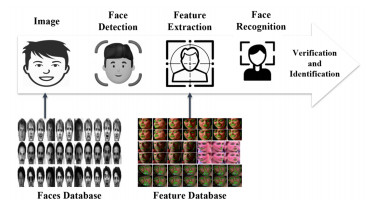
In recent years, the extensive use of facial recognition technology has raised concerns about data privacy and security for various applications, such as improving security and streamlining attendance systems and smartphone access. In this study, a blockchain-based decentralized facial recognition system (DFRS) that has been designed to overcome the complexities of technology. The DFRS takes a trailblazing approach, focusing on finding a critical balance between the benefits of facial recognition and the protection of individuals' private rights in an era of increasing monitoring. First, the facial traits are segmented into separate clusters which are maintained by the specialized node that maintains the data privacy and security. After that, the data obfuscation is done by using generative adversarial networks. To ensure the security and authenticity of the data, the facial data is encoded and stored in the blockchain. The proposed system achieves significant results on the CelebA dataset, which shows the effectiveness of the proposed approach. The proposed model has demonstrated enhanced efficacy over existing methods, attaining 99.80% accuracy on the dataset. The study's results emphasize the system's efficacy, especially in biometrics and privacy-focused applications, demonstrating outstanding precision and efficiency during its implementation. This research provides a complete and novel solution for secure facial recognition and data security for privacy protection.
Citation: Muhammad Ahmad Nawaz Ul Ghani, Kun She, Muhammad Arslan Rauf, Shumaila Khan, Masoud Alajmi, Yazeed Yasin Ghadi, Hend Khalid Alkahtani. Toward robust and privacy-enhanced facial recognition: A decentralized blockchain-based approach with GANs and deep learning[J]. Mathematical Biosciences and Engineering, 2024, 21(3): 4165-4186. doi: 10.3934/mbe.2024184
[1] | Guangmin Sun, Hao Wang, Yu Bai, Kun Zheng, Yanjun Zhang, Xiaoyong Li, Jie Liu . PrivacyMask: Real-world privacy protection in face ID systems. Mathematical Biosciences and Engineering, 2023, 20(2): 1820-1840. doi: 10.3934/mbe.2023083 |
[2] | Huiying Zhang, Jiayan Lin, Lan Zhou, Jiahui Shen, Wenshun Sheng . Facial age recognition based on deep manifold learning. Mathematical Biosciences and Engineering, 2024, 21(3): 4485-4500. doi: 10.3934/mbe.2024198 |
[3] | Hao Wang, Guangmin Sun, Kun Zheng, Hui Li, Jie Liu, Yu Bai . Privacy protection generalization with adversarial fusion. Mathematical Biosciences and Engineering, 2022, 19(7): 7314-7336. doi: 10.3934/mbe.2022345 |
[4] | Songfeng Liu, Jinyan Wang, Wenliang Zhang . Federated personalized random forest for human activity recognition. Mathematical Biosciences and Engineering, 2022, 19(1): 953-971. doi: 10.3934/mbe.2022044 |
[5] | Muhammad Firdaus, Siwan Noh, Zhuohao Qian, Harashta Tatimma Larasati, Kyung-Hyune Rhee . Personalized federated learning for heterogeneous data: A distributed edge clustering approach. Mathematical Biosciences and Engineering, 2023, 20(6): 10725-10740. doi: 10.3934/mbe.2023475 |
[6] | Xiao Dong Yang, Wen Jia Wang, Bin Shu, Mei Juan Li, Rui Xia Liu, Cai Fen Wang . Multi-message multi-receiver signcryption scheme based on blockchain. Mathematical Biosciences and Engineering, 2023, 20(10): 18146-18172. doi: 10.3934/mbe.2023806 |
[7] | Kwabena Owusu-Agyemang, Zhen Qin, Appiah Benjamin, Hu Xiong, Zhiguang Qin . Insuring against the perils in distributed learning: privacy-preserving empirical risk minimization. Mathematical Biosciences and Engineering, 2021, 18(4): 3006-3033. doi: 10.3934/mbe.2021151 |
[8] | Jiahe Xu, Yuan Tian, Tinghuai Ma, Najla Al-Nabhan . Intelligent manufacturing security model based on improved blockchain. Mathematical Biosciences and Engineering, 2020, 17(5): 5633-5650. doi: 10.3934/mbe.2020303 |
[9] | Wenjuan Wang, Deqiang Teng, Ming Chen, Yan Ge, Yibo Zou . A trading matching model for aquatic products based on blockchain and credit mechanisms. Mathematical Biosciences and Engineering, 2023, 20(11): 19732-19762. doi: 10.3934/mbe.2023874 |
[10] | Radi P. Romansky, Irina S. Noninska . Challenges of the digital age for privacy and personal data protection. Mathematical Biosciences and Engineering, 2020, 17(5): 5288-5303. doi: 10.3934/mbe.2020286 |
In recent years, the extensive use of facial recognition technology has raised concerns about data privacy and security for various applications, such as improving security and streamlining attendance systems and smartphone access. In this study, a blockchain-based decentralized facial recognition system (DFRS) that has been designed to overcome the complexities of technology. The DFRS takes a trailblazing approach, focusing on finding a critical balance between the benefits of facial recognition and the protection of individuals' private rights in an era of increasing monitoring. First, the facial traits are segmented into separate clusters which are maintained by the specialized node that maintains the data privacy and security. After that, the data obfuscation is done by using generative adversarial networks. To ensure the security and authenticity of the data, the facial data is encoded and stored in the blockchain. The proposed system achieves significant results on the CelebA dataset, which shows the effectiveness of the proposed approach. The proposed model has demonstrated enhanced efficacy over existing methods, attaining 99.80% accuracy on the dataset. The study's results emphasize the system's efficacy, especially in biometrics and privacy-focused applications, demonstrating outstanding precision and efficiency during its implementation. This research provides a complete and novel solution for secure facial recognition and data security for privacy protection.
Facial recognition technology, a popular tool for recognizing persons in images based on facial traits [1], is crucial in a variety of applications, such as attendance systems, crime prevention, and smartphone unlocking. Its main applications are in authentication, access control, and security-related operations [2]. Nonetheless, its widespread use in a variety of applications raises concerns about user privacy and data security. Because of its direct connection to an individual's identity, this technology raises concerns about significant hazards that must be addressed. The general process of the facial recognition system is presented in Figure 1; this figure shows the system taking images from the image data source as input and going through the various processes including face detection, feature extraction, and facial recognition.
The widespread use of surveillance cameras in public spaces, with an estimated 250 million cameras installed globally [3], exacerbates privacy concerns. Facial recognition technologies built into these cameras can collect and undermine the privacy of people in public places like shopping malls [4]. It is critical to strike a fine balance between the benefits of facial recognition and the preservation of privacy rights. Maintaining user data security, guarding against malicious or accidental data exposure, and avoiding privacy violations are shared responsibilities [5]. To protect sensitive information, robust data encryption and strict access control methods are required. Prioritizing user safety and privacy builds trust in technology and ensures the dependability and integrity of data-driven systems.
Efforts to resolve privacy concerns have resulted in the creation of smart cameras with incorporated privacy protections, and this has been done to improve rather than abandon monitoring practices [6]. In smart surveillance systems, using a lightweight blockchain in conjunction with identity-based distributed data storage in multi-cloud settings can assure privacy and authorized access [7]. The current threat scenario, however, necessitates a holistic strategy that goes beyond basic security measures such as a classic blockchain or simple encryption. A multi-tier authentication structure is required to strengthen security. Using deep learning architectures for data processing gives an extra degree of security. This multi-tiered method ensures that even if one security layer is breached, the remaining levels act as strong barriers, ensuring the safety of essential data and resources. When deep learning and multi-level authentication are integrated, the result is a complete and reliable security solution that is perfectly adapted to the changing digital landscape.
This study advocates for the development of a decentralized facial recognition system (DFRS) underpinned by blockchain technology to ensure robust data security and highly accurate facial recognition capability. To mitigate the limitations of centralized systems, we emphasize the use of privacy computing by generative adversarial networks (GANs) and blockchain technology. This innovative approach involves the segmentation of facial features into distinct clusters, each governed by a dedicated node and empowered by smart contracts to safeguard interactions and preserve privacy. The proposed privacy protection measures constitute a response to the increasing volume of facial data, addressing the critical need for decentralized and secure facial data management. Additionally, the study introduces a multi-level security system that employs unique security protocols at each level to enhance data integrity and access control. Incorporating intricate deep learning architectures improves security, providing the best protection for data stored in a blockchain, which ensures the accuracy of facial recognition data, and elevates security standards.
The main contributions of this study are given as follows:
● Developed a blockchain-based decentralized facial recognition system (DFRS) to ensure privacy and data security.
● Implemented advanced facial detection techniques to identify facial features with precision.
● Utilized a GAN for data obfuscation to protect sensitive information.
● Employed an auto-encoder for data encoding to further enhance data security.
● Established secure blockchain storage for secure data management.
● Attained efficient facial recognition capabilities to match incoming inputs with securely stored facial data.
blockchain technology has received substantial interest in the field of data security and integrity, with applications in a variety of industries such as healthcare, biometric security, and others [8]. Privacy-preserving biometric authentication [9] solutions for smart healthcare are notable for their ability to ensure anonymity and security while providing speedy and precise biometric identification. The authors [10] created a blockchain-based biometric authentication system for electronic health records that provides accurate and cost-effective patient identification and access management. A further study investigated the integration of blockchain with biometric technologies for automatic identity verification, as well as yielded models for blockchain-based attendance systems [11].
The advancement of surveillance technology has resulted in substantial studies into biometric-enhanced security systems, with an emphasis on authentication methods such as facial recognition, fingerprint scanning, and iris scanning. Because it eliminates the need for physical devices or passwords, biometric identification, particularly recognition, has grown in popularity [12]. Chintalapati and Raghunadh [13] suggested an attendance system based on LBPH feature extraction and a distance classifier, with efficiency taking precedence over deep learning's accuracy and generalization. The authors [14] used eigenvalue and eigenvector computations for flexible recognition, although dealing with many facials remains difficult. Deep learning techniques have also gained popularity, notably those influenced by generative adversarial networks. GANs accuracy and flexibility on criminal facial identification tasks were proven [15], while the authors of [16] enhanced facial expression recognition by implementing ongoing adversarial training, optimizing real-time performance and decreasing the computational burden.
Facial recognition technology has greatly enhanced surveillance system authentication, but it has also brought privacy problems to light, leading researchers to create more secure systems to reduce data leakage [17,18]. An innovative method called facialAdv was presented [19]; it uses adversarial stickers to trick facial recognition software. Another creative approach was put out [20], and it integrated deep learning architectures, cryptography, and picture optimization to provide a ground breaking facial feature encryption method with excellent performance metrics and impressive encryption speed. GAN-based architectures do, however, still need additional security measures for complete protection because they are vulnerable to adversarial attacks and algorithm weaknesses, despite their contributions to facial feature security.
Sensitive data security and data privacy issues have been greatly improved by the use of blockchain technology in biometric identification systems [21,22]. Biometric authentication, especially facial recognition, has been the main focus of research in the field of surveillance data protection [23]. The authors of [24] presented a low-weight blockchain smart surveillance system that has been designed to safeguard privacy. A blockchain-based facial recognition and attendance system was proposed [25], where convolutional neural networks were applied to verify attendance and a blockchain database was used to store information that cannot be tampered with Similar to [26]. The eHealth data management system [27] emphasizes the increased security offered by blockchain technology by utilizing facial recognition and a blockchain for secure tracking and identity verification. All security methods offer their advantages, but single-level authentication falls short in terms of protecting facial privacy. A robust system with multiple security levels is essential for comprehensive security. Hence, we present a multi-level authentication system that progressively enhances the security of facial features at each level.
The primary distinction between the data security literature and this study is the comprehensive and multi-level methodology that this research presents. While the literature explores numerous blockchain uses in data security and biometric identification, we present a systematic approach that integrates techniques such as data obfuscation, encoding, and blockchain storage with smart contracts. Furthermore, this method emphasizes privacy protection through applications of data decomposition and privacy-preserving noise, as well as by providing a facial recognition component that combines decoding and de-obfuscation for accurate data recognition. The multi-layered strategy, together with the use of smart contracts and the Ethereum blockchain, distinguishes the study by providing a sophisticated and privacy-focused solution for the secure management of facial biometric data.
This study differs from the previous studies; While the literature focuses on the broader implications of blockchain technology in data security and biometric identification, we present a novel and systematic technique for facial recognition systems. The novelty is in the combination of modern technologies, such as GANs for data obfuscation, neural networks for encoding, and Ethereum blockchain with smart contracts for multi-level security. Unlike previous research, which frequently emphasizes the benefits of blockchain in abstract terms, this study yielded a precise, step-by-step technique that includes facial identification, geometric feature extraction, and a secure master node. The combination of data decomposition, privacy-preserving noise, and the use of ethereum blockchain with smart contracts has yielded as a pioneering effort to solve the constraints and privacy problems associated with existing facial recognition algorithms. This study stands out because it presents a complete and privacy-focused approach, which contributes to the advancement of secure facial recognition systems beyond the realm of standard studies in the literature.
The methodology delineates a secure system by using blockchain for facial feature storage and recognition, emphasizing privacy. It employs GANs for data obfuscation and neural networks for encoding/decoding. Figure 2 visually represents the interconnected steps: gathering input facial images, identifying landmarks, feature engineering for valuable data extraction, segmentation for geometric information, securing the central master node, and conducting facial recognition while prioritizing user privacy. This multi-faceted approach ensures precise recognition of facial attributes within a robust and privacy-centric framework which is vital for secure data storage and retrieval in facial recognition systems.
The first part of this study is focused on facial detection, which is an important step in the process of protecting the privacy of user-provided facial data. Four different kinds of specialized algorithms is knowledge-based [28], appearance-based [29], template matching [30], and feature-based [31] algorithms are used in this process to identify and localize human facials precisely. Among these, appearance-based facial detection is the most accurate due to its use of deep learning algorithms and large datasets, especially when there are difficult settings. Particularly, an important part of this method is accurately identifying important facial characteristics including the mouth, nose, eyes, and eyebrows. By using the MediaPipe architecture and its facial landmarker model, which can precisely anticipate 478 facial landmarks as shown in Figure 3, the accuracy of this landmark recognition is significantly increased. These landmarks are carefully arranged into a 1434-element structured list, where each triplet of values corresponds to a distinct landmark.
In addition, the coordinates are normalized to lie between –1 and 1, guaranteeing maximum effectiveness. A crucial component of the workflow is featuring engineering, which improves model performance by locating, transforming, or producing pertinent features from raw data so that they may be further examined. The data decomposition privacy approach is used to prioritize the privacy of human facial data by breaking the data down into smaller, independent components. In particular, the human facial is finely divided into nine regions, each of which focuses on unique facial traits, making it easier to compute geometric features for individual facial segments. The accurate division of these facial regions makes it easier to extract important geometric features, such as the area and perimeter which can be found by adding the distances between successive landmark points and calculating the centroid which can be found by using Eq (3.1) and the aspect ratio.
Centroid=(∑ni=1xin,∑ni=1yin,∑ni=1zin) | (3.1) |
These four distinctive geometric features, encompassing the area, perimeter, centroid, and aspect ratio, serve as fundamental descriptors for the characterization of the spatial and structural attributes of each facial segment. The overall procedure for facial detection is presented in Algorithm 1. This algorithm starts by loading a human facial-containing input picture and choosing an appearance-based facial detection algorithm. If appearance-based is selected, a deep learning model is used to predict the facial landmarks, after which the landmark coordinates are sorted and normalized. Next, for each of the nine facial areas, the algorithm initializes the arrays for the area, perimeter, centroid, and aspect ratio. It computes the perimeter by adding the distances between successive landmarks, determines the area by measuring the enclosed space for each facial region by using the shoelace formula, and uses certain formulas to determine the centroid coordinates based on these landmarks. Furthermore, the width of the area is divided by its height to find the aspect ratio. The algorithm then builds a master node to integrate these geometric properties and safely store them for further processing, offering a thorough foundation for mathematically analyzing and describing facial traits.
Algorithm 1 Face detection and feature extraction |
1: Input: Image with a human facial. |
2: Load Image: Load the input image. |
3: Select a specialized facial detection algorithm: |
● Knowledge-based, appearance-based, template matching, or feature-based. |
4: if Appearance-based algorithm is Chosen then |
● Utilize a deep learning model for facial landmark detection and prediction. |
● Organize landmark coordinates into a structured list, normalizing values to the range between −1 and 1. |
5: Initialize empty arrays for each of the nine facial regions: |
● Area (A), perimeter (P), centroid (Cx and Cy), and aspect ratio (AR). |
6: end if |
7: for Each facial region (r) do |
● Calculate the area (A) by using the shoelace formula: |
A[r]=n∑i=1(xiyi+1−xi+1yi) |
● Compute the perimeter (P) by summing the distances between consecutive landmark points: |
P[r]=n∑i=1(xi+1−xi)2+(yi+1−yi)2 |
● Calculate the centroid by using the provided equations: |
Cx[r]=13A[r]n∑i=1(xi+xi+1)2+(xiyi+1−xi+1yi)2 |
Cy[r]=13A[r]n∑i=1(yi+yi+1)(xiyi+1−xi+1yi) |
● Compute the aspect ratio by dividing the width by the height: |
AR[r]=max(xi)−min(xi)max(yi)−min(yi) |
8: end for |
9: Create a master node: |
● Consolidate the calculated geometric features for all nine facial regions. |
10: Store securely: |
● Store the master node securely for further processing. |
End Procedure |
The procedure runs in an organized fashion. The data are split into nine sections after landmark and face detection, and these four geometric attributes are then computed for each region. Following calculation, these values are integrated to create a master node, which acts as a single point of contact for the processing and storage of secure data. This master node provides a full representation of the entire facial anatomy with its distinct spatial and structural qualities by integrating the characteristics of all nine regions. The integrated technique is critical in the study, leading to the achievement of the primary aim of secure analysis and recognition of human facial data.
Strict security protocols are in place to protect the "master node, " which houses extensive data on a person's facial characteristics. Given the importance and sensitivity of facial biometric data, data protection is crucial. A two-step strategy has been implemented to ensure security, encompassing the following steps, which are covered in algorithm 2.
Algorithm 2 Compute aspect ratio of a cluster |
1: Procedure (Input: CC (coordinates of a cluster)) |
2: Data: ▷ CC = coordinates of a cluster ▷ n = length of CC ▷ i = current landmark ▷ j = next landmark ▷ AR = aspect ratio |
3: X←[] |
4: Y←[] |
5: for each i from 0 to n−1 do |
6: X←xi |
7: Y←yi |
8: end for |
9: Compute the width of a cluster: W←max(X)−min(X) |
10: Compute the height of a cluster: H←max(Y)−min(Y) |
11: Calculate the aspect ratio of a cluster: AR←WH |
12: return AR |
13: End procedure |
By integrating obfuscation, encoding, and blockchain storage, a strong security framework is created that guarantees the integrity and secrecy of the master node. The implementation of this comprehensive strategy offers a long-term resolution for the secure administration of facial biometric information, while simultaneously acting as a safeguard against future data breaches and unwanted access. For a cluster identified by a collection of landmark coordinates, Algorithm 2 describes how to determine the aspect ratio (AR). First, the method initializes two empty arrays, X and Y, that are intended to store the landmarks' X and Y coordinates. It allocates the matching X and Y coordinates to the appropriate arrays as it iterates through each landmark, starting with the first (ⅰ) and ending with the second to last (n–1). It then calculates the highest (max) and minimum (min) X values inside the cluster to get the width (W) of the cluster. In parallel, it determines the lowest and maximum values of Y to compute the height (H). In the end, the width-to-height ratio (AR = W/H) is used to compute the aspect ratio. The output of the process is the resultant aspect ratio.
In this study, data obfuscation, which renders sensitive information unintelligible to potential attackers plays a critical role in improving data security and privacy. The process of noise injection adds randomness to the data while maintaining its original format and structure, hence improving its security [32]. Data security and diversity are improved by using a variety of noise injection techniques, including random noise, privacy-preserving noise, extreme value noise, and synthetic data. These methods supplement the data with various types of noise, making it a more diverse and secure dataset. Utilizing the capabilities of a GAN, a unique strategy is implemented that combines methods that are proposed to create synthetic data and privacy-preserving noise. Sensitive facial data are well protected by this special combination, which guarantees the master node's privacy throughout data processing.
The fundamental component of this approach is the GAN model, which consists of a generator and discriminator. The generator transforms latent space representation or random noise into valuable data samples through the application of a specific Eq (3.2).
Y=σ(W⋅X+b) | (3.2) |
where W represents weights, X is the input, B is the bias, Y is the output, and σ introduces randomness. The generator employs a linear layer to generate synthetic data, with forward pass calculations being performed as described by the Eq (3.3).
Yn=Hn(WnYn−1+Bn) | (3.3) |
The goal of the GAN is to produce realistic data samples that closely mimic the training data, and this requires synthetic data. In contrast, the discriminator works by applying binary classification (real or fake) and uses the forward approach to cause x to pass through the self.fc, adding learned biases and weights, and then burning. What is important for differentiating between produced and actual data is the sigmoid for probability-like output. The average real and fake accuracy estimations are the result of training the discriminator to distinguish between real and created data and optimizing the generator to produce data that are comparable to genuine data [32]. Within the project, this integrated method facilitates secure and useful data analysis while guaranteeing strong data protection.
Data encoding is used in conjunction with data obfuscation to further improve security by providing an additional line of defense against any intrusions. This strategy combines different encoding techniques for all-encompassing security. To minimize data size and maintain security, compression encoding was used. An encoder and a decoder comprised an auto-encoder neural network. High-dimensional data are compressed by the encoder while key characteristics are maintained. To realize effective data compression and secure storage, the network minimizes discrepancies between input data and decoder reconstructions during training. The encoder's job is to take the original 54-feature set of data and reduce its dimensionality. It does this by building a series of dense, completely linked layers:
Z1=X⋅W1+B1A1=ReLU(Z1)Z2=A1⋅W2+B2A2=ReLU(Z2)Z3=A2⋅W3+B3A3=ReLU(Z3)Z4=A3⋅W4+B4 |
The encoder in this instance has an output layer with linear activation and three hidden layers that use the ReLU activation function. Remarkably, the input data are reduced to four characteristics by the output layer's just four units. The purpose of the decoder is to reconstruct all 54 features by returning the four characteristics that make up the encoded data to their original state. This part is a mirror image of the encoder; however, it is mirrored in reverse:
Z1=X⋅W1+B1A1=ReLU(Z1)Z2=A1⋅W2+B2A2=ReLU(Z2)Z3=A2⋅W3+B3A3=ReLU(Z3)Z4=A3⋅W4+B4 |
The decoder consists of fully linked layers that have linear activation in the output layer and ReLU activation in hidden levels. The dimensionality of the original data is essentially restored by the output layer, which has 54 units. With an emphasis on data reconstruction, this cooperative method creates a comprehensive auto-encoder by learning a compact representation of the input data and utilizing it to decode and approximate the original input. By using encoding techniques in conjunction with data obfuscation, the master node's security and its sensitive facial data are strengthened overall, providing an additional line of defense against any intrusions.
One of the most important steps in ensuring the security and integrity of the sensitive data in this study is the method for storing encoded and obfuscated data on a blockchain. The ethereum blockchain network's use of distributed ledger technology capitalizes on the advantages of decentralization, dependability, and transparency. To guarantee security, data are split up into smaller pieces, replicated, and encrypted by using a private key. These shards are encrypted and dispersed globally across decentralized nodes, ensuring immutability and permanence. A Solidity smart contract named secure-array was created to handle the storage and matching of integer arrays using predetermined scores to enable transparent and secure storage. Adding items, finding the number of arrays that have been saved, comparing elements to find the best match, and retrieving particular arrays by index are all features of this smart contract. This secure data management is based on the ethereum blockchain, which has the native coin ETH. Ganache replicates the ethereum blockchain on a local host in a testing and development environment, making it easier to test and implement smart contracts. Web3, which connects to the ethereum network, loads the application binary interfacial of the smart contract, establishes the contract address, and permits the creation of blockchain transactions by sending integer arrays to the addElements function, assigned with the user's private key for security; this is how interactions with the blockchain are carried out. These transactions are validated and permanently recorded on the blockchain, ensuring data security and transaction reliability.
Facial recognition is used in the last stage of the process to match incoming inputs with securely stored facial data within the blockchain. When a match is found, the system generates a unique ID and extracts the related facial data. In the absence of a match, the input data is safely added to the blockchain via the same established mechanism. A decoding procedure is carried out to ensure the usefulness and accuracy of the data acquired from the blockchain. The compression encoding is reversed in this decoding, returning the data to their original dimensions. Furthermore, de-obfuscation is used to remove synthetic and privacy-protecting noise, assuring exact recognition and returning the facial data to their natural condition. This discussion is also presented in Algorithm 3.
Algorithm 3 Facial recognition and blockchain data management for facial features |
1: Procedure (Input: input data (incoming facial features), securely stored facial features in blockchain) |
2: Compare the incoming Input Data with the securely stored facial features in the blockchain. |
3: if Match is found then |
4: Provide a unique ID associated with the matched facial features. |
5: Retrieve the corresponding facial information from the blockchain. |
6: else |
7: Add the input data securely to the blockchain using the established process. |
8: end if |
9: To ensure data usability and accuracy: |
10: Perform decoding to reverse the compression encoding. |
11: Execute de-obfuscation to remove synthetic and privacy-preserving noise. |
12: Result: Unique ID and facial information for a match, or the input data securely added to the blockchain. |
13: End Procedure |
The secure facial feature storage and recognition system's secure-array smart contract effectively controls data storage, retrieval, and security protocols. To ensure immutability and permanence, the smart contract uses ethereum's decentralized structure to duplicate and distribute data shards worldwide among nodes while securely encrypting integer arrays. Users query the smart contract to obtain certain arrays by index throughout the retrieval process, which offers insight into the data that are stored. One way to ensure that only authorized individuals may access and alter stored facial feature data is through the use of private keys for encryption. Because ethereum's blockchain is decentralized, there is no single point of failure, which improves security. Through the suggested technique, this smart contract strategy guarantees reliable data storage, retrieval, and security.
In this work, data obfuscation is achieved by using a GAN. A discriminator plus a generator make up a GAN. The generator translates latent space representation into synthetic data, while the discriminator checks the authenticity of the created data as compared to real data. The generator and discriminator participate in an adversarial process during training, with the generator's goal being to make data that are identical to actual data and the discriminator's goal being to discriminate between the produced and the real data. Until the generator produces data that are believable and realistic, this iterative procedure is carried out. With the suggested technique, sensitive facial characteristics are protected during storage and identification through the introduction of synthetic data and noise, which is made possible by the design and operation of the GAN.
This section presents the experimental outcomes of the system. The first module discusses facial detection, and it uses image processing to identify 3D landmarks that are expressed as (x, y, z) coordinates. The facial Landmark model quickly and effectively creates a 2D array with 478 rows and three columns, normalizing data to fall between –1 and 1. It does this in only 0.12 seconds. After that use the MediaPipe facialMesh framework to divide this 2D array into nine different facial areas. This procedure takes only 0.0009 seconds to complete. As a result, nine lists are produced, each of which represents a distinct facial area and the corresponding landmark values. The four essential geometric parameters i.e., area, perimeter, centroid, and aspect ratio, are calculated inside of these defined areas. Specific functions are used to handle each region's landmark data, producing an extensive feature vector in the process. With nine pairs assigned to each region, these characteristics are neatly arranged by using a key-value pair structure. The data organization process takes just 0.042 seconds per region. Although the hardware configuration is discussed to provide background information, the main emphasis is on the creative and effective results obtained in facial feature extraction, with opportunities for additional investigation through comparison studies and debates to guarantee a thorough assessment. The creation of the master node involves aggregating data from the nine key-value pairs, a task accomplished in a mere 1.6710-6 seconds. To bolster security measures, data obfuscation is applied through the use of a GAN, requiring only 0.007 seconds. The GAN is meticulously trained on three distinct datasets, namely, Celebfacials Attributes (CelebA) *, Flickr-facials-HQ (FFHQ) †, and Human facials (HF) ‡. These datasets are preprocessed to conform to the master node format, resulting in 54 scalar values, an operation completed in just 1.25 minutes.
*https://mmlab.ie.cuhk.edu.hk/projects/CelebA.html
†https://github.com/NVlabs/ffhq-dataset
‡https://www.kaggle.com/datasets/sajjadhossaint/human-facial-dataset
The configuration of the GAN model incorporates specific parameters, including input and output dimensions, and it is subsequently trained on the CelebA, FFHQ, and HF datasets. This training process demonstrates the exceptional performance of CelebA, yielding real and fake accuracy averages of 99.8 and 99.6%, respectively, all accomplished in 7.12 minutes. For further insight, please refer to Table 1, which displays the classification results for the discriminator within the GAN model during the training process. Additionally, Figures 4–6 illustrate the accuracy graphs obtained during the training process on the FFHQ and HF datasets, providing a visual representation of the model's performance. The GAN trained on the CelebA and FFHQ datasets exhibited an average real accuracy of 99.55% and an average fake accuracy of 98.25%. Training time on the FFHQ dataset was 5.35 minutes. The GAN trained on the Human facials dataset exhibited an average real accuracy of 99.32% and an average fake accuracy of 96.78%. Lastly, the training time was 2.21 minutes for the HF dataset.
Dataset | Real Acc. | Fake Acc. | Train Time | No. of Samples | No. of Epochs | Gen. Time |
CelebA | 99.8% | 99.6% | 7.12 min | 200,000 | 10 | 0.007 Sec |
FFHQ | 99.55% | 98.25% | 5.35 min | 52,000 | 10 | 0.007 Sec |
HF | 99.32% | 96.78% | 2.21 min | 7,200 | 10 | 0.007 Sec |
The experimental configuration has many essential components that are responsible for the GAN's excellent performance. To improve the model's capacity to recognize complex patterns and subtleties, the GAN model was first painstakingly trained on three separate datasets: celebfaces attributes (CelebA), flickr-faces-HQ (FFHQ), and human faces (HF). CelebA is one of the largest and most varied datasets used, and it was crucial in improving the model's overall performance. A richer comprehension of facial traits and variations was made possible by the richness of the CelebA dataset, which helped the GAN perform more accurately. Furthermore, the exact setup of parameters, such as input and output dimensions, and the careful training procedure, which produced amazing actual and fake accuracy averages of 99.8 and 99.6%, respectively, may be credited for the GAN's extraordinary performance. This degree of precision shows how well the GAN can differentiate between real and fake data. The exceptional performance of the GAN was further enhanced by the use of deep learning techniques, which are renowned for their accuracy and flexibility. This is seen in Figures 4–6, which display the accuracy graphs and classification results for the GAN during training.
The CelebA dataset GAN performed exceptionally well due to the dataset's richness. Among the three datasets, CelebA's extensive and diverse nature allowed the model to identify intricate patterns and nuances, greatly enhancing its performance and result quality. A detailed comparison of training outcomes is shown in Table 1, which presents the technical comparison of the training outcomes of three different datasets i.e., CelebA, FFHQ, and HF by using a GAN. It comprises metrics for generation time, training time, number of epochs, true accuracy, and fake accuracy. While false accuracy denotes the network's skill on the task of properly recognizing produced pictures as fake, real accuracy reflects the GAN's capacity to discern actual photos from created ones. The GAN's remarkable genuine accuracy of 99.80% and fake accuracy of 99.60% for the CelebA dataset indicate its capacity to distinguish between actual and produced pictures.
CelebA needs 7.12 minutes to complete its 200,000 epoch training procedure. Real accuracy for the FFHQ dataset was still very high at 99.55%, whereas the fake accuracy is marginally lower at 98.25%. FFHQ takes 5.35 minutes to train, and the GAN passes through 52,000 epochs. Similar results can be seen for the HF dataset, where the fake accuracy is somewhat lower at 96.78% and the genuine accuracy is excellent at 99.32%. The HF dataset had the fastest training procedure, taking 2.21 minutes and covering 7,200 epochs. Interestingly, at 0.007 seconds, the creation time is constant across all three datasets. The consistent generation time seen for all datasets may be ascribed to the particular hardware and architecture employed in the GAN training process, assuring that picture production proceeds at an identical pace irrespective of the dataset. On the other hand, variations in dataset complexity, size, and content are likely to have an impact on the real and fake accuracy discrepancies since they can affect the training process and the network's capacity to discriminate between genuine and false images.
Table 2 provides a thorough comparison of the suggested approach with several other works in the field of facial modification detection, taking into account variables like the number of samples, epochs, training time, accuracy, and dataset. Interestingly, the suggested approach outperformed current models on several datasets, obtaining remarkably high accuracy rates on the HF, FFHQ, and CelebA datasets, respectively, of 99.32, 99.55, and 99.80%. The results demonstrate that the proposed model successfully identifies manipulated facials, achieving higher accuracy than various state-of-the-art techniques. Our investigative proposed model also outperformed several other models including AutoGAN [33], FakeSpotter [33], FID [33], Xception+Reg. [34], Multi-task [35], FWA [36] and HeadPose [37]. Additionally, the proposed approach requires comparatively less training time to reach peak accuracy. This is particularly evident on the HF dataset, where the model obtained maximal accuracy in just 2.21 minutes of training. These findings suggest that the suggested model is a potential development in the field of facial modification detection as it not only performs very well in terms of accuracy, it also shows efficiency in training. The proposed model's strengths include its impressive accuracy over a wide range of datasets and its short training periods, both of which are essential for robust performance and real-world application.
Method | Dataset | No. of Samples | No. of Epochs | Training Time | Accuracy (%) |
HeadPose | UADFV | 198,000 | 10 | 5.49 min. | 89.00 |
FWA | UADFV | 198,000 | 10 | 5.55 min. | 97.40 |
Multi-task | FFHQ | 52,000 | 10 | 5.39 min. | 65.80 |
Xception+Reg. | UADFV | 198,000 | 10 | 5.55 min. | 98.40 |
FID | CelebA | 200,000 | 10 | 7.19 min. | 99.01 |
FakeSpotter | CelebA | 200,000 | 10 | 6.55 min. | 91.90 |
AutoGAN | CelebA | 200,000 | 10 | 7.14 min. | 72.50 |
Proposed Method | HF | 7200 | 10 | 2.21 min. | 99.32 |
Proposed Method | FFHQ | 52,000 | 10 | 5.35 min. | 99.55 |
Proposed Method | CelebA | 200,000 | 10 | 7.12 min. | 99.80 |
After the successful training of the GAN model, the master node is obfuscated by converting the tensor dataset into a NumPy array, ensuring TensorFlow compatibility. It is then efficiently encoded by the auto-encoder, reducing 54 facial data elements into a 4-element array in 1.9710−4seconds, with decoding taking just 5.1110−5 seconds. This training process is completed in just 10 epochs, demonstrating speed and efficiency.
Table 3 presents a technical overview of the setup and outcomes of the auto-encoder training for three different datasets: FFHQ, HF, and CelebA. Important parameters include the mean squared error (MSE) loss, training time, sample count, encoding time, and decoding time are included. The reconstruction error between the original and auto-encoder-generated pictures is measured by MSE loss. The better reconstruction quality is indicated by lower MSE values. With a low MSE loss of 0.005, the auto-encoder demonstrated great reconstruction accuracy on the CelebA dataset. The HF dataset showed the highest MSE loss of 0.04, indicating a somewhat inferior reconstruction quality, whereas the FFHQ dataset had a slightly greater MSE loss of 0.01. The amount of time needed to train the auto-encoder on each dataset is called the training time. It took 5.13 minutes for the CelebA dataset, 3.87 minutes for FFHQ, and 2.45 minutes for HF. Shorter training periods are better since they reveal the efficacy of the auto-encoder training procedure. The size of the training dataset is reflected in the number of samples. Specifically, 200,000 samples are in CelebA, 52,000 in FFHQ, and 7200 in HF. Because it influences the model's capacity to learn from and generalize from the data, the dataset size is important. The terms "encoding time" and "decoding time" refer to how long it takes to encode data into a compressed format and then decode it back into its original format. These intervals demonstrate the effectiveness of the auto-encoder in data transformation and are crucial for real-time applications. This analysis is important to the study since it shows how well the auto-encoder performs across various datasets. By assessing the reconstruction quality (MSE loss) and the auto-encoder's efficiency in terms of training time and data transformation speed, it becomes clear how effectively the auto-encoder can capture and represent the underlying characteristics of the data. The practicality and usefulness of auto-encoders for data encoding and decoding inside the suggested system must be evaluated in light of these results. Within the larger framework of the study's aims, the data in the table assist researchers and practitioners in making well-informed judgments on which dataset and settings are best suitable for certain use cases. Figure 7 shows the loss graph obtained during the training of the auto-encoder on all three datasets. This shows the loss curves for the auto-encoder's training on the FFHQ, CelebA, and HF datasets. The auto-encoder demonstrates low reconstruction error on all datasets, indicating its ability to effectively compress and reconstruct facial data. The HF dataset had a slightly higher loss, suggesting poorer reconstruction quality compared to the CelebA and FFHQ datasets. Overall the auto-encoder performs well in facial data encoding across all datasets.
Dataset | MSE loss | Training Time | Number of Samples | Number of Epochs | Encoding Time | Decoding Time |
CelebA | 0.005 | 5.13 minutes | 200,000 | 10 | 1.97×10−4 Sec | 5.11×10−5 Sec |
FFHQ | 0.01 | 3.87 minutes | 52,000 | 10 | 1.97×10−4 Sec | 5.11×10−5 Sec |
HF | 0.04 | 2.45 minutes | 7200 | 10 | 1.97×10−4 Sec | 5.11×10−5 Sec |
It takes around 1.87 minutes to store the master node in the blockchain by using an Ethereum smart contract to secure it. Multiplying each element by 1015 in a speedy 0.078 seconds yields the desired real numbers from the floating-point tensor values in array format. In facial recognition, the smart contract retrieves the best match from the blockchain, converting the integer array into floating-point values. This floating-point array is passed through the auto-encoder's decoder, efficiently restoring the 4-element array to its original 54-element configuration. The reconstructed array, devoid of synthetic GAN data, returns as a tensor object with only the original data, all in just 0.18 seconds for 3 arrays in the blockchain. Figures 8 and 9 respectively visualize the real and fake accuracies of the generated images.
The results of the analysis provide important insights into the functioning of the system and show it's effectiveness and efficiency at various stages. Interestingly, the GAN model works quite well on the CelebA dataset, with maximum actual and fake accuracy rates of 99.8 and 99.6%, respectively. The richness and diversity of the CelebA dataset greatly improved the model's performance by strengthening its capacity to recognize minute details and complex patterns. Moreover, the exceptional accuracy attained justifies the 7.12-minute training duration for the CelebA dataset. Further boosting the system's efficiency and security was the auto-encoder, which was utilized to obfuscate and encode the master node. It produced amazing results with very little loss. The fastest processing times of the method are further demonstrated by the effective storage of the master node on the blockchain and the subsequent retrieval of the best match. All things considered, the findings show a well-organized and effective system for secure facial feature identification and archiving, which makes it a viable option for biometrics and other fields needing data protection and privacy.
This research represents significant improvements in the fields of secure facial recognition and data management. It has yielded a complete solution that properly balances privacy protection and data security. The construction of a very accurate GAN model, demonstrating outstanding real and fake accuracy rates, notably on the rich and diversified CelebA dataset, is one of the key successes. The study offers a capable auto-encoder for obfuscating and encoding the master node, resulting in fast data transformation. Another significant achievement is the system's capacity to quickly save and retrieve data from the blockchain while assuring the restoration of original, GAN-free data. Overall, this study provides a well-organized and fast method for secure facial feature recognition and archiving, making it a potential alternative for applications that require severe data protection and privacy measures in biometrics and beyond.
While the study offers a promising and new method for secure facial recognition with a focus on privacy, certain drawbacks must be acknowledged, which may influence the proposed system's broader application and robustness. First, the methodology's effectiveness is strongly dependent on the availability of varied and representative datasets for training facial recognition models. Any biases or limitations in the training data may impede the system's effectiveness, especially when dealing with different demographic groups or underrepresented facial traits. Furthermore, the success of facial detection and identification is dependent on the robustness of the algorithms used, and any flaws in these algorithms may jeopardize the overall accuracy and dependability of the system. The use of GANs for data obfuscation adds a new set of issues, such as possible artifacts in the synthetic data and GAN restrictions such as mode collapse. Although the study addresses privacy concerns, it does not go into detail on the potential implications and problems associated with user consent, data control, and inadvertent access.
Theoretical implications. This study demonstrates the efficacy of blockchain technology and GANs for developing a privacy-preserving and secure facial recognition system. The results validate the utility of blockchain decentralization and encryption for robust data security. Additionally, the successful obfuscation of facial imagery using GANs has important implications for sensitive biometric data protection. From a theoretical standpoint, this work provides a blueprint for balancing facial recognition performance with user privacy through the use of state-of-the-art cryptography, blockchain consensus protocols, and artificial intelligence-generated synthetic data.
Practical implications. The DFRS introduced in this paper could provide enhanced security and privacy for the real-world deployment of facial recognition applications. By distributing facial data across blockchain nodes rather than centralized servers, the risk of data compromise or misuse is mitigated. The system's use of AI-powered facial obfuscation also enables practical privacy preservation when handling sensitive user data. Furthermore, the DFRS can match incoming facial images against privacy-protected facial records on the blockchain, enabling practical facial identification while preventing unauthorized access to the original biometric data. Overall, this research demonstrates the real-world potential for blockchain, cryptography and AI to enable robust and privacy-centric facial recognition technology.
A technical ablation study was carried out to evaluate the distinct contributions. Critical components include the use of blockchain for secure data storage, GANs for data obfuscation, and a multi-tiered security system that uses deep learning techniques; they were methodically eliminated and their effects assessed. The ablation study's findings showed that every element is crucial to the system's overall functionality. Due to the degraded data security and integrity caused by the removal of blockchain-based storage, this technology's critical function in protecting private facial data is made clear. Likewise, the lack of GANs resulted in a notable reduction in privacy protection, underscoring their significance in assuring data privacy. The overall defense of the system was weakened by the removal of deep learning architectures and multi-tiered security systems, highlighting the need for these cutting-edge security measures. This ablation research confirms the DFRS efficacy in resolving privacy issues while preserving precise facial recognition capabilities by highlighting the crucial roles played by each component in the system's security and smooth functioning.
In an era of growing surveillance, we have investigated the data security in facial recognition systems, addressing the critical requirement to protect user privacy. We propose a complete and novel solution to the critical topic of secure facial feature storage and recognition, with a particular focus on user privacy. The precise separation of the facial into nine zones, each distinguished by geometric characteristics such as area, perimeter, centroid, and aspect ratio, provides an organized framework for data processing. The next phases of data obfuscation with GANs and encoding with auto-encoders improve data security and privacy even more. To protect sensitive facial data, the system makes use of the Ethereum blockchain's secure and decentralized storage capabilities, which are enabled by smart contracts. The experimental assessment findings highlight the efficacy and efficiency of the system, with the GAN model differentiating genuine from false data with high accuracy and the auto-encoder effectively changing and restoring data. Overall, this study advances biometrics and data privacy by providing a strong and dependable approach for secure facial feature storage and recognition, with implications for larger applications that need data security and privacy preservation.
This study may progress in various important directions in the future. The first goal is to improve the DFRS scalability by tackling the possible difficulties associated with organizing a sizable amount of facial data within a blockchain-based architecture. Second, we plan to investigate how cutting-edge technologies like edge computing and 5G networks may be integrated to provide safe, real-time facial recognition around the globe.
The authors declare that they have not used artificial intelligence tools in the creation of this article.
This project was funded by Princess Nourah bint Abdulrahman University, Riyadh, Saudi Arabia, Princess Nourah bint Abdulrahman University Researchers Supporting Project number (PNURSP2023R384), Princess Nourah bint Abdulrahman University, Riyadh, Saudi Arabia.
The authors declare there is no conflict of interest.
[1] |
I. Adjabi, A. Ouahabi, A. Benzaoui, A. Taleb-Ahmed, Past, present, and future of face recognition: A review, Electronics, 9 (2020), 1188. https://doi.org/10.3390/electronics9081188 doi: 10.3390/electronics9081188
![]() |
[2] |
C. Hu, Y. Zhang, F. Wu, X. Lu, P. Liu, X. Jing, Toward driver face recognition in the intelligent traffic monitoring systems, IEEE Trans. Intell. Transp. Syst., 21 (2019), 4958–4971. https://doi.org/10.1109/TITS.2019.2945923 doi: 10.1109/TITS.2019.2945923
![]() |
[3] |
S. Zhang, L. Wang, H. Xiong, Chaintegrity: blockchain-enabled large-scale e-voting system with robustness and universal verifiability, Int. J. Inform. Secur., 19 (2020), 323–341. https://doi.org/10.1007/s10207-019-00465-8 doi: 10.1007/s10207-019-00465-8
![]() |
[4] | R. Xu, S. Nikouei, Y. Chen, A. Polunchenko, S. Song, C. Deng, et al., Real-time human objects tracking for smart surveillance at the edge, in 2018 IEEE International conference on communications (ICC), (2018), 1–6. https://doi.org/10.1109/ICC.2018.8422970 |
[5] | X. Wu, X. Zhang, Responses to critiques on machine learning of criminality perceptions, preprint, arXiv: 1611.0413. |
[6] |
J. Wang, B. Amos, A. Das, P. Pillai, N. Sadeh, M. Satyanarayanan, Enabling live video analytics with a scalable and privacy-aware framework, ACM Trans. Multim. Comput. Commun. Appl., 14 (2018), 1–24. https://doi.org/10.1145/3209659 doi: 10.1145/3209659
![]() |
[7] |
A. Dorri, S. S. Kanhere, R. Jurdak, P. Gauravaram, Lsb: A lightweight scalable blockchain for iot security and anonymity, J. Parallel Distr. Comput., 134 (2019), 180–197. https://doi.org/10.1016/j.jpdc.2019.08.005 doi: 10.1016/j.jpdc.2019.08.005
![]() |
[8] |
O. Cheikhrouhou, K. Mershad, F. Jamil, R. Mahmud, A. Koubaa, S. Moosavi, A lightweight blockchain and fog-enabled secure remote patient monitoring system, Int. Things, 22 (2023), 100691. https://doi.org/10.1016/j.iot.2023.100691 doi: 10.1016/j.iot.2023.100691
![]() |
[9] |
N. D. Sarier, Privacy preserving biometric authentication on the blockchain for smart healthcare, Perv. Mob. Comput., 86 (2022), 101683. https://doi.org/10.1016/j.pmcj.2022.101683 doi: 10.1016/j.pmcj.2022.101683
![]() |
[10] |
E. Barka, M. A. Baqari, C. A. Kerrache, J. Herrera-Tapia, Implementation of a biometric-based blockchain system for preserving privacy, security, and access control in healthcare records, J. Sensor Actuat. Networks, 11 (2022), 85. https://doi.org/10.3390/jsan11040085 doi: 10.3390/jsan11040085
![]() |
[11] | Q. Zhang, Attendance system based on blockchain and face recognition, in 2022 International Conference on Smart Applications, Communications and Networking (SmartNets), (2022), 1–6. https://doi.org/10.1109/SmartNets55823.2022.9993992 |
[12] |
A. Srivastava, S. Chanda, U. Pal, Aga-gan: Attribute guided attention generative adversarial network with u-net for face hallucination, Image Vision Comput., 126 (2022), 104534. https://doi.org/10.1016/j.imavis.2022.104534 doi: 10.1016/j.imavis.2022.104534
![]() |
[13] | S. Chintalapati, M. V. Raghunadh, Automated attendance management system based on face recognition algorithms, in 2013 IEEE International conference on computational intelligence and computing research, (2013), 1–5. https://doi.org/10.1109/ICCIC.2013.6724266 |
[14] | M. G. Krishnan, S. B. Balaji, Implementation of automated attendance system using face recognition, Int. J. Sci. Eng. Res., 6 (2015), 30–33. |
[15] | R. Jadhav, V. Gokhale, M. Deshpande, A. Gore, A. Gharpure, H. Yadav, High fidelity face generation with style generative adversarial networks, in 2023 2nd International Conference on Smart Technologies and Systems for Next Generation Computing (ICSTSN), (2023), 1–6. https://doi.org/10.1109/ICSTSN57873.2023.10151603 |
[16] |
J. Wang, Improved facial expression recognition method based on gan, Sci. Programm., 2021 (2021), 1–8. https://doi.org/10.1155/2021/2689029 doi: 10.1155/2021/2689029
![]() |
[17] |
F. Boutros, V. Struc, J. Fierrez, N. Damer, Synthetic data for face recognition: Current state and future prospects, Image Vision Comput., 135 (2023), 104688. https://doi.org/10.1016/j.imavis.2023.104688 doi: 10.1016/j.imavis.2023.104688
![]() |
[18] |
E. E. Hemdan, W. El-Shafai, A. Sayed, Integrating digital twins with iot-based blockchain: Concept, architecture, challenges, and future scope, Wireless Pers. Commun., 131 (2023), 2193–2216. https://doi.org/10.1007/s11277-023-10538-6 doi: 10.1007/s11277-023-10538-6
![]() |
[19] |
M. Shen, H. Yu, L. Zhu, K. Xu, Q. Li, J. Hu, Effective and robust physical-world attacks on deep learning face recognition systems, IEEE Trans. Inform. Forens. Secur., 16 (2021), 4063–4077. https://doi.org/10.1109/TIFS.2021.3102492 doi: 10.1109/TIFS.2021.3102492
![]() |
[20] |
M. Alsafyani, F. Alhomayani, H. Alsuwat, E. Alsuwat, Face image encryption based on feature with optimization using secure crypto general adversarial neural network and optical chaotic map, Sensors, 23 (2023), 1415. https://doi.org/10.3390/s23031415 doi: 10.3390/s23031415
![]() |
[21] |
R. Páez, M. Pérez, G. Ramírez, J. Montes, L. Bouvarel, An architecture for biometric electronic identification document system based on blockchain, Future Int., 12 (2020), 10. https://doi.org/10.3390/fi12010010 doi: 10.3390/fi12010010
![]() |
[22] | Y. Liu, G. Sun, S. Schuckers, Enabling secure and privacy preserving identity management via smart contract, in 2019 IEEE conference on communications and network security (CNS), (2019), 1–8. https://doi.org/10.1109/CNS.2019.8802771 |
[23] |
M. Darshan, S. R. Raswanth, S. Skandan, S. S. Saravanan, R. Chandramohanan, P. Kumar, A secured blockchain based facial recognition system for two factor authentication process, Int. Confer. Electr. Electr. Eng., 894 (2022), 492–502. https://doi.org/10.1007/978-981-19-1677-9_44 doi: 10.1007/978-981-19-1677-9_44
![]() |
[24] | A. Fitwi, Y. Chen, S. Zhu, A lightweight blockchain-based privacy protection for smart surveillance at the edge, in 2019 IEEE International Conference on Blockchain (Blockchain), (2019), 552–555. https://doi.org/10.1109/Blockchain.2019.00080 |
[25] | Q. Zhang, Attendance system based on blockchain and face recognition, in 2022 International Conference on Smart Applications, Communications and Networking (SmartNets), (2022), 1–6. https://doi.org/10.1109/SmartNets55823.2022.9993992 |
[26] |
T. Saba, K. Haseeb, A. Rehman, G. Jeon, Blockchain-enabled intelligent iot protocol for high-performance and secured big financial data transaction, IEEE Trans. Comput. Soc. Syst., 2023. https://doi.org/10.1109/TCSS.2023.3268592 doi: 10.1109/TCSS.2023.3268592
![]() |
[27] | U. K. Jannat, M. MohanKumar, S. A. Islam, Human face detection and recognition in ehealth implications for blockchain data theory, in 2022 IEEE International Conference on Data Science and Information System (ICDSIS), (2022), 1–7. https://doi.org/10.1109/ICDSIS55133.2022.9915845 |
[28] |
X. Zhang, Y. Yang, Y. Zhang, H. Luan, J. Li, H. Zhang, et al., Enhancing video event recognition using automatically constructed semantic-visual knowledge base, IEEE Trans. Multim., 17 (2015), 1562–1575. https://doi.org/10.1109/TMM.2015.2449660 doi: 10.1109/TMM.2015.2449660
![]() |
[29] |
O. Starostenko, C. Cruz-Perez, V. Alarcon-Aquino, R. Rosas-Romero, Real-time facial expression recognition using local appearance-based descriptors, J. Intell. Fuzzy Syst., 36 (2019), 5037–5049. https://doi.org/10.3233/JIFS-179049 doi: 10.3233/JIFS-179049
![]() |
[30] | X. Huang, J. Xu, Y. Tai, C. Tang, Fast video object segmentation with temporal aggregation network and dynamic template matching, in Proceedings of the IEEE/CVF conference on computer vision and pattern recognition, (2020), 8879–8889. https://doi.org/10.1109/CVPR42600.2020.00890 |
[31] |
P. Shanthi, S. Nickolas, Facial landmark detection and geometric feature-based emotion recognition, Int. J. Biom., 14 (2022), 138–154. https://doi.org/10.1504/ijbm.2022.121799 doi: 10.1504/ijbm.2022.121799
![]() |
[32] |
P. Sattigeri, S. C. Hoffman, V. Chenthamarakshan, K. R. Varshney, Fairness gan: Generating datasets with fairness properties using a generative adversarial network, IBM J. Res. Dev., 63 (2019), 1–9. https://doi.org/10.1147/JRD.2019.2945519 doi: 10.1147/JRD.2019.2945519
![]() |
[33] |
G. Tang, L. Sun, X. Mao, S. Guo, H. Zhang, X. Wang, Detection of gan-synthesized image based on discrete wavelet transform, Secur. Commun. Networks, 2021 (2021), 1–10. https://doi.org/10.1155/2021/5511435 doi: 10.1155/2021/5511435
![]() |
[34] | H. Dang, F. Liu, J. Stehouwer, X. Liu, A. K. Jain, On the detection of digital face manipulation, in Proceedings of the IEEE/CVF Conference on Computer Vision and Pattern recognition, (2020), 5781–5790. https://doi.org/10.1109/CVPR42600.2020.00582 |
[35] | H. H. Nguyen, F. Fang, J. Yamagishi, I. Echizen, Multi-task learning for detecting and segmenting manipulated facial images and videos, in 2019 IEEE 10th international conference on biometrics theory, applications and systems (BTAS), (2019), 1–8. https://doi.org/10.1109/BTAS46853.2019.9185974 |
[36] | Y. Li, S. Lyu, Exposing deepfake videos by detecting face warping artifacts, preprint, arXiv: 1811.00656. |
[37] | X. Yang, Y. Li, S. Lyu, Exposing deep fakes using inconsistent head poses, in ICASSP 2019-2019 IEEE International Conference on Acoustics, Speech and Signal Processing (ICASSP), (2019), 8261–8265. https://doi.org/10.1109/ICASSP.2019.8683164 |
1. | Sinan Dede, Eleni Vrochidou, Venetis Kanakaris, George A. Papakostas, 2024, Chapter 21, 978-3-031-66704-6, 316, 10.1007/978-3-031-66705-3_21 | |
2. | Rais Abdul Hamid Khan, Yogesh Kantilal Sharma, Mandar S Karyakarte, Bipin Sule, Aarti Amod Agarkar, 2024, chapter 1, 9798369335970, 1, 10.4018/979-8-3693-3597-0.ch001 | |
3. | Juan Luis López Delgado, Juan Antonio López Ramos, A Comprehensive Survey on Generative AI Solutions in IoT Security, 2024, 13, 2079-9292, 4965, 10.3390/electronics13244965 | |
4. | Noor Ali Alshuraify, , Monitoring and surveillance systems based IoTs with Blockchain: Literature Review, 2024, 50, 2411-524X, 42, 10.56714/bjrs.50.2.5 | |
5. | K Mayuri, B S Smitha, Ginni Nijhawan, Bolleddu Devananda Rao, Shubhi Gupta, Ali Albawi, 2024, Blockchain and Machine Learning for Advanced Pattern Recognition in Biometric Security Systems, 979-8-3503-5006-7, 692, 10.1109/IC3I61595.2024.10828862 | |
6. | Naila Latif, Wenping Ma, Hafiz Bilal Ahmad, Advancements in securing federated learning with IDS: a comprehensive review of neural networks and feature engineering techniques for malicious client detection, 2025, 58, 1573-7462, 10.1007/s10462-024-11082-w |
Dataset | Real Acc. | Fake Acc. | Train Time | No. of Samples | No. of Epochs | Gen. Time |
CelebA | 99.8% | 99.6% | 7.12 min | 200,000 | 10 | 0.007 Sec |
FFHQ | 99.55% | 98.25% | 5.35 min | 52,000 | 10 | 0.007 Sec |
HF | 99.32% | 96.78% | 2.21 min | 7,200 | 10 | 0.007 Sec |
Method | Dataset | No. of Samples | No. of Epochs | Training Time | Accuracy (%) |
HeadPose | UADFV | 198,000 | 10 | 5.49 min. | 89.00 |
FWA | UADFV | 198,000 | 10 | 5.55 min. | 97.40 |
Multi-task | FFHQ | 52,000 | 10 | 5.39 min. | 65.80 |
Xception+Reg. | UADFV | 198,000 | 10 | 5.55 min. | 98.40 |
FID | CelebA | 200,000 | 10 | 7.19 min. | 99.01 |
FakeSpotter | CelebA | 200,000 | 10 | 6.55 min. | 91.90 |
AutoGAN | CelebA | 200,000 | 10 | 7.14 min. | 72.50 |
Proposed Method | HF | 7200 | 10 | 2.21 min. | 99.32 |
Proposed Method | FFHQ | 52,000 | 10 | 5.35 min. | 99.55 |
Proposed Method | CelebA | 200,000 | 10 | 7.12 min. | 99.80 |
Dataset | MSE loss | Training Time | Number of Samples | Number of Epochs | Encoding Time | Decoding Time |
CelebA | 0.005 | 5.13 minutes | 200,000 | 10 | 1.97×10−4 Sec | 5.11×10−5 Sec |
FFHQ | 0.01 | 3.87 minutes | 52,000 | 10 | 1.97×10−4 Sec | 5.11×10−5 Sec |
HF | 0.04 | 2.45 minutes | 7200 | 10 | 1.97×10−4 Sec | 5.11×10−5 Sec |
Dataset | Real Acc. | Fake Acc. | Train Time | No. of Samples | No. of Epochs | Gen. Time |
CelebA | 99.8% | 99.6% | 7.12 min | 200,000 | 10 | 0.007 Sec |
FFHQ | 99.55% | 98.25% | 5.35 min | 52,000 | 10 | 0.007 Sec |
HF | 99.32% | 96.78% | 2.21 min | 7,200 | 10 | 0.007 Sec |
Method | Dataset | No. of Samples | No. of Epochs | Training Time | Accuracy (%) |
HeadPose | UADFV | 198,000 | 10 | 5.49 min. | 89.00 |
FWA | UADFV | 198,000 | 10 | 5.55 min. | 97.40 |
Multi-task | FFHQ | 52,000 | 10 | 5.39 min. | 65.80 |
Xception+Reg. | UADFV | 198,000 | 10 | 5.55 min. | 98.40 |
FID | CelebA | 200,000 | 10 | 7.19 min. | 99.01 |
FakeSpotter | CelebA | 200,000 | 10 | 6.55 min. | 91.90 |
AutoGAN | CelebA | 200,000 | 10 | 7.14 min. | 72.50 |
Proposed Method | HF | 7200 | 10 | 2.21 min. | 99.32 |
Proposed Method | FFHQ | 52,000 | 10 | 5.35 min. | 99.55 |
Proposed Method | CelebA | 200,000 | 10 | 7.12 min. | 99.80 |
Dataset | MSE loss | Training Time | Number of Samples | Number of Epochs | Encoding Time | Decoding Time |
CelebA | 0.005 | 5.13 minutes | 200,000 | 10 | 1.97×10−4 Sec | 5.11×10−5 Sec |
FFHQ | 0.01 | 3.87 minutes | 52,000 | 10 | 1.97×10−4 Sec | 5.11×10−5 Sec |
HF | 0.04 | 2.45 minutes | 7200 | 10 | 1.97×10−4 Sec | 5.11×10−5 Sec |