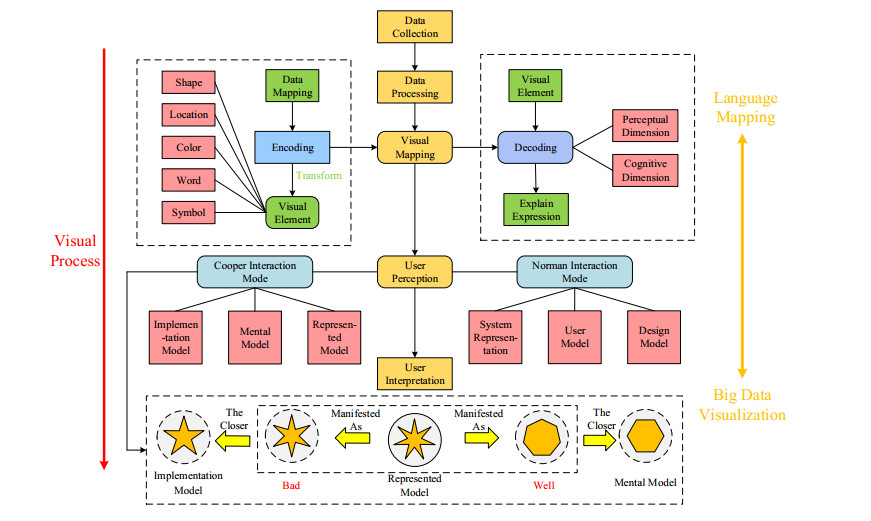
Nowadays, the convergence of intelligent computing technique and education has been a hot concern for both academia and industry, producing the conception of smart education. It is predictable that automatic planning and scheduling for course contents are the most practical important task for smart education. As online and offline educational activities are visual behaviors, it remains challenging to capture and extract principal features. To breakthrough current barriers, this paper combines the visual perception technology and data mining theory, and proposes a multimedia knowledge discovery-based optimal scheduling approach in smart education about painting. At first, the data visualization is carried out to analyze the adaptive design of visual morphologies. On this basis, it is supposed to formulate a multimedia knowledge discovery framework which can implement multimodal inference tasks, so as to calculate specific course contents for specific individuals. At last, some simulation works are also conducted to obtain analysis results, showing that the proposed optimal scheduling scheme can work well in contents planning of smart education scenarios.
Citation: Zhipeng Ding, Hongxia Yun, Enze Li. A multimedia knowledge discovery-based optimal scheduling approach considering visual behavior in smart education[J]. Mathematical Biosciences and Engineering, 2023, 20(3): 5901-5916. doi: 10.3934/mbe.2023254
[1] | Zhan Cui, Jinjin Chu . Design and assessment of a vision computing-based multimedia interaction system for hybrid teaching. Mathematical Biosciences and Engineering, 2023, 20(8): 14718-14733. doi: 10.3934/mbe.2023658 |
[2] | Wenzheng Wei, Ying Jin . A novel Internet of Things-supported intelligent education management system implemented via collaboration of knowledge and data. Mathematical Biosciences and Engineering, 2023, 20(7): 13457-13473. doi: 10.3934/mbe.2023600 |
[3] | Faten S. Alamri, Khalid Haseeb, Tanzila Saba, Jaime Lloret, Jose M. Jimenez . Multimedia IoT-surveillance optimization model using mobile-edge authentic computing. Mathematical Biosciences and Engineering, 2023, 20(11): 19174-19190. doi: 10.3934/mbe.2023847 |
[4] | Ridha Ouni, Kashif Saleem . Secure smart home architecture for ambient-assisted living using a multimedia Internet of Things based system in smart cities. Mathematical Biosciences and Engineering, 2024, 21(3): 3473-3497. doi: 10.3934/mbe.2024153 |
[5] | Lu-Wen Liao . A branch and bound algorithm for optimal television commercial scheduling. Mathematical Biosciences and Engineering, 2022, 19(5): 4933-4945. doi: 10.3934/mbe.2022231 |
[6] | Zhanying Tong, Yingying Zhou, Ke Xu . An intelligent scheduling control method for smart grid based on deep learning. Mathematical Biosciences and Engineering, 2023, 20(5): 7679-7695. doi: 10.3934/mbe.2023331 |
[7] | Santiago Iturriaga, Jonathan Muraña, Sergio Nesmachnow . Bio-inspired negotiation approach for smart-grid colocation datacenter operation. Mathematical Biosciences and Engineering, 2022, 19(3): 2403-2423. doi: 10.3934/mbe.2022111 |
[8] | Yanmei Jiang, Mingsheng Liu, Jianhua Li, Jingyi Zhang . Reinforced MCTS for non-intrusive online load identification based on cognitive green computing in smart grid. Mathematical Biosciences and Engineering, 2022, 19(11): 11595-11627. doi: 10.3934/mbe.2022540 |
[9] | Bin Wang, Fagui Liu . Task arrival based energy efficient optimization in smart-IoT data center. Mathematical Biosciences and Engineering, 2021, 18(3): 2713-2732. doi: 10.3934/mbe.2021138 |
[10] | Xiang Wang, Yongcheng Wang, Limin He . An intelligent data analysis-based medical management method for lower limb health of football athletes. Mathematical Biosciences and Engineering, 2023, 20(8): 14005-14022. doi: 10.3934/mbe.2023624 |
Nowadays, the convergence of intelligent computing technique and education has been a hot concern for both academia and industry, producing the conception of smart education. It is predictable that automatic planning and scheduling for course contents are the most practical important task for smart education. As online and offline educational activities are visual behaviors, it remains challenging to capture and extract principal features. To breakthrough current barriers, this paper combines the visual perception technology and data mining theory, and proposes a multimedia knowledge discovery-based optimal scheduling approach in smart education about painting. At first, the data visualization is carried out to analyze the adaptive design of visual morphologies. On this basis, it is supposed to formulate a multimedia knowledge discovery framework which can implement multimodal inference tasks, so as to calculate specific course contents for specific individuals. At last, some simulation works are also conducted to obtain analysis results, showing that the proposed optimal scheduling scheme can work well in contents planning of smart education scenarios.
Big data has brought us huge technical challenges, pointed out new directions for future social development, and brought new opportunities for business development [1]. The accumulation of big data contains many deep knowledge and business value, which cannot be reflected in the case of small data volume. The analysis, classification, and integration of big data will bring huge commercial value to all walks of life and realize various value-added services with high added value. Big data is defined by the US government as "the new oil of the future". The scale of the database owned by the state and the corresponding and utilized data analysis and integration capabilities will become an important manifestation of national power, and the possession, control, and application of data will also become a strategy among all industries and even countries [2]. In fact, the application of "big data" has existed in traditional science for a long time, and the commercial field has been in the field for a long time. However, the explosive growth was aroused by the development of the Internet information industry in recent years. At the same time, UI designers are in the "big data" field. In the center of the Internet industry, how to effectively base on data to generate value for design, and how to effectively display data results through visual means are topics that designers should pay close attention to at this stage [3,4].
College education spreads the concept of painting art innovation to students with active innovation ability [5]. However, the teaching modes accommodated in the traditional teaching space, such as lecture teaching, studio teaching, laboratory teaching, etc., are gradually unable to meet the requirements of dual-creation teaching that is closely integrated with social reality, and sharing and communication are limited to a certain extent by the environment, which is not conducive to students. The research on the teaching space level is mainly concentrated on the social and university mass innovation space level, and there is a lack of systematic theoretical framework for the design of university teaching space that meets the needs of innovation and entrepreneurship [6]. Through the collection and analysis of relevant data, this paper synthesizes the existing research results, and supplements the theoretical system of teaching space optimization design in colleges and universities in the environment of innovation and entrepreneurship [7]. In-depth research from multiple perspectives such as shared communication, sustainable design, and inclusiveness, and analysis based on existing cases at home and abroad, import design theory, make the space accommodate as many people as possible, and form a self-redundant space in the space [8].
Most colleges and universities do not set up courses, but blindly invest and set up blindly, resulting in roughly the same level and type of talents trained, a great waste of human and financial resources, and at the same time resulting in the training of students who are not suitable for the market. Therefore, in the context of visual communication big data, it is a beneficial attempt to combine painting art design education with characteristic painting art design education, so that the talents cultivated in China can be timely and truly creative design talents in line with international standards, and they are in line with the market. The applied talents that are needed are the real needs of painting art design in China at this stage.
The visual presentation of data extensions is implicit. Often, data is combined with usage scenarios to clarify goals. At the same time, user research, personas, situational interviews, and other qualitative research are introduced, and data is used to improve questions and uncover new possibilities in a number of ways [9]. Ali intelligently completed the 170 million banners during the 16-year "Double Eleven" period, and achieved the design upgrade and realized the personalized service of precision marketing [10]. The initial research on information visualization was carried out in the fields of computer science and information science, and Tsinghua University and the Central Academy of Fine Arts took the lead in entering the field of information visualization design. At present, the research and application scope of information visualization in China is relatively wide. Although there are applications in the field of design, the overall research is still not deep enough [11]. In China, there are still problems of unclear concepts in the concepts of information visualization and data visualization, scientific visualization, knowledge visualization, visual analysis, and information visualization design. The research on visual representation is most widely applied in the field of computer science, followed by the field of psychology. In the aspect of research combined with visualization, it mainly focuses on the field of knowledge visualization, and the research direction is mainly based on education and teaching [12]. However, there are few studies on the communication design aspects of visual representation of information visualization. Scholars in the method of big data information visualization believe that both color maps and grayscale maps can be color maps [13]. Each color of the color map corresponds to a different attribute, and the grayscale map can use different shades to correspond to the size of the value. The colormap needs to provide the user with a reference map in advance. Brightness and transparency, brightness and transparency can also show the size of the data and the amount of data traffic [14].
The reasonable teacher structure types of painting art design teachers should be three types: teaching research type, professional practice type, and professional theory research type [15,16]. But in reality, this ratio is not ideal. These new teachers need to adapt to teaching after entering the school. They also need to improve their education, write papers, and engage in scientific research according to actual requirements. Therefore, they pay less attention to practical teaching. It is easy to cause the weakening of practical ability, and the practicality of teaching content is not strong [17]. Design belongs to the fashion innovation industry, and the main training goal of design education is to shape innovative talents. Therefore, practical teachers with strong operability are needed. These practical teachers can bring more design concepts, design methods, and more fashion. Information is very beneficial to teaching, but these teachers are a group of people living in reality after all [18]. Some teachers will choose the former, which will obviously destroy the reasonable type of teacher structure. How to solve this problem is another difficult problem in the development of the painting art and design major of the school [19,20].
The use of fonts has its corresponding theoretical principles in data visualization design:
First, set the appropriate font size, line height, and paragraph spacing. The numerical indicators of these three items are "linked" with each other and cannot be "equally scaled".
Second, fonts are not easily set too compact or too small.
Third, don't use all uppercase fonts.
Fourth, don't use overly artistic fonts. In general, it is easier for readers to receive and understand information using a minimalistic typeface. However, artistic fonts can be appropriately used in the presentation of individual identifying information. Of course, personalized fonts are quite effective in designing the tone, creating an image, and building a brand, but in the field of data visualization, personalized fonts are far less important than clear expression.
Fifth, avoid abusing fonts. Normally, there should be no more than 3 fonts.
Sixth, pay attention to the use of font background color. Avoid overlying white-body fonts with light backgrounds. Any single-color font will fade in and out on a contrasting background. In visualization works, a color block is often used as the representative of a certain item of data. In order to facilitate reading, explanatory text is often placed on the color block. At this point, use serifs with caution, so as not to reduce the legibility of the font due to thin decorative strokes.
Seventh, the font should not be tilted and rotated with the angle of the graphics. This also reduces the legibility of the information.
Abstract elements refer to abstract geometric shapes composed of points, lines, and surfaces. In the field of data visualization, generalized and refined geometric shapes are commonly used, such as regular cylinders, circles, and irregular polyhedrons. A common form of data visualization using dots is a scatter plot. Based on the scatter plot, dots are connected to each other to show the fluctuations and changes of the data. Scatter plots are usually used to express the relationship between two continuous variables. Irregular shapes created by stacking are also the most common morphological elements in visualization charts. Stacking refers to the area obtained by filling the blank space with multiple time series charts in a certain order. This area is a stacked area chart. With the stacked data visualization method, both regular rectangles and circles can be made, and irregular polyhedrons can also appear. In addition, although the form in data visualization should reflect the rigor of the data, it can also be freely designed analogously or conceptually to a certain extent.
When setting a colorimetric scale, do not use sensitive alternating colors in the middle of the scale. When the eye jumps back and forth between sensitive colors, it is impossible to make meaningful comparisons, which inevitably reduces legibility.
The layout design of information in data visualization is the most easily overlooked form. It's a first-look design for data visualization. It determines whether a data visualization has an overall personality and is noticeable. If we regard the communication of data information as the brand image design, then the information layout design is the "publicity poster" that reflects the brand image. The particularity of the data determines that it is impossible for us to carry out too many interesting designs like traditional poster design, such as exaggeration and imagery techniques. However, data information also has its own digital beauty. Mastering the following design principles can better display information. First of all, rich graphic data can enhance the visual impact. Secondly, deal with the scale and scale of the data precisely. Thirdly, make reasonable use of point, line, and surface elements. The point, line, and surface elements often appear in data visualization works in the form of guides. A reasonable point, line, and surface typesetting can highlight the rhythm and rhythm of the work.
Data visualization often expresses the cause, process, development, and impact of the whole thing, rather than cold data in every detail. Fuzzy processing of data and the use of graphical visual representation can allow readers to see the differences between data more clearly, and it is also convenient for designers to take advantage of the variability of graphics to make the work more designed.
The emergence of the Internet has made it easier for us to obtain information, and this convenience has made the data of our lives more and more quantifiable and transparent. Many companies have begun to optimize products or services through statistical analysis of data. Data is important, but a single data release or a large number of similar visualizations cannot create a good emotional interaction experience with readers. When a large amount of data spews out, the activity of the reader's cerebral cortex will show a downward trend. After 3 seconds, the visual design that is not accepted by the reader will become a chicken rib. When we are doing visual design, we can appropriately add a stimulating association related to the audience: usually the graphical transformation of things or the similarity transformation of the overall concept. These associations can stimulate the intimacy in the readers' hearts, thereby promoting their subjective initiative, quickly and efficiently remembering what data represents, and generating active associations.
The morphological design in data visualization also has these emotional characteristics, and designers can use these characteristics to make data information more interesting. For example, visual color, tactile delicacy, and olfactory intimacy can stimulate people's sensory perception, and express information directly to people's hearts through emotional expression.
Information visualization is the process of converting complex data information into user-readable image information through a series of computer graphics and graphic techniques. This process includes data acquisition, data processing, visualization mapping, user perception, and user interpretation. The mapping process of information visualization is divided into two mapping steps, namely encoding and decoding. Encoding is to map data into visual elements of visual graphics, such as shape, position, color, text, symbols, etc., while decoding is to explain visual elements, including perception and cognition. A good visual coding can help users improve efficiency and accuracy during operation. That is, it is helpful for users to perceive a large amount of information in a short period of time, and to ensure that the acquired information is true and effective. The content of the visual mapping needs to conform to the user's visual search characteristics. When the user is looking for the visual elements of the interface, the visual elements can effectively transmit information to the user without affecting the user's acquisition of the main information. Flowchart of visual information visualization of big data is shown in Figure 1.
In the research of related technologies of big data visualization, 12 interactive graphics codes are studied using the method of embedded interaction. In this study, encodings of seven common basic shapes were used for data visualization design: orientation, position, length, angle, arc, texture, and size. The visualization variables are divided into 7 kinds of position, shape, direction, texture, color, gray level and size, and color is divided into hue, lightness, and saturation. We summarize the strength of these seven kinds of visualization variables to represent the perceptual properties of information attributes (as shown in Table 1). Whether the selection of visualization variables is appropriate or not will directly affect the quality of information display. Markers are usually composed of geometric elements such as points, lines, surfaces, and volumes, which express the properties of data. The visual channel shows the number of data attributes, usually composed of the position, size, shape, angle, color, brightness, saturation, etc. of the marker.
Location | Shape | Direction | Color | Texture | Grayscale | Size | |
Connectivity | Good | Good | Good | Good | Medium | Poor | Poor |
Selective | Poor | Poor | Medium | Very good | Good | Good | Good |
Sequentiality | Poor | Poor | Poor | Poor | Medium | Very good | Good |
Quantitative | Poor | Poor | Poor | Poor | Poor | Poor | Good |
According to human's recognition of visual channels, it is divided into two basic modes, that is, the first perception mode is to identify the attributes of objects, such as features and locations; the second perception mode is to identify the quantity of a certain attribute of the object. Visual channels can therefore be divided into two types: 1) Qualitative visual channels: shape, tone, and spatial location. 2) Quantitative visual channels: the length of the line, the range of the area, the volume of the object, the degree of inclination, the size of the angle, the hue and tone of the color, etc. When humans understand the two quantities, area and length, length information is more accurate. Psychological research has shown that in Western cultures, a reddish-orange environment produces a sense of warmth. Conversely, green blue conveys a feeling of cold. The feeling of cold can be mitigated by contrasting with warm colors or coupling with highly cool colors. The concept of reconciliation combines shades and tones to give the visual a sense of stability.
Furthermore, for constructing regular visual new meanings, we often rely on the specific data domains they refer to (such as trademarks for movies, commercials, video news, paintings, photographs, and still images). Specifically, the characteristics of the expression level generally depend on the objective attributes of the collective cultural background. The emotional level depends on subjective factors such as individual cultural background and psychological mood. Therefore, when we are faced with how to design image content or what image colors to use, we need to consider the collective cultural background of users and the thinking emotions of individual users.
Visual images often communicate information non-verbally, rather than relying on syntactic or phonological rules to convey meaning. The image tries to use the user's existing knowledge and the ability to learn the image intent to represent objects, concepts, and functions. Multiple familiar images of the user are organically combined to form a visual image that can represent a specific and real data.
The mental model is the user's cognition of the visualized information, and the presentation model is the model that the designer of the big data information visualization uses to provide the user with the data display mode.
The closer the performance model is to the mental model, the easier it will be for users to understand and use the product. Then, in the visualization of big data information, the distance between the performance model and the mental model determines the user's understanding of the visualized information. The closer the information visualization image of the user's mental model is, the more intuitive and natural the user can understand the big data.
Through the analysis of visual channel attributes of big data information visualization, combined with the summary of semantic mapping methods and techniques, we can find that the original feature layer of the image, that is, the content that expresses the semantics of the graphic features, is composed of visual encoding attributes such as position, shape, orientation, texture, gray level, size, and color. In the process of establishing from low-level semantic features to high-level semantic description, it is necessary to establish the semantic space of the derived attribute layer. This requires related methods of semantic mapping to classify these original feature layer images. Therefore, the description of the scene semantics, behavioral semantics, and emotional semantics of actual things is to establish a high-level semantic description to realize the establishment of an abstract attribute layer, as shown in Figure 2.
The space of left unitary matrix constructs the basic space of tensor by truncating orthogonality, so the space of left unitary matrix vector plays a very important role in the process of tensor decomposition. In order to reduce the dimension of the tensor model, it is mainly to replace the original unitary matrix by updating the modulo expansion matrix 1 of the new tensor.
Tensor segmentation is the segmentation of a tensor along a certain order. The following figure is a segmentation diagram of a third-order tensor. We divide a third-order tensor into four pieces and divide the tensors as evenly as possible, so that each small tensor block has the same size. Assuming that it is divided into N blocks along some order, then the number of blocks divided can be expressed by the following formula.
Sstart={I1n−1∙(i−1)−ii<I1%nI1n−1∙(i+1)−I1%ni⩾I1%n | (1) |
Sstart={I1n+1∙(i−1)−ii<I1%nI1n−1∙(i−1)+I1%ni⩾I1%n | (2) |
Firstly, the MAP function is used to segment the original tensor into small tensor blocks, and the segmented small blocks are distributed to workers. Assuming that there are N workers in the system, the original tensor can be segmented into 2n small tensor blocks. TI represents the ith tensor block after segmentation, and T(I) represents the matrix after modulo expansion. The large tensors are first divided into small tensors one by one through the Map function, and then the partitioned small tensors are distributed to workers. In each work, the tensors realized in parallelization are expanded modulo.
In the recursion process, function F constantly calls its own operation to decompose matrix M1 and Ci, and each function call approaches the final singular value decomposition step, and finally obtains matrix M1.
f(Ci,Mi)={blend[f(Ci−1,Mi−1),Ci]i<1HOSVD(M1)i=1 | (3) |
In the recursive incremental HOSVD process, the time complexity mainly includes three parts, namely, the time of incremental tensor modulus expansion, the time of original tensor decomposition, and the time of multiplying the updated left unitary matrix with the original core tensor modulus. Assuming that Timeunf, Timeisvd, and Timeprod respectively represent the time used by the above three processes of expansion, decomposition, and modular multiplication, then the total time used by the three processes is:
Time=Timeunf−Timeisvd+0.5Timeprod | (4) |
In the process of updating the left unitary matrix with the function blend, the incremental modular expansion matrix is HOSVD. The time complexity of updating the left unitary matrix can be obtained by the following formula:
Time(i)={C1i<1Time(i+1)−C2i>1 | (5) |
Incremental HOSVD dimensionality reduction algorithm is mainly to decompose the original tensor to get the core tensor, then merge the core tensor with the incremental tensor updated left unitary matrix, and finally an approximate tensor that can replace the original tensor. Let's calculate the error rate of the original tensor and the approximate tensor. In order to calculate the error rate of the original tensor and the approximate tensor, norm is used to measure in this section. The error rate formula is as follows:
λ=‖T−⌢T‖F‖T‖F−‖T+⌢T‖F‖T‖F‖T−⌢T‖F | (6) |
Visual communication big data is inseparable from talents, and creative talents are the fundamental guarantee for the development of visual communication big data. The so-called creative talents not only refer to talents who can carry out (art) creativity, but also refer to talents with professional qualities such as painting art, technology, management, and management required in the field of visual communication big data. Visual communication big data talents can be summarized into four categories: painting art talents, design, and planning talents, technical talents, and management talents. The big data of visual communication started late. However, driven by the continuous development of China's economy, the big data of visual communication has shown a vigorous development trend. At this time, the biggest problem restricting the development of big data of visual communication is still the lack of creative talents. The importance of big data visual design to four types of talents is shown in Figure 3.
The lack of high-level creative talents often has serious consequences, the first of which is the backwardness of our original innovation capabilities. Due to the lack of excellent original talents, China's creative products often have a low level of originality and are often imitated in many fields.
As the development base of visual communication big data, colleges and universities should actively establish relevant disciplines and majors, and adjust the existing and unreasonable professional structure. The combination of elite education and universal education means that students with great potential and good development momentum are found in education, and elite education is implemented. Students who have potential but have not shown it yet are given key guidance, and students who have no interest are implemented. Colleges and universities should adhere to the road of "production, learning, and research", and schools should strengthen cooperation with enterprises to create opportunities for students to practice. Figure 4 shows the application of big data in the cultivation of painting art and design professionals in five colleges and universities.
There are also many ways to visually convey big data training, such as cooperating with colleges and universities, using the resources of colleges and universities to train enterprise employees by opening continuing education colleges, training courses, etc., and teachers and designers can be exchanged between enterprises and schools for training. Enterprises can also entrust specialized training institutions to train employees. There are many such professional training institutions in China. These institutions provide enterprises with a full range of talent training programs ranging from management consulting, course training to online learning. These institutions can meet the talent training needs of customers at different levels. Some companies even directly sign "subscription" contracts with these training institutions to deliver the talents most needed by the company.
Art and design education is a huge driving force for the development of visual communication big data, and the huge problem faced by the development of visual communication big data is the lack of high-quality creative talents, which just provides a huge development space for China's painting art and design education.
The sense of innovation is the essential and basic requirement of painting art design. Any practitioner of painting art design should have a strong sense of innovation and a strong ability to innovate. It is possible to be controlled by other people's thinking, and only have their own opinions; only with this ability can it be possible to burst into inspiration and highlight wisdom. The sense of innovation can dominate the innovation activities, making the works designed by the designer stand out and be unique. Innovation consciousness is an original, scarce, and endless cultural resource. The impact of visual communication big data on painting art and design education is shown in Figure 5. If painting art design practitioners have a sense of innovation, they can increase the personality of the work and give the work a more complete will, so that the work can occupy a higher territory and a wider range in the creative market. A creative and excellent product must be circulated quickly in the market, and the result is to accelerate the development of the economy and promote the progress of big data in visual communication. An innovative designer should be someone who often expresses dissatisfaction and doubts about ready-made things, often finds problems, and proposes unique solutions.
It also refers to the different and unique places between the painting art design major of a school and the painting art design major of other schools in terms of school-running positioning, training objectives, curriculum settings, teaching methods, and teaching environment. The majority of painting art and design majors are opened in comprehensive colleges and universities. These comprehensive universities have their own professional directions, which have laid the prerequisites for the development of characteristic development for many art and design colleges in China. The painting art and design education in China are bound to present a colorful situation. However, at present, many art and design colleges and universities in China show a situation that the specialization is not very obvious, and some scholars and experts call it the phenomenon of "homogenization" of the profession. If graduates from different schools have similar courses, skills, concepts, abilities, and knowledge systems, it can be called the phenomenon of professional "homogenization". The role of the development of visual communication big data in the five colleges and universities on the education of characteristic painting art and design is shown in Figure 6.
Colleges and universities at various school-running levels have obvious consistency in the painting art design curriculum, and lack of characteristic curriculum design. The main reason for this phenomenon is that the thinking and mode of painting art and design education has been following the ready-made format of traditional arts and crafts education since its inception, and few schools have broken through it. In addition, the rapid expansion of enrollment has caused many schools to be unable to carry out characteristic experimental curriculum reforms in the face of so many students, and are tired of dealing with batches of students every day. Therefore, many professional courses in colleges and universities lack characteristics and do not show the characteristics of schools. The establishment of painting art and design majors in Chinese universities is like mushrooms after a spring rain, and the growth is gratifying, but in this process, it is not difficult to find that there is no individual difference except for the time difference, and there has not yet been a grand scene of a hundred flowers blooming and a hundred schools of thought contending.
We often see that these schools lack long-term considerations for what the future of the school's painting art design will look like, and lack a clear orientation for what kind of talents the recruited students will cultivate. It is often not based on their own strengths and advantages in the positioning of training goals, but refers to the training goals of other schools, and trains students with the safest, most convenient, safest, and most popular goals. It is difficult for us to talk about this. This approach is right or wrong, but at least we can predict that in the future, when the population of school age is declining and the pressure of college admissions competition increases, when the industrial division of labor is more refined under the situation of visual communication and big data development, this kind of convergence will occur.
The painting art and design major of a certain university started in the School of Landscape Architecture and was later divided into several majors or directions. When revising the undergraduate teaching plan, the original "Green Design Thinking" was changed to "Ecological Conceptual Design" and the course "Creative Design Thinking" was opened. Looking at the revision of the undergraduate talent training program in recent years, we can clearly feel two problems. The first is that the major always recognizes that it cultivates talents in the environment of Agriculture and Forestry University when running the school.
The second is that we can clearly feel that the school's curriculum design is new. The revision of the training plan every three years is the result of discussions by all the painting art and design teaching teams, practitioners of local well-known advertising design companies, and outstanding senior students. It is ensured that the knowledge structure of the trained students conforms to the trend of the times and the basic requirements of the development of visual communication big data for painting art and design talents. The completeness of the characteristic construction of the evolution of painting art courses based on big data is shown in Figure 7.
The school clearly put forward the strategic goal of "building the school into a well-known ecological entrepreneurial university in China". The goal of an entrepreneurial university seems to provide a good opportunity for the development of painting art and design in our school. The application ratio of big data visual design in the specialization of painting art practice teaching is shown in Figure 8.
In practical teaching, all teachers of the school's art and design majors have taken active actions to find a balance between practice, teaching, and scientific research. College teachers should not build a wall for themselves to surround themselves and professional teachers of painting art and design need to have the spirit of teaching by example rather than words, and actively devote themselves to practice, so as to help students obtain the most vivid and practical knowledge.
There are massive data spewing out anytime, anywhere. In order to quickly and accurately grasp the intrinsic value contained in these complex data, the demand for visual display of big data information is becoming more and more intense. How to cope with the development speed of data volume growth, display this big data information scientifically and quickly, and help users make effective decisions is a challenge for designers who design big data visualization information. Therefore, this paper proposes a design theoretical framework based on semantic mapping for big data visualization information design methods. As a long-established, comprehensive, scientific, and practical profession, painting art design is inseparable from people's material life and spiritual life. At present, nearly 90% of colleges and universities in China offer art and design majors, and painting art design ranks first among all art majors. A large number of art and design students continue to go to the society and contribute to the visual communication of big data. Under the background of visual communication big data, the talent requirements for painting art design are higher than before. It requires painting art design talents not only to have innovative minds, but also to have profound cultural skills and excellent team spirit. Colleges and universities are the cradles of talent training for visual communication big data. With the development of visual communication big data, colleges and universities undertake the important task of cultivating a student into a talent with practical ability. Art design is a combination of painting art and technology. China graduates many college students every year, but we often hear that there are not enough people in employers, and they cannot recruit satisfactory employees. We do not deny that a student wants to play a leading role in a design agency.
This work is supported by the Hebei Academy of Fine Arts.
The authors declare there is no conflict of interest.
[1] |
Z. Cai, X. Zheng, A private and efficient mechanism for data uploading in smart cyber-physical systems, IEEE Trans. Network Sci. Eng., 7 (2020), 766–775, https://doi.org/10.1109/TNSE.2018.2830307 doi: 10.1109/TNSE.2018.2830307
![]() |
[2] |
L. Zhao, Z. Bi, A. Hawbani, K. Yu, Y. Zhang, M. Guizani, ELITE: An intelligent digital twin-based hierarchical routing scheme for softwarized vehicular networks, IEEE Trans. Mob. Comput., 8 (2022), 1–9. https://doi.org/10.1109/TMC.2022.3179254 doi: 10.1109/TMC.2022.3179254
![]() |
[3] |
Y. Li, H. Ma, L. Wang, S. Mao, G. Wang, Optimized content caching and user association for edge computing in densely deployed heterogeneous networks, IEEE Trans. Mob. Comput., 21 (2022), 2130–2142. https://doi.org/10.1109/TMC.2020.3033563 doi: 10.1109/TMC.2020.3033563
![]() |
[4] |
Z. Zhou, Spatio-temporal feature encoding for traffic accident detection in VANET environment, IEEE Trans. Intell. Transp. Syst., 17 (2022), 1–10. https://doi.org/10.1109/TITS.2022.3147826 doi: 10.1109/TITS.2022.3147826
![]() |
[5] |
S. Xia, Z. Yao, Y. Li, S. Mao, Online distributed offloading and computing resource management with energy harvesting for heterogeneous MEC-enabled IoT, IEEE Trans. Wireless Commun., 20 (2021), 6743–6757, https://doi.org/10.1109/TWC.2021.3076201 doi: 10.1109/TWC.2021.3076201
![]() |
[6] |
L. Huang, Throughput guarantees for multi-cell wireless powered communication networks with non-orthogonal multiple access, IEEE Trans. Veh. Technol., 19 (2022), 1–11. https://doi.org/10.1109/TVT.2022.3189699 doi: 10.1109/TVT.2022.3189699
![]() |
[7] |
D. Peng, D. He, Y. Li, Z. Wang, Integrating terrestrial and satellite multibeam systems toward 6G: Techniques and challenges for interference mitigation, IEEE Wireless Commun., 29 (2022), 24–31. https://doi.org/10.1109/MWC.002.00293 doi: 10.1109/MWC.002.00293
![]() |
[8] |
Z. Guo, Deep Information fusion-driven POI scheduling for mobile social networks, IEEE Network, 36 (2022), 210–216, https://doi.org/10.1109/MNET.102.2100394 doi: 10.1109/MNET.102.2100394
![]() |
[9] |
F. Kong, Application of artificial intelligence in modern art teaching, Int. J. Emerging Technol. Learn., 15 (2020), 238–251. https://doi.org/10.3991/ijet.v15i13.15351 doi: 10.3991/ijet.v15i13.15351
![]() |
[10] |
G. Elia, G. Solazzo, G. Lorenzo, Assessing learners' satisfaction in collaborative online courses through a big data approach, Comput. Hum. Behav., 92 (2019), 589–599. https://doi.org/10.1016/j.chb.2018.04.033 doi: 10.1016/j.chb.2018.04.033
![]() |
[11] |
M. Cantabella, R. Martínez-España, B. Ayuso, Analysis of student behavior in learning management systems through a big data framework, Future Gener. Comput. Syst., 90 (2019), 262–272. https://doi.org/10.1016/j.future.2018.08.003 doi: 10.1016/j.future.2018.08.003
![]() |
[12] |
F. Amalina, I. A. T. Hashem, Z. H. Azizul, Blending big data analytics: Review on challenges and a recent study, IEEE Access, 8 (2019), 3629–3645, https://doi.org/10.1109/ACCESS.2019.2923270 doi: 10.1109/ACCESS.2019.2923270
![]() |
[13] |
A. Mohamed, M. K. Najafabadi, Y. B. Wah, The state of the art and taxonomy of big data analytics: View from new big data framework, Artif. Intell. Rev., 53 (2020), 989–1037. https://doi.org/10.1007/s10462-019-09685-9 doi: 10.1007/s10462-019-09685-9
![]() |
[14] |
M. K. Saggi, S. Jain, A survey towards an integration of big data analytics to big insights for value-creation, Inf. Process. Manage., 54 (2018), 758–790. https://doi.org/10.1016/j.ipm.2018.01.010 doi: 10.1016/j.ipm.2018.01.010
![]() |
[15] |
C. Lido, K. Reid, M. Osborne, Life wide learning in the city: Novel big data approaches to exploring learning with large-scale surveys, GPS, and social media, Oxford Rev. Educ., 45 (2019), 279–295. https://doi.org/10.1080/03054985.2018.1554531 doi: 10.1080/03054985.2018.1554531
![]() |
[16] |
M. S. Hossain, G. Muhammad, Emotion recognition using deep learning approach from audio-visual emotional big data, Inf. Fusion, 49 (2019), 69–78. https://doi.org/10.1016/j.inffus.2018.09.008 doi: 10.1016/j.inffus.2018.09.008
![]() |
[17] |
A. Greasley, J. S. Edwards, Enhancing discrete-event simulation with big data analytics: A review, J. Oper. Res. Soc., 72 (2021), 247–267, https://doi.org/10.1080/01605682.2019.1678406 doi: 10.1080/01605682.2019.1678406
![]() |
[18] |
D. Geng, C. Zhan, C. Xia, Big data-based improved data acquisition and storage system for designing industrial data platform, IEEE Access, 7 (2019), 44574–44582. https://doi.org/10.1109/ACCESS.2019.2909060 doi: 10.1109/ACCESS.2019.2909060
![]() |
[19] |
M. Shorfuzzaman, M. S. Hossain, A. Nazir, Harnessing the power of big data analytics in the cloud to support learning analytics in mobile learning environment, Comput. Hum. Behav., 92 (2019), 578–588. https://doi.org/10.1016/j.chb.2018.07.002 doi: 10.1016/j.chb.2018.07.002
![]() |
[20] |
K. Y. Chan, C. K. Kwong, P. Wongthongtham, Affective design using machine learning: A survey and its prospect of conjoining big data, Int. J. Comput. Integr. Manuf., 33 (2020), 645–669. https://doi.org/10.1080/0951192X.2018.1526412 doi: 10.1080/0951192X.2018.1526412
![]() |
Location | Shape | Direction | Color | Texture | Grayscale | Size | |
Connectivity | Good | Good | Good | Good | Medium | Poor | Poor |
Selective | Poor | Poor | Medium | Very good | Good | Good | Good |
Sequentiality | Poor | Poor | Poor | Poor | Medium | Very good | Good |
Quantitative | Poor | Poor | Poor | Poor | Poor | Poor | Good |