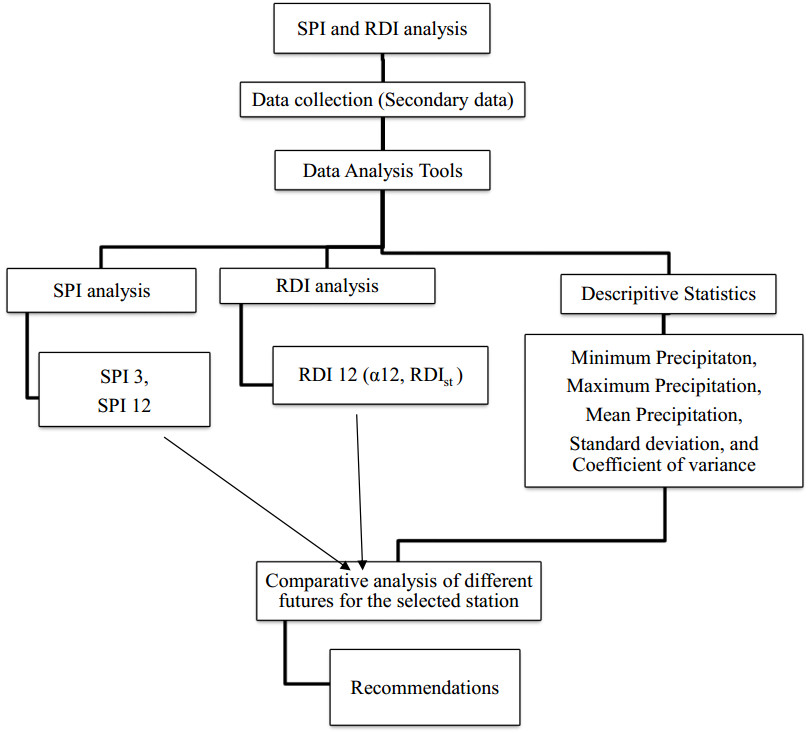
Drought is one of the most natural hazards that cause damage to ecosystems, agricultural production, and water resources. This study has analyzed seasonal and annual rainfall trends using monthly data series of 33 years (1983–2015) in Addis Ababa city over three stations namely; Sendafa, Bole, and Observation. Here, we examined the occurrence of historical drought trends in the study jurisdiction. The Reconnaissance Drought Index (RDI) and the Standardized Precipitation Index (SPI) were employed to find long-term drought trends as well as to examine the occurrence of drought history at a longer duration. The analysis indicated that severe drought conditions were observed for SPI and RDI indices in the year 2013 for Bole station, while medium droughts were recorded for the years 1991 and 2002 for all stations. Similarly, the RDI indices for 1996 was recorded as severe drought for the Observatory station. On the other hand, higher variability (coefficient of variation) of rainfall during winter seasons were 95.8%, 95.9%, and 77.9% for Sendafa, Bole, and Observatory stations respectively. However, the lower coefficient of variation during annual rainfall was 15.59% for Sendafa, 14.38% for Bole, and 13.98% for the Observatory station. Furthermore, the drought severity classification for the long-term drought analysis of annual precipitation shows that 3% of severe drought, 12% of moderate drought, and 85% of the normal condition were recorded in Bole station. The severe and moderate drought indices due to the reduction of rainfall, temperature change, and other factors can cause a shortage of urban water supply. Thus, the results of this study will help the water sector professionals in forecasting weather variations and for better management of urban water resources.
Citation: Zinabu A. Alemu, Emmanuel C. Dioha, Michael O. Dioha. Hydro-meteorological drought in Addis Ababa: A characterization study[J]. AIMS Environmental Science, 2021, 8(2): 148-168. doi: 10.3934/environsci.2021011
[1] | Kaoutar MOUNIR, Isabelle LA JEUNESSE, Haykel SELLAMI, Abdessalam ELKHANCHOUFI . Spatiotemporal analysis of drought occurrence in the Ouergha catchment, Morocco. AIMS Environmental Science, 2023, 10(3): 398-423. doi: 10.3934/environsci.2023023 |
[2] | Nur Hikmah Auliana, Nurtiti Sunusi, Erna Tri Herdiani . Analysis of meteorological drought periods based on the Standardized Precipitation Evapotranspiration Index (SPEI) using the Power Law Process approach. AIMS Environmental Science, 2024, 11(5): 682-702. doi: 10.3934/environsci.2024034 |
[3] | Abebe Kebede Habtegebreal, Abebaw Bizuneh Alemu, U. Jaya Prakash Raju . Examining the Role of Quasi-biennial Oscillation on Rainfall patterns over Upper Blue Nile Basin of Ethiopia. AIMS Environmental Science, 2021, 8(3): 190-203. doi: 10.3934/environsci.2021013 |
[4] | Khaled Hazaymeh, Quazi K. Hassan . Remote sensing of agricultural drought monitoring: A state of art review. AIMS Environmental Science, 2016, 3(4): 604-630. doi: 10.3934/environsci.2016.4.604 |
[5] | Joseph A. Kazery, Dayakar P. Nittala, H. Anwar Ahmad . Meteorological effects on trace element solubility in Mississippi coastal wetlands. AIMS Environmental Science, 2019, 6(1): 1-13. doi: 10.3934/environsci.2019.1.1 |
[6] | Moven Manjowe, Terence Darlington Mushore, Juliet Gwenzi, Collen Mutasa, Electdom Matandirotya, Emmanuel Mashonjowa . Circulation mechanisms responsible for wet or dry summers over Zimbabwe. AIMS Environmental Science, 2018, 5(3): 154-172. doi: 10.3934/environsci.2018.3.154 |
[7] | Bedassa D. Kitessa, Semu M. Ayalew, Geremew S. Gebrie, Solomon T. Teferi . Quantifying water-energy nexus for urban water systems: A case study of Addis Ababa city. AIMS Environmental Science, 2020, 7(6): 486-504. doi: 10.3934/environsci.2020031 |
[8] | Tuan Syaripah Najihah, Mohd Hafiz Ibrahim, Nurul Amalina Mohd Zain, Rosimah Nulit, Puteri Edaroyati Megat Wahab . Activity of the oil palm seedlings exposed to a different rate of potassium fertilizer under water stress condition. AIMS Environmental Science, 2020, 7(1): 46-68. doi: 10.3934/environsci.2020004 |
[9] | Tewodros Woldemariam Tesfaye, C.T. Dhanya, A.K. Gosain . Evaluation of ERA-Interim, MERRA, NCEP-DOE R2 and CFSR Reanalysis precipitation Data using Gauge Observation over Ethiopia for a period of 33 years. AIMS Environmental Science, 2017, 4(4): 596-620. doi: 10.3934/environsci.2017.4.596 |
[10] | Tamara S. Wilson, Benjamin M. Sleeter, Jason Sherba, Dick Cameron . Land-use impacts on water resources and protected areas: applications of state-and-transition simulation modeling of future scenarios. AIMS Environmental Science, 2015, 2(2): 282-301. doi: 10.3934/environsci.2015.2.282 |
Drought is one of the most natural hazards that cause damage to ecosystems, agricultural production, and water resources. This study has analyzed seasonal and annual rainfall trends using monthly data series of 33 years (1983–2015) in Addis Ababa city over three stations namely; Sendafa, Bole, and Observation. Here, we examined the occurrence of historical drought trends in the study jurisdiction. The Reconnaissance Drought Index (RDI) and the Standardized Precipitation Index (SPI) were employed to find long-term drought trends as well as to examine the occurrence of drought history at a longer duration. The analysis indicated that severe drought conditions were observed for SPI and RDI indices in the year 2013 for Bole station, while medium droughts were recorded for the years 1991 and 2002 for all stations. Similarly, the RDI indices for 1996 was recorded as severe drought for the Observatory station. On the other hand, higher variability (coefficient of variation) of rainfall during winter seasons were 95.8%, 95.9%, and 77.9% for Sendafa, Bole, and Observatory stations respectively. However, the lower coefficient of variation during annual rainfall was 15.59% for Sendafa, 14.38% for Bole, and 13.98% for the Observatory station. Furthermore, the drought severity classification for the long-term drought analysis of annual precipitation shows that 3% of severe drought, 12% of moderate drought, and 85% of the normal condition were recorded in Bole station. The severe and moderate drought indices due to the reduction of rainfall, temperature change, and other factors can cause a shortage of urban water supply. Thus, the results of this study will help the water sector professionals in forecasting weather variations and for better management of urban water resources.
Drought is a common natural disaster linked with scarcity of water due to reduction in the amount of rainfall over an extended period of time. Drought occurrences are expected to become more severe and frequent in future years [1]. Rainfall is the primary driver of any drought. Drought leads to a reduction of surface water (reservoir storage level). Surface and subsurface water resources are commonly affected by an extended period of drought and this results from rainfall variability [2,3]. Meteorological droughts are impermanent, recurring natural disasters, which occurs from the shortage of rainfall and can bring significant economic losses. There are numerous indicators and hypothesis that are being employed for drought monitoring [4,5]. Based on drought indices, various categories can be identified–permanent, seasonal, contingent, and invisible drought. Drought maybe meteorological, agricultural or hydrological especially in its manifestation and impact [6].
As a country, Ethiopia is under rapid development both in rural and urban settings; there are enormous constructions in all sectors, rapid urbanization, industrialization, floriculture and big farming are being practiced. These anthropogenic activities along with climate change are affecting the water resources of the country and it also exacerbate the urban water supply shortage. The economic impact of severe drought is that people spend more money to access water for household and industrial purpose, since reservoir level and even ground water levels have decreased. Drought also affects plants, animals, and human health. Hence, many drought indices are proposed to examine the historical drought trends based on rainfall and temperature data which supports water security in the country. Feyissa et al. [7] studied downscaling of future temperature and precipitation extremes in Addis Ababa under climate change. The study result shows that the maximum temperature increases were in the range of 0.9 ℃ (RCP4.5) in 2020 to 2.1 ℃ (CGCM3A2) in 2080 at Addis Ababa Observatory. In Ethiopia, rainfall is very variable both in amount and distribution through regions and seasons [8]. The frequency and ardency of extremes are likely impacted by climate changes and therefore the changes in climate extremes can affect other forms of biological and physical systems and their impacts can even lead to global warming [9]. In Addis Ababa–the capital of Ethiopia, the temperature is usually affected by human activities together with global climate change [10]. The rise in temperature will agammaavate the urban heat highland effects in warm seasons and an increase in rainfall is expected along with a possible risk of flooding [7]. The rising trend of temperature owing to the adverse effects of climate change and other factors can cause climate extremes within the city of Addis Ababa [11]. Hence, the drought in Addis Ababa can be attributed to the fluctuations in the climate pattern of the city.
The prediction of drought can play a vital role in the mitigation of its effects. In practice, drought indices are usually employed in the assessment of drought events. In recent times, drought indices have supported meteorological and hydrological stations easily to predict drought events using the relevant climatic data on the basis of practical applications. Drought indices has become the foremost option for drought monitoring and characterization [12]. In the literature, many studies have used the two common drought indices–the Standardized Precipitation Index (SPI) and the Reconnaissance Drought Index (RDI) for monitoring and forecasting of droughts in numerous areas. The RDI was used to determine the temporal and spatial analysis of meteorological drought in the Awash River Basin (Ethiopia) [13]. Likewise, many research studies has also proposed the RDI as one climatic index for the detection of potential climatic changes [14]. However, drought indices are typically continuous functions of temperature and/or rainfall, river discharge, or additional assessable hydro-meteorological variables. Consequently, the SPI is also used as one of the large numbers of drought indices [15]. SPIs were utilized in drought analysis based on monthly rainfall data to examine the drought characteristics of Meric-Ergene, Gediz, Seyhan, and Ceyhan hydrological basins in Turkey [16]. Pashiardis and Michaelides [17] examined regional drought assessment by calculating the SPI and RDI indices which are based on past monthly rainfall and temperature data. Both indices are similar and can be used efficiently to analyze and monitor drought situations and events. Similarly, in Iran, another study applied the SPI and RDI indices for different time scales in forty meteorological stations. The study found that drought severity was obtainable in the drought year from 1999 to 2000 for the two indices. It also showed that the two indices were more significant in the 3-months, 6-months, and 9-months than longer time scales [18].
There are other numerous examples of SPI and RDI applications such as Ansarifard and Shamsnia [19] who used the SPI and RDI for the analysis of rainfall for drought monitoring. The SPI result showed near-normal condition, while the RDI categorized as moderately dry condition for the year 1999–2000. The SPI has several features that give it an edge over earlier indices, including its simplicity and temporal flexibility [20]. Similarly, the SPI was used for the examination of drought intensity assessment in northeastern Nigeria and the decadal SPI result revealed a mild drought [6]. Zarei et al. [21] employed the modified RDI for the assessment of drought severity in the south of Iran. The study result showed that the trends for annual drought were significantly increasing at 95% confidence level for the areas with normal, dry and extremely dry conditions. Likewise, the SPI was used to examine the rainfall deficit for multiple timescales in Tegal City, Central Java (Indonesia). From all scarcity periods, the result of the study revealed that the foremost severe drought within the study temporal horizon fell in 2015 [22].
We recognize that there are other studies on this subject matter, and thus, we have just presented a snapshot of a few. The foregoing indicates that drought assessment of a given jurisdiction involves the computation of the two (2) well-known drought indices namely; the RDI and SPI. The RDI is computed based on the historic monthly precipitation and temperature data. Whereas, the SPI is calculated only with rainfall data. Both indices can be employed effectively to analyze the occurrence of drought history and used to predict drought trends. However, RDI has more advantage to SPI since it includes temperature data. Accordingly, this study is focused on the precipitation and/or temperature variation in the study area over three stations–Sendafa, Bole, and Observatory in Addis Ababa, Ethiopia. The historic precipitation and temperature data used for the three stations are from 1983 to 2015.
The main objective of this paper is to examine the occurrence of historical drought trend in Addis Ababa city. It seeks to provide scientific information for the water sector professionals and support for better management of urban water resources. With respect to scholarly contributions, this paper adds to the literature in this domain by introducing one of the earliest case studies to examine historical drought trends in Ethiopia. In a useful manner, the study applies the two well-known drought indices for a jurisdiction that have scanty information on its drought trend. Moreover, beyond Ethiopia, the analytical frameworks presented here can be used by other researchers to examine historical drought trends in other locations of the world. Therefore, while this paper is purposed within a national perspective, we believe that it will be relevant for international audience as it also supports the realization of the UN Sustainable Development Goals (SDG) of clean water & sanitation (SDG 6), and climate action (SDG 13).
Section 2 of the paper outlines the study methodology employed in the paper/investigation which contains the study area description, quality control of the data, SPI and RDI etc. Section 3 presents the study results and in-depth discussion. Next is section 4 which states the study conclusions.
We used the Drought Indices Calculator (DrinC) for our study. DrinC is a software package which was established for providing easy, though flexible interface for the computation of drought indices [29]. The Reconnaissance Drought Indices (RDI) and Standard Precipitation Index (SPI) were employed to examine the occurrence of drought history at a longer time scale. Figure 1 shows the simplified study methodological framework.
Addis Ababa is geographically located at 38° 44' East and 9° 1' North longitude and latitude respectively, and has a mild climate. It have three layers of Government hierarchy namely; City Government, Sub City Administrations, and Kebele Administrations at the top, middle, and bottom levels respectively. The average elevation of the city is around 2,500 meters above sea level. The period from March to May, is the warmest of the year. Ethiopia has approximately 1,200 mm of rain fall throughout the year, with the maximum being from June through September. Figure 2 shows the study area.
The data quality was checked visually and statistically. Visually, data cleaning was performed to check the data entry error. Whereas, statistically the quality control were carried out by using boxplot and SPSS software package. The tool is capable of identifying outliers and missed data. An outlier is defined as the value above the upper fence or below the lower fence. The upper and lower fence represent values more than 75th percentile (3rd quartiles) and 25th percentile (1st quartiles) respectively. The rainfall and temperature data were verified for outlier and missing data to avoid mistakes that can cause changes in the final result. Finally, the DrinC was employed for the analysis of drought indices and used to investigate the RDI and SPI indices [29].
The SPI is a widely used meteorological drought index and it was first introduced by [15]. It is basically the transformation of the precipitation (rainfall) time series into a standardized normal distribution. The SPI is calculated as per Eq 1.
SPI=Xi−¯Xσ | (1) |
Where xi is monthly rainfall, ¯X is mean, and σ is the standard deviation of the data.
SPI can be computed for different time scales. SPI is computed for short time (up to 3 month, medium term (3–12 months), and long term accumulation periods (12–24 months). The present study computed the SPI values for medium-term cumulated (3 to 12 months, that is SPI 3, and SPI 12) since it is more suitable for measuring the impact on stream flow and reservoir levels [15,28]. But, the SPI values were also computed for short-term time scales (1-month SPI) to highlight the agricultural drought. Table 1 shows the SPI value used for drought classification.
Drought Category (Classification) | SPI Value |
Extremely Wet | ≥ 2.0 |
Very Wet | 1.5–1.99 |
Moderately Wet | 1.0–1.49 |
Near normal | (-0.99)–(0.99) |
Moderately dry (Moderate Drought) | (-1.0)–(-1.49) |
Severely dry (Sever drought) | (-1.5)–(-1.99) |
Extremely dry (Extreme drought) | ≤ -2.0 |
The Reconnaissance Drought Index (RDI) is one among the meteorological drought indices used to assess drought severity. The RDI is expressed in three forms which are commonly called the initial value of Reconnaissance Drought Index (αk), normalized Reconnaissance Drought Index (RDIn), and standardized Reconnaissance Drought Index (RDIst) [34,29]. The standardized expression of RDI is not significantly affected by the method of potential evapotranspiration (PET) calculation, whereas the initial and the normalized RDI are directly influenced by other methods. However, if precipitation and air temperature data are the only available data, then the simplified version of FAO Penman-Monteith (or Hargreaves) method, could be used [35]. In cases where adequate long time data of several meteorological variables are obtainable, the researchers should use the widely accepted FAO Penman-Monteith method [36].
In this study, the potential evapotranspiration (PET) is computed based on rainfall and temperature data since solar radiation, saturation vapor pressure, relative humidity, soil heat flux density, wind speed, vapor pressure deficit data are not available. Thus, the potential evapotranspiration is calculated by the following Eq 2 [37].
PET=0.0023(Tmax+Tmin2+17.8)√Tmax+Tmin∗Ra | (2) |
Where T max is the maximum temperature, T min is the minimum temperature, Ra is the extra-terrestrial solar radiation, which depends on the year as well as latitude of the observation site.
The initial value of the Reconnaissance Drought Index (αk) is presented in an agammaegated form employing a monthly time step and will be calculated for every month of the hydrological year (or an entire year). The αk will be computed by Eq 3.
α(i)k=∑kj=1Pij∑kj=1PETij,i=1, N and j=k | (3) |
Where: Pij and PETij are the precipitation (rainfall) and potential evapotranspiration (PET) of the jth month of the ith year and i usually starts from September as per Ethiopian hydrological time. Hence, September k = 1 and N is the overall entire number of years for the available data.
The Normalized values of the Reconnaissance Drought Index (RDIn) is calculated using Eq 4.
RDI(i)n=α(i)k¯α(l)k−1 | (4) |
Where, ¯α(l)kis the arithmetic mean of α(i)k values and calculated for the N years of given data. The values of αk follows satisfactorily both the lognormal as well as the gamma distributions in which they were tested [32].
The initial formulation of RDIst used the thought that αk values follow the log-normal (LN) distribution. So, the following equations are often used for the computation of RDIst:
RDIst(i)=y(i)k−¯ykstdαyk | (5) |
In which, yk is ln(αk(i)), ¯yk is the arithmetic mean of yk and std αyk is its standard deviation (SD).
However, the αk values follow both the ln and the gamma distribution values at the majority of several locations and time scales. But in most of the cases, the gamma distribution was proved to be more successful. Therefore, the calculation of RDIst might be performed better by fitting the gamma probability density function (pdf) to the given frequency distribution of ak. The positive values of RDIst indicate the wet periods, whereas the negative values indicate dry periods compared to the conditions of the studied area [38,34]. The RDI value used for drought classification is stated in Table 2.
Drought Category (Classification) | RDI Value |
Extremely Wet | ≥ 2.0 |
Very Wet | 1.5–1.99 |
Moderately Wet | 1.0–1.49 |
Near normal | (-0.99)– (0.99) |
Moderately dry (Moderate Drought) | (-1.0) – (-1.49) |
Severely dry (Sever drought) | (-1.5) – (-1.99) |
Extremely dry (Extreme drought) | ≤ -2.0 |
The given input parameters are derived via descriptive statistical information. The procedure for the descriptive statistical summary analysis can be displayed in the form of a table. The statistical summary of parameters are the sample size (the number of observations), minimum, maximum, mean, standard deviation (SD), variance, and coefficient of variance (CV). The Minimum value shows the lowest category while the Maximum value shows the highest category of the given variable. Hence, the mean is calculated as stated below (Eq. 6).
Mean(ˉX)=∑ni=1Xin | (6) |
Here, Xi is the data value, and n is the sample size.
The sample variance indicates the classical measure of spread. Similar to the mean, it is strongly affected by outliers. In a population, the standard deviation and variance can both be used to measure the variability of the given population. Variance simply refers to the average squared deviations from the arithmetic mean. Whereas, the SD refers to the square root of the variance. Consequently, the variance is simply the square of the SD (Eq. 7). Similarly, assessment of rainfall variability were determine by the CV as stated below (Eq. 9).
Variance (σ2)=∑(X−¯X)2(n−1) | (7) |
Standard Deviation (σ)=√σ2 | (8) |
Coefficient of Variance (CV%)=SD Mean ∗100 | (9) |
The foregoing procedures were adapted from [38,39].
The daily climatic data for temperature and rainfall (precipitation) were taken from the National Meteorological Agency (NMA). The past (historic) precipitation and temperature data were collected from 1983 to 2015 (NY = 33) for all stations (i.e. Sendafa, Bole, and Observatory station). The whole research data for this study were collected based on the secondary data sources to address the objectives of the study. The data was applied to characterize the hydro-meteorological drought and its trend in the city of Addis Ababa.
The SPI and RDI indices were employed to examine the occurrence of drought history at a longer time scale. Both indices were computed using the Drought Indices Calculator (DrinC) software. Meanwhile, the descriptive statistical techniques such as mean, standard deviation, minimum (min), maximum (max), and coefficient of variance (CV), and also annual and seasonal graph were computed with the aid of Microsoft excel and SPSS software package. The analyzed data were used to detect the historic rainfall and drought indices classification.
The RDI and SPI were used to find the long-term drought trends as well as to examine the occurrence of drought history at a longer time scale. Figure 3 shows the graph for mean precipitation of Sendafa, Bole, and Observatory stations from 1983 to 2015, whereas, Table 3 shows annual and seasonal SPI values for Bole, Sendafa, and Observatory stations from 1983 to 2015. Figure 4a, b, and c show annual and seasonal precipitation series from 1983 to 2015 for Bole, Sendafa, and Observatory stations respectively. Additionally, Figure 8 shows the Standardized 12-month RDI value (RDIst_12) for Bole and Observatory stations.
Year | SPI value for Bole Station | SPI value for Sendafa Station | SPI value for Observatory Station | ||||||||||||
SPI 3 | SPI 12 | SPI 3 | SPI 12 | SPI 3 | SPI 12 | ||||||||||
Autumn | Winter | Spring | Summer | Annual | Autumn | Winter | Spring | Summer | Annual | Autumn | Winter | Spring | Summer | Annual | |
1983 | 1.27 | -1.01 | -0.74 | 2.04 | 1.32 | 0.01 | -1.34 | -0.49 | -0.38 | -1.12 | -0.41 | -1.02 | -0.86 | 1.12 | -0.33 |
1984 | -1.33 | 0.14 | 0.65 | -0.11 | -0.24 | -0.26 | -0.85 | 1.67 | 0.80 | 1.43 | -1.09 | -0.31 | -0.20 | 0.05 | -0.78 |
1985 | 0.67 | 0.04 | 1.00 | -0.27 | 0.55 | -0.15 | -0.19 | 1.58 | -0.47 | 0.22 | 0.69 | -0.14 | 1.67 | -0.89 | 0.80 |
1986 | -0.41 | 0.35 | 1.93 | -3.09 | -0.68 | 0.20 | -0.02 | 1.41 | -2.21 | -1.30 | -0.79 | 0.45 | 2.69 | -1.94 | 0.81 |
1987 | -2.05 | 0.05 | -0.19 | -0.52 | -1.34 | -0.19 | -0.03 | -0.09 | 0.63 | 0.33 | -1.27 | 0.44 | -0.58 | -0.25 | -1.01 |
1988 | 1.37 | 0.02 | -0.16 | 0.30 | 0.53 | 1.23 | -0.19 | 0.14 | -0.11 | 0.34 | 0.96 | 0.68 | 0.15 | 1.03 | 1.13 |
1989 | 0.48 | 2.09 | 0.18 | -0.56 | 0.64 | 0.29 | 2.96 | 0.60 | 0.40 | 1.57 | -0.18 | 1.84 | -0.34 | -1.52 | -0.50 |
1990 | 0.55 | -0.17 | -0.76 | 0.75 | 0.15 | 2.88 | 0.08 | -0.12 | -3.34 | -0.98 | -0.12 | 0.64 | -0.21 | 0.09 | -0.10 |
1991 | -0.60 | 0.15 | -0.88 | -0.32 | -1.10 | 0.73 | 0.76 | -1.76 | -0.46 | -0.97 | -1.42 | 1.10 | -1.35 | -0.60 | -1.37 |
1992 | 1.06 | 0.60 | 0.62 | 0.60 | 1.23 | 0.90 | 1.63 | -1.20 | 0.89 | 1.10 | 0.83 | 0.75 | 0.55 | 2.05 | 1.88 |
1993 | 0.92 | -1.03 | -0.83 | -0.38 | -0.80 | 0.49 | -1.34 | -1.20 | -0.63 | -1.46 | 1.14 | -1.22 | 0.13 | -0.54 | -0.08 |
1994 | -1.03 | 0.90 | 0.92 | -0.94 | -0.26 | 0.01 | -0.88 | 0.98 | -0.69 | -0.49 | -0.90 | 0.54 | 0.67 | -1.13 | -0.57 |
1995 | -1.38 | 0.63 | 1.40 | 2.89 | 2.70 | -0.08 | 1.01 | 0.92 | 0.52 | 1.03 | -1.29 | 0.76 | 1.27 | 1.62 | 1.33 |
1996 | 0.88 | -0.19 | -1.12 | -0.46 | -0.80 | -0.08 | 0.31 | -0.35 | -0.33 | -0.75 | 1.01 | -0.08 | -1.33 | -1.59 | -1.23 |
1997 | 0.16 | 1.29 | 1.28 | 0.25 | 1.35 | 0.74 | 0.51 | -1.26 | -0.25 | -0.58 | 0.16 | 0.68 | 0.36 | -0.40 | 0.10 |
1998 | 2.26 | -0.95 | -2.09 | 0.70 | 0.27 | 1.30 | -1.32 | -0.91 | 0.94 | 0.91 | 1.52 | -1.27 | -2.23 | 0.15 | -0.48 |
1999 | 0.53 | -1.03 | -0.17 | -0.76 | -0.90 | 1.13 | -1.34 | 0.04 | 0.65 | 0.88 | -0.79 | -1.34 | -0.44 | -0.11 | -1.11 |
2000 | 0.44 | -0.77 | 0.96 | 0.64 | 0.89 | 0.02 | 0.04 | 1.25 | -0.01 | 0.52 | 1.28 | -0.92 | 1.62 | 1.88 | 2.31 |
2001 | -0.73 | 0.45 | -0.63 | -0.29 | -0.89 | -1.76 | -0.32 | -0.17 | -0.47 | -1.49 | -1.12 | -0.17 | -0.07 | -0.68 | -1.11 |
2002 | -2.07 | 0.74 | -0.46 | -0.38 | -1.14 | -0.64 | 1.52 | -0.11 | 0.18 | 0.06 | -1.86 | 0.74 | -0.38 | -0.32 | -1.03 |
2003 | -0.49 | 0.73 | -0.45 | -0.07 | -0.43 | -0.07 | 0.24 | -0.52 | -0.33 | -0.84 | -0.28 | 1.04 | 0.04 | -0.59 | -0.25 |
2004 | 0.23 | 0.70 | 1.11 | -0.11 | 0.78 | -1.99 | 0.37 | 1.25 | -0.23 | -0.19 | 0.35 | 1.00 | 1.43 | 0.38 | 1.44 |
2005 | -1.04 | 0.06 | 0.28 | 1.46 | 0.79 | -0.28 | -0.41 | -0.53 | 1.07 | 0.56 | 0.39 | -0.93 | 0.61 | 0.48 | 0.45 |
2006 | 0.16 | -0.13 | 0.73 | 0.57 | 0.71 | -0.87 | 0.12 | -0.46 | 0.16 | -0.59 | 1.00 | 0.70 | 0.38 | 1.12 | 1.33 |
2007 | 0.15 | -1.03 | -1.55 | 0.32 | -0.92 | -0.19 | 0.12 | -1.88 | -0.44 | -1.86 | -0.65 | -0.89 | -0.88 | 0.23 | -0.98 |
2008 | 1.69 | 0.11 | -1.53 | -0.07 | -0.19 | 1.41 | 0.12 | -0.46 | 0.16 | 0.51 | 2.33 | 0.10 | -0.56 | 1.20 | 1.45 |
2009 | -0.59 | 2.33 | 1.18 | -0.26 | 1.26 | -0.19 | 0.47 | 1.26 | -0.25 | 0.30 | -0.81 | 1.65 | 0.12 | 0.90 | 0.72 |
2010 | -0.84 | -0.09 | -1.07 | 0.32 | -0.84 | -0.99 | 0.47 | 0.64 | -0.75 | -0.93 | 0.66 | -0.01 | -1.04 | -0.02 | -0.40 |
2011 | -0.13 | -1.03 | -0.60 | -0.09 | -0.92 | -0.99 | 0.47 | 0.64 | -0.75 | -0.93 | -0.39 | -1.34 | -0.97 | -0.15 | -1.24 |
2012 | 0.33 | -0.78 | 0.47 | -0.63 | -0.41 | -0.99 | 0.42 | -0.97 | 1.59 | 0.95 | 0.04 | -0.84 | 0.09 | 0.31 | -0.11 |
2013 | 0.28 | 0.13 | -1.26 | -1.21 | -1.61 | -2.11 | -0.32 | -0.59 | 1.26 | 0.34 | 0.81 | 0.15 | -0.39 | -1.87 | -0.97 |
2014 | -0.60 | -1.03 | 0.74 | -1.01 | -0.91 | 0.23 | -0.56 | -0.23 | 1.91 | 1.84 | 1.09 | -1.34 | -0.94 | 0.38 | -0.16 |
2015 | -0.13 | 0.74 | 1.07 | 0.71 | 1.20 | 0.23 | -1.01 | 1.32 | 0.99 | 1.56 | -0.92 | 0.60 | 0.95 | -0.40 | 0.05 |
From climatological or hydrological perspective, rainfall during the summer season is very common and it is important for deciding the total amount of rainfall received annually. In Ethiopia, the rainy season is usually during the summer ("Kiremt" season) and as Figure 3 shows, the average summer precipitation was very high for Sendafa, Bole, and Observatory stations in comparison with other time scales (i.e Autumn, Winter, and the Spring season). The result found in this study is in line with the results of a previous study whose outcomes show that in the study area, the seasonal cycle shows more rain in May and June [42].
The SPI values for 12 months for Bole Station in Addis Ababa was high in 1995 and the result found in this SPI value plot agrees with the outcomes of standardized RDI indices (RDI st) for the year of 1995 for Bole station in Addis Ababa (Figures 5a and Figure 8).
SPI can be computed for different time scales. The current study computed SPI related to medium-term cumulated values (3 to 12 months, that is SPI 3 and SPI 12) and the SPI indices is also recommended by other authors as it is more appropriate for measuring the impact on stream flow and reservoir storage [5,28]. Figure 5a, b, and c show the plot of SPI values for 12 months for Bole, Sendafa, and Observatory Station in Addis Ababa city. Likewise, the SPI values for 3 months range (seasonal) and 12-month (annual) SPI values for Bole, Sendafa, and Observatory Station in Addis Ababa city is computed as shown in Table 3.
Furthermore, Figure 6 shows the plot of the initial value of RDI_12 (α12) for a time series of 33 years for Bole and Observatory station. The dashed line represents the average of α12 for each station. The research finding suggests the Reconnaissance Drought Index (RDI) as a climatic index for detecting possible drought classification for a given data. Moreover, the RDI is more advantageous than SPI since the RDI incorporates both temperature and precipitation data in a single index whereas the SPI includes only Precipitation (rainfall) data (Figures 5–7, Tables 1 and 2). Similar to this study, for drought monitoring in given stations, Jamshidi et al. [43] showed that the RDI is more sensitive than the SPI to climatic conditions and therefore the authors recommended that the role of evapotranspiration is very important in drought assessment and should not be ignored. However, many scholars from different countries such as Algeria, India, USA, Nepal, Tunisia, Kuwait, etc., also recommend the importance and suitability of SPI and RDI indices for drought monitoring, assessing and comparing for meteorological and hydrological droughts [44,45,46,47,48,49].
Figure 7 revealed that both SPI and RDI show an identical plot/ graph. Both indices did not show large difference and thus in the present study the primary driver of the severe drought is both rainfall and temperature even if RDI is more sensitive to drought severity. The meteorological variable of both rainfall and temperature are interrelated (Figure. 7).
The RDI value is used for drought severity classification (Table 2). Therefore, Figure 9 shows the pie chart of drought conditions for Addis Ababa (Ethiopia) expressed by annual RDIst for 33 hydrological years and each drought class represented by a different color. The drought severity classification for the normal condition for Bole and Observatory station were 85% and 83% respectively. Whereas, the moderate drought classifications were 12% for Bole and 15% for Observatory station. Similarly, the severe drought classification for both stations (i.e. Bole and Observation) were 3% (Figure 9). The foregoing result concurs with the findings of an earlier study on this subject matter, which showed that the temperature trend was significantly increased due to climate change in Bole station (Addis Ababa) [10]. Accordingly, the severe drought and moderate drought indices due to reduction of rainfall, climate change and other variables can lead to a further indication of a shortage of urban water supply.
The calculated SPI and RDI values were classified based on the drought classification range (Tables 1 and 2). However, Table 4 shows the drought classes classified by SPI and RDI values which are analyzed from the monthly rainfall and temperature data and therefore can be used for drought monitoring. The results indicate that severe drought conditions were observed for SPI and RDI in the year 2013 for Bole station, but for the same year, the drought condition for Observatory station was normal for SPI and RDI indices. Similarly, the SPI and RDI indices of drought classification for all stations were medium droughts for the years 1991 and 2002. On the other hand, for the year 1996, the SPI indices displayed moderate drought conditions for the Observatory station. Whereas for RDI indices for the year (1996), the drought classification indicated severe drought for the Observatory station (Table 4 and Figure 8). Likewise, the SPI and RDI indices of drought classification for all stations were at normal conditions for the year 2015. The results found in this study agrees with the discoveries of a previous study [42] on this subject matter, which showed that for the year 2015 in Addis Ababa city (no significant trend), the seasonal cycle shows more rain in May and June than in the same months in Combolcha (risk ratio of 2) and this occurs from the difference in location.
SPI based drought Classification | RDI based drought Classification | |||
Year | Bole station | Observatory station | Bole station | Observatory station |
1983–1986 | Normal | Normal | Normal | Normal |
1987 | Moderate Drought | Moderate Drought | Moderate Drought | Normal |
1988–1990 | Normal | Normal | Normal | Normal |
1991 | Moderate Drought | Moderate Drought | Moderate Drought | Moderate Drought |
1992–1995 | Normal | Normal | Normal | Normal |
1996 | Normal | Moderate Drought | Normal | Severe Drought |
1997–1998 | Normal | Normal | Normal | Normal |
1999 | Normal | Moderate Drought | Normal | Moderate Drought |
2000 | Normal | Normal | Normal | Normal |
2001 | Normal | Moderate Drought | Moderate Drought | Moderate Drought |
2002 | Moderate Drought | Moderate Drought | Moderate Drought | Moderate Drought |
2003–2010 | Normal | Normal | Normal | Normal |
2011 | Normal | Moderate Drought | Normal | Moderate Drought |
2012 | Normal | Normal | Normal | Normal |
2013 | Severe Drought | Normal | Severe Drought | Normal |
2014–2015 | Normal | Normal | Normal | Normal |
The SPI and RDI of drought classification for both stations (i.e. Bole and Observation) in Addis Ababa were normal drought conditions for the year 2004. In contrast to this result, NMSA [50] reported that in Addis Ababa, Robe, Adigrat etc., for the year 2004, the extreme minimum temperature below 5 ℃ were displayed and therefore this minimum temperature caused crop damage over same areas of Ethiopia (i.e. central, eastern, and northeastern Ethiopia). Also, as shown in Table 4, the SPI and RDI indices for the year 1984 and 2015 indicated that normal drought conditions were observed for Bole and Observatory stations (Addis Ababa). In contrast, our result disagrees with the findings of an earlier study in this domain which opined that the year 1984 was extreme drought whereas in 2015 the extremity and severity of drought is very high in the upper part of Awash River basin, Ethiopia [13]. The differences in the findings of these studies can be partly attributed to the climatic and geographical variations.
Table 5 shows the statistical summary of annual and seasonal rainfall at the three meteorological stations. The average annual minimum precipitation for Sendafa, Bole, and Observatory stations are computed as 836.5 mm, 817.5 mm, and 999.2 mm respectively. Similarly, the average annual maximum precipitation for Sendafa, Bole, and Observatory station was 1481.4 mm, 1466.8 mm, and 1624.7 mm respectively. In Ethiopia, hydrological time starts from September and ends in August. Hence, the rainy season usually occurs in the summer ("Kiremt" season). Table 5 shows that the average summer precipitation was 778.58 mm, 399.12 mm, and 709.52 mm for Sendafa, Bole, and Observatory station respectively. Whereas, the time scale of spring ("Bega" season) are low raining season and hence the average spring precipitation for Sendafa, Bole, and Observatory station were 41.81 mm, 46.86 mm, and 53.95 mm respectively (Figure 4 and Table 5). These are in line with the findings of the previous study of Temam et al. [51], whose results show that the "Bega" season (from October to January) has the lowest rainfall amounts compared to other seasons in the given year (~2% of the annual precipitation of the country). Similar assumptions is obtainable in the work by Cermak et al. [52].
Descriptive Statistics | ||||||
Station | Time Scale | Min | Max | Mean | SD | % CV |
Sendafa | Autumn | 27.50 | 479.90 | 142.18 | 86.06 | 60.53 |
Winter | 0.00 | 212.50 | 41.81 | 40.06 | 95.82 | |
Spring | 0.00 | 355.40 | 176.59 | 94.21 | 53.35 | |
Summer | 298.90 | 1173.90 | 778.58 | 177.44 | 22.79 | |
Annual | 836.50 | 1481.40 | 1139.16 | 177.60 | 15.59 | |
Bole | Autumn | 73.10 | 312.80 | 165.82 | 55.03 | 33.18 |
Winter | 0.00 | 195.50 | 46.86 | 44.95 | 95.93 | |
Spring | 83.30 | 420.40 | 225.73 | 85.80 | 38.01 | |
Summer | 325.90 | 949.20 | 599.12 | 108.25 | 18.07 | |
Annual | 817.50 | 1466.80 | 1037.52 | 149.15 | 14.38 | |
Observation | Autumn | 109.00 | 424.30 | 222.60 | 74.09 | 33.28 |
Winter | 0.00 | 164.30 | 53.95 | 42.03 | 77.91 | |
Spring | 68.90 | 572.60 | 229.34 | 104.23 | 45.45 | |
Summer | 543.80 | 908.80 | 709.52 | 92.85 | 13.09 | |
Annual | 999.20 | 1624.70 | 1215.41 | 169.94 | 13.98 |
Table 3 and 5 show SPI values and seasonal rainfall at the three Meteorological Stations calculated for the 3-month period (i.e. Sep. to Nov. for autumn, Dec. to Feb. for winter, Mar. to May for spring, and Jun. to Aug. for summer). Similarly, Table 5 revealed that Observatory station, Sendafa Station, and Bole station have highest annual mean rainfall and highest standard deviations. In comparison, similar results were obtained by Yahaya et al. [6], who found that the highest monthly mean rainfall and highest standard deviation were recorded in Bauchi station, Ibi station, and Gombe station (Nigeria)–indicating high variability of annual rainfall for the study year.
Larger coefficient of variation (higher variability) of rainfall during winter seasons (scarcity of rainfall observed) were 95.82%, 95.93%, and 77.91% for Sendafa, Bole, and Observatory station respectively. Whereas, the lower coefficient of variation during annual rainfall (this is most commonly affected with the summer season) was 15.59% for Sendafa station, 14.38% for Bole station, and 13.98% for Observatory station. As a result, this statistical summary gives a good snapshot of precipitation variability for the region and this has important implications for urban water management while showing an intensification of the adverse impact of climate change. Table 5 also indicates a larger coefficient of variation (higher variability) of rainfall during winter seasons over all stations.
The study indicated that severe drought conditions were observed for SPI and RDI indices in the year 2013 for Bole station, but for the same year, the drought condition for Observatory station was normal for SPI and RDI indices and this happens due to the temperature and latitude variation of the station. In a similar bid, the SPI and RDI of drought classification for all stations show medium droughts for the years 1991 and 2002. On the other hand, for the year 1996, the SPI indices showed moderate drought conditions for the Observatory station. Whereas for RDI indices for 1996, the drought classification indicated severe drought for the Observatory station. The drought classification difference in the year 1996 occurred due to the indices method difference. It can therefore be concluded that the long-term drought trend analysis of annual precipitation shows that almost three percent of severe drought and twelve percent of moderate drought were recorded in the study area. The severe and moderate drought indices due to the reduction of rainfall, climate change, and other factors can lead to an extra indication of urban water supply shortage. Lack of analyzed long term drought trends were observed in many African countries and this remains the limitation of water sector professionals in managing the water supply sector. Thus, the results of this study will be of importance to water sector professionals in forecasting weather variations and better management of urban water resources.
Further research study is recommended to conduct drought analysis using the application of soil moisture which is used to estimate the severity of agricultural droughts particularly for sub-Saharan African countries.
We want to express our utmost gratitude to the National Meteorological Agency (NMA) for providing essential data. The view expressed herein are the authors' own and do not necessarily reflect the view of NMA.
The authors have no competing interest to declare.
[1] |
Najihah TS, Ibrahim M H, Zain NAM, et al. (2020) Activity of the oil palm seedlings exposed to a different rate of potassium fertilizer under water stress condition. AIMS Environ Sci 7: 46-68. doi: 10.3934/environsci.2020004
![]() |
[2] |
Sönmez FK, Kömüscü AÜ, Erkan A, et al. (2005) An analysis of spatial and temporal dimension of drought vulnerability in Turkey using the standardized precipitation index. Nat Hazards 35: 243-264. doi: 10.1007/s11069-004-5704-7
![]() |
[3] |
Manjowe M, Mushore TD, Gwenzi J, et al. (2018) Circulation mechanisms responsible for wet or dry summers over Zimbabwe. AIMS Environ Sci 5: 154-172. doi: 10.3934/environsci.2018.3.154
![]() |
[4] |
Smakhtin VU, Hughes DA, (2007) Automated estimation and analyses of meteorological drought characteristics from monthly rainfall data. Environ Modell Softw 22: 880-890. doi: 10.1016/j.envsoft.2006.05.013
![]() |
[5] |
Christy JR (2019) Examination of extreme rainfall events in two regions of the United States since the 19th century. AIMS Environ Sci 6: 109-126. doi: 10.3934/environsci.2019.2.109
![]() |
[6] | Yahaya I, Adamu SJ, Muhammed BB, (2017) The use of Standardized Precipitation Index (SPI) for Drought Intensity Assessment in North-Eastern Nigeria. Researchjournal's J Geo 4:1-13 |
[7] |
Feyissa G, Zeleke G, Bewket W, et al. (2018) Downscaling of future temperature and precipitation extremes in Addis Ababa under climate change. Climate 6: 58. doi: 10.3390/cli6030058
![]() |
[8] | Engida M (1999) Annual rainfall and evapotranspiration in Ethiopia. Ethiopian Journal of Natural Resources 1: 137-154. |
[9] | IPCC (2007) Climate Change 2007: Impacts, Adaptation and Vulnerability. Contribution of Working Group Ⅱ to the Fourth Assessment Report of the Intergovernmental Panel on Climate Change. Edited by Parry, M., Canziani, O., Palutikof, J., Linden, P.vd., Hanson, C., Cambridge University Press 32 Avenue of the Americas, New York, USA, 10013-2473. |
[10] | Alemu ZA, Dioha MO, (2020a) Climate change and trend analysis of temperature: the case of Addis Ababa, Ethiopia. Environ Syst Res 9: 1-15. |
[11] | FDRE, Ethiopian Government Portal, 2018. Available from: http://www.ethiopia.gov.et/addis-ababa-city-administration. |
[12] | Niemeyer S, New drought indices. European Commission, DG Joint Research Centre, Institute for Environment and Sustainability, T.P. 261, JRC-IES, I-21020 Ispra (VA), Italy, 2020 Availablefrom: https://om.ciheam.org/om/pdf/a80/00800451.pdf |
[13] | Gebreyesus M, Cherint A, Ashine T, et al. Drought Analysis Using Reconnaissance Drought Index (RDI): In the case of Awash River Basin, Ethiopia2020. Available from: https://www.researchgate.net/publication/344440865_Drought_analysis_using_reconnaissance_drought_index_RDI_In_the_case_of_Awash_River_Basin_Ethiopia |
[14] | Tigkas D, Vangelis H, Tsakiris G (2013) The RDI as a composite climatic index. E.W. Publications. European Water 41: 17-22. |
[15] | McKee TB, Doesken NJ, Kleist J (1993) The relationship of drought frequency and duration of time scales. Eighth Conference on Applied Climatology, American Meteorological Society, Anaheim CA. |
[16] | Aksoy H, Onoz B, Cetin M, et al. (2018) SPI-based Drought Severity-Duration-Frequency Analysis. 13th International Congress on Advances in Civil Engineering, Izmir/Turkey. |
[17] | Pashiardis S, Michaelides S (2008) Implementation of the Standardized Precipitation Index (SPI) and the Reconnaissance Drought Index (RDI) for Regional Drought Assessment: A case study for Cyprus. E.W. Publications. Eur Water 23/24: 57-65. |
[18] |
Asadi-Zarch MA, Malekinezhad H, Mobin MH, et al. (2011) Drought Monitoring by Reconnaissance Drought Index (RDI) in Iran. Water Resour Manag 25: 3485-3504. doi: 10.1007/s11269-011-9867-1
![]() |
[19] | Ansarifard S, Shamsnia SA (2018) Monitoring drought by Reconnaissance Drought Index (RDI) and Standardized Precipitation Index (SPI) using DrinC software. Water Utility J 20: 29-35. |
[20] |
Hayes MJ, Svoboda MD, Wilhite DA, et al. (1999) Monitoring the 1996 Drought Using the Standardized Precipitation Index. B Am Meteorol Soc 80: 429-438. doi: 10.1175/1520-0477(1999)080<0429:MTDUTS>2.0.CO;2
![]() |
[21] |
Zarei AR, Moghimi MM, Mahmoudi MR (2016) Analysis of Changes in Spatial Pattern of Drought Using RDI Index in south of Iran. Water Resour Manag 30: 3723-3743. doi: 10.1007/s11269-016-1380-0
![]() |
[22] | Pramudya Y, Onishi T, (2018) Assessment of the Standardized Precipitation Index (SPI) in Tegal City, Central Java, Indonesia. IOP Conf. Series: Earth Environ Sci 129: 012-019. |
[23] | Maroua BA, Nouiri I, (2018) Study of trends in historical variation and mapping of drought events in Tunisia: A global assessment of Standardized precipitation index (SPI) and Reconnaissance drought index (RDI) for the period 1973-2016. 3rd International Conference on Integrated Environmental Management for Sustainable Development. ISSN 1737-3638. |
[24] |
Vangelis H, Tigkas D, Tsakiris G, (2012) The effect of PET method on Reconnaissance Drought Index (RDI) calculation. J Arid Environ 88: 130-140. doi: 10.1016/j.jaridenv.2012.07.020
![]() |
[25] |
Tsakiris G, Vangelis H, Pangalou D, (2007) Regional Drought Assessment Based on the Reconnaissance Drought Index (RDI). Water Resour Manage 21: 821-833. doi: 10.1007/s11269-006-9105-4
![]() |
[26] | Fitsume Y, (2014) Precipitation Extremes and their Pattern in the Central Highlands of Ethiopia: SPI Based Analysis. J Nat Sci Res 4: 92-97. |
[27] |
Gidey E, Dikinya O, Sebego R, et al. (2018) Modeling the Spatio-Temporal Meteorological Drought Characteristics Using the Standardized Precipitation Index (SPI) in Raya and Its Environs, Northern Ethiopia. Earth Sys Environ 2: 281-292. doi: 10.1007/s41748-018-0057-7
![]() |
[28] |
Spinoni J, Naumann G, Carrao H, et al. (2013) World drought frequency, duration, and severity for 1951-2010. Int J Climatol 34: 2792-2804. doi: 10.1002/joc.3875
![]() |
[29] |
Tigkas D, Vangelis H, Tsakiris G (2015) DrinC: a software for drought analysis based on drought indices. Earth Sci Inform 8: 697-709. doi: 10.1007/s12145-014-0178-y
![]() |
[30] | Climate-Ethiopia, Climates to travel world climate guide, 2021. Available from: https://www.climatestotravel.com/climate/ethiopia. |
[31] | Alemu ZA, Dioha MO (2020b) Modelling scenarios for sustainable water supply and demand in Addis Ababa city, Ethiopia. Environ Syst Res 9: 1-14. |
[32] | FDRE, City Map of Addis Ababa City Administration, Ethiopia, 2020. Available from: http://www.addisababa.gov.et/de/web/guest/city-map |
[33] | Rossi G, Bonaccorso B, Vega T (2007) Methods and tools for drought analysis and management, Springer Science and Business Media, Berlin. vol 62. ISBN 978-1-4020-5923-0 |
[34] | Tsakiris G, Nalbantis I, Pangalou D, et al. (2008) Drought meteorological monitoring network design for the reconnaissance drought index (RDI). In: Franco Lopez A. (Ed.), Proceedings of the 1st International Conference "Drought Management: scientific and technological innovations". Zaragoza, Spain: Option Méditerranéennes, Series A, 80: 57-62. |
[35] |
Vangelis H, Tigkas D, Tsakiris G (2013) The effect of PET method on Reconnaissance Drought Index (RDI) calculation. J Arid Environ 88: 130-140. doi: 10.1016/j.jaridenv.2012.07.020
![]() |
[36] | Allen RG, Pereira LS, Raes D, et al. (1998) Crop evapotranspiration: guidelines for computing crop water requirements. FAO irrigation and drainage paper 56, 1st edition. Rome, Italy. |
[37] |
Hargreaves GH, Samani ZA (1985) Reference crop evapotranspiration from temperature. Appl Eng Agric 1: 96-99. doi: 10.13031/2013.26773
![]() |
[38] | Tigkas D (2008) Drought Characterization and Monitoring in Regions of Greece. Eur Water 23/24: 29-39. |
[39] |
Khanmohammadi N, Rezaie H, Montaseri M, et al. (2017) The Effect of Temperature Adjustment on Reference Evapotranspiration and Reconnaissance Drought Index (RDI) in Iran. Water Resour Manag 31: 5001-5017. doi: 10.1007/s11269-017-1793-4
![]() |
[40] | Gupta SP (2007) Statistical Methods. Seventh Revised and Enlarged Edition ed. Sultan Chand and Sons, Educational Publisher. New Delhi. |
[41] | Helsel DR, Hirsch RM (2002) Statistical methods in water resources. Techniques of water-resources investigations of the United States geological survey, book 4, hydrologic analysis and interpretation. U. S. Geological survey |
[42] | Philip S, Kew SF, Oldenborgh GJ, et al. (2018) Attribution Analysis of the Ethiopian Drought of 2015. American Meteorological Society 2465-2486. |
[43] | Jamshidi H, Khalili D, Zadeh MR, et al. (2011) Assessment and comparison of SPI and RDI meteorological drought indices in selected synoptic stations of Iran. In World Environmental and Water Resources Congress 2011: Bearing Knowledge for Sustainability, 1161-1173. |
[44] |
Haied N, Foufou A, Chaab S, et al. (2017) Drought assessment and monitoring using meteorological indices in a semi-arid region. Energy Procedia 119: 518-529. doi: 10.1016/j.egypro.2017.07.064
![]() |
[45] |
Shah R, Bharadiya N, Manekar V (2015) Drought index computation using Standardized Precipitation Index (SPI) method for Surat district, Gujarat. J Aquat Proced 4: 1243-1249. doi: 10.1016/j.aqpro.2015.02.162
![]() |
[46] |
Thilakarathne M, Sridhar V (2017) Characterization of future drought conditions in the Lower Mekong River Basin. Weather Climate Extremes 17: 47-58. doi: 10.1016/j.wace.2017.07.004
![]() |
[47] |
Khatiwada KR, Pandey VP (2019) Characterization of hydro-meteorological drought in Nepal Himalaya: A case of Karnali River Basin. Weather Climate Extremes 26: 100239. doi: 10.1016/j.wace.2019.100239
![]() |
[48] |
Abdelmalek MB, Nouiri I (2020) Study of trends and mapping of drought events in Tunisia and their impacts on agricultural production. Sci Total Environ 734: 139311. doi: 10.1016/j.scitotenv.2020.139311
![]() |
[49] | Almedeij J, (2014) Drought analysis for kuwait using standardized precipitation index. The Sc World J. 2014 |
[50] | NMSA, (1996) Climatic and agro climatic resources of Ethiopia. National Meteorology Service Agency of Ethiopia, Addis Ababa. 1: 137. |
[51] |
Temam D, Uddameri V, Mohammadi G, et al. (2019) Long-Term Drought Trends in Ethiopia with Implications for Dryland Agriculture. MDPI-Water 11: 2571. doi: 10.3390/w11122571
![]() |
[52] |
Cermak V, Bodri L, Safanda J, et al. (2019) Variability trends in the daily air temperatures series Running head: Variability trends prague. AIMS Environ Sci 6: 167-185. doi: 10.3934/environsci.2019.3.167
![]() |
1. | Milica Stojanovic, Getachew Mehabie Mulualem, Rogert Sorí, Marta Vázquez, Raquel Nieto, Luis Gimeno, Precipitation Moisture Sources of Ethiopian River Basins and Their Role During Drought Conditions, 2022, 10, 2296-6463, 10.3389/feart.2022.929497 | |
2. | Mostafa Morsy, Fawzia Ibrahim Moursy, Tarek Sayad, Shouk Shaban, Climatological Study of SPEI Drought Index Using Observed and CRU Gridded Dataset over Ethiopia, 2022, 179, 0033-4553, 3055, 10.1007/s00024-022-03091-z | |
3. | Kassa Abera Tareke, Admasu Gebeyehu Awoke, Upaka Rathnayake, Hydrological Drought Analysis using Streamflow Drought Index (SDI) in Ethiopia, 2022, 2022, 1687-9317, 1, 10.1155/2022/7067951 | |
4. | Mostafa Abd El-Hameed Mohamed, Fawzia Ibrahim Moursy, Mohammad Hamdy Darrag, Mohamed El-Sayed El-Mahdy, Assessment of long-term trends and mapping of drought events in Tunisia, 2023, 21, 24682276, e01766, 10.1016/j.sciaf.2023.e01766 |
Drought Category (Classification) | SPI Value |
Extremely Wet | ≥ 2.0 |
Very Wet | 1.5–1.99 |
Moderately Wet | 1.0–1.49 |
Near normal | (-0.99)–(0.99) |
Moderately dry (Moderate Drought) | (-1.0)–(-1.49) |
Severely dry (Sever drought) | (-1.5)–(-1.99) |
Extremely dry (Extreme drought) | ≤ -2.0 |
Drought Category (Classification) | RDI Value |
Extremely Wet | ≥ 2.0 |
Very Wet | 1.5–1.99 |
Moderately Wet | 1.0–1.49 |
Near normal | (-0.99)– (0.99) |
Moderately dry (Moderate Drought) | (-1.0) – (-1.49) |
Severely dry (Sever drought) | (-1.5) – (-1.99) |
Extremely dry (Extreme drought) | ≤ -2.0 |
Year | SPI value for Bole Station | SPI value for Sendafa Station | SPI value for Observatory Station | ||||||||||||
SPI 3 | SPI 12 | SPI 3 | SPI 12 | SPI 3 | SPI 12 | ||||||||||
Autumn | Winter | Spring | Summer | Annual | Autumn | Winter | Spring | Summer | Annual | Autumn | Winter | Spring | Summer | Annual | |
1983 | 1.27 | -1.01 | -0.74 | 2.04 | 1.32 | 0.01 | -1.34 | -0.49 | -0.38 | -1.12 | -0.41 | -1.02 | -0.86 | 1.12 | -0.33 |
1984 | -1.33 | 0.14 | 0.65 | -0.11 | -0.24 | -0.26 | -0.85 | 1.67 | 0.80 | 1.43 | -1.09 | -0.31 | -0.20 | 0.05 | -0.78 |
1985 | 0.67 | 0.04 | 1.00 | -0.27 | 0.55 | -0.15 | -0.19 | 1.58 | -0.47 | 0.22 | 0.69 | -0.14 | 1.67 | -0.89 | 0.80 |
1986 | -0.41 | 0.35 | 1.93 | -3.09 | -0.68 | 0.20 | -0.02 | 1.41 | -2.21 | -1.30 | -0.79 | 0.45 | 2.69 | -1.94 | 0.81 |
1987 | -2.05 | 0.05 | -0.19 | -0.52 | -1.34 | -0.19 | -0.03 | -0.09 | 0.63 | 0.33 | -1.27 | 0.44 | -0.58 | -0.25 | -1.01 |
1988 | 1.37 | 0.02 | -0.16 | 0.30 | 0.53 | 1.23 | -0.19 | 0.14 | -0.11 | 0.34 | 0.96 | 0.68 | 0.15 | 1.03 | 1.13 |
1989 | 0.48 | 2.09 | 0.18 | -0.56 | 0.64 | 0.29 | 2.96 | 0.60 | 0.40 | 1.57 | -0.18 | 1.84 | -0.34 | -1.52 | -0.50 |
1990 | 0.55 | -0.17 | -0.76 | 0.75 | 0.15 | 2.88 | 0.08 | -0.12 | -3.34 | -0.98 | -0.12 | 0.64 | -0.21 | 0.09 | -0.10 |
1991 | -0.60 | 0.15 | -0.88 | -0.32 | -1.10 | 0.73 | 0.76 | -1.76 | -0.46 | -0.97 | -1.42 | 1.10 | -1.35 | -0.60 | -1.37 |
1992 | 1.06 | 0.60 | 0.62 | 0.60 | 1.23 | 0.90 | 1.63 | -1.20 | 0.89 | 1.10 | 0.83 | 0.75 | 0.55 | 2.05 | 1.88 |
1993 | 0.92 | -1.03 | -0.83 | -0.38 | -0.80 | 0.49 | -1.34 | -1.20 | -0.63 | -1.46 | 1.14 | -1.22 | 0.13 | -0.54 | -0.08 |
1994 | -1.03 | 0.90 | 0.92 | -0.94 | -0.26 | 0.01 | -0.88 | 0.98 | -0.69 | -0.49 | -0.90 | 0.54 | 0.67 | -1.13 | -0.57 |
1995 | -1.38 | 0.63 | 1.40 | 2.89 | 2.70 | -0.08 | 1.01 | 0.92 | 0.52 | 1.03 | -1.29 | 0.76 | 1.27 | 1.62 | 1.33 |
1996 | 0.88 | -0.19 | -1.12 | -0.46 | -0.80 | -0.08 | 0.31 | -0.35 | -0.33 | -0.75 | 1.01 | -0.08 | -1.33 | -1.59 | -1.23 |
1997 | 0.16 | 1.29 | 1.28 | 0.25 | 1.35 | 0.74 | 0.51 | -1.26 | -0.25 | -0.58 | 0.16 | 0.68 | 0.36 | -0.40 | 0.10 |
1998 | 2.26 | -0.95 | -2.09 | 0.70 | 0.27 | 1.30 | -1.32 | -0.91 | 0.94 | 0.91 | 1.52 | -1.27 | -2.23 | 0.15 | -0.48 |
1999 | 0.53 | -1.03 | -0.17 | -0.76 | -0.90 | 1.13 | -1.34 | 0.04 | 0.65 | 0.88 | -0.79 | -1.34 | -0.44 | -0.11 | -1.11 |
2000 | 0.44 | -0.77 | 0.96 | 0.64 | 0.89 | 0.02 | 0.04 | 1.25 | -0.01 | 0.52 | 1.28 | -0.92 | 1.62 | 1.88 | 2.31 |
2001 | -0.73 | 0.45 | -0.63 | -0.29 | -0.89 | -1.76 | -0.32 | -0.17 | -0.47 | -1.49 | -1.12 | -0.17 | -0.07 | -0.68 | -1.11 |
2002 | -2.07 | 0.74 | -0.46 | -0.38 | -1.14 | -0.64 | 1.52 | -0.11 | 0.18 | 0.06 | -1.86 | 0.74 | -0.38 | -0.32 | -1.03 |
2003 | -0.49 | 0.73 | -0.45 | -0.07 | -0.43 | -0.07 | 0.24 | -0.52 | -0.33 | -0.84 | -0.28 | 1.04 | 0.04 | -0.59 | -0.25 |
2004 | 0.23 | 0.70 | 1.11 | -0.11 | 0.78 | -1.99 | 0.37 | 1.25 | -0.23 | -0.19 | 0.35 | 1.00 | 1.43 | 0.38 | 1.44 |
2005 | -1.04 | 0.06 | 0.28 | 1.46 | 0.79 | -0.28 | -0.41 | -0.53 | 1.07 | 0.56 | 0.39 | -0.93 | 0.61 | 0.48 | 0.45 |
2006 | 0.16 | -0.13 | 0.73 | 0.57 | 0.71 | -0.87 | 0.12 | -0.46 | 0.16 | -0.59 | 1.00 | 0.70 | 0.38 | 1.12 | 1.33 |
2007 | 0.15 | -1.03 | -1.55 | 0.32 | -0.92 | -0.19 | 0.12 | -1.88 | -0.44 | -1.86 | -0.65 | -0.89 | -0.88 | 0.23 | -0.98 |
2008 | 1.69 | 0.11 | -1.53 | -0.07 | -0.19 | 1.41 | 0.12 | -0.46 | 0.16 | 0.51 | 2.33 | 0.10 | -0.56 | 1.20 | 1.45 |
2009 | -0.59 | 2.33 | 1.18 | -0.26 | 1.26 | -0.19 | 0.47 | 1.26 | -0.25 | 0.30 | -0.81 | 1.65 | 0.12 | 0.90 | 0.72 |
2010 | -0.84 | -0.09 | -1.07 | 0.32 | -0.84 | -0.99 | 0.47 | 0.64 | -0.75 | -0.93 | 0.66 | -0.01 | -1.04 | -0.02 | -0.40 |
2011 | -0.13 | -1.03 | -0.60 | -0.09 | -0.92 | -0.99 | 0.47 | 0.64 | -0.75 | -0.93 | -0.39 | -1.34 | -0.97 | -0.15 | -1.24 |
2012 | 0.33 | -0.78 | 0.47 | -0.63 | -0.41 | -0.99 | 0.42 | -0.97 | 1.59 | 0.95 | 0.04 | -0.84 | 0.09 | 0.31 | -0.11 |
2013 | 0.28 | 0.13 | -1.26 | -1.21 | -1.61 | -2.11 | -0.32 | -0.59 | 1.26 | 0.34 | 0.81 | 0.15 | -0.39 | -1.87 | -0.97 |
2014 | -0.60 | -1.03 | 0.74 | -1.01 | -0.91 | 0.23 | -0.56 | -0.23 | 1.91 | 1.84 | 1.09 | -1.34 | -0.94 | 0.38 | -0.16 |
2015 | -0.13 | 0.74 | 1.07 | 0.71 | 1.20 | 0.23 | -1.01 | 1.32 | 0.99 | 1.56 | -0.92 | 0.60 | 0.95 | -0.40 | 0.05 |
SPI based drought Classification | RDI based drought Classification | |||
Year | Bole station | Observatory station | Bole station | Observatory station |
1983–1986 | Normal | Normal | Normal | Normal |
1987 | Moderate Drought | Moderate Drought | Moderate Drought | Normal |
1988–1990 | Normal | Normal | Normal | Normal |
1991 | Moderate Drought | Moderate Drought | Moderate Drought | Moderate Drought |
1992–1995 | Normal | Normal | Normal | Normal |
1996 | Normal | Moderate Drought | Normal | Severe Drought |
1997–1998 | Normal | Normal | Normal | Normal |
1999 | Normal | Moderate Drought | Normal | Moderate Drought |
2000 | Normal | Normal | Normal | Normal |
2001 | Normal | Moderate Drought | Moderate Drought | Moderate Drought |
2002 | Moderate Drought | Moderate Drought | Moderate Drought | Moderate Drought |
2003–2010 | Normal | Normal | Normal | Normal |
2011 | Normal | Moderate Drought | Normal | Moderate Drought |
2012 | Normal | Normal | Normal | Normal |
2013 | Severe Drought | Normal | Severe Drought | Normal |
2014–2015 | Normal | Normal | Normal | Normal |
Descriptive Statistics | ||||||
Station | Time Scale | Min | Max | Mean | SD | % CV |
Sendafa | Autumn | 27.50 | 479.90 | 142.18 | 86.06 | 60.53 |
Winter | 0.00 | 212.50 | 41.81 | 40.06 | 95.82 | |
Spring | 0.00 | 355.40 | 176.59 | 94.21 | 53.35 | |
Summer | 298.90 | 1173.90 | 778.58 | 177.44 | 22.79 | |
Annual | 836.50 | 1481.40 | 1139.16 | 177.60 | 15.59 | |
Bole | Autumn | 73.10 | 312.80 | 165.82 | 55.03 | 33.18 |
Winter | 0.00 | 195.50 | 46.86 | 44.95 | 95.93 | |
Spring | 83.30 | 420.40 | 225.73 | 85.80 | 38.01 | |
Summer | 325.90 | 949.20 | 599.12 | 108.25 | 18.07 | |
Annual | 817.50 | 1466.80 | 1037.52 | 149.15 | 14.38 | |
Observation | Autumn | 109.00 | 424.30 | 222.60 | 74.09 | 33.28 |
Winter | 0.00 | 164.30 | 53.95 | 42.03 | 77.91 | |
Spring | 68.90 | 572.60 | 229.34 | 104.23 | 45.45 | |
Summer | 543.80 | 908.80 | 709.52 | 92.85 | 13.09 | |
Annual | 999.20 | 1624.70 | 1215.41 | 169.94 | 13.98 |
Drought Category (Classification) | SPI Value |
Extremely Wet | ≥ 2.0 |
Very Wet | 1.5–1.99 |
Moderately Wet | 1.0–1.49 |
Near normal | (-0.99)–(0.99) |
Moderately dry (Moderate Drought) | (-1.0)–(-1.49) |
Severely dry (Sever drought) | (-1.5)–(-1.99) |
Extremely dry (Extreme drought) | ≤ -2.0 |
Drought Category (Classification) | RDI Value |
Extremely Wet | ≥ 2.0 |
Very Wet | 1.5–1.99 |
Moderately Wet | 1.0–1.49 |
Near normal | (-0.99)– (0.99) |
Moderately dry (Moderate Drought) | (-1.0) – (-1.49) |
Severely dry (Sever drought) | (-1.5) – (-1.99) |
Extremely dry (Extreme drought) | ≤ -2.0 |
Year | SPI value for Bole Station | SPI value for Sendafa Station | SPI value for Observatory Station | ||||||||||||
SPI 3 | SPI 12 | SPI 3 | SPI 12 | SPI 3 | SPI 12 | ||||||||||
Autumn | Winter | Spring | Summer | Annual | Autumn | Winter | Spring | Summer | Annual | Autumn | Winter | Spring | Summer | Annual | |
1983 | 1.27 | -1.01 | -0.74 | 2.04 | 1.32 | 0.01 | -1.34 | -0.49 | -0.38 | -1.12 | -0.41 | -1.02 | -0.86 | 1.12 | -0.33 |
1984 | -1.33 | 0.14 | 0.65 | -0.11 | -0.24 | -0.26 | -0.85 | 1.67 | 0.80 | 1.43 | -1.09 | -0.31 | -0.20 | 0.05 | -0.78 |
1985 | 0.67 | 0.04 | 1.00 | -0.27 | 0.55 | -0.15 | -0.19 | 1.58 | -0.47 | 0.22 | 0.69 | -0.14 | 1.67 | -0.89 | 0.80 |
1986 | -0.41 | 0.35 | 1.93 | -3.09 | -0.68 | 0.20 | -0.02 | 1.41 | -2.21 | -1.30 | -0.79 | 0.45 | 2.69 | -1.94 | 0.81 |
1987 | -2.05 | 0.05 | -0.19 | -0.52 | -1.34 | -0.19 | -0.03 | -0.09 | 0.63 | 0.33 | -1.27 | 0.44 | -0.58 | -0.25 | -1.01 |
1988 | 1.37 | 0.02 | -0.16 | 0.30 | 0.53 | 1.23 | -0.19 | 0.14 | -0.11 | 0.34 | 0.96 | 0.68 | 0.15 | 1.03 | 1.13 |
1989 | 0.48 | 2.09 | 0.18 | -0.56 | 0.64 | 0.29 | 2.96 | 0.60 | 0.40 | 1.57 | -0.18 | 1.84 | -0.34 | -1.52 | -0.50 |
1990 | 0.55 | -0.17 | -0.76 | 0.75 | 0.15 | 2.88 | 0.08 | -0.12 | -3.34 | -0.98 | -0.12 | 0.64 | -0.21 | 0.09 | -0.10 |
1991 | -0.60 | 0.15 | -0.88 | -0.32 | -1.10 | 0.73 | 0.76 | -1.76 | -0.46 | -0.97 | -1.42 | 1.10 | -1.35 | -0.60 | -1.37 |
1992 | 1.06 | 0.60 | 0.62 | 0.60 | 1.23 | 0.90 | 1.63 | -1.20 | 0.89 | 1.10 | 0.83 | 0.75 | 0.55 | 2.05 | 1.88 |
1993 | 0.92 | -1.03 | -0.83 | -0.38 | -0.80 | 0.49 | -1.34 | -1.20 | -0.63 | -1.46 | 1.14 | -1.22 | 0.13 | -0.54 | -0.08 |
1994 | -1.03 | 0.90 | 0.92 | -0.94 | -0.26 | 0.01 | -0.88 | 0.98 | -0.69 | -0.49 | -0.90 | 0.54 | 0.67 | -1.13 | -0.57 |
1995 | -1.38 | 0.63 | 1.40 | 2.89 | 2.70 | -0.08 | 1.01 | 0.92 | 0.52 | 1.03 | -1.29 | 0.76 | 1.27 | 1.62 | 1.33 |
1996 | 0.88 | -0.19 | -1.12 | -0.46 | -0.80 | -0.08 | 0.31 | -0.35 | -0.33 | -0.75 | 1.01 | -0.08 | -1.33 | -1.59 | -1.23 |
1997 | 0.16 | 1.29 | 1.28 | 0.25 | 1.35 | 0.74 | 0.51 | -1.26 | -0.25 | -0.58 | 0.16 | 0.68 | 0.36 | -0.40 | 0.10 |
1998 | 2.26 | -0.95 | -2.09 | 0.70 | 0.27 | 1.30 | -1.32 | -0.91 | 0.94 | 0.91 | 1.52 | -1.27 | -2.23 | 0.15 | -0.48 |
1999 | 0.53 | -1.03 | -0.17 | -0.76 | -0.90 | 1.13 | -1.34 | 0.04 | 0.65 | 0.88 | -0.79 | -1.34 | -0.44 | -0.11 | -1.11 |
2000 | 0.44 | -0.77 | 0.96 | 0.64 | 0.89 | 0.02 | 0.04 | 1.25 | -0.01 | 0.52 | 1.28 | -0.92 | 1.62 | 1.88 | 2.31 |
2001 | -0.73 | 0.45 | -0.63 | -0.29 | -0.89 | -1.76 | -0.32 | -0.17 | -0.47 | -1.49 | -1.12 | -0.17 | -0.07 | -0.68 | -1.11 |
2002 | -2.07 | 0.74 | -0.46 | -0.38 | -1.14 | -0.64 | 1.52 | -0.11 | 0.18 | 0.06 | -1.86 | 0.74 | -0.38 | -0.32 | -1.03 |
2003 | -0.49 | 0.73 | -0.45 | -0.07 | -0.43 | -0.07 | 0.24 | -0.52 | -0.33 | -0.84 | -0.28 | 1.04 | 0.04 | -0.59 | -0.25 |
2004 | 0.23 | 0.70 | 1.11 | -0.11 | 0.78 | -1.99 | 0.37 | 1.25 | -0.23 | -0.19 | 0.35 | 1.00 | 1.43 | 0.38 | 1.44 |
2005 | -1.04 | 0.06 | 0.28 | 1.46 | 0.79 | -0.28 | -0.41 | -0.53 | 1.07 | 0.56 | 0.39 | -0.93 | 0.61 | 0.48 | 0.45 |
2006 | 0.16 | -0.13 | 0.73 | 0.57 | 0.71 | -0.87 | 0.12 | -0.46 | 0.16 | -0.59 | 1.00 | 0.70 | 0.38 | 1.12 | 1.33 |
2007 | 0.15 | -1.03 | -1.55 | 0.32 | -0.92 | -0.19 | 0.12 | -1.88 | -0.44 | -1.86 | -0.65 | -0.89 | -0.88 | 0.23 | -0.98 |
2008 | 1.69 | 0.11 | -1.53 | -0.07 | -0.19 | 1.41 | 0.12 | -0.46 | 0.16 | 0.51 | 2.33 | 0.10 | -0.56 | 1.20 | 1.45 |
2009 | -0.59 | 2.33 | 1.18 | -0.26 | 1.26 | -0.19 | 0.47 | 1.26 | -0.25 | 0.30 | -0.81 | 1.65 | 0.12 | 0.90 | 0.72 |
2010 | -0.84 | -0.09 | -1.07 | 0.32 | -0.84 | -0.99 | 0.47 | 0.64 | -0.75 | -0.93 | 0.66 | -0.01 | -1.04 | -0.02 | -0.40 |
2011 | -0.13 | -1.03 | -0.60 | -0.09 | -0.92 | -0.99 | 0.47 | 0.64 | -0.75 | -0.93 | -0.39 | -1.34 | -0.97 | -0.15 | -1.24 |
2012 | 0.33 | -0.78 | 0.47 | -0.63 | -0.41 | -0.99 | 0.42 | -0.97 | 1.59 | 0.95 | 0.04 | -0.84 | 0.09 | 0.31 | -0.11 |
2013 | 0.28 | 0.13 | -1.26 | -1.21 | -1.61 | -2.11 | -0.32 | -0.59 | 1.26 | 0.34 | 0.81 | 0.15 | -0.39 | -1.87 | -0.97 |
2014 | -0.60 | -1.03 | 0.74 | -1.01 | -0.91 | 0.23 | -0.56 | -0.23 | 1.91 | 1.84 | 1.09 | -1.34 | -0.94 | 0.38 | -0.16 |
2015 | -0.13 | 0.74 | 1.07 | 0.71 | 1.20 | 0.23 | -1.01 | 1.32 | 0.99 | 1.56 | -0.92 | 0.60 | 0.95 | -0.40 | 0.05 |
SPI based drought Classification | RDI based drought Classification | |||
Year | Bole station | Observatory station | Bole station | Observatory station |
1983–1986 | Normal | Normal | Normal | Normal |
1987 | Moderate Drought | Moderate Drought | Moderate Drought | Normal |
1988–1990 | Normal | Normal | Normal | Normal |
1991 | Moderate Drought | Moderate Drought | Moderate Drought | Moderate Drought |
1992–1995 | Normal | Normal | Normal | Normal |
1996 | Normal | Moderate Drought | Normal | Severe Drought |
1997–1998 | Normal | Normal | Normal | Normal |
1999 | Normal | Moderate Drought | Normal | Moderate Drought |
2000 | Normal | Normal | Normal | Normal |
2001 | Normal | Moderate Drought | Moderate Drought | Moderate Drought |
2002 | Moderate Drought | Moderate Drought | Moderate Drought | Moderate Drought |
2003–2010 | Normal | Normal | Normal | Normal |
2011 | Normal | Moderate Drought | Normal | Moderate Drought |
2012 | Normal | Normal | Normal | Normal |
2013 | Severe Drought | Normal | Severe Drought | Normal |
2014–2015 | Normal | Normal | Normal | Normal |
Descriptive Statistics | ||||||
Station | Time Scale | Min | Max | Mean | SD | % CV |
Sendafa | Autumn | 27.50 | 479.90 | 142.18 | 86.06 | 60.53 |
Winter | 0.00 | 212.50 | 41.81 | 40.06 | 95.82 | |
Spring | 0.00 | 355.40 | 176.59 | 94.21 | 53.35 | |
Summer | 298.90 | 1173.90 | 778.58 | 177.44 | 22.79 | |
Annual | 836.50 | 1481.40 | 1139.16 | 177.60 | 15.59 | |
Bole | Autumn | 73.10 | 312.80 | 165.82 | 55.03 | 33.18 |
Winter | 0.00 | 195.50 | 46.86 | 44.95 | 95.93 | |
Spring | 83.30 | 420.40 | 225.73 | 85.80 | 38.01 | |
Summer | 325.90 | 949.20 | 599.12 | 108.25 | 18.07 | |
Annual | 817.50 | 1466.80 | 1037.52 | 149.15 | 14.38 | |
Observation | Autumn | 109.00 | 424.30 | 222.60 | 74.09 | 33.28 |
Winter | 0.00 | 164.30 | 53.95 | 42.03 | 77.91 | |
Spring | 68.90 | 572.60 | 229.34 | 104.23 | 45.45 | |
Summer | 543.80 | 908.80 | 709.52 | 92.85 | 13.09 | |
Annual | 999.20 | 1624.70 | 1215.41 | 169.94 | 13.98 |