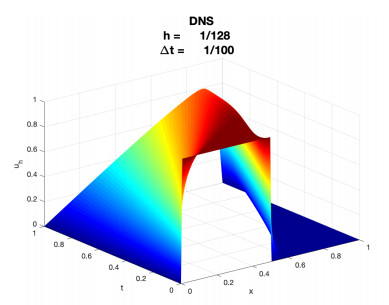
Citation: Luigi C. Berselli, Traian Iliescu, Birgul Koc, Roger Lewandowski. Long-time Reynolds averaging of reduced order models for fluid flows: Preliminary results[J]. Mathematics in Engineering, 2020, 2(1): 1-25. doi: 10.3934/mine.2020001
[1] | Stefania Fresca, Federico Fatone, Andrea Manzoni . Long-time prediction of nonlinear parametrized dynamical systems by deep learning-based reduced order models. Mathematics in Engineering, 2023, 5(6): 1-36. doi: 10.3934/mine.2023096 |
[2] | Michele Dolce, Ricardo Grande . On the convergence rates of discrete solutions to the Wave Kinetic Equation. Mathematics in Engineering, 2024, 6(4): 536-558. doi: 10.3934/mine.2024022 |
[3] | Jason Howell, Katelynn Huneycutt, Justin T. Webster, Spencer Wilder . A thorough look at the (in)stability of piston-theoretic beams. Mathematics in Engineering, 2019, 1(3): 614-647. doi: 10.3934/mine.2019.3.614 |
[4] | Ludovica Cicci, Stefania Fresca, Stefano Pagani, Andrea Manzoni, Alfio Quarteroni . Projection-based reduced order models for parameterized nonlinear time-dependent problems arising in cardiac mechanics. Mathematics in Engineering, 2023, 5(2): 1-38. doi: 10.3934/mine.2023026 |
[5] | Boubacar Fall, Filippo Santambrogio, Diaraf Seck . Shape derivative for obstacles in crowd motion. Mathematics in Engineering, 2022, 4(2): 1-16. doi: 10.3934/mine.2022012 |
[6] | Simon Lemaire, Julien Moatti . Structure preservation in high-order hybrid discretisations of potential-driven advection-diffusion: linear and nonlinear approaches. Mathematics in Engineering, 2024, 6(1): 100-136. doi: 10.3934/mine.2024005 |
[7] | Francesca Tedeschi, Giulio G. Giusteri, Leonid Yelash, Mária Lukáčová-Medvid'ová . A multi-scale method for complex flows of non-Newtonian fluids. Mathematics in Engineering, 2022, 4(6): 1-22. doi: 10.3934/mine.2022050 |
[8] | Alessia Nota, Juan J. L. Velázquez . Homoenergetic solutions of the Boltzmann equation: the case of simple-shear deformations. Mathematics in Engineering, 2023, 5(1): 1-25. doi: 10.3934/mine.2023019 |
[9] | François Alouges, Aline Lefebvre-Lepot, Jessie Levillain . A limiting model for a low Reynolds number swimmer with $ N $ passive elastic arms. Mathematics in Engineering, 2023, 5(5): 1-20. doi: 10.3934/mine.2023087 |
[10] | Yangyang Qiao, Qing Li, Steinar Evje . On the numerical discretization of a tumor progression model driven by competing migration mechanisms. Mathematics in Engineering, 2022, 4(6): 1-24. doi: 10.3934/mine.2022046 |
In this note we combine some results on the long-time averaging of fluid equations with the recent techniques for reduced order model (ROM) development. In this preliminary work we start proving some analytical results that characterize the time-averaged effect of the exchange of energy between various modes, both in the case of the computable decomposition made with proper orthogonal decomposition (POD) type basis functions and with the abstract basis made with eigenfunctions. We will show that the results obtainable with a generic (but computable) basis are less precise than those obtainable with the abstract spectral basis, the difference coming from the lack of orthogonality of the gradients of the POD basis functions.
We then provide a few numerical examples. Concerning the analytical results we will prove partial results for the energy exchange between large and small scales, showing the difference between the use of a spectral type basis and a POD one. In particular, we are interested in results connected to the statistical equilibrium problem, which can be deduced in a computable way by a long-time averaging of the solutions. We want to investigate the possible forward and backward average transfer of energy. The properties of a turbulent flow are computable (and relevant) only in an average sense. In this respect, we want to follow the classical approach dating back to Stokes, Reynolds, and Prandtl of considering long-time averages of the solution as the key quantity to be computed or observed. Therefore, we do not need to consider statistical averaging and link it with time averaging by means of (unproved) ergodic hypotheses.
To introduce the problem that we will consider, we recall that a Newtonian incompressible flow (with constant density) can be described by the Navier-Stokes equations (NSE in the sequel)
{∂tu−νΔu+(u⋅∇)u+∇p=fin Ω×(0,T),∇⋅u=0in Ω×(0,T),u=0on Γ×(0,T),u(⋅,0)=u(0)in Ω, | (1.1) |
with Dirichlet conditions when the motion takes place in a smooth and bounded domain Ω⊂R3 with solid walls Γ:=∂Ω. The unknowns are the velocity field u and the scalar pressure p, while the positive constant ν>0 is the kinematic viscosity. The key parameter to detect the nature of the problem is the non-dimensional Reynolds number, which is defined as
Re=ULν, |
where U and L are a characteristic velocity and length of the problem. In realistic problems, the Reynolds number can be extremely large (in many cases of the order of 108, but up to the order of 1020 in certain geophysical problems). For simplicity in the notation, we use the viscosity as a control parameter and assume that it is very small. Hence, the effect of the regularization (similar to the diffusion in heat transfer) due to the Laplacian is negligible and the behavior of solutions is really turbulent and rather close to the motion of ideal fluids. Due to the well-known difficulties in performing direct numerical simulations (DNS), it is nowadays a well-established technique that of trying to reduce the computational efforts by simulating only the largest scales, which, for limited computational and experimental resources, are the only ones computable and observable. In this framework, the large eddy simulation (LES) methods, which emerged in the last 30 years, are among the most popular, and they found a very relevant role within both theorists' and practitioners' communities. For recent LES reviews, see, for instance, the monographs [2,5,27,39].
The LES methods are, in many cases, very well developed and both theoretically and computationally appealing, especially for problems without boundaries, but many difficulties and open problems arise when facing solid boundaries. In most cases the design of efficient LES methods is guided by deep properties of the solutions, as emerging from deep theorems of mathematical analysis. Furthermore, the ultimate goal of having a golden standard is far from being obtained, and large families of methods (wave-number asymptotics, differential filters, α-models) attracted the interest of different communities, spanning from the pure mathematicians, to the applied geophysicist and mechanical engineers, to the computational practitioners. For these reasons, we believe that it is important to have some well-defined and clearly stated guidelines, that can be adapted to different problems. In this way the methods can be improved with insight not only from experts in modeling, but also from mathematicians, physicists, and computational scientists.
In this respect, we point out that very recently the use of other (more flexible and computationally simpler) ways of finding approximate systems has become very popular. The LES methods itself can be specialized or even glued with other ways of determining much smaller approximate systems, which are computable in a very efficient way. For instance, reduced order modeling is increasingly becoming an accepted paradigm, in which applications to fluids are still being developed [15,25,35,36,38].
The basic ansatz at the basis of the use of these models is the approximation of the velocity by a truncation of the series
u=∞∑k=1ukwk, |
where {wk}k∈N is a basis constructed by using the POD, not necessarily made with eigenfunctions of the Stokes operator, and the coefficients of the L2-projections are evaluated as follows
uk=∫Ωu⋅wkdx∫Ω|wk|2dx. |
The appealing property of this approach is that the choice of the basis is adapted to and determined by the problem itself. Generally the basis is chosen after a preliminary numerical computation, hence it contains at least the basic features of the solution and geometry of the problem to be studied. The other basic fact is that the kinetic energy of the problem is the key quantity under consideration; in fact the L2-projection is generally used to determine the approximate velocity and also the energy content in the basis construction. To determine the number r∈N such that the solution is projected over the space generated by the (orthogonal) functions Vr:=span{w1,…,wr}, generally it is assumed that the projection of the solution over Vr contains a large percentage (say 80%) of the total kinetic energy of the underlying system.
It turns out that a basis associated with the problem at hand can greatly improve the effectiveness of the ROM. Its proper choice can be of great interest in applications to fluid flows [41,42,44]. The present paper combines mathematical results on the long-time behavior of fluid flows (especially in the case of statistical equilibrium) with reduced order modeling. The main goal of this approach is that it provides a mathematical description of both the long-time averages of ROMs and the energy exchange between ROM modes. Furthermore, preliminary numerical results that support the theoretical developments are presented. Specifically, we are extending to the POD setting the results for statistical solutions by Foias et al. [11,12] and those more recently obtained for time-averages in [3,28]. In this respect, the main theoretical results of this paper, stated in Theorems 4.2 and 4.3 below, can be viewed as a spectral version of the results of [3,28]. These results show that low frequency modes yield a Reynolds stress that is dissipative in the mean, its total spatial mean work being larger than the long time average of the dissipation of the fluctuations, which is consistent with observations and results in [3,11]. However, the analysis shows that the triad interaction between high and low frequency modes yields an additional non positive term in the budget between the Reynolds stress of high modes and the corresponding mean dissipation. This term may be non dissipative and may permit an inverse energy cascade, which is not in contradiction with the fact that the total Reynolds stress is dissipative in mean.
The paper is organized as follows: In Section 2, we outline the general framework for ROMs of fluid flows, and we display the exchange of energy between large scales and small scales for two ROM bases: the POD and the Stokes eigenfunctions. In Section 3, we present some preliminaries on long-time averages. In Section 4, we prove the main theoretical results for the average transfer of energy for ROMs constructed with the POD and the Stokes eigenfunctions. In Section 5, we perform a preliminary numerical study in which we investigate the theoretical results in the numerical simulation of the one-dimensional Burgers equation. Finally, in Section 6, we draw conclusions and outline future research directions.
As outlined in the introduction, one key quantity in the pure and applied analysis of the Navier-Stokes equations is the kinetic energy, since it is a meaningful physical quantity and the analysis of its budget is at the basis of abstract existence results for weak solutions (cf. Constantin and Foias [6]) and also of the conventional turbulence theories of Kolmogorov [19]. It is well-known that after testing the NSE (1.1) by u and integrating over the space-time, one (formally) obtains the global energy balance
12‖u(t)‖2+ν∫t0‖∇u(s)‖2ds=12‖u(0)‖2+∫t0∫Ωf⋅udxds. |
At present, it is possible to prove that the above balance holds true with the sign of "less or equal" for the class of weak (or turbulent) solutions for which there are results of existence globally in time, without restrictions on the viscosity and on the size of square summable initial velocity u(0) and external force f. It is of fundamental importance in many problems in pure mathematics to understand under which hypotheses the equality holds true. We are now focusing on the "global energy" which is an averaged quantity, since it is the integral of the square modulus of the velocity over the entire domain. We also point out that at the other extreme one can deduce, without the integration over the domain, the point-wise relation
∂t|u|22+|∇u|2−Δ|u|22+div (u|u|22+pu)=f⋅u. |
In between there is the so called "local energy" which can be obtained by multiplying the NSE by uϕ, where ϕ is a bump function, before integrating in the space-time variables. The goal is to show that
∫T0∫Ω|∇u|2ϕdxdt=∫T0∫Ω[|u|22(∂tϕ+Δϕ)+(|u|22+p)u⋅∇ϕ+f⋅uϕ]dxdt |
holds (at least with the inequality sign) for all smooth scalar functions ϕ∈C∞0((0,T)×Ω) such that ϕ≥0. The validity of such an inequality is one of the requirements to prove partial regularity results, but it is also one of the conditions to be satisfied by weak solutions constructed by numerical or LES methods. In this respect, see Guermond, Oden and Prudhomme [14] and [4].
In this paper we study the global energy in the perspective that it can be reconstructed in a computable way or it can be well approximated by the POD basis functions {wk}.
The fact that the functions {wk} can be constructed to be orthonormal with respect to the scalar product in (L2(Ω)3,‖⋅‖) allows us to evaluate the kinetic energy easily by the following numerical series
E(u)=12∞∑k=1‖uk‖2. |
Since we are going to use only a reduced number of ROM modes, it is relevant to consider the energy contained in functions described by a restricted set of indices. Hence, following the notation in [12], if we define
um′,m″:=m″∑k=m′ukwk, |
then the kinetic energy content of um′,m″ is simply evaluated as follows
E(um′,m″)=12m″∑k=m′‖uk‖2. |
We want to investigate the energy transfer between the various modes, together with averaged long-time behavior associated with this splitting.
We are going to adapt well-known studies on the decomposition in small and large eddies. This would be the case if the functions wk are chosen to be the eigenfunctions of the Stokes operator, hence associated with large and small frequencies. In our setting the basis is determined by the solution of a simplified problem, which can be treated computationally, and the basis functions are orthonormal in L2(Ω), but we cannot expect that their gradients are also orthogonal.
For the NSE, the standard ROM is constructed as follows:
(ⅰ) choose modes {w1,…,wd}, which represent the recurrent spatial structures of the given flow;
(ⅱ) choose the dominant modes {w1,…,wm}, with m≤d, as basis functions for the ROM;
(ⅲ) use a Galerkin truncation um=∑mj=1ajwj;
(ⅳ) replace u with um in the NSE;
(ⅴ) use a Galerkin projection of NSE (um) onto the ROM space Vm:=span{w1,…,wm} to obtain a low-dimensional dynamical system, which represents the ROM:
˙a=Aa+a⊤Ba, |
where a is the vector of unknown ROM coefficients and A,B are ROM operators;
(ⅵ) in an offline stage, compute the ROM operators;
(ⅶ) in an online stage, repeatedly use the ROM (for various parameter settings and/or longer time intervals).
Hence, there is a very natural splitting of the velocity field u into two components, the part coherent with the basis expansion associated with the more energetic modes (y), and the remainder (z). This can be formalized as follows:
u=y+z, |
where
y=m∑k=1ukwk=Pmuandz=+∞∑k=m+1ukwk=(I−Pm)u=:Qmu. | (2.1) |
In (2.1), m∈N can be selected computationally (based, e.g., on the relative kinetic energy content in the first m POD-modes, but other choices relative to the enstrophy are possible) in order to have a significant amount of the energy content of the flow in y. Furthermore, Pm is the projection operator over the subspace Vm spanned by the first m-functions {wk}k=1,…,m.
We observe that we are considering the functions {wk}k as divergence-free, at least in a weak and/or approximate sense. Even if generally they are not "exactly divergence-free", numerically we can consider that they have vanishing divergence, by assuming that the solution of the problems used to construct the basis is accurate enough to have negligible divergence. Hence, in the computations that will follow, we will drop the pressure terms by a standard Leray projection. It will be nevertheless interesting to extend our study to bases that are not divergence-free, e.g., those derived by the combination of ROM with artificial compressibility methods [8,9,13].
In addition, we consider the external force as stationary, that is f=f(x)∈L2(Ω)3 and we look for conditions holding at statistical equilibrium. Our purpose is to determine –if possible– the long-time behavior of y and to analyze the energy budget between low and high modes in the orthogonal decomposition determined by the functions wk.
As usual in many problems fluid mechanics, we use the Hilbert space functional setting with
V={φ∈D(Ω)3,∇⋅φ=0},H={u∈L2(Ω)3, ∇⋅u=0, u⋅n=0 on Γ},V={u∈H10(Ω)3,∇⋅u=0}, |
where n denotes the outward normal unit vector. Moreover, V′ is the topological dual space of V. We will also denote by <⋅,⋅> the duality pairing between V and V′. We recall that V is dense in H and V for their respective topologies [10,29].
Once we project L2(Ω)3 over the subspace H of divergence-free and tangential vector fields by means of the Leray projection operator P, we have the following abstract (functional) equation in H
dudt+νAu+B(u,u)=Pf, |
where A:=−PΔ, while B(u,u):=P((u⋅∇)u). As usual in this analysis (see for instance [12]), we can start by assuming that the input force can be decomposed within a finite sum of basis functions (or that it belongs to Vm, which will be clarified in the next section, in particular by Theorem 2.1), hence
Pmf=f. |
We split the Navier-Stokes equations into a coupled system for y∈PmH and z∈(PmH)⊥=QmH as follows
dydt−νPm(Δu)+PmB(y+z,y+z)=Pmf,dzdt−νQm(Δu)+QmB(y+z,y+z)=0, | (2.2) |
where we used that both Pm and Qm commute with the time derivative.
Once we evaluate the kinetic energy, since Pmy=y and Qmz=z we get (by integrating by parts and by using the fact that functions vanish at the boundary) that
−ν∫ΩPm(Δu)⋅ydx=−ν∫Ω(Δu)⋅ydx=−ν∫Ω(Δy+Δz)⋅ydx=ν‖∇y‖2+ν∫Ω∇y:∇zdx,−ν∫ΩQm(Δu)⋅zdx=−ν∫Ω(Δu)⋅zdx=−ν∫Ω(Δy+Δz)⋅zdx=ν‖∇z‖2+ν∫Ω∇y:∇zdx. |
In this way we can obtain the following equality and inequality
12ddt‖y‖2+ν‖∇y‖2+ν(∇y,∇z)+(B(y+z,y+z),y)=(f,y),12ddt‖z‖2+ν‖∇z‖2+ν(∇y,∇z)+(B(y+z,y+z),z)≤0, | (2.3) |
These are the two basic balance equations that we will use to infer the behavior and transfer of the kinetic energy between y and z. Notice that the balance relation for y, involving just a finite combination of rather smooth functions is an equality, while the second one is an inequality. In fact, the second one can be derived by a limiting argument and in the limit the lower semi-continuity of the norm will produce the inequality.
Since the tri-linear term (B(u,u),w) is skew-symmetric with respect to the last two variables, we obtain from (2.3)
12ddtE(y)+ν‖∇y‖2+ν(∇y,∇z)=(B(y,y),z)−(B(z,z),y)+(f,y),12ddtE(z)+ν‖∇z‖2+ν(∇y,∇z)≤−(B(y,y),z)+(B(z,z),y). | (2.4) |
This is a formal setting, which is obviously true for strong solutions of the NSE, where the inequality in (2.4) is an equality. When considering weak solutions, the integral (B(z,z),z) might be not defined in L1(0,T) for regularity issues. However, one can still rigorously derive (2.4) by a double frequency truncation or a regularization of the operator B by considering (B(z⋆ρε,z),z) for a given standard mollifier ρε and passing to the limit when ε→0. Details are standard and out of the scope of the present paper.
We observe that −(B(y,y),z) is the energy flux induced in the more energetic terms by the inertial forces associated to less energetic modes, while −(B(z,z),y) is the energy flux induced in the less energetic terms by the inertial forces associated to more energetic modes. In a schematic way we can decompose the rate of transfer of kinetic energy em(u) into two terms as follows
em(u):=e↑(u)−e↓(u) withe↑(u):=−(B(y,y),z),e↓(u):=(B(z,z),y). | (2.5) |
We also use the following notation:
Em(u):=−ν(∇y,∇z). | (2.6) |
Hence, we can rewrite (2.4) as follows
12ddtE(y)+ν‖∇y‖2=Em(u)−em(u)+(f,y),12ddtE(z)+ν‖∇z‖2≤Em(u)+em(u). | (2.7) |
Remark 2.1. We recall that apart from extremely simple geometries and provided one is willing to use in a systematic way special functions as the Bessel ones or the spherical harmonics (which are nevertheless time consuming in their evaluation), the explicit calculations in numerical tests will not be so easy to be obtained in a precise and efficient way. Hence, the solution of (2.2) and the long-time integration of its solution pose hard numerical problems.
We point out for the reader that we have a first fundamental difference with respect to the classical splitting based on the use of a spectral basis (which will be recalled in Section 2.1), where the latter integral vanishes exactly. For this reason, in the next section we will show the derivation of the corresponding system of equations, which holds when the eigenfunctions are used.
In this section, we compare the results of the previous section with the well-established ones that can be proved if the spectral decomposition (i.e., that made with eigenfunctions of the Stokes operator {Wk}) is used instead of utilizing a generic POD basis. We recall that, by classical results about compact operators in Hilbert spaces there exists a sequence of smooth functions {Wk} (and their regularity is depending on the smoothness of the bounded domain Ω) and an increasing sequence of positive numbers {λk} such that
AWk=λkWkand∫ΩWk⋅Wjdx=δkj. |
Since each function Wk solves the following Stokes system AWk=λkWk, it follows by an integration by parts that
∫Ω∇Wk:∇Wjdx=0for k≠j, |
hence also the V-orthogonality of the family {Wk}k∈N.
We consider now the usual decomposition by eigenfunctions associated with low and high frequencies
u=y+z:=m∑k=1ckWk+∞∑k=m+1 ckWk=Pmu+Qmu, |
where Pm is the projection over the subspace generated by {Wk}k=1,…,m. Our main result is based on a standard result about the projector Pm, that can be found in [30,App. A.4,Thm. 4.11]:
Theorem 2.1. The projector Pm can be defined as a continuous endomorphism over V, H and V′, and one has
‖Pm‖L(V,V)≤1,‖Pm‖L(H,H)≤1,‖Pm‖L(V′,V′)≤1. |
The result is mainly based on the regularity of solutions of elliptic equations, and thanks to this fact, it is possible to decompose the equations for the velocity, which yields,
−ν∫ΩPm(Δu)⋅ydx=−ν∫ΩΔy⋅ydx=ν‖∇y‖2,−ν∫ΩQm(Δu)⋅zdx=−ν∫ΩΔz⋅zdx=ν‖∇z‖2, |
since PmΔu=ΔPmu=Δy and also Qm(Δu)=ΔQmu=Δz.
Thus, we directly obtain the system
12ddt‖y‖2+ν‖∇y‖2+(B(y+z,y+z),y)=(f,y),12ddt‖z‖2+ν‖∇z‖2+(B(y+z,y+z),z)≤0, | (2.8) |
which can be rewritten also as
12ddtE(y)+ν‖∇y‖2=−em(u)+(f,y),12ddtE(z)+ν‖∇z‖2≤em(u). | (2.9) |
We note that the equations in (2.9) do not contain the term Em(u), whereas the equations in (2.7) contain Em(u). This will have important consequences in the analysis in Section 4.
Since we consider long-time averages for the NSE, we must consider solutions which are global-in-time (defined for all positive times). Due to the well-known open problems related to the NSE, this forces us to restrict ourselves to Leray-Hopf weak solutions [6,29]. By using a natural setting, we take the initial datum u(0) in H. The classical Leray-Hopf result of existence (but not uniqueness) of a global weak solution u to the NSE holds when f∈V′, and the velocity u satisfies
u∈L2(R+;V)∩L∞(R+;H). |
Notice that we consider in this paper the case where f is time-independent, for simplicity. However, the following results can be extended to the case where f=f(t) is time dependent, for f belonging to a suitable class (see [3]).
In order to properly set what we mean by long-time-averaging, let ψ: R+×Ω→RN be any tensor field related to a given turbulent flow (N being its order). The time-average of ψ over a time interval [0,t] is defined by
Mt(ψ)(x):=1t∫t0ψ(s,x)dsfor t>0. | (3.1) |
According to the standard turbulence modeling process, we then apply the averaging operator Mt to NSE (1.1) and also to (2.2), to study the limits when t→+∞. We recall that the long-time averages represent one of the few observable and computable quantities associated to a highly variable turbulent flow. We will adopt the following standard notation for the long-time average of any field ψ
¯ψ(x):=limt→+∞Mt(ψ)(x), |
whenever the limit exists. (Without too much restrictions, we can suppose that the limits we write do exist, at least after extracting sub-sequences leaving the mathematical difficulties, which can be treated with generalized Banach limits, for a more general and abstract framework.) Within this theory we can decompose the velocity as follows
u=¯u+u′, |
where u′:=u−¯u represents the so-called turbulent fluctuations. We recall that time-averaging has been introduced by O. Reynolds [37], at least for large values of t, and the ideas have been widely developed by L. Prandtl [32] in the case of turbulent channel flows. The same ideas have been also later considered in the case of fully developed homogeneous and isotropic turbulence, such as grid-generated turbulence. In this case the velocity field is postulated as oscillating around a mean smoother steady state, see for instance G.-K. Batchelor [1]. For further details on the role of time averaging in turbulence, after the work of Stokes and Reynolds, we can recall a few recent papers and books [2,3,5,11,18,26,28], where aspects of computation and modeling are studied.
We now observe that, by taking the time-averages of the NSE we have the following estimates, see [28,Prop. 2.1]
‖u(t)‖2≤‖u(0)‖2e−νCPt+‖f‖2ν2CP(1−e−νCPt),∀t>01t∫t0‖∇u(s)‖2ds≤‖f‖2ν2+‖u(0)‖2νt,∀t>0, | (3.2) |
where CP is the best constant in the Poincaré inequality
CP‖u‖2≤‖∇u‖2∀u∈H10(Ω). |
The above inequalities show that both ‖u(t)‖2 and 1t∫t0‖∇u(s)‖2ds are uniformly bounded for all t≥1 (any other positive time will be enough), hence we have the following result:
Theorem 3.1 (cf. [3,28]). Let u(0)∈H, f∈V′, and let u be a global-in-time weak solution to the NSE (1.1). Then, there exist
1. a sequence {tn}n∈N such that limn→∞tn=+∞;
2. a vector field ¯u∈V;
3. a vector field B∈L3/2(Ω)3;
4. a second order tensor field σ(R)∈L3(Ω)9;
such that it holds:
i) When n→∞,
Mtn(u)⇀¯uweakly in V,Mtn((u⋅∇)u)⇀Bweakly in L3/2(Ω)3,Mtn(u′⊗u′)⇀σ(R)weakly in L3(Ω)9; |
ii) The Reynolds averaged equations:
{(¯u⋅∇)¯u−νΔ¯u+∇¯p+∇⋅σ(R)=¯fin Ω,∇⋅¯u=0in Ω,¯u=0on Γ, | (3.3) |
hold true in the weak sense;
iii) The equality B−(¯u⋅∇)¯u=∇⋅σ(R) is valid in D′(Ω);
iv) The following energy balance (equality) holds true
ν‖∇¯u‖2+(∇⋅σ(R),¯u)=<¯f,¯u>; |
v) The tensor σ(R) is dissipative on the average or, more precisely, the following inequality
ϵ:=ν¯‖∇u′‖2≤∫Ω(∇⋅σ(R))⋅¯udx, | (3.4) |
holds true.
It is important to observe that the long-time limit is characterized by the solution of the system (3.3), which is similar to the Navier-Stokes equations, but which contains an extra term, coming from the effect of fluctuations, which has the mean effect of increasing the dissipation.
We observe that this is related to the long-time behavior of solutions close to statistical equilibrium. The study of the long-time behavior dates back to pioneering works of Foias and Prodi on deterministic statistical solutions, see, for instance [12]. Their interest is mainly devoted to finding measure in the space of initial data to be connected with the long-time limits. Here, we follow a slightly different path, as in [3,28], in order to characterize in a less technical way the long-time behavior, without resorting to any ergodic-type result and also with the perspective that long time averages are computable or at least can be approximated in a clear way.
Our goal in this section is to characterize in some sense the energy transfer between the two functions y and z of the expansion and to determine –if possible– the sign of em(u), at least in an average sense. We will consider both the POD case and the spectral one.
The point concerning the exchange of energy between low and high modes is in the same spirit as the results recalled in Foias, Manley, Rosa, and Temam [12,Chap. 5] and follows from results obtained in a more heuristic way by Kolmogorov [19].
We first observe that the L2-orthogonality of the POD decomposition implies that
‖u‖2=‖y+z‖2=‖y‖2+‖z‖2. |
Hence, from the uniform L2-bound on u it follows that both y and z are uniformly bounded in time. From this observation we can deduce the following result, reminding that em and Em are defined by equations (2.5) and (2.5), and Mt is defined by equation (3.1).
Theorem 4.1. Let z be the projection onto the less energetic POD modes of a weak solution u to the Navier-Stokes equations. Then, there exists a sequence {tn} such that tn→+∞ and a field ¯z∈H such that
Ztn=Mtn(z)⇀¯zweakly in H, | (4.1) |
and
lim infn→+∞Mtn(em(u)+Em(u))≥0. | (4.2) |
Proof. Let us observe first that by the energy inequality (3.2), we easily deduce that (Mt(z))t>0 is bounded in H, hence the first assertion of the statement and (4.1). We next prove (4.2). To do so, we average with respect to time with the operator Mt the balance equation (2.7) for E(z), which yields
12t‖z(t)‖2−12t‖z(0)‖2+νMt(‖∇z‖2)≤Mt(em(u)+Em(u)). | (4.3) |
By using the energy inequality (3.2) once again, we see that the first two terms vanish as t→+∞ and also that Mt(‖∇z‖2) is bounded. Therefore, (4.3) yields
0≤νlim infn→+∞Mtn(‖∇z‖2)≤lim infn→+∞Mtn(em(u)+Em(u)), |
hence (4.2). We observe that in this case we do not have any direct estimation on the behavior of the H1-norm.
In the case we can assume that the limit exists, we also have the following result.
Corollary 4.1. Let us assume the limit of Mtn(em(u)) for n→+∞ exists, and that
lim infT→+∞νT∫T0(∇z(s),∇y(s))ds=lim inft→∞Em(u)≥0. |
Then, it follows
¯em(u)=limn→+∞1tn∫tn0em(u(s))ds≥0. |
This result can be interpreted as that, beyond the range of injection of energy, the average net transfer of energy occurs only into the small scales. This occurs if the term of interaction between gradients of large and small scales is negligible, in the limit of long time. This latter assumption is not proved rigorously, but we will see it is satisfied in the numerical tests, with a good degree of approximation (see Section 5). However, when one uses the eigenfunctions of the Stokes operator as POD basis, this is automatically satisfied since this basis is also orthogonal for the H1-scalar product, so that in this case Em(u)=0.
The results of the previous section can be made much more precise in the case of decomposition made by a spectral basis of eigenfunctions of the Stokes operator. We present the results, which are in some sense new and not fully completely included in [12], in the sense of time-averaging. This procedure is applied to u, which is a weak solution of the Navier-Stokes equations, satisfying the uniform estimates (3.2). In this way, the orthogonality (in both H and V) of the basis implies that
‖u‖2=‖y‖2+‖z‖2and‖∇u‖2=‖∇y‖2+‖∇z‖2. |
The results in this case are more precise than those from Theorem 4.1, since we have at disposal a larger set of a priori estimates and also the set of equations (2.8) has a better structure than (2.3).
We now prove the following results in the case of a decomposition of the velocity into small and large frequencies. The first one aims at taking the time average and then let t go to infinity in the equations (2.3) satisfied by y and z. The second one aims at comparing the amount of turbulent dissipation of small and large frequencies with respect to the total work of the corresponding Reynolds stresses σ(R)y and σ(R)z.
Theorem 4.2. Let u(0)∈H, f∈PmH, and let u be a global-in-time weak solution to the NSE (1.1). Then, there exist
1. a sequence {tn}n∈N such that limn→∞tn=+∞;
2. vector fields ¯y,¯z∈V;
3. vector fields B1,B2∈V′;
such that it holds:
i) When n→∞,
Mtn(y)⇀¯yweakly in V,Mtn((y⋅∇)y)⇀B1weakly in V′,Mtn(z)⇀¯zweakly in V,Mtn((z⋅∇)z)⇀B2weakly in V′, |
ii) The Reynolds averaged equations:
{−νΔ¯y+∇¯py+B1=Pmfin Ω,∇⋅¯y=0in Ω,¯y=0on Γ, | (4.4) |
and
{−νΔ¯z+∇¯pz+B2=0in Ω,∇⋅¯z=0in Ω,¯z=0on Γ, | (4.5) |
holds true in V′.
Arguing as in [3,28], using the relations (¯z⋅∇)¯z=∇⋅(¯z⊗¯z) and (¯y⋅∇)¯y=∇⋅(¯y⊗¯y), we get the existence of "small frequencies" and "large frequencies" Reynolds stresses σ(R)y and σ(R)z in V′, such that
B1=∇⋅σ(R)y+(¯y⋅∇)¯yandB2=∇⋅σ(R)z+(¯z⋅∇)¯z, |
or equivalently, if we write the Reynolds decomposition as
y=¯y+y′andz=¯z+z′, |
then
σ(R)y=¯y′⊗y′andσ(R)z=¯z′⊗z′, |
where the bar operator denotes the limit of the Mtn's in V′ as n→∞ (eventually after having extracted another sub-sequence).
According to the budget (3.4), we aim to compare the turbulent dissipation of small and large scales, denoted as ε↓ and ε↑ respectively, to the total work of the Reynolds stresses, namely (∇⋅σ(R)y,¯y) and (∇⋅σ(R)z,¯z), where
ϵ↓:=ν¯‖∇y′‖2andϵ↑:=ν¯‖∇z′‖2. |
When we compare to (3.4), we observe that triad nonlinear effect between small and large frequencies will be felt, that means the nonlinear interactions due to the convection will be provided by the term
Φz(y):=(Qm[(y+z)⋅∇(y+z)],z)=−(Pm[(y+z)⋅∇(y+z)],y)=−Φy(z). | (4.6) |
Notice that due to the regularity of y, it is easily checked that the following energy balance holds true (this property will be shown with more details in the proof of Theorem 4.2 below)
ν‖∇¯y‖2+(∇⋅σ(R)y,¯y)=<f,¯y>. |
Finally, to prove Theorem 4.3 we will use the following orthogonality relation (see e.g., [3,Lemma 4.4]), formally written as follows
¯‖∇ψ‖2=‖∇¯ψ‖2+¯‖∇ψ′‖2. | (4.7) |
which is valid for any field ψ:R+→V, such that ¯ψ is well-defined and the fluctuations are defined as ψ′=ψ−¯ψ.
Theorem 4.3. The families (Mt(Φz(y)))t>0 and (Mt(Φy(z)))t>0 converge (along certain subsequences) as t→∞. Let ¯Φz(y) and ¯Φy(z) denote the corresponding limits. One has
¯Φz(y)=−¯Φy(z)≤0, | (4.8) |
and the following dissipation balances hold true
ϵ↓+¯Φy(z)=(∇⋅σ(R)y,¯y), | (4.9) |
ϵ↑+¯Φz(y)≤(∇⋅σ(R)z,¯z). | (4.10) |
Remark 4.1. Notice that by equations (4.8) and (4.9) we see that σ(R)y is dissipative in mean, and follows the same law (3.4) as the complete Reynolds stress, namely
ϵ↓≤(∇⋅σ(R)y,¯y). |
However, nothing similar can be concluded from (4.9) about σ(R)z, that might be at this stage non dissipative in mean, which permits an inverse energy cascade to occur.
The results of Theorems 4.2 and 4.3 are original, even if similar results have been already obtained in [11] and reported also in [12]. In that case, the results are based on the notion of deterministic statistical solutions and on a sort of ergodic hypothesis. Even if statements could look very similar to ours, the main difference is that we do not average over the set H of initial data, and we do not introduce probability measures on H, as suggested by the work by Prodi [33,34]. Our approach is based on a more elementary functional setting and also amenable to include treatment of sets of external forces, as those in several numerical or practical experiments. The main point is an extension of the results in [3].
Proof of Theorem 4.2. We know, from the results in [3,28] that Ut=Mt(u) is such that
Ut⇀¯uweakly in V,Mt((u⋅∇)u)⇀B in L3/2(Ω)⊂V′, |
hence, if we define F:=B−(¯u⋅∇)¯u, we get
ν(∇¯u,∇ϕϕ)+((¯u⋅∇)¯u,ϕϕ)+<F,ϕϕ>=<f,ϕϕ>, |
and using ¯u∈V as test function we obtain
ν‖∇¯u‖2+<F,¯u>=<f,¯u>. |
We assume now that Pmf=f, and we consider the equations satisfied by y=Pmu and z=Qmu. In particular, the equation for y reads, as an abstract equation in Vm=PmV, as follows:
dydt+νAy+Pm[(y+z)⋅∇(y+z)]=Pmf. |
The uniform estimates on u from Theorem 3.1 combined with Theorem 2.1, about the properties of the projection operator Pm as a continuous operator over V′, yield
Mt(y)=Yt⇀¯yweakly in V,PmMt((u⋅∇)u)=PmMt[(y+z)⋅∇(y+z)]⇀PmB=B1weakly in V′, |
in such a way that ¯y satisfies, for the spectral basis Wk introduced in Section 2.1,
ν(∇¯y,∇Wk)+<(¯y⋅∇)¯y,Wk>+<Fy,Wk>=<f,Wk>for all 1≤k≤m, |
where Fy:=B1−(¯y⋅∇)¯y, which leads to (4.4) by De Rham Theorem, if written in a strong formulation. Arguing as in [3] (which was already mentioned above), it is easily checked that there exists σ(R)y such that Fy=∇⋅σ(R)y. Hence, being ¯y∈Vm⊂V a legitimate test function, we get
ν‖∇¯y‖2+<Fy,¯y>=ν‖∇¯y‖2+(∇⋅σ(R)y,¯y)=<f,¯y>. | (4.11) |
The other term z of the decomposition satisfies
ddtz+νAz+Qm[(y+z)⋅∇(y+z)]=0. |
The uniform estimates on u and the boundedness of the linear operator Pm imply the following convergence (up to a sub-sequence), as already shown in Theorem 4.1
Mt(z)=Zt⇀¯zweakly in V,QmMt((u⋅∇)u)=QmMt[(y+z)⋅∇(y+z)]⇀QmB=B2weakly in V′. |
By using that B=PmB+QmB, we get
ν(∇¯z,∇Wj)+<(¯z⋅∇)¯z,Wj>+<Fz,Wj>=0for all j≥m+1, | (4.12) |
for Fz=B2−(¯z⋅∇)¯z=∇⋅σ(R)z. Hence, (4.5) follows again by De Rham Theorem. Notice that, ¯z∈V⊥m⊂V and in particular ¯z∈span{Wj}j≥m+1, so by linearity it can be used as test function in (4.12). Next, since ∇⋅¯z=0, it follows that <(¯z⋅∇)¯z,¯z>=0 and we have the following energy equality:
ν‖∇¯z‖2+(∇⋅σ(R)z,¯z)=0, | (4.13) |
which concludes the proof.
Proof of Theorem 4.3. We now write the energy inequality for z, obtaining
12ddt‖z‖2+ν‖∇z‖2+(Qm[(y+z)⋅∇(y+z)],z)≤0, |
and hence, by using the orthogonality of the basis, we have that Qmz=z and
12ddt‖z‖2+ν‖∇z‖2+([(y+z)⋅∇(y+z)],z)=12ddt‖z‖2+‖∇z‖2+Φz(y)≤0, |
recalling the definition of Φz(y) in (4.6).
Averaging the above equation over a fixed time interval (0,t), we get
12t‖z(t)‖2−12t‖z(0)‖2+νMt(‖∇z‖2)+Mt(Φz(y))≤0. |
The L2-uniform bounds on z imply that 12t‖z(t)‖2→0, hence, possibly after having extracted another sub-sequence to ensure the convergence of the term Mt(‖∇z‖2) (that is known to be bounded by the energy inequality (3.2)) we get
lim supn→∞Mtn(Φz(y))≤−ν¯‖∇z‖2≤0. | (4.14) |
We now combine the orthogonality relation (4.7) with the energy balance (4.13), so that (4.14) yields
ϵ↑+lim supn→∞Mtn(Φz(y))≤(∇⋅σ(R)z,¯z), |
which is almost inequality (4.10), up to the convergence of (Mt(Φz(y)))t>0 that remains to be proved. To prove this, we deal with the budget for y, recall the definition of Φz(y) and Φy(z) from (4.6).
Then, averaging the energy equality (in this case we have equality since y solves a finite dimensional system of ordinary differential equations) which is satisfied for y, we get
12t‖y(t)‖2−12t‖y(0)‖2+νMt(‖∇y‖2)+Mt(Φy(z))=<f,Mt(y)>. | (4.15) |
By the same argument, eventually after having extracted a further sub-sequence, (Mt(‖∇y‖2))t>0 is convergent as tn→∞, as well as (<f,Mt(y)>)t>0. Therefore, {Mtn(Φy(z))} is also convergent by (4.15). Let ¯Φy(z) denote its limit. In particular, by (4.6), {Mtn(Φz(y)))} is also convergent, with limit ¯Φz(y)=−¯Φy(z). We are done with (4.10).
It remains to check (4.9). Taking the limit as n→∞ in (4.15) gives the equality
ν¯‖∇y‖2+¯Φz(y)=<f,¯y>, |
which, combined with the energy balance (4.11) and the orthogonality relation (4.7), yields (4.9), ending the proof.
In Theorem 4.1, we showed that
lim infT→+∞1T∫T0em(u(s))+Em(u(s))ds≥0. | (5.1) |
In this section, we investigate numerically whether the inequality (5.1) holds. To this end, we consider the one-dimensional Burgers equation with homogeneous Dirichlet boundary conditions as a simplified, yet relevant test case [16,17,20,21,22,23,24,31,40,43]:
{ut−νuxx+uux=f(x,t)∈Ω×[0,1],u=0(x,t)∈∂Ω×[0,1]. | (5.2) |
To calculate the long-time average of em(u) in (5.1), we use the composite trapezoidal rule:
1T∫T0em(u(s))ds≈12nn∑i=1(em(u(ti))+em(u(ti+1))), | (5.3) |
where ti=(i−1)∗Tn,i=1,…,n+1. We also use the composite trapezoidal rule to calculate the long-time average of Em(u).
Our numerical results are obtained by using the one-dimensional Burgers equation (5.2) with a step function initial condition [16,43]:
u0(x)={1,x∈(0,1/2],0,x∈(1/2,1]. | (5.4) |
We use the following parameters in the finite element discretization of the Burgers equation (5.2): Ω=[0,1], ν=10−2, f=0, mesh size h=1/128, piecewise linear finite element spatial discretization, and backward Euler time discretization.
For this test case, we consider the time interval [0,T]=[0,1] and the time step Δt=10−2. We utilize all the snapshots to build the POD basis, whose dimension is d=37. In the composite trapezoidal rule, we use n=100. In Figure 1, we plot the DNS results (which are used to generate the snapshots). In Table 1, we list the time-averages of em(u) and Em(u) for different m values. We note that the time-average of em(u) is positive for all m values. The time-average of Em(u) is positive for the low m values and negative for the largest m values. Furthermore, the magnitude of the time-average of Em(u) is generally lower than the magnitude of the time-average of em(u). Thus, we conclude that the time average 1T∫T0em(u(s))+Em(u(s))ds in (5.1) is positive for all m values.
m | ∫10em(u(s))ds | ∫10Em(u(s))ds |
3 | 2.6170e-02 | 1.0451e-03 |
6 | 7.3208e-03 | 2.2524e-03 |
9 | 1.4934e-03 | 1.5977e-03 |
15 | 3.6181e-05 | 3.6287e-05 |
20 | 1.2776e-06 | 7.0356e-07 |
25 | 4.6207e-08 | 9.5638e-09 |
30 | 2.0257e-09 | -1.1127e-10 |
35 | 8.2806e-11 | -2.2595e-11 |
In Case 1, we showed that the time average 1T∫T0em(u(s))+Em(u(s))ds in (5.1) is positive. In the remainder of this section, we investigate whether this time average remains positive if we make the following changes in our computational setting: (ⅰ) we increase/decrease the time-interval; and (ⅱ) we use more quadrature points (i.e., subintervals) in the composite trapezoidal rule (5.3).
In this case, we use a longer time interval, i.e., [0,T]=[0,10] (instead of [0,T]=[0,1], as we used in Case 1). We also use different time step (Δt) values to generate the snapshots and a different number of quadrature points to evaluate the time average 1T∫T0em(u(s))+Em(u(s))ds.
In Tables 2–5, we list the time-averages of em(u) and Em(u) for different time steps (Δt) values, different number of equally spaced quadrature points (n), and different m values. We note that the time-averages of em(u) and Em(u) are positive for all Δt, n, and m values. Moreover, the magnitude of the time-average of Em(u) is generally lower than the magnitude of the time-average of em(u). Thus, we conclude that the time average 1T∫T0em(u(s))+Em(u(s))ds in (5.1) is positive for all Δt, n, and m values. Furthermore, we note that decreasing the time step while keeping the same number of snapshots (i.e., n=10000) does not change the time average 1T∫T0em(u(s))+Em(u(s))ds significantly (see Tables 3–5).
m | 110∫100em(u(s))ds | 110∫100Em(u(s))ds |
3 | 3.3652e-03 | 8.6523e-05 |
6 | 1.0001e-03 | 2.1570e-04 |
9 | 2.1132e-04 | 1.8614e-04 |
15 | 5.4224e-06 | 5.5724e-06 |
20 | 4.3119e-07 | 2.5667e-07 |
25 | 5.6884e-08 | 1.0451e-08 |
30 | 1.1327e-09 | 3.2736e-10 |
35 | 2.0469e-11 | 2.6396e-12 |
m | 110∫100em(u(s))ds | 110∫100Em(u(s))ds |
3 | 3.5296e-03 | 3.0515e-06 |
6 | 1.1402e-03 | 8.8746e-06 |
9 | 2.6359e-04 | 1.5090e-05 |
15 | 1.1126e-05 | 1.1156e-05 |
20 | 9.5913e-07 | 1.5183e-06 |
25 | 1.8140e-07 | 1.8704e-07 |
30 | 4.8765e-08 | 1.3491e-08 |
35 | 1.0291e-09 | 9.7629e-10 |
40 | 2.4680e-11 | 2.0416e-11 |
m | 110∫100em(u(s))ds | 110∫100Em(u(s))ds |
3 | 3.5637e-03 | 2.7841e-06 |
6 | 1.1664e-03 | 8.1109e-06 |
9 | 2.7346e-04 | 1.3956e-05 |
15 | 1.2084e-05 | 1.1937e-05 |
20 | 1.1785e-06 | 1.6370e-06 |
25 | 2.2727e-07 | 1.2913e-07 |
30 | 6.2606e-08 | 9.5191e-09 |
35 | 2.1106e-09 | 6.1538e-10 |
40 | 1.0330e-10 | 2.0241e-11 |
m | 110∫100em(u(s))ds | 110∫100Em(u(s))ds |
3 | 3.5668e-03 | 2.7594e-06 |
6 | 1.1688e-03 | 8.0390e-06 |
9 | 2.7436e-04 | 1.3843e-05 |
15 | 1.2172e-05 | 1.2002e-05 |
20 | 1.2030e-06 | 1.6405e-06 |
25 | 2.3339e-07 | 1.2463e-07 |
30 | 6.4120e-08 | 8.8971e-09 |
35 | 2.2654e-09 | 5.6187e-10 |
40 | 1.1211e-10 | 1.8216e-11 |
In this case, we use an even longer time interval, i.e., [0,T]=[0,100], and compare the time-averages for this time interval to those for the time intervals [0,T]=[0,1] (Case 1) and [0,T]=[0,10] (Case 2). For each time interval, we use the same time step values (Δt=10−2) to generate the snapshots and all the subintervals in the composite trapezoidal rule utilized in the evaluation of the time average 1T∫T0em(u(s))+Em(u(s))ds. In Table 6, we list the time-averages of em(u) and Em(u) for all three time intervals and different m values. We note that the time-averages of em(u) and Em(u) are positive for all time intervals and m values. Furthermore, the magnitude of the time-average of Em(u) is generally lower than the magnitude of the time-average of em(u). Thus, we conclude that the time-average 1T∫T0em(u(s))+Em(u(s))ds in (5.1) is positive for all time intervals and m values. Furthermore, we note that the time-averages of em(u) and Em(u) for the time intervals [0,T]=[0,100] and [0,T]=[0,10] are close, whereas those for the time interval [0,T]=[0,1] are slightly different. Thus, we conclude that the time interval [0,T]=[0,10] is adequate for the approximation of the long time-average 1T∫T0em(u(s))+Em(u(s))ds.
m | 1100∫1000em(u(s))ds | 110∫100em(u(s))ds | ∫10em(u(s))ds |
3 | 3.3687e-04 | 3.3683e-04 | 1.7634e-04 |
6 | 1.0001e-04 | 1.0001e-04 | 7.3142e-05 |
9 | 2.1146e-05 | 2.1147e-05 | 1.6701e-05 |
15 | 5.5621e-07 | 5.5742e-07 | 4.7129e-07 |
20 | 7.9605e-08 | 7.9605e-08 | 6.3799e-08 |
25 | 8.3483e-09 | 8.3489e-09 | 5.7426e-09 |
30 | 1.0839e-10 | 1.0840e-10 | 9.2430e-11 |
35 | 2.7710e-12 | 2.7718e-12 | 2.9575e-12 |
m | 1100∫1000Em(u(s))ds | 110∫100Em(u(s))ds | ∫10Em(u(s))ds |
3 | 8.6270e-06 | 7.0320e-06 | 8.1057e-05 |
6 | 2.1568e-05 | 2.1520e-05 | 3.2405e-05 |
9 | 1.8614e-05 | 1.8599e-05 | 2.0255e-05 |
15 | 5.5718e-07 | 5.4232e-07 | 5.8207e-07 |
20 | 5.6044e-08 | 5.5874e-08 | 6.1423e-08 |
25 | 1.0936e-09 | 9.9763e-10 | 5.9386e-10 |
30 | 3.3046e-11 | 3.2478e-11 | 4.0949e-11 |
35 | 6.4170e-13 | 6.3739e-13 | 1.2125e-12 |
In this case, we use a much shorter time interval, i.e., [0,T]=[0,0.1], and compare the time-averages for this time interval to those for the time intervals [0,T]=[0,1],[0,T]=[0,10], and [0,T]=[0,100] (Case 3). We use two different time step values to generate the snapshots, but the same (i.e., n=5000) equally spaced subintervals in the composite trapezoidal rule utilized in the evaluation of the time average 1T∫T0em(u(s))+Em(u(s))ds. In Tables 8–9, we list the time-averages of em(u) and Em(u) for two different time step values and different m values. We emphasize that, this time, the time-average of em(u) is negative for some m values. Furthermore, the magnitude of the time-average of Em(u) is larger than the magnitude of the time-average of em(u). This is in stark contrast with the previous cases.
m | 10.1∫0.10em(u(s))ds | 10.1∫0.10Em(u(s))ds |
3 | -6.8687e-04 | 2.7378e-05 |
5 | -2.6333e-05 | 1.7795e-05 |
7 | -9.1458e-07 | 4.2432e-06 |
9 | -9.8188e-09 | 4.1220e-07 |
13 | 2.3800e-10 | 1.5749e-09 |
15 | 8.5423e-12 | 4.8043e-11 |
m | 10.1∫0.10em(u(s))ds | 10.1∫0.10Em(u(s))ds |
3 | -6.8807e-04 | 2.7338e-05 |
5 | -2.6447e-05 | 1.7812e-05 |
7 | -9.1691e-07 | 4.2755e-06 |
9 | -9.5609e-09 | 4.1694e-07 |
13 | 2.5287e-10 | 1.5941e-09 |
15 | 1.0725e-11 | 4.7030e-11 |
In this preliminary study, we investigated theoretically and numerically the time-average of the exchange of energy among modes of reduced order models (ROMs) of fluid flows. In particular, we were interested in the statistical equilibrium problem, and especially in the long-time averaging of the ROM solutions. The main goal of the paper was to deduce the possible forward and backward average transfer of the energy among ROM basis functions (modes). We considered two types of ROM modes: Eigenfunctions of the Stokes operator and proper orthogonal decomposition (POD) modes. In Theorem 4.1 and Theorem 4.2, we proved analytical results for both types of ROM modes and we highlighted the differences between them, especially those stemming from the lack of orthogonality of the gradients of the POD basis functions.
In Section 5, we performed a preliminary numerical investigation aiming at determining whether the time-average energy exchange between POD modes (i.e., 1T∫T0em(u(s))+Em(u(s))ds) in Theorem 4.1 is positive. To this end, we used the one-dimensional Burgers equation as a mathematical model. We utilized a piecewise linear FE spatial discretization and a backward Euler temporal discretization. To compute the time-averages, we used the composite trapezoidal rule. We tested different time steps, different number of subintervals in the composite trapezoidal rule, and, most importantly, different time intervals, to ensure that the computed quantities are indeed approximations of the time-averages and not numerical artifacts. The main conclusion of our numerical study is that, for long enough time intervals (i.e., time intervals longer than [0,T]=[0,10]), the time-average 1T∫T0em(u(s))+Em(u(s))ds in (5.1) is positive. Furthermore, the magnitude of the time-average of Em(u) is much lower than the magnitude of the time-average of em(u).
There are several research directions that we plan to pursue. Probably the most important one is the numerical investigation of the theoretical results in three-dimensional, high Reynolds number flows, which could shed new light on the energy transfer among ROM modes. A related, but different numerical investigation was performed in [7].
Luigi C. Berselli and Roger Lewandowski for the research that led to the present paper were partially supported by a grant of the group GNAMPA of INdAM and by the project the University of Pisa within the grant PRA_2018_52 UNIPI Energy and regularity: New techniques for classical PDE problems. Traian Iliescu and Birgul Koc gratefully acknowledge the support provided by the NSF DMS-1821145 grant. We thank the two reviewers for the comments and suggestions that improved the paper.
The authors declare no conflict of interest.
[1] | Batchelor GK (1953) The Theory of Homogeneous Turbulence, Cambridge University Press. |
[2] | Berselli LC, Iliescu T, Layton WJ (2006) Mathematics of Large Eddy Simulation of Turbulent Flows, Berlin: Springer-Verlag. |
[3] |
Berselli LC, Lewandowski R (2019) On the Reynolds time-averaged equations and the long-time behavior of Leray-Hopf weak solutions, with applications to ensemble averages. Nonlinearity 32: 4579-4608. doi: 10.1088/1361-6544/ab32bc
![]() |
[4] | Berselli LC, Fagioli S, Spirito S (2019) Suitable weak solutions of the Navier-Stokes equations constructed by a space-time numerical discretization. J Math Pures Appl 125: 189-208. |
[5] | Rebollo TC, Lewandowski R (2014) Mathematical and Numerical Foundations of Turbulence Models and Applications, New York: Springer. |
[6] | Constantin P, Foias C (1988) Navier-Stokes Equations, Chicago: University of Chicago Press. |
[7] | Couplet M, Sagaut P, Basdevant C (2003) Intermodal energy transfers in a proper orthogonal decomposition-Galerkin representation of a turbulent separated flow. J Fluid Mech 491: 275-284. |
[8] | DeCaria V, Layton WJ, McLaughlin M (2017) A conservative, second order, unconditionally stable artificial compression method. Comput Methods Appl Mech Engrg 325: 733-747. |
[9] | DeCaria V, Iliescu T, Layton W, et al. (2019) An artificial compression reduced order model. arXiv preprint arXiv:1902.09061. |
[10] | Girault V, Raviart PA (1986) Finite Element Methods for Navier-Stokes Equations, Berlin: Springer-Verlag. |
[11] | Foiaş C (1972/73) Statistical study of Navier-Stokes equations. I, Ⅱ. Rend Sem Mat Univ Padova 48: 219-348; 49: 9-123. |
[12] | Foias C, Manley O, Rosa R, et al. (2001) Navier-Stokes Equations and Turbulence, Cambridge: Cambridge University Press. |
[13] | Guermond JL, Minev P, Shen J (2006) An overview of projection methods for incompressible flows. Comput Methods Appl Mech Engrg 195: 6011-6045. |
[14] | Guermond JL, Oden JT, Prudhomme S (2004) Mathematical perspectives on large eddy simulation models for turbulent flows. J Math Fluid Mech 6: 194-248. |
[15] | Hesthaven JS, Rozza G, Stamm B (2016) Certified Reduced Basis Methods for Parametrized Partial Differential Equations, Berlin: Springer. |
[16] | Iliescu T, Wang Z (2014) Are the snapshot difference quotients needed in the proper orthogonal decomposition? SIAM J Sci Comput 36: A1221-A1250. |
[17] | Iliescu T, Liu H, Xie X (2018) Regularized reduced order models for a stochastic Burgers equation Int J Numer Anal Mod 15: 594-607. |
[18] | Jiang N, Layton WJ (2016) Algorithms and models for turbulence not at statistical equilibrium. Comput Math Appl 71: 2352-2372. |
[19] | Kolmogorov AN (1941) The local structure of turbulence in incompressible viscous fluids for very large Reynolds number. Dokl Akad Nauk SSR 30: 9-13. |
[20] | Kunisch K, Volkwein S (1999) Control of the Burgers equation by a reduced-order approach using proper orthogonal decomposition. J Optim Theory Appl 102: 345-371. |
[21] | Kunisch K, Volkwein S (2001) Galerkin proper orthogonal decomposition methods for parabolic problems. Numer Math 90: 117-148. |
[22] | Kunisch K, Volkwein S, Xie L (2004) HJB-POD-based feedback design for the optimal control of evolution problems. SIAM J Appl Dyn Syst 3: 701-722. |
[23] | Kunisch K, Xie L (2005) POD-based feedback control of the Burgers equation by solving the evolutionary HJB equation. Comput Math Appl 49: 1113-1126. |
[24] | Kunisch K, Volkwein S (2008) Proper orthogonal decomposition for optimality systems. ESAIM: Math Model Numer Anal 42: 1-23. |
[25] | Lassila T, Manzoni A, Quarteroni A, et al. (2014) Model order reduction in fluid dynamics: Challenges and perspectives. In: Reduced order methods for modeling and computational reduction, Springer, 9: 235-273. |
[26] | Layton WJ (2014) The 1877 Boussinesq conjecture: Turbulent fluctuations are dissipative on the mean flow. Technical Report TR-MATH 14-07, Pittsburgh Univ. |
[27] | Layton WJ, Rebholz L (2012) Approximate Deconvolution Models of Turbulence Approximate Deconvolution Models of Turbulence, Heidelberg: Springer. |
[28] | Lewandowski R (2015) Long-time turbulence model deduced from the Navier-Stokes equations. Chin Ann Math Ser B 36: 883-894. |
[29] | Lions JL, (1969) Quelques Méthodes de Résolution des Problèmes aux Limites Non Linéaires, Paris: Dunod. |
[30] | Málek J, Nečas J, Rokyta M, et al. (1996) Weak and Measure-valued Solutions to Evolutionary PDEs, London: Chapman & Hall. |
[31] | Park HM, Jang YD (2000) Control of Burgers equation by means of mode reduction. Int J of Eng Sci 38: 785-805. |
[32] |
Prandtl L (1925) Bericht über Untersuchungen zur ausgebildeten Turbulenz. Z Angew Math Mech 5: 136-139. doi: 10.1002/zamm.19250050212
![]() |
[33] | Prodi G (1960) Teoremi ergodici per le equazioni della idrodinamica, In: Sistemi Dinamici e Teoremi Ergodici, Berlin: Springer, 159-177. |
[34] | Prodi G (1961) On probability measures related to the Navier-Stokes equations in the 3-dimensional case. Technical Report AF61(052)-414, Trieste Univ. |
[35] | Quarteroni A, Manzoni A, Negri F (2016) Reduced Basis Methods for Partial Differential Equations, Berlin: Springer. |
[36] | Quarteroni A, Rozza G, Manzoni A (2011) Certified reduced basis approximation for parametrized partial differential equations and applications. J Math Ind 1: 3. |
[37] | Reynolds O (1895) On the dynamic theory of the incompressible viscous fluids and the determination of the criterion. Philos Trans Roy Soc London Ser A 186: 123-164. |
[38] | Rozza G (2014) Fundamentals of reduced basis method for problems governed by parametrized PDEs and applications, In: Separated Representations and PGD-based Model Reduction, Vienna: Springer, 153-227. |
[39] | Sagaut P (2001) Large Eddy Simulation for Incompressible Flows. Berlin: Springer-Verlag. |
[40] | San O, Maulik R (2018) Neural network closures for nonlinear model order reduction. Adv Comput Math 44: 1717-1750. |
[41] | Wells D, Wang Z, Xie X, et al. (2017) An evolve-then-filter regularized reduced order model for convection-dominated flows. Internat J. Numer Methods Fluids 84: 598-615. |
[42] | Xie X, Wells D, Wang Z, et al. (2017) Approximate deconvolution reduced order modeling. Comput Methods Appl Mech Engrg 313: 512-534. |
[43] | Xie X, Mohebujjaman M, Rebholz LG, et al. (2018) Data-driven filtered reduced order modeling of fluid flows. SIAM J Sci Comput 40: B834-B857. |
[44] | Xie X, Mohebujjaman M, Rebholz LG, et al. (2018) Lagrangian data-driven reduced order modeling of finite time Lyapunov exponents. arXiv preprint arXiv:1808.05635. |
1. | Ping Guo, Wei Tian, Huimin Li, Guangmin Zhang, Jianhui Li, Global characteristics and trends of research on construction dust: based on bibliometric and visualized analysis, 2020, 27, 0944-1344, 37773, 10.1007/s11356-020-09723-y | |
2. | Luigi C. Berselli, 2021, 9780128219546, 255, 10.1016/B978-0-12-821954-6.00011-9 | |
3. | Shady E. Ahmed, Suraj Pawar, Omer San, Adil Rasheed, Traian Iliescu, Bernd R. Noack, On closures for reduced order models—A spectrum of first-principle to machine-learned avenues, 2021, 33, 1070-6631, 091301, 10.1063/5.0061577 | |
4. | Huiping Wang, Yi Wang, Estimating CO2 emissions using a fractional grey Bernoulli model with time power term, 2022, 29, 0944-1344, 47050, 10.1007/s11356-022-18803-0 | |
5. | Thierry Delisle, Peter A. Buhr, Advanced control‐flow and concurrency in C∀, 2021, 51, 0038-0644, 1005, 10.1002/spe.2925 | |
6. | Junqi Zhu, Haotian Zheng, Li Yang, Shanshan Li, Liyan Sun, Jichao Geng, Evaluation of deep coal and gas outburst based on RS-GA-BP, 2023, 115, 0921-030X, 2531, 10.1007/s11069-022-05652-w | |
7. | Rahmat Ali Khan, Shaista Gul, Fahd Jarad, Hasib Khan, Existence results for a general class of sequential hybrid fractional differential equations, 2021, 2021, 1687-1847, 10.1186/s13662-021-03444-3 | |
8. | Hongjun Han, Liyuan Wang, Diffraction of SV waves by a surface effective elliptic cavity, 2022, 17, 1559-3959, 65, 10.2140/jomms.2022.17.65 | |
9. | Xingyue Deng, Jiuyuan Huo, Ling Wu, 2022, Chapter 20, 978-981-19-5208-1, 298, 10.1007/978-981-19-5209-8_20 | |
10. | Pradeep Kumar Dabla, Kamal Upreti, Divakar Singh, Anju Singh, Jitender Sharma, Aashima Dabas, Damien Gruson, Bernard Gouget, Sergio Bernardini, Evgenija Homsak, Sanja Stankovic, Target association rule mining to explore novel paediatric illness patterns in emergency settings, 2022, 82, 0036-5513, 595, 10.1080/00365513.2022.2148121 | |
11. | Mingsheng Yang, Jie Yang, Weihong Han, Jiawei Zhang, 2024, Chapter 24, 978-981-97-4518-0, 336, 10.1007/978-981-97-4519-7_24 |
m | ∫10em(u(s))ds | ∫10Em(u(s))ds |
3 | 2.6170e-02 | 1.0451e-03 |
6 | 7.3208e-03 | 2.2524e-03 |
9 | 1.4934e-03 | 1.5977e-03 |
15 | 3.6181e-05 | 3.6287e-05 |
20 | 1.2776e-06 | 7.0356e-07 |
25 | 4.6207e-08 | 9.5638e-09 |
30 | 2.0257e-09 | -1.1127e-10 |
35 | 8.2806e-11 | -2.2595e-11 |
m | 110∫100em(u(s))ds | 110∫100Em(u(s))ds |
3 | 3.3652e-03 | 8.6523e-05 |
6 | 1.0001e-03 | 2.1570e-04 |
9 | 2.1132e-04 | 1.8614e-04 |
15 | 5.4224e-06 | 5.5724e-06 |
20 | 4.3119e-07 | 2.5667e-07 |
25 | 5.6884e-08 | 1.0451e-08 |
30 | 1.1327e-09 | 3.2736e-10 |
35 | 2.0469e-11 | 2.6396e-12 |
m | 110∫100em(u(s))ds | 110∫100Em(u(s))ds |
3 | 3.5296e-03 | 3.0515e-06 |
6 | 1.1402e-03 | 8.8746e-06 |
9 | 2.6359e-04 | 1.5090e-05 |
15 | 1.1126e-05 | 1.1156e-05 |
20 | 9.5913e-07 | 1.5183e-06 |
25 | 1.8140e-07 | 1.8704e-07 |
30 | 4.8765e-08 | 1.3491e-08 |
35 | 1.0291e-09 | 9.7629e-10 |
40 | 2.4680e-11 | 2.0416e-11 |
m | 110∫100em(u(s))ds | 110∫100Em(u(s))ds |
3 | 3.5637e-03 | 2.7841e-06 |
6 | 1.1664e-03 | 8.1109e-06 |
9 | 2.7346e-04 | 1.3956e-05 |
15 | 1.2084e-05 | 1.1937e-05 |
20 | 1.1785e-06 | 1.6370e-06 |
25 | 2.2727e-07 | 1.2913e-07 |
30 | 6.2606e-08 | 9.5191e-09 |
35 | 2.1106e-09 | 6.1538e-10 |
40 | 1.0330e-10 | 2.0241e-11 |
m | 110∫100em(u(s))ds | 110∫100Em(u(s))ds |
3 | 3.5668e-03 | 2.7594e-06 |
6 | 1.1688e-03 | 8.0390e-06 |
9 | 2.7436e-04 | 1.3843e-05 |
15 | 1.2172e-05 | 1.2002e-05 |
20 | 1.2030e-06 | 1.6405e-06 |
25 | 2.3339e-07 | 1.2463e-07 |
30 | 6.4120e-08 | 8.8971e-09 |
35 | 2.2654e-09 | 5.6187e-10 |
40 | 1.1211e-10 | 1.8216e-11 |
m | 1100∫1000em(u(s))ds | 110∫100em(u(s))ds | ∫10em(u(s))ds |
3 | 3.3687e-04 | 3.3683e-04 | 1.7634e-04 |
6 | 1.0001e-04 | 1.0001e-04 | 7.3142e-05 |
9 | 2.1146e-05 | 2.1147e-05 | 1.6701e-05 |
15 | 5.5621e-07 | 5.5742e-07 | 4.7129e-07 |
20 | 7.9605e-08 | 7.9605e-08 | 6.3799e-08 |
25 | 8.3483e-09 | 8.3489e-09 | 5.7426e-09 |
30 | 1.0839e-10 | 1.0840e-10 | 9.2430e-11 |
35 | 2.7710e-12 | 2.7718e-12 | 2.9575e-12 |
m | 1100∫1000Em(u(s))ds | 110∫100Em(u(s))ds | ∫10Em(u(s))ds |
3 | 8.6270e-06 | 7.0320e-06 | 8.1057e-05 |
6 | 2.1568e-05 | 2.1520e-05 | 3.2405e-05 |
9 | 1.8614e-05 | 1.8599e-05 | 2.0255e-05 |
15 | 5.5718e-07 | 5.4232e-07 | 5.8207e-07 |
20 | 5.6044e-08 | 5.5874e-08 | 6.1423e-08 |
25 | 1.0936e-09 | 9.9763e-10 | 5.9386e-10 |
30 | 3.3046e-11 | 3.2478e-11 | 4.0949e-11 |
35 | 6.4170e-13 | 6.3739e-13 | 1.2125e-12 |
m | 10.1∫0.10em(u(s))ds | 10.1∫0.10Em(u(s))ds |
3 | -6.8687e-04 | 2.7378e-05 |
5 | -2.6333e-05 | 1.7795e-05 |
7 | -9.1458e-07 | 4.2432e-06 |
9 | -9.8188e-09 | 4.1220e-07 |
13 | 2.3800e-10 | 1.5749e-09 |
15 | 8.5423e-12 | 4.8043e-11 |
m | 10.1∫0.10em(u(s))ds | 10.1∫0.10Em(u(s))ds |
3 | -6.8807e-04 | 2.7338e-05 |
5 | -2.6447e-05 | 1.7812e-05 |
7 | -9.1691e-07 | 4.2755e-06 |
9 | -9.5609e-09 | 4.1694e-07 |
13 | 2.5287e-10 | 1.5941e-09 |
15 | 1.0725e-11 | 4.7030e-11 |
m | ∫10em(u(s))ds | ∫10Em(u(s))ds |
3 | 2.6170e-02 | 1.0451e-03 |
6 | 7.3208e-03 | 2.2524e-03 |
9 | 1.4934e-03 | 1.5977e-03 |
15 | 3.6181e-05 | 3.6287e-05 |
20 | 1.2776e-06 | 7.0356e-07 |
25 | 4.6207e-08 | 9.5638e-09 |
30 | 2.0257e-09 | -1.1127e-10 |
35 | 8.2806e-11 | -2.2595e-11 |
m | 110∫100em(u(s))ds | 110∫100Em(u(s))ds |
3 | 3.3652e-03 | 8.6523e-05 |
6 | 1.0001e-03 | 2.1570e-04 |
9 | 2.1132e-04 | 1.8614e-04 |
15 | 5.4224e-06 | 5.5724e-06 |
20 | 4.3119e-07 | 2.5667e-07 |
25 | 5.6884e-08 | 1.0451e-08 |
30 | 1.1327e-09 | 3.2736e-10 |
35 | 2.0469e-11 | 2.6396e-12 |
m | 110∫100em(u(s))ds | 110∫100Em(u(s))ds |
3 | 3.5296e-03 | 3.0515e-06 |
6 | 1.1402e-03 | 8.8746e-06 |
9 | 2.6359e-04 | 1.5090e-05 |
15 | 1.1126e-05 | 1.1156e-05 |
20 | 9.5913e-07 | 1.5183e-06 |
25 | 1.8140e-07 | 1.8704e-07 |
30 | 4.8765e-08 | 1.3491e-08 |
35 | 1.0291e-09 | 9.7629e-10 |
40 | 2.4680e-11 | 2.0416e-11 |
m | 110∫100em(u(s))ds | 110∫100Em(u(s))ds |
3 | 3.5637e-03 | 2.7841e-06 |
6 | 1.1664e-03 | 8.1109e-06 |
9 | 2.7346e-04 | 1.3956e-05 |
15 | 1.2084e-05 | 1.1937e-05 |
20 | 1.1785e-06 | 1.6370e-06 |
25 | 2.2727e-07 | 1.2913e-07 |
30 | 6.2606e-08 | 9.5191e-09 |
35 | 2.1106e-09 | 6.1538e-10 |
40 | 1.0330e-10 | 2.0241e-11 |
m | 110∫100em(u(s))ds | 110∫100Em(u(s))ds |
3 | 3.5668e-03 | 2.7594e-06 |
6 | 1.1688e-03 | 8.0390e-06 |
9 | 2.7436e-04 | 1.3843e-05 |
15 | 1.2172e-05 | 1.2002e-05 |
20 | 1.2030e-06 | 1.6405e-06 |
25 | 2.3339e-07 | 1.2463e-07 |
30 | 6.4120e-08 | 8.8971e-09 |
35 | 2.2654e-09 | 5.6187e-10 |
40 | 1.1211e-10 | 1.8216e-11 |
m | 1100∫1000em(u(s))ds | 110∫100em(u(s))ds | ∫10em(u(s))ds |
3 | 3.3687e-04 | 3.3683e-04 | 1.7634e-04 |
6 | 1.0001e-04 | 1.0001e-04 | 7.3142e-05 |
9 | 2.1146e-05 | 2.1147e-05 | 1.6701e-05 |
15 | 5.5621e-07 | 5.5742e-07 | 4.7129e-07 |
20 | 7.9605e-08 | 7.9605e-08 | 6.3799e-08 |
25 | 8.3483e-09 | 8.3489e-09 | 5.7426e-09 |
30 | 1.0839e-10 | 1.0840e-10 | 9.2430e-11 |
35 | 2.7710e-12 | 2.7718e-12 | 2.9575e-12 |
m | 1100∫1000Em(u(s))ds | 110∫100Em(u(s))ds | ∫10Em(u(s))ds |
3 | 8.6270e-06 | 7.0320e-06 | 8.1057e-05 |
6 | 2.1568e-05 | 2.1520e-05 | 3.2405e-05 |
9 | 1.8614e-05 | 1.8599e-05 | 2.0255e-05 |
15 | 5.5718e-07 | 5.4232e-07 | 5.8207e-07 |
20 | 5.6044e-08 | 5.5874e-08 | 6.1423e-08 |
25 | 1.0936e-09 | 9.9763e-10 | 5.9386e-10 |
30 | 3.3046e-11 | 3.2478e-11 | 4.0949e-11 |
35 | 6.4170e-13 | 6.3739e-13 | 1.2125e-12 |
m | 10.1∫0.10em(u(s))ds | 10.1∫0.10Em(u(s))ds |
3 | -6.8687e-04 | 2.7378e-05 |
5 | -2.6333e-05 | 1.7795e-05 |
7 | -9.1458e-07 | 4.2432e-06 |
9 | -9.8188e-09 | 4.1220e-07 |
13 | 2.3800e-10 | 1.5749e-09 |
15 | 8.5423e-12 | 4.8043e-11 |
m | 10.1∫0.10em(u(s))ds | 10.1∫0.10Em(u(s))ds |
3 | -6.8807e-04 | 2.7338e-05 |
5 | -2.6447e-05 | 1.7812e-05 |
7 | -9.1691e-07 | 4.2755e-06 |
9 | -9.5609e-09 | 4.1694e-07 |
13 | 2.5287e-10 | 1.5941e-09 |
15 | 1.0725e-11 | 4.7030e-11 |