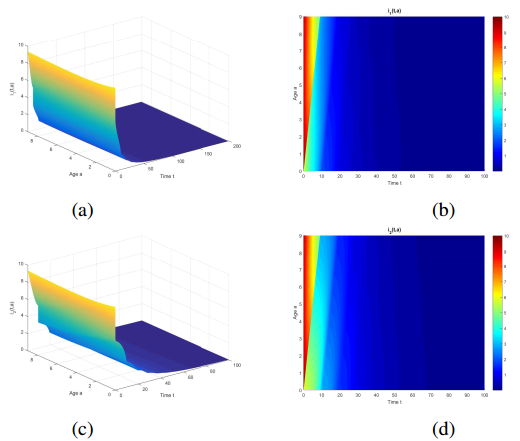
An entropically-driven binding interaction at a certain temperature may change to an enthalpically-driven process at another temperature, depending on the polarization state of the groups that are involved in binding. The streptavidin-biotin complex has been extensively studied across biological, medical, chemical and material science fields using various techniques, however, not much has been reported on this interaction across a broad temperature range, between 2 °C and 40 °C using biophysical techniques. In this study, we determined how the forces involved in the streptavidin-biotin complex formation are affected by the reaction temperature using the Affinity ITC (TA Instruments). We observed that this complex formation is a spontaneous binding process, indicated by a negative Gibbs energy (ΔG) at all temperatures tested. The observed negative heat capacity (ΔCp) ∼ −459.9 cal/mol K highlights the polar solvation of the interaction that corresponds to a decreasing enthalpy (more negative) (ΔH) with increasing reaction temperature. The stoichiometry (n) of 0.98 was estimated at 25 °C. An increase in reaction temperature resulted in an almost two-fold increase or more in n, notably from 1.59 to 3.41 between 30 °C and 40 °C. Whereas, at lower reaction temperatures, 2 °C to 10 °C, higher molar binding ratios were recorded, i.e. 2.74 to 5.76. We report an enthalpically-driven interaction between 30 °C and 40 °C whereas, an entropically-favourable interaction is observed at lower temperatures, suggestive of an interaction dominated by nonpolar interactions at lower temperatures and polar interactions at higher temperatures. Consequently, alterations in the polarisation state of streptavidin result in moderate binding affinity of biotin to streptavidin at higher reaction temperatures, KD 10−4 ≤ 10−5 M.
Citation: Keleabetswe L. Mpye, Samantha Gildenhuys, Salerwe Mosebi. The effects of temperature on streptavidin-biotin binding using affinity isothermal titration calorimetry[J]. AIMS Biophysics, 2020, 7(4): 236-247. doi: 10.3934/biophy.2020018
[1] | Abigail Gbemisola Adeyonu, Olubunmi Lawrence Balogun, Babatunde Oluseyi Ajiboye, Isaac Busayo Oluwatayo, Abiodun Olanrewaju Otunaiya . Sweet potato production efficiency in Nigeria: Application of data envelopment analysis. AIMS Agriculture and Food, 2019, 4(3): 672-684. doi: 10.3934/agrfood.2019.3.672 |
[2] | Saeed Hosseini Nejad, Reza Moghaddasi, Amir Mohammadi Nejad . On the role of credit in agricultural growth: An Iranian panel data analysis. AIMS Agriculture and Food, 2018, 3(1): 1-11. doi: 10.3934/agrfood.2018.1.1 |
[3] | Lindie V. Maltitz, Yonas T. Bahta . Empowerment of smallholder female livestock farmers and its potential impacts to their resilience to agricultural drought. AIMS Agriculture and Food, 2021, 6(2): 603-630. doi: 10.3934/agrfood.2021036 |
[4] | Ogunniyi Adebayo, Omonona Bolarin, Abioye Oyewale, Olagunju Kehinde . Impact of irrigation technology use on crop yield, crop income and household food security in Nigeria: A treatment effect approach. AIMS Agriculture and Food, 2018, 3(2): 154-171. doi: 10.3934/agrfood.2018.2.154 |
[5] | Raulston Derrick Gillette, Norio Sakai, Godfrid Erasme Ibikoule . Role and impact of contract farming under various pricing standards: A case of Guyana's rice sector. AIMS Agriculture and Food, 2024, 9(1): 336-355. doi: 10.3934/agrfood.2024020 |
[6] | Hien Thi Vu, Ke-Chung Peng, Rebecca H. Chung . Efficiency measurement of edible canna production in Vietnam. AIMS Agriculture and Food, 2020, 5(3): 466-479. doi: 10.3934/agrfood.2020.3.466 |
[7] | Abbas Ali Chandio, Yuansheng Jiang . Factors influencing the adoption of improved wheat varieties by rural households in Sindh, Pakistan. AIMS Agriculture and Food, 2018, 3(3): 216-228. doi: 10.3934/agrfood.2018.3.216 |
[8] | Marcello Mastrorilli, Raffaella Zucaro . Towards sustainable use of water in rainfed and irrigated cropping systems: review of some technical and policy issues. AIMS Agriculture and Food, 2016, 1(3): 294-314. doi: 10.3934/agrfood.2016.3.294 |
[9] | Radhwane Derraz, Farrah Melissa Muharam, Noraini Ahmad Jaafar . Uncertainty sources affecting operational efficiency of ML algorithms in UAV-based precision agriculture: A 2013–2020 systematic review. AIMS Agriculture and Food, 2023, 8(2): 687-719. doi: 10.3934/agrfood.2023038 |
[10] | Boris Boincean, Amir Kassam, Gottlieb Basch, Don Reicosky, Emilio Gonzalez, Tony Reynolds, Marina Ilusca, Marin Cebotari, Grigore Rusnac, Vadim Cuzeac, Lidia Bulat, Dorian Pasat, Stanislav Stadnic, Sergiu Gavrilas, Ion Boaghii . Towards Conservation Agriculture systems in Moldova. AIMS Agriculture and Food, 2016, 1(4): 369-386. doi: 10.3934/agrfood.2016.4.369 |
An entropically-driven binding interaction at a certain temperature may change to an enthalpically-driven process at another temperature, depending on the polarization state of the groups that are involved in binding. The streptavidin-biotin complex has been extensively studied across biological, medical, chemical and material science fields using various techniques, however, not much has been reported on this interaction across a broad temperature range, between 2 °C and 40 °C using biophysical techniques. In this study, we determined how the forces involved in the streptavidin-biotin complex formation are affected by the reaction temperature using the Affinity ITC (TA Instruments). We observed that this complex formation is a spontaneous binding process, indicated by a negative Gibbs energy (ΔG) at all temperatures tested. The observed negative heat capacity (ΔCp) ∼ −459.9 cal/mol K highlights the polar solvation of the interaction that corresponds to a decreasing enthalpy (more negative) (ΔH) with increasing reaction temperature. The stoichiometry (n) of 0.98 was estimated at 25 °C. An increase in reaction temperature resulted in an almost two-fold increase or more in n, notably from 1.59 to 3.41 between 30 °C and 40 °C. Whereas, at lower reaction temperatures, 2 °C to 10 °C, higher molar binding ratios were recorded, i.e. 2.74 to 5.76. We report an enthalpically-driven interaction between 30 °C and 40 °C whereas, an entropically-favourable interaction is observed at lower temperatures, suggestive of an interaction dominated by nonpolar interactions at lower temperatures and polar interactions at higher temperatures. Consequently, alterations in the polarisation state of streptavidin result in moderate binding affinity of biotin to streptavidin at higher reaction temperatures, KD 10−4 ≤ 10−5 M.
As history indicated, infectious diseases have been becoming a main enemy affecting human's health and economic development. Mathematical modelling is an useful tool to investigate the mechanisms of transmission of diseases and make optimal control measures [1]. There are many ways to suppress the disease transmission, such as media propagation, vaccination, quarantine and so on [2]. As we know, vaccination is one of effective methods to control and prevent disease prevalence. Indeed, vaccination has succeeded in slowing down transmission of diseases such as tuberculosis, hepatitis, and some children diseases [3]. However, it has been reported that vaccination immunity waning has caused some diseases reemergence such as measles, rubella and pertussis. There is no doubt that vaccine waning has great effects on understanding the evolution of diseases. Based on the epidemic compartment knowledge in Kermack and McKendrick [4,5,6], an SIVS epidemic model can be written as follows [7]:
dS(t)dt=b−βSI−(μ+p)S+ϵV,dI(t)dt=βSI−(μ+γ)I,dR(t)dt=γI(t)−μR,dV(t)dt=pS−(μ+ϵ)V(t), | (1) |
where the total population is splited into four classes (Susceptible, Infected, Recovered and Vaccinated). b is the birth rate, μ is the natural death rate, β is the transmission rate, γ is the recovery rate, p is the vaccinated rate, ϵ is the vaccine waning rate. In [7], Li and Yang investigated two vaccine strategies consisting of continuous and impulsive styles, and they obtained the global stability of equilibria by constructing Lyapunov functionals. Zaleta and Hernández proposed an SIVS model with a standard incidence rate and a disease-induced death rate [8]. They showed that their model exhibits a backward bifurcation. Based on model (1), many researchers have evolved many different structures and successfully captured the key characters of diseases transmission and evaluated the risk of their prevalence (see, for examples, [9,10,11]).
We note that all models mentioned above are based on the homogeneous assumptions for host population. However, host heterogeneity plays an important role in exploring their dynamics. Many diseases such as tuberculosis, hepatitis C, HIV/AIDS and so on infect their host for a long time and sometimes for the duration of lifespan. During the long infectious period, the variability of infectivity with age-since-infection has been studied most extensively in HIV infection [12]. The immunity waning process of pertussis satisfies Gamma distributions [13], which can be expressed by the vaccinated age. In this case, the densities of the infected and vaccinated in time t and age a are denoted by i(t,a), and v(t,a), respectively. The parameters β,γ, and ϵ in system (1) are associated with age a. Based on system (1), the model can be described as follows:
dS(t)dt=b−S(t)∫∞0β(a)i(t,a)da−(μ+p)S(t)+∫∞0ϵ(a)v(t,a)da,∂i(t,a)∂t+∂i(t,a)∂a=−(μ+γ(a))i(t,a),∂v(t,a)∂t+∂v(t,a)∂a=−(μ+ϵ(a))v(t,a),i(t,0)=S(t)∫∞0β(a)i(t,a)da,v(t,0)=pS(t),dR(t)dt=∫∞0γ(a)i(t,a)da−μR(t),S(0)=S0∈R+,i(0,a)=i0(a)∈L1+(R+),v(0,a)=v0(a)∈L1+(R+), | (2) |
where R+=(0,+∞), and L1+(R+) denotes the space of all the integral functions in L1 and maintaining positivity after integral. Obviously, system (2) is a hybrid system combining an ordinary differential equation and two partial differential equations. Global dynamics of such a system has been becoming a challenging issue due to lack of well posed mathematical techniques.
On the other hand, some diseases such as mumps, measles, gonorrhea, HIV/AIDS etc exhibit heterogeneity in host populations. Groups can be geographical such as counties, cities, communities, or epidemiological as different infectivity and multi-stain agents. Many authors introduced an irreducible matrix and summing elements of the matrix together as a kernel function to describe the inter-group and intra-group infections. In [14], Lajmanovich and Yorke proposed the earliest multi-group model for gonorrhea spread in a community and investigated the global stability. They assumed that the total size of population doesn't change and maintain a constant. Under this assumption their model can be simplified to just consider the infected classes. Since then, many multi-group epidemic models have been studied (see, for example [15,19,20]). In [21], Guo et al proposed a multi-group SIR epidemic model and used the graph-theoretic approach to investigate the global stability of endemic equilibrium. This method combining with nonnegative irreducible matrix is an effective tool in solving the global behavior of endemic equilibrium. In [20], Kuniya considered a multi-group SVIR model to explore the global behavior of equilibria by constructing Lyapunov functional and using a developed graph-theoretic method. There are few literatures incorporating age structure into multi-group epidemic models [15].
Motivated by the discussions above, we separate the total population into n groups and four compartments: susceptible, infected, and vaccinated, recovered, denoted by Sk(t),ik(t,a) and vk(t,a) and Rk(t), respectively. ik(t,a) denotes the infected individuals at time t and infection age a in group k. vk(t,a) denotes the vaccinated individuals at time t and vaccinated age a in group k. Rk(t) represents the recovered individuals at time t. Susceptible individuals in group k can be infected by infected individuals in group j at rate βkj(a). Hence, we denote the incidence rate in group k in the form of
λk(t)=n∑j=1∫∞0βkj(a)ij(t,a)da. |
Susceptible individuals in group k can be vaccinated at rate pk and become vaccinated individuals with immunity. The vaccinated individuals in group k lose its immunity at rate ϵk(a) and become susceptible individuals. A multi-group SIRVS epidemic model is formulated by the following differential equations:
dSk(t)dt=bk−Sk(t)λk(t)−(μk+pk)Sk(t)+∫∞0ϵk(a)vk(t,a)da,∂ik(t,a)∂t+∂ik(t,a)∂a=−(μk+γk(a))ik(t,a),∂vk(t,a)∂t+∂vk(t,a)∂a=−(μk+ϵk(a))vk(t,a),ik(t,0)=Sk(t)λk(t),vk(t,0)=pkSk(t),dRk(t)dt=∫∞0γk(a)ik(t,a)da−μkRk(t), | (3) |
where bk denotes the birth rate, γk is the recovery rate with respect to infection age a, μk is the natural death rate in group k. In order to satisfy the biological meaning, all the parameters are assumed to be nonnegative and bk>0 and μk>0. Note that the total population Nk(t)=Sk(t)+∫∞0ik(t,a)da+Rk(t)+∫∞0vk(t,a)da satisfies the following equation:
dNk(t)dt=bk−μkNk(t), | (4) |
which yields limt→∞Nk(t)=bkμk. Without loss of generality, we assume that the total population is Nk=bkμk. Since the first four equations in (3) do not contain the variable Rk, we can consider the following closed subsystem:
dSk(t)dt=bk−Sk(t)λk(t)−(μk+pk)Sk(t)+∫∞0ϵk(a)vk(t,a)da,∂ik(t,a)∂t+∂ik(t,a)∂a=−(μk+γk(a))ik(t,a),∂vk(t,a)∂t+∂vk(t,a)∂a=−(μk+ϵk(a))vk(t,a),ik(t,0)=Sk(t)λk(t),vk(t,0)=pkSk(t),Sk(0)=Sk0∈R+,ik(0,a)=ik0(a)∈L1+(R+),vk(0,a)=vk0(a)∈L1+(R+). | (5) |
Once behaviors of Sk(t), ik(t,a) and vk(t,a) are known, those of Rk(t) can be derived from the fourth equation in (3). For convenience, we make the following assumption:
Assumption 1.1. For system (5), we assume
(ⅰ) For each j,k∈{1,2,⋯,n}, βjk(a),γk(a),ϵk(a)∈L∞+(0,∞). That is, there exist positive constants β+jk and ϵ+k such that
esssupa∈[0,+∞)βjk(a)=β+jk,esssupa∈[0,∞)γk(a)=γ+k,esssupa∈[0,∞)ϵk(a)=ϵ+k. |
(ⅱ) For each j,k∈{1,2,⋯,n}, βjk(a) satisfies the following property:
limh→0∫∞0|βjk(a+h)−βjk(a)|da=0. |
(ⅲ) For each j,k∈{1,2,⋯,n}, there exists an ε0>0 such that for almost all a∈[0,+∞),βjk(a)≥ε0.
(ⅳ) For each j,k∈{1,2,⋯,n}, the matrix (βjk)n×n is irreducible.
The assumptions above on the parameters in system (5) are naturally satisfied for some real diseases. Evidence exists that the infectivity βjk(a) has been addressed by Gamma and Log-normal distributions for smallpox [16,17], Weibull distribution for Ebola [18]. It is easy to find that all of these probability distribution functions have peak values and they are continuous. Hence, (ⅰ) and (ⅱ) in Assumption 1.1 readily hold. As for (ⅲ) in Assumption 1.1, we can modify it in a more generalized form:
(ⅲ)' There exists a positive constant}aβ such that βjk(a)(k,j∈N) is positive in a neighbourhood of aβ.
Obviously, the distribution functions mentioned above have this property. If we assume that every group keeps up close exchanges in mutual contact, then the generated graph is strongly connected. (ⅳ) in Assumption 1.1 is satisfied automatically.
In order to investigate the dynamic behavior of system (5), define the functional spaces
X=(R×L1(R+,R))n,Y=X×X,Z=Rn×X×X, |
and
X0=({0}×L1(R+,R))n,Y0=X0×X0,Z0=Rn×X0×X0 |
with the norm
‖ϕ‖Rn=n∑j=1|ϕj|,ϕ=(ϕ1,ϕ2,⋯,ϕn)T∈Rn, |
‖ϕ‖X0=n∑j=1∫∞0|ϕj(a)|da,ϕ=(ϕ1,ϕ2,⋯,ϕn)T∈L1(R+,Rn), |
‖ψ‖Y0=‖ψ1‖X0+‖ψ2‖X0,‖ψ‖Z=‖ψ1‖Rn+‖ψ2‖X0+‖ψ3‖X0, |
where ψi=(ψi1,ψi2,⋯,ψin)T∈Rn or L1(R+,Rn)(i=1,2,3). In addition, denote X+,Y+ and Z+ as the positive cones of X,Y and Z. We define
X0+=X0⋂X+,Y0+=Y0⋂Y+,Z0+=Z0⋂Z+. |
Under Assumption 1.1 we see that the set
Ω={(S(t),0,i(t,⋅),0,v(t,⋅))∈Z0+|Sk(t)+∫∞0ik(t,a)da+∫∞0vk(t,a)da≤bkμk} |
is invariant, where S=(S1,S2,⋯,Sn)T, i=(i1,i2,⋯,in)T and v=(v1,v2,⋯,vn)T. In the following, we just assume that all the initial values are taken from Ω.
Next, we will show that system (5) has a globally classic solution in Ω. Let b,p,μ,γ(a), and ϵ(a) be diagonal matrixes given by
b=diag(b1,b2,⋯,bn),p=diag(p1,p2,⋯,pn),μ=diag(μ1,μ2,⋯,μn),γ(a)=diag(γ1(a),γ2(a),⋯,γn(a)),ϵ(a)=diag(ϵ1(a),ϵ2(a),⋯,ϵn(a)). | (6) |
Then let us define a linear operator Ai:D(Ai)⊂X→X as
Ai(0ψ)=(0Aiψ)=(0−ddaψ−(μ+γ(a))ψ), |
and
Av(0ψ)=(0Avψ)=(0−ddaψ−(μ+ϵ(a))ψ), |
where D(Aj)={(0,ψ)∈X0|ψ is obsolutely continuous,ψ′∈L1(R+,Rn),ψ(0)=0}, j=i,v. If λ∈ρ(Ai) (ρ(Ai) denotes the resolvent set of Ai), for any initial value (θi,ϕi)T∈X, we have
ψi(a)=e−∫a0[μi+γi(s)+λ]dsθi+∫a0ϕi(s)e−∫as[μi+γi(ξ)+λ]dξds. | (7) |
Similarly, for any λ∈ρ(Av) and any initial value (θv,ϕv)T∈X, we have
ψv(a)=e−∫a0[μi+ϵi(s)+λ]dsθv+∫a0ϕi(s)e−∫as[μi+ϵi(ξ)+λi]dξds. | (8) |
Furthermore, define two nonlinear operators as
Fj(0ψ)=(Bj(ψ)0),j=i,v, |
where Bi(ϕ)=Sn∑j=1∫∞0βj(a)ϕij(a)da and Bv(ϕ)=pϕS. We further define another nonlinear operator
FS(ψ)=b−pψS−Bi(ψi)+∫∞0ϵ(a)ψv(a)da. |
If we set u=(S,(0i),(0v))T∈Z, A=diag(−μ,Ai,Av), and F=(Fs,Fi,Fv), (5) can be rewritten as the following abstract Cauchy problem
du(t)dt=Au(t)+F(u(t)),u(0)=u0∈Ω. | (9) |
Proposition 2.1. There exists a uniquely determined semiflow {U(t)}t≥0 on Z0+ such that, for each u=(S(t),(0i(t,⋅)),(0v(t,⋅)))∈Z0+, there exists a unique continuous map U∈C(R+,Z0+) which is an integral solution of the Cauchy problem (9), that is, for all t≥0,
U(t)u=u0+A∫t0U(s)uds+∫t0F(U(s)u)ds. | (10) |
Proof. By the definition of A and equations (7) and (8), A is dense in part of X0 (see Page 1117, , [22]), and the resolvent operator is bounded. From Proposition 3.2 in, [23], we need only to verify that F satisfies Lipschitz condition. Denote
xk=(ψSk,(0ψik),(0ψvk))T∈Z,ˉxk=(ˉψSk,(0ˉψik),(0ˉψvk))T∈Z, |
and
LSk=max{pk+pk∫∞0ϵk(a)da+bkμkn∑j=1β+kj,bkμkn∑j=1β+kj}. |
By calculation, we have
|FSk(xk)−FSk(ˉxk)|=|−pk(ψSk−ˉψSk)+ψSkn∑j=1∫∞0βkj(a)ψij(a)da−ˉψSkn∑j=1∫∞0βkj(a)ψij(a)da+∫∞0ϵk(a)dapk(ψSk−ˉψSk)|≤pk|ψSk−ˉψSk|+n∑j=1∫∞0βkj(a)ψij(a)da|ψSk−ˉψSk|+ψSkn∑j=1∫∞0βkj(a)|ψij(a)−ˉψij(a)|da+p∫∞0ϵk(a)da|ψSk−ˉψSk)|≤pk|ψSk−ˉψSk|+bkμkn∑j=1β+kj|ψSk−ˉψSk|+bkμkn∑j=1β+kj‖ψij−ˉψij‖L1+pk∫∞0ϵk(a)da|ψSk−ˉψSk)|≤LSk‖x−ˉx‖. | (11) |
Similarly, we have ‖Flk(xk)−Flk(ˉx)‖≤Llk‖x−ˉx‖,l=i,v, where Lik=bkμkn∑j=1β+kj, and Lvk=pk. Therefore, ‖F(x)−F(ˉx)‖≤n∑k=1‖Fk(xk)−F(ˉxk)‖≤L‖x−ˉx‖, where L=max{LSk,Lik,Lvk}.
In order to prove the positivity of the solution of (5), we first rewrite the operator Av and the nonlinear function Fvk as follows:
A′v(0ψ)=(0Avψ)=(0−ddaψ−μψ) |
and
F′v(0ψ)=(pS−∫∞0ϵ(a)ψ(a)da0). |
Denote A′=diag(μ,Ai,A′v), and F′=(FS,Fi,F′v). Then system (9) can be written as
du(t)dt=A′u(t)+F′(u(t)),u(0)=u0. | (12) |
It is obvious that eA′tu0∈Ω+ if u0∈Ω+, where Ω is the positive cone of Ω. Then we need to show that
∫t0eA(t−s)F′k(Ω+)ds∈Ω+. |
For any ψlk∈R and ϕlk∈Ω+, it follows from the definitions of A′ and F′ that
ψ1+ψ2+ψ3=∫t0e−μ(t−s)FS(ϕ(s))ds+∫t0e−μ(t−s)−∫tsγ(a)daFi(ϕ(s))ds+∫t0e−μ(t−s)F′v(ϕ(s))ds≤∫t0bke−μ(t−s)ds=bkμk(1−eμkt)≤bkμk. | (13) |
On the other hand, choose a κk∈R+ and redefine the operator in (12) as follows: Aκk=diag(−(μk+κk),Aiκk,Avκk), where
Aiκk(0ψ)=(0−ddaψ−(μk+γk(a)+κk)ψ), |
and
Avκk(0ψ)=(0−ddaψ−(μk+κk)ψ). |
The nonlinear functions in (12) are defined as Flκk(ϕ)=Flk+κk‖ϕk‖(l=S,i,v) for any ϕ∈Z. Hence, we have
∫t0e−(μk+κk)(t−s)FSκk(ϕk(s))ds=∫t0e−(μk+κk)(t−s)[bk−pkϕSk(s)−ϕSk(s)n∑j=1∫∞0βkj(a)ϕik(t,a)da+∫t0ϵk(a)ϕvk(a)da+κk‖ϕSk‖]ds≥∫t0e−(μk+κk)(t−s)[bk+(κk−pk−n∑k=1β+kj)‖ϕij‖)]ϕSk(s)ds, | (14) |
∫t0e−(μk+κk)(t−s)e−∫s0γk(a)daFiκk(ϕk(s))ds=∫t0e−(μk+κk)(t−s)[ϕSk(s)n∑j=1∫∞0βkj(a)ϕij(t,a)da+κk‖ϕik‖]ds≥∫t0e−(μk+κk)(t−s)[ϕSk(s)ε0+κk]‖ϕik‖ds, | (15) |
and
∫t0e−(μk+κk)(t−s)Fvκk(x)ds=∫t0e−(μk+κk)(t−s)[pkϕSk(s)−∫∞0ϵk(a)ϕvk(s,a)da+κ‖ϕvk‖]ds≥∫t0e−(μk+κk)(t−s)[pkϕSk(s)+(κk−ϵ+k)‖ϕvk‖]ds. | (16) |
If we choose κk>max{pk+n∑j=1β+kj‖ϕij‖,ϵ+k}, it follows from (14)-(16) that
∫t0eAκk(t−s)Fκk(ϕ(s))ds>0,ifϕ>0. |
From what has been discussed above, we have the following result.
Proposition 2.2. If Assumption 1.1 holds, system (5) has a unique positive solution in Ω.
In this section, we show the computation process of the basic reproduction number R0, which is the average number of secondary cases produced by a classical infected individual during its infectious period in a fully susceptible population. Many literatures have given different methods to solve this problem. In this paper, it follows from the renewal process mentioned in Diekman et al in [24] that its value is determined by a next generation operator. Note that E0=(ˆS0,0,0,0,ˆv0(a)) is the disease-free equilibrium of system (5) where ˆS0=(S10,S02,⋯,S0n) and ˆv0(a)=(v01(a),v02(a),⋯,v0n(a)) with S0k=bkμk+pk(1−K1k),v0k(a)=pkS0kπ1k(a), and π1k(a)=e−∫a0(μk+ϵk(s))ds, K1k=∫∞0ϵk(a)π1k(a)da. Linearizing system (5) at the disease-free equilibrium E0, the subsystem (5) can be rewritten as
∂i(t,a)∂t+∂i(t,a)∂a=−(μ+γ(a))i(t,a),i(t,0)=S0Λ(i(t,⋅)), | (17) |
where
Λ(i(t,⋅))=diagj∈N(n∑k=1∫∞0βjk(a)ik(t,a)da), |
and
S0=diag(S01,S02,⋯,S0n). |
Let B:D(B)⊂X→X be a linear operator defined by
B(0ϕ(a))=(−ϕ(0)−ddaϕ(a)−{μ+γ(a)}ϕ(a)),D(B)={ϕ∈X:ϕis absolutely continuous, ϕ′∈L1(R+)}, | (18) |
where μ and γ are defined in (6). Based on the above definition, (7) can be rewritten as the following linear Cauchy problem in Z0:
ddtI(t)=BI(t)+S0ΛI(t),I(0)=I0∈X, | (19) |
Let u(t)=etB be the C0 semigroup generated by the generator B. By the variation of constants formula, we have
I(t)=u(t)I0+∫t0u(t−s)S0ΛI(s)ds. |
Mapping S0Λ on both sides of the above equation yields
v(t)=f(t)+∫t0Ψ(s)v(t−s)ds, | (20) |
where v(t)=S0ΛI denotes the density of newly infectives in the linear invasion phase, f(t)=S0Λu(t)I0 and Ψ(s)=ΛS0u(s). Then the next generation operator is defined by
K=∫∞0Ψ(s)ds=Λ(−S0B)−1, |
where we used the relation (z−B)−1=∫∞0e−zsV(s)ds, for z∈ρ(B)(ρ(B) denotes the resolvent set of B) and 0∈ρ(B). In fact, the next generation operator is calculated as follows:
K=(K1,K2,⋯,Kn)T, | (21) |
where
Kj=n∑k=1S0k∫∞0βjk(a)πk(a)da,j=1,2,⋯,n. | (22) |
Based on Diekmann et al [24], the basic reproduction number R0=r(K) is the spectral radius of the next generation operator K, where r(A) denotes the spectral radius of a bounded operator A. It follows from Inaba [25] that Malthusian parameter or asymptotic growth rate of infectives is positive if R0>1, otherwise it is negative.
In this section, we focus on the existence of the endemic equilibrium E∗ of system (5). It follows from Section 2 that equilibria of system (5) satisfy Au∗+F(u∗)=0. Actually, it satisfies the following equations
0=bk−(μk+pk)S∗k−S∗kλ∗k+∫∞0ϵk(a)v∗k(a)da,di∗k(a)da=−(μk+γk(a))i∗k(a),i∗k(0)=S∗kλ∗k,λ∗k=n∑j=1∫∞0βkj(a)i∗k(a)da,dv∗k(a)da=−(μk+ϵk(a))v∗k(a),v∗k(0)=pkS∗k. | (23) |
From the last two equations of (23), we have
v∗k(a)=pkS∗kπ1k(a),π1k(a)=e−∫a0[μk+ϵk(s)]ds. | (24) |
Substituting (24) into the first equation of (23) yields
S∗k=bkμk+pk(1−K1k)+λ∗k. | (25) |
It follows from the second and the third equations of (23) that
i∗k(a)=i∗k(0)πk(a)=S∗kλ∗kπk(a),πk(a)=e−∫a0[μk+γk(s)]ds. |
Note that
λ∗k=n∑j=1∫∞0βkj(a)i∗j(a)da=n∑j=1i∗j(0)Kkj=n∑j=1bjλ∗jμj+pj(1−K1j)+λ∗jKkj, | (26) |
where Kkj=∫∞0βkj(a)πj(a)da. Hence, we can define a nonlinear operator
H(ϕ):=(H1(ϕ),H2(ϕ),⋯,Hn(ϕ))T∈X,ϕ∈R, | (27) |
where
Hk(ϕ)=n∑j=1bjϕjμj+pj(1−K1j)+ϕjKkj. |
In fact, it follows from (26) that the endemic equilibrium of (23) is a positively nontrivial fixed point of the operator H. Note that the Fréchet derivative of H at ϕ=0 is given by
H′k[0]=limh→0Hk[h]−Hk[0]h=limh→0n∑j=1hbjKkjh(μj+pj(1−K1j)+h)=limh→0n∑j=1bjKkjμj+pj(1−K1j)+h=Kk. |
Let
H′[0]=(ˆH1,ˆH2,⋯,ˆHn), |
where
ˆHk=n∑j=1bjμj+pj(1−K1j)Kkj=n∑j=1S0jKkj=Kk,k=1,2,⋯,n. |
Consequently, H′[0] is equal to the next generation operator K defined by (21).
Next, we show that R0 determines the existence of the positive fixed point of operator H. Under Assumption 1.1, the following lemma holds.
Lemma 4.1. Let K be defined by (21). We have
(a) K is compact.
(b)K is nonsupporting.
Proof. Assume that B0 is an arbitrary bounded subset of R. Then there exists a positive constant c0 such that ‖ϕ‖≤c0 for all ϕ∈B0. Note that
‖K(ϕ)‖=n∑j=1|Kj(ϕ)|≤n∑j=1n∑k=1S0k∫∞0βjk(a)|ϕk(a)|da≤n∑j=1n∑k=1S0kβ+jk∫∞0|ϕk(a)|da=n∑j=1n∑k=1S0kβ+jk‖ϕk‖L1. | (28) |
(28) implies that the operator K is bounded. It follows from Fréchet-Kolmogorov Theorem [31] that the operator K is compact. It is obvious that K is nonsupporting under (ⅲ) or (ⅲ)' of Assumption 1.1.
Lemma 4.1, together with the monotonicity of the operator H with respect to ϕ manifests that the following lemma holds.
Lemma 4.2. Let H be defined (27). H is compact and H(R+) is bounded.
Employing Theorem 4.11 in [26] (Krasnoselskii fixed theorem) and Krein-Rutman Theorem in [27], Lemma 4.2 implies that r(K) is the uqiue eigenvalue of the operator K associated with a positive eigenvector and there is no eigenvector of K associated with eigenvalue 1. As a consequence of Corollary 5.2 in [15], the following result is immediate.
Proposition 4.1. If R0>1, then H has at least one nontrivial fixed point in Z0+.
Corollary 4.1. If R0>1, then system (5) has at least one positive endemic equilibrium E∗=(S∗,0,i∗,0,v∗)∈Z0+, where S∗=(S∗1,S∗2,⋯,S∗n),i∗=(i∗1,i∗2,⋯,i∗n),v∗=(v∗1,v∗2,⋯,v∗n).
In order to determine the uniqueness of the solution of system (23), we define two operators by (ⅱ) and (ⅲ) of Assumption 1.1 as follows:
H+k(ϕ)=n∑j=1bjβ+kjϕjμj+pj(1−K1j)+ϕj∫∞0πj(a)da,k∈N, |
and for a small positive value δ
H−k(ϕ)=n∑j=1bjε0ϕjμj+pj(1−K1j)+ϕj∫aβ+δaβ−δπj(a)da,k∈N. |
For convenience, define
H+(ϕ)=diag(H+1(ϕ),H+2(ϕ),⋯,H+n(ϕ)), |
and
H−(ϕ)=diag(H−1(ϕ),H−2(ϕ),⋯,H−n(ϕ)). |
It is obvious that 0≤H−(ϕ)e≤H(ϕ)e≤H+(ϕ)e, where e=(1,1,⋯,1)T.
Theorem 4.3. If R0>1, then the operator H defined in (27) has at most one nontrivial fixed point in Z0+.
Proof. By Proposition 4.1, we assume that there are two different nontrivial fixed points in R+, denoted by ϕ∗ and ˆϕ∗. Borrowing the definitions of H− and H+, we have
ϕ∗=H(ϕ∗)≥H−(ϕ∗)=H−(ϕ∗)H+(ϕ∗)H+(ϕ∗)≥H−(ϕ∗)H+(ϕ∗)H(ϕ∗)=H−(ϕ∗)H+(ϕ∗)ϕ∗. |
Hence, there exists a positive constant q=sup{r≥0,ϕ∗≥rˆϕ∗}>0. Suppose 0<q<1, for all ϕ∈R+ and k∈N, then
Hk(qϕ)=qHk(ϕ)+ξk(ϕ,q), | (29) |
where ξk(ϕ,q)=q(1−q)n∑j=1bjϕj(μj+pj(1−K1j)+ϕj)(μj+pj(1−K1j)+qϕj)Kkj. It follows from 0<q<1 and (ⅲ) of Assumption 1.1 that ξk is positive for all k∈N+ and ϕ∈R+. Furthermore,
H(qϕ)≥qH(ϕ)+ξ(q,ϕ)e,ξ=diag(ξ1,ξ2,⋯,ξn). | (30) |
Inequality (30), together with the monotonicity of H implies that
ϕ∗=H(ϕ∗)≥qH(ϕ∗)+ξ(ˆϕ∗,q)e=qˆϕ∗+ξ(ˆϕ∗,q){H+(ˆϕ∗)}−1H+(ˆϕ∗)e≥qˆϕ∗+ξ(ˆϕ∗,q){H+(ˆϕ∗)}−1H(ˆϕ∗)=qˆϕ∗+ξ(ˆϕ∗,q){H+(ˆϕ∗)}−1ˆϕ∗. | (31) |
This contradicts the definition of q. Therefore, q≥1 and ϕ∗≥qˆϕ∗≥ˆϕ∗. Exchanging the role of ϕ∗ and ˆϕ∗, we can prove ϕ∗≤ˆϕ∗. This implies that ϕ∗=ˆϕ∗.
In this section, we will show the relative compactness of the positive orbit {U(t,u0)}t≥0 defined by (5). This process is spurred by Lemma 19 in [28] and Theorem 2.46 in [29]. To apply them, we define
˜ϕk(t,a):={0,t>a,ik(t,a),t≤a,˜ψk(t,a):={0,t>a,vk(t,a),t≤a, |
and
˜ik(t,a)=ik(t,a)−˜ϕk(t,a),˜vk(t,a)=vk(t,a)−˜ψk(t,a). |
Then we can divide the solution of semigroup U(t) into two parts:
V(t)u0=(0,0,˜ϕ(t,⋅),0,˜ψ(t,⋅)) | (32) |
and
W(t)u0=(S(t),0,˜i(t,⋅),0,˜v(t,⋅))T | (33) |
where
˜ϕ=(˜ϕ1,˜ϕ2,⋯,˜ϕn)T,˜ψ=(˜ψ1,˜ψ2,⋯,˜ψn)T,˜i=(˜i1,˜i2,⋯,˜in)T,˜v=(˜v1,˜v2,⋯,˜vn)T. |
This implies that U(t)u0=V(t)u0+W(t)u0.
Theorem 5.1. The semiflow U:R+×X0→X0 is asymptotically smooth if there are maps V(t),W(t):R+×X0→X0 such that U(t)u0=V(t)u0+W(t)u0, and the following statements hold for any bounded closed set Ω that is forward invariant under U:
(i) For any u0∈Ω, there exists a function δ:R+×R+→R+ such that for any r>0 limt→+∞δ(t,r)=0 with ‖u0‖Ω≤r, then ‖V(t,u0)‖Ω≤δ(t,r);
(ii) there exists a tΩ≥0 such that W(t)(Ω) has a compact closure for each t≥tΩ.
Lemma 5.2. There exists a function δ:R+×R+→R+ such that for any r>0,
limt→+∞δ(t,r)=0 | (34) |
and
‖V(t)u0‖Ω≤δ(t,r),∀u0∈Z,‖u0‖Ω≤r,t≥0. | (35) |
Proof. Integrating ik and vk equations along the characteristic line t−a= const. yields
˜ϕ(t,a)={0,t>a,ik0(a−t)πk(a)πk(a−t),t≤a,˜ψ(t,a)={0,t>a,vk0(a−t)π1k(a)π1k(a−t),t≤a |
for all k∈N. Hence for any u0∈Y and ‖u0‖Y≤r,
‖V(t)x0‖Ω=‖0‖+‖˜ϕ(t,⋅)‖X+‖˜ψ(t,⋅)‖X=n∑j=1{∫∞tij0(a−t)πj(a)πj(a−t)da+∫∞tvj0(a−t)π1j(a)π1j(a−t)da}≤eμ_tn∑j=1∫∞0[ij0(a)+vj0(a)]da=e−μ_t{‖i0‖X+‖v0‖X}≤e−μ_tr |
where δ(t,r)=e−μ_tr and μ_=mink∈N{μk}. Obviously, ‖V(t)u0‖Ω approaches 0 as t goes to infinity.
Lemma 5.3. W(t) maps any bounded subsets of Ω into sets with compact closure in Y.
Proof. It follows from the first equation of (5) that Sk(t) remains in the compact set {ϕ∈Rn+|0≤ϕk≤bkμk,k∈N} for all t≥0. Therefore, we need to show that ˜i and ˜v still remain in pre-compact subsets of Y0 which is independent of u0. Note that
˜ik(t,a)={ik(t−a,0)πk(a),t>a,0,t≤a,˜vk(t,a)={vk(t−a,0)π1k(a),t>a,0,t≤a. |
Since for all k∈N+, 0≤˜ik(t,a)=ik(t−a,0)πk(a)≤bkμ_2n∑j=1β+kjbje−μ_a:=Δke−μ_a where Δk=bkμ_2n∑j=1β+kjbj, and 0≤˜vk(t,a)≤bkpkμke−μ_a. It is easy to see that (ⅰ) - (ⅲ) of Theorem B.2 in [29] hold.
In what follows, we need to show that (iv) of Theorem B.2 in [29] also holds. Assume that h∈(0,t) without loss of generality. Then
n∑j=1∫∞0|˜ij(t,a+h)−˜ij(t,a)|da=n∑j=1∫tt−h|0−˜ij(t,a)|da+n∑j=1∫t−h0|˜ij(t,a+h)−˜ij(t,a)|da≤n∑j=1Δjh+n∑j=1∫t−h0˜ij(t,a)|π(h)−1|da≤n∑j=1Δjh+n∑j=1Δj(t−h)h=n∑k=1Δjh(1+t−h). | (36) |
Similarly,
n∑j=1∫∞0|˜vj(t,a+h)−˜vj(t,a)|da≤n∑k=1bjpjμjh(1+t−h). | (37) |
Obviously, both (36) and (48) uniformly converge to 0 as h→0 which is independent of u0.
Lemmas 5.2 and 5.3, together with ‖U(t)u0‖Z0≤n∑k=1bkμk, imply that U(t) has compact closure in Z for u0∈Ω. It follows from Proposition 3.13 in [32] that the solution orbit is relatively compact and the semiflow U(t) is asymptotically smooth.
Proposition 5.1. The semiflow U(t) defined in (10) is asymptotically smooth.
In this section, we establish the uniform persistence of (5) when R0>1. This property guarantees the well-definition of the Lyapunov functionals in Section 7. For some k∈N, define ρk:Γ→R+ and u0=(S0,0,i0(⋅),0,v0(⋅))∈Ω0 by
ρk(S(t),0,i(t,⋅),0,v(t,⋅))=λk(t)=n∑j=1∫∞0βkj(a)ij(t,a)daforu0∈Ω. |
Let
Ω0={(S0,0,i0(⋅),0,v0(⋅))∈Ω:ρk(U(t0,u0))>0for somet0∈R+}. |
Obviously, if u0∈Ω∖Ω0, then (S(t),0,i(t,⋅),0,v(t,⋅))→E0 as t→∞. Hence, if U has a global compact attractor in Ω0, then it also has a global compact attractor in Ω.
Assumption 6.1. The support of the initial age-since-infection value ik0(a)∈L1(R+) lies to the right of the support the infectivity function βkj for all k,j∈N.
Proposition 6.1. If R0>1 and Assumption 6.1 hold, then system (5) is uniformly weakly ρ-persistent for some k∈N.
Proof. Since R0>1, there exists an ηj0>0 and ϕj∈R+ for k,j∈N such that
n∑j=1˜S0j(ηj0)ˆKkj(ηj0)ϕj>ϕk, | (38) |
where ˜S0k(ηk0)=bkμ+ηk0+pk(1−K1k)−ηk0(>0) and ^Kkj(⋅)=∫∞0e−λaβkj(a)πk(a)da.
Suppose that, for any ηk0>0, there exists an u0∈Ω0 such that
lim supt→∞ρk(U(t,u0))≤ηk0 |
and show a contradiction. Therefore, there exists a t0∈R+ such that ρk(U(t,u0))≤ηk0 for t≥t0 and all k∈N. Without loss of generality, we shift the time to t0=0. Then λk(t)≤ηk0 for t≥t0=0 and k∈N.
Next, we show that Sk∞≥S0k(ηk0):=bkμk+ηk0+pk(1−K1k), where Sk∞=lim inft→∞Sk(t). By the Fluctuation Lemma [35], we can pick up a sequence {tn} such that Sk(tn)→Sk∞, dSk(tn)dt→0 as n→∞. Then from the first equation of (5), it follows that
dSk(tn)dt≥bk−(μk+pk)Sk(tn)−ηk0Sk(tn)+∫∞0εk(a)v(tn,a)da. |
This, combined with (40), gives
dSk(tn)dt≥bk−(μk+pk)Sk(tn)−ηk0Sk(tn)+∫tn0εk(a)pkSk(tn−a)π1k(a)da. |
Letting n→∞ leads to
0≥bk−(μ+ϕ)Sk∞−ηk0Sk∞+pkSk∞K1k. |
This implies that Sk∞≥S0k(ηk0).
Finally, since Sk∞≥S0k(ηk0), there exists a t1∈R+ such that Sk(t)≥˜S0k(ηk0) for t≥t1. Again, without loss of generality, we can assume t1=0. Solving ik(t,a) by the characteristic line method yields
ik(t,a)={bk(t−a)πk(a),t≥a,ik0(a−t)πk(a)πk(a−t),t<a, | (39) |
where bk(t)=Sk(t)λk(t). Then
λk(t)=n∑j=1∫∞0βkj(a)ij(t,a)da≥n∑j=1∫t0βkj(a)Sj(t−a)λj(t−a)πj(a)da≥n∑j=1˜Sj(ηj0)∫t0βkj(a)πj(a)λj(t−a)da. |
Taking Laplace transforms on both sides of the above inequality gives
^λk(ξ)≥n∑j=1˜Sj(ηj0)^Kk(ξ)^λj(ξ). |
This inequality holds for any ξ and ηk0. If we take ξ and ηk0 small enough, then this is a contradiction with (38). This completes the proof.
Clearly, for all k∈N, ρk is a continuous function on R+. Proposition 5.1 implies that {U}t≥0 has a global attractor. From Theorem A.34 in [34], we need to show that for any bounded total orbit h(t+s)=U(s,u(t)) of Ut such that ρk(h(t))>0 for all t∈R and s∈R+. For the total trajectory, we have
ik(t,a)=bk(t−a)πk(a),vk(t,a)=pSk(t−a)π1k(a) |
for t∈R and a∈R+. In order to prove the strongly uniform persistence, the following lemma is helpful.
Lemma 6.1. Let (S(t),0,i(t,⋅),0,v(t,⋅)) be a solution of (5). Then S∞k≤S0k, where S∞k=lim supt→∞Sk(t) for k∈N.
Proof. By Fluctuate Lemma in [35], there exists {tn} such that tn→∞, Sk(tn)→S∞k, and dSk(tn)dt→0 as n→∞. Integrating vk equation in (5) along the characteristic equation t−a=const., we have
vk(t,a)={pkSk(t−a)π1k(a),t≥a,vk0(a−t)π1k(a)π1k(a−t),t<a,for allk∈N. | (40) |
Substituting vk into Sk equation yields
dSk(tn)dt=bk−(μk+pk)Sk(tn)−Sk(tn)λk(tn)+∫∞0εk(a)vk(tn,a)da≤bk−(μk+pk)Sk(tn)+∫tn0εk(a)pkSk(tn−a)π1k(a)da+∫∞tnεk(a)vk0(a−tn)π1k(a)π1k(a−tn)da≤bk−(μk+pk)Sk(tn)+∫tn0εk(a)pkSk(tn−a)π1k(a)da+∫∞0εk(a+tn)vk0(a)π1k(a+tn)π1k(a)da. |
Applying Fluctuate Lemma, we immediately obtain
0≤bk−(μk+pk)S∞k+pkS∞kK1k |
or S∞k≤bkμk+pk(1−K1k)=S0k as required. This completes the proof.
Lemma 6.2. Let us define Θ(b(t))=n∑k=1bk(t). Suppose that Assumption 6.1 holds, then Θ(b(t)) for total trajectory h(t) is identically zero on R, or it is strictly positive on R.
Proof. Suppose that there exists a t1>0 such that bk(t)=0 for all t≤t1. By the definition of λk, we have
bk(t)=Sk(t)n∑j=1∫∞0βkj(a)ij(t,a)da=Sk(t)n∑j=1∫∞0βkj(a)b(t−a)πj(a)da≤S0kˉβ∫∞0Θ(b(t−a))da=S0kˉβ∫t−t10Θ(b(t−a))da≤S0kˉβ∫t0Θ(b(a))da, | (41) |
where ˉβ=maxj,k∈N{β+kj}. Summing k from 1 to n on both sides of (41) yields
Θ(b(t))≤∫t0Θ(b(a))daS0ˉβ,S0ˉβ=n∑k=1ˉβS0k |
for all t>t1. It follows from Gronwall inequality that Θ(b(t))=0 for all t≥t1.
Suppose there doesn't exist a t1 such that Θ(b(t))=0 for all t≤t1. Thus, there exists a sequence {tm} towards −∞ such that Θ(b(tm))>0 for each m. That means that bk(tm)>0 for each m. Moreover, there exists a sequence am such that ik(tm,am)=ik(tm−am,0)πk(am)>0 for each m. In view of the first equation, with the dissipative property of system (5), we obtain
S′k(t)≥bk−(μk+pk)Sk(t). |
Hence, there exists a positive constant ζ>0 such that Sk(t)>ζ>0 holds for all t∈R. Let bkm(t)=bk(t+t∗m) for each n, where t∗m=tm−am. Recalling equation (41), we arrive at
bkm(t)≥ζ[n∑j=1∫t0βkj(a)bjm(t−a)πj(a)da+˜Θkm(t)]≥ζ[ε0∫t0Θ(bm(t−a))da+˜Θkm(t)], | (42) |
where ε0 is defined in (ⅲ) of Assumption 1.1 and
˜Θkm(t)=n∑j=1∫∞tβkj(a)ijm0(a−t)πj(a)πj(a−t)da. |
Consequently,
Θ(bm(t))≥ζ[ε0n∫t0Θ(bm(a))da+˜Θ(t)],˜Θm(t)=n∑k=1˜Θkm(t). | (43) |
Since ˜Θm(0)=n∑k=1n∑j=1∫∞0βkj(a)ijm0(a)da>0 and ˜Θm(t) is continuous at t=0, it follows from Assumption [29] and Gronwall inequality that Θ(bm(t)) and ˜Θm(t) are positive for sufficiently small t. Furthermore, from Corollary B.6 [29], we conclude that there exists a constant l>0 such that Θ(bm(t))>0 for all t>l. Since Θ(bm(t)) is a time shift of Θ(b(t)) by t∗m with t∗m→−∞ as m→∞, it follows that Θ(b(t))>0 for all t∈R. This completes the proof.
Corollary 6.1. Suppose that Assumption 6.1 holds. Then for all k∈N, bk(t) for the total trajectory h(t) is strictly positive for every t∈R.
From Propositions 5.1 and 6.1, together with Corollary 6.1, we apply Theorem 5.2 in [29] to illustrate the ρ− strongly uniform persistence of system (5).
Lemma 6.3. Suppose that R0>1 and the assumption of Corollary 6.1 hold. Then system (5) persists uniformly strongly, in this sense, there exists some ηk0 such that
lim inft→∞λk(t)≥ηk0 |
for some k∈N and λk(0)≠0.
Proof. From Corollary 6.1, we readily see that infλk(0)>0 for some k∈N. Applying Theorem A.34 in [34], we conclude that there exists a constant ηk0>0 such that lim inft→+∞ρk(U(t,u0))>ηk0.
By Lemma 6.3, we present the following theorem to state the uniformly strong persistence of system (5).
Theorem 6.4. Suppose that R0>1 and Assumption 6.1 hold. There exist some positive constants ηk0>0(k∈N) such that for all t∈R and a∈R+
lim inft→∞Sk(t)≥ηk0,lim inft→∞ik(t,a)≥ηk0πk(a),lim inft→∞vk(t,a)≥ηk0π1k(a). |
In this section, we will show the global behavior of the equilibria of system (5). To achieve this goal, we employ a Volterra type functional defined by g(x)=x−1−lnx in [22], which is positive and attains minimum value 0 at x=1. In what follows, we check this Volterra type functional is well-defined in infinite dimension and make the following assumption.
Assumption 7.1. For all j∈N+, Sj0∈R+, ∫∞0|lnhj0(a)|da<+∞, h=i,v.
Lemma 7.1. If Assumption 7.1 holds, then ∫∞0v0j(a)lnvj(t,a)v0j(a)da is bounded.
Proof. For t>a,
|v0jlnvj(t,a)v0j(a)|=|v0j(a)lnvj(t,a)−v0j(a)lnv0j(a)|≤|v0j(a)lnvj(t,a)|+|v0j(a)lnv0j(a)|=|pjS0jπ1j(a)lnpjS0j(t−a)π1j(a)+|pjS0jπ1j(a)lnpjS0jπ1j(a)|≤2pjS0jlnpjΛjμje−μja+2pjS0je−μjaμja. | (44) |
For t≤a,
|v0jlnvj(t,a)v0j(a)|≤|v0j(a)lnvj(t,a)|+|v0j(a)lnv0j(a)|=|pjS0jπ1j(a)lnvj0(a−t)π1j(t)|+|pjS0jπ1j(a)lnpjS0jπ1j(a)|≤pjS0j|lnvj0(a−t)π1j(t)|+pjS0jlnpjΛjμje−μja+2pjS0je−μjaμja. | (45) |
It follows from (44) - (45) that
∫∞0|v0j(a)lnvj(t,a)v0j(a)|da=∫∞0|v0j(a)lnvj(t,a)−v0j(a)lnv0j(a)|da≤pjS0jlnpjΛjμj∫t0e−μjada+pjS0j∫∞t|lnvj0(a−t)|e−μjtda+pjS0jlnpjΛjμj∫∞0e−μjada+2pjS0j∫∞0ae−μjada=pjS0jlnpjΛjμj(1−e−μjt)+pjS0je−μjt∫∞0|lnvj0(a)|da+pjS0jμjlnpjΛjμj+2pjS0jμj≤2pjS0jlnpjΛjμj+pjS0je−μjt∫∞0|lnvj0(a)|da+2pjS0jμj. | (46) |
Therefore, it follows from Assumption 7.1 that ∫∞0v0j(a)lnvj(t,a)v0j(a)da is bounded.
Theorem 7.2. Let Assumption 7.1 hold. If R0=r(K)<1, the disease-free equilibrium E0 is a global attractor in Ω.
Proof. For j∈N, define
Vj(t)=n∑k=1KjkS0kg(Sk(t)S0k)+n∑k=1∫∞0αjk(a)ik(t,a)da+n∑k=1Kjk∫∞0δk(a)g(vk(t,a)v0k(a))da, |
where αkj(a)=∫∞aβkj(s)πj(s)πj(a)ds and δk(a)=∫∞aεk(s)v0k(s)da. Lemma 7.1 ensures Vj(t) is well-defined. Then
α′kj(a)=−βkj(a)+(μk+ϵk(a))αkj(a),δ′k(a)=−ϵk(a)i0k(a). | (47) |
From Lemma 7.1, together with 6.4, Vj(t) is well-defined. Deviating it along the solution of (5) yields
dVj(t)dt|(5)=n∑k=1{Kjk(1−S0kSk(t))S′k+∫∞0αjk(a)∂ijt(t,a)∂tda+Kjk∫∞0δk(a)∂vk(t,a)∂tda}=n∑k=1[Kjk(1−S0kSk(t))(bk−(μk+pk)Sk−ik(t,0)+∫∞0ϵk(a)vk(t,a)da)]+n∑k=1[Kjkik(t,0)−n∑j=1∫∞0βkj(a)ik(t,a)da]+n∑k=1[Kjk∫∞0ϵk(a)(g(vk(t,0)v0k)−g(vk(t,a)v0k(a)))da]=−n∑k=1[Kjk(μk+pk(1−K1k))(2−Sk(t)S0k−S0kSk(t))]−n∑k=1[Kjk∫∞0ϵk(a)g(vk(t,a)S0kv0k(a)Sk(t))da]+n∑k=1(S0kKjk−1)∫∞0βjk(a)ik(t,a)da. | (48) |
Note that R0=r(K)<1 implies that n∑k=1S0kKjk<1. Therefore, V′j(t)≤0 and it is easy to see that the equality holds if and only if (S(t),0,i(t,⋅),0,v(t,⋅))=(S0,0,0,0,v0(⋅)). This implies that the largest positive invariant subset of {u(t)∈Ω|V′j(t)=0} is the singleton {(S0,0,0,0,v0(⋅))}. This shows that the disease-free equilibrium E0 is a global attractor.
In the following, we give a lemma to show the boundedness of the Lyapunov functional for proving the global attractivity of the endemic equilibrium E∗.
Lemma 7.3. If Assumption 7.1 holds, then ∫∞0h∗j(a)lnhj(t,a)h∗j(a)da (h=i,v) is bounded.
Proof. Prior to this proof, denote β+j=maxk∈Ness.supa∈R+βjk(a) for all j∈N. For t>a, it follows from bj(t)≤β+jΛ2jμ2j that
|i∗j(a)lnij(t,a)i∗j(a)|=|i∗j(a)lnij(t,a)−i∗j(a)lni∗j(a)|≤|i∗j(a)lnij(t,a)|+|i∗j(a)lni∗j(a)|=|i∗j(0)πj(a)lnbj(t−a)πj(a)+|i∗j(0)πj(a)lni∗j(0)πj(a)|≤4(Λjμj)2e−μjaβ+jlnβ+jΛjμj+2(Λjμj)2e−μjaμja. | (49) |
For a>t,
|i∗j(a)lnij(t,a)i∗j(a)|=|i∗j(a)lnij(t,a)−i∗j(a)lni∗j(a)|≤|i∗j(a)lnij(t,a)|+|i∗j(a)lni∗j(a)|=|i∗j(0)πj(a)lnij0(a−t)πj(a)+|i∗j(0)πj(a)lni∗j(a)πj(a)|≤2(Λjμj)2e−μja|lnij0(a−t)|+2(Λjμj)2e−μjaβ+jlnβ+jΛjμj+2(Λjμj)2e−μjaμja. | (50) |
Then,
∫∞0|i∗j(a)lnij(t,a)i∗j(a)|da=∫∞0|i∗j(a)lnij(t,a)−i∗j(a)lni∗j(a)|≤2Λ2jμ3jβ+jlnβ+jΛjμj∫t0e−μjada+2(Λjμj)2∫∞t|lnij0(a−t)|e−μjtda+2Λ2jμ3jβ+jlnβ+jΛjμj∫∞0e−μjada+2(Λjμj)2μj∫∞0ae−μjada=2Λ2jμ3jβ+jlnβ+jΛjμj(1−e−μjt)+2(Λjμj)2∫∞0|lnij0(a)|e−μjtda+2Λ2jμ3jβ+jlnβ+jΛjμj+2Λ2jμ3j≤4Λ2jμ3jβ+jlnβ+jΛjμj+2(Λjμj)2e−μjt∫∞0|lnij0(a)|da+2Λ2jμ3j. | (51) |
By the assumption, it follows that ∫∞0i∗j(a)lnij(t,a)i∗j(a)da is bounded.
Similarly, ∫∞0v∗j(a)lnvj(t,a)v∗j(a)da is also bounded.
Assume that f(t,a)∈R×L1(R+) is a solution of the following system
∂f(t,a)∂t+∂f(t,a)∂a=−m(a)f(t,a),f(t,0)=L(t)∫∞0η(a)f(t,a)da,f(0,a)=f0(a), | (52) |
where m(a),η(a)∈L1(R+) and L(t)∈R. Obviously, the nontrivial equilibrium E∗1 of system (52) satisfies the following equations:
df∗(a)da=−m(a)f∗(a),f∗(0)=L∗∫∞0η(a)f∗(a)da. | (53) |
Define a Lyapunov functional V1(t)=∫∞0M(a)g(f(t,a)f∗(a))da, where M(a)=∫∞aβ(s) f∗(s)ds.
Lemma 7.4. Suppose that ∫∞0|lnf0(a)|da is bounded. There exists a positive value η0>0 such that f(t,a)>η0e−∫a0m(s)ds, then
dV1(t)dt=∫∞0M(a)f∗(a)[g(f(t,0)f∗(0))−g(f(t,a)f∗(a))]da, | (54) |
where M(a)=∫∞aη(s)f∗(s)da.
Proof. Note that
∂g(f(t,a)f∗(a))∂a=∂∂a(f(t,a)f∗(a)−1−lnf(t,a)f∗(a))=∂∂af(t,a)f∗(a)−∂∂alnf(t,a)f∗(a)=f′a(t,a)f∗(a)−f(t,a)f∗a(a)(f∗(a))2−f∗(a)f(t,a)f′a(t,a)f∗(a)−f(t,a)f∗′a(a)(f∗(a))2=(1f∗(a)−1f(t,a))(f′a(t,a)−f(t,a))f∗′a(a)f∗(a)=(1f∗(a)−1f(t,a))f′a(t,a)+(1f∗(a)−1f(t,a))m(a)f(t,a), | (55) |
where we denote h′l(s,l)=∂h(s,l)∂l.
It follows from the proving process of Lemma 7.3, together the assumption of Lemma 7.4 that V1(t) is well-defined. Deviating it along the solution of (52), we obtain
dV1(t)dt|(52)=∫∞0M(a)∂g(f(t,a)f∗(a))∂tda=∫∞0M(a)(1−f∗(a)f(t,a))f′t(t,a)f∗(a)da=∫∞0M(a)(1f∗(a)−1f(t,a))(−f′a(t,a)−m(a)f(t,a))da=−∫∞0M(a)(1f∗(a)−1f(t,a))f′a(t,a)da−∫∞0M(a)m(a)f(t,a)da. | (56) |
Observing (55), we have
dV1(t)dt|(52)=−∫∞0M(a)∂g(f(t,a)f∗(a))∂ada. | (57) |
With the help of integral by parts, we obtain
dV1(t)dt|(52)=−M(a)g(f(t,a)f∗(a))|∞0+∫∞0M′a(a)g(f(t,a)f∗(a))da=M(0)g(f(t,0)f∗(0))−∫∞0η(a)f∗(a)g(f(t,a)f∗(a))da=∫∞0η(a)f∗(a)[g(f(t,0)f∗(0))−g(f(t,a)f∗(a))]da, | (58) |
here we used the fact M(0)=∫∞0η(a)f∗(a)da, and M′(a)=−η(a)f∗(a).
Next, we will give the global attractivity of the endemic equilibrium E∗.
Theorem 7.5. Suppose R0>1, (iv) of Assumption 1.1 and Assumption 7.1 hold. Then the endemic equilibrium E∗ is a global attractor in Ω0.
Proof. Define
V(t)=n∑j=1κj{S∗jg(Sj(t)S∗j)+∫∞0αj(a)g(ij(t,a)i∗j(a))da+∫∞0δj(a)g(vj(t,a)v∗j(a))da}, |
where αj(a)=n∑k=1∫∞aβjk(s)i∗k(s)da and δj(a)=∫∞aεj(s)v∗j(s)ds. κj will be determined later and considered as a weighted coefficient. The well-definition of V(t) follows from Lemma 7.3. From the definitions of αj(a) and δj(a), it follows that
α′j(a)=−n∑k=1βjk(a)i∗k(a), |
and
δ′j(a)=−εj(a)v∗j(a). |
Assisting with Lemma 7.4 and deviating V(t) along the solution of (5) yield
dV(t)dt|(5)=n∑j=1κj{(1−S∗jSj(t))S′j(t)+n∑k=1∫∞0βjk(a)i∗k(a)[g(ij(t,0)i∗j(0))−g(ij(t,a)i∗j(a))]da+∫∞0ϵj(a)v∗j(a)[g(vj(t,0)v∗j(0))−g(vj(t,a)v∗j(a))]da}=n∑j=1κj{(1−S∗jSj(t))[−(μj+pj)(Sj(t)−S∗j)−(Sj(t)n∑k=1∫∞0βjk(a)ik(t,a)da−S∗jn∑k=1∫∞0βjk(a)i∗k(a)da)+∫∞0ϵj(a)(vj(t,a)−v∗j(a))da]+n∑k=1∫∞0βjk(a)i∗k(a)[g(ij(t,0)i∗j(0))−g(ij(t,a)i∗j(a))]da+∫∞0ϵj(a)v∗j(a)[g(vj(t,0)v∗j(0))−g(vj(t,a)v∗j(a))]da}=n∑j=1κj{−(μk+pk)(2−Sk(t)S∗k−S∗kSk(t))+S∗jn∑k=1∫∞0βjk(a)i∗k(a)×[ij(t,0)i∗j(0)−ij(t,a)i∗j(a)−ik(t,a)Sj(t)i∗k(a)S∗j+ik(t,a)i∗k(a)−S∗jSj(t)]da+∫∞0ϵj(a)v∗j(a)[S∗jSj(t)+Sj(t)S∗J−1−S∗jvj(t,a)Sj(t)v∗j(a)+lnS∗jvj(t,a)Sj(t)v∗j(a)]da}. | (59) |
Note that
S∗jn∑k=1∫∞0βjk(a)i∗k(a)ik(t,a)Sj(t)i∗k(a)S∗jda=Sj(t)n∑k=1∫∞0βjk(a)ik(t,a)da=ij(t,0)=S∗jn∑k=1∫∞0βjk(a)i∗k(a)ij(t,0)i∗j(0)da. | (60) |
Equation (60) implies that
S∗jn∑k=1∫∞0βjk(a)i∗k(a)ik(t,a)Sj(t)i∗j(0)i∗k(a)S∗jij(t,0)da=S∗jn∑k=1∫∞0βjk(a)i∗k(a)da. | (61) |
Noting that ∫∞0ϵk(a)v∗k(a)da=pkS∗k∫∞0ϵk(a)π1k(a)da=pkS∗kK1k, it follows from (60) and (61) that
dV(t)dt|(5)=n∑j=1κj{−(μj+pj(1−K1j))(2−Sj(t)S∗j−S∗jSj(t))−S∗jn∑k=1∫∞0βjk(a)i∗k(a)[g(S∗jSj(t))+g(ik(t,a)Sj(t)i∗j(0)i∗k(a)S∗jij(t,0))]da+S∗jn∑k=1∫∞0βjk(a)i∗k(a)[Hk(i(t,a))−Hj(i(t,a))]da−∫∞0ϵj(a)v∗j(a)g(S∗jvj(t,a)Sj(t)v∗j(a))da}, | (62) |
where Hj(i)=ij(t,a)i∗j(a)−lnij(t,a)i∗j(a). Define Θjk=S∗j∫∞0βjk(a)i∗k(a)[Hk(i)−Hj(i)]da, (j,k=1,2,⋯,n) and a Laplacian matrix
Θ=(∑k≠1θ1k−θ21⋯−θn1−θ12∑k≠2θ2k⋯−θn2⋮⋮⋱⋮−θ1n−θ2n⋯∑k≠nθnk). |
By Lemma 2.1 in [21], we have that the solution space of the linear system Θκ=0 is 1 and one of its basis is given by
κ=(κ1,κ2,⋯,κn)T=(c11,c22,⋯,cnn)T, |
where cjj>0(j=1,2,⋯,n) denotes the cofactor of the j−th diagonal element of matrix Θ. This implies that n∑k=1θjkκj=n∑j=1θkjκk and
n∑j=1κjn∑k=1∫∞0βjk(a)i∗k(a)[Hk(i(t,a))−Hj(i(t,a))]da=n∑j=1n∑k=1κk∫∞0βkj(a)i∗j(a)[Hj(i(t,a))−Hk(i(t,a))]da. | (63) |
Employing the graph-theoretic approach mentioned in [21], (63) is equal to zero. Therefore, V′(t)≤0. The equality holds if and only if ik(t,a)i∗k(a)=ij(t,0)i∗j(0)=ij(t,a)i∗j(a). This implies that i=ci∗. It follows from the first equation of (5) with respect to the monotonicity of c that c=1. Hence, the largest invariant set of {u(t)∈Ω0|V′(t)=0} is the singleton E∗. Combining the relative compactness of the solution orbit (see Lemma 5.3) with the invariance principle (see [Theorem 4.2, [30]]), we see that the endemic equilibrium E∗ is a global attractor in Ω0.
In this section, we perform some numerical experiments to illustrate our theoretical results. For the experimental operability, we set amax=10 instead of infinity. For simplicity, we assume system (5) describes some sexually transmission diseases, such as Zika, Ebola and genital warts etc, which consist of two groups - male group and female group. As some news reported in [33], human papillomaviruses (HPV) is an effective and safe vaccine to control some sexually transmitted diseases inducing by virus. In order to illustrate system (5), we firstly fix the demographic parameters as follows:
b1=20.5,b2=40.5,μ1=μ2=0.01. |
Hence the total male population maintains the size N1(t)=2050 and the size of the total female population is N2(t)=4050. It has been reported that nearly half of newly infections are diagnosed as females in age range between 15 to 24. Therefore, we assume that the vaccination rates p1=0.21 for male and p2=0.22 for female. Other parameters are associated with infection age in the form of
y(x,A,B)=1BAΓ(A)xA−1e−xB |
where Γ(n)=(n−1)!, or Γ(z)=∫∞0xz−1e−xdx(z∈N). Obviously, y(x,A,B) satisfies (ⅲ)' of Assumption 1.1. We fix γ(a)=y(a,2,5), ε(a)=y(a,1,1) and verify the transmission rate β(a)=y(a,6,B). If we choose B=9, this implies that the mean of the transmission function is 54 and the variance is 486. It follows from Figure 1 that the disease-free equilibrium E0 is asymptotically stable. Then we decrease B=8 associated with the variance changed as 384. Figure 2 shows that the endemic equilibrium E∗ is asymptotically stable.
Comparing infected male and female populations in Figure 2, we find that the total infected number for female population is larger than that for male population. However, the first peak time for female population is later than the time for male population. Although the vaccination rate for female designed is higher than such rate for male, the level of infected peak and the total number for female population are still larger than those for male population. Consequently, the government should pay more attentions to female population.
In this paper, we proposed a multi-group SIVS epidemic model with age-since-infection. We calculated the basic reproduction number R0 by the renewal equation, which is the spectral radius of the next generation operator K. From Theorems 7.2 and 7.5, we see that the global attractivity of system (5) is totally determined by R0. This implies that the basic reproduction number is a sharp threshold determining that the disease prevails or vanishes. Implicit Euler method performed illustrates the theoretical results. Numerical experiment shows that the number of the infected females is larger than the number of the infected male although the vaccination rate for female group is higher than that for male group. The government should pay much more concerns on female group for suppressing sexual diseases prevalence.
Irreducibility of the transmission matrix βjk,j,k∈N has been highly influential in analyzing the global stability of equilibria. This assumption is still a basic assumption of epidemics spreading on scale free networks. Therefore, we hope our analysis method proving theoretical results and numerical method can be generalized to investigate the dynamics of some epidemic models on complex networks [36]. Besides, oscillation is one of the important phenomena in diseases transmission. What mechanisms resulting in oscillation has been becoming an increasing trend in investigating epidemic models with age-since-infection [37]. We leave this for our future work.
The authors are very grateful to the Editor-in-Chief, Professor Yang Kuang, and the anonymous referees for their valuable comments and suggestions, which helped us to improve the presentation of this work significantly.
This work was supported by the National Natural Science Foundation of China (Nos. 61573016, 11871316), Shanxi Scholarship Council of China (2015-094), Shanxi Scientific Data Sharing Platform for Animal Diseases, and Startup foundation for High-level Personal of Shanxi University.
The authors declare there is no conflict of interest.
[1] | González M, Bagatolli LA, Echabe I, et al. (1997) Interaction of biotin with streptavidin. Thermostability and conformational changes upon binding. J Biol Chem 272: 11288-11294. |
[2] | Wilchek M, Bayer EA (1990) Introduction to avidin-biotin technology. Methods Enzymol 184: 5-13. |
[3] | Kurzban GP, Gitlin G, Bayer EA, et al. (1990) Biotin binding changes the conformation and decreases tryptophan accessibility of streptavidin. J Protein Chem 9: 673-682. |
[4] | Green NM (1990) Streptomyces avidinii. Methods Enzymol 184: 51-67. |
[5] | Delgadillo RF, Mueser TC, Zaleta-Rivera K, et al. (2019) Detailed characterization of the solution kinetics and thermodynamics of biotin, biocytin and HABA binding to avidin and streptavidin. Plos One 14: e0204194. |
[6] | Hyre DE, Stayton PS, Trong IL, et al. (2000) Ser45 plays an important role in managing both the equilibrium and transition state energetics of the streptavidin-biotin system. Protein Sci 9: 878-885. |
[7] | Chilkoti A, Tan PH, Stayton PS (1995) Site-directed mutagenesis studies of the high-affinity streptavidin-biotin complex: Contributions of tryptophan residues 79, 108, and 120. Proc Natl Acad Sci 92: 1754-1758. |
[8] | Klumb LA, Chu V, Stayton PS (1998) Energetic roles of hydrogen bonds at the ureido oxygen binding pocket in the streptavidin-biotin complex. Biochemistry 37: 7657-7663. |
[9] | Qureshi MH, Yeung JC, Wu SC, et al. (2001) Development and characterization of a series of soluble tetrameric and monomeric streptavidin muteins with differential biotin binding affinities. J Biol Chem 276: 46422-46428. |
[10] | Laitinen OH, Nordlund HR, Hytönen VP, et al. (2003) Rational design of an active avidin monomer. J Biol Chem 278: 4010-4014. |
[11] | Diamandis EP, Christopoulos TK (1991) The biotin-(strept) avidin system: principles and applications in biotechnology. Clin Chem 37: 625-636. |
[12] | Sano T, Glazer AN, Cantor CR (1992) A streptavidin-metallothionein chimera that allows specific labeling of biological materials with many different heavy metal ions. Proc Natl Acad Sci 89: 1534-1538. |
[13] | Chaiet L, Miller TW, Tausig F, et al. (1963) Antibiotic MSD-235. II. separation and purification of synergistic components. Antimicrob Agents Chemother 161: 28-32. |
[14] | Green NM (1975) Avidin. Adv Protein Chem 29: 85-133. |
[15] | Kuo TC, Tsai CW, Lee PC, et al. (2015) Revisiting the streptavidin-biotin binding by using an aptamer and displacement isothermal calorimetry titration. J Mol Recognit 28: 125-128. |
[16] | Pérez-Luna VH, O'Brien MJ, Opperman KA, et al. (1999) Molecular recognition between genetically engineered streptavidin and surface-bound biotin. J Am Chem Soc 121: 6469-6478. |
[17] | Lo YS, Simons J, Beebe TP (2002) Temperature dependence of the biotin−avidin bond-rupture force studied by atomic force microscopy. J Phys Chem B 106: 9847-9852. |
[18] | Neish CS, Martin IL, Henderson RM, et al. (2002) Direct visualization of ligand-protein interactions using atomic force microscopy. Br J Pharmacol 135: 1943-1950. |
[19] | Liu F, Zhang JZH, Mei Y (2016) The origin of the cooperativity in the streptavidin-biotin system: A computational investigation through molecular dynamics simulations. Sci Rep 6: 27190. |
[20] | Green N (1966) Thermodynamics of the binding of biotin and some analogues by avidin. Biochem J 101: 774-780. |
[21] | Du X, Li Y, Xia YL, et al. (2016) Insights into protein–ligand interactions: Mechanisms, models, and methods. Int J Mol Sci 17: 1-34. |
[22] | Bouchemal K (2008) New challenges for pharmaceutical formulations and drug delivery systems characterization using isothermal titration calorimetry. Drug Discov Today 13: 960-972. |
[23] | Roselin LS, Lin M-S, Lin PH, et al. (2010) Recent trends and some applications of isothermal titration calorimetry in biotechnology. Biotechnol J 5: 85-98. |
[24] | Velazquez-Campoy A, Ohtaka H, Nezami A, et al. (2004) Isothermal titration calorimetry. Curr Protoc Cell Biol 23: 17.81-17.8.24. |
[25] | Velazquez-Campoy A, Freire E (2006) Isothermal titration calorimetry to determine association constants for high-affinity ligands. Nat Protoc 1: 186-191. |
[26] | Kuo TC, Lee PC, Tsai CW, et al. (2013) Salt bridge exchange binding mechanism between streptavidin and its DNA aptamer--thermodynamics and spectroscopic evidences. J Mol Recognit 26: 149-159. |
[27] | Rajarathnam K, Rosgen J (2014) Isothermal titration calorimetry of membrane proteins-progress and challenges. Biochim Biophys Acta 1838: 69-77. |
[28] | Laemmli UK (1970) Cleavage of structural proteins during the assembly of the head of bacteriophage T4. Nature 227: 680-685. |
[29] | Gill SC, von Hippel PH (1989) Calculation of protein extinction coefficients from amino acid sequence data. Anal Biochem 182: 319-326. |
[30] | Swamy MJ (1995) Thermodynamic analysis of biotin binding to avidin. A high sensitivity titration calorimetric study. Biochem Mol Biol Int 36: 219-225. |
[31] | Privalov PL, Gill SJ (1988) Stability of protein structure and hydrophobic interaction. Adv Protein Chem 39: 191-234. |
[32] | Katz BA (1997) Binding of biotin to streptavidin stabilizes intersubunit salt bridges between Asp61 and His87 at low pH. J Mol Biol 274: 776-800. |
[33] | Hyre DE (2006) Cooperative hydrogen bond interactions in the streptavidin-biotin system. Protein Sci 15: 459-467. |
[34] | Prabhu NV, Sharp KA (2005) Heat capacity in proteins. Annu Rev Phys Chem 56: 521-548. |
[35] | Kauzmann W (1959) Some factors in the interpretation of protein denaturation. Adv Protein Chem 14: 1-63. |
[36] | Huang B, Muy S, Feng S, et al. (2018) Non-covalent interactions in electrochemical reactions and implications in clean energy applications. Phys Chem Chem Phys 20: 15680-15686. |
[37] | Yumura K, Ui M, Doi H, et al. (2013) Mutations for decreasing the immunogenicity and maintaining the function of core streptavidin. Protein Sci 22: 213-221. |
[38] | Sano T, Cantor CR (1990) Expression of a cloned streptavidin gene in Escherichia coli. PNAS 87: 142-146. |
1. | Wei Lv, Hanfei He, Kezan Li, Robust optimal control of a network-based SIVS epidemic model with time delay, 2022, 161, 09600779, 112378, 10.1016/j.chaos.2022.112378 | |
2. | Jing Yang, Shaojuan Ma, Juan Ma, Jinhua Ran, Xinyu Bai, Stochastic Analysis for the Dual Virus Parallel Transmission Model with Immunity Delay, 2024, 1557-8666, 10.1089/cmb.2024.0662 |