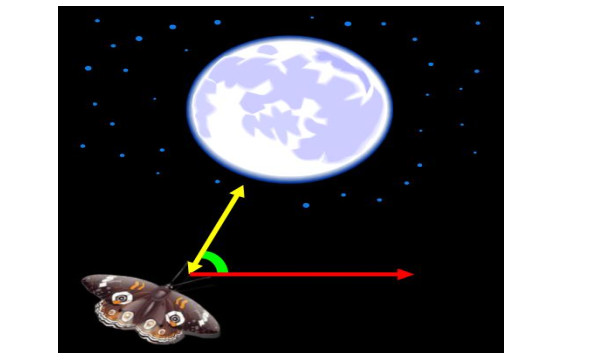
Citation: Thomas Bintsis. Lactic acid bacteria as starter cultures: An update in their metabolism and genetics[J]. AIMS Microbiology, 2018, 4(4): 665-684. doi: 10.3934/microbiol.2018.4.665
[1] | Shikai Wang, Heming Jia, Xiaoxu Peng . Modified salp swarm algorithm based multilevel thresholding for color image segmentation. Mathematical Biosciences and Engineering, 2020, 17(1): 700-724. doi: 10.3934/mbe.2020036 |
[2] | Shikai Wang, Kangjian Sun, Wanying Zhang, Heming Jia . Multilevel thresholding using a modified ant lion optimizer with opposition-based learning for color image segmentation. Mathematical Biosciences and Engineering, 2021, 18(4): 3092-3143. doi: 10.3934/mbe.2021155 |
[3] | Xiaoxu Peng, Heming Jia, Chunbo Lang . Modified dragonfly algorithm based multilevel thresholding method for color images segmentation. Mathematical Biosciences and Engineering, 2019, 16(6): 6467-6511. doi: 10.3934/mbe.2019324 |
[4] | Nilkanth Mukund Deshpande, Shilpa Gite, Biswajeet Pradhan, Ketan Kotecha, Abdullah Alamri . Improved Otsu and Kapur approach for white blood cells segmentation based on LebTLBO optimization for the detection of Leukemia. Mathematical Biosciences and Engineering, 2022, 19(2): 1970-2001. doi: 10.3934/mbe.2022093 |
[5] | Hong Qi, Guanglei Zhang, Heming Jia, Zhikai Xing . A hybrid equilibrium optimizer algorithm for multi-level image segmentation. Mathematical Biosciences and Engineering, 2021, 18(4): 4648-4678. doi: 10.3934/mbe.2021236 |
[6] | Shenghan Li, Linlin Ye . Multi-level thresholding image segmentation for rubber tree secant using improved Otsu's method and snake optimizer. Mathematical Biosciences and Engineering, 2023, 20(6): 9645-9669. doi: 10.3934/mbe.2023423 |
[7] | Yating Fang, Baojiang Zhong . Cell segmentation in fluorescence microscopy images based on multi-scale histogram thresholding. Mathematical Biosciences and Engineering, 2023, 20(9): 16259-16278. doi: 10.3934/mbe.2023726 |
[8] | Jiande Zhang, Chenrong Huang, Ying Huo, Zhan Shi, Tinghuai Ma . Multi-population cooperative evolution-based image segmentation algorithm for complex helical surface image. Mathematical Biosciences and Engineering, 2020, 17(6): 7544-7561. doi: 10.3934/mbe.2020385 |
[9] | Yijun Yin, Wenzheng Xu, Lei Chen, Hao Wu . CoT-UNet++: A medical image segmentation method based on contextual transformer and dense connection. Mathematical Biosciences and Engineering, 2023, 20(5): 8320-8336. doi: 10.3934/mbe.2023364 |
[10] | Xueyuan Li, MiaoYu, Xiaoling Zhou, Yi Li, Hong Chen, Liping Wang, Jianghui Dong . A method of ultrasound diagnosis for unilateral peripheral entrapment neuropathy based on multilevel side-to-side image contrast. Mathematical Biosciences and Engineering, 2019, 16(4): 2250-2265. doi: 10.3934/mbe.2019111 |
Image segmentation is an important connection from image handling to image investigation, and the intention is to segment a provided image into multiple and unique regions and extricate the objects of interest according to characteristic, color, grain, histogram, grayscale and margin [1,2,3,4,5]. The quality of image segmentation is critical for the accuracy of target feature extraction and target detection, and the processing quality required in image investigation, target identification and machine vision is high. The main image segmentation strategies include thresholding, region, edge, clustering and graphs [6,7,8,9,10]. Compared with other image segmentation strategies, thresholding has the advantages of simple operation, high computational productivity, small capacity space and strong robustness, which have been utilized to illuminate image segmentation. Many algorithms have been applied to settle image segmentation, including the bat algorithm (BA) [11], flower pollination algorithm (FPA) [12], moth swarm algorithm (MSA) [13], particle swarm algorithm (PSO) [14] and water wave optimization (WWO) [15].
Yan et al. designed an improved water wave optimization algorithm to solve the underwater image segmentation, the proposed algorithm had a better segmentation performance [16]. Li et al. presented a fuzzy c-means method to clarify the image segmentation, the algorithm was practical and efficacious [17]. Bao et al. introduced an alternative crossover algorithm to illuminate the color image segmentation, the proposed algorithm had a better segmentation effect [18]. Gao et al. conducted a study on an improved artificial bee colony algorithm based on the Otsu segmentation method for multi-level threshold image segmentation, the results have demonstrated the productiveness and feasibleness of the method [19]. Akay et al. combined the particle swarm optimization with the artificial bee colony algorithm for multilevel thresholding, the mixed algorithm contained higher calculation precision and better segmentation effect [20]. Pare et al. proposed the cuckoo search algorithm to puzzle out the color image thresholding, the proposed algorithm produced a high feature of the segmented images [21]. Lu et al. designed a neutrosophic C-means clustering method to explain the image segmentation, the algorithm had an excellent effect [22]. Galdran et al. presented a red channel approach to resolve the underwater image restoration, the method handled smoothly falsely enlightened zones and achieved a characteristic color adjustment [23]. Vasamsetti et al. proposed a variational improvement mechanism to unravel the underwater image, the algorithm captured the better segmentation result [24]. Bohat et al. proposed novel thresholding heuristic algorithms to settle the multilevel thresholding of images, these algorithms obtained better fitness value and segmentation effect [25]. Ouadfel et al. applied the blended method to resolve the multilevel thresholding, the proposed algorithm was powerful and achievable [26]. Pare et al. designed the Lévy flight firefly algorithm to perform the color image segmentation, the algorithm had better optimization execution in terms of distinctive constancy parameters and estimation time [27]. Satapathy et al. combined the chaotic bat algorithm with the Otsu method to clarify the image thresholding, the algorithm obtained the optimum thresholds [28]. Emberton et al. recommended a novel algorithm to improve permeability by recognizing and portioning image districts, and the algorithm achieved better optimization execution [29]. Sambandam et al. introduced a self-adaptive dragonfly algorithm to perform the digital image multilevel segmentation, the algorithm gained the threshold values [30]. Sun et al. presented a novel compound algorithm to settle the multi-level thresholding, the proposed algorithm significantly reduced the computational complexity of the given segmented images [31]. Díaz-Cortés et al. employed the dragonfly algorithm to calculate the best thresholds of the segmented image, and the proposed algorithm provided a highly reliable clinical decision support [32]. Shen et al. proposed an adjusted flower pollination algorithm to multi-level image thresholding, the results illustrated the superiority of the algorithm in terms of image quality measures, fitness values and convergence calculation [33]. Hao et al. designed a variational pattern to solve the underwater image restoration and evaluate the effectiveness and robustness [34]. Zhou et al. proposed the moth swarm algorithm to optimize the thresholds and obtain a better segmentation effect [35]. Kalyani et al. introduced an exchange market algorithm to solve the image segmentation, and the results indicate that the proposed algorithm balances exploration and exploitation to find the global threshold values [36]. Duan et al. used a modified cuckoo search algorithm to solve the multilevel thresholding image segmentation, and the proposed algorithm had strong robustness and stability to obtain the best results [37]. Elaziz et al. combined the improved volleyball premier league algorithm with the whale optimization algorithm for image segmentation, and the hybrid algorithm had the better segmentation accuracy [38]. Li et al. tried to solve the fuzzy multilevel image segmentation using an improved coyote optimization algorithm, and the proposed algorithm had better threshold levels and segmentation effect [39]. Luo et al. applied the enhanced moth swarm algorithm to address the global optimization problem, and the proposed algorithm had a fast convergence speed and higher calculation accuracy [40]. Li et al. presented Lévy-flight moth-flame algorithm to solve the function optimization and engineering design problems, and the proposed algorithm balanced exploration and exploitation to obtain better optimization results [41]. Wang et al. designed eight complex-valued encoding metaheuristic optimization algorithms to solve the function optimization and engineering optimization problems, and the superiority of the algorithms has been proved [42].
Moth-flame optimization (MFO) simulates the transverse orientation navigation mechanism of moths and overcomes premature convergence to attain the global extremum in the optimization area [43]. MFO has some advantages of simple operation, easy implementation, few adjustment parameters, high search efficiency and strong robustness. MFO has a fast convergence speed and high calculation accuracy. MFO based on Kapur's entropy strategy is utilized to achieve multilevel thresholding image segmentation. MFO can efficiently utilize exploration and exploitation to obtain a better segmentation effect and convergence precision. Ten test images are applied to evaluate the overall segmentation performance of the proposed algorithm. To prove the productivity and practicability of the MFO, the MFO is compared with BA, FPA, MSA, PSO and WWO. The experimental results show that MFO consumes less execution time to obtain a better optimization effect, and MFO is an efficient algorithm.
The remaining sections are as follows: Section 2 provides multilevel thresholding. Section 3 surveys MFO. Section 4 describes the MFO-based multilevel threshold method. Section 5 gives the experimental results and analysis. Finally, conclusions and future research are drawn in Section 6.
The bilevel thresholding method separates an allotted image into foreground and background and can only effectively process a simple image that contains an object. However, this is a poor choice to handle a complex image that contains multiple objects. Therefore, the multilevel thresholding method replaces the bilevel thresholding method to perform image segmentation and obtains the best threshold values in the solution space. Kapur's entropy is a valuable and feasible metric for multilevel thresholding segmentation. Kapur's entropy divides the image into different classes, and the size of the entropy determines whether the category is uniform. Kapur's entropy has a low calculation process, easy implementation, strong stability, fast processing speed and high segmentation accuracy. The entropy of the original image is compactness and separateness between different classes. Kapur's entropy finds the best threshold values by maximizing the fitness value. The selection of the segmentation threshold for MFO is manual. Gao et al. designed an effective genetic first-order statistical image segmentation method to solve quantitative crack detection. The proposed method can automatically determine the best statistical feature and threshold selection [44]. Assuming that $ [{t_1}, {t_2}, ..., {t_n}] $ are the excellent threshold values, an image is separated into diverse classes [45]. The formula is described as
${p_i} = \frac{{{h_i}}}{{\sum\nolimits_{i = 0}^{L - 1} {h(i)} }}$ | (1) |
where $ {h_i} $ denotes the number of pixels, $ i $ denotes gray level, and $ L $ denotes the number of levels.
$f({t_1}, {t_2}, ..., {t_n}) = {H_0} + {H_1} + {H_2} + ... + {H_n}$ | (2) |
where
${H_0} = - \sum\limits_{i = 0}^{{t_1} - 1} {\frac{{{p_i}}}{{{\omega _0}}}} \ln \frac{{{p_i}}}{{{\omega _0}}}, {\kern 1pt} {\kern 1pt} {\kern 1pt} {\kern 1pt} {\kern 1pt} {\kern 1pt} {\kern 1pt} {\kern 1pt} {\omega _0} = \sum\limits_{i = 0}^{{t_1} - 1} {{p_i}} $ | (3) |
${H_1} = - \sum\limits_{i = {t_1}}^{{t_2} - 1} {\frac{{{p_i}}}{{{\omega _1}}}} \ln \frac{{{p_i}}}{{{\omega _1}}}, {\kern 1pt} {\kern 1pt} {\kern 1pt} {\kern 1pt} {\kern 1pt} {\kern 1pt} {\kern 1pt} {\kern 1pt} {\omega _1} = \sum\limits_{i = {t_1}}^{{t_2} - 1} {{p_i}} $ | (4) |
${H_2} = - \sum\limits_{i = {t_2}}^{{t_3} - 1} {\frac{{{p_i}}}{{{\omega _2}}}} \ln \frac{{{p_i}}}{{{\omega _2}}}, {\kern 1pt} {\kern 1pt} {\kern 1pt} {\kern 1pt} {\kern 1pt} {\kern 1pt} {\kern 1pt} {\kern 1pt} {\omega _2} = \sum\limits_{i = {t_2}}^{{t_3} - 1} {{p_i}} $ | (5) |
${H_n} = - \sum\limits_{i = {t_n}}^{L - 1} {\frac{{{p_i}}}{{{\omega _n}}}} \ln \frac{{{p_i}}}{{{\omega _n}}}, {\kern 1pt} {\kern 1pt} {\kern 1pt} {\kern 1pt} {\kern 1pt} {\kern 1pt} {\kern 1pt} {\kern 1pt} {\omega _n} = \sum\limits_{i = {t_n}}^{L - 1} {{p_i}} $ | (6) |
$ {H_0}, {H_1}, ..., {H_n} $ denote the Kapur's entropies of the distinct classes, and $ {\omega _0}, {\omega _1}, ..., {\omega _n} $ denote the probabilities of each class.
The unique flight approach of moths at night is called transverse orientation. In MFO, the moths are regarded as a candidate solution, and the positions are regarded as the variables. The moth maintains a fixed flight angle relative to the moon, which is a powerful flight strategy that travels long distances along a straight line. Due to the presence of artificial light sources in nature, the flames are regarded not only as the optimal solutions but also as flags or pins in the search area. The position of each moth is refreshed by the logarithmic spiral movement formula. The transverse orientation is given in Figure 1, and the spiral flying path is given in Figure 2. The corresponding relationship between the algorithm's description and moth-flame's elements is given in Table 1.
Algorithm's description | Moth-flame's elements |
Decision variable | Moth's position in each dimension |
Solutions | Moth's position |
Initial solutions | Random positions of moths |
Current solutions | Current positions of moths |
New solutions | New positions of moths |
Best solution | Flame's position |
Fitness function | Distance between moth and flame |
Process of generating a new solution | Flying in a spiral path toward a flame |
The moths fly in one-dimensional, two-dimensional, three-dimensional or n-dimensional space. MFO is a population-based optimization algorithm, the formula is described as
$M = \left[ {m1,1m1,2⋯⋯m1,dm2,1m2,2⋯⋯m2,d⋮⋮⋮⋮⋮mn,1mn,2⋯⋯mn,d } \right]$
|
(7) |
where $ n $ denotes the number of moths and $ d $ denotes the number of dimensions.
The target values of the moths are sorted by an array.
$OM = \left[ {OM1OM2⋮OMn } \right]$
|
(8) |
The flames are the core component of the MFO, and its matrix is similar to the moth matrix.
$F = \left[ {F1,1F1,2⋯⋯F1,dF2,1F2,2⋯⋯F2,d⋮⋮⋮⋮⋮Fn,1Fn,2⋯⋯Fn,d } \right]$
|
(9) |
The target values of the flames are sorted by an array.
$OF = \left[ {OF1OF2⋮OFn } \right]$
|
(10) |
Moths and flames are selected as candidate solutions. The main distinction is that the position update method is different during the iteration process. The moths are chosen as the actual search agents to move in the search area. The flames are chosen as flags or pins that crashed by moths, which is the best solution currently obtained by the moths. Therefore, the moths perform a global search around the flames until the moths find the optimal positions. With the flight mechanism, the moths never lose their best solutions.
The framework of MFO contains three-tuple approximation functions to realize the best solution. The location is described as
$MFO = (I, P, T)$ | (11) |
where $ I $ denotes a randomly generated moth swarm and the target function $ (I:\phi \to \left\{ {M, OM} \right\}) $, $ P $ denotes the central function of the moth $ (P:M \to M) $, and $ T $ denotes the end of the search $ (T:M \to true, false) $. The $ I $ is used to implement the random distribution.
$M(i, j) = (ub(i) - lb(i)) * rand() + lb(i)$ | (12) |
where $ lb $ and $ ub $ denote the lower and upper bounds of the search space, respectively. In MFO, the moths not only use the transverse orientation to fly but also adopt a logarithmic spiral function to update the flight mechanism.
The logarithmic spiral function is described as
$S({M_i}, {F_j}){\rm{ = }}{D_i} \cdot {e^{bt}} \cdot (\cos 2\pi t) + {F_j}$ | (13) |
where $ {D_i} $ denotes the length between the i-th moth and the j-th flame, $ b $ denotes a fixed value of the logarithmic spiral, and $ t $ denotes an arbitrary value in [-1, 1].
$ D $ is described as
${D_i}{\rm{ = }}\left| {{F_j} - {M_i}} \right|$ | (14) |
where $ {M_i} $ denotes the i-th moth and $ {F_j} $ denotes the j-th flame.
In MFO, $ n $ different positions are used to update the positions of the moths, which will reduce the exploitation of the MFO. Therefore, decreasing the number of flames is beneficial to tackle this issue. The formula is described as
$flame{\kern 1pt} {\kern 1pt} {\kern 1pt} {\kern 1pt} no = round(N - l * \frac{{N - 1}}{T})$ | (15) |
where $ N $ denotes the number of flames, $ l $ denotes the current number of iterations, and $ T $ denotes the maximum number of iterations.
The MFO is shown in Algorithm 1.
Algorithm 1. MFO. |
Randomly initialize the position of moths and the parameters for Moth-flame |
While $iteration \le Max\_iteration$ do |
Renew flame no utilizing Eq (15) |
$OM = FitnessFunction(M)$ |
If $iteration =1$ then |
$F = sort(M)$ |
$OF = sort(OM)$ |
else |
$F = sort({M_{t - 1}}, {M_t})$ |
$OF = sort({M_{t - 1}}, {M_t})$ |
end if |
for $i=1: n$ do |
for $j=1: d$ do |
Renew $ r $ and $ t $ |
Estimate $ D $ utilizing Eq (14) |
Renew $ M(i, j) $ utilizing Eq (13) |
end for |
end for |
end while |
Return the best solution |
The position of each moth denotes the threshold value of the segmented image. The moth revises the position to determine the global optimal solution by the threshold level. The correspondence between the threshold segmentation and MFO is given in Table 2. The MFO-based Kapur's entropy is shown in Algorithm 2. The flowchart of MFO for multilevel threshold is shown in Figure 3.
Threshold segmentation | MFO |
A collection $ ({x_1}, {x_2}, ..., {x_k}) $ scheduling schemes | A moth population $ ({n_1}, {n_2}, ..., {n_k}) $ moths |
An optimal scheme to resolve the image segmentation | An optimal flame's position |
The objective function of the image segmentation | The fitness function of the MFO |
Algorithm 2. MFO-based Kapur entropy. |
Randomly initialize the position of moths and the parameters for Moth-flame |
While $iteration \le Max\_iteration$ do |
Renew flame no utilizing Eq (15) |
Estimate the fitness value of each moth utilizing Eq (2) for the Kapur entropy and attain the best solution |
$OM = FitnessFunction(M)$ |
If $iteration = 1$ then |
$F = sort(M)$ |
$OF = sort(OM)$ |
else |
$F = sort({M_{t - 1}}, {M_t})$ |
$OF = sort({M_{t - 1}}, {M_t})$ |
end if |
for $i = 1:n$ do |
for $j = 1:d$ do |
Renew $ r $ and $ t $ |
Estimate $ D $ utilizing Eq (14) |
Renew $ M(i, j) $ utilizing Eq (13) |
end for |
end for |
end while |
Return the best solution or the optimal threshold value |
In this section, the time and spatial complexity of the MFO are studied.
The time complexity of MFO is based on the calculation workload and operational structure of the algorithm, which is used as an important indicator to estimate the optimization efficiency. In MFO, $ n $ denotes the number of moths, $ t $ denotes the maximum number of iterations, $ d $ denotes the number of variables, and the sorting mechanism of flames in each iteration. MFO uses the quicksort method, the best time complexity of MFO is $ O(n\log n) $, and the worst time complexity of MFO is $ O({n^2}) $. Therefore, the total time complexity of MFO is described as
$O(MFO) = O(t(O(Quick{\kern 1pt} {\kern 1pt} {\kern 1pt} sort) + O(position{\kern 1pt} {\kern 1pt} {\kern 1pt} update)))$ | (16) |
$O(MFO) = O(t({n^2} + n \times d)) = O(t{n^2} + tnd)$ | (17) |
The spatial complexity denotes the storage space that requires to be executed by an algorithm. The total spatial complexity of MFO is $ O(t{n^2} + tnd) $, which is valuable and achievable.
The numerical test is conducted on a computer with a 2.2 GHz Intel Core i7-8750H processor and 8 GB of RAM using MATLAB R2018(b).
Image segmentation is an effective method for character extraction and object identification recognition. It is also an important step from image handling to image investigation. Image segmentation segments a provided preprocessed image to obtain a more intuitive target and background. The experiments select ten images to detect the overall segmentation effect, and they are given in Figure 4.
To prove the effectiveness and practicability, the MFO is compared with BA, FPA, MSA, PSO and WWO. These control parameters are some representative empirical values and are derived from the original articles. The parameters of all algorithms are given in Table 3.
Algorithm | Parameter | Value |
BA [11] | Pulse frequency scope $ f $ | [0, 2] |
Echo loudness $ A $ | 0.25 | |
Reducing coefficient $ \gamma $ | 0.5 | |
FPA [12] | Switch probability $ \rho $ | 0.8 |
MSA [13] | An arbitrary value $ \theta $ | [-2, 1] |
An arbitrary value $ {\varepsilon _2} $ | [0, 1] | |
An arbitrary value $ {\varepsilon _3} $ | [0, 1] | |
An arbitrary value $ {r_1} $ | [0, 1] | |
An arbitrary value $ {r_2} $ | [0, 1] | |
PSO [14] | Constant inertia $ \omega $ | 0.3 |
First acceleration coefficient $ {c_1} $ | 1.4962 | |
Second acceleration coefficient $ {c_2} $ | 1.4962 | |
WWO [15] | Wavelength $ \lambda $ | 0.5 |
Wave height $ {h_{\max }} $ | 6 | |
Wavelength attenuation factor $ \alpha $ | 1.0026 | |
Breaking factor $ \beta $ | [0.001, 0.25] | |
Maximum value $ {k_{\max }} $ of breaking directions | $ min(12, {D \mathord{\left/ {\vphantom {D 2}} \right. } 2}) $ | |
MFO [43] | Logarithmic spiral $ b $ | 1 |
An arbitrary value $ t $ | [-1, 1] |
Five important indicators are used to estimate the image segmentation effect of different algorithms as follows:
1) Fitness value. The fitness value shows the calculation precision of each algorithm. The fitness value is proportional to the segmented image information.
2) Execution time. The algorithm consumes less time, which means that the algorithm has a faster calculation process.
3) Peak signal-to-noise ratio (PSNR). The PSNR based on the intensity value is used to measure the variation between the processed image and the reference image. A larger PSNR indicates that the processed image has less distortion. The image segmentation effect of the higher PSNR may be lower than the image segmentation effect of the lower PSNR. The PSNR is described as [46]
$PSNR = 10{\log _{10}}\left( {\frac{{{{255}^2}}}{{MSE}}} \right)$ | (18) |
where MSE denotes the mean squared error. It is described as follows:
$MSE = \frac{1}{{MN}}{\sum\limits_{i = 1}^M {\sum\limits_{j = 1}^N {\left[ {I\left( {i, j} \right) - K\left( {i, j} \right)} \right]} } ^2}$ | (19) |
where $ M $ and $ N $ denote the size of the provided image and the processed image, respectively.
4) Structure similarity index (SSIM). The SSIM is a similarity measure between the provided image and the processed image. If the SSIM is close to 1, then the image segmentation result is better. The SSIM is described as [47]
$SSIM(x, y) = \frac{{(2{\mu _x}{\mu _y} + {c_1})(2{\sigma _{xy}} + {c_2})}}{{(\mu _x^2 + \mu _y^2 + {c_1})(\sigma _x^2 + \sigma _y^2 + {c_2})}}$ | (20) |
where for the provided image and the processed image, $ {\mu _x} $ and $ {\mu _y} $ denote the mean intensity. $ \sigma _x^2 $ and $ \sigma _y^2 $ denote the standard deviation. $ {\sigma _{xy}} $ denotes the covariance. $ {c_1} $ and $ {c_2} $ denote constants.
5) Wilcoxon's rank-sum test is utilized to identify whether there is a noteworthy distinction between the two algorithms [48]. There is a noteworthy distinction if the p value is lower than 0.05. There is no noteworthy distinction if the p value is higher than 0.05.
To ensure the segmentation effect of the MFO, the population size of each algorithm is set to 30, the maximum number of iterations is set to 100, and the number of independent runs is set to 30. The threshold levels are defined as 2, 3, 4, 5 and 6. The effectiveness and feasibility of the MFO are verified by comparing it with other algorithms. The experimental results of the comparison algorithms are given in Tables 4-9, and the experimental results of some given segmented images are given in Figures 5-14.
Images | k | Fitness values | ||||||
BA | FPA | MSA | PSO | WWO | MFO | Rank | ||
Test 1 | 2 | 12.3364 | 12.3198 | 12.2127 | 12.3479 | 12.3112 | 12.3487 | 1 |
3 | 15.2194 | 15.3081 | 15.2704 | 15.2018 | 15.1970 | 15.3172 | 1 | |
4 | 17.7102 | 17.7705 | 17.8821 | 17.7208 | 17.8752 | 17.9921 | 1 | |
5 | 20.3813 | 20.0295 | 20.2961 | 21.1111 | 20.2899 | 21.2798 | 1 | |
6 | 22.4683 | 22.3940 | 21.9694 | 22.4280 | 22.4488 | 22.6422 | 1 | |
Test 2 | 2 | 12.5842 | 12.5331 | 12.5997 | 12.6346 | 12.5916 | 12.6346 | 1 |
3 | 15.6189 | 15.5130 | 15.6003 | 15.4587 | 15.4813 | 15.6531 | 1 | |
4 | 18.1117 | 18.3233 | 18.1107 | 18.3061 | 18.2180 | 18.4397 | 1 | |
5 | 20.5250 | 20.9349 | 20.9719 | 20.9611 | 20.9605 | 21.0539 | 1 | |
6 | 23.1116 | 23.3068 | 23.3549 | 23.2826 | 23.2950 | 23.3837 | 1 | |
Test 3 | 2 | 12.1651 | 12.1623 | 12.1384 | 12.2017 | 12.0067 | 12.2061 | 1 |
3 | 15.3462 | 15.3160 | 14.9498 | 15.2155 | 15.1669 | 15.5039 | 1 | |
4 | 17.9605 | 18.1165 | 18.0610 | 17.8602 | 17.7274 | 18.3107 | 1 | |
5 | 20.0693 | 20.3889 | 20.6938 | 20.7448 | 20.0828 | 20.8056 | 1 | |
6 | 22.2665 | 22.6040 | 22.7055 | 23.0135 | 22.2510 | 24.1228 | 1 | |
Test 4 | 2 | 11.6162 | 11.0138 | 11.6151 | 11.6170 | 11.5649 | 11.6170 | 1 |
3 | 14.4375 | 14.1046 | 14.4277 | 14.4640 | 14.4074 | 14.6363 | 1 | |
4 | 17.1661 | 17.1351 | 17.2543 | 17.2182 | 16.5648 | 17.5047 | 1 | |
5 | 19.3315 | 19.0672 | 19.5998 | 19.9435 | 19.4292 | 20.0589 | 1 | |
6 | 22.1115 | 21.7571 | 21.8045 | 21.9815 | 21.7166 | 22.1257 | 1 | |
Test 5 | 2 | 12.5987 | 9.17771 | 12.4965 | 12.6016 | 12.5880 | 12.6016 | 1 |
3 | 15.8446 | 15.6981 | 15.6613 | 15.7945 | 15.6387 | 15.9201 | 1 | |
4 | 18.6330 | 18.5687 | 18.6837 | 18.7647 | 18.4739 | 18.8308 | 1 | |
5 | 21.5729 | 21.4283 | 21.6418 | 21.3651 | 21.5618 | 21.6809 | 1 | |
6 | 24.1845 | 24.0339 | 24.0773 | 23.7893 | 23.9359 | 24.3074 | 1 | |
Test 6 | 2 | 12.9609 | 12.9185 | 12.9393 | 12.9682 | 12.9668 | 12.9682 | 1 |
3 | 15.9835 | 16.0781 | 16.0526 | 16.1034 | 16.1034 | 16.1252 | 1 | |
4 | 18.5746 | 18.7090 | 18.7443 | 18.6889 | 18.7069 | 19.0165 | 1 | |
5 | 21.3011 | 21.1524 | 21.2343 | 21.0410 | 20.8741 | 21.4711 | 1 | |
6 | 24.1403 | 23.8479 | 23.9266 | 23.7607 | 24.0573 | 24.1842 | 1 | |
Test 7 | 2 | 12.5779 | 12.3517 | 12.5367 | 12.5936 | 12.3939 | 12.5936 | 1 |
3 | 15.3831 | 15.4011 | 15.3516 | 15.3938 | 15.0022 | 15.4274 | 1 | |
4 | 18.3603 | 18.0115 | 17.8258 | 17.9950 | 18.2520 | 18.4235 | 1 | |
5 | 20.9092 | 20.9076 | 20.6714 | 20.5292 | 20.7942 | 21.0217 | 1 | |
6 | 22.8604 | 23.3690 | 23.1421 | 22.9799 | 23.0124 | 23.5484 | 1 | |
Test 8 | 2 | 12.8868 | 12.8947 | 12.8930 | 12.9208 | 12.8957 | 12.9208 | 1 |
3 | 15.8248 | 15.9079 | 15.8297 | 15.8527 | 15.8992 | 16.0552 | 1 | |
4 | 18.5661 | 18.7317 | 18.6622 | 18.7469 | 18.5391 | 19.0449 | 1 | |
5 | 21.2946 | 21.1664 | 21.1186 | 21.1555 | 21.5266 | 21.5719 | 1 | |
6 | 23.5705 | 23.8786 | 24.2027 | 23.8717 | 23.6695 | 24.3206 | 1 | |
Test 9 | 2 | 11.9271 | 11.8657 | 11.4150 | 11.9285 | 11.7951 | 11.9286 | 1 |
3 | 14.7762 | 14.7811 | 14.7560 | 14.8629 | 14.7074 | 14.9331 | 1 | |
4 | 17.1365 | 17.2515 | 17.1703 | 17.3163 | 17.3681 | 17.4062 | 1 | |
5 | 19.8132 | 19.5984 | 19.8790 | 19.7153 | 19.8422 | 19.8973 | 2 | |
6 | 21.9515 | 22.0255 | 22.1325 | 21.8282 | 21.9971 | 22.2318 | 1 | |
Test 10 | 2 | 11.5248 | 11.4683 | 11.4326 | 11.5288 | 11.4273 | 11.5288 | 1 |
3 | 14.3849 | 13.9338 | 14.1944 | 14.4104 | 14.3475 | 14.5453 | 1 | |
4 | 16.8579 | 17.2591 | 17.3456 | 17.3302 | 17.2842 | 17.3904 | 1 | |
5 | 19.3943 | 19.2793 | 18.9721 | 19.3799 | 19.3881 | 19.8757 | 1 | |
6 | 21.5514 | 21.6964 | 21.5692 | 21.3715 | 21.8951 | 22.2800 | 1 |
Images | k | Best threshold values | ||||||
BA | FPA | MSA | PSO | WWO | MFO | |||
Test 1 | 2 | 95, 168 | 95, 171 | 113, 157 | 98, 165 | 103, 173 | 97, 164 | |
3 | 67, 121, 181 | 88, 134, 179 | 76, 133, 176 | 73, 124, 160 | 90, 139, 168 | 81, 126, 174 | ||
4 | 68, 110, 130, 163 | 60, 103, 160, 200 | 55, 91, 139, 180 | 61, 83, 116, 181 | 79, 121, 146, 175 | 73, 112, 147, 184 | ||
5 | 60, 98, 144, 172, 204 |
46, 65, 109, 142, 176 |
78, 98, 135, 157, 183 |
39, 100, 163, 165, 239 |
63, 91, 144, 168, 187 |
65, 101, 125, 165, 239 |
||
6 | 38, 58, 98, 140, 171, 195 |
71, 89, 127, 176, 194, 216 |
44, 91, 101, 133, 155, 200 |
66, 106, 137, 159, 196, 224 |
66, 115, 140, 162, 178, 193 |
77, 98, 132, 156, 181, 208 |
||
Test 2 | 2 | 62, 148 | 64, 121 | 79, 138 | 75, 147 | 66, 153 | 75, 147 | |
3 | 76, 132, 174 | 42, 83, 158 | 76, 119, 158 | 45, 130, 169 | 43, 109, 146 | 59, 106, 170 | ||
4 | 43, 86, 144, 206 | 55, 108, 133, 190 | 62, 89, 118, 192 | 53, 121, 152, 188 | 47, 118, 159, 203 | 50, 84, 141, 179 | ||
5 | 24, 93, 134, 173, 192 |
35, 93, 126, 155, 191 |
32, 79, 101, 136, 188 |
34, 73, 95, 129, 176 |
56, 84, 104, 145, 175 |
50, 80, 119, 163, 204 |
||
6 | 35, 80, 106, 137, 191, 203 |
56, 101, 126, 160, 177, 200 |
44, 76, 122, 162, 177, 196 |
22, 38, 82, 110, 151, 193 |
65, 83, 107, 145, 179, 199 |
37, 52, 76, 126, 169, 195 |
||
Test 3 | 2 | 82, 162 | 88, 164 | 85, 157 | 69, 177 | 110, 163 | 69, 174 | |
3 | 87, 135, 186 | 62, 141, 178 | 102, 159, 184 | 73, 141, 195 | 81, 154, 192 | 69, 127, 183 | ||
4 | 89, 123, 152, 189 | 64, 114, 151, 176 | 54, 95, 135, 169 | 68, 120, 134, 185 | 38, 93, 148, 192 | 66, 106, 146, 186 | ||
5 | 78, 101, 118, 133, 186 |
67, 98, 135, 149, 174 |
56, 80, 111, 152, 194 |
34, 87, 165, 182, 231 |
75, 101, 132, 169, 213 |
70, 104, 132, 160, 190 |
||
6 | 82, 114, 131, 154, 174, 212 |
49, 80, 94, 114, 174, 198 |
41, 53, 73, 113, 146, 181 |
55, 93, 128, 153, 183, 196 |
58, 71, 78, 118, 147, 181 |
56, 69, 127, 163, 183, 231 |
||
Test 4 | 2 | 101, 174 | 146, 164 | 95, 175 | 98, 174 | 93, 154 | 95, 171 | |
3 | 72, 136, 169 | 117, 177, 212 | 69, 115, 180 | 99, 152, 181 | 70, 113, 169 | 85, 137, 180 | ||
4 | 41, 84, 115, 164 | 50, 80, 146, 183 | 43, 91, 124, 172 | 40, 86, 133, 167 | 63, 96, 159, 172 | 38, 86, 138, 180 | ||
5 | 49, 82, 122, 181, 224 |
29, 75, 104, 120, 205 |
49, 75, 105, 160, 181 |
32, 91, 125, 171, 208 |
37, 100, 116, 152, 210 |
43, 98, 125, 173, 245 |
||
6 | 75, 102, 125, 161, 188, 237 |
43, 110, 129, 150, 181, 211 |
79, 120, 135, 167, 221, 237 |
43, 59, 85, 127, 183, 212 |
32, 61, 78, 122, 148, 211 |
28, 53, 77, 114, 146, 182 |
||
Test 5 | 2 | 74, 135 | 110, 248 | 83, 157 | 70, 135 | 64, 129 | 70, 135 | |
3 | 56, 134, 215 | 61, 120, 184 | 59, 145, 230 | 62, 119, 197 | 80, 127, 180 | 69, 133, 204 | ||
4 | 52, 131, 206, 231 | 95, 145, 200, 234 | 70, 94, 146, 207 | 74, 127, 186, 226 | 81, 114, 190, 221 | 70, 108, 150, 216 | ||
5 | 61, 110, 168, 199, 231 |
33, 71, 97, 154, 225 |
37, 101, 137, 179, 217 |
21, 79, 150, 195, 231 |
63, 93, 134, 188, 233 |
41, 94, 131, 177, 225 |
||
6 | 29, 62, 117, 143, 188, 211 |
23, 75, 110, 142, 208, 238 |
38, 61, 127, 167, 212, 233 |
51, 84, 124, 147, 187, 200 |
32, 72, 91, 116, 192, 228 |
27, 77, 109, 140, 179, 225 |
||
Test 6 | 2 | 84, 168 | 111, 175 | 106, 175 | 91, 170 | 92, 171 | 91, 170 | |
3 | 55, 126, 191 | 84, 145, 188 | 85, 138, 200 | 71, 124, 188 | 68, 128, 177 | 75, 131, 185 | ||
4 | 37, 117, 168, 211 | 60, 137, 164, 194 | 39, 77, 132, 186 | 94, 121, 157, 214 | 54, 139, 178, 219 | 68, 105, 157, 204 | ||
5 | 50, 75, 110, 184, 206 |
41, 82, 122, 189, 234 |
35, 78, 131, 156, 183 |
37, 124, 154, 182, 208 |
42, 108, 120, 179, 205 |
80, 111, 132, 163, 204 |
||
6 | 59, 89, 114, 146, 187, 207 |
32, 58, 116, 146, 186, 214 |
60, 80, 105, 135, 168, 189 |
47, 88, 111, 165, 191, 204 |
44, 65, 113, 146, 170, 199 |
42, 84, 116, 136, 170, 209 |
||
Test 7 | 2 | 97, 166 | 124, 164 | 97, 170 | 96, 163 | 70, 156 | 96, 163 | |
3 | 75, 123, 158 | 53, 94, 176 | 54, 130, 162 | 80, 107, 170 | 62, 178, 219 | 81, 166, 198 | ||
4 | 71, 127, 166, 218 | 66, 121, 145, 184 | 77, 129, 187, 236 | 43, 110, 162, 181 | 95, 123, 166, 209 | 46, 104, 167, 197 | ||
5 | 46, 87, 123, 161, 225 |
40, 99, 130, 175, 198 |
40, 59, 97, 143, 196 |
24, 73, 137, 166, 214 |
65, 122, 146, 175, 214 |
79, 107, 137, 166, 212 |
||
6 | 37, 69, 131, 145, 174, 236 |
47, 63, 112, 162, 186, 223 |
53, 101, 125, 160, 202, 214 |
62, 123, 149, 174, 190, 233 |
62, 110, 167, 193, 204, 233 |
44, 82, 132, 171, 200, 234 |
||
Test 8 | 2 | 91, 170 | 83, 162 | 100, 168 | 93, 161 | 94, 169 | 93, 161 | |
3 | 80, 164, 225 | 109, 165, 201 | 66, 102, 157 | 69, 105, 171 | 59, 114, 161 | 95, 159, 206 | ||
4 | 46, 104, 144, 217 | 73, 109, 175, 225 | 83, 136, 177, 203 | 42, 109, 159, 191 | 47, 81, 124, 156 | 65, 111, 160, 207 | ||
5 | 62, 120, 172, 204, 223 |
36, 55, 115, 157, 217 |
80, 143, 175, 209, 231 |
55, 112, 132, 173, 194 |
68, 102, 131, 164, 223 |
74, 107, 159, 196, 223 |
||
6 | 29, 47, 87, 110, 157, 212 |
58, 85, 113, 133, 165, 229 |
52, 75, 102, 131, 160, 205 |
59, 74, 108, 145, 171, 204 |
58, 98, 144, 157, 181, 201 |
50, 85, 126, 158, 202, 227 |
||
Test 9 | 2 | 103, 155 | 93, 160 | 128, 163 | 104, 156 | 92, 166 | 105, 156 | |
3 | 110, 152, 213 | 92, 152, 212 | 104, 166, 207 | 111, 153, 193 | 69, 115, 167 | 104, 154, 203 | ||
4 | 55, 105, 135, 161 | 44, 86, 150, 205 | 55, 96, 121, 174 | 97, 132, 155, 192 | 63, 99, 173, 203 | 57, 106, 172, 201 | ||
5 | 96, 117, 150, 179, 298 |
92, 121, 136, 152, 204 |
56, 91, 114, 165, 220 |
56, 116, 153, 169, 186 |
92, 129, 154, 172, 201 |
61, 84, 102, 163, 197 |
||
6 | 45, 74, 86, 120, 161, 220 |
66, 84, 96, 128, 156, 184 |
72, 95, 116, 162, 172, 209 |
78, 96, 107, 133, 151, 199 |
53, 84, 102, 111, 165, 211 |
56, 82, 115, 164, 187, 198 |
||
Test 10 | 2 | 74, 148 | 72, 138 | 77, 133 | 75, 153 | 96, 168 | 75, 153 | |
3 | 86, 154, 184 | 75, 104, 213 | 84, 129, 211 | 71, 154, 196 | 97, 143, 196 | 83, 143, 187 | ||
4 | 76, 95, 171, 222 | 83, 125, 164, 203 | 77, 123, 164, 213 | 74, 112, 138, 177 | 74, 123, 156, 210 | 72, 116, 151, 181 | ||
5 | 94, 132, 147, 188, 222 |
85, 146, 168, 202, 230 |
71, 88, 101, 179, 213 |
50, 83, 152, 179, 248 |
81, 132, 174, 214, 230 |
74, 105, 136, 163, 186 |
||
6 | 70, 82, 114, 172, 194, 230 |
74, 110, 126, 163, 194, 203 |
89, 120, 133, 167, 180, 223 |
64, 81, 128, 162, 202, 230 |
72, 126, 162, 192, 213, 233 |
75, 103, 144, 176, 191, 222 |
Images | k | Execution time (in second) | ||||||
BA | FPA | MSA | PSO | WWO | MFO | Rank | ||
Test 1 | 2 | 2.0504 | 2.5449 | 4.1430 | 3.2668 | 2.6219 | 1.8838 | 1 |
3 | 2.1806 | 2.4652 | 3.6404 | 3.7600 | 3.0271 | 2.1470 | 1 | |
4 | 2.3493 | 2.5466 | 3.6591 | 4.2612 | 3.5488 | 2.3476 | 1 | |
5 | 2.3526 | 2.4754 | 3.6553 | 4.4621 | 4.1037 | 2.3232 | 1 | |
6 | 2.4534 | 2.5140 | 3.6961 | 4.7721 | 4.6332 | 2.4476 | 1 | |
Test 2 | 2 | 2.0546 | 2.2233 | 3.5972 | 3.0943 | 2.4066 | 1.9862 | 1 |
3 | 2.2834 | 2.4729 | 3.7247 | 3.7189 | 3.0388 | 2.2371 | 1 | |
4 | 2.4856 | 2.4565 | 3.5933 | 4.0514 | 3.7730 | 2.3832 | 1 | |
5 | 2.6244 | 2.5519 | 3.6495 | 13.892 | 4.2132 | 2.5896 | 2 | |
6 | 2.7085 | 2.6344 | 3.6366 | 4.6383 | 4.9811 | 2.6217 | 1 | |
Test 3 | 2 | 2.0428 | 2.1661 | 3.5012 | 10.081 | 2.3834 | 2.0128 | 1 |
3 | 2.1067 | 2.2636 | 3.6970 | 3.5344 | 2.9690 | 2.1931 | 2 | |
4 | 2.3168 | 2.4309 | 3.6582 | 4.1890 | 3.5014 | 2.2318 | 1 | |
5 | 2.4964 | 2.4430 | 3.6781 | 4.2050 | 4.1567 | 2.4421 | 1 | |
6 | 2.5751 | 2.5232 | 3.7181 | 4.4494 | 4.7794 | 2.5211 | 1 | |
Test 4 | 2 | 2.1245 | 2.2784 | 3.5689 | 3.1980 | 2.4759 | 1.8874 | 1 |
3 | 2.2841 | 2.3142 | 3.6728 | 3.9206 | 3.1605 | 2.1235 | 1 | |
4 | 2.4620 | 2.4784 | 3.7133 | 4.4914 | 3.6540 | 2.4128 | 1 | |
5 | 2.5981 | 2.5782 | 3.7064 | 4.5182 | 4.2552 | 2.5252 | 1 | |
6 | 2.5760 | 2.6113 | 3.7157 | 4.6266 | 4.6624 | 2.5645 | 1 | |
Test 5 | 2 | 2.1369 | 2.3193 | 3.6874 | 3.3801 | 2.5160 | 1.9748 | 1 |
3 | 2.3770 | 2.4655 | 3.8206 | 3.9882 | 3.1202 | 2.1927 | 1 | |
4 | 2.6246 | 2.5708 | 3.7468 | 4.6477 | 3.7042 | 2.4725 | 1 | |
5 | 2.6867 | 2.6756 | 3.7018 | 4.8451 | 4.2924 | 2.6573 | 1 | |
6 | 3.0574 | 2.6619 | 3.7099 | 5.2076 | 4.9496 | 2.6989 | 2 | |
Test 6 | 2 | 2.0659 | 2.2878 | 3.7082 | 3.4298 | 2.4881 | 1.8933 | 1 |
3 | 2.3009 | 2.4216 | 3.7141 | 3.7289 | 3.1803 | 2.1388 | 1 | |
4 | 2.5690 | 2.5297 | 3.7186 | 4.4016 | 3.6365 | 2.5144 | 1 | |
5 | 2.7012 | 2.7481 | 3.8449 | 4.6251 | 4.4892 | 2.5788 | 1 | |
6 | 2.7363 | 2.6549 | 3.5908 | 5.0158 | 5.0763 | 2.6390 | 1 | |
Test 7 | 2 | 2.1999 | 2.2860 | 3.7677 | 3.4719 | 2.5184 | 1.7930 | 1 |
3 | 2.3913 | 2.4617 | 3.8282 | 4.4295 | 3.1260 | 2.1823 | 1 | |
4 | 2.5758 | 2.5069 | 3.6892 | 4.5830 | 3.6773 | 2.5712 | 2 | |
5 | 2.8661 | 2.6928 | 3.7420 | 4.9113 | 4.3654 | 2.6331 | 1 | |
6 | 2.8314 | 2.7552 | 3.8166 | 4.7869 | 4.8823 | 2.7347 | 1 | |
Test 8 | 2 | 2.1196 | 2.2375 | 3.5308 | 3.2347 | 2.6986 | 1.8208 | 1 |
3 | 2.1790 | 2.4691 | 3.6472 | 4.1744 | 3.2448 | 2.0801 | 1 | |
4 | 2.4523 | 2.5377 | 3.6976 | 4.4788 | 3.7100 | 2.3825 | 1 | |
5 | 2.5949 | 2.5873 | 3.7993 | 4.6852 | 4.3499 | 2.5537 | 1 | |
6 | 2.6545 | 2.6189 | 3.7323 | 5.1430 | 4.7387 | 2.5936 | 1 | |
Test 9 | 2 | 2.1158 | 2.2435 | 3.7132 | 3.0533 | 2.3750 | 1.8010 | 1 |
3 | 2.2377 | 2.3223 | 3.7861 | 3.8355 | 2.9901 | 2.1133 | 1 | |
4 | 2.3979 | 2.4493 | 3.6818 | 4.1490 | 3.5694 | 2.3356 | 1 | |
5 | 2.4748 | 2.4971 | 3.6920 | 4.3074 | 4.2244 | 2.4209 | 1 | |
6 | 2.4707 | 2.5587 | 3.7407 | 4.6462 | 4.5649 | 2.5010 | 2 | |
Test 10 | 2 | 2.0775 | 2.1429 | 3.4231 | 3.0237 | 2.3839 | 1.6726 | 1 |
3 | 2.2550 | 2.2918 | 3.5819 | 3.4502 | 2.9528 | 2.2082 | 1 | |
4 | 2.3523 | 2.3987 | 3.6269 | 4.0056 | 3.4883 | 2.3009 | 1 | |
5 | 2.3653 | 2.4887 | 3.7718 | 4.1497 | 4.0963 | 2.3358 | 1 | |
6 | 2.42209 | 2.5584 | 3.7138 | 4.3912 | 4.6485 | 2.4192 | 1 |
Images | k | PSNR values | ||||||
BA | FPA | MSA | PSO | WWO | MFO | Rank | ||
Test 1 | 2 | 54.2052 | 54.0193 | 52.6804 | 53.9194 | 53.3728 | 54.2052 | 1 |
3 | 55.2116 | 54.7434 | 55.5773 | 55.8071 | 54.6025 | 56.2391 | 1 | |
4 | 56.1645 | 56.9214 | 55.8071 | 56.8053 | 55.3545 | 57.8253 | 1 | |
5 | 56.9214 | 57.2139 | 55.4283 | 67.4037 | 56.5877 | 61.6781 | 2 | |
6 | 57.5946 | 55.9464 | 63.0931 | 56.3249 | 56.3249 | 68.3558 | 1 | |
Test 2 | 2 | 56.8302 | 55.6667 | 55.2570 | 55.6667 | 56.4413 | 56.8496 | 1 |
3 | 55.5716 | 55.8390 | 55.5716 | 56.9284 | 58.4671 | 58.5125 | 1 | |
4 | 56.8302 | 57.5587 | 56.8302 | 57.7738 | 57.7738 | 58.6671 | 1 | |
5 | 60.8783 | 57.6678 | 59.6180 | 57.5587 | 57.4521 | 58.5125 | 3 | |
6 | 58.7405 | 57.4521 | 58.5890 | 61.3621 | 56.5356 | 59.3017 | 2 | |
Test 3 | 2 | 61.6543 | 60.6947 | 61.1888 | 59.1452 | 57.4414 | 63.7976 | 1 |
3 | 60.8653 | 64.1985 | 58.3970 | 63.3619 | 61.8294 | 65.2923 | 1 | |
4 | 60.5327 | 65.0068 | 64.6841 | 64.3602 | 66.0216 | 66.2020 | 1 | |
5 | 62.3681 | 65.6347 | 65.9875 | 70.5641 | 62.9357 | 67.5475 | 2 | |
6 | 61.6543 | 66.8489 | 65.6347 | 66.0853 | 65.7478 | 68.2531 | 1 | |
Test 4 | 2 | 53.7298 | 51.8359 | 53.9307 | 53.8281 | 53.3635 | 54.0080 | 1 |
3 | 55.7727 | 53.2812 | 56.2939 | 53.7936 | 54.4415 | 56.3931 | 1 | |
4 | 69.8724 | 64.0939 | 68.4585 | 70.6684 | 57.7874 | 70.6684 | 1 | |
5 | 64.6339 | 76.5339 | 64.6339 | 75.2461 | 54.0512 | 72.8879 | 3 | |
6 | 55.3686 | 68.4585 | 62.6342 | 59.8785 | 71.2461 | 71.5255 | 1 | |
Test 5 | 2 | 55.9353 | 54.4501 | 55.5723 | 56.1030 | 56.1944 | 56.3859 | 1 |
3 | 56.1489 | 56.5395 | 56.6408 | 56.1489 | 55.6932 | 56.7947 | 1 | |
4 | 56.0035 | 55.0787 | 56.1030 | 55.9353 | 55.6534 | 56.4888 | 1 | |
5 | 57.1093 | 57.6783 | 57.8691 | 59.3191 | 56.4366 | 58.1327 | 2 | |
6 | 58.4300 | 59.0280 | 57.8027 | 57.0566 | 57.3278 | 59.0280 | 1 | |
Test 6 | 2 | 52.4037 | 50.9764 | 51.2852 | 52.4037 | 52.3196 | 52.9992 | 1 |
3 | 53.7675 | 52.9992 | 52.9146 | 54.0808 | 54.3233 | 55.5443 | 1 | |
4 | 56.9588 | 55.0405 | 54.3233 | 52.1517 | 55.6405 | 57.5981 | 1 | |
5 | 55.2454 | 54.8447 | 55.8429 | 57.9588 | 56.4138 | 58.3841 | 1 | |
6 | 55.1430 | 57.0950 | 54.9352 | 56.4138 | 56.7949 | 59.1946 | 1 | |
Test 7 | 2 | 52.1853 | 50.1439 | 52.1853 | 52.2305 | 52.2305 | 53.6248 | 1 |
3 | 53.1369 | 52.7825 | 54.3929 | 52.8286 | 54.1279 | 54.4252 | 1 | |
4 | 53.5238 | 53.9356 | 52.9913 | 54.1279 | 52.2737 | 54.9081 | 1 | |
5 | 54.7169 | 55.1408 | 54.7169 | 52.7825 | 53.9928 | 57.7724 | 1 | |
6 | 54.4130 | 54.6643 | 54.4252 | 54.4600 | 54.1279 | 55.1408 | 1 | |
Test 8 | 2 | 51.2625 | 51.1401 | 50.7696 | 51.1401 | 51.0819 | 51.8484 | 1 |
3 | 52.1217 | 50.3589 | 53.9395 | 53.4810 | 51.0247 | 55.1889 | 1 | |
4 | 58.3667 | 52.9207 | 51.8484 | 52.6689 | 58.1344 | 59.3198 | 1 | |
5 | 54.6097 | 54.7941 | 52.1217 | 60.9018 | 53.6305 | 61.3007 | 1 | |
6 | 62.1126 | 55.4021 | 56.8943 | 55.1889 | 55.4021 | 64.4974 | 1 | |
Test 9 | 2 | 54.7684 | 56.0844 | 50.9610 | 54.6524 | 54.5365 | 56.2376 | 1 |
3 | 53.9616 | 56.2376 | 54.6524 | 53.8444 | 54.6524 | 61.7530 | 1 | |
4 | 67.6683 | 73.5879 | 67.6683 | 55.5133 | 58.1149 | 68.0342 | 2 | |
5 | 62.8685 | 59.5786 | 67.1721 | 67.1721 | 56.2376 | 73.5879 | 1 | |
6 | 72.9963 | 62.8685 | 68.6413 | 57.8977 | 68.6413 | 79.2437 | 1 | |
Test 10 | 2 | 49.4261 | 49.4702 | 49.3850 | 49.4109 | 49.2129 | 49.4809 | 1 |
3 | 49.3039 | 49.4109 | 49.3191 | 49.3437 | 49.2012 | 49.4261 | 1 | |
4 | 49.3976 | 49.3267 | 49.3850 | 49.4261 | 49.4261 | 49.5080 | 1 | |
5 | 49.2362 | 49.3115 | 49.4261 | 49.1974 | 49.3437 | 49.5080 | 1 | |
6 | 49.5634 | 49.4261 | 49.2816 | 49.3850 | 49.4702 | 50.5952 | 1 |
Images | k | SSIM values | ||||||
BA | FPA | MSA | PSO | WWO | MFO | Rank | ||
Test 1 | 2 | 0.5811 | 0.5630 | 0.4666 | 0.5589 | 0.5389 | 0.5827 | 1 |
3 | 0.6174 | 0.6028 | 0.6291 | 0.6196 | 0.5792 | 0.6677 | 1 | |
4 | 0.6275 | 0.6713 | 0.6450 | 0.6841 | 0.6040 | 0.6962 | 1 | |
5 | 0.6706 | 0.6692 | 0.6240 | 0.6779 | 0.6761 | 0.7428 | 1 | |
6 | 0.6579 | 0.6752 | 0.7295 | 0.6565 | 0.6272 | 0.7853 | 1 | |
Test 2 | 2 | 0.5934 | 0.5618 | 0.5486 | 0.5624 | 0.5854 | 0.5951 | 1 |
3 | 0.5849 | 0.5892 | 0.5737 | 0.6229 | 0.6440 | 0.6549 | 1 | |
4 | 0.6118 | 0.6431 | 0.6084 | 0.6336 | 0.6302 | 0.6680 | 1 | |
5 | 0.6700 | 0.6321 | 0.6721 | 0.6239 | 0.6315 | 0.6953 | 1 | |
6 | 0.6764 | 0.6305 | 0.6926 | 0.7184 | 0.6484 | 0.7095 | 2 | |
Test 3 | 2 | 0.7776 | 0.7700 | 0.7735 | 0.7433 | 0.7326 | 0.7782 | 1 |
3 | 0.7694 | 0.7963 | 0.7380 | 0.7321 | 0.7388 | 0.8075 | 1 | |
4 | 0.7661 | 0.8171 | 0.8107 | 0.7983 | 0.7966 | 0.8286 | 1 | |
5 | 0.8008 | 0.7352 | 0.8087 | 0.7841 | 0.7682 | 0.8266 | 1 | |
6 | 0.7468 | 0.7928 | 0.8434 | 0.7941 | 0.8482 | 0.8560 | 1 | |
Test 4 | 2 | 0.5682 | 0.4613 | 0.5752 | 0.5724 | 0.5494 | 0.5840 | 1 |
3 | 0.6295 | 0.5643 | 0.6447 | 0.5538 | 0.5765 | 0.6573 | 1 | |
4 | 0.7995 | 0.7457 | 0.8018 | 0.7963 | 0.7050 | 0.8387 | 1 | |
5 | 0.8021 | 0.7892 | 0.7802 | 0.8431 | 0.5767 | 0.8509 | 1 | |
6 | 0.6250 | 0.8207 | 0.8056 | 0.7640 | 0.8148 | 0.8493 | 1 | |
Test 5 | 2 | 0.5874 | 0.5694 | 0.5478 | 0.5923 | 0.5947 | 0.6025 | 1 |
3 | 0.6513 | 0.6167 | 0.6558 | 0.6536 | 0.5760 | 0.6752 | 1 | |
4 | 0.6629 | 0.5762 | 0.6594 | 0.6147 | 0.6122 | 0.6669 | 1 | |
5 | 0.6686 | 0.6775 | 0.6918 | 0.7207 | 0.6737 | 0.7221 | 1 | |
6 | 0.7354 | 0.7299 | 0.7183 | 0.6835 | 0.7114 | 0.7593 | 1 | |
Test 6 | 2 | 0.3856 | 0.3073 | 0.3285 | 0.3856 | 0.3823 | 0.4098 | 1 |
3 | 0.4862 | 0.4423 | 0.4438 | 0.5025 | 0.5060 | 0.5417 | 1 | |
4 | 0.6023 | 0.5147 | 0.5425 | 0.4154 | 0.5323 | 0.6141 | 1 | |
5 | 0.5798 | 0.5765 | 0.5771 | 0.5794 | 0.6314 | 0.6562 | 1 | |
6 | 0.6170 | 0.6676 | 0.6141 | 0.6412 | 0.6737 | 0.7035 | 1 | |
Test 7 | 2 | 0.5412 | 0.2944 | 0.5452 | 0.5357 | 0.5357 | 0.6049 | 1 |
3 | 0.5439 | 0.5643 | 0.6308 | 0.5533 | 0.6497 | 0.6713 | 1 | |
4 | 0.5819 | 0.5867 | 0.5474 | 0.6396 | 0.5011 | 0.6571 | 1 | |
5 | 0.6752 | 0.6858 | 0.6817 | 0.5044 | 0.5725 | 0.7308 | 1 | |
6 | 0.6826 | 0.6776 | 0.6360 | 0.6650 | 0.5908 | 0.6938 | 1 | |
Test 8 | 2 | 0.3781 | 0.3656 | 0.3403 | 0.3656 | 0.3661 | 0.4100 | 1 |
3 | 0.4250 | 0.2956 | 0.4948 | 0.4927 | 0.3533 | 0.5583 | 1 | |
4 | 0.6528 | 0.4934 | 0.3981 | 0.4760 | 0.6109 | 0.6803 | 1 | |
5 | 0.5484 | 0.5568 | 0.3853 | 0.4986 | 0.5249 | 0.6793 | 1 | |
6 | 0.7181 | 0.5974 | 0.6489 | 0.6071 | 0.5787 | 0.7293 | 1 | |
Test 9 | 2 | 0.5502 | 0.6010 | 0.2334 | 0.5494 | 0.5547 | 0.6075 | 1 |
3 | 0.4929 | 0.5707 | 0.5475 | 0.4852 | 0.5360 | 0.6251 | 1 | |
4 | 0.5860 | 0.6949 | 0.6196 | 0.4427 | 0.5586 | 0.7198 | 1 | |
5 | 0.6465 | 0.6827 | 0.7318 | 0.6505 | 0.4812 | 0.7441 | 1 | |
6 | 0.7208 | 0.6556 | 0.7322 | 0.5454 | 0.7166 | 0.7698 | 1 | |
Test 10 | 2 | 0.1722 | 0.1666 | 0.1571 | 0.1729 | 0.1502 | 0.1733 | 1 |
3 | 0.1611 | 0.1719 | 0.1644 | 0.1708 | 0.1475 | 0.1772 | 1 | |
4 | 0.1822 | 0.1678 | 0.1775 | 0.1753 | 0.1804 | 0.1927 | 1 | |
5 | 0.1486 | 0.1511 | 0.1786 | 0.6364 | 0.1645 | 0.1915 | 2 | |
6 | 0.2091 | 0.1812 | 0.1565 | 0.1697 | 0.1755 | 0.2901 | 1 |
Images | k | Wilcoxon test | ||||
MFO vs BA | MFO vs FPA | MFO vs MSA | MFO vs PSO | MFO vs WWO | ||
Test 1 | 2 | 4.1486E-08 | 2.9135E-07 | 3.7215E-07 | 1.0905E-02 | 4.9558E-08 |
3 | 4.1406E-03 | 8.8674E-01 | 2.7328E-02 | 5.9956E-04 | 1.8056E-02 | |
4 | 3.9271E-03 | 6.8298E-03 | 2.3871E-05 | 5.2530E-04 | 5.9054E-05 | |
5 | 3.5064E-03 | 4.4599E-02 | 7.7394E-06 | 2.5972E-04 | 7.7394E-06 | |
6 | 1.5429E-04 | 1.6017E-04 | 2.1821E-11 | 1.6475E-04 | 2.1821E-11 | |
Test 2 | 2 | 1.5649E-11 | 4.1040E-11 | 4.5618E-11 | 5.7016E-03 | 2.6819E-11 |
3 | 3.7061E-02 | 1.4931E-03 | 4.5940E-02 | 1.5957E-02 | 7.3007E-03 | |
4 | 2.1572E-02 | 7.2382E-04 | 1.1435E-02 | 9.2276E-03 | 1.8454E-02 | |
5 | 3.0221E-07 | 1.2754E-02 | 4.4680E-10 | 3.9238E-02 | 4.4680E-10 | |
6 | 2.1287E-05 | 6.0511E-04 | 4.8389E-10 | 1.9143E-02 | 4.8389E-10 | |
Test 3 | 2 | 1.6510E-11 | 1.2118E-12 | 1.2118E-12 | 2.7793E-03 | 1.2118E-12 |
3 | 1.7047E-02 | 7.9323E-05 | 9.8208E-03 | 5.1474E-03 | 3.8910E-02 | |
4 | 1.5568E-02 | 4.8012E-02 | 6.1697E-05 | 6.0010E-03 | 1.8438E-03 | |
5 | 1.2075E-04 | 1.1553E-03 | 8.9553E-08 | 8.6976E-06 | 8.9553E-08 | |
6 | 4.2458E-05 | 1.6691E-02 | 3.9562E-10 | 1.4187E-05 | 3.9562E-10 | |
Test 4 | 2 | 1.4119E-10 | 3.6912E-11 | 4.5618E-11 | 2.7605E-02 | 1.5672E-11 |
3 | 9.6851E-08 | 1.0617E-03 | 1.3782E-02 | 1.8815E-02 | 2.0296E-11 | |
4 | 2.3687E-03 | 2.6206E-03 | 3.3040E-02 | 2.2534E-02 | 1.8354E-02 | |
5 | 1.3187E-02 | 6.9403E-03 | 6.0393E-02 | 3.3072E-02 | 1.3187E-02 | |
6 | 1.0490E-02 | 3.1661E-03 | 6.3076E-09 | 6.0661E-05 | 3.0311E-04 | |
Test 5 | 2 | 4.7773E-09 | 1.4148E-08 | 1.8780E-09 | 6.9419E-03 | 1.0569E-09 |
3 | 1.0226E-06 | 1.5514E-03 | 9.3662E-04 | 1.7753E-02 | 2.8427E-10 | |
4 | 1.2338E-04 | 6.8037E-03 | 9.0570E-05 | 6.0755E-04 | 6.6120E-03 | |
5 | 1.9974E-02 | 1.5408E-02 | 1.9284E-05 | 1.8299E-02 | 1.1010E-02 | |
6 | 2.3675E-02 | 1.9315E-02 | 9.3069E-08 | 2.8298E-02 | 2.0096E-02 | |
Test 6 | 2 | 5.4162E-09 | 5.4162E-09 | 2.4736E-08 | 2.8298E-02 | 8.3512E-09 |
3 | 1.2298E-07 | 1.5879E-06 | 3.6108E-02 | 7.8961E-03 | 7.6552E-10 | |
4 | 1.3692E-02 | 1.2204E-02 | 6.7678E-04 | 3.3418E-02 | N/A | |
5 | 1.5319E-02 | 1.3228E-05 | 3.7759E-02 | 8.2918E-03 | 8.0670E-03 | |
6 | 7.9518E-01 | 7.6105E-05 | 8.8807E-06 | 1.6308E-03 | 7.6285E-03 | |
Test 7 | 2 | 1.1999E-12 | 1.2118E-12 | 1.2118E-12 | 5.5398E-03 | 1.2118E-12 |
3 | 1.0043E-08 | 3.2704E-04 | 1.7181E-04 | 4.5838E-04 | 1.8783E-11 | |
4 | 2.2155E-05 | 1.3080E-04 | 2.0545E-03 | 1.8360E-02 | 1.8586E-03 | |
5 | 9.2817E-05 | 5.9629E-04 | 2.6611E-02 | 2.7791E-02 | 7.1075E-03 | |
6 | 8.1845E-01 | 1.8944E-02 | 1.0237E-07 | 7.3815E-04 | 2.6871E-02 | |
Test 8 | 2 | 1.1067E-08 | 1.2380E-09 | 1.2377E-09 | 9.5909E-03 | 1.2377E-09 |
3 | 5.0104E-03 | 5.7108E-04 | 7.4568E-04 | 2.5069E-02 | 7.4568E-03 | |
4 | 6.0746E-03 | 7.7156E-05 | 4.5418E-04 | 1.2662E-02 | 7.5196E-03 | |
5 | 1.2619E-02 | 7.7958E-05 | 4.3177E-10 | 5.6943E-07 | 4.3177E-10 | |
6 | 1.0049E-02 | 1.1403E-02 | 2.5562E-11 | 9.6312E-05 | 2.5562E-11 | |
Test 9 | 2 | 4.1906E-10 | 7.2256E-10 | 1.2384E-09 | 4.5164E-02 | 1.1236E-09 |
3 | 2.0185E-02 | 5.6566E-03 | 6.7067E-03 | 8.2284E-02 | N/A | |
4 | 1.7717E-02 | 8.1726E-03 | 2.2107E-06 | 2.0015E-02 | 7.4528E-03 | |
5 | 1.2740E-02 | 3.7302E-05 | 4.5563E-10 | 2.6262E-05 | 4.5563E-10 | |
6 | 1.4911E-02 | 1.7368E-03 | 4.3348E-10 | 9.9941E-04 | 4.3348E-10 | |
Test 10 | 2 | 2.5301E-10 | 1.2384E-09 | 1.2384E-09 | N/A | 7.5947E-10 |
3 | 8.1850E-03 | 1.5376E-02 | 1.4949E-02 | 9.7602E-01 | 6.5949E-04 | |
4 | 1.3894E-02 | 1.3909E-02 | 3.7115E-05 | 1.2287E-02 | 1.8341E-03 | |
5 | 9.3482E-01 | 1.5129E-02 | 1.2758E-05 | 6.5560E-03 | 3.6908E-04 | |
6 | 2.4276E-02 | 1.5559E-02 | 3.9225E-08 | 4.9250E-04 | 2.3328E-05 |
The optimal fitness of each algorithm is given in Table 4. The optimization goal is to achieve the best threshold levels by maximizing the desired value of Kapur's entropy. For given segmented images, the threshold levels are set to 2, 3, 4, 5 and 6. The fitness value of each algorithm increases as the threshold level increases, and each algorithm can achieve a better fitness value under a relatively high threshold level. The processed images embody more segmentation information. The ranking based on the fitness value is applied to reflect the optimization performance and segmentation ability of the MFO. The fitness value and ranking of MFO are better than those of the other algorithms, which indicates that MFO can utilize exploration and exploitation to realize the maximum fitness value. The processed images of the MFO have more segmentation information. The best threshold values of each algorithm are given in Table 5. The choice of the threshold level directly affects the effect and quality of the processed image. MFO finds the optimal objective value according to the best threshold level. To summarize, MFO can dodge immature convergence to achieve better segmentation accuracy and effect.
The average execution time of each algorithm is given in Table 6. The execution time reflects the computational complexity of each algorithm and the realization ability of the problem. At the same threshold level, the algorithm consumes less time and has a better optimization performance. As the segmentation threshold level increases, the time consumption gradually increases. Compared with other algorithms, the execution time of the MFO is better. MFO consumes less time to address image segmentation.
The PSNR of each algorithm is given in Table 7. The selection of the threshold level is crucial for the accuracy and effect of image segmentation. The overall segmentation performance improves if the threshold level increases. The PSNR is an effective criterion to determine the distortion between the provided image and the processed image and the segmentation ability of the multilevel thresholding image. The PSNR increases accordingly when the threshold level becomes larger, and the algorithm has lower distortion. To demonstrate the advantage of the MFO, the ranking is based upon the PSNR. For a given image, the threshold levels are defined as 2, 3, 4, 5 and 6; each algorithm has 50 PSNR values; and 43 PSNR values of the MFO are better. The PSNR values and ranking of the MFO are better than those of other algorithms, which indicates that the MFO has better overall segmentation performance and better superiority. The experimental results indicate that MFO has stable practicability and robustness to obtain a better segmentation effect.
The SSIM of each algorithm is given in Table 8. SSIM based on the brightness, contrast and structural information is used to determine the visual similarity between the provided image and the processed image. When the threshold level increases, the SSIM value becomes larger. The optimization algorithm obtains the processed image with less distortion, and the processed image is close to the provided image. The ranking based on the SSIM value is used to detect the segmentation ability. The ranking of the MFO is better, which indicates that the segmented images of the MFO contain more segmentation information. Each algorithm has 50 SSIM values, the first-place ranking of MFO is 48 and the second-place ranking of MFO is 2. The SSIM values and segmentation effect of the MFO are better than those of other algorithms. MFO obtains a segmented image that is close to the original image. The experimental results indicate that MFO has better calculation precision and overall segmentation performance.
The p value of the Wilcoxon rank-sum is given in Table 9. Wilcoxon's rank-sum is utilized to identify whether there is a noteworthy distinction between the two algorithms. If $ p < 0.05 $, then there is a noteworthy distinction between MFO and other algorithms. If $ p > 0.05 $ is expressed in bold, then there is no noteworthy distinction between MFO and other algorithms. There is a noteworthy distinction in most cases, and the experimental data are valid.
The segmented images of Tests 1-10 for different algorithms using the Kapur method at levels 2, 3, 4, 5 and 6 are given in Figures 5-14. The segmentation quality of the provided images is closely related to the threshold level. A segmented image with a higher threshold level includes more segmentation information. MFO has a strong enhancement and optimization performance to obtain better segmented images that are close to the original images. The MFO can find excellent fitness values and higher calculation accuracy under a given threshold level. The population size of each algorithm is set to 30, the maximum number of iterations is set to 100 and the number of independent runs is set to 30. MFO consumes less execution time to achieve a smaller computational complexity. The PSNR value and SSIM value of the MFO are better than those of other algorithms so that the MFO has better distortion and structural similarity. Wilcoxon's rank-sum can effectively verify the noteworthy distinction between MFO and other algorithms. In summary, MFO has stronger robustness and a better segmentation effect to solve the multilevel thresholding image segmentation problem.
Statistically, the MFO simulates the transverse orientation navigation behavior of the moths to effectively search for the global optimal solution in the search space. MFO resolves multilevel thresholding image segmentation for the following reasons. First, for MFO, the algorithm framework is simple, the amount of calculation is small, the control parameters are few and the algorithm is easy to implement. The MFO has better search ability and strong robustness. Second, MFO has a better position update strategy and overall optimization performance. MFO can effectively avoid falling into a local optimal solution or premature convergence. MFO balances the global search ability and the local search ability in the optimization space to improve the convergence speed and calculation accuracy. Third, the combination of MFO and Kapur's entropy method realizes complementary advantages to enhance the segmentation effect and optimization performance. To summarize, MFO is the optimal choice to solve the multilevel thresholding image segmentation problem.
The objective of image segmentation is to consume less time to attain the best threshold values by maximizing the objective value of Kapur's entropy. This paper proposes an MFO based on Kapur's entropy to resolve multithreshold image segmentation. The MFO simulates the transverse orientation navigation mechanism of moths to perform global search optimization. MFO has high search efficiency and strong robustness to avoid immature convergence or falling into the local solution, which balances exploration and exploitation to find the best solution in the optimization space. For image segmentation, as the threshold level increases, the difference between the MFO and other algorithms is significant. To verify the overall segmentation performance, compared with other algorithms, MFO has a faster convergence rate and higher calculation precision to obtain segmented images that contain more useful segmentation information. The experimental results indicate that the MFO has strong stability and a better segmentation effect in terms of the fitness value, threshold values, execution time, PSNR, SSIM and Wilcoxon's rank-sum. Meanwhile, MFO has stronger robustness and better practicality to effectively achieve the image segmentation problem.
In future research, we will develop a system that can automatically detect the optimal number of levels for the input image. MFO will be applied to settle complicated high-threshold or color image segmentation. Meanwhile, different segmentation methods will be introduced into the MFO such as Tsallis entropy, Renyi entropy, cross entropy, fuzzy entropy and Otsu. These research works will further demonstrate the effectiveness and feasibility of MFO.
The authors declare that they have no known competing financial interests or personal relationships that could have appeared to influence the work reported in this paper. This work was partially funded by the Research on the Application of Internet of Things Technology based on Smart Chain in University Management under Grant No. 2021-KYYWF-E005.
The authors declare that we do not have any commercial or associative interest that represents a conflict of interest in connection with the work submitted.
[1] | Bintsis T (2018) Lactic acid bacteria: their applications in foods. J Bacteriol Mycol 5: 1065. |
[2] | Hayek SA, Ibrahim SA (2013) Current limitations and challenges with lactic acid bacteria: a review. Food Nutr Sci 4: 73–87. |
[3] | Khalid K (2011) An overview of lactic acid bacteria. Int J Biosci 1: 1–13. |
[4] | Bintsis T, Athanasoulas A (2015) Dairy starter cultures, In: Papademas P, Editor, Dairy Microbiology, A Practical Approach, Boca Raton: CRC Press, 114–154. |
[5] | Von Wright A, Axelsson L (2011) Lactic acid bacteria: An introduction, In: Lahtinne S, Salminen S, Von Wright A, et al., Editors, Lactic Acid Bacteria: Microbiological and Functional Aspects, London: CRC Press, 1–17. |
[6] | Sheehan JJ (2007) What are starters and what starter types are used for cheesemaking? In: McSweeney PLH, Editor, Cheese problems solved, Boca Raton: Woodhead Publishing Ltd., 36–37. |
[7] | Tamime AY (2002) Microbiology of starter cultures, In: Robinson RK, Editor, Dairy Microbiology Handbook, 3 Eds., New York: John Wiley & Sons Inc., 261–366. |
[8] | Parente E, Cogan TM (2004) Starter cultures: General aspects, In: Fox PF, McSweeney PLH, Cogan TM, et al., Cheese: Chemistry, Physics and Microbiology, 4 Eds., London: Elsevier Academic Press, 23–147. |
[9] | Bourdichon F, Boyaval P, Casaregola J, et al. (2012) The 2012 Inventory of Microbial Species with technological beneficial role in fermented food products. B Int Dairy Fed 455: 22–61. |
[10] | Bourdichon F, Berger B, Casaregola S, et al. (2012) A safety assessment of microbial food cultures with history of use in fermented dairy products. B Int Dairy Fed 455: 2–12. |
[11] | Ricci A, Allende A, Bolton D, et al. (2017) Scientific Opinion on the update of the list of QPS-recommended biological agents intentionally added to food or feed as notified to EFSA. EFSA J 15: 4664. |
[12] | Beresford T, Cogan T (1997) Improving Cheddar cheese flavor, In: Proceedings of the 5th Cheese Symposium, Cork: Teagasc/University College Cork, 53–61. |
[13] | Picon A (2018) Cheese Microbial Ecology and Safety, In: Papademas P, Bintsis T, Editors, Global Cheesemaking Technology, Cheese Quality and Characteristics, Chichester: John Wiley & Sons Ltd., 71–99. |
[14] | Wedajo B (2015) Lactic acid bacteria: benefits, selection criteria and probiotic potential in fermented food. J Prob Health 3: 129. |
[15] |
Grattepanche F, Miescher-Schwenninger S, Meile L, et al. (2008) Recent developments in cheese cultures with protective and probiotic functionalities. Dairy Sci Technol 88: 421–444. doi: 10.1051/dst:2008013
![]() |
[16] | Law BA (1999) Cheese ripening and cheese flavour technology, In: Law BA, Editor, Technology of Cheesemaking, Sheffield: Sheffield Academic Press Ltd., 163–192. |
[17] |
Smit G, Smit BA, Engels WJ (2005) Flavour formation by lactic acid bacteria and biochemical flavour profiling of cheese products. FEMS Microbiol Rev 29: 591–610. doi: 10.1016/j.fmrre.2005.04.002
![]() |
[18] | Tamime AY, Robinson RK (1999) Yoghurt Science and Technology, 2 Eds., Cambridge: Woodhead Publishing Ltd. |
[19] | Ammor MS, Mayo B (2006) Selection criteria for lactic acid bacteria to be used as functional starter cultures in dry sausage production: An update. Meat Sci 76: 138–146. |
[20] | Souza MJ, Ardo Y, McSweeney PLH (2001) Advances in the study of proteolysis in cheese. Int Dairy J 11: 327–345. |
[21] |
Martinez FAC, Balciunas EM, Salgado JM, et al. (2013) Lactic acid properties, applications and production: A review. Trends Food Sci Tech 30: 70–83. doi: 10.1016/j.tifs.2012.11.007
![]() |
[22] |
Burgos-Rubio CN, Okos MR, Wankat PC (2000) Kinetic study of the conversion of different substrates to lactic acid using Lactobacillus bulgaricus. Biotechnol Progr 16: 305–314. doi: 10.1021/bp000022p
![]() |
[23] |
Hofvendahl K, Hahn-Hägerda B (2000) Factors affecting the fermentative lactic acid production from renewable resources. Enzyme Microb Tech 26: 87–107. doi: 10.1016/S0141-0229(99)00155-6
![]() |
[24] | Crow VL, Davey GP, Pearce LE, et al. (1983) Plasmid linkage of the D-tagatose 6-phosphate pathway in Streptococcus lactis: Effect on lactose and galactose metabolism. J Bacteriol 153: 76–83. |
[25] |
Hugenholtz J (1993) Citrate metabolism in lactic acid bacteria. FEMS Microbiol Rev 12: 165–178. doi: 10.1111/j.1574-6976.1993.tb00017.x
![]() |
[26] |
McFeeters RF, Fleming HP, Thompson RL (1982) Malic acid as a source of carbon dioxide in cucumber fermentations. J Food Sci 47: 1862–1865. doi: 10.1111/j.1365-2621.1982.tb12900.x
![]() |
[27] | Daeschel MA, McFeeters RF, Fleming HP, et al. (1984) Mutation and selection of Lactobacillus plantarum strains that do not produce carbon dioxide from malate. Appl Environ Microb 47: 419–420. |
[28] | Li KY (2004) Fermentation: Principles and Microorganisms, In: Hui YH, Meunier-Goddik L, Hansen LM, et al., Editors, Handbook of Food and Beverage Fermentation Technology, New York: Marcel Dekker Inc., 594–608. |
[29] |
Chopin A (1993) Organization and regulation of genes for amino acid biosynthesis in lactic acid bacteria. FEMS Microbiol Rev 12: 21–38. doi: 10.1111/j.1574-6976.1993.tb00011.x
![]() |
[30] |
Kunji ERS, Mierau I, Hagting A, et al. (1996) The proteolytic system of lactic acid bacteria. Anton Leeuw 70: 187–221. doi: 10.1007/BF00395933
![]() |
[31] | Upadhyay VK, McSweeney PLH, Magboul AAA, et al. (2004) Proteolysis in Cheese during Ripening, In: Fox PF, McSweeney PLH, Cogan TM, et al., Editors, Cheese: Chemistry, Physics and Microbiology, 4 Eds., London: Elsevier Academic Press, 391–433. |
[32] | Curtin AC, McSweeney PLH (2004) Catabolism of Amino Acids in Cheese during Ripening, In: Fox PF, McSweeney PLH, Cogan TM, et al., Editors, Cheese: Chemistry, Physics and Microbiology, 4 Eds., London: Elsevier Academic Press, 435–454. |
[33] |
Christensen JE, Dudley EG, Pederson JA, et al. (1999) Peptidases and amino acid catabolism in lactic acid bacteria. Anton Leeuw 76: 217–246. doi: 10.1023/A:1002001919720
![]() |
[34] | Fox PF, Wallace JM (1997) Formation of flavour compounds in cheese. Adv Appl Microbiol 45: 17–85. |
[35] |
Khalid NM, Marth EM (1990) Lactobacilli-their enzymes and role in ripening and spoilage of cheese: A review. J Dairy Sci 73: 2669–2684. doi: 10.3168/jds.S0022-0302(90)78952-7
![]() |
[36] |
Bintsis T, Robinson RK (2004) A study of the effects of adjunct cultures on the aroma compounds of Feta-type cheese. Food Chem 88: 435–441. doi: 10.1016/j.foodchem.2004.01.057
![]() |
[37] |
Broadbent JR, McMahon DJ, Welker DL, et al. (2003) Biochemistry, genetics, and applications of exopolysaccharide production in Streptococcus thermophilus: A review. J Dairy Sci 86: 407–423. doi: 10.3168/jds.S0022-0302(03)73619-4
![]() |
[38] | Callanan MJ, Ross RP (2004) Starter Cultures: Genetics, In: Fox PF, McSweeney PLH, Cogan TM, et al., Editors, Cheese: Chemistry, Physics and Microbiology, 4 Eds., London: Elsevier Academic Press, 149–161. |
[39] |
Klaenhammer T, Altermann E, Arigoni F, et al. (2002) Discovering lactic acid bacteria by genomics. Anton Leeuw 82: 29–58. doi: 10.1023/A:1020638309912
![]() |
[40] | Morelli L, Vogensen FK, Von Wright A (2011) Genetics of Lactic Acid Bacteria, In: Salminen S, Von Wright A, Ouwehand A, Editors, Lactic Acid Bacteria-Microbiological and Functional Aspects, 3 Eds., New York: Marcel Dekker Inc., 249–293. |
[41] |
Mills S, O'Sullivan O, Hill C, et al. (2010) The changing face of dairy starter culture research: From genomics to economics. Int J Dairy Tech 63: 149–170. doi: 10.1111/j.1471-0307.2010.00563.x
![]() |
[42] | NCBI, Data collected from Genbank, 2018. Available from: https://www.ncbi.nlm.nih.gov/genome/browse/. |
[43] | Oliveira AP, Nielsen J, Förster J (2005) Modeling Lactococcus lactis using a genome-scale flux model. BMC Microbiol 55: 39. |
[44] | Salama M, Sandine WE, Giovannoni S (1991) Development and application of oligonucleotide probes for identification of Lactococcus lactis subsp. cremoris. Appl Environ Microb 57: 1313–1318. |
[45] | McKay LL (1985) Roles of plasmids in starter cultures, In: Gilliland SE, Editor, Bacterial Starter Cultures for Food, Boca Raton: CRC Press, 159–174. |
[46] |
Davidson B, Kordis N, Dobos M, et al. (1996) Genomic organization of lactic acid bacteria. Anton Leeuw 70: 161–183. doi: 10.1007/BF00395932
![]() |
[47] |
Dunny G, McKay LL (1999) Group II introns and expression of conjugative transfer functions in lactic acid bacteria. Anton Leeuw 76: 77–88. doi: 10.1023/A:1002085605743
![]() |
[48] | Hughes D (2000) Evaluating genome dynamics: The constraints on rearrangements within bacterial genomes. Genome Biol 1: 1–8. |
[49] |
Hols P, Kleerebezem M, Schanck AN, et al. (1999) Conversion of Lactococcus lactis from homolactic to homoalanine fermentation through metabolic engineering. Nat Biotechnol 17: 588–592. doi: 10.1038/9902
![]() |
[50] |
Bolotin A, Quinquis B, Renault P, et al. (2004) Complete sequence and comparative genome analysis of the dairy bacterium Streptococcus thermophilus. Nat Biotechnol 22: 1554–1558. doi: 10.1038/nbt1034
![]() |
[51] |
Pastink MI, Teusink B, Hols P, et al. (2009) Genome-scale model of Streptococcus thermophilus LMG18311 for metabolic comparison of lactic acid bacteria. Appl Environ Microb 75: 3627–3633. doi: 10.1128/AEM.00138-09
![]() |
[52] |
Madera C, Garcia P, Janzen T, et al. (2003) Characterization of technologically proficient wild Lactococcus lactis strains resistant to phage infection. Int J Food Microbiol 86: 213–222. doi: 10.1016/S0168-1605(03)00042-4
![]() |
[53] |
Turgeon N, Frenette M, Moineau S (2004) Characterization of a theta-replicating plasmid from Streptococcus thermophilus. Plasmid 51: 24–36. doi: 10.1016/j.plasmid.2003.09.004
![]() |
[54] | Hols P, Hancy F, Fontaine L, et al. (2005) New insights in the molecular biology and physiology of Streptococcus thermophilus revealed by comparative genomics. FEMS Microbiol Rev 29: 435–463. |
[55] | O'Sullivan DJ (1999) Methods for analysis of the intestinal microflora, In: Tannock GW, Editor, Probiotics: A critical review, Norfolk: Horizon Scientific Press. |
[56] | Solow BT, Somkuti GA (2000) Molecular properties of Streptococcus thermophilus plasmid pER35 encoding a restriction modification system. Curr Microbiol 42: 122–128. |
[57] |
El Demerdash HAM, Oxmann J, Heller KJ, et al. (2006) Yoghurt fermentation at elevated temperatures by strains of Streptococcus thermophilus expressing a small heat-shock protein: Application of a two-plasmid system for constructing food-grade strains of Streptococcus thermophilus. Biotechnol J 1: 398–404. doi: 10.1002/biot.200600018
![]() |
[58] |
Chevallier B, Hubert JC, Kammerer B (1994) Determination of chromosome size and number of rrn loci in Lactobacillus plantarum by pulsed-field gel electrophoresis. FEMS Microbiol Lett 120: 51–56. doi: 10.1111/j.1574-6968.1994.tb07006.x
![]() |
[59] |
Daniel P (1995) Sizing the Lactobacillus plantarum genome and other lactic bacteria species by transverse alternating field electrophoresis. Curr Microbiol 30: 243–246. doi: 10.1007/BF00293640
![]() |
[60] |
Oliveira PM, Zannini E, Arendt EK (2014) Cereal fungal infection, mycotoxins, and lactic acid bacteria mediated bioprotection: From crop farming to cereal products. Food Microbiol 37: 78–95. doi: 10.1016/j.fm.2013.06.003
![]() |
[61] |
Johnson JL, Phelps CF, Cummins CS, et al. (1980) Taxonomy of the Lactobacillus acidophilus group. Int J Syst Bacteriol 30: 53–68. doi: 10.1099/00207713-30-1-53
![]() |
[62] | Fujisawa T, Benno Y, Yaeshima T, et al. (1992) Taxonomic study of the Lactobacillus acidophilus group, with recognition of Lactobacillus gallinarum sp. nov. and Lactobacillus johnsonii sp. nov. and synonymy of Lactobacillus acidophilus group A3 with the type strain of Lactobacillus amylovorus. Int J Syst Bacteriol 42: 487–491. |
[63] |
Link-Amster H, Rochat F, Saudan KY, et al. (1994) Modulation of a specific humoral immune response and changes in intestinal flora mediated through fermented milk intake. FEMS Immunol Med Mic 10: 55–64. doi: 10.1111/j.1574-695X.1994.tb00011.x
![]() |
[64] |
Schiffrin EJ, Rochat F, Link-Amster H, et al. (1995) Immunomodulation of human blood cells following the ingestion of lactic acid bacteria. J Dairy Sci 78: 491–497. doi: 10.3168/jds.S0022-0302(95)76659-0
![]() |
[65] | Bernet-Camard MF, Liévin V, Brassart D, et al. (1997) The human Lactobacillus acidophilus strain La1 secretes a non bacteriocin antibacterial substance active in vitro and in vivo. Appl Environ Microb 63: 2747–2753. |
[66] |
Felley CP, Corthésy-Theulaz I, Rivero JL, et al. (2001) Favourable effect of an acidified milk (LC-1) on Helicobacter pylori gastritis in man. Eur J Gastroenterol Hepatol 13: 25–29. doi: 10.1097/00042737-200101000-00005
![]() |
[67] | Pérez PF, Minnaard J, Rouvet M, et al. (2001) Inhibition ofGiardia intestinalisby extracellularfactors from lactobacilli: An in vitro study. Appl Environ Microb 67: 5037–5042. |
[68] | Schleifer KH, Ludwig W (1995) Phylogenetic relationships of lactic acid bacteria, In: Wood BJB, Holzapfel WH, Editors, The Genera of Lactic Acid Bacteria, London: Chapman & Hall, 7–18. |
[69] | Hassan AN, Frank JF (2001) Starter cultures and their use, In: Marth EH, Steele JL, Editors, Applied Dairy Microbiology, 2 Eds., New York: Marcel Dekker Inc., 151–206. |
[70] | Hammes WP, Vogel RF (1995) The genus Lactobacillus, In: Wood BJB, Holzapfel WH, Editors, The Genera of Lactic Acid Bacteria, London: Blackie Academic and Professional, 19–54. |
[71] |
Park JS, Shin E, Hong H, et al. (2015) Characterization of Lactobacillus fermentum PL9988 isolated from healthy elderly Korean in a longevity village. J Microbiol Biotechnol 25: 1510–1518. doi: 10.4014/jmb.1505.05015
![]() |
[72] | Simpson WJ, Taguchi H (1995) The genus Pediococcus, with notes on the genera Tetratogenococcus and Aerococcus, In: Wood BJB, Holzapfel WH, Editors, The Genera of Lactic Acid Bacteria, London: Chapman & Hall, 125–172. |
[73] |
Beresford TP, Fitzsimons NA, Brennan NL, et al. (2001) Recent advances in cheese microbiology. Int Dairy J 11: 259–274. doi: 10.1016/S0958-6946(01)00056-5
![]() |
[74] | Caldwell S, McMahon DL, Oberg CL, et al. (1996) Development and characterization of lactose-positive Pediococcus species for milk fermentation. Appl Environ Microb 62: 936–941. |
[75] |
Caldwell S, Hutkins RW, McMahon DJ, et al. (1998) Lactose and galactose uptake by genetically engineered Pediococcus species. Appl Microbiol Biot 49: 315–320. doi: 10.1007/s002530051175
![]() |
[76] | Graham DC, McKay LL (1985) Plasmid DNA in strains of Pediococcus cerevisiae and Pediococcus pentosaceus. Appl Environ Microb 50: 532–534. |
[77] | Daeschel MA, Klaenhammer TR (1985) Association of a 13.6-megadalton plasmid in Pediococcus pentosaceus with bacteriocin activity. Appl Environ Microb 50: 1528–1541. |
[78] | Gonzalez CF, Kunka BS (1986) Evidence for plasmid linkage of raffinose utilization and associated α-galactosidase and sucrose hydrolase activity in Pediococcus pentosaceus. Appl Environ Microb 51: 105–109. |
[79] | De Roos J, De Vuyst L (2018) Microbial acidification, alcoholization, and aroma production during spontaneous limbic beer production. J Sci Food Agr 99: 25–38. |
[80] |
Sakamoto K, Margolles A, van Veen HW, et al. (2001) Hop resistance in the beer spoilage bacterium Lactobacillus brevis is mediated by the ATP-binding cassette multidrug transporter HorA. J Bacteriol 183: 5371–5375. doi: 10.1128/JB.183.18.5371-5375.2001
![]() |
[81] |
Snauwaert I, Stragier P, De Vuyst L, et al. (2015) Comparative genome analysis of Pediococcus damnosus LMG 28219, a strain well-adapted to the beer environment. BMC Genomics 16: 267. doi: 10.1186/s12864-015-1438-z
![]() |
[82] |
Suzuki K, Ozaki K, Yamashita H (2004) Comparative analysis of conserved genetic markers and adjacent DNA regions identified in beer spoilage lactic acid bacteria. Lett Appl Microbiol 39: 240–245. doi: 10.1111/j.1472-765X.2004.01572.x
![]() |
[83] | Bergsveinson J, Friesen V, Ziola B (2017) Transcriptome analysis of beer-spoiling Lactobacillus brevis BSO 464 during growth in degassed and gassed beer. Int J Food Microbiol 235: 28–35. |
[84] | Garvie EI (1986) Genus Leuconostoc, In: Sneath PHA, Mair NS, Sharpe ME, et al., Editors, Bergey's Manual of Systematic Bacteriology, 9 Eds., Baltimore: Williams and Wilkins, 1071–1075. |
[85] | Cogan TM, O'Dowd M, Mellerick D (1981) Effects of sugar on acetoin production from citrate by Leuconostoc lactis. Appl Environ Microb 41: 1–8. |
[86] |
Klare I, Werner G, Witte W (2001) Enterococci: Habitats, infections, virulence factors, resistances to antibiotics, transfer of resistance determinants. Contrib Microbiol 8: 108–122. doi: 10.1159/000060406
![]() |
[87] |
Endtz HP, van den Braak N, Verbrugh HA, et al. (1999) Vancomycin resistance: Status quo and quo vadis. Eur J Clin Microbiol 18: 683–690. doi: 10.1007/s100960050379
![]() |
[88] |
Moreno MRF, Sarantinopoulos P, Tsakalidou E, et al. (2006) The role and application of enterococci in food and health. Int J Food Microbiol 106: 1–24. doi: 10.1016/j.ijfoodmicro.2005.06.026
![]() |
[89] |
Cappello MS, Zapparoli G, Logrieco A, et al. (2017) Linking wine lactic acid bacteria diversity with wine aroma and flavor. Int J Food Microbiol 243: 16–27. doi: 10.1016/j.ijfoodmicro.2016.11.025
![]() |
[90] |
Hugenholtz J (2008) The lactic acid bacterium as a cell factory for food ingredient production. Int Dairy J 18: 466–475. doi: 10.1016/j.idairyj.2007.11.015
![]() |
[91] | Hugenholtz J, Kleerebezem M, Starrenburg M, et al. (2000) Lactococcus lactis as a cell-factory for high level diacetyl production. Appl Environ Microb 66: 4112–4114. |
[92] |
Hugenholtz J, Smid EJ (2002) Neutraceutical production with food-grade microorganisms. Curr Opin Biotech 13: 497–507. doi: 10.1016/S0958-1669(02)00367-1
![]() |
[93] |
Hugenholtz J, Sybesma W, Groot MN, et al. (2002) Metabolic engineering of lactic acid bacteria for the production of nutraceuticals. Anton Leeuw 82: 217–235. doi: 10.1023/A:1020608304886
![]() |
[94] |
Hols P, Kleerebezem M, Schranck AN, et al. (1999) Conversion of Lactococcus lactis from homolactic to homoalanine fermentation through metabolic engineering. Nat Biotechnol 17: 588–592. doi: 10.1038/9902
![]() |
[95] | Börner RA, Kandasamy V, Axelsen AM, et al. (2018) High-throughput genome editing tools for lactic acid bacteria: Opportunities for food, feed, pharma and biotech. Available from: www.preprints.org. |
[96] | Johansen E (2018) Use of natural selection and evolution to develop new starter cultures for fermented foods. Annu Rev Food Sci T 9: 411–428. |
[97] | EC (2013) Regulation (EC) No 1829/2003 of the European Parliament and of the council on genetically modified food and feed. Off J Eur Union L 268: 1–23. |
[98] |
Derkx PM, Janzen T, Sørensen KI, et al. (2014) The art of strain improvement of industrial lactic acid bacteria without the use of recombinant DNA technology. Microb Cell Fact 13: S5. doi: 10.1186/1475-2859-13-S1-S5
![]() |
[99] | Bachmann H, Pronk JT, Kleerebezem M, et al. (2015) Evolutionary engineering to enhance starter culture performance in food fermentations. Curr Opin Biotech 32: 1–7. |
[100] |
Zeidan AA, Poulsen VK, Janzen T, et al. (2017) Polysaccharide production by lactic acid bacteria: From genes to industrial applications. FEMS Microbiol Rev 41: S168–S200. doi: 10.1093/femsre/fux017
![]() |
[101] |
De Angelis M, de Candia S, Calasso MP, et al. (2008) Selection and use of autochthonous multiple strain cultures for the manufacture of high-moisture traditional Mozzarella cheese. Int J Food Microbiol 125: 123–132. doi: 10.1016/j.ijfoodmicro.2008.03.043
![]() |
[102] |
Terzić-Vidojević A, Tonković K, Leboš A, et al. (2015) Evaluation of autochthonous lactic acid bacteria as starter cultures for production of white pickled and fresh soft cheeses. LWT-Food Sci Technol 63: 298–306. doi: 10.1016/j.lwt.2015.03.050
![]() |
[103] |
Frau F, Nuñez M, Gerez L, et al. (2016) Development of an autochthonous starter culture for spreadable goat cheese. Food Sci Technol 36: 622–630. doi: 10.1590/1678-457x.08616
![]() |
[104] |
Kargozari M, Moini S, Basti AA, et al. (2014) Effect of autochthonous starter cultures isolated from Siahmazgi cheese on physicochemical, microbiological and volatile compound profiles and sensorial attributes of sucuk, a Turkish dry-fermented sausage. Meat Sci 97: 104–114. doi: 10.1016/j.meatsci.2014.01.013
![]() |
[105] | Casquete R, Benito MJ, Martin A, et al. (2012) Use of autochthonous Pediococcus acidilactici and Staphylococcus vitulus starter cultures in the production of "Chorizo" in 2 different traditional industries. J Food Sci 77: 70–79. |
[106] | Talon R, Leroy S, Lebert I, et al. (2008) Safety improvement and preservation of typical sensory qualities of traditional dry fermented sausages using autochthonous starter cultures. Int J Food Microbiol 126: 227–234. |
[107] | EFSA (2008) Scientific opinion of the panel on biological hazards on the request from EFSA on the maintenance of the list of QPS microorganisms intentionally added to food or feed. EFSA J 928: 1–48. |
1. | Jie Xing, Hanli Zhao, Huiling Chen, Ruoxi Deng, Lei Xiao, Boosting Whale Optimizer with Quasi-Oppositional Learning and Gaussian Barebone for Feature Selection and COVID-19 Image Segmentation, 2023, 20, 1672-6529, 797, 10.1007/s42235-022-00297-8 | |
2. | Nilkanth Mukund Deshpande, Shilpa Gite, Biswajeet Pradhan, Ketan Kotecha, Abdullah Alamri, Improved Otsu and Kapur approach for white blood cells segmentation based on LebTLBO optimization for the detection of Leukemia, 2021, 19, 1551-0018, 1970, 10.3934/mbe.2022093 | |
3. | Rebika Rai, Arunita Das, Krishna Gopal Dhal, Nature-inspired optimization algorithms and their significance in multi-thresholding image segmentation: an inclusive review, 2022, 13, 1868-6478, 889, 10.1007/s12530-022-09425-5 | |
4. | Simrandeep Singh, Harbinder Singh, Gloria Bueno, Oscar Deniz, Sartajvir Singh, Himanshu Monga, P.N. Hrisheekesha, Anibal Pedraza, A Review of Image Fusion: Methods, Applications and Performance Metrics, 2023, 10512004, 104020, 10.1016/j.dsp.2023.104020 | |
5. | Simrandeep Singh, Nitin Mittal, Harbinder Singh, Diego Oliva, Improving the segmentation of digital images by using a modified Otsu’s between-class variance, 2023, 1380-7501, 10.1007/s11042-023-15129-y | |
6. | Nagamani Gonthina, L V Narasimha Prasad, 2024, A Study of Various Optimization Algorithms and Entropy Measures for Image Segmentation, 978-93-80544-51-9, 1828, 10.23919/INDIACom61295.2024.10498431 | |
7. | Emir Turajlic, 2024, Multilevel Image Thresholding based on Particle Swarm Optimization Algorithm with Chaotic Cognitive and Social Acceleration Coefficients, 979-8-3503-8756-8, 1, 10.1109/MECO62516.2024.10577873 | |
8. | Hanyue He, An enhanced seagull algorithm for multi-threshold image segmentation based on Kapur entropy, 2024, 2858, 1742-6588, 012043, 10.1088/1742-6596/2858/1/012043 | |
9. | Saifuddin Ahmed, Anupam Biswas, Abdul Kayom Md Khairuzzaman, An experimentation of objective functions used for multilevel thresholding based image segmentation using particle swarm optimization, 2024, 16, 2511-2104, 1717, 10.1007/s41870-023-01606-y | |
10. | Linguo Li, Mingyu Zhang, Qinghe Li, Shujing Li, 2024, Chapter 33, 978-981-99-9238-6, 334, 10.1007/978-981-99-9239-3_33 |
Algorithm's description | Moth-flame's elements |
Decision variable | Moth's position in each dimension |
Solutions | Moth's position |
Initial solutions | Random positions of moths |
Current solutions | Current positions of moths |
New solutions | New positions of moths |
Best solution | Flame's position |
Fitness function | Distance between moth and flame |
Process of generating a new solution | Flying in a spiral path toward a flame |
Algorithm 1. MFO. |
Randomly initialize the position of moths and the parameters for Moth-flame |
While $iteration \le Max\_iteration$ do |
Renew flame no utilizing Eq (15) |
$OM = FitnessFunction(M)$ |
If $iteration =1$ then |
$F = sort(M)$ |
$OF = sort(OM)$ |
else |
$F = sort({M_{t - 1}}, {M_t})$ |
$OF = sort({M_{t - 1}}, {M_t})$ |
end if |
for $i=1: n$ do |
for $j=1: d$ do |
Renew $ r $ and $ t $ |
Estimate $ D $ utilizing Eq (14) |
Renew $ M(i, j) $ utilizing Eq (13) |
end for |
end for |
end while |
Return the best solution |
Threshold segmentation | MFO |
A collection $ ({x_1}, {x_2}, ..., {x_k}) $ scheduling schemes | A moth population $ ({n_1}, {n_2}, ..., {n_k}) $ moths |
An optimal scheme to resolve the image segmentation | An optimal flame's position |
The objective function of the image segmentation | The fitness function of the MFO |
Algorithm 2. MFO-based Kapur entropy. |
Randomly initialize the position of moths and the parameters for Moth-flame |
While $iteration \le Max\_iteration$ do |
Renew flame no utilizing Eq (15) |
Estimate the fitness value of each moth utilizing Eq (2) for the Kapur entropy and attain the best solution |
$OM = FitnessFunction(M)$ |
If $iteration = 1$ then |
$F = sort(M)$ |
$OF = sort(OM)$ |
else |
$F = sort({M_{t - 1}}, {M_t})$ |
$OF = sort({M_{t - 1}}, {M_t})$ |
end if |
for $i = 1:n$ do |
for $j = 1:d$ do |
Renew $ r $ and $ t $ |
Estimate $ D $ utilizing Eq (14) |
Renew $ M(i, j) $ utilizing Eq (13) |
end for |
end for |
end while |
Return the best solution or the optimal threshold value |
Algorithm | Parameter | Value |
BA [11] | Pulse frequency scope $ f $ | [0, 2] |
Echo loudness $ A $ | 0.25 | |
Reducing coefficient $ \gamma $ | 0.5 | |
FPA [12] | Switch probability $ \rho $ | 0.8 |
MSA [13] | An arbitrary value $ \theta $ | [-2, 1] |
An arbitrary value $ {\varepsilon _2} $ | [0, 1] | |
An arbitrary value $ {\varepsilon _3} $ | [0, 1] | |
An arbitrary value $ {r_1} $ | [0, 1] | |
An arbitrary value $ {r_2} $ | [0, 1] | |
PSO [14] | Constant inertia $ \omega $ | 0.3 |
First acceleration coefficient $ {c_1} $ | 1.4962 | |
Second acceleration coefficient $ {c_2} $ | 1.4962 | |
WWO [15] | Wavelength $ \lambda $ | 0.5 |
Wave height $ {h_{\max }} $ | 6 | |
Wavelength attenuation factor $ \alpha $ | 1.0026 | |
Breaking factor $ \beta $ | [0.001, 0.25] | |
Maximum value $ {k_{\max }} $ of breaking directions | $ min(12, {D \mathord{\left/ {\vphantom {D 2}} \right. } 2}) $ | |
MFO [43] | Logarithmic spiral $ b $ | 1 |
An arbitrary value $ t $ | [-1, 1] |
Images | k | Fitness values | ||||||
BA | FPA | MSA | PSO | WWO | MFO | Rank | ||
Test 1 | 2 | 12.3364 | 12.3198 | 12.2127 | 12.3479 | 12.3112 | 12.3487 | 1 |
3 | 15.2194 | 15.3081 | 15.2704 | 15.2018 | 15.1970 | 15.3172 | 1 | |
4 | 17.7102 | 17.7705 | 17.8821 | 17.7208 | 17.8752 | 17.9921 | 1 | |
5 | 20.3813 | 20.0295 | 20.2961 | 21.1111 | 20.2899 | 21.2798 | 1 | |
6 | 22.4683 | 22.3940 | 21.9694 | 22.4280 | 22.4488 | 22.6422 | 1 | |
Test 2 | 2 | 12.5842 | 12.5331 | 12.5997 | 12.6346 | 12.5916 | 12.6346 | 1 |
3 | 15.6189 | 15.5130 | 15.6003 | 15.4587 | 15.4813 | 15.6531 | 1 | |
4 | 18.1117 | 18.3233 | 18.1107 | 18.3061 | 18.2180 | 18.4397 | 1 | |
5 | 20.5250 | 20.9349 | 20.9719 | 20.9611 | 20.9605 | 21.0539 | 1 | |
6 | 23.1116 | 23.3068 | 23.3549 | 23.2826 | 23.2950 | 23.3837 | 1 | |
Test 3 | 2 | 12.1651 | 12.1623 | 12.1384 | 12.2017 | 12.0067 | 12.2061 | 1 |
3 | 15.3462 | 15.3160 | 14.9498 | 15.2155 | 15.1669 | 15.5039 | 1 | |
4 | 17.9605 | 18.1165 | 18.0610 | 17.8602 | 17.7274 | 18.3107 | 1 | |
5 | 20.0693 | 20.3889 | 20.6938 | 20.7448 | 20.0828 | 20.8056 | 1 | |
6 | 22.2665 | 22.6040 | 22.7055 | 23.0135 | 22.2510 | 24.1228 | 1 | |
Test 4 | 2 | 11.6162 | 11.0138 | 11.6151 | 11.6170 | 11.5649 | 11.6170 | 1 |
3 | 14.4375 | 14.1046 | 14.4277 | 14.4640 | 14.4074 | 14.6363 | 1 | |
4 | 17.1661 | 17.1351 | 17.2543 | 17.2182 | 16.5648 | 17.5047 | 1 | |
5 | 19.3315 | 19.0672 | 19.5998 | 19.9435 | 19.4292 | 20.0589 | 1 | |
6 | 22.1115 | 21.7571 | 21.8045 | 21.9815 | 21.7166 | 22.1257 | 1 | |
Test 5 | 2 | 12.5987 | 9.17771 | 12.4965 | 12.6016 | 12.5880 | 12.6016 | 1 |
3 | 15.8446 | 15.6981 | 15.6613 | 15.7945 | 15.6387 | 15.9201 | 1 | |
4 | 18.6330 | 18.5687 | 18.6837 | 18.7647 | 18.4739 | 18.8308 | 1 | |
5 | 21.5729 | 21.4283 | 21.6418 | 21.3651 | 21.5618 | 21.6809 | 1 | |
6 | 24.1845 | 24.0339 | 24.0773 | 23.7893 | 23.9359 | 24.3074 | 1 | |
Test 6 | 2 | 12.9609 | 12.9185 | 12.9393 | 12.9682 | 12.9668 | 12.9682 | 1 |
3 | 15.9835 | 16.0781 | 16.0526 | 16.1034 | 16.1034 | 16.1252 | 1 | |
4 | 18.5746 | 18.7090 | 18.7443 | 18.6889 | 18.7069 | 19.0165 | 1 | |
5 | 21.3011 | 21.1524 | 21.2343 | 21.0410 | 20.8741 | 21.4711 | 1 | |
6 | 24.1403 | 23.8479 | 23.9266 | 23.7607 | 24.0573 | 24.1842 | 1 | |
Test 7 | 2 | 12.5779 | 12.3517 | 12.5367 | 12.5936 | 12.3939 | 12.5936 | 1 |
3 | 15.3831 | 15.4011 | 15.3516 | 15.3938 | 15.0022 | 15.4274 | 1 | |
4 | 18.3603 | 18.0115 | 17.8258 | 17.9950 | 18.2520 | 18.4235 | 1 | |
5 | 20.9092 | 20.9076 | 20.6714 | 20.5292 | 20.7942 | 21.0217 | 1 | |
6 | 22.8604 | 23.3690 | 23.1421 | 22.9799 | 23.0124 | 23.5484 | 1 | |
Test 8 | 2 | 12.8868 | 12.8947 | 12.8930 | 12.9208 | 12.8957 | 12.9208 | 1 |
3 | 15.8248 | 15.9079 | 15.8297 | 15.8527 | 15.8992 | 16.0552 | 1 | |
4 | 18.5661 | 18.7317 | 18.6622 | 18.7469 | 18.5391 | 19.0449 | 1 | |
5 | 21.2946 | 21.1664 | 21.1186 | 21.1555 | 21.5266 | 21.5719 | 1 | |
6 | 23.5705 | 23.8786 | 24.2027 | 23.8717 | 23.6695 | 24.3206 | 1 | |
Test 9 | 2 | 11.9271 | 11.8657 | 11.4150 | 11.9285 | 11.7951 | 11.9286 | 1 |
3 | 14.7762 | 14.7811 | 14.7560 | 14.8629 | 14.7074 | 14.9331 | 1 | |
4 | 17.1365 | 17.2515 | 17.1703 | 17.3163 | 17.3681 | 17.4062 | 1 | |
5 | 19.8132 | 19.5984 | 19.8790 | 19.7153 | 19.8422 | 19.8973 | 2 | |
6 | 21.9515 | 22.0255 | 22.1325 | 21.8282 | 21.9971 | 22.2318 | 1 | |
Test 10 | 2 | 11.5248 | 11.4683 | 11.4326 | 11.5288 | 11.4273 | 11.5288 | 1 |
3 | 14.3849 | 13.9338 | 14.1944 | 14.4104 | 14.3475 | 14.5453 | 1 | |
4 | 16.8579 | 17.2591 | 17.3456 | 17.3302 | 17.2842 | 17.3904 | 1 | |
5 | 19.3943 | 19.2793 | 18.9721 | 19.3799 | 19.3881 | 19.8757 | 1 | |
6 | 21.5514 | 21.6964 | 21.5692 | 21.3715 | 21.8951 | 22.2800 | 1 |
Images | k | Best threshold values | ||||||
BA | FPA | MSA | PSO | WWO | MFO | |||
Test 1 | 2 | 95, 168 | 95, 171 | 113, 157 | 98, 165 | 103, 173 | 97, 164 | |
3 | 67, 121, 181 | 88, 134, 179 | 76, 133, 176 | 73, 124, 160 | 90, 139, 168 | 81, 126, 174 | ||
4 | 68, 110, 130, 163 | 60, 103, 160, 200 | 55, 91, 139, 180 | 61, 83, 116, 181 | 79, 121, 146, 175 | 73, 112, 147, 184 | ||
5 | 60, 98, 144, 172, 204 |
46, 65, 109, 142, 176 |
78, 98, 135, 157, 183 |
39, 100, 163, 165, 239 |
63, 91, 144, 168, 187 |
65, 101, 125, 165, 239 |
||
6 | 38, 58, 98, 140, 171, 195 |
71, 89, 127, 176, 194, 216 |
44, 91, 101, 133, 155, 200 |
66, 106, 137, 159, 196, 224 |
66, 115, 140, 162, 178, 193 |
77, 98, 132, 156, 181, 208 |
||
Test 2 | 2 | 62, 148 | 64, 121 | 79, 138 | 75, 147 | 66, 153 | 75, 147 | |
3 | 76, 132, 174 | 42, 83, 158 | 76, 119, 158 | 45, 130, 169 | 43, 109, 146 | 59, 106, 170 | ||
4 | 43, 86, 144, 206 | 55, 108, 133, 190 | 62, 89, 118, 192 | 53, 121, 152, 188 | 47, 118, 159, 203 | 50, 84, 141, 179 | ||
5 | 24, 93, 134, 173, 192 |
35, 93, 126, 155, 191 |
32, 79, 101, 136, 188 |
34, 73, 95, 129, 176 |
56, 84, 104, 145, 175 |
50, 80, 119, 163, 204 |
||
6 | 35, 80, 106, 137, 191, 203 |
56, 101, 126, 160, 177, 200 |
44, 76, 122, 162, 177, 196 |
22, 38, 82, 110, 151, 193 |
65, 83, 107, 145, 179, 199 |
37, 52, 76, 126, 169, 195 |
||
Test 3 | 2 | 82, 162 | 88, 164 | 85, 157 | 69, 177 | 110, 163 | 69, 174 | |
3 | 87, 135, 186 | 62, 141, 178 | 102, 159, 184 | 73, 141, 195 | 81, 154, 192 | 69, 127, 183 | ||
4 | 89, 123, 152, 189 | 64, 114, 151, 176 | 54, 95, 135, 169 | 68, 120, 134, 185 | 38, 93, 148, 192 | 66, 106, 146, 186 | ||
5 | 78, 101, 118, 133, 186 |
67, 98, 135, 149, 174 |
56, 80, 111, 152, 194 |
34, 87, 165, 182, 231 |
75, 101, 132, 169, 213 |
70, 104, 132, 160, 190 |
||
6 | 82, 114, 131, 154, 174, 212 |
49, 80, 94, 114, 174, 198 |
41, 53, 73, 113, 146, 181 |
55, 93, 128, 153, 183, 196 |
58, 71, 78, 118, 147, 181 |
56, 69, 127, 163, 183, 231 |
||
Test 4 | 2 | 101, 174 | 146, 164 | 95, 175 | 98, 174 | 93, 154 | 95, 171 | |
3 | 72, 136, 169 | 117, 177, 212 | 69, 115, 180 | 99, 152, 181 | 70, 113, 169 | 85, 137, 180 | ||
4 | 41, 84, 115, 164 | 50, 80, 146, 183 | 43, 91, 124, 172 | 40, 86, 133, 167 | 63, 96, 159, 172 | 38, 86, 138, 180 | ||
5 | 49, 82, 122, 181, 224 |
29, 75, 104, 120, 205 |
49, 75, 105, 160, 181 |
32, 91, 125, 171, 208 |
37, 100, 116, 152, 210 |
43, 98, 125, 173, 245 |
||
6 | 75, 102, 125, 161, 188, 237 |
43, 110, 129, 150, 181, 211 |
79, 120, 135, 167, 221, 237 |
43, 59, 85, 127, 183, 212 |
32, 61, 78, 122, 148, 211 |
28, 53, 77, 114, 146, 182 |
||
Test 5 | 2 | 74, 135 | 110, 248 | 83, 157 | 70, 135 | 64, 129 | 70, 135 | |
3 | 56, 134, 215 | 61, 120, 184 | 59, 145, 230 | 62, 119, 197 | 80, 127, 180 | 69, 133, 204 | ||
4 | 52, 131, 206, 231 | 95, 145, 200, 234 | 70, 94, 146, 207 | 74, 127, 186, 226 | 81, 114, 190, 221 | 70, 108, 150, 216 | ||
5 | 61, 110, 168, 199, 231 |
33, 71, 97, 154, 225 |
37, 101, 137, 179, 217 |
21, 79, 150, 195, 231 |
63, 93, 134, 188, 233 |
41, 94, 131, 177, 225 |
||
6 | 29, 62, 117, 143, 188, 211 |
23, 75, 110, 142, 208, 238 |
38, 61, 127, 167, 212, 233 |
51, 84, 124, 147, 187, 200 |
32, 72, 91, 116, 192, 228 |
27, 77, 109, 140, 179, 225 |
||
Test 6 | 2 | 84, 168 | 111, 175 | 106, 175 | 91, 170 | 92, 171 | 91, 170 | |
3 | 55, 126, 191 | 84, 145, 188 | 85, 138, 200 | 71, 124, 188 | 68, 128, 177 | 75, 131, 185 | ||
4 | 37, 117, 168, 211 | 60, 137, 164, 194 | 39, 77, 132, 186 | 94, 121, 157, 214 | 54, 139, 178, 219 | 68, 105, 157, 204 | ||
5 | 50, 75, 110, 184, 206 |
41, 82, 122, 189, 234 |
35, 78, 131, 156, 183 |
37, 124, 154, 182, 208 |
42, 108, 120, 179, 205 |
80, 111, 132, 163, 204 |
||
6 | 59, 89, 114, 146, 187, 207 |
32, 58, 116, 146, 186, 214 |
60, 80, 105, 135, 168, 189 |
47, 88, 111, 165, 191, 204 |
44, 65, 113, 146, 170, 199 |
42, 84, 116, 136, 170, 209 |
||
Test 7 | 2 | 97, 166 | 124, 164 | 97, 170 | 96, 163 | 70, 156 | 96, 163 | |
3 | 75, 123, 158 | 53, 94, 176 | 54, 130, 162 | 80, 107, 170 | 62, 178, 219 | 81, 166, 198 | ||
4 | 71, 127, 166, 218 | 66, 121, 145, 184 | 77, 129, 187, 236 | 43, 110, 162, 181 | 95, 123, 166, 209 | 46, 104, 167, 197 | ||
5 | 46, 87, 123, 161, 225 |
40, 99, 130, 175, 198 |
40, 59, 97, 143, 196 |
24, 73, 137, 166, 214 |
65, 122, 146, 175, 214 |
79, 107, 137, 166, 212 |
||
6 | 37, 69, 131, 145, 174, 236 |
47, 63, 112, 162, 186, 223 |
53, 101, 125, 160, 202, 214 |
62, 123, 149, 174, 190, 233 |
62, 110, 167, 193, 204, 233 |
44, 82, 132, 171, 200, 234 |
||
Test 8 | 2 | 91, 170 | 83, 162 | 100, 168 | 93, 161 | 94, 169 | 93, 161 | |
3 | 80, 164, 225 | 109, 165, 201 | 66, 102, 157 | 69, 105, 171 | 59, 114, 161 | 95, 159, 206 | ||
4 | 46, 104, 144, 217 | 73, 109, 175, 225 | 83, 136, 177, 203 | 42, 109, 159, 191 | 47, 81, 124, 156 | 65, 111, 160, 207 | ||
5 | 62, 120, 172, 204, 223 |
36, 55, 115, 157, 217 |
80, 143, 175, 209, 231 |
55, 112, 132, 173, 194 |
68, 102, 131, 164, 223 |
74, 107, 159, 196, 223 |
||
6 | 29, 47, 87, 110, 157, 212 |
58, 85, 113, 133, 165, 229 |
52, 75, 102, 131, 160, 205 |
59, 74, 108, 145, 171, 204 |
58, 98, 144, 157, 181, 201 |
50, 85, 126, 158, 202, 227 |
||
Test 9 | 2 | 103, 155 | 93, 160 | 128, 163 | 104, 156 | 92, 166 | 105, 156 | |
3 | 110, 152, 213 | 92, 152, 212 | 104, 166, 207 | 111, 153, 193 | 69, 115, 167 | 104, 154, 203 | ||
4 | 55, 105, 135, 161 | 44, 86, 150, 205 | 55, 96, 121, 174 | 97, 132, 155, 192 | 63, 99, 173, 203 | 57, 106, 172, 201 | ||
5 | 96, 117, 150, 179, 298 |
92, 121, 136, 152, 204 |
56, 91, 114, 165, 220 |
56, 116, 153, 169, 186 |
92, 129, 154, 172, 201 |
61, 84, 102, 163, 197 |
||
6 | 45, 74, 86, 120, 161, 220 |
66, 84, 96, 128, 156, 184 |
72, 95, 116, 162, 172, 209 |
78, 96, 107, 133, 151, 199 |
53, 84, 102, 111, 165, 211 |
56, 82, 115, 164, 187, 198 |
||
Test 10 | 2 | 74, 148 | 72, 138 | 77, 133 | 75, 153 | 96, 168 | 75, 153 | |
3 | 86, 154, 184 | 75, 104, 213 | 84, 129, 211 | 71, 154, 196 | 97, 143, 196 | 83, 143, 187 | ||
4 | 76, 95, 171, 222 | 83, 125, 164, 203 | 77, 123, 164, 213 | 74, 112, 138, 177 | 74, 123, 156, 210 | 72, 116, 151, 181 | ||
5 | 94, 132, 147, 188, 222 |
85, 146, 168, 202, 230 |
71, 88, 101, 179, 213 |
50, 83, 152, 179, 248 |
81, 132, 174, 214, 230 |
74, 105, 136, 163, 186 |
||
6 | 70, 82, 114, 172, 194, 230 |
74, 110, 126, 163, 194, 203 |
89, 120, 133, 167, 180, 223 |
64, 81, 128, 162, 202, 230 |
72, 126, 162, 192, 213, 233 |
75, 103, 144, 176, 191, 222 |
Images | k | Execution time (in second) | ||||||
BA | FPA | MSA | PSO | WWO | MFO | Rank | ||
Test 1 | 2 | 2.0504 | 2.5449 | 4.1430 | 3.2668 | 2.6219 | 1.8838 | 1 |
3 | 2.1806 | 2.4652 | 3.6404 | 3.7600 | 3.0271 | 2.1470 | 1 | |
4 | 2.3493 | 2.5466 | 3.6591 | 4.2612 | 3.5488 | 2.3476 | 1 | |
5 | 2.3526 | 2.4754 | 3.6553 | 4.4621 | 4.1037 | 2.3232 | 1 | |
6 | 2.4534 | 2.5140 | 3.6961 | 4.7721 | 4.6332 | 2.4476 | 1 | |
Test 2 | 2 | 2.0546 | 2.2233 | 3.5972 | 3.0943 | 2.4066 | 1.9862 | 1 |
3 | 2.2834 | 2.4729 | 3.7247 | 3.7189 | 3.0388 | 2.2371 | 1 | |
4 | 2.4856 | 2.4565 | 3.5933 | 4.0514 | 3.7730 | 2.3832 | 1 | |
5 | 2.6244 | 2.5519 | 3.6495 | 13.892 | 4.2132 | 2.5896 | 2 | |
6 | 2.7085 | 2.6344 | 3.6366 | 4.6383 | 4.9811 | 2.6217 | 1 | |
Test 3 | 2 | 2.0428 | 2.1661 | 3.5012 | 10.081 | 2.3834 | 2.0128 | 1 |
3 | 2.1067 | 2.2636 | 3.6970 | 3.5344 | 2.9690 | 2.1931 | 2 | |
4 | 2.3168 | 2.4309 | 3.6582 | 4.1890 | 3.5014 | 2.2318 | 1 | |
5 | 2.4964 | 2.4430 | 3.6781 | 4.2050 | 4.1567 | 2.4421 | 1 | |
6 | 2.5751 | 2.5232 | 3.7181 | 4.4494 | 4.7794 | 2.5211 | 1 | |
Test 4 | 2 | 2.1245 | 2.2784 | 3.5689 | 3.1980 | 2.4759 | 1.8874 | 1 |
3 | 2.2841 | 2.3142 | 3.6728 | 3.9206 | 3.1605 | 2.1235 | 1 | |
4 | 2.4620 | 2.4784 | 3.7133 | 4.4914 | 3.6540 | 2.4128 | 1 | |
5 | 2.5981 | 2.5782 | 3.7064 | 4.5182 | 4.2552 | 2.5252 | 1 | |
6 | 2.5760 | 2.6113 | 3.7157 | 4.6266 | 4.6624 | 2.5645 | 1 | |
Test 5 | 2 | 2.1369 | 2.3193 | 3.6874 | 3.3801 | 2.5160 | 1.9748 | 1 |
3 | 2.3770 | 2.4655 | 3.8206 | 3.9882 | 3.1202 | 2.1927 | 1 | |
4 | 2.6246 | 2.5708 | 3.7468 | 4.6477 | 3.7042 | 2.4725 | 1 | |
5 | 2.6867 | 2.6756 | 3.7018 | 4.8451 | 4.2924 | 2.6573 | 1 | |
6 | 3.0574 | 2.6619 | 3.7099 | 5.2076 | 4.9496 | 2.6989 | 2 | |
Test 6 | 2 | 2.0659 | 2.2878 | 3.7082 | 3.4298 | 2.4881 | 1.8933 | 1 |
3 | 2.3009 | 2.4216 | 3.7141 | 3.7289 | 3.1803 | 2.1388 | 1 | |
4 | 2.5690 | 2.5297 | 3.7186 | 4.4016 | 3.6365 | 2.5144 | 1 | |
5 | 2.7012 | 2.7481 | 3.8449 | 4.6251 | 4.4892 | 2.5788 | 1 | |
6 | 2.7363 | 2.6549 | 3.5908 | 5.0158 | 5.0763 | 2.6390 | 1 | |
Test 7 | 2 | 2.1999 | 2.2860 | 3.7677 | 3.4719 | 2.5184 | 1.7930 | 1 |
3 | 2.3913 | 2.4617 | 3.8282 | 4.4295 | 3.1260 | 2.1823 | 1 | |
4 | 2.5758 | 2.5069 | 3.6892 | 4.5830 | 3.6773 | 2.5712 | 2 | |
5 | 2.8661 | 2.6928 | 3.7420 | 4.9113 | 4.3654 | 2.6331 | 1 | |
6 | 2.8314 | 2.7552 | 3.8166 | 4.7869 | 4.8823 | 2.7347 | 1 | |
Test 8 | 2 | 2.1196 | 2.2375 | 3.5308 | 3.2347 | 2.6986 | 1.8208 | 1 |
3 | 2.1790 | 2.4691 | 3.6472 | 4.1744 | 3.2448 | 2.0801 | 1 | |
4 | 2.4523 | 2.5377 | 3.6976 | 4.4788 | 3.7100 | 2.3825 | 1 | |
5 | 2.5949 | 2.5873 | 3.7993 | 4.6852 | 4.3499 | 2.5537 | 1 | |
6 | 2.6545 | 2.6189 | 3.7323 | 5.1430 | 4.7387 | 2.5936 | 1 | |
Test 9 | 2 | 2.1158 | 2.2435 | 3.7132 | 3.0533 | 2.3750 | 1.8010 | 1 |
3 | 2.2377 | 2.3223 | 3.7861 | 3.8355 | 2.9901 | 2.1133 | 1 | |
4 | 2.3979 | 2.4493 | 3.6818 | 4.1490 | 3.5694 | 2.3356 | 1 | |
5 | 2.4748 | 2.4971 | 3.6920 | 4.3074 | 4.2244 | 2.4209 | 1 | |
6 | 2.4707 | 2.5587 | 3.7407 | 4.6462 | 4.5649 | 2.5010 | 2 | |
Test 10 | 2 | 2.0775 | 2.1429 | 3.4231 | 3.0237 | 2.3839 | 1.6726 | 1 |
3 | 2.2550 | 2.2918 | 3.5819 | 3.4502 | 2.9528 | 2.2082 | 1 | |
4 | 2.3523 | 2.3987 | 3.6269 | 4.0056 | 3.4883 | 2.3009 | 1 | |
5 | 2.3653 | 2.4887 | 3.7718 | 4.1497 | 4.0963 | 2.3358 | 1 | |
6 | 2.42209 | 2.5584 | 3.7138 | 4.3912 | 4.6485 | 2.4192 | 1 |
Images | k | PSNR values | ||||||
BA | FPA | MSA | PSO | WWO | MFO | Rank | ||
Test 1 | 2 | 54.2052 | 54.0193 | 52.6804 | 53.9194 | 53.3728 | 54.2052 | 1 |
3 | 55.2116 | 54.7434 | 55.5773 | 55.8071 | 54.6025 | 56.2391 | 1 | |
4 | 56.1645 | 56.9214 | 55.8071 | 56.8053 | 55.3545 | 57.8253 | 1 | |
5 | 56.9214 | 57.2139 | 55.4283 | 67.4037 | 56.5877 | 61.6781 | 2 | |
6 | 57.5946 | 55.9464 | 63.0931 | 56.3249 | 56.3249 | 68.3558 | 1 | |
Test 2 | 2 | 56.8302 | 55.6667 | 55.2570 | 55.6667 | 56.4413 | 56.8496 | 1 |
3 | 55.5716 | 55.8390 | 55.5716 | 56.9284 | 58.4671 | 58.5125 | 1 | |
4 | 56.8302 | 57.5587 | 56.8302 | 57.7738 | 57.7738 | 58.6671 | 1 | |
5 | 60.8783 | 57.6678 | 59.6180 | 57.5587 | 57.4521 | 58.5125 | 3 | |
6 | 58.7405 | 57.4521 | 58.5890 | 61.3621 | 56.5356 | 59.3017 | 2 | |
Test 3 | 2 | 61.6543 | 60.6947 | 61.1888 | 59.1452 | 57.4414 | 63.7976 | 1 |
3 | 60.8653 | 64.1985 | 58.3970 | 63.3619 | 61.8294 | 65.2923 | 1 | |
4 | 60.5327 | 65.0068 | 64.6841 | 64.3602 | 66.0216 | 66.2020 | 1 | |
5 | 62.3681 | 65.6347 | 65.9875 | 70.5641 | 62.9357 | 67.5475 | 2 | |
6 | 61.6543 | 66.8489 | 65.6347 | 66.0853 | 65.7478 | 68.2531 | 1 | |
Test 4 | 2 | 53.7298 | 51.8359 | 53.9307 | 53.8281 | 53.3635 | 54.0080 | 1 |
3 | 55.7727 | 53.2812 | 56.2939 | 53.7936 | 54.4415 | 56.3931 | 1 | |
4 | 69.8724 | 64.0939 | 68.4585 | 70.6684 | 57.7874 | 70.6684 | 1 | |
5 | 64.6339 | 76.5339 | 64.6339 | 75.2461 | 54.0512 | 72.8879 | 3 | |
6 | 55.3686 | 68.4585 | 62.6342 | 59.8785 | 71.2461 | 71.5255 | 1 | |
Test 5 | 2 | 55.9353 | 54.4501 | 55.5723 | 56.1030 | 56.1944 | 56.3859 | 1 |
3 | 56.1489 | 56.5395 | 56.6408 | 56.1489 | 55.6932 | 56.7947 | 1 | |
4 | 56.0035 | 55.0787 | 56.1030 | 55.9353 | 55.6534 | 56.4888 | 1 | |
5 | 57.1093 | 57.6783 | 57.8691 | 59.3191 | 56.4366 | 58.1327 | 2 | |
6 | 58.4300 | 59.0280 | 57.8027 | 57.0566 | 57.3278 | 59.0280 | 1 | |
Test 6 | 2 | 52.4037 | 50.9764 | 51.2852 | 52.4037 | 52.3196 | 52.9992 | 1 |
3 | 53.7675 | 52.9992 | 52.9146 | 54.0808 | 54.3233 | 55.5443 | 1 | |
4 | 56.9588 | 55.0405 | 54.3233 | 52.1517 | 55.6405 | 57.5981 | 1 | |
5 | 55.2454 | 54.8447 | 55.8429 | 57.9588 | 56.4138 | 58.3841 | 1 | |
6 | 55.1430 | 57.0950 | 54.9352 | 56.4138 | 56.7949 | 59.1946 | 1 | |
Test 7 | 2 | 52.1853 | 50.1439 | 52.1853 | 52.2305 | 52.2305 | 53.6248 | 1 |
3 | 53.1369 | 52.7825 | 54.3929 | 52.8286 | 54.1279 | 54.4252 | 1 | |
4 | 53.5238 | 53.9356 | 52.9913 | 54.1279 | 52.2737 | 54.9081 | 1 | |
5 | 54.7169 | 55.1408 | 54.7169 | 52.7825 | 53.9928 | 57.7724 | 1 | |
6 | 54.4130 | 54.6643 | 54.4252 | 54.4600 | 54.1279 | 55.1408 | 1 | |
Test 8 | 2 | 51.2625 | 51.1401 | 50.7696 | 51.1401 | 51.0819 | 51.8484 | 1 |
3 | 52.1217 | 50.3589 | 53.9395 | 53.4810 | 51.0247 | 55.1889 | 1 | |
4 | 58.3667 | 52.9207 | 51.8484 | 52.6689 | 58.1344 | 59.3198 | 1 | |
5 | 54.6097 | 54.7941 | 52.1217 | 60.9018 | 53.6305 | 61.3007 | 1 | |
6 | 62.1126 | 55.4021 | 56.8943 | 55.1889 | 55.4021 | 64.4974 | 1 | |
Test 9 | 2 | 54.7684 | 56.0844 | 50.9610 | 54.6524 | 54.5365 | 56.2376 | 1 |
3 | 53.9616 | 56.2376 | 54.6524 | 53.8444 | 54.6524 | 61.7530 | 1 | |
4 | 67.6683 | 73.5879 | 67.6683 | 55.5133 | 58.1149 | 68.0342 | 2 | |
5 | 62.8685 | 59.5786 | 67.1721 | 67.1721 | 56.2376 | 73.5879 | 1 | |
6 | 72.9963 | 62.8685 | 68.6413 | 57.8977 | 68.6413 | 79.2437 | 1 | |
Test 10 | 2 | 49.4261 | 49.4702 | 49.3850 | 49.4109 | 49.2129 | 49.4809 | 1 |
3 | 49.3039 | 49.4109 | 49.3191 | 49.3437 | 49.2012 | 49.4261 | 1 | |
4 | 49.3976 | 49.3267 | 49.3850 | 49.4261 | 49.4261 | 49.5080 | 1 | |
5 | 49.2362 | 49.3115 | 49.4261 | 49.1974 | 49.3437 | 49.5080 | 1 | |
6 | 49.5634 | 49.4261 | 49.2816 | 49.3850 | 49.4702 | 50.5952 | 1 |
Images | k | SSIM values | ||||||
BA | FPA | MSA | PSO | WWO | MFO | Rank | ||
Test 1 | 2 | 0.5811 | 0.5630 | 0.4666 | 0.5589 | 0.5389 | 0.5827 | 1 |
3 | 0.6174 | 0.6028 | 0.6291 | 0.6196 | 0.5792 | 0.6677 | 1 | |
4 | 0.6275 | 0.6713 | 0.6450 | 0.6841 | 0.6040 | 0.6962 | 1 | |
5 | 0.6706 | 0.6692 | 0.6240 | 0.6779 | 0.6761 | 0.7428 | 1 | |
6 | 0.6579 | 0.6752 | 0.7295 | 0.6565 | 0.6272 | 0.7853 | 1 | |
Test 2 | 2 | 0.5934 | 0.5618 | 0.5486 | 0.5624 | 0.5854 | 0.5951 | 1 |
3 | 0.5849 | 0.5892 | 0.5737 | 0.6229 | 0.6440 | 0.6549 | 1 | |
4 | 0.6118 | 0.6431 | 0.6084 | 0.6336 | 0.6302 | 0.6680 | 1 | |
5 | 0.6700 | 0.6321 | 0.6721 | 0.6239 | 0.6315 | 0.6953 | 1 | |
6 | 0.6764 | 0.6305 | 0.6926 | 0.7184 | 0.6484 | 0.7095 | 2 | |
Test 3 | 2 | 0.7776 | 0.7700 | 0.7735 | 0.7433 | 0.7326 | 0.7782 | 1 |
3 | 0.7694 | 0.7963 | 0.7380 | 0.7321 | 0.7388 | 0.8075 | 1 | |
4 | 0.7661 | 0.8171 | 0.8107 | 0.7983 | 0.7966 | 0.8286 | 1 | |
5 | 0.8008 | 0.7352 | 0.8087 | 0.7841 | 0.7682 | 0.8266 | 1 | |
6 | 0.7468 | 0.7928 | 0.8434 | 0.7941 | 0.8482 | 0.8560 | 1 | |
Test 4 | 2 | 0.5682 | 0.4613 | 0.5752 | 0.5724 | 0.5494 | 0.5840 | 1 |
3 | 0.6295 | 0.5643 | 0.6447 | 0.5538 | 0.5765 | 0.6573 | 1 | |
4 | 0.7995 | 0.7457 | 0.8018 | 0.7963 | 0.7050 | 0.8387 | 1 | |
5 | 0.8021 | 0.7892 | 0.7802 | 0.8431 | 0.5767 | 0.8509 | 1 | |
6 | 0.6250 | 0.8207 | 0.8056 | 0.7640 | 0.8148 | 0.8493 | 1 | |
Test 5 | 2 | 0.5874 | 0.5694 | 0.5478 | 0.5923 | 0.5947 | 0.6025 | 1 |
3 | 0.6513 | 0.6167 | 0.6558 | 0.6536 | 0.5760 | 0.6752 | 1 | |
4 | 0.6629 | 0.5762 | 0.6594 | 0.6147 | 0.6122 | 0.6669 | 1 | |
5 | 0.6686 | 0.6775 | 0.6918 | 0.7207 | 0.6737 | 0.7221 | 1 | |
6 | 0.7354 | 0.7299 | 0.7183 | 0.6835 | 0.7114 | 0.7593 | 1 | |
Test 6 | 2 | 0.3856 | 0.3073 | 0.3285 | 0.3856 | 0.3823 | 0.4098 | 1 |
3 | 0.4862 | 0.4423 | 0.4438 | 0.5025 | 0.5060 | 0.5417 | 1 | |
4 | 0.6023 | 0.5147 | 0.5425 | 0.4154 | 0.5323 | 0.6141 | 1 | |
5 | 0.5798 | 0.5765 | 0.5771 | 0.5794 | 0.6314 | 0.6562 | 1 | |
6 | 0.6170 | 0.6676 | 0.6141 | 0.6412 | 0.6737 | 0.7035 | 1 | |
Test 7 | 2 | 0.5412 | 0.2944 | 0.5452 | 0.5357 | 0.5357 | 0.6049 | 1 |
3 | 0.5439 | 0.5643 | 0.6308 | 0.5533 | 0.6497 | 0.6713 | 1 | |
4 | 0.5819 | 0.5867 | 0.5474 | 0.6396 | 0.5011 | 0.6571 | 1 | |
5 | 0.6752 | 0.6858 | 0.6817 | 0.5044 | 0.5725 | 0.7308 | 1 | |
6 | 0.6826 | 0.6776 | 0.6360 | 0.6650 | 0.5908 | 0.6938 | 1 | |
Test 8 | 2 | 0.3781 | 0.3656 | 0.3403 | 0.3656 | 0.3661 | 0.4100 | 1 |
3 | 0.4250 | 0.2956 | 0.4948 | 0.4927 | 0.3533 | 0.5583 | 1 | |
4 | 0.6528 | 0.4934 | 0.3981 | 0.4760 | 0.6109 | 0.6803 | 1 | |
5 | 0.5484 | 0.5568 | 0.3853 | 0.4986 | 0.5249 | 0.6793 | 1 | |
6 | 0.7181 | 0.5974 | 0.6489 | 0.6071 | 0.5787 | 0.7293 | 1 | |
Test 9 | 2 | 0.5502 | 0.6010 | 0.2334 | 0.5494 | 0.5547 | 0.6075 | 1 |
3 | 0.4929 | 0.5707 | 0.5475 | 0.4852 | 0.5360 | 0.6251 | 1 | |
4 | 0.5860 | 0.6949 | 0.6196 | 0.4427 | 0.5586 | 0.7198 | 1 | |
5 | 0.6465 | 0.6827 | 0.7318 | 0.6505 | 0.4812 | 0.7441 | 1 | |
6 | 0.7208 | 0.6556 | 0.7322 | 0.5454 | 0.7166 | 0.7698 | 1 | |
Test 10 | 2 | 0.1722 | 0.1666 | 0.1571 | 0.1729 | 0.1502 | 0.1733 | 1 |
3 | 0.1611 | 0.1719 | 0.1644 | 0.1708 | 0.1475 | 0.1772 | 1 | |
4 | 0.1822 | 0.1678 | 0.1775 | 0.1753 | 0.1804 | 0.1927 | 1 | |
5 | 0.1486 | 0.1511 | 0.1786 | 0.6364 | 0.1645 | 0.1915 | 2 | |
6 | 0.2091 | 0.1812 | 0.1565 | 0.1697 | 0.1755 | 0.2901 | 1 |
Images | k | Wilcoxon test | ||||
MFO vs BA | MFO vs FPA | MFO vs MSA | MFO vs PSO | MFO vs WWO | ||
Test 1 | 2 | 4.1486E-08 | 2.9135E-07 | 3.7215E-07 | 1.0905E-02 | 4.9558E-08 |
3 | 4.1406E-03 | 8.8674E-01 | 2.7328E-02 | 5.9956E-04 | 1.8056E-02 | |
4 | 3.9271E-03 | 6.8298E-03 | 2.3871E-05 | 5.2530E-04 | 5.9054E-05 | |
5 | 3.5064E-03 | 4.4599E-02 | 7.7394E-06 | 2.5972E-04 | 7.7394E-06 | |
6 | 1.5429E-04 | 1.6017E-04 | 2.1821E-11 | 1.6475E-04 | 2.1821E-11 | |
Test 2 | 2 | 1.5649E-11 | 4.1040E-11 | 4.5618E-11 | 5.7016E-03 | 2.6819E-11 |
3 | 3.7061E-02 | 1.4931E-03 | 4.5940E-02 | 1.5957E-02 | 7.3007E-03 | |
4 | 2.1572E-02 | 7.2382E-04 | 1.1435E-02 | 9.2276E-03 | 1.8454E-02 | |
5 | 3.0221E-07 | 1.2754E-02 | 4.4680E-10 | 3.9238E-02 | 4.4680E-10 | |
6 | 2.1287E-05 | 6.0511E-04 | 4.8389E-10 | 1.9143E-02 | 4.8389E-10 | |
Test 3 | 2 | 1.6510E-11 | 1.2118E-12 | 1.2118E-12 | 2.7793E-03 | 1.2118E-12 |
3 | 1.7047E-02 | 7.9323E-05 | 9.8208E-03 | 5.1474E-03 | 3.8910E-02 | |
4 | 1.5568E-02 | 4.8012E-02 | 6.1697E-05 | 6.0010E-03 | 1.8438E-03 | |
5 | 1.2075E-04 | 1.1553E-03 | 8.9553E-08 | 8.6976E-06 | 8.9553E-08 | |
6 | 4.2458E-05 | 1.6691E-02 | 3.9562E-10 | 1.4187E-05 | 3.9562E-10 | |
Test 4 | 2 | 1.4119E-10 | 3.6912E-11 | 4.5618E-11 | 2.7605E-02 | 1.5672E-11 |
3 | 9.6851E-08 | 1.0617E-03 | 1.3782E-02 | 1.8815E-02 | 2.0296E-11 | |
4 | 2.3687E-03 | 2.6206E-03 | 3.3040E-02 | 2.2534E-02 | 1.8354E-02 | |
5 | 1.3187E-02 | 6.9403E-03 | 6.0393E-02 | 3.3072E-02 | 1.3187E-02 | |
6 | 1.0490E-02 | 3.1661E-03 | 6.3076E-09 | 6.0661E-05 | 3.0311E-04 | |
Test 5 | 2 | 4.7773E-09 | 1.4148E-08 | 1.8780E-09 | 6.9419E-03 | 1.0569E-09 |
3 | 1.0226E-06 | 1.5514E-03 | 9.3662E-04 | 1.7753E-02 | 2.8427E-10 | |
4 | 1.2338E-04 | 6.8037E-03 | 9.0570E-05 | 6.0755E-04 | 6.6120E-03 | |
5 | 1.9974E-02 | 1.5408E-02 | 1.9284E-05 | 1.8299E-02 | 1.1010E-02 | |
6 | 2.3675E-02 | 1.9315E-02 | 9.3069E-08 | 2.8298E-02 | 2.0096E-02 | |
Test 6 | 2 | 5.4162E-09 | 5.4162E-09 | 2.4736E-08 | 2.8298E-02 | 8.3512E-09 |
3 | 1.2298E-07 | 1.5879E-06 | 3.6108E-02 | 7.8961E-03 | 7.6552E-10 | |
4 | 1.3692E-02 | 1.2204E-02 | 6.7678E-04 | 3.3418E-02 | N/A | |
5 | 1.5319E-02 | 1.3228E-05 | 3.7759E-02 | 8.2918E-03 | 8.0670E-03 | |
6 | 7.9518E-01 | 7.6105E-05 | 8.8807E-06 | 1.6308E-03 | 7.6285E-03 | |
Test 7 | 2 | 1.1999E-12 | 1.2118E-12 | 1.2118E-12 | 5.5398E-03 | 1.2118E-12 |
3 | 1.0043E-08 | 3.2704E-04 | 1.7181E-04 | 4.5838E-04 | 1.8783E-11 | |
4 | 2.2155E-05 | 1.3080E-04 | 2.0545E-03 | 1.8360E-02 | 1.8586E-03 | |
5 | 9.2817E-05 | 5.9629E-04 | 2.6611E-02 | 2.7791E-02 | 7.1075E-03 | |
6 | 8.1845E-01 | 1.8944E-02 | 1.0237E-07 | 7.3815E-04 | 2.6871E-02 | |
Test 8 | 2 | 1.1067E-08 | 1.2380E-09 | 1.2377E-09 | 9.5909E-03 | 1.2377E-09 |
3 | 5.0104E-03 | 5.7108E-04 | 7.4568E-04 | 2.5069E-02 | 7.4568E-03 | |
4 | 6.0746E-03 | 7.7156E-05 | 4.5418E-04 | 1.2662E-02 | 7.5196E-03 | |
5 | 1.2619E-02 | 7.7958E-05 | 4.3177E-10 | 5.6943E-07 | 4.3177E-10 | |
6 | 1.0049E-02 | 1.1403E-02 | 2.5562E-11 | 9.6312E-05 | 2.5562E-11 | |
Test 9 | 2 | 4.1906E-10 | 7.2256E-10 | 1.2384E-09 | 4.5164E-02 | 1.1236E-09 |
3 | 2.0185E-02 | 5.6566E-03 | 6.7067E-03 | 8.2284E-02 | N/A | |
4 | 1.7717E-02 | 8.1726E-03 | 2.2107E-06 | 2.0015E-02 | 7.4528E-03 | |
5 | 1.2740E-02 | 3.7302E-05 | 4.5563E-10 | 2.6262E-05 | 4.5563E-10 | |
6 | 1.4911E-02 | 1.7368E-03 | 4.3348E-10 | 9.9941E-04 | 4.3348E-10 | |
Test 10 | 2 | 2.5301E-10 | 1.2384E-09 | 1.2384E-09 | N/A | 7.5947E-10 |
3 | 8.1850E-03 | 1.5376E-02 | 1.4949E-02 | 9.7602E-01 | 6.5949E-04 | |
4 | 1.3894E-02 | 1.3909E-02 | 3.7115E-05 | 1.2287E-02 | 1.8341E-03 | |
5 | 9.3482E-01 | 1.5129E-02 | 1.2758E-05 | 6.5560E-03 | 3.6908E-04 | |
6 | 2.4276E-02 | 1.5559E-02 | 3.9225E-08 | 4.9250E-04 | 2.3328E-05 |
Algorithm's description | Moth-flame's elements |
Decision variable | Moth's position in each dimension |
Solutions | Moth's position |
Initial solutions | Random positions of moths |
Current solutions | Current positions of moths |
New solutions | New positions of moths |
Best solution | Flame's position |
Fitness function | Distance between moth and flame |
Process of generating a new solution | Flying in a spiral path toward a flame |
Algorithm 1. MFO. |
Randomly initialize the position of moths and the parameters for Moth-flame |
While $iteration \le Max\_iteration$ do |
Renew flame no utilizing Eq (15) |
$OM = FitnessFunction(M)$ |
If $iteration =1$ then |
$F = sort(M)$ |
$OF = sort(OM)$ |
else |
$F = sort({M_{t - 1}}, {M_t})$ |
$OF = sort({M_{t - 1}}, {M_t})$ |
end if |
for $i=1: n$ do |
for $j=1: d$ do |
Renew $ r $ and $ t $ |
Estimate $ D $ utilizing Eq (14) |
Renew $ M(i, j) $ utilizing Eq (13) |
end for |
end for |
end while |
Return the best solution |
Threshold segmentation | MFO |
A collection $ ({x_1}, {x_2}, ..., {x_k}) $ scheduling schemes | A moth population $ ({n_1}, {n_2}, ..., {n_k}) $ moths |
An optimal scheme to resolve the image segmentation | An optimal flame's position |
The objective function of the image segmentation | The fitness function of the MFO |
Algorithm 2. MFO-based Kapur entropy. |
Randomly initialize the position of moths and the parameters for Moth-flame |
While $iteration \le Max\_iteration$ do |
Renew flame no utilizing Eq (15) |
Estimate the fitness value of each moth utilizing Eq (2) for the Kapur entropy and attain the best solution |
$OM = FitnessFunction(M)$ |
If $iteration = 1$ then |
$F = sort(M)$ |
$OF = sort(OM)$ |
else |
$F = sort({M_{t - 1}}, {M_t})$ |
$OF = sort({M_{t - 1}}, {M_t})$ |
end if |
for $i = 1:n$ do |
for $j = 1:d$ do |
Renew $ r $ and $ t $ |
Estimate $ D $ utilizing Eq (14) |
Renew $ M(i, j) $ utilizing Eq (13) |
end for |
end for |
end while |
Return the best solution or the optimal threshold value |
Algorithm | Parameter | Value |
BA [11] | Pulse frequency scope $ f $ | [0, 2] |
Echo loudness $ A $ | 0.25 | |
Reducing coefficient $ \gamma $ | 0.5 | |
FPA [12] | Switch probability $ \rho $ | 0.8 |
MSA [13] | An arbitrary value $ \theta $ | [-2, 1] |
An arbitrary value $ {\varepsilon _2} $ | [0, 1] | |
An arbitrary value $ {\varepsilon _3} $ | [0, 1] | |
An arbitrary value $ {r_1} $ | [0, 1] | |
An arbitrary value $ {r_2} $ | [0, 1] | |
PSO [14] | Constant inertia $ \omega $ | 0.3 |
First acceleration coefficient $ {c_1} $ | 1.4962 | |
Second acceleration coefficient $ {c_2} $ | 1.4962 | |
WWO [15] | Wavelength $ \lambda $ | 0.5 |
Wave height $ {h_{\max }} $ | 6 | |
Wavelength attenuation factor $ \alpha $ | 1.0026 | |
Breaking factor $ \beta $ | [0.001, 0.25] | |
Maximum value $ {k_{\max }} $ of breaking directions | $ min(12, {D \mathord{\left/ {\vphantom {D 2}} \right. } 2}) $ | |
MFO [43] | Logarithmic spiral $ b $ | 1 |
An arbitrary value $ t $ | [-1, 1] |
Images | k | Fitness values | ||||||
BA | FPA | MSA | PSO | WWO | MFO | Rank | ||
Test 1 | 2 | 12.3364 | 12.3198 | 12.2127 | 12.3479 | 12.3112 | 12.3487 | 1 |
3 | 15.2194 | 15.3081 | 15.2704 | 15.2018 | 15.1970 | 15.3172 | 1 | |
4 | 17.7102 | 17.7705 | 17.8821 | 17.7208 | 17.8752 | 17.9921 | 1 | |
5 | 20.3813 | 20.0295 | 20.2961 | 21.1111 | 20.2899 | 21.2798 | 1 | |
6 | 22.4683 | 22.3940 | 21.9694 | 22.4280 | 22.4488 | 22.6422 | 1 | |
Test 2 | 2 | 12.5842 | 12.5331 | 12.5997 | 12.6346 | 12.5916 | 12.6346 | 1 |
3 | 15.6189 | 15.5130 | 15.6003 | 15.4587 | 15.4813 | 15.6531 | 1 | |
4 | 18.1117 | 18.3233 | 18.1107 | 18.3061 | 18.2180 | 18.4397 | 1 | |
5 | 20.5250 | 20.9349 | 20.9719 | 20.9611 | 20.9605 | 21.0539 | 1 | |
6 | 23.1116 | 23.3068 | 23.3549 | 23.2826 | 23.2950 | 23.3837 | 1 | |
Test 3 | 2 | 12.1651 | 12.1623 | 12.1384 | 12.2017 | 12.0067 | 12.2061 | 1 |
3 | 15.3462 | 15.3160 | 14.9498 | 15.2155 | 15.1669 | 15.5039 | 1 | |
4 | 17.9605 | 18.1165 | 18.0610 | 17.8602 | 17.7274 | 18.3107 | 1 | |
5 | 20.0693 | 20.3889 | 20.6938 | 20.7448 | 20.0828 | 20.8056 | 1 | |
6 | 22.2665 | 22.6040 | 22.7055 | 23.0135 | 22.2510 | 24.1228 | 1 | |
Test 4 | 2 | 11.6162 | 11.0138 | 11.6151 | 11.6170 | 11.5649 | 11.6170 | 1 |
3 | 14.4375 | 14.1046 | 14.4277 | 14.4640 | 14.4074 | 14.6363 | 1 | |
4 | 17.1661 | 17.1351 | 17.2543 | 17.2182 | 16.5648 | 17.5047 | 1 | |
5 | 19.3315 | 19.0672 | 19.5998 | 19.9435 | 19.4292 | 20.0589 | 1 | |
6 | 22.1115 | 21.7571 | 21.8045 | 21.9815 | 21.7166 | 22.1257 | 1 | |
Test 5 | 2 | 12.5987 | 9.17771 | 12.4965 | 12.6016 | 12.5880 | 12.6016 | 1 |
3 | 15.8446 | 15.6981 | 15.6613 | 15.7945 | 15.6387 | 15.9201 | 1 | |
4 | 18.6330 | 18.5687 | 18.6837 | 18.7647 | 18.4739 | 18.8308 | 1 | |
5 | 21.5729 | 21.4283 | 21.6418 | 21.3651 | 21.5618 | 21.6809 | 1 | |
6 | 24.1845 | 24.0339 | 24.0773 | 23.7893 | 23.9359 | 24.3074 | 1 | |
Test 6 | 2 | 12.9609 | 12.9185 | 12.9393 | 12.9682 | 12.9668 | 12.9682 | 1 |
3 | 15.9835 | 16.0781 | 16.0526 | 16.1034 | 16.1034 | 16.1252 | 1 | |
4 | 18.5746 | 18.7090 | 18.7443 | 18.6889 | 18.7069 | 19.0165 | 1 | |
5 | 21.3011 | 21.1524 | 21.2343 | 21.0410 | 20.8741 | 21.4711 | 1 | |
6 | 24.1403 | 23.8479 | 23.9266 | 23.7607 | 24.0573 | 24.1842 | 1 | |
Test 7 | 2 | 12.5779 | 12.3517 | 12.5367 | 12.5936 | 12.3939 | 12.5936 | 1 |
3 | 15.3831 | 15.4011 | 15.3516 | 15.3938 | 15.0022 | 15.4274 | 1 | |
4 | 18.3603 | 18.0115 | 17.8258 | 17.9950 | 18.2520 | 18.4235 | 1 | |
5 | 20.9092 | 20.9076 | 20.6714 | 20.5292 | 20.7942 | 21.0217 | 1 | |
6 | 22.8604 | 23.3690 | 23.1421 | 22.9799 | 23.0124 | 23.5484 | 1 | |
Test 8 | 2 | 12.8868 | 12.8947 | 12.8930 | 12.9208 | 12.8957 | 12.9208 | 1 |
3 | 15.8248 | 15.9079 | 15.8297 | 15.8527 | 15.8992 | 16.0552 | 1 | |
4 | 18.5661 | 18.7317 | 18.6622 | 18.7469 | 18.5391 | 19.0449 | 1 | |
5 | 21.2946 | 21.1664 | 21.1186 | 21.1555 | 21.5266 | 21.5719 | 1 | |
6 | 23.5705 | 23.8786 | 24.2027 | 23.8717 | 23.6695 | 24.3206 | 1 | |
Test 9 | 2 | 11.9271 | 11.8657 | 11.4150 | 11.9285 | 11.7951 | 11.9286 | 1 |
3 | 14.7762 | 14.7811 | 14.7560 | 14.8629 | 14.7074 | 14.9331 | 1 | |
4 | 17.1365 | 17.2515 | 17.1703 | 17.3163 | 17.3681 | 17.4062 | 1 | |
5 | 19.8132 | 19.5984 | 19.8790 | 19.7153 | 19.8422 | 19.8973 | 2 | |
6 | 21.9515 | 22.0255 | 22.1325 | 21.8282 | 21.9971 | 22.2318 | 1 | |
Test 10 | 2 | 11.5248 | 11.4683 | 11.4326 | 11.5288 | 11.4273 | 11.5288 | 1 |
3 | 14.3849 | 13.9338 | 14.1944 | 14.4104 | 14.3475 | 14.5453 | 1 | |
4 | 16.8579 | 17.2591 | 17.3456 | 17.3302 | 17.2842 | 17.3904 | 1 | |
5 | 19.3943 | 19.2793 | 18.9721 | 19.3799 | 19.3881 | 19.8757 | 1 | |
6 | 21.5514 | 21.6964 | 21.5692 | 21.3715 | 21.8951 | 22.2800 | 1 |
Images | k | Best threshold values | ||||||
BA | FPA | MSA | PSO | WWO | MFO | |||
Test 1 | 2 | 95, 168 | 95, 171 | 113, 157 | 98, 165 | 103, 173 | 97, 164 | |
3 | 67, 121, 181 | 88, 134, 179 | 76, 133, 176 | 73, 124, 160 | 90, 139, 168 | 81, 126, 174 | ||
4 | 68, 110, 130, 163 | 60, 103, 160, 200 | 55, 91, 139, 180 | 61, 83, 116, 181 | 79, 121, 146, 175 | 73, 112, 147, 184 | ||
5 | 60, 98, 144, 172, 204 |
46, 65, 109, 142, 176 |
78, 98, 135, 157, 183 |
39, 100, 163, 165, 239 |
63, 91, 144, 168, 187 |
65, 101, 125, 165, 239 |
||
6 | 38, 58, 98, 140, 171, 195 |
71, 89, 127, 176, 194, 216 |
44, 91, 101, 133, 155, 200 |
66, 106, 137, 159, 196, 224 |
66, 115, 140, 162, 178, 193 |
77, 98, 132, 156, 181, 208 |
||
Test 2 | 2 | 62, 148 | 64, 121 | 79, 138 | 75, 147 | 66, 153 | 75, 147 | |
3 | 76, 132, 174 | 42, 83, 158 | 76, 119, 158 | 45, 130, 169 | 43, 109, 146 | 59, 106, 170 | ||
4 | 43, 86, 144, 206 | 55, 108, 133, 190 | 62, 89, 118, 192 | 53, 121, 152, 188 | 47, 118, 159, 203 | 50, 84, 141, 179 | ||
5 | 24, 93, 134, 173, 192 |
35, 93, 126, 155, 191 |
32, 79, 101, 136, 188 |
34, 73, 95, 129, 176 |
56, 84, 104, 145, 175 |
50, 80, 119, 163, 204 |
||
6 | 35, 80, 106, 137, 191, 203 |
56, 101, 126, 160, 177, 200 |
44, 76, 122, 162, 177, 196 |
22, 38, 82, 110, 151, 193 |
65, 83, 107, 145, 179, 199 |
37, 52, 76, 126, 169, 195 |
||
Test 3 | 2 | 82, 162 | 88, 164 | 85, 157 | 69, 177 | 110, 163 | 69, 174 | |
3 | 87, 135, 186 | 62, 141, 178 | 102, 159, 184 | 73, 141, 195 | 81, 154, 192 | 69, 127, 183 | ||
4 | 89, 123, 152, 189 | 64, 114, 151, 176 | 54, 95, 135, 169 | 68, 120, 134, 185 | 38, 93, 148, 192 | 66, 106, 146, 186 | ||
5 | 78, 101, 118, 133, 186 |
67, 98, 135, 149, 174 |
56, 80, 111, 152, 194 |
34, 87, 165, 182, 231 |
75, 101, 132, 169, 213 |
70, 104, 132, 160, 190 |
||
6 | 82, 114, 131, 154, 174, 212 |
49, 80, 94, 114, 174, 198 |
41, 53, 73, 113, 146, 181 |
55, 93, 128, 153, 183, 196 |
58, 71, 78, 118, 147, 181 |
56, 69, 127, 163, 183, 231 |
||
Test 4 | 2 | 101, 174 | 146, 164 | 95, 175 | 98, 174 | 93, 154 | 95, 171 | |
3 | 72, 136, 169 | 117, 177, 212 | 69, 115, 180 | 99, 152, 181 | 70, 113, 169 | 85, 137, 180 | ||
4 | 41, 84, 115, 164 | 50, 80, 146, 183 | 43, 91, 124, 172 | 40, 86, 133, 167 | 63, 96, 159, 172 | 38, 86, 138, 180 | ||
5 | 49, 82, 122, 181, 224 |
29, 75, 104, 120, 205 |
49, 75, 105, 160, 181 |
32, 91, 125, 171, 208 |
37, 100, 116, 152, 210 |
43, 98, 125, 173, 245 |
||
6 | 75, 102, 125, 161, 188, 237 |
43, 110, 129, 150, 181, 211 |
79, 120, 135, 167, 221, 237 |
43, 59, 85, 127, 183, 212 |
32, 61, 78, 122, 148, 211 |
28, 53, 77, 114, 146, 182 |
||
Test 5 | 2 | 74, 135 | 110, 248 | 83, 157 | 70, 135 | 64, 129 | 70, 135 | |
3 | 56, 134, 215 | 61, 120, 184 | 59, 145, 230 | 62, 119, 197 | 80, 127, 180 | 69, 133, 204 | ||
4 | 52, 131, 206, 231 | 95, 145, 200, 234 | 70, 94, 146, 207 | 74, 127, 186, 226 | 81, 114, 190, 221 | 70, 108, 150, 216 | ||
5 | 61, 110, 168, 199, 231 |
33, 71, 97, 154, 225 |
37, 101, 137, 179, 217 |
21, 79, 150, 195, 231 |
63, 93, 134, 188, 233 |
41, 94, 131, 177, 225 |
||
6 | 29, 62, 117, 143, 188, 211 |
23, 75, 110, 142, 208, 238 |
38, 61, 127, 167, 212, 233 |
51, 84, 124, 147, 187, 200 |
32, 72, 91, 116, 192, 228 |
27, 77, 109, 140, 179, 225 |
||
Test 6 | 2 | 84, 168 | 111, 175 | 106, 175 | 91, 170 | 92, 171 | 91, 170 | |
3 | 55, 126, 191 | 84, 145, 188 | 85, 138, 200 | 71, 124, 188 | 68, 128, 177 | 75, 131, 185 | ||
4 | 37, 117, 168, 211 | 60, 137, 164, 194 | 39, 77, 132, 186 | 94, 121, 157, 214 | 54, 139, 178, 219 | 68, 105, 157, 204 | ||
5 | 50, 75, 110, 184, 206 |
41, 82, 122, 189, 234 |
35, 78, 131, 156, 183 |
37, 124, 154, 182, 208 |
42, 108, 120, 179, 205 |
80, 111, 132, 163, 204 |
||
6 | 59, 89, 114, 146, 187, 207 |
32, 58, 116, 146, 186, 214 |
60, 80, 105, 135, 168, 189 |
47, 88, 111, 165, 191, 204 |
44, 65, 113, 146, 170, 199 |
42, 84, 116, 136, 170, 209 |
||
Test 7 | 2 | 97, 166 | 124, 164 | 97, 170 | 96, 163 | 70, 156 | 96, 163 | |
3 | 75, 123, 158 | 53, 94, 176 | 54, 130, 162 | 80, 107, 170 | 62, 178, 219 | 81, 166, 198 | ||
4 | 71, 127, 166, 218 | 66, 121, 145, 184 | 77, 129, 187, 236 | 43, 110, 162, 181 | 95, 123, 166, 209 | 46, 104, 167, 197 | ||
5 | 46, 87, 123, 161, 225 |
40, 99, 130, 175, 198 |
40, 59, 97, 143, 196 |
24, 73, 137, 166, 214 |
65, 122, 146, 175, 214 |
79, 107, 137, 166, 212 |
||
6 | 37, 69, 131, 145, 174, 236 |
47, 63, 112, 162, 186, 223 |
53, 101, 125, 160, 202, 214 |
62, 123, 149, 174, 190, 233 |
62, 110, 167, 193, 204, 233 |
44, 82, 132, 171, 200, 234 |
||
Test 8 | 2 | 91, 170 | 83, 162 | 100, 168 | 93, 161 | 94, 169 | 93, 161 | |
3 | 80, 164, 225 | 109, 165, 201 | 66, 102, 157 | 69, 105, 171 | 59, 114, 161 | 95, 159, 206 | ||
4 | 46, 104, 144, 217 | 73, 109, 175, 225 | 83, 136, 177, 203 | 42, 109, 159, 191 | 47, 81, 124, 156 | 65, 111, 160, 207 | ||
5 | 62, 120, 172, 204, 223 |
36, 55, 115, 157, 217 |
80, 143, 175, 209, 231 |
55, 112, 132, 173, 194 |
68, 102, 131, 164, 223 |
74, 107, 159, 196, 223 |
||
6 | 29, 47, 87, 110, 157, 212 |
58, 85, 113, 133, 165, 229 |
52, 75, 102, 131, 160, 205 |
59, 74, 108, 145, 171, 204 |
58, 98, 144, 157, 181, 201 |
50, 85, 126, 158, 202, 227 |
||
Test 9 | 2 | 103, 155 | 93, 160 | 128, 163 | 104, 156 | 92, 166 | 105, 156 | |
3 | 110, 152, 213 | 92, 152, 212 | 104, 166, 207 | 111, 153, 193 | 69, 115, 167 | 104, 154, 203 | ||
4 | 55, 105, 135, 161 | 44, 86, 150, 205 | 55, 96, 121, 174 | 97, 132, 155, 192 | 63, 99, 173, 203 | 57, 106, 172, 201 | ||
5 | 96, 117, 150, 179, 298 |
92, 121, 136, 152, 204 |
56, 91, 114, 165, 220 |
56, 116, 153, 169, 186 |
92, 129, 154, 172, 201 |
61, 84, 102, 163, 197 |
||
6 | 45, 74, 86, 120, 161, 220 |
66, 84, 96, 128, 156, 184 |
72, 95, 116, 162, 172, 209 |
78, 96, 107, 133, 151, 199 |
53, 84, 102, 111, 165, 211 |
56, 82, 115, 164, 187, 198 |
||
Test 10 | 2 | 74, 148 | 72, 138 | 77, 133 | 75, 153 | 96, 168 | 75, 153 | |
3 | 86, 154, 184 | 75, 104, 213 | 84, 129, 211 | 71, 154, 196 | 97, 143, 196 | 83, 143, 187 | ||
4 | 76, 95, 171, 222 | 83, 125, 164, 203 | 77, 123, 164, 213 | 74, 112, 138, 177 | 74, 123, 156, 210 | 72, 116, 151, 181 | ||
5 | 94, 132, 147, 188, 222 |
85, 146, 168, 202, 230 |
71, 88, 101, 179, 213 |
50, 83, 152, 179, 248 |
81, 132, 174, 214, 230 |
74, 105, 136, 163, 186 |
||
6 | 70, 82, 114, 172, 194, 230 |
74, 110, 126, 163, 194, 203 |
89, 120, 133, 167, 180, 223 |
64, 81, 128, 162, 202, 230 |
72, 126, 162, 192, 213, 233 |
75, 103, 144, 176, 191, 222 |
Images | k | Execution time (in second) | ||||||
BA | FPA | MSA | PSO | WWO | MFO | Rank | ||
Test 1 | 2 | 2.0504 | 2.5449 | 4.1430 | 3.2668 | 2.6219 | 1.8838 | 1 |
3 | 2.1806 | 2.4652 | 3.6404 | 3.7600 | 3.0271 | 2.1470 | 1 | |
4 | 2.3493 | 2.5466 | 3.6591 | 4.2612 | 3.5488 | 2.3476 | 1 | |
5 | 2.3526 | 2.4754 | 3.6553 | 4.4621 | 4.1037 | 2.3232 | 1 | |
6 | 2.4534 | 2.5140 | 3.6961 | 4.7721 | 4.6332 | 2.4476 | 1 | |
Test 2 | 2 | 2.0546 | 2.2233 | 3.5972 | 3.0943 | 2.4066 | 1.9862 | 1 |
3 | 2.2834 | 2.4729 | 3.7247 | 3.7189 | 3.0388 | 2.2371 | 1 | |
4 | 2.4856 | 2.4565 | 3.5933 | 4.0514 | 3.7730 | 2.3832 | 1 | |
5 | 2.6244 | 2.5519 | 3.6495 | 13.892 | 4.2132 | 2.5896 | 2 | |
6 | 2.7085 | 2.6344 | 3.6366 | 4.6383 | 4.9811 | 2.6217 | 1 | |
Test 3 | 2 | 2.0428 | 2.1661 | 3.5012 | 10.081 | 2.3834 | 2.0128 | 1 |
3 | 2.1067 | 2.2636 | 3.6970 | 3.5344 | 2.9690 | 2.1931 | 2 | |
4 | 2.3168 | 2.4309 | 3.6582 | 4.1890 | 3.5014 | 2.2318 | 1 | |
5 | 2.4964 | 2.4430 | 3.6781 | 4.2050 | 4.1567 | 2.4421 | 1 | |
6 | 2.5751 | 2.5232 | 3.7181 | 4.4494 | 4.7794 | 2.5211 | 1 | |
Test 4 | 2 | 2.1245 | 2.2784 | 3.5689 | 3.1980 | 2.4759 | 1.8874 | 1 |
3 | 2.2841 | 2.3142 | 3.6728 | 3.9206 | 3.1605 | 2.1235 | 1 | |
4 | 2.4620 | 2.4784 | 3.7133 | 4.4914 | 3.6540 | 2.4128 | 1 | |
5 | 2.5981 | 2.5782 | 3.7064 | 4.5182 | 4.2552 | 2.5252 | 1 | |
6 | 2.5760 | 2.6113 | 3.7157 | 4.6266 | 4.6624 | 2.5645 | 1 | |
Test 5 | 2 | 2.1369 | 2.3193 | 3.6874 | 3.3801 | 2.5160 | 1.9748 | 1 |
3 | 2.3770 | 2.4655 | 3.8206 | 3.9882 | 3.1202 | 2.1927 | 1 | |
4 | 2.6246 | 2.5708 | 3.7468 | 4.6477 | 3.7042 | 2.4725 | 1 | |
5 | 2.6867 | 2.6756 | 3.7018 | 4.8451 | 4.2924 | 2.6573 | 1 | |
6 | 3.0574 | 2.6619 | 3.7099 | 5.2076 | 4.9496 | 2.6989 | 2 | |
Test 6 | 2 | 2.0659 | 2.2878 | 3.7082 | 3.4298 | 2.4881 | 1.8933 | 1 |
3 | 2.3009 | 2.4216 | 3.7141 | 3.7289 | 3.1803 | 2.1388 | 1 | |
4 | 2.5690 | 2.5297 | 3.7186 | 4.4016 | 3.6365 | 2.5144 | 1 | |
5 | 2.7012 | 2.7481 | 3.8449 | 4.6251 | 4.4892 | 2.5788 | 1 | |
6 | 2.7363 | 2.6549 | 3.5908 | 5.0158 | 5.0763 | 2.6390 | 1 | |
Test 7 | 2 | 2.1999 | 2.2860 | 3.7677 | 3.4719 | 2.5184 | 1.7930 | 1 |
3 | 2.3913 | 2.4617 | 3.8282 | 4.4295 | 3.1260 | 2.1823 | 1 | |
4 | 2.5758 | 2.5069 | 3.6892 | 4.5830 | 3.6773 | 2.5712 | 2 | |
5 | 2.8661 | 2.6928 | 3.7420 | 4.9113 | 4.3654 | 2.6331 | 1 | |
6 | 2.8314 | 2.7552 | 3.8166 | 4.7869 | 4.8823 | 2.7347 | 1 | |
Test 8 | 2 | 2.1196 | 2.2375 | 3.5308 | 3.2347 | 2.6986 | 1.8208 | 1 |
3 | 2.1790 | 2.4691 | 3.6472 | 4.1744 | 3.2448 | 2.0801 | 1 | |
4 | 2.4523 | 2.5377 | 3.6976 | 4.4788 | 3.7100 | 2.3825 | 1 | |
5 | 2.5949 | 2.5873 | 3.7993 | 4.6852 | 4.3499 | 2.5537 | 1 | |
6 | 2.6545 | 2.6189 | 3.7323 | 5.1430 | 4.7387 | 2.5936 | 1 | |
Test 9 | 2 | 2.1158 | 2.2435 | 3.7132 | 3.0533 | 2.3750 | 1.8010 | 1 |
3 | 2.2377 | 2.3223 | 3.7861 | 3.8355 | 2.9901 | 2.1133 | 1 | |
4 | 2.3979 | 2.4493 | 3.6818 | 4.1490 | 3.5694 | 2.3356 | 1 | |
5 | 2.4748 | 2.4971 | 3.6920 | 4.3074 | 4.2244 | 2.4209 | 1 | |
6 | 2.4707 | 2.5587 | 3.7407 | 4.6462 | 4.5649 | 2.5010 | 2 | |
Test 10 | 2 | 2.0775 | 2.1429 | 3.4231 | 3.0237 | 2.3839 | 1.6726 | 1 |
3 | 2.2550 | 2.2918 | 3.5819 | 3.4502 | 2.9528 | 2.2082 | 1 | |
4 | 2.3523 | 2.3987 | 3.6269 | 4.0056 | 3.4883 | 2.3009 | 1 | |
5 | 2.3653 | 2.4887 | 3.7718 | 4.1497 | 4.0963 | 2.3358 | 1 | |
6 | 2.42209 | 2.5584 | 3.7138 | 4.3912 | 4.6485 | 2.4192 | 1 |
Images | k | PSNR values | ||||||
BA | FPA | MSA | PSO | WWO | MFO | Rank | ||
Test 1 | 2 | 54.2052 | 54.0193 | 52.6804 | 53.9194 | 53.3728 | 54.2052 | 1 |
3 | 55.2116 | 54.7434 | 55.5773 | 55.8071 | 54.6025 | 56.2391 | 1 | |
4 | 56.1645 | 56.9214 | 55.8071 | 56.8053 | 55.3545 | 57.8253 | 1 | |
5 | 56.9214 | 57.2139 | 55.4283 | 67.4037 | 56.5877 | 61.6781 | 2 | |
6 | 57.5946 | 55.9464 | 63.0931 | 56.3249 | 56.3249 | 68.3558 | 1 | |
Test 2 | 2 | 56.8302 | 55.6667 | 55.2570 | 55.6667 | 56.4413 | 56.8496 | 1 |
3 | 55.5716 | 55.8390 | 55.5716 | 56.9284 | 58.4671 | 58.5125 | 1 | |
4 | 56.8302 | 57.5587 | 56.8302 | 57.7738 | 57.7738 | 58.6671 | 1 | |
5 | 60.8783 | 57.6678 | 59.6180 | 57.5587 | 57.4521 | 58.5125 | 3 | |
6 | 58.7405 | 57.4521 | 58.5890 | 61.3621 | 56.5356 | 59.3017 | 2 | |
Test 3 | 2 | 61.6543 | 60.6947 | 61.1888 | 59.1452 | 57.4414 | 63.7976 | 1 |
3 | 60.8653 | 64.1985 | 58.3970 | 63.3619 | 61.8294 | 65.2923 | 1 | |
4 | 60.5327 | 65.0068 | 64.6841 | 64.3602 | 66.0216 | 66.2020 | 1 | |
5 | 62.3681 | 65.6347 | 65.9875 | 70.5641 | 62.9357 | 67.5475 | 2 | |
6 | 61.6543 | 66.8489 | 65.6347 | 66.0853 | 65.7478 | 68.2531 | 1 | |
Test 4 | 2 | 53.7298 | 51.8359 | 53.9307 | 53.8281 | 53.3635 | 54.0080 | 1 |
3 | 55.7727 | 53.2812 | 56.2939 | 53.7936 | 54.4415 | 56.3931 | 1 | |
4 | 69.8724 | 64.0939 | 68.4585 | 70.6684 | 57.7874 | 70.6684 | 1 | |
5 | 64.6339 | 76.5339 | 64.6339 | 75.2461 | 54.0512 | 72.8879 | 3 | |
6 | 55.3686 | 68.4585 | 62.6342 | 59.8785 | 71.2461 | 71.5255 | 1 | |
Test 5 | 2 | 55.9353 | 54.4501 | 55.5723 | 56.1030 | 56.1944 | 56.3859 | 1 |
3 | 56.1489 | 56.5395 | 56.6408 | 56.1489 | 55.6932 | 56.7947 | 1 | |
4 | 56.0035 | 55.0787 | 56.1030 | 55.9353 | 55.6534 | 56.4888 | 1 | |
5 | 57.1093 | 57.6783 | 57.8691 | 59.3191 | 56.4366 | 58.1327 | 2 | |
6 | 58.4300 | 59.0280 | 57.8027 | 57.0566 | 57.3278 | 59.0280 | 1 | |
Test 6 | 2 | 52.4037 | 50.9764 | 51.2852 | 52.4037 | 52.3196 | 52.9992 | 1 |
3 | 53.7675 | 52.9992 | 52.9146 | 54.0808 | 54.3233 | 55.5443 | 1 | |
4 | 56.9588 | 55.0405 | 54.3233 | 52.1517 | 55.6405 | 57.5981 | 1 | |
5 | 55.2454 | 54.8447 | 55.8429 | 57.9588 | 56.4138 | 58.3841 | 1 | |
6 | 55.1430 | 57.0950 | 54.9352 | 56.4138 | 56.7949 | 59.1946 | 1 | |
Test 7 | 2 | 52.1853 | 50.1439 | 52.1853 | 52.2305 | 52.2305 | 53.6248 | 1 |
3 | 53.1369 | 52.7825 | 54.3929 | 52.8286 | 54.1279 | 54.4252 | 1 | |
4 | 53.5238 | 53.9356 | 52.9913 | 54.1279 | 52.2737 | 54.9081 | 1 | |
5 | 54.7169 | 55.1408 | 54.7169 | 52.7825 | 53.9928 | 57.7724 | 1 | |
6 | 54.4130 | 54.6643 | 54.4252 | 54.4600 | 54.1279 | 55.1408 | 1 | |
Test 8 | 2 | 51.2625 | 51.1401 | 50.7696 | 51.1401 | 51.0819 | 51.8484 | 1 |
3 | 52.1217 | 50.3589 | 53.9395 | 53.4810 | 51.0247 | 55.1889 | 1 | |
4 | 58.3667 | 52.9207 | 51.8484 | 52.6689 | 58.1344 | 59.3198 | 1 | |
5 | 54.6097 | 54.7941 | 52.1217 | 60.9018 | 53.6305 | 61.3007 | 1 | |
6 | 62.1126 | 55.4021 | 56.8943 | 55.1889 | 55.4021 | 64.4974 | 1 | |
Test 9 | 2 | 54.7684 | 56.0844 | 50.9610 | 54.6524 | 54.5365 | 56.2376 | 1 |
3 | 53.9616 | 56.2376 | 54.6524 | 53.8444 | 54.6524 | 61.7530 | 1 | |
4 | 67.6683 | 73.5879 | 67.6683 | 55.5133 | 58.1149 | 68.0342 | 2 | |
5 | 62.8685 | 59.5786 | 67.1721 | 67.1721 | 56.2376 | 73.5879 | 1 | |
6 | 72.9963 | 62.8685 | 68.6413 | 57.8977 | 68.6413 | 79.2437 | 1 | |
Test 10 | 2 | 49.4261 | 49.4702 | 49.3850 | 49.4109 | 49.2129 | 49.4809 | 1 |
3 | 49.3039 | 49.4109 | 49.3191 | 49.3437 | 49.2012 | 49.4261 | 1 | |
4 | 49.3976 | 49.3267 | 49.3850 | 49.4261 | 49.4261 | 49.5080 | 1 | |
5 | 49.2362 | 49.3115 | 49.4261 | 49.1974 | 49.3437 | 49.5080 | 1 | |
6 | 49.5634 | 49.4261 | 49.2816 | 49.3850 | 49.4702 | 50.5952 | 1 |
Images | k | SSIM values | ||||||
BA | FPA | MSA | PSO | WWO | MFO | Rank | ||
Test 1 | 2 | 0.5811 | 0.5630 | 0.4666 | 0.5589 | 0.5389 | 0.5827 | 1 |
3 | 0.6174 | 0.6028 | 0.6291 | 0.6196 | 0.5792 | 0.6677 | 1 | |
4 | 0.6275 | 0.6713 | 0.6450 | 0.6841 | 0.6040 | 0.6962 | 1 | |
5 | 0.6706 | 0.6692 | 0.6240 | 0.6779 | 0.6761 | 0.7428 | 1 | |
6 | 0.6579 | 0.6752 | 0.7295 | 0.6565 | 0.6272 | 0.7853 | 1 | |
Test 2 | 2 | 0.5934 | 0.5618 | 0.5486 | 0.5624 | 0.5854 | 0.5951 | 1 |
3 | 0.5849 | 0.5892 | 0.5737 | 0.6229 | 0.6440 | 0.6549 | 1 | |
4 | 0.6118 | 0.6431 | 0.6084 | 0.6336 | 0.6302 | 0.6680 | 1 | |
5 | 0.6700 | 0.6321 | 0.6721 | 0.6239 | 0.6315 | 0.6953 | 1 | |
6 | 0.6764 | 0.6305 | 0.6926 | 0.7184 | 0.6484 | 0.7095 | 2 | |
Test 3 | 2 | 0.7776 | 0.7700 | 0.7735 | 0.7433 | 0.7326 | 0.7782 | 1 |
3 | 0.7694 | 0.7963 | 0.7380 | 0.7321 | 0.7388 | 0.8075 | 1 | |
4 | 0.7661 | 0.8171 | 0.8107 | 0.7983 | 0.7966 | 0.8286 | 1 | |
5 | 0.8008 | 0.7352 | 0.8087 | 0.7841 | 0.7682 | 0.8266 | 1 | |
6 | 0.7468 | 0.7928 | 0.8434 | 0.7941 | 0.8482 | 0.8560 | 1 | |
Test 4 | 2 | 0.5682 | 0.4613 | 0.5752 | 0.5724 | 0.5494 | 0.5840 | 1 |
3 | 0.6295 | 0.5643 | 0.6447 | 0.5538 | 0.5765 | 0.6573 | 1 | |
4 | 0.7995 | 0.7457 | 0.8018 | 0.7963 | 0.7050 | 0.8387 | 1 | |
5 | 0.8021 | 0.7892 | 0.7802 | 0.8431 | 0.5767 | 0.8509 | 1 | |
6 | 0.6250 | 0.8207 | 0.8056 | 0.7640 | 0.8148 | 0.8493 | 1 | |
Test 5 | 2 | 0.5874 | 0.5694 | 0.5478 | 0.5923 | 0.5947 | 0.6025 | 1 |
3 | 0.6513 | 0.6167 | 0.6558 | 0.6536 | 0.5760 | 0.6752 | 1 | |
4 | 0.6629 | 0.5762 | 0.6594 | 0.6147 | 0.6122 | 0.6669 | 1 | |
5 | 0.6686 | 0.6775 | 0.6918 | 0.7207 | 0.6737 | 0.7221 | 1 | |
6 | 0.7354 | 0.7299 | 0.7183 | 0.6835 | 0.7114 | 0.7593 | 1 | |
Test 6 | 2 | 0.3856 | 0.3073 | 0.3285 | 0.3856 | 0.3823 | 0.4098 | 1 |
3 | 0.4862 | 0.4423 | 0.4438 | 0.5025 | 0.5060 | 0.5417 | 1 | |
4 | 0.6023 | 0.5147 | 0.5425 | 0.4154 | 0.5323 | 0.6141 | 1 | |
5 | 0.5798 | 0.5765 | 0.5771 | 0.5794 | 0.6314 | 0.6562 | 1 | |
6 | 0.6170 | 0.6676 | 0.6141 | 0.6412 | 0.6737 | 0.7035 | 1 | |
Test 7 | 2 | 0.5412 | 0.2944 | 0.5452 | 0.5357 | 0.5357 | 0.6049 | 1 |
3 | 0.5439 | 0.5643 | 0.6308 | 0.5533 | 0.6497 | 0.6713 | 1 | |
4 | 0.5819 | 0.5867 | 0.5474 | 0.6396 | 0.5011 | 0.6571 | 1 | |
5 | 0.6752 | 0.6858 | 0.6817 | 0.5044 | 0.5725 | 0.7308 | 1 | |
6 | 0.6826 | 0.6776 | 0.6360 | 0.6650 | 0.5908 | 0.6938 | 1 | |
Test 8 | 2 | 0.3781 | 0.3656 | 0.3403 | 0.3656 | 0.3661 | 0.4100 | 1 |
3 | 0.4250 | 0.2956 | 0.4948 | 0.4927 | 0.3533 | 0.5583 | 1 | |
4 | 0.6528 | 0.4934 | 0.3981 | 0.4760 | 0.6109 | 0.6803 | 1 | |
5 | 0.5484 | 0.5568 | 0.3853 | 0.4986 | 0.5249 | 0.6793 | 1 | |
6 | 0.7181 | 0.5974 | 0.6489 | 0.6071 | 0.5787 | 0.7293 | 1 | |
Test 9 | 2 | 0.5502 | 0.6010 | 0.2334 | 0.5494 | 0.5547 | 0.6075 | 1 |
3 | 0.4929 | 0.5707 | 0.5475 | 0.4852 | 0.5360 | 0.6251 | 1 | |
4 | 0.5860 | 0.6949 | 0.6196 | 0.4427 | 0.5586 | 0.7198 | 1 | |
5 | 0.6465 | 0.6827 | 0.7318 | 0.6505 | 0.4812 | 0.7441 | 1 | |
6 | 0.7208 | 0.6556 | 0.7322 | 0.5454 | 0.7166 | 0.7698 | 1 | |
Test 10 | 2 | 0.1722 | 0.1666 | 0.1571 | 0.1729 | 0.1502 | 0.1733 | 1 |
3 | 0.1611 | 0.1719 | 0.1644 | 0.1708 | 0.1475 | 0.1772 | 1 | |
4 | 0.1822 | 0.1678 | 0.1775 | 0.1753 | 0.1804 | 0.1927 | 1 | |
5 | 0.1486 | 0.1511 | 0.1786 | 0.6364 | 0.1645 | 0.1915 | 2 | |
6 | 0.2091 | 0.1812 | 0.1565 | 0.1697 | 0.1755 | 0.2901 | 1 |
Images | k | Wilcoxon test | ||||
MFO vs BA | MFO vs FPA | MFO vs MSA | MFO vs PSO | MFO vs WWO | ||
Test 1 | 2 | 4.1486E-08 | 2.9135E-07 | 3.7215E-07 | 1.0905E-02 | 4.9558E-08 |
3 | 4.1406E-03 | 8.8674E-01 | 2.7328E-02 | 5.9956E-04 | 1.8056E-02 | |
4 | 3.9271E-03 | 6.8298E-03 | 2.3871E-05 | 5.2530E-04 | 5.9054E-05 | |
5 | 3.5064E-03 | 4.4599E-02 | 7.7394E-06 | 2.5972E-04 | 7.7394E-06 | |
6 | 1.5429E-04 | 1.6017E-04 | 2.1821E-11 | 1.6475E-04 | 2.1821E-11 | |
Test 2 | 2 | 1.5649E-11 | 4.1040E-11 | 4.5618E-11 | 5.7016E-03 | 2.6819E-11 |
3 | 3.7061E-02 | 1.4931E-03 | 4.5940E-02 | 1.5957E-02 | 7.3007E-03 | |
4 | 2.1572E-02 | 7.2382E-04 | 1.1435E-02 | 9.2276E-03 | 1.8454E-02 | |
5 | 3.0221E-07 | 1.2754E-02 | 4.4680E-10 | 3.9238E-02 | 4.4680E-10 | |
6 | 2.1287E-05 | 6.0511E-04 | 4.8389E-10 | 1.9143E-02 | 4.8389E-10 | |
Test 3 | 2 | 1.6510E-11 | 1.2118E-12 | 1.2118E-12 | 2.7793E-03 | 1.2118E-12 |
3 | 1.7047E-02 | 7.9323E-05 | 9.8208E-03 | 5.1474E-03 | 3.8910E-02 | |
4 | 1.5568E-02 | 4.8012E-02 | 6.1697E-05 | 6.0010E-03 | 1.8438E-03 | |
5 | 1.2075E-04 | 1.1553E-03 | 8.9553E-08 | 8.6976E-06 | 8.9553E-08 | |
6 | 4.2458E-05 | 1.6691E-02 | 3.9562E-10 | 1.4187E-05 | 3.9562E-10 | |
Test 4 | 2 | 1.4119E-10 | 3.6912E-11 | 4.5618E-11 | 2.7605E-02 | 1.5672E-11 |
3 | 9.6851E-08 | 1.0617E-03 | 1.3782E-02 | 1.8815E-02 | 2.0296E-11 | |
4 | 2.3687E-03 | 2.6206E-03 | 3.3040E-02 | 2.2534E-02 | 1.8354E-02 | |
5 | 1.3187E-02 | 6.9403E-03 | 6.0393E-02 | 3.3072E-02 | 1.3187E-02 | |
6 | 1.0490E-02 | 3.1661E-03 | 6.3076E-09 | 6.0661E-05 | 3.0311E-04 | |
Test 5 | 2 | 4.7773E-09 | 1.4148E-08 | 1.8780E-09 | 6.9419E-03 | 1.0569E-09 |
3 | 1.0226E-06 | 1.5514E-03 | 9.3662E-04 | 1.7753E-02 | 2.8427E-10 | |
4 | 1.2338E-04 | 6.8037E-03 | 9.0570E-05 | 6.0755E-04 | 6.6120E-03 | |
5 | 1.9974E-02 | 1.5408E-02 | 1.9284E-05 | 1.8299E-02 | 1.1010E-02 | |
6 | 2.3675E-02 | 1.9315E-02 | 9.3069E-08 | 2.8298E-02 | 2.0096E-02 | |
Test 6 | 2 | 5.4162E-09 | 5.4162E-09 | 2.4736E-08 | 2.8298E-02 | 8.3512E-09 |
3 | 1.2298E-07 | 1.5879E-06 | 3.6108E-02 | 7.8961E-03 | 7.6552E-10 | |
4 | 1.3692E-02 | 1.2204E-02 | 6.7678E-04 | 3.3418E-02 | N/A | |
5 | 1.5319E-02 | 1.3228E-05 | 3.7759E-02 | 8.2918E-03 | 8.0670E-03 | |
6 | 7.9518E-01 | 7.6105E-05 | 8.8807E-06 | 1.6308E-03 | 7.6285E-03 | |
Test 7 | 2 | 1.1999E-12 | 1.2118E-12 | 1.2118E-12 | 5.5398E-03 | 1.2118E-12 |
3 | 1.0043E-08 | 3.2704E-04 | 1.7181E-04 | 4.5838E-04 | 1.8783E-11 | |
4 | 2.2155E-05 | 1.3080E-04 | 2.0545E-03 | 1.8360E-02 | 1.8586E-03 | |
5 | 9.2817E-05 | 5.9629E-04 | 2.6611E-02 | 2.7791E-02 | 7.1075E-03 | |
6 | 8.1845E-01 | 1.8944E-02 | 1.0237E-07 | 7.3815E-04 | 2.6871E-02 | |
Test 8 | 2 | 1.1067E-08 | 1.2380E-09 | 1.2377E-09 | 9.5909E-03 | 1.2377E-09 |
3 | 5.0104E-03 | 5.7108E-04 | 7.4568E-04 | 2.5069E-02 | 7.4568E-03 | |
4 | 6.0746E-03 | 7.7156E-05 | 4.5418E-04 | 1.2662E-02 | 7.5196E-03 | |
5 | 1.2619E-02 | 7.7958E-05 | 4.3177E-10 | 5.6943E-07 | 4.3177E-10 | |
6 | 1.0049E-02 | 1.1403E-02 | 2.5562E-11 | 9.6312E-05 | 2.5562E-11 | |
Test 9 | 2 | 4.1906E-10 | 7.2256E-10 | 1.2384E-09 | 4.5164E-02 | 1.1236E-09 |
3 | 2.0185E-02 | 5.6566E-03 | 6.7067E-03 | 8.2284E-02 | N/A | |
4 | 1.7717E-02 | 8.1726E-03 | 2.2107E-06 | 2.0015E-02 | 7.4528E-03 | |
5 | 1.2740E-02 | 3.7302E-05 | 4.5563E-10 | 2.6262E-05 | 4.5563E-10 | |
6 | 1.4911E-02 | 1.7368E-03 | 4.3348E-10 | 9.9941E-04 | 4.3348E-10 | |
Test 10 | 2 | 2.5301E-10 | 1.2384E-09 | 1.2384E-09 | N/A | 7.5947E-10 |
3 | 8.1850E-03 | 1.5376E-02 | 1.4949E-02 | 9.7602E-01 | 6.5949E-04 | |
4 | 1.3894E-02 | 1.3909E-02 | 3.7115E-05 | 1.2287E-02 | 1.8341E-03 | |
5 | 9.3482E-01 | 1.5129E-02 | 1.2758E-05 | 6.5560E-03 | 3.6908E-04 | |
6 | 2.4276E-02 | 1.5559E-02 | 3.9225E-08 | 4.9250E-04 | 2.3328E-05 |