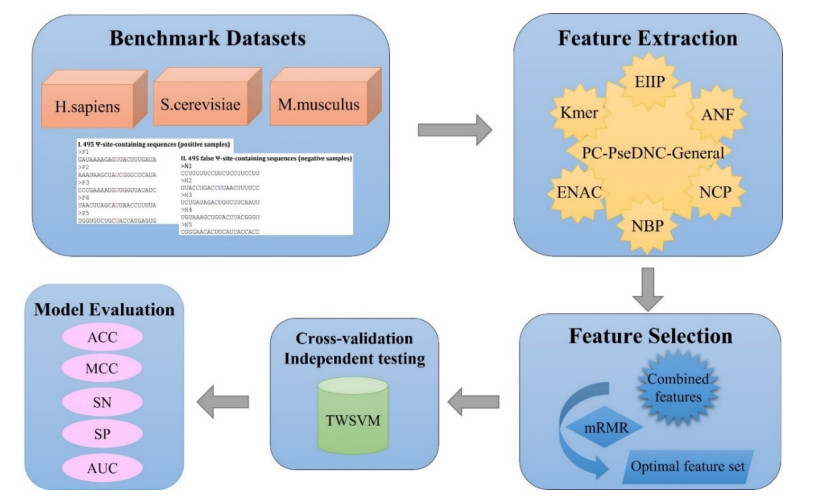
Citation: Nicola Bellomo, Raluca Eftimie, Guido Forni. What is the in-host dynamics of the SARS-CoV-2 virus? A challenge within a multiscale vision of living systems[J]. Networks and Heterogeneous Media, 2024, 19(2): 655-681. doi: 10.3934/nhm.2024029
[1] | Pingping Sun, Yongbing Chen, Bo Liu, Yanxin Gao, Ye Han, Fei He, Jinchao Ji . DeepMRMP: A new predictor for multiple types of RNA modification sites using deep learning. Mathematical Biosciences and Engineering, 2019, 16(6): 6231-6241. doi: 10.3934/mbe.2019310 |
[2] | Honglei Wang, Wenliang Zeng, Xiaoling Huang, Zhaoyang Liu, Yanjing Sun, Lin Zhang . MTTLm6A: A multi-task transfer learning approach for base-resolution mRNA m6A site prediction based on an improved transformer. Mathematical Biosciences and Engineering, 2024, 21(1): 272-299. doi: 10.3934/mbe.2024013 |
[3] | Hasan Zulfiqar, Rida Sarwar Khan, Farwa Hassan, Kyle Hippe, Cassandra Hunt, Hui Ding, Xiao-Ming Song, Renzhi Cao . Computational identification of N4-methylcytosine sites in the mouse genome with machine-learning method. Mathematical Biosciences and Engineering, 2021, 18(4): 3348-3363. doi: 10.3934/mbe.2021167 |
[4] | Huili Yang, Wangren Qiu, Zi Liu . Anoikis-related mRNA-lncRNA and DNA methylation profiles for overall survival prediction in breast cancer patients. Mathematical Biosciences and Engineering, 2024, 21(1): 1590-1609. doi: 10.3934/mbe.2024069 |
[5] | Yong Ding, Jian-Hong Liu . The signature lncRNAs associated with the lung adenocarcinoma patients prognosis. Mathematical Biosciences and Engineering, 2020, 17(2): 1593-1603. doi: 10.3934/mbe.2020083 |
[6] | Xiangzheng Fu, Yifan Chen, Sha Tian . DlncRNALoc: A discrete wavelet transform-based model for predicting lncRNA subcellular localization. Mathematical Biosciences and Engineering, 2023, 20(12): 20648-20667. doi: 10.3934/mbe.2023913 |
[7] | Yunxiang Wang, Hong Zhang, Zhenchao Xu, Shouhua Zhang, Rui Guo . TransUFold: Unlocking the structural complexity of short and long RNA with pseudoknots. Mathematical Biosciences and Engineering, 2023, 20(11): 19320-19340. doi: 10.3934/mbe.2023854 |
[8] | Shuai Miao, Lijun Wang, Siyu Guan, Tianshu Gu, Hualing Wang, Wenfeng Shangguan, Weiding Wang, Yu Liu, Xue Liang . Integrated whole transcriptome analysis for the crucial regulators and functional pathways related to cardiac fibrosis in rats. Mathematical Biosciences and Engineering, 2023, 20(3): 5413-5429. doi: 10.3934/mbe.2023250 |
[9] | Xuesi Chen, Qijun Zhang, Qin Zhang . Predicting potential biomarkers and immune infiltration characteristics in heart failure. Mathematical Biosciences and Engineering, 2022, 19(9): 8671-8688. doi: 10.3934/mbe.2022402 |
[10] | Xiaoshan Qian, Lisha Xu, Xinmei Yuan . Soft-sensing modeling of mother liquor concentration in the evaporation process based on reduced robust least-squares support-vector machine. Mathematical Biosciences and Engineering, 2023, 20(11): 19941-19962. doi: 10.3934/mbe.2023883 |
Various chemical modifications, including cytosine modification, uridine isomerization, and adenosine methylation, have been found in cellular RNA [1] and have been linked to important biological and physiological functions in cells [2]. У modification is a common posttranscriptional RNA modification known as the fifth base in RNA [3]. It is commonly present in a variety of species, and research has revealed that tRNA and rRNA contain large amounts of it [4]. Numerous biological processes have shown У to be crucial, and distinct У modifications serve different purposes at various places [5,6,7]. Therefore, the discovery of У sites in RNA sequences is crucial for both fundamental and applied biological research.
Initially, researchers identified У modification sites based on biochemical experiments. At first, researchers used paper chromatography to find У modification sites in the RNA of yeast, which was achieved by using RNA decomposition enzymes to decompose RNA and electrophoresis to separate out column chromatography on the upper layer of paper [3,4,5,6,7,8]. Later researchers successively used high-performance liquid chromatography and mass spectrometry to detect modification sites [9]. With the growing interest in this field, researchers have proposed a variety of high-throughput sequencing technologies, including Ψ-seq [10,11], PseudoU-seq [12] and CeU-Seq [13], and successfully used them to detect У sites. However, the methods described above are reliant on time-consuming, expensive, and difficult biochemical experiments, which are susceptible to environmental factors, and the sequencing process becomes increasingly difficult as the sequence length increases. Therefore, robust, fast, and inexpensive calculation methods are needed to predict У sites in RNA sequences.
First, Panwar and colleagues proposed a tRNAmod model to predict У sites in tRNA [14]. Then, a web server (PPUS) based on support vector machine (SVM) was proposed by Li et al. to identify У sites in S.cerevisiae and H.sapiens [15]. The frequency composition of nucleotides and the pseudo K-tuple nucleotide composition (PseKNC) were merged for feature representation in the iRNA-PseU model that Chen et al. created [16]. Subsequently, He et al. developed the SVM model (PseUI) to identify У sites in H.sapiens, S.cerevisiae and M.musculus, which combined a variety of feature extraction techniques including position-specific dinucleotide propensity (PSDP) [17]. Later, utilizing convolutional neural networks, Tahir et al. created a predictor (iPseU-CNN) [18]. Extreme gradient boosting (XGboost) was used by Liu et al. to create a new model known as XG-PseU [19]. Lv et al. also proposed a method called RF-PseU, which utilizes the LGBM algorithm for feature selection while combining the random forest algorithm for classification [20]. Saad et al. proposed a convolutional neural network model MU-PseUDeep [21], which combines sequence and secondary structural features to predict У sites. Li et al. built the model Porpoise by utilizing multiple type features and inputting them into the stacked ensemble learning framework [22]. Although the aforementioned techniques have proven successful in correctly identifying У sites in RNA sequences, they might still use more work in comparison to high-performance predictors [23,24,25,26,27,28].
In this study, we build a У site identification model (iPseU-TWSVM) based on TWSVM, and Figure 1 depicts the model construction process. The model combines multiple feature representation methods, including Kmer, ENAC and EIIP. To obtain the best subset of features, the mRMR approach is utilized. The model is then evaluated using 10-fold cross-validation (10-CV) and independent testing (Ⅰ-testing). The average Ⅰ-testing accuracy of the iPseU-TWSVM is 3.4% higher than that of current advanced predictors, demonstrating the better generalization performance of our model. Therefore, iPseU-TWSVM may become an effective tool for У site identification.
In this work, we train and evaluate our models using datasets created by Chen et al. [29]. The steps of constructing the benchmark dataset were as follows: 1307 positive samples and 33,280 negative samples were obtained at first, and then the subset-balancing treatment was adopted to reduce the number of negative samples according to Euclidean distance. The obtained distance values were sorted in ascending order, and the first 1307 negative samples were selected to form the negative subset. The training datasets contained data from three species, namely, H.sapiens, S.cerevisiae and M.musculus. The H.sapiens training dataset included 495 positive samples and 495 negative samples; the S.cerevisiae dataset included 314 positive samples and 314 negative samples; and the M.musculus dataset included 944 samples, half of which were positive samples. There were just two species in the Ⅰ-testing datasets: H.sapiens and S.cerevisiae. Each of them included 200 samples, of which only half were positive and half were negative.
Different types of features reflect biological significance from different perspectives, including sequence composition and physicochemical properties. In this work, a variety of types of features are used to comprehensively consider the composition, distribution and physicochemical properties of nucleotides in the sequence from various aspects to further improve the prediction performance of subsequent work.
One effective technique for extracting RNA sequence characteristics is Kmer, which reflects the frequency of k adjacent nucleotides in the sequence. The frequencies of the k-neighboring nucleotides are used to generate the feature vector [30]. The method is provided by the web server Pse-in-One2.0 (http://bioinformatics.hitsz.edu.cn/Pse-in-One2.0/) [31].
The approach offers 22 different physicochemical properties to create the pseudo-dinucleotide composition [32,33,34]. It overwrites the local sequence order and the global sequence order information into the feature vector. The relevant features are expressed in this form:
Vector=(m1m2⋯m16m16+1⋯m16+λ)T | (1) |
with
mi={qi∑16j=1qj+α∑λk=1ρk(1≤i≤16)αρσ−16∑16j=1qj+α∑λk=1ρk(16+1≤i≤16+λ) | (2) |
where qi(i=1,2,⋯,16) represents the 16 dinucleotides' normalized frequency of occurrence; α(0≤α≤1) is the weight factor; and λ is the highest counted rank. ρk is the k-tier correlation factor.
ρk displays the relationship between the sequence orders of all neighboring dinucleotides along a specific RNA sequence, which can be written as
ρk=1l−k−1∑l−k−1j=1C(Rj,Rj+k)(k=1,2,⋯,λ;λ<l−1) | (3) |
where C(Rj,Rj+k) indicates the correlation function expressed as
C(Rj,Rj+k)=1σ∑σg=1[Pg(Rj)−Pg(Rj+k)]2 | (4) |
where parameter σ is the number of physicochemical properties studied; Pg(Rj) and Pg(Rj+k) are the related values of the gth property for the dinucleotides Rj at position j and Rj+k at position j+k.
The coding method reflects that each nucleotide in the sequence has different chemical structures and binding properties. The ring structures of the four RNA nucleotides (ACGU) differ from one another, hydrogen bond, and functional group. Based on these differences, they may be represented with a 3D coordinate [35].
The method incorporates data on each nucleotide's distribution in the RNA sequence as well as its frequency [35]. We can calculate the density di of an RNA sequence's ith prefix subsequence. It is defined as
di=1i∑ij=1f(xj),wheref(xj)={1,ifxj=xi0,otherwise | (5) |
where i is the length of the sliding string and xj represents the nucleotide at the jth position.
The EIIP values represent the energy of the delocalized electron in the nucleotide. The nucleotides in the DNA sequence have previously been denoted by the EIIP values of A, G, C and T [36]. In the RF-PseU method [20], each nucleotide in an RNA sequence was also coded by EIIP feature vectors.
Using a fixed length window, the approach was used to determine the nucleotide composition [20,35]. Afterward, RNA sequences were converted into equally long feature vectors. Sequence length and sliding window size are two factors that affect the dimension of ENAC coding.
E=(b1,b2,⋯,bn),wherebi=NiN,i∈{A,C,G,U} | (6) |
where N is the sliding window size and n is the coding dimension.
Binary profiles provide the position specific composition of nucleotides in RNA fragments [35,36]. A four-digit binary vector is used to encode each nucleotide. Dibinary profiles are different from binary profiles in that they are encoded for 16 dinucleotides, i.e., AA is denoted by (0, 0, 0, 0).
mRMR [37] is a commonly used feature selection method for compressing feature vector space. The goal of this technique is to identify a subset of features from the initial feature set that have the lowest correlation between features and the highest correlation with the output result. It considers the connection between features as well as the association between features and labels. The mechanism of feature selection is as follows.
The mutual information is used to find the feature subset S containing m features first, so that the m features found have the maximum correlation with the category c. The correlation between the feature subset S and the category c is defined by the average value of all mutual information between each feature and category as shown in (7).
maxD(S,c),D=1|S|∑xi∈SI(xi;c) | (7) |
where I(xi;c) is mutual information; S is a subset of features of length m; xi is the ith feature in S and c is category variable.
Then the features selected by the maximum correlation may be redundant, and (8) is used to eliminate the redundancy among m features.
minR(S),R=1|S|2∑xi,xj∈SI(xi;xj) | (8) |
The final feature subset S is obtained by combining the maximum correlation D with the minimum redundancy R.
mRMR=max[1|S|∑xi∈SI(xi;c)−1|S|2∑xi,xj∈SI(xi;xj)] | (9) |
Compared with other feature selection methods, the proposed algorithm considers the redundancy among features, further optimizes the feature subset, and solves the problem that the maximum dependency is difficult to achieve. However, only approximate optimal solutions can be obtained in practical applications.
Consider the binary classification issue using the training datasets
Dtrain={(u1,1),(u2,1),⋯,(um,1),(um+1,−1),(um+2,−1),⋯,(um+n,−1)}, | (10) |
where ui∈Rn,i=1,2,⋯,m+n.
Let T=(u1,u2,⋯,um)T∈Rm×n,F=(um+1,um+2,⋯,um+n)T∈Rn×n and l=m+n.
TWSVM [38] looks for a pair of nonparallel hyperplanes in the linear case.
w+u+b+=0andw−u+b−=0 | (11) |
where w+∈Rn,w−∈Rn,b+∈R,b−∈R by solving the following pair of QPPs:
minw+,b+,ξ−12(Tw++e+b+)T(Tw++e+b+)+c1eT−ξ−s.t.−(Fw++e−b+)+ξ−≥e−,ξ−≥0 | (12) |
and
minw−,b−,ξ+12(Fw−+e−b−)T(Fw−+e−b−)+c2eT+ξ+s.t.(Tw−+e+b−)+ξ+≥e+,ξ+≥0 | (13) |
where c1,c2 are the penalty parameters, e+,e− are all 1 vectors (e+,e−=[1⋯1]T) whose dimensions are the same as the number of positive and negative samples respectively, and ξ+,ξ− are slack vectors of appropriate dimension.
Minimizing the objective function means making a hyperplane as close as possible to one type of data, and the constraint requires that the distance between the hyperplane and the other type of data is at least greater than 1. Their corresponding Lagrange dual problems are
maxαeT−α−12αTJ(KTK)−1JTαs.t.0≤α≤c1e− | (14) |
and
maxγeT+γ−12γTK(JTJ)−1KTγs.t.0≤γ≤c2e+ | (15) |
where
K=[Te+]∈Rm×(n+1),J=[Fe−]∈Rn×(n+1). | (16) |
The solution to the primary problem can be acquired by addressing the dual problem, which can be obtained by
(wT+,b+)T=−(KTK)−1JTα, | (17) |
(wT−,b−)T=−(JTJ)−1KTγ. | (18) |
Therefore, an unknown point u∈Rn is predicted to the Class by
Class=argmins=−,+|wsu+bs|, | (19) |
where |⋅| is the perpendicular distance of point u from the planes wsu+bs=0,s=−,+.
This method not only divides a large quadratic programming problem into two small quadratic programming problems, which improves the training speed, but also is not very sensitive to noise.
Five indicators were widely used to assess how well the built models performed [39,40,41], accuracy (ACC), sensitivity (SN), specificity (SP), Matthew correlation coefficient (MCC), and integral area under the receiver operating characteristic curve (auROC), which were calculated using the following equations.
ACC=TP+TNTP+TN+FP+FN | (20) |
SN=TPFN+TP | (21) |
SP=TNTN+FP | (22) |
MCC=TN×TP−FN×FP√(TP+FP)×(TP+FN)×(TN+FN)×(TN+FP) | (23) |
where TP, TN, FP, and FN represent true positive, true negative, false positive and false negative, respectively.
We use 10-CV for comparison [42,43,44,45,46,47,48,49,50,51,52,53,54,55,56,57,58,59,60,61,62]. The training datasets are equally divided into ten subsets. The remaining one subset is tested after the proposed model has been trained using nine subsets. After each subset is tested once, the procedure is repeated ten times, and the average results represent the final performance. Finally, Ⅰ-testing was used in the testing datasets to evaluate the training model.
Feature extraction affects the results of subsequent sequence classification. To obtain better performance, this paper studied seven different features, including Kmer, PC-PseDNC-General and ANF, EIIP, ENAC, NCP + NBP from the RF-PseU method [20]. These features were first used in the experiment separately, and then multiple features were selected for different combinations according to the test results to obtain better experimental results.
Table 1 lists the results of feature combination for the H_990 dataset using the TWSVM methods. The first six rows are the performance of single features, with Kmer, EIIP, ENAC and NCP + NBP returning the best results, which are roughly distributed in the range of 0.57–0.59. Since the test results of single features were lower than those of the RF-PseU predictor, we combined several features that perform well and used the mRMR method to select the best feature for model construction. For the H_990 dataset, the combined characteristics listed in Table 1 have four results, including Kmer + ENAC, Kmer + EIIP, Kmer + NCP + NBP and Kmer + PC-PseDNC-General + ANF + EIIP + ENAC + NCP + NBP. Using the TWSVM method, the result of combined features was usually approximately 1–3% higher than that of single features, with a maximum ACC value of 0.65 and the best feature combination being KMER + PC-PseDNC-Generel +ANF + EIIP + ENAC + NCP + NBP. Compared with the RF-PseU predictor, the accuracy was improved by 0.7%. We chose this feature combination for the 10-CV of the training set H_990 and applied it to the Ⅰ-testing of the testing set H_200.
Feature Subset | TWSVM | ||||
ACC | MCC | SN | SP | AUC | |
Kmer | 0.59 | 0.181 | 0.533 | 0.646 | 0.618 |
PC-PseDNC-General | 0.534 | 0.07 | 0.434 | 0.635 | 0.543 |
ANF | 0.526 | 0.053 | 0.568 | 0.485 | 0.518 |
EIIP | 0.572 | 0.144 | 0.525 | 0.618 | 0.6 |
ENAC | 0.587 | 0.178 | 0.472 | 0.7 | 0.59 |
NCP + NBP | 0.584 | 0.172 | 0.53 | 0.639 | 0.582 |
Kmer + NCP + NBP | 0.59 | 0.182 | 0.532 | 0.648 | 0.587 |
Kmer + ENAC | 0.603 | 0.208 | 0.582 | 0.624 | 0.62 |
Kmer + EIIP | 0.606 | 0.212 | 0.585 | 0.626 | 0.625 |
Kmer + PC-PseDNC-Generel + ANF + EIIP + ENAC + NCP + NBP |
0.65 | 0.301 | 0.697 | 0.602 | 0.682 |
Table 2 lists the test results of different feature combinations on the S_628 dataset using the TWSVM method. Similar to the results in Table 1, the test results of Kmer, EIIP, ENAC and NCP + NBP were better. We combined those features that reported better performance. Except for Kmer + NCP + NBP, the results of other combined features were improved compared with the single feature results. Among them, the performance of the feature combination Kmer + PC – PseDNC – Generel + ANF + EIIP + ENAC + NCP + NBP was the best, with test results improved by approximately 6% compared to other combinations. We also used the independent test set S_200 to test this feature combination.
Feature Subset | TWSVM | ||||
ACC | MCC | SN | SP | AUC | |
Kmer | 0.627 | 0.259 | 0.625 | 0.63 | 0.67 |
PC-PseDNC-General | 0.574 | 0.153 | 0.666 | 0.483 | 0.589 |
ANF | 0.584 | 0.175 | 0.716 | 0.452 | 0.584 |
EIIP | 0.614 | 0.238 | 0.473 | 0.755 | 0.632 |
ENAC | 0.634 | 0.326 | 0.435 | 0.836 | 0.693 |
NCP + NBP | 0.669 | 0.34 | 0.659 | 0.678 | 0.711 |
Kmer + NCP + NBP | 0.664 | 0.33 | 0.653 | 0.675 | 0.683 |
Kmer + ENAC | 0.653 | 0.308 | 0.631 | 0.675 | 0.683 |
Kmer + EIIP | 0.631 | 0.263 | 0.628 | 0.634 | 0.662 |
Kmer + PC-PseDNC-Generel+ANF + EIIP + ENAC + NCP + NBP | 0.722 | 0.45 | 0.656 | 0.786 | 0.758 |
Table 3 shows the test results for the dataset M_994. The feature combination Kmer + PC-PseDNC-Generel + ANF + EIIP + ENAC + NCP + NBP had the best performance, and all test indicators were higher than the rest of the feature combinations. The combination was increased by approximately 5%, the MCC was increased by approximately 10%, and the AUC was also significantly improved.
Feature Subset | TWSVM | ||||
ACC | MCC | SN | SP | AUC | |
Kmer | 0.584 | 0.17 | 0.676 | 0.49 | 0.614 |
PC-PseDNC-General | 0.541 | 0.084 | 0.517 | 0.566 | 0.539 |
ANF | 0.553 | 0.113 | 0.71 | 0.396 | 0.527 |
EIIP | 0.625 | 0.276 | 0.826 | 0.424 | 0.632 |
ENAC | 0.664 | 0.329 | 0.667 | 0.661 | 0.7 |
NCP + NBP | 0.662 | 0.326 | 0.636 | 0.688 | 0.703 |
Kmer + NCP + NBP | 0.677 | 0.36 | 0.623 | 0.731 | 0.73 |
Kmer + ENAC | 0.662 | 0.326 | 0.657 | 0.667 | 0.704 |
Kmer + EIIP | 0.636 | 0.274 | 0.697 | 0.574 | 0.667 |
Kmer + PC-PseDNC-Generel + ANF + EIIP + ENAC + NCP + NBP | 0.728 | 0.462 | 0.795 | 0.661 | 0.775 |
In this study, we contrast the mRMR approach and the LightGBM method [64] since feature selection is a crucial component of model construction. Figure 2 shows their accuracy on the training datasets of the three species. The findings demonstrate that the performance of the mRMR technique is superior, which further enhances the classification accuracy of the model. The accuracy of the mRMR method on the three species is greater than that of the LightGBM approach.
The accuracy of classification results may be successfully increased by feature selection. We initially utilized the mRMR technique to pick feature subsets with high correlation with class labels and low feature redundancy to obtain the optimum feature dimension. To further obtain the feature dimension with the best precision, the incremental feature selection approach was applied. After many experiments, we found that the accuracy of Ⅰ-testing and 10-CV fluctuates as the number of characteristics rises and the highest accuracy mostly appeared within 100 or 150 dimensions, as illustrated in Figure 3. The accuracy of each species initially increases rapidly as the feature dimension increases, and then fluctuates continuously. For H.sapiens species, the highest 10-CV accuracy of 0.65 was obtained when the feature dimension reached 33, while the highest independent test accuracy of 0.763 was obtained at relatively low dimensions. The highest 10-CV accuracy of 0.722 and independent test accuracy of 0.825 for S. cerevisiae species were between 60–80 dimensions, obtained in 72 and 76 dimensions, respectively. M. musculus species only showed 10-CV results with the highest value at a feature dimension of 62.
Tables 4–6 show the changes of feature dimensions after feature selection and the distribution of feature subsets after optimization for the three species. It can be found that ENAC and EIIP occupy a large number in the optimized feature subset of the three species, followed by NCP and NBP, ANF and Kmer occupy a small number, and there is no PC-PseDNC-General in the optimized feature subset. It indicates that each feature has different contributions in the model, and ENAC and EIIP play an important role in the model.
Feature | The original dimension | The dimension after feature selection |
NCP | 63 | 14 |
EIIP | 21 | 21 |
NBP | 84 | 15 |
ENAC | 105 | 41 |
ANF | 21 | 8 |
Kmer | 64 | 1 |
PC-PseDNC-general | 22 | 0 |
Feature | The Original Dimension | The dimension after feature selection |
NCP | 93 | 22 |
EIIP | 31 | 31 |
NBP | 124 | 25 |
ENAC | 155 | 53 |
ANF | 31 | 19 |
Kmer | 256 | 0 |
PC-PseDNC-general | 18 | 0 |
Feature | The original dimension | The dimension after feature selection |
NCP | 63 | 11 |
EIIP | 21 | 21 |
NBP | 84 | 17 |
ENAC | 105 | 43 |
ANF | 21 | 7 |
Kmer | 64 | 1 |
PC-PseDNC-general | 22 | 0 |
Since many previous researchers built У sites recognition models based on support vector machines, we employed SVM [65] as a classifier in the same feature space to compare the performance of TWSVM with that of SVM. Figure 4 displays how it performed. The ACC, MCC, and AUC based on the TWSVM model were found to be larger than those based on the SVM model for the 10-CV results of the three species, while the independent test results may have more clearly indicated the difference between the two. All of the evaluation metrics outperformed the SVM model. As a result, we concluded that the TWSVM model performs much better than the SVM model, suggesting that it may be better suited for identifying У sites in RNA sequences.
The effectiveness of iPseU-TWSVM was also evaluated in comparison to other advanced predictors, such as iRNA-PseU [16], PseUI [17], iPseU-CNN [18], XG-PseU [19] and RF-PseU [20]. The 10-CV and Ⅰ-testing results of the advanced У site predictors using iPseU-TWSVM are contrasted in Tables 7 and 8, respectively. The 10-CV results reveal that the accuracy of iPseU-TWSVM on H.sapiens is 1.7% less accurate than that of the best predictor iPseU-CNN on this species, and the accuracy on S.cerevisiae and M.musculus is 0.722 and 0.728, respectively, which is 2.6 and 2.0% less accurate than that of the best predictor RF-PseU. Although iPseU-TWSVM does not perform optimally on the training set, iPseU-TWSVM has higher accuracy than other predictors on all species in terms of Ⅰ-testing. H.sapiens and S.cerevisiae are 1.3 and 5.5% more accurate in Ⅰ-testing than the best predictor RF-PseU, with corresponding accuracy values of 0.763 and 0.825, respectively. We also calculated the average accuracy of several species so that we could compare the predictors' performance in depth. As shown in Table 9, the 10-CV accuracy of iPseU-TWSVM is 1.3% less than that of RF-PseU. In terms of Ⅰ-testing, iPseU-TWSVM is significantly improved by 3.4% compared with RF-PseU. iPseU-TWSVM performs much better overall than the other predictors. The findings demonstrate that iPseU-TWSVM, a very practical technique, has greater generalization performance and is more appropriate for recognizing У sites in RNA sequences.
Species | Classifier | Cross-validation | ||||
ACC | MCC | SN | SP | AUC | ||
H.sapiens | iRNA-PseU | 0.604 | 0.21 | 0.61 | 0.598 | 0.64 |
PseUI | 0.642 | 0.28 | 0.649 | 0.636 | 0.68 | |
iRNA-CNN | 0.667 | 0.34 | 0.65 | 0.688 | / | |
XG-PseU | 0.661 | 0.32 | 0.635 | 0.687 | 0.7 | |
RF-PseU | 0.643 | 0.29 | 0.661 | 0.626 | 0.7 | |
iPseU-TWSVM | 0.65 | 0.301 | 0.697 | 0.602 | 0.682 | |
S.cerevisiae | iRNA-PseU | 0.645 | 0.29 | 0.647 | 0.643 | 0.81 |
PseUI | 0.641 | 0.3 | 0.647 | 0.675 | 0.69 | |
iRNA-CNN | 0.682 | 0.37 | 0.664 | 0.705 | / | |
XG-PseU | 0.682 | 0.37 | 0.668 | 0.695 | 0.77 | |
RF-PseU | 0.748 | 0.49 | 0.772 | 0.724 | 0.81 | |
iPseU-TWSVM | 0.722 | 0.45 | 0.656 | 0.786 | 0.758 | |
M.musculus | iRNA-PseU | 0.691 | 0.38 | 0.733 | 0.648 | 0.75 |
PseUI | 0.704 | 0.41 | 0.799 | 0.703 | 0.71 | |
iRNA-CNN | 0.718 | 0.44 | 0.748 | 0.691 | / | |
XG-PseU | 0.72 | 0.45 | 0.765 | 0.676 | 0.74 | |
RF-PseU | 0.748 | 0.5 | 0.731 | 0.765 | 0.796 | |
iPseU-TWSVM | 0.728 | 0.462 | 0.795 | 0.661 | 0.775 |
Species | Classifier | Independent testing | ||||
ACC | MCC | SN | SP | AUC | ||
H.sapiens | iRNA-PseU | 0.65 | 0.3 | 0.6 | 0.7 | / |
PseUI | 0.655 | 0.31 | 0.63 | 0.7 | / | |
iRNA-CNN | 0.69 | 0.4 | 0.777 | 0.68 | / | |
XG-PseU | 0.675 | / | / | 0.608 | / | |
RF-PseU | 0.75 | 0.5 | 0.78 | 0.72 | 0.8 | |
iPseU-TWSVM | 0.763 | 0.529 | 0.825 | 0.7 | 0.786 | |
S.cerevisiae | iRNA-PseU | 0.6 | 0.2 | 0.63 | 0.57 | / |
PseUI | 0.685 | 0.37 | 0.65 | 0.72 | / | |
iRNA-CNN | 0.735 | 0.47 | 0.688 | 0.778 | / | |
XG-PseU | 0.71 | / | / | / | / | |
RF-PseU | 0.77 | 0.54 | 0.75 | 0.79 | 0.838 | |
iPseU-TWSVM | 0.825 | 0.65 | 0.85 | 0.8 | 0.905 |
Scores type | iPseU-TWSVM | RF-PseU | XG-PseU | iRNA-CNN | PseUI | iRNA-PseU |
Cross-validation | 0.7 | 0.713 | 0.687 | 0.689 | 0.662 | 0.647 |
Independent testing | 0.794 | 0.76 | 0.693 | 0.713 | 0.7 | 0.625 |
This work proposes the use of a novel model called iPseU-TWSVM to identify RNA У sites across various species. We have used an efficient feature selection method to obtain the best feature subset and selected TWSVM as the classifier to increase recognition accuracy. Finally, we compared advanced predictors and found that iPseU-TWSVM significantly improved the independent test accuracy by 3.4%, while the accuracy of cross validation was lower by 1.3%. Through comprehensive analysis, it was concluded that the relatively poor performance of the training datasets was due to the following two reasons. One is that the features used by the best predictor are different, and the other is that the classifier of the model is different. The above results indicate that iPseU-TWSVM had better generalization performance and could more accurately identify У sites from RNA sequences. It is anticipated that iPseU-TWSVM will be effective in identifying RNA У sites.
The contribution of this work has the following three aspects: (ⅰ) the model uses TWSVM as a classifier, which improves the accuracy of the model and improves the training speed; (ⅱ) the model has good generalization performance and can be applied to the prediction of other sites in the sequence; (ⅲ) further accurate identification of У sites in the sequence lays the foundation for disease control and related drug development. At the same time, this work also has the following shortcomings: (ⅰ) in the feature selection part, only two algorithms are compared, and subsequent research can try other algorithms to further improve the feature subset; (ⅱ) the model uses TWSVM as a classifier. In the original problem of TWSVM, only empirical risk is minimized, but structural risk is not minimized. Moreover, the algorithm can only obtain approximate solutions. Subsequent research can consider improving TWSVM or try other classification algorithms as the classifier of the model to improve the prediction performance. Future work will study emerging methods [66,67,68,69,70,71,72,73,74,75,76,77,78,79,80,81] to further improve the accuracy of the model.
This paper is supported by the National Natural Science Foundation of China (NSFC 62172076, 62072385), and the Municipal Government of Quzhou under Grant Number 2020D003 and 2021D004.
The authors declare there is no conflict of interest.
[1] |
J. P. Agnelli, B. Buffa, D. A. Knopoff, G. Torres, A spatial kinetic model of crowd evacuation dynamics with infectious disease contagion, Bull Math Biol, 85(2023), 23. https://doi.org/10.1007/s11538-023-01127-6 doi: 10.1007/s11538-023-01127-6
![]() |
[2] |
M. Aguiar, G. Dosi, D. A. Knopoff, M.E. Virgillito, A multiscale network-based model of contagion dynamics: heterogeneity, spatial distancing and vaccination, Math Models Methods Appl Sci, 31 (2021), 2425–2570. https://doi.org/10.1142/S0218202521500524 doi: 10.1142/S0218202521500524
![]() |
[3] |
K. G. Andersen, A. Rambaut, W. Ian Lipkin, E. C. Holmes, R. F. Garry, The proximal origin of SARS-CoV-2, Nat. Med., 26 (2020), 450–452. https://doi.org/10.1038/s41591-020-0820-9 doi: 10.1038/s41591-020-0820-9
![]() |
[4] |
S. Asgari, L. A. Pousaz, Human genetic variants identified that affect Covid susceptibility and severity, Nature, 600 (2021), 690–691. https://doi.org/10.1038/s41586-021-04210-x doi: 10.1038/s41586-021-04210-x
![]() |
[5] |
A. Atifa, M. A. Khan, K. Isakakova, F. S. Al-Duais, I. Ahmad, Mathematical modelling and analysis of the SARS-CoV-2 disease with reinfection, Comput. Biol. Chem., 98 (2022), 107678. https://doi.org/10.1016/j.compbiolchem.2022.107678 doi: 10.1016/j.compbiolchem.2022.107678
![]() |
[6] | B. Avishai, The pandemic isn't a black swan but a portent of a more fragile global system. The New Yorker, 2020. Available from: https://www.newyorker.com/news/daily-comment/the-pandemic-isnt-a-black-swan-but-a-portent-of-a-more-fragile-global-system |
[7] | Y. M. Bar-On, A. Flamholz, R. Phillips, R. Milo, SARS-CoV-2 (COVID-19) by the numbers, eLife, 9, e57309, (2020). https://doi.org/10.7554/eLife.57309 |
[8] |
N. Bellomo, R. Bingham, M. Chaplain, G. Dosi, G. Forni, D. Knopoff, et al., A multi-scale model of virus pandemic: Heterogeneous interactive entities in a globally connected world, Math Models Methods Appl Sci, 30 (2020), 1591–1651. https://doi.org/10.1142/S0218202520500323 doi: 10.1142/S0218202520500323
![]() |
[9] |
N. Bellomo, F. Brezzi, M. Chaplain, Modelling Virus pandemics in a globally connected world, a challenge towards a mathematics for living lystems, Math Models Methods Appl Sci, 31 (2021), 2391–2397. https://doi.org/10.1142/S0218202521020024 doi: 10.1142/S0218202521020024
![]() |
[10] |
N. Bellomo, D. Burini, G. Dosi, L. Gibelli, D. A. Knopoff, N. Outada, et al., What is life? A perspective of the mathematical kinetic theory of active particles, Math Models Methods Appl Sci, 31 (2021), 1821–1866. https://doi.org/10.1142/S0218202521500408 doi: 10.1142/S0218202521500408
![]() |
[11] |
N. Bellomo, D. Burini, N. Outada, Multiscale models of Covid-19 with mutations and variants, Netw. Heterog. Media., 17 (2022), 293–310. https://doi.org/10.3934/nhm.2022008 doi: 10.3934/nhm.2022008
![]() |
[12] |
N. Bellomo, D. Burini, N. Outada, Pandemics of Mutating Virus and Society: A multi-scale active particles approach, Philos. Trans. Royal Soc. A, 380 (2022), 20210161. https://doi.org/10.1098/rsta.2021.0161 doi: 10.1098/rsta.2021.0161
![]() |
[13] |
N. Bellomo, L. Gibelli, N. Outada, On the interplay between behavioral dynamics and social interactions in human crowds, Kinet. Relat. Models, 12 (2019), 397–409. https://doi.org/10.3934/krm.2019017 doi: 10.3934/krm.2019017
![]() |
[14] |
G. Bertaglia, L. Pareschi, Hyperbolic compartmental models for epidemic spread on networks with uncertain data: application to the emergence of Covid-19 in Italy, Math Models Methods Appl Sci, 31 (2021), 2495–2531. https://doi.org/10.1142/S0218202521500548 doi: 10.1142/S0218202521500548
![]() |
[15] | G. Bertaglia, A. Bondesan, D. Burini, R. Eftimie, L. Pareschi, G. Toscani, New trends on the systems approach to modeling SARS-CoV-2 pandemics in a globally connected planet, Math Models Methods Appl Sci, (2024). https://doi.org/10.1142/S0218202524500301 |
[16] |
A. L. Bertozzi, E. Franco, G. Mohler, M. B. Short, D. Sledge, The challenges of modeling and forecasting the spread of COVID-19, Proc. Natl. Acad. Sci., 117 (2020), 16732–16738. https://doi.org/10.1073/pnas.2006520117 doi: 10.1073/pnas.2006520117
![]() |
[17] | J. Borghans, R. M. Ribeiro, The maths of memory, eLife, 6 (2017), e26754. https://doi.org/10.7554/eLife.26754 |
[18] |
D. Burini, D. A. Knopoff, Epidemics and society—A Multiscale vision from the small world to the globally interconnected world, Math Models Methods Appl Sci, 34 (2024), 1564–1594. https://doi.org/10.1142/S0218202524500295 doi: 10.1142/S0218202524500295
![]() |
[19] |
J. D. Challenger, C. Y. Foo, Y. Wu, A. W. C. Yan, M. M. Marjaneh, F. Liew, et al., Modelling upper respiratory viral load dynamics of SARS-CoV-2, BMC Med, 20 (2022), 25. https://doi.org/10.1186/s12916-021-02220-0 doi: 10.1186/s12916-021-02220-0
![]() |
[20] |
R. J. De Boer, D. Homann, A. S. Perelson, Different dynamics of CD4+ and CD8+ T cell responses during and after acute lymphocytic choriomeningitis virus infection, J Immunol, 171 (2003), 3928–3935. https://doi.org/10.4049/jimmunol.171.8.3928 doi: 10.4049/jimmunol.171.8.3928
![]() |
[21] |
J. Demongeot, Q. Griette, P. Magal, G. Webb, Vaccine efficacy for COVID-19 outbreak in New York City, Biology, 11 (2022), 345. https://doi.org/10.3390/biology11030345 doi: 10.3390/biology11030345
![]() |
[22] |
M. S. Diamond, T. D. Kanneganti, Innate immunity: the first line of defense against SARS-CoV-2, Nat Immunol, 23 (2022), 165–176. https://doi.org/10.1038/s41590-021-01091-0 doi: 10.1038/s41590-021-01091-0
![]() |
[23] |
G. Dosi, L. Fanti, M. E. Virgillito, Unequal societies in usual times, unjust societies in pandemic ones, J. Ind. Bus. Econ., 47 (2020), 371–389. https://doi.org/10.1007/s40812-020-00173-8 doi: 10.1007/s40812-020-00173-8
![]() |
[24] |
R. Eftimie, Grand challenges in mathematical biology: Integrating multi-scale modeling and data, Front Ecol Environ, 8 (2022), 1010622. https://doi.org/10.3389/fams.2022.1010622 doi: 10.3389/fams.2022.1010622
![]() |
[25] |
I. Eizenberg-Magar, I. Rimer, I. Zaretsky, N. Friedman, Diverse continuum of CD4+ T-cell states is determined by hierarchical additive integration of cytokine signals, Proc. Natl. Acad. Sci., 114 (2017), E6447–E6456. https://doi.org/10.1073/pnas.1615590114 doi: 10.1073/pnas.1615590114
![]() |
[26] |
S. El Zein, O. Chehab, A. Kanj, S. Akrawe, S. Alkassis, T. Mishra et al., SARS-CoV-2 infection: Initial viral load (iVL) predicts severity of illness/outcome, and declining trend of iVL in hospitalized patients corresponds with slowing of the pandemic, PLoS One, 16 (2021), e0255981. https://doi.org/10.1371/journal.pone.0255981 doi: 10.1371/journal.pone.0255981
![]() |
[27] | M. Elemans, N. K. S. Al Basatena, B. Asquith, The efficiency of the human CD8+ T cell response: how should we quantify it, what determines it, and does it matter? Plos Comput Biol, 8 (2012), e1002381. https://doi.org/10.1371/journal.pcbi.1002381 |
[28] |
F. Flandoli, E. La Fauci, M. Riva, Individual-based Markov model of virus diffusion: Comparison with COVID-19 incubation period, serial interval and regional time series, Math Models Methods Appl Sci, 31 (2021), 907–939. https://doi.org/10.1142/S0218202521500226 doi: 10.1142/S0218202521500226
![]() |
[29] | J. F. Fontanari, A stochastic model for the influence of social distancing on loneliness, Physica A, 584 (2021), 126367. |
[30] |
M. Gatto, E. Bertuzzo, L. Mari, S. Miccoli, L. Carraro, R. Casagrandi, et al., Spread and dynamics of the COVID-19 epidemic in Italy: Effects of emergency containment measures, Proc. Natl. Acad. Sci., 117 (2020), 10484–10491. https://doi.org/10.1073/pnas.2004978117 doi: 10.1073/pnas.2004978117
![]() |
[31] |
N. M. Gerhards, J. B. W. J. Cornelissen, L. J. M. van Keulen, J. Harders-Westerveen, R. Vloet, B. Smid, et. al., Predictive value of precision-cut lung slices for the susceptibility of three animal species for SARS-CoV-2 and validation in a refined hamster model, Pathogens, 10 (2021), 824. https://doi.org/10.3390/pathogens10070824 doi: 10.3390/pathogens10070824
![]() |
[32] |
G. Gessain, C. Blériot, F. Ginhoux, Non-genetic heterogeneity of macrophages in diseases–a medical perspective, Front. Cell. Dev. Biol., 8 (2020), 613116. https://doi.org/10.3389/fcell.2020.613116 doi: 10.3389/fcell.2020.613116
![]() |
[33] |
C. Franceschi, S. Salvioli, P. Garagnani, M de Eguileor, D. Monti, M.Capri, Immunobiography and the heterogeneity of immune responses in the elderly: a focus on inflammaging and trained immunity, Front. Immunol., 8 (2017), 982. https://doi.org/10.3389/fimmu.2017.00982 doi: 10.3389/fimmu.2017.00982
![]() |
[34] |
J. F. Gianlupi, T. Mapder, T. J. Sego, J. P. Sluka, S. K. Quinney, M. Craig, et al., Multiscale model of antiviral timing, potency, and heterogeneity effects on an epithelial tissue patch infected by SARS-CoV-2, Viruses, 14 (2022), 605. https://doi.org/10.3390/v14030605 doi: 10.3390/v14030605
![]() |
[35] |
C. H. Hansen, D. Michlmayr, S. M. Gubbels, K. Mølbak, S. Ethelberg, Assessment of protection against reinfection with SARS-CoV-2 among 4 million PCR-tested individuals in Denmark in 2020: a population-level observational study, Lancet, 397 (2021), 1204–1212. https://doi.org/10.1016/S0140-6736(21)00575-4 doi: 10.1016/S0140-6736(21)00575-4
![]() |
[36] |
P. Hardy, L. S. Marcolino, J. F. Fontanari, The paradox of productivity during quarantine: an agent-based simulation, Eur. Phys. J. B., 94 (2021), 40. https://doi.org/10.1140/epjb/s10051-020-00016-4 doi: 10.1140/epjb/s10051-020-00016-4
![]() |
[37] |
S. Karimzadeh, R. Bophal, H. N. Tien, Review of infective dose, routes of transmission and outcome of COVID-19 caused by the SARS-CoV-2: comparison with other respiratory viruses, Epidemiol. Infect., 149 (2021), e96. https://doi.org/10.1017/S0950268821000790 doi: 10.1017/S0950268821000790
![]() |
[38] |
R. Karki, B. R. Sharma, S. Tuladhar, E. P. Williams, L. Zalduondo, P. Samir, et al., Synergism of TNF-α and IFN-γ triggers inflammatory cell death, tissue damage, and mortality in SARS-CoV-2 infection and cytokine shock syndrome, Cell, 184 (2021), 149–168. https://doi.org/10.1016/j.cell.2020.11.025 doi: 10.1016/j.cell.2020.11.025
![]() |
[39] |
D. Kim, A. Quaini, Coupling kinetic theory approaches for pedestrian dynamics and disease contagion in a confined environment, Math Models Methods Appl Sci, 30 (2020), 1893–1915. https://doi.org/10.1142/S0218202520400126 doi: 10.1142/S0218202520400126
![]() |
[40] |
S. M. Kissler, C. Tedijanto, E. Goldstein, Y. H. Grad, M. Lipsitch, Projecting the transmission dynamics of SARS-CoV-2 through the postpandemic period, Science, 368 (2020), 860–868. https://doi.org/10.1126/science.abb5793 doi: 10.1126/science.abb5793
![]() |
[41] | Z. Liu, P. Magal, O. Seydi, G. Webb, A model to predict COVID-19 epidemics with applications to South Korea, Italy, and Spain, SIAM News (2020). Available from: https://sinews.siam.org/Details-Page/a-model-to-predict-covid-19-epidemics-with-applications-to-south-korea-italy-and-spain |
[42] |
S. M. Lynch, G. Guo, D. S. Gibson, A. J. Bjourson, T. Singh Ra, Role of Senescence and Aging in SARS-CoV-2 Infection and COVID-19 Disease, Cells, 10 (2021), 3367–3372. https://doi.org/10.3390/cells10123367 doi: 10.3390/cells10123367
![]() |
[43] |
D. C. Macallan, R. Busch, B. Asquith, Current estimates of T cell kinetics in humans, Curr. Opin. Syst. Biol., 18 (2019), 77–86. https://doi.org/10.1016/j.coisb.2019.10.002 doi: 10.1016/j.coisb.2019.10.002
![]() |
[44] |
A. Mantovani, M. Rescigno, G. Forni, F. Tognon, G. Putoto, J. Ictho, P. Lochoro, COVID-19 vaccines and a perspective on Africa, Trends Immunol, 44 (2023), 172–187. https://doi.org/10.1016/j.it.2023.01.005 doi: 10.1016/j.it.2023.01.005
![]() |
[45] |
J. S. Marshall, R. Warrington, W. Watson, H. L. Kim, An introduction to immunology and immunopathology, Allergy Asthma CL IM, 14 (2018), 49. https://doi.org/10.1186/s13223-018-0289-y doi: 10.1186/s13223-018-0289-y
![]() |
[46] |
M. Massard, R. Eftimie, A. Perasso, B. Saussereau, A multi-strain epidemic model for COVID-19 with infected and asymptomatic cases: application to French data, J. Theor. Biol., 545 (2022), 111117. https://doi.org/10.1016/j.jtbi.2022.111117 doi: 10.1016/j.jtbi.2022.111117
![]() |
[47] |
P. M. Matricardi, R. W. Dal Negro, R. Nisini, The first, holistic immunological model of COVID-19: Implications for prevention, diagnosis, and public health measures, Pediatr Allergy Immunol, 31 (2020), 454–470. https://doi.org/10.1111/pai.13271 doi: 10.1111/pai.13271
![]() |
[48] |
E. Meffre, A. Iwasaki, Interferon deficiency can lead to severe COVID, Nature, 587 (2020), 374–376. https://doi.org/10.1038/d41586-020-03070-1 doi: 10.1038/d41586-020-03070-1
![]() |
[49] |
P. Moss, The T cell immune response against SARS-CoV-2, Nat. Immunol., 23 (2022), 186–193. https://doi.org/10.1038/s41590-021-01122-w doi: 10.1038/s41590-021-01122-w
![]() |
[50] | P. Musiani, G. Forni, Basic Immunology, Padua: Piccin, 2018. |
[51] |
M. G. Netea, J. Dominguez-Andrés, L. B. Barreiro, T. Chavakis, M. Divangahi, E. Fuchs, et al., Defining trained immunity and its role in health and disease, Nat. Rev. Immunol., 20 (2020), 375–388. https://doi.org/10.1038/s41577-020-0285-6 doi: 10.1038/s41577-020-0285-6
![]() |
[52] |
A. H. Newton, A. Cardani, T. J. Braciale, The host immune response in respiratory virus infection: balancing virus clearance and immunopathology, Semin. Immunol., 38 (2016), 471–482. https://doi.org/10.1007/s00281-016-0558-0 doi: 10.1007/s00281-016-0558-0
![]() |
[53] |
J. Niessl, T. Sekine, M. Buggert, T cell immunity to SARS-CoV-2, Seminars in Immunology, 55 (2021), 101505. https://doi.org/10.1016/j.smim.2021.101505 doi: 10.1016/j.smim.2021.101505
![]() |
[54] |
A. Paolini, R. Borella, S. De Biasi, A. Neroni, M. Mattioli, D. Lo Tartaro, et al., Cell death in coronavirus infections: uncovering its role during COVID-19, Cells, 10 (2021), 1585. https://doi.org/10.3390/cells10071585 doi: 10.3390/cells10071585
![]() |
[55] | B. Perthame, Transport Equations in Biology, Boston: Birkhäuser Basel, 2006. |
[56] |
J. J. Pinney, F. Rivera-Escalera, C. C. Chu, H. E. Whitehead, K.R. VanDerMeid, A.M. Nelson, et al., Macrophage hypophagia as a mechanism of innate immune exhaustion in mAb-induced cell clearance, Blood, 136 (2020), 2065–2079. https://doi.org/10.1182/blood.2020005571 doi: 10.1182/blood.2020005571
![]() |
[57] |
D. Pople, E. J. M. Monk, S. Evans, S. Foulkes, J. Islam, E. Wllington, et al., Burden of SARS-CoV-2 infection in healthcare workers during second wave in England and impact of vaccines: prospective multicentre cohort study (SIREN) and mathematical model, BMJ, 378 (2022), e070379. https://doi.org/10.1136/bmj-2022-070379 doi: 10.1136/bmj-2022-070379
![]() |
[58] |
M. Renardy, C. Hult, S. Evans, J. J. Linderman, D. E. Kirschner, Global sensitivity analysis of biological multiscale models, Curr. Opin. Biomed. Eng., 11 (2019), 109–116. https://doi.org/10.1016/j.cobme.2019.09.012 doi: 10.1016/j.cobme.2019.09.012
![]() |
[59] |
D. Ricci, M. P. Etna, F. Rizzo, S. Sandini, M. Severa, E. M. Coccia, Innate immune response to SARS-CoV-2 infection: from cells to soluble mediators, Int. J. Mol. Sci., 22 (2021), 7017. https://doi.org/10.3390/ijms22137017 doi: 10.3390/ijms22137017
![]() |
[60] |
R. Robinot, M. Hubert, G. Dias de Melo, F. Lazarini, T. Bruel, N. Smith, et al., SARS-CoV-2 infection induces the dedifferentiation of multiciliated cells and impairs mucociliary clearance, Nat Commun, 12 (2021), 4354. https://doi.org/10.1038/s41467-021-24521-x doi: 10.1038/s41467-021-24521-x
![]() |
[61] | Royal Society (Coordinator), RAMP: A call for assistance, addressed to the scientific modelling community. Coordinated by Mark Chaplain, 2021. Available from: https://epcced.github.io/ramp/ |
[62] |
G. Seminara, B. Carli, G. Forni, S. Fuzzi, A. Mazzino, A. Rinaldo, Biological fluid dynamics of airborne COVID.19 infection, Rend. Fis. Acc. Lincei, 31 (2020), 505–537. https://doi.org/10.1007/s12210-020-00938-2 doi: 10.1007/s12210-020-00938-2
![]() |
[63] |
A. Seller, C. Hackenbruch, J. S. Walz, A. Nelde, J. S. Heitmann, Long-term follow-up of COVID-19 convalescents–immune response associated with reinfection rate and symptoms, Viruses, 15 (2023), 2100. https://doi.org/10.3390/v15102100 doi: 10.3390/v15102100
![]() |
[64] |
R. Sender, Y. M. Bar-On, S. Gleizer, B. Bernshtein, A. Flamholz, R. Phillips, et al., The total number and mass of SARS-CoV-2 virions, Proc. Natl. Acad. Sci., 118 (2021), e2024815118. https://doi.org/10.1073/pnas.2024815118 doi: 10.1073/pnas.2024815118
![]() |
[65] |
A. Sette, S. Crotty, Adaptive immunity to SARS-CoV-2 and COVID-19, Cell, 184 (2021), 861–880. https://doi.org/10.1016/j.cell.2021.01.007 doi: 10.1016/j.cell.2021.01.007
![]() |
[66] |
H. Shen, D. Chen, C. Li, T. Huang, W. Ma, A mini review of reinfection with the SARS-CoV-2 Omicron variant, Health Sci. Rep., 7 (2024), e2016. https://doi.org/10.1002/hsr2.2016 doi: 10.1002/hsr2.2016
![]() |
[67] |
A. T. Tan, M. Linster, C. W. Tan, N. L. Bert, W. N. Chia, K. Kunasegaran, et al., Early induction of functional SARS-CoV-2-specific T cells associates with rapid viral clearance and mild disease in COVID-19 patients, Cell Rep., 34 (2021), 108728. https://doi.org/10.1016/j.celrep.2021.108728 doi: 10.1016/j.celrep.2021.108728
![]() |
[68] |
G. Toscani, P. Sen, S. Biswas, Kinetic exchange models of societies and economies, Philos. Trans. Royal Soc. A, 380 (2022), 20210170. https://doi.org/10.1098/rsta.2021.0170 doi: 10.1098/rsta.2021.0170
![]() |
[69] | The University of Edinburgh, Review: what is the infectious dose of SARS-CoV-2? Usher Institute, (2021). Available from: https://www.ed.ac.uk/files/atoms/files/uncover_029-01_review_infectious_dose_of_covid-19.pdf. |
[70] |
J. D. Van Belleghem, P. L. Bollyky, Macrophages and innate immune memory against Staphylococcus skin infections, Proc. Natl. Acad. Sci., 115 (2018), 11865–11867. https://doi.org/10.1073/pnas.1816935115 doi: 10.1073/pnas.1816935115
![]() |
[71] |
E. Vazquez-Alejo, L. Tarancon-Diez, M. de la Sierra Espinar-Buitrago, M. Genebat, A. Calderón, G. Pérez-Cabeza, et al., Persistent exhausted T-cell immunity after severe COVID-19: 6-month evaluation in a prospective observational study, J. Clin. Med., 12 (2023), 3539. https://doi.org/10.3390/jcm12103539 doi: 10.3390/jcm12103539
![]() |
[72] |
D. J. Verdon, M. Mulazzani, M.R. Jenkins, Cellular and molecular mechanisms of CD8+ T cell differentiation, dysfunction and exhaustion, Int. J. Mol. Sci., 21 (2020), 7357. https://doi.org/10.3390/ijms21197357 doi: 10.3390/ijms21197357
![]() |
[73] | W. Van Damme, R. Dahake, R. van de Pas, G. Vanham, Y. Assefa, COVID-19: Does the infectious inoculum dose-response relationship contribute to understanding heterogeneity in disease severity and transmission dynamics? Med. Hypotheses, 146 (2021), 110431. https://doi.org/10.1016/j.mehy.2020.110431 |
[74] |
S. Wang, M. Hao, Z. Pan, J. Lei, X. Zou, Data-driven multiscale mathematical modeling of SARS-CoV-2 infection revels heterogeneity among COVID-19 patients, PLoS Comput. Biol., 17 (2021), e1009587. https://doi.org/10.1371/journal.pcbi.1009587 doi: 10.1371/journal.pcbi.1009587
![]() |
1. | Mingzhao Wang, Haider Ali, Yandi Xu, Juanying Xie, Shengquan Xu, BiPSTP: Sequence feature encoding method for identifying different RNA modifications with bidirectional position-specific trinucleotides propensities, 2024, 300, 00219258, 107140, 10.1016/j.jbc.2024.107140 |
Feature Subset | TWSVM | ||||
ACC | MCC | SN | SP | AUC | |
Kmer | 0.59 | 0.181 | 0.533 | 0.646 | 0.618 |
PC-PseDNC-General | 0.534 | 0.07 | 0.434 | 0.635 | 0.543 |
ANF | 0.526 | 0.053 | 0.568 | 0.485 | 0.518 |
EIIP | 0.572 | 0.144 | 0.525 | 0.618 | 0.6 |
ENAC | 0.587 | 0.178 | 0.472 | 0.7 | 0.59 |
NCP + NBP | 0.584 | 0.172 | 0.53 | 0.639 | 0.582 |
Kmer + NCP + NBP | 0.59 | 0.182 | 0.532 | 0.648 | 0.587 |
Kmer + ENAC | 0.603 | 0.208 | 0.582 | 0.624 | 0.62 |
Kmer + EIIP | 0.606 | 0.212 | 0.585 | 0.626 | 0.625 |
Kmer + PC-PseDNC-Generel + ANF + EIIP + ENAC + NCP + NBP |
0.65 | 0.301 | 0.697 | 0.602 | 0.682 |
Feature Subset | TWSVM | ||||
ACC | MCC | SN | SP | AUC | |
Kmer | 0.627 | 0.259 | 0.625 | 0.63 | 0.67 |
PC-PseDNC-General | 0.574 | 0.153 | 0.666 | 0.483 | 0.589 |
ANF | 0.584 | 0.175 | 0.716 | 0.452 | 0.584 |
EIIP | 0.614 | 0.238 | 0.473 | 0.755 | 0.632 |
ENAC | 0.634 | 0.326 | 0.435 | 0.836 | 0.693 |
NCP + NBP | 0.669 | 0.34 | 0.659 | 0.678 | 0.711 |
Kmer + NCP + NBP | 0.664 | 0.33 | 0.653 | 0.675 | 0.683 |
Kmer + ENAC | 0.653 | 0.308 | 0.631 | 0.675 | 0.683 |
Kmer + EIIP | 0.631 | 0.263 | 0.628 | 0.634 | 0.662 |
Kmer + PC-PseDNC-Generel+ANF + EIIP + ENAC + NCP + NBP | 0.722 | 0.45 | 0.656 | 0.786 | 0.758 |
Feature Subset | TWSVM | ||||
ACC | MCC | SN | SP | AUC | |
Kmer | 0.584 | 0.17 | 0.676 | 0.49 | 0.614 |
PC-PseDNC-General | 0.541 | 0.084 | 0.517 | 0.566 | 0.539 |
ANF | 0.553 | 0.113 | 0.71 | 0.396 | 0.527 |
EIIP | 0.625 | 0.276 | 0.826 | 0.424 | 0.632 |
ENAC | 0.664 | 0.329 | 0.667 | 0.661 | 0.7 |
NCP + NBP | 0.662 | 0.326 | 0.636 | 0.688 | 0.703 |
Kmer + NCP + NBP | 0.677 | 0.36 | 0.623 | 0.731 | 0.73 |
Kmer + ENAC | 0.662 | 0.326 | 0.657 | 0.667 | 0.704 |
Kmer + EIIP | 0.636 | 0.274 | 0.697 | 0.574 | 0.667 |
Kmer + PC-PseDNC-Generel + ANF + EIIP + ENAC + NCP + NBP | 0.728 | 0.462 | 0.795 | 0.661 | 0.775 |
Feature | The original dimension | The dimension after feature selection |
NCP | 63 | 14 |
EIIP | 21 | 21 |
NBP | 84 | 15 |
ENAC | 105 | 41 |
ANF | 21 | 8 |
Kmer | 64 | 1 |
PC-PseDNC-general | 22 | 0 |
Feature | The Original Dimension | The dimension after feature selection |
NCP | 93 | 22 |
EIIP | 31 | 31 |
NBP | 124 | 25 |
ENAC | 155 | 53 |
ANF | 31 | 19 |
Kmer | 256 | 0 |
PC-PseDNC-general | 18 | 0 |
Feature | The original dimension | The dimension after feature selection |
NCP | 63 | 11 |
EIIP | 21 | 21 |
NBP | 84 | 17 |
ENAC | 105 | 43 |
ANF | 21 | 7 |
Kmer | 64 | 1 |
PC-PseDNC-general | 22 | 0 |
Species | Classifier | Cross-validation | ||||
ACC | MCC | SN | SP | AUC | ||
H.sapiens | iRNA-PseU | 0.604 | 0.21 | 0.61 | 0.598 | 0.64 |
PseUI | 0.642 | 0.28 | 0.649 | 0.636 | 0.68 | |
iRNA-CNN | 0.667 | 0.34 | 0.65 | 0.688 | / | |
XG-PseU | 0.661 | 0.32 | 0.635 | 0.687 | 0.7 | |
RF-PseU | 0.643 | 0.29 | 0.661 | 0.626 | 0.7 | |
iPseU-TWSVM | 0.65 | 0.301 | 0.697 | 0.602 | 0.682 | |
S.cerevisiae | iRNA-PseU | 0.645 | 0.29 | 0.647 | 0.643 | 0.81 |
PseUI | 0.641 | 0.3 | 0.647 | 0.675 | 0.69 | |
iRNA-CNN | 0.682 | 0.37 | 0.664 | 0.705 | / | |
XG-PseU | 0.682 | 0.37 | 0.668 | 0.695 | 0.77 | |
RF-PseU | 0.748 | 0.49 | 0.772 | 0.724 | 0.81 | |
iPseU-TWSVM | 0.722 | 0.45 | 0.656 | 0.786 | 0.758 | |
M.musculus | iRNA-PseU | 0.691 | 0.38 | 0.733 | 0.648 | 0.75 |
PseUI | 0.704 | 0.41 | 0.799 | 0.703 | 0.71 | |
iRNA-CNN | 0.718 | 0.44 | 0.748 | 0.691 | / | |
XG-PseU | 0.72 | 0.45 | 0.765 | 0.676 | 0.74 | |
RF-PseU | 0.748 | 0.5 | 0.731 | 0.765 | 0.796 | |
iPseU-TWSVM | 0.728 | 0.462 | 0.795 | 0.661 | 0.775 |
Species | Classifier | Independent testing | ||||
ACC | MCC | SN | SP | AUC | ||
H.sapiens | iRNA-PseU | 0.65 | 0.3 | 0.6 | 0.7 | / |
PseUI | 0.655 | 0.31 | 0.63 | 0.7 | / | |
iRNA-CNN | 0.69 | 0.4 | 0.777 | 0.68 | / | |
XG-PseU | 0.675 | / | / | 0.608 | / | |
RF-PseU | 0.75 | 0.5 | 0.78 | 0.72 | 0.8 | |
iPseU-TWSVM | 0.763 | 0.529 | 0.825 | 0.7 | 0.786 | |
S.cerevisiae | iRNA-PseU | 0.6 | 0.2 | 0.63 | 0.57 | / |
PseUI | 0.685 | 0.37 | 0.65 | 0.72 | / | |
iRNA-CNN | 0.735 | 0.47 | 0.688 | 0.778 | / | |
XG-PseU | 0.71 | / | / | / | / | |
RF-PseU | 0.77 | 0.54 | 0.75 | 0.79 | 0.838 | |
iPseU-TWSVM | 0.825 | 0.65 | 0.85 | 0.8 | 0.905 |
Scores type | iPseU-TWSVM | RF-PseU | XG-PseU | iRNA-CNN | PseUI | iRNA-PseU |
Cross-validation | 0.7 | 0.713 | 0.687 | 0.689 | 0.662 | 0.647 |
Independent testing | 0.794 | 0.76 | 0.693 | 0.713 | 0.7 | 0.625 |
Feature Subset | TWSVM | ||||
ACC | MCC | SN | SP | AUC | |
Kmer | 0.59 | 0.181 | 0.533 | 0.646 | 0.618 |
PC-PseDNC-General | 0.534 | 0.07 | 0.434 | 0.635 | 0.543 |
ANF | 0.526 | 0.053 | 0.568 | 0.485 | 0.518 |
EIIP | 0.572 | 0.144 | 0.525 | 0.618 | 0.6 |
ENAC | 0.587 | 0.178 | 0.472 | 0.7 | 0.59 |
NCP + NBP | 0.584 | 0.172 | 0.53 | 0.639 | 0.582 |
Kmer + NCP + NBP | 0.59 | 0.182 | 0.532 | 0.648 | 0.587 |
Kmer + ENAC | 0.603 | 0.208 | 0.582 | 0.624 | 0.62 |
Kmer + EIIP | 0.606 | 0.212 | 0.585 | 0.626 | 0.625 |
Kmer + PC-PseDNC-Generel + ANF + EIIP + ENAC + NCP + NBP |
0.65 | 0.301 | 0.697 | 0.602 | 0.682 |
Feature Subset | TWSVM | ||||
ACC | MCC | SN | SP | AUC | |
Kmer | 0.627 | 0.259 | 0.625 | 0.63 | 0.67 |
PC-PseDNC-General | 0.574 | 0.153 | 0.666 | 0.483 | 0.589 |
ANF | 0.584 | 0.175 | 0.716 | 0.452 | 0.584 |
EIIP | 0.614 | 0.238 | 0.473 | 0.755 | 0.632 |
ENAC | 0.634 | 0.326 | 0.435 | 0.836 | 0.693 |
NCP + NBP | 0.669 | 0.34 | 0.659 | 0.678 | 0.711 |
Kmer + NCP + NBP | 0.664 | 0.33 | 0.653 | 0.675 | 0.683 |
Kmer + ENAC | 0.653 | 0.308 | 0.631 | 0.675 | 0.683 |
Kmer + EIIP | 0.631 | 0.263 | 0.628 | 0.634 | 0.662 |
Kmer + PC-PseDNC-Generel+ANF + EIIP + ENAC + NCP + NBP | 0.722 | 0.45 | 0.656 | 0.786 | 0.758 |
Feature Subset | TWSVM | ||||
ACC | MCC | SN | SP | AUC | |
Kmer | 0.584 | 0.17 | 0.676 | 0.49 | 0.614 |
PC-PseDNC-General | 0.541 | 0.084 | 0.517 | 0.566 | 0.539 |
ANF | 0.553 | 0.113 | 0.71 | 0.396 | 0.527 |
EIIP | 0.625 | 0.276 | 0.826 | 0.424 | 0.632 |
ENAC | 0.664 | 0.329 | 0.667 | 0.661 | 0.7 |
NCP + NBP | 0.662 | 0.326 | 0.636 | 0.688 | 0.703 |
Kmer + NCP + NBP | 0.677 | 0.36 | 0.623 | 0.731 | 0.73 |
Kmer + ENAC | 0.662 | 0.326 | 0.657 | 0.667 | 0.704 |
Kmer + EIIP | 0.636 | 0.274 | 0.697 | 0.574 | 0.667 |
Kmer + PC-PseDNC-Generel + ANF + EIIP + ENAC + NCP + NBP | 0.728 | 0.462 | 0.795 | 0.661 | 0.775 |
Feature | The original dimension | The dimension after feature selection |
NCP | 63 | 14 |
EIIP | 21 | 21 |
NBP | 84 | 15 |
ENAC | 105 | 41 |
ANF | 21 | 8 |
Kmer | 64 | 1 |
PC-PseDNC-general | 22 | 0 |
Feature | The Original Dimension | The dimension after feature selection |
NCP | 93 | 22 |
EIIP | 31 | 31 |
NBP | 124 | 25 |
ENAC | 155 | 53 |
ANF | 31 | 19 |
Kmer | 256 | 0 |
PC-PseDNC-general | 18 | 0 |
Feature | The original dimension | The dimension after feature selection |
NCP | 63 | 11 |
EIIP | 21 | 21 |
NBP | 84 | 17 |
ENAC | 105 | 43 |
ANF | 21 | 7 |
Kmer | 64 | 1 |
PC-PseDNC-general | 22 | 0 |
Species | Classifier | Cross-validation | ||||
ACC | MCC | SN | SP | AUC | ||
H.sapiens | iRNA-PseU | 0.604 | 0.21 | 0.61 | 0.598 | 0.64 |
PseUI | 0.642 | 0.28 | 0.649 | 0.636 | 0.68 | |
iRNA-CNN | 0.667 | 0.34 | 0.65 | 0.688 | / | |
XG-PseU | 0.661 | 0.32 | 0.635 | 0.687 | 0.7 | |
RF-PseU | 0.643 | 0.29 | 0.661 | 0.626 | 0.7 | |
iPseU-TWSVM | 0.65 | 0.301 | 0.697 | 0.602 | 0.682 | |
S.cerevisiae | iRNA-PseU | 0.645 | 0.29 | 0.647 | 0.643 | 0.81 |
PseUI | 0.641 | 0.3 | 0.647 | 0.675 | 0.69 | |
iRNA-CNN | 0.682 | 0.37 | 0.664 | 0.705 | / | |
XG-PseU | 0.682 | 0.37 | 0.668 | 0.695 | 0.77 | |
RF-PseU | 0.748 | 0.49 | 0.772 | 0.724 | 0.81 | |
iPseU-TWSVM | 0.722 | 0.45 | 0.656 | 0.786 | 0.758 | |
M.musculus | iRNA-PseU | 0.691 | 0.38 | 0.733 | 0.648 | 0.75 |
PseUI | 0.704 | 0.41 | 0.799 | 0.703 | 0.71 | |
iRNA-CNN | 0.718 | 0.44 | 0.748 | 0.691 | / | |
XG-PseU | 0.72 | 0.45 | 0.765 | 0.676 | 0.74 | |
RF-PseU | 0.748 | 0.5 | 0.731 | 0.765 | 0.796 | |
iPseU-TWSVM | 0.728 | 0.462 | 0.795 | 0.661 | 0.775 |
Species | Classifier | Independent testing | ||||
ACC | MCC | SN | SP | AUC | ||
H.sapiens | iRNA-PseU | 0.65 | 0.3 | 0.6 | 0.7 | / |
PseUI | 0.655 | 0.31 | 0.63 | 0.7 | / | |
iRNA-CNN | 0.69 | 0.4 | 0.777 | 0.68 | / | |
XG-PseU | 0.675 | / | / | 0.608 | / | |
RF-PseU | 0.75 | 0.5 | 0.78 | 0.72 | 0.8 | |
iPseU-TWSVM | 0.763 | 0.529 | 0.825 | 0.7 | 0.786 | |
S.cerevisiae | iRNA-PseU | 0.6 | 0.2 | 0.63 | 0.57 | / |
PseUI | 0.685 | 0.37 | 0.65 | 0.72 | / | |
iRNA-CNN | 0.735 | 0.47 | 0.688 | 0.778 | / | |
XG-PseU | 0.71 | / | / | / | / | |
RF-PseU | 0.77 | 0.54 | 0.75 | 0.79 | 0.838 | |
iPseU-TWSVM | 0.825 | 0.65 | 0.85 | 0.8 | 0.905 |
Scores type | iPseU-TWSVM | RF-PseU | XG-PseU | iRNA-CNN | PseUI | iRNA-PseU |
Cross-validation | 0.7 | 0.713 | 0.687 | 0.689 | 0.662 | 0.647 |
Independent testing | 0.794 | 0.76 | 0.693 | 0.713 | 0.7 | 0.625 |