.2009.02.003
![]() |
[20] |
Key TJ (1995) Hormones and cancer in humans. Mutat Res 333: 59-67. doi: 10.1016/0027-5107(95)00132-8
![]() |
[21] |
Mravec B, Tibensky M, Horvathova L (2020) Stress and cancer. Part I: Mechanisms mediating the effect of stressors on cancer. J Neuroimmunol 346: 577311. doi: 10.1016/j.jneuroim.2020.577311
![]() |
[22] |
Yin W, Gore AC (2006) Neuroendocrine control of reproductive aging: roles of GnRH neurons. Reproduction 131: 403-414. doi: 10.1530/rep.1.00617
![]() |
[23] | Reed BG, Carr BR (2000) The Normal Menstrual Cycle and the Control of Ovulation. Endotext South Dartmouth (MA): MDText.com, Inc. |
[24] |
Del Río JP, Alliende MI, Molina N, et al. (2018) Steroid Hormones and Their Action in Women's Brains: The Importance of Hormonal Balance. Front Public Health 6: 141. doi: 10.3389/fpubh.2018.00141
![]() |
[25] |
Djahanbakhch O, Ezzati M, Zosmer A (2007) Reproductive ageing in women. J Pathol 211: 219-231. doi: 10.1002/path.2108
![]() |
[26] | Braunwald E, Isselbacher KJ, Petersdorf RG, et al. (1987) Harrison's Principles of Internal Medicine New York: McGraw-Hill, 1818-1837. |
[27] |
Priyanka HP, Nair RS (2020) Neuroimmunomodulation by estrogen in health and disease. AIMS Neurosci 7: 401-417. doi: 10.3934/Neuroscience.2020025
![]() |
[28] |
Sherman BM, West JH, Korenman SG (1976) The menopausal transition: analysis of LH, FSH, estradiol, and progesterone concentrations during menstrual cycles of older women. J Clin Endocrinol Metab 42: 629-636. doi: 10.1210/jcem-42-4-629
![]() |
[29] |
Hall JE, Gill S (2001) Neuroendocrine aspects of aging of aging in women. Endocrinol Metab Clin North Am 30: 631-646. doi: 10.1016/S0889-8529(05)70205-X
![]() |
[30] |
Lang TJ (2004) Estrogen as an immunomodulator. Clin Immunol 113: 224-230. doi: 10.1016/j.clim.2004.05.011
![]() |
[31] |
Salem ML (2004) Estrogen, a double-edged sword: modulation of TH1- and TH2-mediated inflammations by differential regulation of TH1/TH2 cytokine production. Curr Drug Targets Inflamm Allergy 3: 97-104. doi: 10.2174/1568010043483944
![]() |
[32] |
Wise PM, Scarbrough K, Lloyd J, et al. (1994) Neuroendocrine concomitants of reproductive aging. Exp Gerontol 29: 275-283. doi: 10.1016/0531-5565(94)90007-8
![]() |
[33] |
Chakrabarti M, Haque A, Banik NL, et al. (2014) Estrogen receptor agonists for attenuation of neuroinflammation and neurodegeneration. Brain Res Bull 109: 22. doi: 10.1016/j.brainresbull.2014.09.004
![]() |
[34] |
ThyagaRajan S, Madden KS, Teruya B, et al. (2011) Age-associated alterations in sympathetic noradrenergic innervation of primary and secondary lymphoid organs in female Fischer 344 rats. J Neuroimmunol 233: 54-64. doi: 10.1016/j.jneuroim.2010.11.012
![]() |
[35] |
Teilmann SC, Clement CA, Thorup J, et al. (2006) Expression and localization of the progesterone receptor in mouse and human reproductive organs. J Endocrinol 191: 525-535. doi: 10.1677/joe.1.06565
![]() |
[36] |
Butts CL, Shukair SA, Duncan KM, et al. (2007) Progesterone inhibits mature rat dendritic cells in a receptor-mediated fashion. Int Immunol 19: 287-296. doi: 10.1093/intimm/dxl145
![]() |
[37] |
Jones LA, Kreem S, Shweash M, et al. (2010) Differential modulation of TLR3- and TLR4-mediated dendritic cell maturation and function by progesterone. J Immunol 185: 4525-4534. doi: 10.4049/jimmunol.0901155
![]() |
[38] |
Menzies FM, Henriquez FL, Alexander J, et al. (2011) Selective inhibition and augmentation of alternative macrophage activation by progesterone. Immunology 134: 281-291. doi: 10.1111/j.1365-2567.2011.03488.x
![]() |
[39] |
Hardy DB, Janowski BA, Corey DR, et al. (2006) Progesterone receptor plays a major antiinflammatory role in human myometrial cells by antagonism of nuclear factor-κB activation of cyclooxygenase 2 expression. Mol Endocrinol 20: 2724-2733. doi: 10.1210/me.2006-0112
![]() |
[40] |
Arruvito L, Giulianelli S, Flores AC, et al. (2008) NK cells expressing a progesterone receptor are susceptible to progesterone-induced apoptosis. J Immunol 180: 5746-5753. doi: 10.4049/jimmunol.180.8.5746
![]() |
[41] | Piccinni MP, Giudizi MG, Biagiotti R, et al. (1995) Progesterone favors the development of human T helper cells producing TH2-type cytokines and promotes both IL-4 production and membrane CD30 expression in established TH1 cell clones. J Immunol 155: 128-133. |
[42] |
Özdemir BC, Dotto GP (2019) Sex hormones an anticancer immunity. Clin Cancer Res 25: 4603-4610. doi: 10.1158/1078-0432.CCR-19-0137
![]() |
[43] |
Beral V (2003) Breast cancer and hormone-replacement therapy in the Million Women Study. Lancet 362: 419-427. doi: 10.1016/S0140-6736(03)14596-5
![]() |
[44] |
Grady D, Gebretsadik T, Kerlikowske K, et al. (1995) Hormone replacement therapy and endometrial cancer risk: a meta-analysis. Obstet Gynecol 85: 304-313. doi: 10.1016/0029-7844(94)00383-O
![]() |
[45] |
Lacey JV, Mink PJ, Lubin JH, et al. (2002) Menopausal hormone replacement therapy and risk of ovarian cancer. JAMA 288: 334-341. doi: 10.1001/jama.288.3.334
![]() |
[46] |
Fournier A, Berrino F, Clavel-Chapelon F (2008) Unequal risks for breast cancer associated with different hormone replacement therapies results from the E3N cohort study. Breast Cancer Res Treat 107: 103-111. doi: 10.1007/s10549-007-9523-x
![]() |
[47] |
Anderson GL, Limacher M, Assaf AR, et al. (2004) Effects of conjugated equine estrogen in postmenopausal women with hysterectomy: the Women's Health Initiative randomized controlled trial. JAMA 291: 1701-1712. doi: 10.1001/jama.291.14.1701
![]() |
[48] |
Key TJ, Pike MC (1988) The dose-effect relationship between ‘unopposed’ oestrogens and endometrial mitotic rate: its central role in explaining and predicting endometrial cancer risk. Br J Cancer 57: 205-212. doi: 10.1038/bjc.1988.44
![]() |
[49] |
Cook MB, Dawsey SM, Freedman ND, et al. (2009) Sex disparities in cancer incidence by period and age. Cancer Epidemiol Biomarkers Prev 18: 1174-1182. doi: 10.1158/1055-9965.EPI-08-1118
![]() |
[50] |
Cook MB, McGlynn KA, Devesa SS, et al. (2011) Sex disparities in cancer mortality and survival. Cancer Epidemiol. Biomarkers Prev 20: 1629-1637. doi: 10.1158/1055-9965.EPI-11-0246
![]() |
[51] |
Lista P, Straface E, Brunelleschi S, et al. (2011) On the role of autophagy in human diseases: a gender perspective. J Cell Mol Med 15: 1443-1457. doi: 10.1111/j.1582-4934.2011.01293.x
![]() |
[52] |
Lin PY, Sun L, Thibodeaux SR, et al. (2010) B7-H1-dependent sex-related differences in tumor immunity and immunotherapy responses. J Immunol 185: 2747-2753. doi: 10.4049/jimmunol.1000496
![]() |
[53] |
Polanczyk MJ, Hopke C, Vandenbark AA, et al. (2006) Estrogen-mediated immunomodulation involves reduced activation of effector T cells, potentiation of Treg cells, and enhanced expression of the PD-1 costimulatory pathway. J Neurosci Res 84: 370-378. doi: 10.1002/jnr.20881
![]() |
[54] |
Klein SL, Flanagan KL (2016) Sex differences in immune responses. Nature Rev Immunol 16: 626-638. doi: 10.1038/nri.2016.90
![]() |
[55] |
Nance DM, Sanders VM (2007) Autonomic innervation and regulation of the immune system. Brain Behav Immun 21: 736-745. doi: 10.1016/j.bbi.2007.03.008
![]() |
[56] |
Priyanka HP, Pratap UP, Singh RV, et al. (2014) Estrogen modulates β2-adrenoceptor-induced cell-mediated and inflammatory immune responses through ER-α involving distinct intracellular signaling pathways, antioxidant enzymes, and nitric oxide. Cell Immunol 292: 1-8. doi: 10.1016/j.cellimm.2014.08.001
![]() |
[57] |
Priyanka HP, Krishnan HC, Singh RV, et al. (2013) Estrogen modulates in vitro T cell responses in a concentration- and receptor dependent manner: effects on intracellular molecular targets and antioxidant enzymes. Mol Immunol 56: 328-339. doi: 10.1016/j.molimm.2013.05.226
![]() |
[58] |
Pratap UP, Patil A, Sharma HR, et al. (2016) Estrogen-induced neuroprotective and anti-inflammatory effects are dependent on the brain areas of middle-aged female rats. Brain Res Bull 124: 238-253. doi: 10.1016/j.brainresbull.2016.05.015
![]() |
[59] |
Kale P, Mohanty A, Mishra M, et al. (2014) Estrogen modulates neural–immune interactions through intracellular signaling pathways and antioxidant enzyme activity in the spleen of middle-aged ovariectomized female rats. J Neuroimmunol 267: 7-15. doi: 10.1016/j.jneuroim.2013.11.003
![]() |
[60] |
Priyanka HP, Sharma U, Gopinath S, et al. (2013) Menstrual cycle and reproductive aging alters immune reactivity, NGF expression, antioxidant enzyme activities, and intracellular signaling pathways in the peripheral blood mononuclear cells of healthy women. Brain Behav Immun 32: 131-143. doi: 10.1016/j.bbi.2013.03.008
![]() |
[61] |
Ulrich-Lai YM, Herman JP (2009) Neural regulation of endocrine and autoimmune stress responses. Nat Rev Neurosci 10: 397-409. doi: 10.1038/nrn2647
![]() |
[62] |
Herman JP, Flak J, Jankord R (2008) Chronic stress plasticity in the hypothalamic paraventricular nucleus. Prog Brain Res 170: 353-364. doi: 10.1016/S0079-6123(08)00429-9
![]() |
[63] |
Iftikhar A, Islam M, Shepherd S, et al. (2021) Cancer and Stress: Does It Make a Difference to the Patient When These Two Challenges Collide? Cancers (Basel) 13: 163. doi: 10.3390/cancers13020163
![]() |
[64] |
Lin KT, Wang LH (2016) New dimension of glucocorticoids in cancer treatment. Steroids 111: 84-88. doi: 10.1016/j.steroids.2016.02.019
![]() |
[65] |
Antoni MH, Lutgendorf SK, Cole SW, et al. (2006) The influence of bio-behavioural factors on tumour biology: pathways and mechanisms. Nat Rev Cancer 6: 240-248. doi: 10.1038/nrc1820
![]() |
[66] |
Lutgendorf SK, Costanzo E, Siegel S (2007) Psychosocial influences in oncology: An expanded model of biobehavioral mechanisms. Psychoneuroimmunology New York, NY, USA: Academic Press, 869-895. doi: 10.1016/B978-012088576-3/50048-4
![]() |
[67] |
Xie H, Li B, Li L, et al. (2014) Association of increased circulating catecholamine and glucocorticoid levels with risk of psychological problems in oral neoplasm patients. PLoS One 9: e99179. doi: 10.1371/journal.pone.0099179
![]() |
[68] |
Lutgendorf SK, DeGeest K, Dahmoush L, et al. (2011) Social isolation is associated with elevated tumor norepinephrine in ovarian carcinoma patients. Brain Behav Immun 25: 250-255. doi: 10.1016/j.bbi.2010.10.012
![]() |
[69] |
Obeid EI, Conzen SD (2013) The role of adrenergic signaling in breast cancer biology. Cancer Biomark 13: 161-169. doi: 10.3233/CBM-130347
![]() |
[70] |
Mravec B, Horvathova L, Hunakova L (2020) Neurobiology of cancer: the role of β-adrenergic receptor signaling in various tumor environments. Int J Mol Sci 21: 7958. doi: 10.3390/ijms21217958
![]() |
[71] |
Thaker PH, Han LY, Kamat AA, et al. (2006) Chronic stress promotes tumor growth and angiogenesis in a mouse model of ovarian carcinoma. Nat Med 12: 939-944. doi: 10.1038/nm1447
![]() |
[72] |
Lin Q, Wang F, Yang R, et al. (2013) Effect of chronic restraint stress on human colorectal carcinoma growth in mice. PLoS One 8: e61435. doi: 10.1371/journal.pone.0061435
![]() |
[73] |
Landen CN, Lin YG, Armaiz Pena GN, et al. (2007) Neuroendocrine modulation of signal transducer and activator of transcription-3 in ovarian cancer. Cancer Res 67: 10389-10396. doi: 10.1158/0008-5472.CAN-07-0858
![]() |
[74] |
Shang ZJ, Liu K, Liang DF (2009) Expression of beta2-adrenergic receptor in oral squamous cell carcinoma. J Oral Pathol Med 38: 371-376. doi: 10.1111/j.1600-0714.2008.00691.x
![]() |
[75] |
Saul AN, Oberyszyn TM, Daugherty C, et al. (2005) Chronic stress and susceptibility to skin cancer. J Natl Cancer Inst 97: 1760-1767. doi: 10.1093/jnci/dji401
![]() |
[76] |
Ben-Eliyahu S, Page GG, Yirmiya R, et al. (1999) Evidence that stress and surgical interventions promote tumor development by suppressing natural killer cell activity. Int J Cancer 80: 880-888. doi: 10.1002/(SICI)1097-0215(19990315)80:6<880::AID-IJC14>3.0.CO;2-Y
![]() |
[77] |
Ben-Eliyahu S, Yirmiya R, Liebeskind JC, et al. (1991) Stress increases metastatic spread of a mammary tumor in rats: evidence for mediation by the immune system. Brain Behav Immun 5: 193-205. doi: 10.1016/0889-1591(91)90016-4
![]() |
[78] |
Greenfeld K, Avraham R, Benish M, et al. (2007) Immune suppression while awaiting surgery and following it: dissociations between plasma cytokine levels, their induced production, and NK cell cytotoxicity. Brain Behav Immun 21: 503-513. doi: 10.1016/j.bbi.2006.12.006
![]() |
[79] |
Morris N, Moghaddam N, Tickle A, et al. (2018) The relationship between coping style and psychological distress in people with head and neck cancer: A systematic review. Psychooncology 27: 734-747. doi: 10.1002/pon.4509
![]() |
[80] | Nogueira TE, Adorno M, Mendonça E, et al. (2018) Factors associated with the quality of life of subjects with facial disfigurement due to surgical treatment of head and neck cancer. Med Oral Patol Oral Cir Bucal 23: e132-e137. |
[81] |
Hagedoorn M, Molleman E (2006) Facial disfigurement in patients with head and neck cancer: the role of social self-efficacy. Health Psychol 25: 643-647. doi: 10.1037/0278-6133.25.5.643
![]() |
[82] |
Lackovicova L, Gaykema RP, Banovska L, et al. (2013) The time-course of hindbrain neuronal activity varies according to location during either intraperitoneal or subcutaneous tumor growth in rats: Single Fos and dual Fos/dopamine beta-hydroxylase immunohistochemistry. J Neuroimmunol 260: 37-46. doi: 10.1016/j.jneuroim.2013.04.010
![]() |
[83] |
Horvathova L, Tillinger A, Padova A, et al. (2020) Changes in gene expression in brain structures related to visceral sensation, autonomic functions, food intake, and cognition in melanoma-bearing mice. Eur J Neurosci 51: 2376-2393. doi: 10.1111/ejn.14661
![]() |
[84] |
Kin NW, Sanders VM (2006) It takes nerve to tell T and B cells what to do. J Leukocyte Biol 79: 1093-1104. doi: 10.1189/jlb.1105625
![]() |
[85] |
Straub RH (2004) Complexity of the bi-directional neuroimmune junction in the spleen. Trends Pharmacol Sci 25: 640-646. doi: 10.1016/j.tips.2004.10.007
![]() |
[86] |
Nance DM, Sanders VM (2007) Autonomic innervation and regulation of the immune system (1987–2007). Brain Behav Immun 21: 736-745. doi: 10.1016/j.bbi.2007.03.008
![]() |
[87] |
Madden KS (2003) Catecholamines, sympathetic innervation, and immunity. Brain Behav Immun 17: S5-10. doi: 10.1016/S0889-1591(02)00059-4
![]() |
[88] |
ThyagaRajan S, Felten DL (2002) Modulation of neuroendocrine--immune signaling by L-deprenyl and L-desmethyldeprenyl in aging and mammary cancer. Mech Ageing Dev 123: 1065-1079. doi: 10.1016/S0047-6374(01)00390-6
![]() |
[89] |
Pratap U, Hima L, Kannan T, et al. (2020) Sex-Based Differences in the Cytokine Production and Intracellular Signaling Pathways in Patients With Rheumatoid Arthritis. Arch Rheumatol 35: 545-557. doi: 10.46497/ArchRheumatol.2020.7481
![]() |
[90] |
Hima L, Patel M, Kannan T, et al. (2020) Age-associated decline in neural, endocrine, and immune responses in men and women: Involvement of intracellular signaling pathways. J Neuroimmunol 345: 577290. doi: 10.1016/j.jneuroim.2020.577290
![]() |
[91] |
Chen DS, Mellman I (2013) Oncology meets immunology: the cancer-immunity cycle. Immunity 39: 1-10. doi: 10.1016/j.immuni.2013.07.012
![]() |
[92] |
Schreiber RD, Old LJ, Smyth MJ (2011) Cancer immunoediting: integrating immunity's roles in cancer suppression and promotion. Science 331: 1565-1570. doi: 10.1126/science.1203486
![]() |
[93] |
Sanders VM (1995) The role of adrenoceptor-mediated signals in the modulation of lymphocyte function. Adv Neuroimmunol 5: 283-298. doi: 10.1016/0960-5428(95)00019-X
![]() |
[94] |
Madden KS, Felten DL (1995) Experimental basis for neural-immune interactions. Physiol Rev 75: 77-106. doi: 10.1152/physrev.1995.75.1.77
![]() |
[95] |
Madden KS (2003) Catecholamines, sympathetic innervation, and immunity. Brain Behav Immun 17: S5-10. doi: 10.1016/S0889-1591(02)00059-4
![]() |
[96] |
Callahan TA, Moynihan JA (2002) The effects of chemical sympathectomy on T-cell cytokine responses are not mediated by altered peritoneal exudate cell function or an inflammatory response. Brain Behav Immun 16: 33-45. doi: 10.1006/brbi.2000.0618
![]() |
[97] |
Madden KS, Felten SY, Felten DL, et al. (1989) Sympathetic neural modulation of the immune system. I. Depression of T cell immunity in vivo and vitro following chemical sympathectomy. Brain Behav Immun 3: 72-89. doi: 10.1016/0889-1591(89)90007-X
![]() |
[98] |
Alaniz RC, Thomas SA, Perez-Melgosa M, et al. (1999) Dopamine beta-hydroxylase deficiency impairs cellular immunity. Proc Natl Acad Sci U S A 96: 2274-2278. doi: 10.1073/pnas.96.5.2274
![]() |
[99] |
Pongratz G, McAlees JW, Conrad DH, et al. (2006) The level of IgE produced by a B cell is regulated by norepinephrine in a p38 MAPK- and CD23-dependent manner. J Immunol 177: 2926-2938. doi: 10.4049/jimmunol.177.5.2926
![]() |
[100] |
Chen F, Zhuang X, Lin L, et al. (2015) New horizons in tumor microenvironment biology: challenges and opportunities. BMC Med 13: 45. doi: 10.1186/s12916-015-0278-7
![]() |
[101] |
Wang M, Zhao J, Zhang L, et al. (2017) Role of tumor microenvironment in tumorigenesis. J Cancer 8: 761-773. doi: 10.7150/jca.17648
![]() |
[102] |
Del Prete A, Schioppa T, Tiberio L, et al. (2017) Leukocyte trafficking in tumor microenvironment. Curr Opin Pharmacol 35: 40-47. doi: 10.1016/j.coph.2017.05.004
![]() |
[103] |
Jiang Y, Li Y, Zhu B (2015) T-cell exhaustion in the tumor microenvironment. Cell Death Dis 6: e1792. doi: 10.1038/cddis.2015.162
![]() |
[104] |
Maimela NR, Liu S, Zhang Y (2019) Fates of CD8+ T cells in tumor microenvironment. Comput Struct Biotechnol J 17: 1-13. doi: 10.1016/j.csbj.2018.11.004
![]() |
[105] |
Zhang Z, Liu S, Zhang B, et al. (2020) T Cell Dysfunction and Exhaustion in Cancer. Front Cell Dev Biol 8: 17. doi: 10.3389/fcell.2020.00017
![]() |
[106] |
Gonzalez H, Robles I, Werb Z (2018) Innate and acquired immune surveillance in the postdissemination phase of metastasis. FEBS J 285: 654-664. doi: 10.1111/febs.14325
![]() |
[107] |
Komohara Y, Fujiwara Y, Ohnishi K, et al. (2016) Tumor-associated macrophages: Potential therapeutic targets for anti-cancer therapy. Adv Drug Delivery Rev 99: 180-185. doi: 10.1016/j.addr.2015.11.009
![]() |
[108] |
Mantovani A, Marchesi F, Malesci A, et al. (2017) Tumour-associated macrophages as treatment targets in oncology. Nat Rev Clin Oncol 14: 399-416. doi: 10.1038/nrclinonc.2016.217
![]() |
[109] |
Linde N, Lederle W, Depner S, et al. (2012) Vascular endothelial growth factor-induced skin carcinogenesis depends on recruitment and alternative activation of macrophages. J Pathol 227: 17-28. doi: 10.1002/path.3989
![]() |
[110] |
Komohara Y, Jinushi M, Takeya M (2014) Clinical significance of macrophage heterogeneity in human malignant tumors. Cancer Sci 105: 1-8. doi: 10.1111/cas.12314
![]() |
[111] |
Ruffell B, Coussens LM (2015) Macrophages and therapeutic resistance in cancer. Cancer Cell 27: 462-472. doi: 10.1016/j.ccell.2015.02.015
![]() |
[112] |
Noy R, Pollard JW (2014) Tumor-associated macrophages: from mechanisms to therapy. Immunity 41: 49-61. doi: 10.1016/j.immuni.2014.06.010
![]() |
[113] |
Long KB, Gladney WL, Tooker GM, et al. (2016) IFN-γ and CCL2 Cooperate to Redirect Tumor-Infiltrating Monocytes to Degrade Fibrosis and Enhance Chemotherapy Efficacy in Pancreatic Carcinoma. Cancer Discov 6: 400-413. doi: 10.1158/2159-8290.CD-15-1032
![]() |
[114] |
Muz B, de la Puente P, Azab F, et al. (2015) The role of hypoxia in cancer progression, angiogenesis, metastasis, and resistance to therapy. Hypoxia (Auckl) 3: 83-92. doi: 10.2147/HP.S93413
![]() |
[115] |
Zhang CC, Sadek HA (2014) Hypoxia and metabolic properties of hematopoietic stem cells. Antioxid Redox Signal 20: 1891-1901. doi: 10.1089/ars.2012.5019
![]() |
[116] |
Henze AT, Mazzone M (2016) The impact of hypoxia on tumor-associated macrophages. J Clin Invest 126: 3672-3679. doi: 10.1172/JCI84427
![]() |
[117] |
Chen P, Zuo H, Xiong H, et al. (2017) Gpr132 sensing of lactate mediates tumor-macrophage interplay to promote breast cancer metastasis. Proc Natl Acad Sci 114: 580-585. doi: 10.1073/pnas.1614035114
![]() |
[118] |
Albo D, Akay CL, Marshall CL, et al. (2011) Neurogenesis in colorectal cancer is a marker of aggressive tumor behavior and poor outcomes. Cancer 117: 4834-4845. doi: 10.1002/cncr.26117
![]() |
[119] |
Kamiya A, Hiyama T, Fujimura A, et al. (2021) Sympathetic and parasympathetic innervation in cancer: therapeutic implications. Clin Auton Res 31: 165-178. doi: 10.1007/s10286-020-00724-y
![]() |
[120] |
Ceyhan GO, Schäfer KH, Kerscher AG, et al. (2010) Nerve growth factor and artemin are paracrine mediators of pancreatic neuropathy in pancreatic adenocarcinoma. Ann Surg 251: 923-931. doi: 10.1097/SLA.0b013e3181d974d4
![]() |
[121] |
Zhao CM, Hayakawa Y, Kodama Y, et al. (2014) Denervation suppresses gastric tumorigenesis. Sci Transl Med 6: 250ra115. doi: 10.1126/scitranslmed.3009569
![]() |
[122] |
Liebig C, Ayala G, Wilks JA, et al. (2009) Perineural invasion in cancer: a review of the literature. Cancer 115: 3379-3391. doi: 10.1002/cncr.24396
![]() |
[123] |
Dai H, Li R, Wheeler T, et al. (2007) Enhanced survival in perineural invasion of pancreatic cancer: an in vitro approach. Hum Pathol 38: 299-307. doi: 10.1016/j.humpath.2006.08.002
![]() |
[124] |
Liebig C, Ayala G, Wilks J, et al. (2009) Perineural invasion is an independent predictor of outcome in colorectal cancer. J Clin Oncol 27: 5131-5137. doi: 10.1200/JCO.2009.22.4949
![]() |
[125] |
Conceição F, Sousa DM, Paredes J, et al. (2021) Sympathetic activity in breast cancer and metastasis: partners in crime. Bone Res 9: 9. doi: 10.1038/s41413-021-00137-1
![]() |
[126] |
Mauffrey P, Tchitchek N, Barroca V, et al. (2019) Progenitors from the central nervous system drive neurogenesis in cancer. Nature 569: 672-678. doi: 10.1038/s41586-019-1219-y
![]() |
[127] |
Ruscica M, Dozio E, Motta M, et al. (2007) Role of neuropeptide Y and its receptors in the progression of endocrine-related cancer. Peptides 28: 426-434. doi: 10.1016/j.peptides.2006.08.045
![]() |
[128] |
Hayakawa Y, Sakitani K, Konishi M, et al. (2017) Nerve Growth Factor Promotes Gastric Tumorigenesis through Aberrant Cholinergic Signaling. Cancer Cell 31: 21-34. doi: 10.1016/j.ccell.2016.11.005
![]() |
[129] |
Barabutis N (2021) Growth Hormone Releasing Hormone in Endothelial Barrier Function. Trends Endocrinol Metab 32: 338-340. doi: 10.1016/j.tem.2021.03.001
![]() |
[130] |
ThyagaRajan S, Madden KS, Kalvass JC, et al. (1998) L-deprenyl-induced increase in IL-2 and NK cell activity accompanies restoration of noradrenergic nerve fibers in the spleens of old F344 rats. J Neuroimmunol 92: 9-21. doi: 10.1016/S0165-5728(98)00039-3
![]() |
[131] |
Zumoff B (1998) Does postmenopausal estrogen administration increase the risk of breast cancer? Contributions of animal, biochemical, and clinical investigative studies to a resolution of the controversy. Proc Soc Exp Biol Med 217: 30-37. doi: 10.3181/00379727-217-44202
![]() |
[132] |
Hollingsworth AB, Lerner MR, Lightfoot SA, et al. (1998) Prevention of DMBA-induced rat mammary carcinomas comparing leuprolide, oophorectomy, and tamoxifen. Breast Cancer Res Treat 47: 63-70. doi: 10.1023/A:1005872132373
![]() |
[133] |
Sun G, Wu L, Sun G, et al. (2021) WNT5a in Colorectal Cancer: Research Progress and Challenges. Cancer Manag Res 13: 2483-2498. doi: 10.2147/CMAR.S289819
![]() |
[134] |
Schlange T, Matsuda Y, Lienhard S, et al. (2007) Autocrine WNT signaling contributes to breast cancer cell proliferation via the canonical WNT pathway and EGFR transactivation. Breast Cancer Res 9: R63. doi: 10.1186/bcr1769
![]() |
[135] |
Sun X, Bernhardt SM, Glynn DJ, et al. (2021) Attenuated TGFB signalling in macrophages decreases susceptibility to DMBA-induced mammary cancer in mice. Breast Cancer Res 23: 39. doi: 10.1186/s13058-021-01417-8
![]() |
[136] |
Heasley L (2001) Autocrine and paracrine signaling through neuropeptide receptors in human cancer. Oncogene 20: 1563-1569. doi: 10.1038/sj.onc.1204183
![]() |
[137] |
Cuttitta F, Carney DN, Mulshine J, et al. (1985) Bombesin-like peptides can function as autocrine growth factors in human small-cell lung cancer. Nature 316: 823-826. doi: 10.1038/316823a0
![]() |
[138] |
Moody TW, Pert CB, Gazdar AF, et al. (1981) Neurotensin is produced by and secreted from classic small cell lung cancer cells. Science 214: 1246-1248. doi: 10.1126/science.6272398
![]() |
[139] | Cardona C, Rabbitts PH, Spindel ER, et al. (1991) Production of neuromedin B and neuromedin B gene expression in human lung tumor cell lines. Cancer Res 51: 5205-5211. |
[140] |
Sun B, Halmos G, Schally AV, et al. (2000) Presence of receptors for bombesin/gastrin-releasing peptide and mRNA for three receptor subtypes in human prostate cancers. Prostate 42: 295-303. doi: 10.1002/(SICI)1097-0045(20000301)42:4<295::AID-PROS7>3.0.CO;2-B
![]() |
[141] | Markwalder R, Reubi JC (1999) Gastrin-releasing peptide receptors in the human prostate: relation to neoplastic transformation. Cancer Res 59: 1152-1159. |
[142] |
Moody TW, Pert CB, Gazdar AF, et al. (1981) Neurotensin is produced by and secreted from classic small cell lung cancer cells. Science 214: 1246-1248. doi: 10.1126/science.6272398
![]() |
[143] |
Goetze JP, Nielsen FC, Burcharth F, et al. (2000) Closing the gastrin loop in pancreatic carcinoma: Co-expression of gastrin and its receptor in solid human pancreatic adenocarcinoma. Cancer 88: 2487-2494. doi: 10.1002/1097-0142(20000601)88:11<2487::AID-CNCR9>3.0.CO;2-E
![]() |
[144] |
Blackmore M, Hirst B (1992) Autocrine stimulation of growth of AR4-2J rat pancreatic tumour cells by gastrin. Br J Cancer 66: 32-38. doi: 10.1038/bjc.1992.212
![]() |
[145] | Smith JP, Liu G, Soundararajan V, et al. (1994) Identification and characterization of CCK-B/gastrin receptors in human pancreatic cancer cell lines. Am J Physiol 266: R277-283. |
[146] |
Seethalakshmi L, Mitra SP, Dobner PR, et al. (1997) Neurotensin receptor expression in prostate cancer cell line and growth effect of NT at physiological concentrations. Prostate 31: 183-192. doi: 10.1002/(SICI)1097-0045(19970515)31:3<183::AID-PROS7>3.0.CO;2-M
![]() |
[147] |
Kawaguchi K, Sakurai M, Yamamoto Y, et al. (2019) Alteration of specific cytokine expression patterns in patients with breast cancer. Sci Rep 9: 2924. doi: 10.1038/s41598-019-39476-9
![]() |
[148] |
Smyth MJ, Cretney E, Kershaw MH, et al. (2004) Cytokines in cancer immunity and immunotherapy. Immunol Rev 202: 275-293. doi: 10.1111/j.0105-2896.2004.00199.x
![]() |
[149] |
Wang X, Lin Y (2008) Tumor necrosis factor and cancer, buddies or foes? Acta Pharmacol Sin 29: 1275-1288. doi: 10.1111/j.1745-7254.2008.00889.x
![]() |
[150] |
Ohri CM, Shikotra A, Green RH, et al. (2010) Tumour necrosis factor-alpha expression in tumour islets confers a survival advantage in non-small cell lung cancer. BMC Cancer 10: 323. doi: 10.1186/1471-2407-10-323
![]() |
[151] |
Woo CH, Eom YW, Yoo MH, et al. (2000) Tumor necrosis factor-α generates reactive oxygen species via a cytosolic phospholipase A2-linked cascade. J Biol Chem 275: 32357-32362. doi: 10.1074/jbc.M005638200
![]() |
[152] |
Suganuma M, Watanabe T, Yamaguchi K, et al. (2012) Human gastric cancer development with TNF-α-inducing protein secreted from Helicobacter pylori. Cancer Lett 322: 133-138. doi: 10.1016/j.canlet.2012.03.027
![]() |
[153] |
Cai X, Cao C, Li J, et al. (2017) Inflammatory factor TNF-α promotes the growth of breast cancer via the positive feedback loop of TNFR1/NF-κB (and/or p38)/p-STAT3/HBXIP/TNFR1. Oncotarget 8: 58338-58352. doi: 10.18632/oncotarget.16873
![]() |
[154] |
Fiorentino DF, Bond MW, Mosmann TR (1989) Two types of mouse T helper cell. IV. Th2 clones secrete a factor that inhibits cytokine production by Th1 clones. J Exp Med 170: 2081-2095. doi: 10.1084/jem.170.6.2081
![]() |
[155] |
Ikeguchi M, Hatada T, Yamamoto M, et al. (2009) Serum interleukin-6 and -10 levels in patients with gastric cancer. Gastric Cancer 12: 95-100. doi: 10.1007/s10120-009-0509-8
![]() |
[156] |
Hassuneh MR, Nagarkatti M, Nagarkatti PS (2013) Role of interleukin-10 in the regulation of tumorigenicity of a T cell lymphoma. Leuk Lymphoma 54: 827-834. doi: 10.3109/10428194.2012.726721
![]() |
[157] |
Hodge DR, Hurt EM, Farrar WL (2005) The role of IL-6 and STAT3 in inflammation and cancer. Eur J Cancer 41: 2502-2512. doi: 10.1016/j.ejca.2005.08.016
![]() |
[158] |
Kim SY, Kang JW, Song X, et al. (2013) Role of the IL-6-JAK1-STAT3-Oct-4 pathway in the conversion of non-stem cancer cells into cancer stem-like cells. Cell Signaling 25: 961-969. doi: 10.1016/j.cellsig.2013.01.007
![]() |
[159] |
Mravec B, Horvathova L, Cernackova A (2019) Hypothalamic Inflammation at a Crossroad of Somatic Diseases. Cell Mol Neurobiol 39: 11-29. doi: 10.1007/s10571-018-0631-4
![]() |
[160] |
Morrison CD, Parvani JG, Schiemann WP (2013) The relevance of the TGF-β Paradox to EMT-MET programs. Cancer Lett 341: 30-40. doi: 10.1016/j.canlet.2013.02.048
![]() |
[161] |
Bierie B, Moses HL (2006) TGF-β and cancer. Cytokine Growth Factor Rev 17: 29-40. doi: 10.1016/j.cytogfr.2005.09.006
![]() |
[162] |
Levy L, Hill CS (2006) Alterations in components of the TGF-β superfamily signaling pathways in human cancer. Cytokine Growth Factor Rev 17: 41-58. doi: 10.1016/j.cytogfr.2005.09.009
![]() |
Weights | w123 | w124 | w134 | w234 | w12 | w13 | w14 | w23 | w24 | w34 | w1 | w2 | w3 | w4 |
P | 116 | 116 | 0 | 0 | 0 | 18 | −18 | 18 | −18 | 0 | 14 | 14 | 18 | 18 |
Weights | Cl1 | Cl2 | Cl3 | Cl4 | Cl5 | Cl6 | Cl7 | Cl8 |
wi | 1/8 | −1/8 | 1/8 | 1/8 | −1/8 | −1/8 | 1/8 | −1/8 |
wj | 1/8 | 1/8 | −1/8 | 1/8 | −1/8 | 1/8 | −1/8 | −1/8 |
wk | 1/8 | 1/8 | 1/8 | −1/8 | 1/8 | −1/8 | −1/8 | −1/8 |
wij | −1/8 | 1/8 | 1/8 | −1/8 | −1/8 | 1/8 | 1/8 | −1/8 |
wik | −1/8 | −1/8 | 1/8 | 1/8 | 1/8 | −1/8 | 1/8 | −1/8 |
wjk | −1/8 | 1/8 | −1/8 | 1/8 | 1/8 | −1/8 | 1/8 | −1/8 |
wijk | 1/16 | −1/16 | −1/16 | −1/16 | 1/16 | 1/16 | 1/6 | −1/16 |
Weights | C11 | C12 | C13 | C14 | C15 | C16 | C17 | C21 | P |
w1 | 1/8 | −1/8 | 1/8 | 1/8 | −1/8 | −1/8 | 1/8 | 1/8 | 1/4 |
w2 | 1/8 | 1/8 | −1/8 | 1/8 | −1/8 | 1/8 | −1/8 | 1/8 | 1/4 |
w3 | 1/8 | 1/8 | 1/8 | −1/8 | 1/8 | −1/8 | −1/8 | 0 | 1/8 |
w4 | 0 | 0 | 0 | 0 | 0 | 0 | 0 | 1/8 | 1/8 |
w12 | −1/8 | 1/8 | 1/8 | −1/8 | −1/8 | 1/8 | 1/8 | −1/8 | 0 |
w13 | −1/8 | −1/8 | 1/8 | 1/8 | 1/8 | −1/8 | 1/8 | 0 | 1/8 |
w14 | 0 | 0 | 0 | 0 | 0 | 0 | 0 | −1/8 | −1/8 |
w23 | −1/8 | 1/8 | −1/8 | 1/8 | 1/8 | −1/8 | 1/8 | 0 | 1/8 |
w24 | 0 | 0 | 0 | 0 | 0 | 0 | 0 | −1/8 | −1/8 |
w34 | 0 | 0 | 0 | 0 | 0 | 0 | 0 | 0 | 0 |
w123 | 1/16 | −1/16 | −1/16 | −1/16 | 1/16 | 1/16 | 1/16 | 0 | 1/16 |
w124 | 0 | 0 | 0 | 0 | 0 | 0 | 0 | 1/16 | 1/16 |
w234 | 0 | 0 | 0 | 0 | 0 | 0 | 0 | 0 | 0 |
Weights | P | C22 | C23 | Padd | C12 | C13 | Pdec | C17 | C21 | C18 | C31 | Pupd |
w1 | 1/4 | −1/8 | 1/8 | 1/4 | −1/8 | 1/8 | 1/4 | 1/8 | 1/8 | −1/8 | 0 | −1/8 |
w2 | 1/4 | 1/8 | −1/8 | 1/4 | 1/8 | −1/8 | 1/4 | −1/8 | 1/8 | −1/8 | 1/8 | 1/4 |
w3 | 1/8 | 0 | 0 | 1/8 | 1/8 | 1/8 | −1/8 | −1/8 | 0 | −1/8 | 1/8 | 1/4 |
w4 | 1/8 | 1/8 | 1/8 | 3/8 | 0 | 0 | 1/8 | 0 | 1/8 | 0 | 1/8 | 1/8 |
w12 | 0 | 1/8 | 1/8 | 1/4 | 1/8 | 1/8 | −1/4 | 1/8 | −1/8 | −1/8 | 0 | −1/8 |
w13 | 1/8 | 0 | 0 | 1/8 | −1/8 | 1/8 | 1/8 | 1/8 | 0 | −1/8 | 0 | −1/8 |
w14 | −1/8 | −1/8 | 1/8 | −1/8 | 0 | 0 | −1/8 | 0 | −1/8 | 0 | 0 | 0 |
w23 | 1/8 | 0 | 0 | 1/8 | 1/8 | −1/8 | 1/8 | 1/8 | 0 | −1/8 | −1/8 | −1/4 |
w24 | −1/8 | 1/8 | −1/8 | −1/8 | 0 | 0 | −1/8 | 0 | −1/8 | 0 | −1/8 | −1/8 |
w34 | 0 | 0 | 0 | 0 | 0 | 0 | 0 | 0 | 0 | 0 | −1/8 | −1/8 |
w123 | 1/16 | 0 | 0 | 1/16 | −1/16 | −1/16 | 3/16 | 1/16 | 0 | −1/16 | 0 | −1/16 |
w124 | 1/16 | −1/16 | −1/16 | −1/16 | 0 | 0 | 1/16 | 0 | 1/16 | 0 | 0 | 0 |
w234 | 0 | 0 | 0 | 0 | 0 | 0 | 0 | 0 | 0 | 0 | 1/16 | 1/16 |
No. | Instance | variables | Clauses | No. | Instance | variables | Clauses |
1 | aim-50-1_6-yes1-1 | 50 | 80 | 7 | aim-100-3_4-yes1-1 | 100 | 340 |
2 | aim-50-2_0-yes1-1 | 50 | 100 | 8 | aim-100-6_0-yes1-1 | 100 | 600 |
3 | aim-50-3_4-yes1-1 | 50 | 170 | 9 | aim-200-1_6-yes1-1 | 200 | 320 |
4 | aim-50-6_0-yes1-1 | 50 | 300 | 10 | aim-200-2_0-yes1-1 | 200 | 400 |
5 | aim-100-1_6-yes1-1 | 100 | 160 | 11 | aim-200-3_4-yes1-1 | 200 | 680 |
6 | aim-100-2_0-yes1-1 | 100 | 200 | 12 | aim-200-6_0-yes1-1 | 200 | 1200 |
parametric | parameter value | parametric | parameter value |
Initial assigned amount | 10000 | tolerance value δ | 0.001 |
CPU time threshold | 24 hours | - | - |
parametric | parameter value | parametric | parameter value |
Initial assigned amount | 10000 | probability of mutation pm | 0.05 |
population size | 50 | Maximum Iterations t | 100 |
crossover probability pc | 0.6 | - | - |
parametric | parameter value | parametric | parameter value |
Initial assigned amount | 10000 | revolutionary rate α | 0.3 |
population size | 50 | Maximum Iterations t | 100 |
parametric | parameter value | parametric | parameter value |
Initial assigned amount | 10000 | crossover probability pc | 0.6 |
population size | 50 | probability of mutation pm | 0.05 |
Initial number of clusters | 3 | Maximum Iterations t | 100 |
Indicators | calculation formula | instructions |
GMR | GMR=NGMT | GMR represents the ratio of the global minimum solution to the total number of runs [16]. GMR is an effective metric for assessing the efficiency of an algorithm. A model is considered robust when its GMR value is close to 1 [23]. Here, NGM represents the number of times the global minimum is converged, and T represents the total number of runs. |
MCT | MCT=1NGM∑TGMiNTi | The MCT refers to the average time needed for each model to reach the global minimum. A smaller MCT indicates that the model is more efficient in finding the global minimum. NTi represents the CPU time needed to find the global minimum at the i th retrieval result, and NGM represents the number of times the global minimum converged. |
MMHD | MMHD=1T∑TiminjD(Xi,Zj) | The MMHD value represents the mean minimum Hamming distance, which is the average of the smallest bit difference between the retrieval result of each run and the global minimum. When the MMHD value is closer to 0, it indicates that the model retrieves a result closer to the global minimum. In this context, D(Xi,Zj) represents the Hamming distance between the retrieval result of the i th run and the global minimum, and T represents the total number of runs. |
MLSR | MLSR=1T∑TiNsat(i)m | The MLSR value indicates the average proportion of the total number of clauses that can be satisfied by the retrieval results. The closer the MLSR value is to 1, the closer the model retrieval results are to the global minimum. Here, Nsat(i) denotes the number of satisfying clauses for the i th retrieval result, and m denotes the total number of clauses. |
No. |
GMR | MCT | MMHD | MLSR | ||||
DHNN-3SAT-WA | DHNN-3SAT-GA | DHNN-3SAT-WA | DHNN-3SAT-GA | DHNN-3SAT-WA | DHNN-3SAT-GA | DHNN-3SAT-WA | DHNN-3SAT-GA | |
1 | 1.0000 | 1.0000 | 4.83 | 2.51 | 0.0000 | 0.0000 | 1 | 1 |
2 | 0.9123 | 0.9323 | 16.67 | 11.70 | 1.1000 | 1.0900 | 0.9744 | 0.9745 |
3 | 0.8234 | 0.8456 | 26.67 | 22.83 | 3.6000 | 3.4900 | 0.9355 | 0.9578 |
4 | 0.5802 | 0.6204 | 48.82 | 45.80 | 3.7200 | 3.7100 | 0.8923 | 0.8966 |
5 | 1.0000 | 1.0000 | 11.72 | 6.31 | 0.0000 | 0.0000 | 1 | 1 |
6 | 0.8812 | 0.9011 | 46.51 | 16.01 | 2.5000 | 2.4000 | 0.9433 | 0.9533 |
7 | 0.5467 | 0.6041 | 113.28 | 35.50 | 3.6200 | 3.6100 | 0.9288 | 0.9363 |
8 | 0.2018 | 0.2188 | 440.39 | 171.23 | 4.7400 | 4.2300 | 0.9139 | 0.9231 |
9 | 0.4114 | 0.4222 | 537.92 | 431.04 | 4.8600 | 4.4500 | 0.9835 | 0.9844 |
10 | 0.2261 | 0.2352 | 978.78 | 773.75 | 5.9800 | 5.6700 | 0.9312 | 0.9474 |
11 | 0.1616 | 0.1653 | 1369.44 | 1100.94 | 7.1000 | 6.8900 | 0.8537 | 0.8775 |
12 | 0.1413 | 0.1518 | 1566.18 | 1198.85 | 8.2200 | 8.1100 | 0.8234 | 0.8641 |
No. |
GMR | MCT | MMHD | MLSR | ||||
DHNN-3SAT-ICA | DHNN-3SAT-GAKM | DHNN-3SAT-ICA | DHNN-3SAT-GAKM | DHNN-3SAT-ICA | DHNN-3SAT-GAKM | DHNN-3SAT-ICA | DHNN-3SAT-GAKM | |
1 | 1.0000 | 1.0000 | 2.49 | 2.21 | 0.0000 | 0.0000 | 1 | 1 |
2 | 0.9441 | 0.9658 | 10.64 | 8.29 | 1.0700 | 1.0400 | 0.9761 | 0.9783 |
3 | 0.8542 | 0.9126 | 20.45 | 15.08 | 3.2700 | 1.1400 | 0.9662 | 0.9751 |
4 | 0.6356 | 0.7229 | 40.18 | 26.87 | 3.3700 | 2.9700 | 0.8978 | 0.9354 |
5 | 1.0000 | 1.0000 | 5.49 | 4.68 | 0.0000 | 0.0000 | 1 | 1 |
6 | 0.9124 | 0.9256 | 14.02 | 11.06 | 2.2000 | 2.1000 | 0.9644 | 0.9881 |
7 | 0.6205 | 0.6898 | 30.59 | 22.03 | 3.2000 | 2.9300 | 0.9375 | 0.9523 |
8 | 0.2291 | 0.3211 | 141.54 | 74.60 | 3.9800 | 3.7600 | 0.9251 | 0.9336 |
9 | 0.4301 | 0.4503 | 435.12 | 396.82 | 4.0800 | 3.8900 | 0.9851 | 0.9928 |
10 | 0.2488 | 0.2632 | 752.20 | 678.91 | 5.0800 | 4.7200 | 0.9488 | 0.9557 |
11 | 0.1689 | 0.2203 | 1108.03 | 935.02 | 6.0800 | 5.5500 | 0.8809 | 0.9028 |
12 | 0.1612 | 0.2097 | 1160.96 | 982.28 | 7.0800 | 6.3800 | 0.8732 | 0.8822 |
Weights | w123 | w124 | w134 | w234 | w12 | w13 | w14 | w23 | w24 | w34 | w1 | w2 | w3 | w4 |
P | 116 | 116 | 0 | 0 | 0 | 18 | −18 | 18 | −18 | 0 | 14 | 14 | 18 | 18 |
Weights | Cl1 | Cl2 | Cl3 | Cl4 | Cl5 | Cl6 | Cl7 | Cl8 |
wi | 1/8 | −1/8 | 1/8 | 1/8 | −1/8 | −1/8 | 1/8 | −1/8 |
wj | 1/8 | 1/8 | −1/8 | 1/8 | −1/8 | 1/8 | −1/8 | −1/8 |
wk | 1/8 | 1/8 | 1/8 | −1/8 | 1/8 | −1/8 | −1/8 | −1/8 |
wij | −1/8 | 1/8 | 1/8 | −1/8 | −1/8 | 1/8 | 1/8 | −1/8 |
wik | −1/8 | −1/8 | 1/8 | 1/8 | 1/8 | −1/8 | 1/8 | −1/8 |
wjk | −1/8 | 1/8 | −1/8 | 1/8 | 1/8 | −1/8 | 1/8 | −1/8 |
wijk | 1/16 | −1/16 | −1/16 | −1/16 | 1/16 | 1/16 | 1/6 | −1/16 |
Weights | C11 | C12 | C13 | C14 | C15 | C16 | C17 | C21 | P |
w1 | 1/8 | −1/8 | 1/8 | 1/8 | −1/8 | −1/8 | 1/8 | 1/8 | 1/4 |
w2 | 1/8 | 1/8 | −1/8 | 1/8 | −1/8 | 1/8 | −1/8 | 1/8 | 1/4 |
w3 | 1/8 | 1/8 | 1/8 | −1/8 | 1/8 | −1/8 | −1/8 | 0 | 1/8 |
w4 | 0 | 0 | 0 | 0 | 0 | 0 | 0 | 1/8 | 1/8 |
w12 | −1/8 | 1/8 | 1/8 | −1/8 | −1/8 | 1/8 | 1/8 | −1/8 | 0 |
w13 | −1/8 | −1/8 | 1/8 | 1/8 | 1/8 | −1/8 | 1/8 | 0 | 1/8 |
w14 | 0 | 0 | 0 | 0 | 0 | 0 | 0 | −1/8 | −1/8 |
w23 | −1/8 | 1/8 | −1/8 | 1/8 | 1/8 | −1/8 | 1/8 | 0 | 1/8 |
w24 | 0 | 0 | 0 | 0 | 0 | 0 | 0 | −1/8 | −1/8 |
w34 | 0 | 0 | 0 | 0 | 0 | 0 | 0 | 0 | 0 |
w123 | 1/16 | −1/16 | −1/16 | −1/16 | 1/16 | 1/16 | 1/16 | 0 | 1/16 |
w124 | 0 | 0 | 0 | 0 | 0 | 0 | 0 | 1/16 | 1/16 |
w234 | 0 | 0 | 0 | 0 | 0 | 0 | 0 | 0 | 0 |
Weights | P | C22 | C23 | Padd | C12 | C13 | Pdec | C17 | C21 | C18 | C31 | Pupd |
w1 | 1/4 | −1/8 | 1/8 | 1/4 | −1/8 | 1/8 | 1/4 | 1/8 | 1/8 | −1/8 | 0 | −1/8 |
w2 | 1/4 | 1/8 | −1/8 | 1/4 | 1/8 | −1/8 | 1/4 | −1/8 | 1/8 | −1/8 | 1/8 | 1/4 |
w3 | 1/8 | 0 | 0 | 1/8 | 1/8 | 1/8 | −1/8 | −1/8 | 0 | −1/8 | 1/8 | 1/4 |
w4 | 1/8 | 1/8 | 1/8 | 3/8 | 0 | 0 | 1/8 | 0 | 1/8 | 0 | 1/8 | 1/8 |
w12 | 0 | 1/8 | 1/8 | 1/4 | 1/8 | 1/8 | −1/4 | 1/8 | −1/8 | −1/8 | 0 | −1/8 |
w13 | 1/8 | 0 | 0 | 1/8 | −1/8 | 1/8 | 1/8 | 1/8 | 0 | −1/8 | 0 | −1/8 |
w14 | −1/8 | −1/8 | 1/8 | −1/8 | 0 | 0 | −1/8 | 0 | −1/8 | 0 | 0 | 0 |
w23 | 1/8 | 0 | 0 | 1/8 | 1/8 | −1/8 | 1/8 | 1/8 | 0 | −1/8 | −1/8 | −1/4 |
w24 | −1/8 | 1/8 | −1/8 | −1/8 | 0 | 0 | −1/8 | 0 | −1/8 | 0 | −1/8 | −1/8 |
w34 | 0 | 0 | 0 | 0 | 0 | 0 | 0 | 0 | 0 | 0 | −1/8 | −1/8 |
w123 | 1/16 | 0 | 0 | 1/16 | −1/16 | −1/16 | 3/16 | 1/16 | 0 | −1/16 | 0 | −1/16 |
w124 | 1/16 | −1/16 | −1/16 | −1/16 | 0 | 0 | 1/16 | 0 | 1/16 | 0 | 0 | 0 |
w234 | 0 | 0 | 0 | 0 | 0 | 0 | 0 | 0 | 0 | 0 | 1/16 | 1/16 |
No. | Instance | variables | Clauses | No. | Instance | variables | Clauses |
1 | aim-50-1_6-yes1-1 | 50 | 80 | 7 | aim-100-3_4-yes1-1 | 100 | 340 |
2 | aim-50-2_0-yes1-1 | 50 | 100 | 8 | aim-100-6_0-yes1-1 | 100 | 600 |
3 | aim-50-3_4-yes1-1 | 50 | 170 | 9 | aim-200-1_6-yes1-1 | 200 | 320 |
4 | aim-50-6_0-yes1-1 | 50 | 300 | 10 | aim-200-2_0-yes1-1 | 200 | 400 |
5 | aim-100-1_6-yes1-1 | 100 | 160 | 11 | aim-200-3_4-yes1-1 | 200 | 680 |
6 | aim-100-2_0-yes1-1 | 100 | 200 | 12 | aim-200-6_0-yes1-1 | 200 | 1200 |
parametric | parameter value | parametric | parameter value |
Initial assigned amount | 10000 | tolerance value δ | 0.001 |
CPU time threshold | 24 hours | - | - |
parametric | parameter value | parametric | parameter value |
Initial assigned amount | 10000 | probability of mutation pm | 0.05 |
population size | 50 | Maximum Iterations t | 100 |
crossover probability pc | 0.6 | - | - |
parametric | parameter value | parametric | parameter value |
Initial assigned amount | 10000 | revolutionary rate α | 0.3 |
population size | 50 | Maximum Iterations t | 100 |
parametric | parameter value | parametric | parameter value |
Initial assigned amount | 10000 | crossover probability pc | 0.6 |
population size | 50 | probability of mutation pm | 0.05 |
Initial number of clusters | 3 | Maximum Iterations t | 100 |
Indicators | calculation formula | instructions |
GMR | GMR=NGMT | GMR represents the ratio of the global minimum solution to the total number of runs [16]. GMR is an effective metric for assessing the efficiency of an algorithm. A model is considered robust when its GMR value is close to 1 [23]. Here, NGM represents the number of times the global minimum is converged, and T represents the total number of runs. |
MCT | MCT=1NGM∑TGMiNTi | The MCT refers to the average time needed for each model to reach the global minimum. A smaller MCT indicates that the model is more efficient in finding the global minimum. NTi represents the CPU time needed to find the global minimum at the i th retrieval result, and NGM represents the number of times the global minimum converged. |
MMHD | MMHD=1T∑TiminjD(Xi,Zj) | The MMHD value represents the mean minimum Hamming distance, which is the average of the smallest bit difference between the retrieval result of each run and the global minimum. When the MMHD value is closer to 0, it indicates that the model retrieves a result closer to the global minimum. In this context, D(Xi,Zj) represents the Hamming distance between the retrieval result of the i th run and the global minimum, and T represents the total number of runs. |
MLSR | MLSR=1T∑TiNsat(i)m | The MLSR value indicates the average proportion of the total number of clauses that can be satisfied by the retrieval results. The closer the MLSR value is to 1, the closer the model retrieval results are to the global minimum. Here, Nsat(i) denotes the number of satisfying clauses for the i th retrieval result, and m denotes the total number of clauses. |
No. |
GMR | MCT | MMHD | MLSR | ||||
DHNN-3SAT-WA | DHNN-3SAT-GA | DHNN-3SAT-WA | DHNN-3SAT-GA | DHNN-3SAT-WA | DHNN-3SAT-GA | DHNN-3SAT-WA | DHNN-3SAT-GA | |
1 | 1.0000 | 1.0000 | 4.83 | 2.51 | 0.0000 | 0.0000 | 1 | 1 |
2 | 0.9123 | 0.9323 | 16.67 | 11.70 | 1.1000 | 1.0900 | 0.9744 | 0.9745 |
3 | 0.8234 | 0.8456 | 26.67 | 22.83 | 3.6000 | 3.4900 | 0.9355 | 0.9578 |
4 | 0.5802 | 0.6204 | 48.82 | 45.80 | 3.7200 | 3.7100 | 0.8923 | 0.8966 |
5 | 1.0000 | 1.0000 | 11.72 | 6.31 | 0.0000 | 0.0000 | 1 | 1 |
6 | 0.8812 | 0.9011 | 46.51 | 16.01 | 2.5000 | 2.4000 | 0.9433 | 0.9533 |
7 | 0.5467 | 0.6041 | 113.28 | 35.50 | 3.6200 | 3.6100 | 0.9288 | 0.9363 |
8 | 0.2018 | 0.2188 | 440.39 | 171.23 | 4.7400 | 4.2300 | 0.9139 | 0.9231 |
9 | 0.4114 | 0.4222 | 537.92 | 431.04 | 4.8600 | 4.4500 | 0.9835 | 0.9844 |
10 | 0.2261 | 0.2352 | 978.78 | 773.75 | 5.9800 | 5.6700 | 0.9312 | 0.9474 |
11 | 0.1616 | 0.1653 | 1369.44 | 1100.94 | 7.1000 | 6.8900 | 0.8537 | 0.8775 |
12 | 0.1413 | 0.1518 | 1566.18 | 1198.85 | 8.2200 | 8.1100 | 0.8234 | 0.8641 |
No. |
GMR | MCT | MMHD | MLSR | ||||
DHNN-3SAT-ICA | DHNN-3SAT-GAKM | DHNN-3SAT-ICA | DHNN-3SAT-GAKM | DHNN-3SAT-ICA | DHNN-3SAT-GAKM | DHNN-3SAT-ICA | DHNN-3SAT-GAKM | |
1 | 1.0000 | 1.0000 | 2.49 | 2.21 | 0.0000 | 0.0000 | 1 | 1 |
2 | 0.9441 | 0.9658 | 10.64 | 8.29 | 1.0700 | 1.0400 | 0.9761 | 0.9783 |
3 | 0.8542 | 0.9126 | 20.45 | 15.08 | 3.2700 | 1.1400 | 0.9662 | 0.9751 |
4 | 0.6356 | 0.7229 | 40.18 | 26.87 | 3.3700 | 2.9700 | 0.8978 | 0.9354 |
5 | 1.0000 | 1.0000 | 5.49 | 4.68 | 0.0000 | 0.0000 | 1 | 1 |
6 | 0.9124 | 0.9256 | 14.02 | 11.06 | 2.2000 | 2.1000 | 0.9644 | 0.9881 |
7 | 0.6205 | 0.6898 | 30.59 | 22.03 | 3.2000 | 2.9300 | 0.9375 | 0.9523 |
8 | 0.2291 | 0.3211 | 141.54 | 74.60 | 3.9800 | 3.7600 | 0.9251 | 0.9336 |
9 | 0.4301 | 0.4503 | 435.12 | 396.82 | 4.0800 | 3.8900 | 0.9851 | 0.9928 |
10 | 0.2488 | 0.2632 | 752.20 | 678.91 | 5.0800 | 4.7200 | 0.9488 | 0.9557 |
11 | 0.1689 | 0.2203 | 1108.03 | 935.02 | 6.0800 | 5.5500 | 0.8809 | 0.9028 |
12 | 0.1612 | 0.2097 | 1160.96 | 982.28 | 7.0800 | 6.3800 | 0.8732 | 0.8822 |