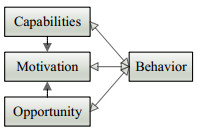
The sedentary behavior among college students has become one of the most important factors affecting the development of physical and mental health. Chronic lack of physical activity may lead to health problems such as decreased physical fitness, and increased psychological disorders. In the post-epidemic era, it is necessary for college students to have a strong immune system, and a strong body cannot be achieved without regular leisure physical activity. Therefore, it is necessary to explore the relationship between relevant health factors and physical activity. This paper presents an optimized COM-B model. And the experimental results show that the optimized model is well applied in describing the current situation of physical activity participation among college students, analyzing the distribution characteristics of socio-demographic variables related to physical activity, and exploring the correlation between physical activity and the subhealth status of college students.
Citation: Hanying Zhang, Zhongqiu Xu. The correlation between physical inactivity and students' health based on data mining and related influencing factors[J]. Mathematical Biosciences and Engineering, 2023, 20(4): 6735-6750. doi: 10.3934/mbe.2023290
[1] | Xu Lu, Chuan Yang, Yujing Zhang, Shanqiu Huang, Li Li, Haoqun Chen, Long Gao, Yan Ma, Wei Song . Test method for health-related physical fitness of college students in mobile internet environment. Mathematical Biosciences and Engineering, 2019, 16(4): 2189-2201. doi: 10.3934/mbe.2019107 |
[2] | Zhenghong Xu, Juan Du . A mental health informatics study on the mediating effect of the regulatory emotional self-efficacy. Mathematical Biosciences and Engineering, 2021, 18(3): 2775-2788. doi: 10.3934/mbe.2021141 |
[3] | Meiting Qu, Shaohui Liu, Lei Li . Urban public health spatial planning using big data technology and visual communication in IoT. Mathematical Biosciences and Engineering, 2023, 20(5): 8583-8600. doi: 10.3934/mbe.2023377 |
[4] | Xiaoli Yuan, Wenzhu Song, Yaheng Li, Qili Wang, Jianbo Qing, Wenqiang Zhi, Huimin Han, Zhiqi Qin, Hao Gong, Guohua Hou, Yafeng Li . Using Bayesian networks with tabu algorithm to explore factors related to chronic kidney disease with mental illness: A cross-sectional study. Mathematical Biosciences and Engineering, 2023, 20(9): 16194-16211. doi: 10.3934/mbe.2023723 |
[5] | Lu-Ting Xia, Chun-Heng Ho, Xing-Min Lin . Evaluation of the elderly health examination app based on the comprehensive evaluation method of AHP-fuzzy theory. Mathematical Biosciences and Engineering, 2021, 18(4): 4731-4742. doi: 10.3934/mbe.2021240 |
[6] | Xiaoke Li, Fuhong Yan, Jun Ma, Zhenzhong Chen, Xiaoyu Wen, Yang Cao . RBF and NSGA-II based EDM process parameters optimization with multiple constraints. Mathematical Biosciences and Engineering, 2019, 16(5): 5788-5803. doi: 10.3934/mbe.2019289 |
[7] | Yu-Mei Han, Hui Yang, Qin-Lai Huang, Zi-Jie Sun, Ming-Liang Li, Jing-Bo Zhang, Ke-Jun Deng, Shuo Chen, Hao Lin . Risk prediction of diabetes and pre-diabetes based on physical examination data. Mathematical Biosciences and Engineering, 2022, 19(4): 3597-3608. doi: 10.3934/mbe.2022166 |
[8] | Yi Yin, Ting Zeng, Miao Lai, Zemin Luan, Kai Wang, Yuhang Ma, Zhiliang Hu, Kai Wang, Zhihang Peng . Impact of antibody-level on viral shedding in B.1.617.2 (Delta) variant-infected patients analyzed using a joint model of longitudinal and time-to-event data. Mathematical Biosciences and Engineering, 2023, 20(5): 8875-8891. doi: 10.3934/mbe.2023390 |
[9] | Xiujun Zhang, H. G. Govardhana Reddy, Arcot Usha, M. C. Shanmukha, Mohammad Reza Farahani, Mehdi Alaeiyan . A study on anti-malaria drugs using degree-based topological indices through QSPR analysis. Mathematical Biosciences and Engineering, 2023, 20(2): 3594-3609. doi: 10.3934/mbe.2023167 |
[10] | Richard J. Braun, Rayanne A. Luke, Tobin A. Driscoll, Carolyn G. Begley . Dynamics and mechanisms for tear breakup (TBU) on the ocular surface. Mathematical Biosciences and Engineering, 2021, 18(5): 5146-5175. doi: 10.3934/mbe.2021262 |
The sedentary behavior among college students has become one of the most important factors affecting the development of physical and mental health. Chronic lack of physical activity may lead to health problems such as decreased physical fitness, and increased psychological disorders. In the post-epidemic era, it is necessary for college students to have a strong immune system, and a strong body cannot be achieved without regular leisure physical activity. Therefore, it is necessary to explore the relationship between relevant health factors and physical activity. This paper presents an optimized COM-B model. And the experimental results show that the optimized model is well applied in describing the current situation of physical activity participation among college students, analyzing the distribution characteristics of socio-demographic variables related to physical activity, and exploring the correlation between physical activity and the subhealth status of college students.
Physical activity is any form of activities that result from increased energy expenditure due to skeletal muscle contraction, including four areas: occupational activities, household chores, transportation to and from, and sports [1,2,3]. For the current college students, their autonomy of choice, living conditions, and social networks have significantly changed. However, college students do not choose to lead active lifestyles, and studies have shown that leisure physical activity among them declines rapidly during college [4]. Leisure physical activity is the most significant part of physical activity in terms of energy expenditure, and the benefits of physical activity are all met by leisure physical activity [5].
Competitive sports can cultivate students' sense of competition. Modern society is full of competition everywhere, and the sense of competition is closely related to competitive sports; In the competition activities, there is no inequality other than individual physical and psychological. Students can fully exert their potential and reflect their strength in physical exercise, sports, and competition. In sports, the rule of law is the most important; The most realistic, regardless of qualifications; The most practical, not vain. This requires that each participant should try his best to compete, especially in some directly antagonistic sports, such as football, basketball, boxing, etc., thus gradually enhancing the students' sense of competition and tenacity. It is helpful to cultivate students' good social morality and cooperative spirit in competitive sports, all sports must abide by strict rules. People can only make efforts to create achievements when the rules allow. Any behavior that violates the rules will be condemned and punished. At the same time, in a collective project, everyone has his or her own role, each role has its own specific behavior requirements, and must act according to the role requirements, which is very conducive to the socialization of people, and is conducive to the cultivation of people's concept and behavior of abiding by the law. Competitive sports always follow the basic principle of "equal opportunity, survival of the fittest". Strict rules and fair referees can cultivate students' concept of fair competition; Through competitive sports, we can also learn and practice how to correctly deal with the mistakes and failures in the competition, temper our strong will, enhance our ability to resist setbacks, and make students have strong vitality in the fierce society. Therefore, students can cultivate good sports ethics and social morality by participating in competitive sports. That is, how to make contributions to the public welfare of social groups and organizations, how to improve social awareness, and how to develop respect and understanding for the rights and feelings of others.
The study [6] has found that current college students have high levels of malnutrition, overweight, and correspondingly the number of the failures of physical fitness and function continues to increase. And the lack of physical activity among college students at the college level can cause physical health problems not only during college, but also lead to increased health risks later in life [7]. For example, the proportion of myopia among high school students is 83.28%, while that of college students is as high as 87.67% [8]. The most influential factor in this is the serious lack of physical activity among students, as shown in a report by the World Health Organization that more than 80% of school adolescents worldwide do not meet the recommended amount [9,10]. What means can be used to effectively reverse the serious decline in physical fitness of college students and improve their physical health is the primary issue that needs to be addressed now. In conclusion, the physical activity has a protective effect on reducing the risk of subhealth status among college students. The physique of college students refers to the quality of their physical health. It not only includes congenital factors, but also has the influence of acquired exercise. It is a comprehensive sports index. The current definition of health is no longer limited to the absence of physical diseases, but also includes psychological health, interpersonal health, moral health, etc. It is a comprehensive performance indicator. Although health is not equal to physique, they are an organic whole connected with each other. Only a healthy body can guarantee a good constitution, but the health of the constitution is not only affected by its own conditions, but also restricted by the environment, health, diet, habits, and other aspects.
To quantify and model the physical ability of college students more holistically, we introduced the COM-B model and proposed a physical activity behavior intervention model from the relationship among capability, opportunity, motivation, and behavior. The aim is to develop a way to promote student exercise awareness and abilities from a behavioral intervention perspective. Compared with the existing studies, the proposed method is more systematic and holistic, and the results of the analysis of physical ability and behavior of the student group are more accurate.
At present, there have been some studies on the measurement indicators and models of educational environment levels. The study [11] mentioned the student fitness indicators among normal weight college students and the relationship between physical activity and student fitness indicators. The study [12] shows that it provides a theoretical and practical basis for a more comprehensive, scientific, simple, and practical evaluation of students' physical fitness levels, and has positive practical implications for the smooth implementation of physical education reform in schools. The study [13] suggested that the interrelationship between school's physical education environment and students' physical activity and the influencing factors are needed before student physical activity interventions and instruments.
However, those research on the factors and models is focused on the physical environment and teachers' strength, and there is less research on other factors that can influence students' physical activity in schools.
Some researchers attempted to explore quantitative methods of students' physical activities. The study [14] concluded that, in contrast, the campus physical education environment is richer for students in China's colleges and universities, and in view of this, the research object of this paper was chosen to be students in colleges and universities, and there is a more in-depth exploration of the interrelationship between student health and the lack of physical activity among students. The study [15] concluded that the double-labeled water method is considered the gold standard for the measurement of human energy expenditure and indirect calorimetry is often used to calibrate other methods of measurement, but these two methods are difficult to apply in large populations. The study [16] concluded that students should be guided to carry out planned, purposeful, and regular physical exercise according to their age, gender, and physical condition, and strive to improve their physical form and function, enhance their motor ability, and meet physical fitness standards, requiring that Chinese secondary school students should spend no less than one hour per day on physical activity. The study [17] suggested that the vast majority of college students do not spend more than 1 hour of physical activity per day, and among them, those who spend less than 30 min of physical activity per day account for about 60%. Subhealth is a state of low quality of health between health and disease and its experience. Individual subhealth is mainly manifested in physical subhealth and psychological subhealth [18,19]. In addition, national and regional policies and laws play an important role in improving students' physical activity [20,21,22,23,24,25].
In summary, those research is mainly carried out from the above three perspectives, including the students' physical fitness, the educational environment, and the policy and law. However, few studies have integrated the perspectives and clarified the relationship to comprehensively explore the students' physical fitness and guide a positive behavior.
The COM-B model, is a behavior change model of the assumes that behavior occurs through three necessary components: capability, opportunity, and motivation as shown in Figure 1. 1) Capability, refers to an individual's ability to participate in related activities, divided into physical capability (physical skills, strength, and endurance) and mental capability (knowledge and psychological skills involved in the thought processes): physical capability can be achieved through the development of physical skills. 2) Opportunity is the environment. It refers to all external factors that guarantee or promote the occurrence of individual behavior, including physical environment (time, resources, geographical location, materials, etc.) and social environment (words, ideas, interpersonal influences, social and cultural practices, etc.). 3) Motivation refers to the process of brain activity that motivates and directs the behavior. It is divided into reflexive motivation (including planning and evaluation) and spontaneous motivation (including emotional responses, wishes, impulses, etc.).
As shown in Figure 1 that the model reflects that when people perform a particular behavior (B), they must have the physical and psychological capability (C), and have the opportunity (O) to achieve the behavior. Meanwhile, the motivation (M) for this behavior will prevail over any other behaviors' motivation at this moment. The capability, the opportunity, the motivation, and the behavior are the components of the COM-B model, and the four components interact and react. If one or more components are changed by a specific intervention, the other components will also change.
This study conducted an empirical study on the capability, opportunity, and motivation-behavior (COM-B) model of BCW theory to further enrich the research and application of this theoretical model in China. The aerobic exercise intervention program based on the COM-B model provides an empirical reference for the development of physical activity guidelines for physically inactive elderly people with chronic diseases in China. A model of physical activity exercise behavior intervention for middle school students was constructed based on the COM-B model, as shown in Figure 2.
As shown in Figure 3, the relationship between the influencing factors can be elaborated as follows: based on the judgment of the benefits and disadvantages brought by the target behavior and the internal preference of the behavior, combined with the attitude of the important people around them whether they approve of engaging in the behavior and their behavior role model, as well as the prediction of the possible facilitating or hindering factors in the process of engaging in the target behavior, and judge their own coping capability, and then generate the willingness to engage in the target behavior. In this process, demographic characteristics such as individual age, gender, and race moderate the effect. On the one hand, demographic characteristics help to partly reflect individuals' capabilities, opportunities, and motivations from the perspective of the whole. However, the richness of information contained in demographic characteristics is limited, and it is difficult to reflect the differentiated characteristics of individuals, as well as the complexity and dynamics of behavior and cognition.
The weights of the corresponding indicators can be found in Eq (1):
ˉwi=(n∏j=1aij)1/n | (1) |
And from this, the weight vector is obtained as Eq (2).
ˉW=(w1,w2,…,wn)T | (2) |
The skewness coefficient is defined as calculated by the formula.
SK=NN∑i=1(xi−ˉx)3(N−1)(N−2)s3 | (3) |
The wavelet energy (E) is calculated as:
E=4∑i=3‖d(i)‖2 | (4) |
The acceleration data of the waist is preprocessed and the feature values are extracted for training to obtain the corresponding daily physical activity detection model. The radial basis function is defined as shown in the calculation equation.
k(xi,x)=exp{−|xi−ˉx|2σ2} | (5) |
In this paper, after calculating the storage space required by the wearable device software, several consecutive 50 pages of the Flash of MCU CC2541 are used as data storage areas. In this method, the storage structure of Flash is designed to contain Flash start address, end address, current read pointer, current write pointer, and the number of bytes currently present in Flash, which avoids repeated erasure of the fixed address of Flash and improves the lifetime of Flash, as Figure 4.
At the same level as high school and college students, with only 25% of adults meeting the minimum recommended amount of vigorous physical activity, about 39.6% of young adults aged 18–24 met the recommended amount of vigorous physical activity level. The optimized model was shown to have a good fit. This resulted in the determination of the effect relationships among the factors shown in Figure 5.
In terms of model fit, the test values for the four control indicators were RMSEA = 0.076, SRMR = 0.082, CFI = 0.883, and TLI = 0.843, showing that the analyzed model has a good fit to the data. This judged that the structural relationship shown in Figure 6 holds.
For secondary school students, self-efficacy is also strongly associated with physical activity and is a determinant of physical activity participation among secondary school students, while perceived behavioral control is a determinant, but there is no conclusive evidence of an effect on physical activity participation among secondary school students. Personal physical activity history and exercise purpose were also directly associated with physical activity participation in adults. In addition, age, gender, education level, race, and social support were also associated with individual physical activity, but these were not determinants of individual participation in physical activity. The results of the validated factorial analysis of the COM-B model by measuring the COM-B model in the previous section showed that the construct validity of the COM-B model was relatively good, and then the entire COM-B model needed to be analyzed. In this study, the hypothetical model was fitted and analyzed by using AMOS 24.0 software. And the corresponding residual terms were set, the maximum fit method was used to fit the model, and the final model was obtained for the secondary school students' physical education The structural equation COM-B model fitting diagram of the influencing factors of health behavior cultivation was finally obtained, as shown in Figure 7.
In summary, the leisure physical activities of college students in this study were mainly walking, with the least amount of moderate intensity physical activities, and most of them did not meet the recommended amount of leisure physical activities. Significant differences existed among college students in terms of gender, body mass index classification, level of social support, and obese people had better leisure physical activity than low weight people.
The test results were summarized in Figure 8, In Figure 8, the x axis is the grouping of different students, and the y axis is the score. and the statistical analysis showed that the overall distribution of test scores showed a trend of less at both ends and more in the middle. The excellent rate of the tested college students was 5.7%, the good rate was 35.2%, the passing rate was 40.7%, and the failing rate was 18.4%. The percentage of excellent and good accounted for 40.9% of the total number of students, and the percentage of pass and fail accounted for 59.1%, indicating that the proportion of college students' physical health in these universities was relatively small in good, and the proportion of pass and fail was relatively large. In terms of gender, the number of students who reach good is not as many as that of female students.
Height is a morphological indicator of the longitudinal dimension of the human body, reflecting the height and skeletal growth and development; weight is an indicator showing the transverse dimension of the human body, reflecting the weight and development of bones, muscles, and fat. In Figures 9 and 10, the x axis is the number of people and the y axis is the probability. Height is affected by age, gender, genetic factors, dietary habits, exercise, disease, living conditions, geography, and other factors; in general, an increase in weight indicates an increase in muscle, muscle strength growth, and improved nutritional status. The comparison of the statistical graphs of height distribution (Figure 9) and weight distribution (Figure 10) shows that the tested college students are higher than the national average in both height and weight [26,27].
The International Obesity Expert Group jointly issued the obesity criteria defined in the guiding manual "Redefinition and treatment of obesity in the Asia-Pacific region" in February 2002 (as Table 1).
Indicators | Indicators |
BMI < 18.5 | Underweight |
18.5 ≤ BMI < 23 | Standard weight |
23 ≤ BMI < 25 | Overweight |
25 ≤ BMI < 30 | degree Ⅰ |
BMI ≥ 30 | degree Ⅱ |
The statistical analysis showed that the overall mean value of BMI of college students was 20.5 kg/m2, among which the mean value for male students was 23 kg/m2 and for female students was 20.5 kg/m2. Comparing the students of different grades separately, the analysis of the distribution of the tested BMI values (Table 2) showed that the BMI value of 2011 grade (junior) students reached the highest, and the distribution showed a trend of ascending and then the distribution showed a trend of "decreasing" shape. The BMI values of both male and female students increased more in 2011 class than in 2012 class, and decreased in 2010 class than in 2011 class.
Grade | 2012 | 2011 | 2010 | M±SD |
Male students | 21.8 ± 22 | 21.4 ± 2.3 | 22.9 ± 1.6 | 23 ± 18 |
Female | 18.2 ± 1.2 | 20.5 ± 1.2 | 21.6 ± 2.2 | 20.5 ± 3.0 |
Average value | 19.5 ± 2.8 | 21.2 ± 3.5 | 22.6 ± 2.2 | 21.5 ± 2.6 |
The analysis of the data (as Table 3) shows that the overall mean spirometry of the tested college students was 3294.8, 3971.1 ml for males and 2684.2 ml for females, and the development trend shows that the spirometry of males increased with grade from 2012 to 2010, with an overall increase of 100 ml over two years; the change in spirometry of females was the same as that of males, with the same increase of 130 ml over two years. It shows that the lung capacity of the tested college students generally increased gradually with the increase of the grade [26,27].
Grade | 2012 | 2011 | 2010 | M±SD |
Male students | 21.8 ± 22 | 21.4 ± 2.3 | 22.9 ± 1.6 | 23 ± 18 |
Female | 18.2 ± 1.2 | 20.5 ± 1.2 | 21.6 ± 2.2 | 20.5 ± 3.0 |
Average value | 19.5 ± 2.8 | 21.2 ± 3.5 | 22.6 ± 2.2 | 21.5 ± 2.6 |
Aerobic exercise should last at least 10 minutes at a time. Based on the above criteria, the students in this study did not achieve the recommended amount of physical activity time. Physical fitness was divided into three main categories: endurance, flexibility and strength, and speed and agility. The scores of these three categories were aggregated to obtain the total physical fitness score of college students. The national data were obtained from the results of the 2005 and 2010 China Student Physical Fitness and Health Survey published by the Ministry of Education by calculating the scores (as Figure 11).
The summary results of the endurance quality test scores of different grades are shown in Figure 12. Figure 12 shows that the overall score of endurance quality is not high, just above the passing line. The endurance quality of male students tended to increase with age, and the overall score of male students in grade 2011 exceeded 70, while the overall scores of grades 2012 and 2011 were between 60 and 65; the endurance quality of female students rose and then decreased, and the endurance quality of female students in grade 2011 was better than that of grades 2012 and 2010, but none of the three grades exceeded 70. The trend of the three-dimensional acceleration values of the voltages is shown in Figure 13.
Although ambulation is also a stationary type of physical activity, the three axes are different in terms of values compared to lying down, with the reverse acceleration values in the coronal axis of ambulation being greater than in the sagittal axis, which is well screened by SWA.
The trend of walking 3D acceleration values as shown in Figure 14.
The overall image of the walking waveform shows a jagged distribution, with obvious peaks and troughs in the three axes, no intersection area in the three axes, and regular frequency of fluctuations in each axis. The trend of running 3D acceleration value is shown in Figure 15.
The three axes of the running waveform plot clearly overlap, and the amplitude of each axis increases significantly, reaching 0.0–3.0 for the vertical axis, 0.0–1.0 for the coronal axis, and -0.5–1.0 for the sagittal axis, which has both positive and negative acceleration.
To sum up, increasing the time of physical activity in middle and high school is beneficial to physical health, and through regular physical activity of certain intensity and adjusting the dietary structure of middle and high school students, thus preventing obesity and reducing body fat rate have a positive impact on the physical health of middle and high school students. Changes in body composition, especially body fat, can affect the development of physical and mental health of college students. An appropriate level of physical activity not only helps to improve the level of body health, but also helps to maintain a balanced body composition.
Physical activity is undoubtedly related to the positive results of the metabolism of the three major nutrients in the human body, but there are numerous factors that affect the intestinal flora, including diet, age, development, genetics, emotions, etc. The role of the composition of the intestinal microbiota and its metabolites as regulators still needs further research, and a sedentary lifestyle for a long time can lead to a variety of health problems in the organism and can have an interactive effect on other diseases, increasing physical activity and moderate exercise are beneficial for material-energy metabolic processes. However, the proposed method still has some limitations. The model in this paper is suitable for understanding the relationship among the students' behavior change, capability, environment, and motivation from the perspective of the whole. But for the differentiated individuals, its performance is limited.
In the future, we will attempt to further study the method of rapid modeling of each individual's physical ability to achieve personalized sports-related cognition and behavior intervention.
The authors declared that they have no conflicts of interest regarding this work.
[1] |
A. K. Ghrouz, M. M. Noohu, D. Manzar, D. W. Spence, A. S. BaHammam, S. R. Pandi-Perumal, Physical activity and sleep quality in relation to mental health among college students, Sleep Breathing, 23 (2019), 627–634. https://doi.org/10.1007/s11325-019-01780-z doi: 10.1007/s11325-019-01780-z
![]() |
[2] |
C. Romero-Blanco, J. Rodríguez-Almagro, M. D. Onieva-Zafra, M. L. Parra-Fernández, M. D. C. Prado-Laguna, A. Hernández-Martínez, Physical activity and sedentary lifestyle in university students: changes during confinement due to the COVID-19 pandemic, Int. J. Environ. Res. Public Health, 17 (2020), 6567. https://doi.org/10.3390/ijerph17186567 doi: 10.3390/ijerph17186567
![]() |
[3] |
T. R. Snedden, J. Scerpella, S. A. Kliethermes, R. S. Norman, L. Blyholder, J. Sanfilippo, et al., Sport and physical activity level impacts health-related quality of life among collegiate students, Am. J. Health Promot., 33 (2019), 675–682. https://doi.org/10.1177/0890117118817715 doi: 10.1177/0890117118817715
![]() |
[4] |
Y. Zhang, H. Zhang, X. Ma, Q. Di, Mental health problems during the COVID-19 pandemics and the mitigation effects of exercise: a longitudinal study of college students in China, Int. J. Environ. Res. Public Health, 17 (2020), 3722. https://doi.org/10.3390/ijerph17103722 doi: 10.3390/ijerph17103722
![]() |
[5] |
L. Bertrand, K. A. Shaw, J. Ko, D. Deprez, P. D. Chilibeck, G. A. Zello, The impact of the coronavirus disease 2019 (COVID-19) pandemic on university students' dietary intake, physical activity, and sedentary behaviour, Appl. Physiol. Nutr. Metab., 46 (2021), 265–272. https://doi.org/10.1139/apnm-2020-0990 doi: 10.1139/apnm-2020-0990
![]() |
[6] |
N. E. Peterson, J. R. Sirard, P. A. Kulbok, M. D. DeBoer, J. M. Erickson, Sedentary behavior and physical activity of young adult university students, Res. Nurs. Health, 41 (2018), 30–38. https://doi.org/10.1002/nur.21845 doi: 10.1002/nur.21845
![]() |
[7] |
S. Sukys, V. J. Cesnaitiene, A. Emeljanovas, B. Mieziene, I. Valantine, Z. M. Ossowski, Reasons and barriers for university students' leisure-time physical activity: moderating effect of health education, Perceptual Motor Skills, 126 (2019), 1084–1100. https://doi.org/10.1177/0031512519869089 doi: 10.1177/0031512519869089
![]() |
[8] |
V. Violant-Holz, M. G. Gallego-Jiménez, C. S. González-González, S. Muñoz-Violant, M. J. Rodríguez, O. Sansano-Nadal, et al., Psychological health and physical activity levels during the COVID-19 pandemic: a systematic review, Int. J. Environ. Res. Public Health, 17 (2020), 9419. https://doi.org/10.3390/ijerph17249419 doi: 10.3390/ijerph17249419
![]() |
[9] |
M. H. Murphy, A. Carlin, C. Woods, A. Nevill, C. MacDonncha, K. Ferguson, et al., Active students are healthier and happier than their inactive peers: the results of a large representative cross-sectional study of university students in Ireland, J. Phys. Act. Health, 15 (2018), 737–746. https://doi.org/10.1123/jpah.2017-0432 doi: 10.1123/jpah.2017-0432
![]() |
[10] |
D. Schultchen, J. Reichenberger, T. Mittl, T. R. Weh, J. M. Smyth, J. Blechert, et al., Bidirectional relationship of stress and affect with physical activity and healthy eating, Br. J. Health Psychol., 24 (2019), 315–333. https://doi.org/10.1111/bjhp.12355 doi: 10.1111/bjhp.12355
![]() |
[11] |
Z. Zhang, W. Chen, A systematic review of the relationship between physical activity and happiness, J. Happiness Stud., 2 (2019), 1305–1322. https://doi.org/10.1007/s10902-018-9976-0 doi: 10.1007/s10902-018-9976-0
![]() |
[12] |
F. Wang, S. Boros, The effect of physical activity on sleep quality: a systematic review, Eur. J. Physiother., 23 (2021), 11–18. https://doi.org/10.1080/21679169.2019.1623314 doi: 10.1080/21679169.2019.1623314
![]() |
[13] |
F. Zurita-Ortega, S. San Román-Mata, R. Chacón-Cuberos, M. Castro-Sánchez, J. J. Muros, Adherence to the mediterranean diet is associated with physical activity, self-concept and sociodemographic factors in university student, Nutrients, 10 (2018), 966. https://doi.org/10.3390/nu10080966 doi: 10.3390/nu10080966
![]() |
[14] |
S. Dogra, L. MacIntosh, C. O'Neill, C. D'Silva, H. Shearer, K. Smith, et al., The association of physical activity with depression and stress among post-secondary school students: A systematic review, Mental Health Phys. Act., 14 (2018), 146–156. https://doi.org/10.1016/j.mhpa.2017.11.001 doi: 10.1016/j.mhpa.2017.11.001
![]() |
[15] |
E. Sharara, C. Akik, H. Ghattas, C. Makhlouf Obermeyer, Physical inactivity, gender and culture in Arab countries: a systematic assessment of the literature, BMC Public Health, 18 (2018), 1–19. https://doi.org/10.1186/s12889-018-5472-z doi: 10.1186/s12889-017-4524-0
![]() |
[16] |
E. L. Caputo, F. F. Reichert, Studies of physical activity and COVID-19 during the pandemic: a scoping review, J. Phys. Act. Health, 17 (2020), 1275–1284. https://doi.org/10.1123/jpah.2020-0406 doi: 10.1123/jpah.2020-0406
![]() |
[17] |
M. Pascoe, A. P. Bailey, M. Craike, T. Carter, R. Patten, N. Stepto, et al., Physical activity and exercise in youth mental health promotion: A scoping review, BMJ Open Sport Exercise Med., 6 (2020), e000677. https://doi.org/10.1136/bmjsem-2019-000677 doi: 10.1136/bmjsem-2019-000677
![]() |
[18] |
G. Maugeri, P. Castrogiovanni, G. Battaglia, R. Pippi, V. D'Agata, A. Palma, et al., The impact of physical activity on psychological health during Covid-19 pandemic in Italy, Heliyon, 6 (2020), e04315. https://doi.org/10.1016/j.heliyon.2020.e04315 doi: 10.1016/j.heliyon.2020.e04315
![]() |
[19] |
S. Stockwell, M. Trott, M. Tully, J. Shint, Y. Barnet, L. Butler, et al., Changes in physical activity and sedentary behaviours from before to during the COVID-19 pandemic lockdown: a systematic review, BMJ Open Sport Exercise Med., 7 (2021), e000960. https://doi.org/10.1136/bmjsem-2020-000960 doi: 10.1136/bmjsem-2020-000960
![]() |
[20] |
M. J. Savage, R. James, D. Magistro, J. Donaldson, L. C. Healy, M. Nevill, et al., Mental health and movement behaviour during the COVID-19 pandemic in UK university students: Prospective cohort study, Mental Health Phys. Act., 19 (2020), 100357. https://doi.org/10.1016/j.mhpa.2020.100357 doi: 10.1016/j.mhpa.2020.100357
![]() |
[21] |
B. Sañudo, C. Fennell, A. J. Sánchez-Oliver, Objectively-assessed physical activity, sedentary behavior, smartphone use, and sleep patterns pre-and during-COVID-19 quarantine in young adults from Spain, Sustainability, 12 (2020), 5890. https://doi.org/10.3390/su12155890 doi: 10.3390/su12155890
![]() |
[22] |
H. A. Alzamil, M. A. Alhakbany, N. A. Alfadda, S. M. Almusallam, H. M. Al-Hazzaa, A profile of physical activity, sedentary behaviors, sleep, and dietary habits of Saudi college female students, J. Fam. Community Med., 26 (2019), 1. https://doi.org/10.4103/jfcm.JFCM_58_18 doi: 10.4103/jfcm.JFCM_58_18
![]() |
[23] |
S. Panahi, A. Tremblay, Sedentariness and health: is sedentary behavior more than just physical inactivity, Front. Public Health, 6 (2018), 258. https://doi.org/10.3389/fpubh.2018.00258 doi: 10.3389/fpubh.2018.00258
![]() |
[24] |
Ó. Martínez-de-Quel, D. Suárez-Iglesias, M. López-Flores, C. A. Pérez, Physical activity, dietary habits and sleep quality before and during COVID-19 lockdown: A longitudinal study, Appetite, 158 (2021), 105019. https://doi.org/10.1016/j.appet.2020.105019 doi: 10.1016/j.appet.2020.105019
![]() |
[25] |
P. J. Puccinelli, T. S. da Costa, A. Seffrin, C. A. B. de Lira, R. L. Vancini, P. T. Nikolaidis, et al., Reduced level of physical activity during COVID-19 pandemic is associated with depression and anxiety levels: an internet-based survey, BMC Public Health, 21 (2021), 1–11. https://doi.org/10.1186/s12889-021-10684-1 doi: 10.1186/s12889-020-10013-y
![]() |
[26] |
J. Zhou, D. Zhang, W. Ren, W. Zhang, Auto color correction of underwater images utilizing depth information, IEEE Geosci. Remote Sens. Lett., 19 (2022), 1–5. https://doi.org/10.1109/LGRS.2022.3170702 doi: 10.1109/LGRS.2022.3170702
![]() |
[27] |
Y. Sun, J. Xu, H. Wu, G. Lin, S. Mumtaz, Deep learning based semi-supervised control for vertical security of maglev vehicle with guaranteed bounded airgap, IEEE Trans. Intell. Transp. Syst., 22 (2021), 4431–4442. https://doi.org/10.1109/TITS.2020.3045319 doi: 10.1109/TITS.2020.3045319
![]() |
1. | Yunus Emre Kundakcı, Sultan Karaman, Medine Sıla Ateş, Physical activity, leisure-time management, perceived barriers to physical activity and mental well-being among Turkish university students, 2024, 4, 2731-4383, 10.1007/s44192-024-00109-x | |
2. | Chao Wang, 2024, Student Physical Health Assessment Based on Puzzle Optimization Algorithm with Artificial Neural Network, 979-8-3503-7289-2, 1, 10.1109/NMITCON62075.2024.10699287 | |
3. | Andrii Chernozub, Eduard Syvokhop, Oleksandr Тymochko, Сучасні проблеми моделювання, контролю та корекції системи фізичного виховання студентів із гіпокінезією, 2024, 2410-2156, 30, 10.29038/2220-7481-2024-02-30-38 | |
4. | Guoyuan Huang, Yang Chen, ByungChan Lee, Yipeng Qiu, Aqiang Mao, Maolong Liang, Maojie Liu, A study on the effects of modified sprint interval trainingon physical fitness test scores and the quantitative and dose-response relationships among Chinese male university students, 2025, 16, 1664-042X, 10.3389/fphys.2025.1555019 |
Indicators | Indicators |
BMI < 18.5 | Underweight |
18.5 ≤ BMI < 23 | Standard weight |
23 ≤ BMI < 25 | Overweight |
25 ≤ BMI < 30 | degree Ⅰ |
BMI ≥ 30 | degree Ⅱ |
Grade | 2012 | 2011 | 2010 | M±SD |
Male students | 21.8 ± 22 | 21.4 ± 2.3 | 22.9 ± 1.6 | 23 ± 18 |
Female | 18.2 ± 1.2 | 20.5 ± 1.2 | 21.6 ± 2.2 | 20.5 ± 3.0 |
Average value | 19.5 ± 2.8 | 21.2 ± 3.5 | 22.6 ± 2.2 | 21.5 ± 2.6 |
Grade | 2012 | 2011 | 2010 | M±SD |
Male students | 21.8 ± 22 | 21.4 ± 2.3 | 22.9 ± 1.6 | 23 ± 18 |
Female | 18.2 ± 1.2 | 20.5 ± 1.2 | 21.6 ± 2.2 | 20.5 ± 3.0 |
Average value | 19.5 ± 2.8 | 21.2 ± 3.5 | 22.6 ± 2.2 | 21.5 ± 2.6 |
Indicators | Indicators |
BMI < 18.5 | Underweight |
18.5 ≤ BMI < 23 | Standard weight |
23 ≤ BMI < 25 | Overweight |
25 ≤ BMI < 30 | degree Ⅰ |
BMI ≥ 30 | degree Ⅱ |
Grade | 2012 | 2011 | 2010 | M±SD |
Male students | 21.8 ± 22 | 21.4 ± 2.3 | 22.9 ± 1.6 | 23 ± 18 |
Female | 18.2 ± 1.2 | 20.5 ± 1.2 | 21.6 ± 2.2 | 20.5 ± 3.0 |
Average value | 19.5 ± 2.8 | 21.2 ± 3.5 | 22.6 ± 2.2 | 21.5 ± 2.6 |
Grade | 2012 | 2011 | 2010 | M±SD |
Male students | 21.8 ± 22 | 21.4 ± 2.3 | 22.9 ± 1.6 | 23 ± 18 |
Female | 18.2 ± 1.2 | 20.5 ± 1.2 | 21.6 ± 2.2 | 20.5 ± 3.0 |
Average value | 19.5 ± 2.8 | 21.2 ± 3.5 | 22.6 ± 2.2 | 21.5 ± 2.6 |