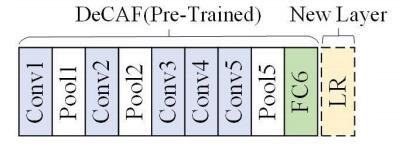
Citation: Ming Wei, Bo Sun, Wei Wu, Binbin Jing. A multiple objective optimization model for aircraft arrival and departure scheduling on multiple runways[J]. Mathematical Biosciences and Engineering, 2020, 17(5): 5545-5560. doi: 10.3934/mbe.2020298
[1] | Long Wen, Yan Dong, Liang Gao . A new ensemble residual convolutional neural network for remaining useful life estimation. Mathematical Biosciences and Engineering, 2019, 16(2): 862-880. doi: 10.3934/mbe.2019040 |
[2] | Guanghua Fu, Qingjuan Wei, Yongsheng Yang . Bearing fault diagnosis with parallel CNN and LSTM. Mathematical Biosciences and Engineering, 2024, 21(2): 2385-2406. doi: 10.3934/mbe.2024105 |
[3] | Xueyan Wang . A fuzzy neural network-based automatic fault diagnosis method for permanent magnet synchronous generators. Mathematical Biosciences and Engineering, 2023, 20(5): 8933-8953. doi: 10.3934/mbe.2023392 |
[4] | Yajing Zhou, Xinyu Long, Mingwei Sun, Zengqiang Chen . Bearing fault diagnosis based on Gramian angular field and DenseNet. Mathematical Biosciences and Engineering, 2022, 19(12): 14086-14101. doi: 10.3934/mbe.2022656 |
[5] | Kunli Zhang, Shuai Zhang, Yu Song, Linkun Cai, Bin Hu . Double decoupled network for imbalanced obstetric intelligent diagnosis. Mathematical Biosciences and Engineering, 2022, 19(10): 10006-10021. doi: 10.3934/mbe.2022467 |
[6] | Jinyi Tai, Chang Liu, Xing Wu, Jianwei Yang . Bearing fault diagnosis based on wavelet sparse convolutional network and acoustic emission compression signals. Mathematical Biosciences and Engineering, 2022, 19(8): 8057-8080. doi: 10.3934/mbe.2022377 |
[7] | Xu Zhang, Wei Huang, Jing Gao, Dapeng Wang, Changchuan Bai, Zhikui Chen . Deep sparse transfer learning for remote smart tongue diagnosis. Mathematical Biosciences and Engineering, 2021, 18(2): 1169-1186. doi: 10.3934/mbe.2021063 |
[8] | Shizhen Huang, ShaoDong Zheng, Ruiqi Chen . Multi-source transfer learning with Graph Neural Network for excellent modelling the bioactivities of ligands targeting orphan G protein-coupled receptors. Mathematical Biosciences and Engineering, 2023, 20(2): 2588-2608. doi: 10.3934/mbe.2023121 |
[9] | Jun Gao, Qian Jiang, Bo Zhou, Daozheng Chen . Convolutional neural networks for computer-aided detection or diagnosis in medical image analysis: An overview. Mathematical Biosciences and Engineering, 2019, 16(6): 6536-6561. doi: 10.3934/mbe.2019326 |
[10] | Suqi Zhang, Wenfeng Wang, Ningning Li, Ningjing Zhang . Multi-behavioral recommendation model based on dual neural networks and contrast learning. Mathematical Biosciences and Engineering, 2023, 20(11): 19209-19231. doi: 10.3934/mbe.2023849 |
Prognostic Health Management (PHM) system has become a vital part in modern industry. The goals of PHM are to reduce the risks to avoid the dangerous situations and improve the safety and reliability of the smart equipment and the systems [1]. Over the past decades, various attempts have been made to design effective methods to achieve the superior diagnosis performance. With the development of the smart manufacturing, the machines and equipment are more automatic and complicate, the intelligent fault diagnosis of these smart machines and equipment became necessary [2]. The data from the machine are boosting, and it can be collected much faster and more widely than ever before, the data-driven fault diagnosis has attracted more and more attentions from both academic and engineering fields [3].
Traditional learning-based approaches need to extract features of signals from time, frequency, and time-frequency domains [4]. The feature extraction is an essential step and the upper-bound performances of the leaning methods rely on the feature extraction process [5]. However, the traditional handcrafted feature extraction techniques need considerable domain knowledge, and the feature extraction process is very time-consuming and labor-intensive [6]. In recent years, deep learning (DL) has achieved huge success in image recognition and speech recognition [7]. It can learn the feature-representation from raw data automatically, and the key aspect is that this process is not depended on human engineers, which can eliminate the experts' effect as more as possible. DL has been widely applied in the machine health-monitoring field [3].
Even though the applications of deep learning have achieved remarkable results in fault diagnosis, there are still some problems for the further improvements. Firstly, the deep learning models implemented by many researchers only have less than five hidden layers [8], which limits their final prediction accuracies. However, the well-trained deep learning can reach hundreds of layers on ImageNet. How to bridge the gap between the deep models in fault diagnosis and those in ImageNet could promote the performance of deep models in fault diagnosis. Secondly, the individual deep learning models for fault diagnosis still suffers from the generalization ability [9]. As stated by the no-free-lunch theorem [10,11,12], no single model can perform best on every dataset. To improve the generalization ability of deep learning method is essential.
To overcome these two drawbacks, a new ensemble version of deep learning method is proposed. Firstly, the transfer learning is applied to bridge the network gap between fault diagnosis and ImageNet. TL can learn a learning system from a dataset (source domain) and then applies this system to solve a new problem (target domain) more quickly and effectively. It should be noted that the new target domain can be irrelative with the source domain [13]. So the ResNet-50 which is pre-trained on the ImageNet can also perform well in fault diagnosis. The ResNet-50 has the depth of 50 layers, which is much deeper than traditional DL model applied in fault diagnosis, and it could improve the predication accuracy on fault diagnosis field. Secondly, the ensemble learning is also investigated in this research. Ensemble learning is an effective way to improve the generalization ability. Several classifiers are trained cooperatively using negative correlation learning (NCL), and then these classifiers are combined to form a powerful fault classifier. In this research, the transfer learning technique and the NCL technique are combined, and a new negative correlation transfer ensemble model (NCTE) is proposed for fault diagnosis.
The rest of this paper is organized as follows. Section 2 discusses literature review. Section 3 presents the methodologies of negative correlation learning. Section 4 presents the proposed NCTE. Section 5 presents the case studies. The conclusion and future researches are presented in Section 6.
With the development of smart manufacturing, the data-driven fault diagnosis has received more and more attentions. It is very suitable for the complicated industrial systems, since the data-driven fault diagnosis applied the learning-based approaches to learn from the historic data without the models about the system [14,15,16]. The learning-based approaches can be classified into statistical analysis, machining learning methods and their joint paradigm. Principal component analysis (PCA), partial least squares (PLS), and independent Component Correlation (ICA) have received considerable attentions on the industrial process monitoring [17]. The machine learning methods also achieved good applications in fault diagnosis, such as support vector machine (SVM) [18,19], artificial neural network (ANN) [20], Bayesian network [21].
Since deep learning (DL) methods can obtain the feature-representations of raw data in an automatically way, it has shown a great potential in machine health monitoring field [3,22]. Wang et al. [23] investigated an adaptive deep CNN model, and the main parameters were determined by particle swarm optimization. Shao et al. [2] studied deep belief network based fault diagnosis on rolling bearing. Wang et al. [24] studied a new type of bilateral long short-term memory model (LSTM) for the cycle time prediction of re-entrant manufacturing system. Pan et al. [25] proposed a LiftingNet for mechanical data analysis and the results showed that LiftingNet has a good performance on different rotating speeds. Li [26] studied IDSCNN with D-S evidence for bearing fault diagnosis. This method is also an ensemble CNN, and it has a good adaptability on different load conditions. Lu et al. [27] applied Convolutional Neural Network (CNN) to fault diagnosis, and the comparison experiments showed that the accuracy of greater than 90% was achieved with fewer computational resource. Zhang et al. [28] studied the intelligent fault diagnosis under varying working conditions using domain adaptive CNN method.
However, due to the fact that the volume of labeled samples in fault diagnosis is relatively small compared with ten million annotated images in ImageNet, the DL models for fault diagnosis are shallow compared with benchmark deep learning models in ImageNet. However, it is hard to train a deep model without the large amount of well-organized training dataset like ImageNet, so to train a very deep model on fault diagnosis field is almost impossible. To deal with this challenge, by applying transfer learning and taking the deep CNN model trained on ImageNet as the feature extractor, the deep learning model that trained on ImageNet can also perform well on small data in another domain.
Transfer learning (TL) is a new paradigm in machine learning field. TL can learn a learning system from a dataset (source domain) and then applies this system to solve a new problem (target domain) more quickly and effectively. It should be noted that the new target domain can be irrelative with the source domain [13].
TL has been studied by many researchers. Donahue et al. [29] investigated the generic tasks, which may be suffered by insufficient labeled data for training a deep DL model, and they released DeCAF as generic image features across many visual recognition tasks. Based on DeCAF, Ren et al. [30] studied a feature transferring learning method using pre-trained DeCAF for Automated Surface Inspection, as shown in Figure 1. They tested the proposed methods on NEU surface defect database, weld defect database, wood defect database and micro-structure defect dataset, and the results showed that the proposed algorithm outperforms several best benchmarks in literature.
Many other famous CNN models that trained on ImageNet are also investigated for transfer learning, such as CifarNet, AlexNet, GoogleNet, ResNet and so on. Wehrmann et al. [31] studied a novel approach for adult content detection in videos and applied both pre-trained GoogleNet and ResNet architectures as the feature extractor. The results shown that the proposed method outperformed the current state-of-the-art methods for adult content detection. Shin et al. [32] applied CifarNet, AlexNet and GoogLeNet for the computer-aided detection in medical imaging tasks. They also investigated when and why transfer learning from pre-trained ImageNet (via fine-tuning) can be useful, and the results have achieved the state-of-the-art performance. Rezende et al. [33] investigated the transfer learning on ResNet-50 on the classification of malicious software, and the results showed that this approach can effectively classify the malware families with the accuracy of 98.62%.
Applying the pre-trained CNN models that trained on ImageNet to fault diagnosis has investigated by many researchers. Janssens et al. [34] selected the pre-trained VGG-16 as the feature extractor and fine-tuning all the weights of the network. The proposed transfer learning method has been applied to use the infrared thermal video to automatically determine the condition of the machine. Shao et al. [8] proposed a VGG-16 based deep transfer learning fault diagnosis and the structure of their method has been shown in Figure 2. The proposed method is applied on induction motors, gearboxes, and bearings dataset and the results showed that it has achieved a significant improvement by using the transfer learning technique. The application of transfer learning on fault diagnosis has great potential to improve the prediction accuracies.
The advantage of TL on fault diagnosis can be summarized as two aspects. Firstly, the labeled data in fault diagnosis is also small, and it is hard to train deep models in fault diagnosis, which could limit the prediction of deep learning in fault diagnosis. With transfer learning, the deep models can extract better features on fault diagnosis and then improve the accuracy on fault diagnosis. Secondly, the deeper models has much more parameters than shallow models. The training of a deep model requires considerable computational and time resources as well as a large amount of labelled data. However, by using transfer learning, only the fine-tuning process is necessary, which could reduce the requirements on hardware and training process.
Even the great improvement has been achieved by the transfer learning on fault diagnosis field, the application of transfer learning on fault diagnosis is only at the beginning. The further investigation and improvement on the transfer learning is necessary. In this research, a new ensemble transfer learning by using negative correlation ensemble is proposed.
Ensemble method is a learning pattern in which a group of base learners is trained for the same task, and they worked together as the committee to give the final results. As stated by the no-free-lunch theorem [10,35,36], no single model can perform best on every dataset. The ensemble learning becomes an effective way to improve the performance. The ensemble learning was proposed by Hansen and Salamons [37], and their results provided the solid support that the generalization ability of a neural network can be significantly improved through combining a number of neural networks.
Ensemble learning has been studied by many researchers, and these ensemble algorithms can be classified into three categories [38]. In the first category, each base learner is trained with a subset of training samples, and then these base learners are combined at advance. The typical ensemble algorithm is Bagging and its variants. In the second category, the weights are introduced on the training samples and the training samples that are misclassified by the former base learner would be paid more attention in the next training stage. The algorithms in the second categories include adaboosting and its variants. In the third category, the interaction and cooperation among the base learners are necessary to generate a more diverse group of base learner. One of the typical algorithm in the third category is the negative correlation learning (NCL). NCL emphasizes the cooperation and specialization among different base learners during the base learner design. It provides an opportunity for different base learner to interact with each other to solve one single problem. The accuracy and the diversity of the group of base learner, and the results of NCL has shown a good potential [39].
The ensemble learning in fault diagnosis has also been investigated. Hu et al. [40] proposed a new ensemble approach for the data-driven remaining useful life estimation. Their ensemble method is the first category, and the member algorithms are weighted to form the final ensemble algorithm. The accuracy-based weighting, diversity-based weighting and optimization-based weighting are applied and the results showed that the ensemble approach with any weighting scheme gives more accurate RUL predictions compared to any sole member algorithm. Wang et al. [9] studied the selective ensemble neural networks (PSOSEN) for the fault diagnosis of bearings and pumps. In their method, the adaptive particle swarm optimization (APSO) is developed for not only determining the optimal weights but also selecting superior base learners. The results demonstrated that PSOSEN has achieved desirable accuracies and robustness under the environmental noise and working condition fluctuations. Wu et al. [41] proposed the Easy-SMT ensemble algorithm based on synthesizing SMOTE-based data augmentation policy. The method is tested on the PHM 2015 challenge datasets and the results showed that it could achieve good performance on multi-class imbalance learning task.
However, even though the ensemble learning has achieved remarkable results in the fault diagnosis field, as far as I know, the NCL technique has not been applied on fault diagnosis. In this research, the NCL is combined with transfer learning to construct the high accuracy classifier for fault diagnosis.
NCL introduces a correlation penalty term to the error function of each individual network in the ensemble so that all the networks can be trained interactively on the same training dataset. Given the training dataset{xn,yn}Nn=1, NCL combines M neural networks fi(x) to constitute the ensemble.
fens(xn)=1MM∑i=1fi(xn) | (1) |
To train network fi, the cost function ei for network i is defined by Eq 2. Where λ is a weighting parameter on the penalty term pi as shown in Eq 3.
ei=N∑n=1(fi(xn)−yn)2+λpi | (2) |
pi=N∑n=1{(fi(xn)−fens(xn))∑j≠i(fj(xn)−fens(xn))}=−N∑n=1(fi(xn)−fens(xn))2 | (3) |
From Eq 2, it can be seen that NCL uses a penalty term in the error function to produce base learners whose errors tend to be negatively correlated. So the NCL model can cooperate the training of base learner and the whole ensemble model simultaneously. λ control the degree of the negatively correlated. If set λ=0, then the error Eq 2 will become Eq 4, and each individual models would be trained separately. When set λ=1, then error Eq 2 can be trained as a whole ensemble model.
ei=N∑n=1(fi(xn)−yn)2 | (4) |
ei=N∑n=1(fi(xn)−yn)2−N∑n=1(fi(xn)−fens(xn))2=N∑n=1(fens(xn)−yn)2 | (5) |
In this research, the NCL technique is applied with transfer learning technique to obtain a new ensemble method for fault diagnosis.
In this section, a new negative correlation transfer ensemble model (NCTE) is proposed.
The whole flowchart of the proposed NCTE consists of four parts, the data preprocessing part, the feature transferring part, the fine-tuning part and the hyper-parameter selection part.
(1) Data preprocessing part: Since the input of ResNet-50 is the RGB images, it is essential to convert the time-domain signals to 3D matrix in order to use the pre-trained ResNet-50 network.
(2) Feature transferring part: Establish the structure of ResNet-50, and keep the layers weights in ResNet-50 unchanged. Since the output of ResNet-50 is 2048, the feature obtained by ResNet-50 is also a 2048 vector.
(3) Training part: Adding the several separated fully-connected (FC) layers at the end of ResNet-50, and then training these FC layers using the NCL technique.
(4) Hyper-parameter selection part: It is vital to select the key parameter, λ, in the NCL technique. In this research, the cross validation is applied to test the most proper λ.
The flowchart of the proposed NCTE is presented in Figure 3. The details of these four parts are given as following:
Data preprocessing is the essential part in the data-driven fault diagnosis. Since the input of ResNet-50 is the 3D natural image, so it is essential to transfer the time-domain signals to the 3D format. Chong [42] proposed the data preprocessing methods to convert the time-domain raw fault signals to 2D images. Wen et al [43] studied a new time domain signal to gray image method. Suppose the raw fault signals of all fault types are collected and then segmented to obtain the data samples. Let m×m denote the gray image size and Li(a), i = 1…N, a = 1…m2, denote the strength value of signal samples. N the number of samples. GP(j, k), j = 1...m, k = 1…m is matrix of 2D gray images. The time domain signals to gray images can be formulated by Eq 6.
GP(j,k)=L((j−1)×m+k)−Min(L)Max(L)−Min(L)×255 | (6) |
However, RGB image is 3D matrix format. Let RP(j, k, p), p = 1, 2, 3 presents this 3D matrix. The third elements of the RGB image are the strength of red (p = 1), green (p = 2) and blue (p = 3) channels. In this research, the data preprocessing method that transfers the time domain raw signals to 3D RGB images is presented as Eqs 7–10.
NMi(j,k)=Li((j−1)×M+k)−Mini,j,k(Li((j−1)×M+k))Maxi,j,k(Li((j−1)×M+k))−Mini,j,k(Li((j−1)×M+k)) | (7) |
RPi(j,k,1)=NMi(j,k)×255 | (8) |
RPi(j,k,2)=NMi(j,k)×255 | (9) |
RPi(j,k,3)=NMi(j,k)×255 | (10) |
The difference between Eq 6 and Eq 7 is that Eq 6 applies the maximum and minimum values of the data sample while Eq 7 selects the maximum and minimum values of the whole samples. Then scale the normalized matrix (NM(j, k)) to 0-255 and copy the scaled results to RP(j, k, p), as shown in Eq 8–10.
Residual Networks (ResNet) [44] is a very famous Convolutional Neural Network developed in recent years. Since the vanishing/exploding gradient problem is also found in deep learning algorithms using gradient-based learning methods and backpropagation [45], the ResNet applied the shortcut connections to construct the deep networks to avoid this problem, and it has shown a great performance in image recognition.
ResNet-50 is a released version of ResNet, which has 50 layers. The input of ResNet-50 is 224 × 224, and the detail structure of ResNet-50 is shown in Figure 4. The output of ResNet-50 is 1000. In this research, the transfer learning is combined with ResNet-50 and the NCL technique is applied to train several newly constructed FC layers and softmax classifiers.
Based on the ResNet-50, a new structure of NCTE is proposed. For most transfer learning method, there are only one softmax classifier. However, in this research, total M and softmax classifier are conducted in order to form the inherit ensemble version of transfer learning. As shown in Figure 5, along with the sofmax classifiers, one FC layer is also constructed for each softmax classifier, and the hidden neurons are 128 for all FC layers. FC layers of each softmax are separate and they have no interaction to each other.
Since there are M classifiers in the structure, the final output of the NCTE is the ensemble version of all M classifiers, and the bagging ensemble is applied, as shown in Eq 1. The training of these M classifiers are based on the NCL training process. For the training of each softmax classifier, there are two parts in the error function. The first part is the error function between the output of softmax classifier and the labels. The second part is the diversity term, and it tries to make M classifiers to be as diversity as possible. The second part worked as the penalty term in the loss function. The training method of NCTE is presented in Algorithm (1).
Algorithm (1), Training method for NCLE |
Step 1: Let M be the final number of classifiers Step 2: Take a training dataset {xn,yn}Nn=1 and the hyper-parameter λ. Step 3: For the training dataset, repeat the following (a) to (d) steps until the maximal epochs is reached: (a) Calculate the ensemble output of M softmax classifiers. fens(xn)=1MM∑i=1fi(xn) (b) For each softmax classifiers, from i=1 to M, for each weight wij in FC layer and softmax classifiers i, perform the update of the i-th FC layer and softmax classifiers: ei=N∑n=1(fi(xn)−yn)2−λN∑n=1(fi(xn)−fens(xn))2 ∂ei∂wij=2N∑n=1(fi(xn)−yn)∂fi∂wij−2λN∑n=1(fi(xn)−fens(xn))(1−1M)∂fi∂wij (c) Calculate the new output of the i-th softmax classifiers. (d) Repeat (a)-(c) until all M FC layer and softmax classifiers are updated. Step 4: Combine all softmax classifiers to formulate the final ensemble classifiers. |
As shown in Eq 2, hyper-parameter λ control the degree of the negative correlate rate of the NCTE, so to select a proper hyper-parameter λ is vital for NCTE. In this research, the λ is selected according to its model performance. In many data-driven fault diagnosis methods, the performance is evaluated by the testing dataset, and the model that has the best performance on the testing dataset are selected. However, this model selection method has the following shortcomings: (1) It requires the testing dataset in addition to the training data. However, the testing dataset should be untouched during the training method and model selection period. (2) The selected standalone algorithm may not be robust, since no statistical analysis of the results are conducted. To overcome the above shortcoming, the cross validation technique is applied in these researches to obtain a reliable performance evaluation method for the model selection.
Cross validation (CV) is a popular technique to obtain a reliable model [46]. The CV technique divides the training dataset into two parts, and they are the training part and the validation part. The typical CV techniques includes Leave-one-out CV, Generalized CV, K-Fold CV and so on [47]. K-fold CV is the most popular technique of CV techniques. It divides the whole data into K subsamples with approximately equal cardinality N/K samples. Each subsample successively plays the role of validation part, while the rest K-1 subsamples are used for train part. However, the selection of K has no theoretical analysis [48], and the popular value of K are set to be 3, 5 and 10. In this research, the five-fold cross validation is applied.
Suppose Yv and ˆYv denote the actual and prediction labels on the validate part, and Nv is the sample number of validate dataset. The accuracy of CV (Acccv) is the mean of five-fold accuracy, and it can be shown by Eq 11.
Acccv=K∑k=11Nv(Nv∑i=11{Yv==ˆYv}) | (11) |
The Acccv is applied to the selection of the proper λ. After finishing this selection, the obtained fault diagnosis classifier would be tested on a separated testing dataset, and the accuracy of testing dataset is the final results (Acc) of NCTE for comparison.
The KAT bearing damage dataset provided by KAT datacenter in Paderborn University [45]. The hardware of this experiment is shown in [45], and there are 15 datasets and they can be categorized as three healthy classifications as shown in Table 1. The K0-series (K001–K005) are the healthy condition, the KA-series (KA04, KA15, KA16, KA22, KA30) are the outer bearing ring with damage and the KI-series (KI04, KI14, KI16, KI18, KI21) are the inner bearing ring with damage. The experiments are conducted with four different operating parameters, and the operating parameters are shown in Table 2. Each experiment is conducted 20 repeated and the vibrations signals are collected for analysis, and the sampling rate is 64 kHz. It should be noted that the damage of this dataset is real damages caused by accelerated lifetime test.
Healthy (Class 1) | Outer ring damage (Class 2) | Inner ring damage (Class 3) |
K001 | KA04 | KI04 |
K002 | KA15 | KI14 |
K003 | KA16 | KI16 |
K004 | KA22 | KI18 |
K005 | KA30 | KI21 |
No. | Rotational speed | Load torque | Radial force |
0 | 1500 | 0.7 | 1000 |
1 | 900 | 0.7 | 1000 |
2 | 1500 | 0.1 | 1000 |
3 | 1500 | 0.7 | 400 |
During the experiments, the algorithm is written in python 3.5 using Tensorflow. The hidden neurons in the FC layers are set to be 128, the L2 regulations rate is 1e-5, m is set to be 64. The learning rate scheduler is the momentum optimizer and the initial learning rate is 0.005 and the momentum value is 0.9. The batch size is 200, and the total epoch is 40. In this research, the five-fold cross validation is applied for selection the proper λ. The tested λ are from 0 to 1 with the increment of 0.1.
During the cross validation process, the number of the softmax classifiers are set to be 2, and the effect of the λ on the cross validation process is presented in Table 3 and Figure 6. From Table 3, it can be seen that the mean (mean), the minimum (min) and the stand deviation (std) of the Acccv is the best on all the values of λ. Since the results of λ = 0.4 have the best mean and std, the selection of λ is 0.4 in this round. Figure 6 presents the mean value of Acccv along with the increase of λ. It can be seen that the whole curve like an inverse 'U' type, and the peak of this curve is also at λ = 0.4.
λ | 0 | 0.1 | 0.2 | 0.3 | 0.4 | 0.5 |
max | 98.67% | 98.62% | 98.71% | 98.68% | 98.68% | 98.66% |
mean | 98.52% | 98.56% | 98.49% | 98.52% | 98.62% | 98.55% |
min | 98.14% | 98.46% | 98.13% | 98.21% | 98.59% | 98.44% |
std | 0.0022 | 0.0006 | 0.0024 | 0.0018 | 0.0004 | 0.0009 |
λ | 0.6 | 0.7 | 0.8 | 0.9 | 1.0 | |
max | 98.68% | 98.63% | 98.64% | 98.64% | 98.67% | |
mean | 98.60% | 98.52% | 98.47% | 98.29% | 98.56% | |
min | 98.50% | 98.27% | 97.98% | 97.76% | 98.48% | |
std | 0.0008 | 0.0016 | 0.0028 | 0.0039 | 0.0007 |
The convergence of two classifiers and the final ensemble classifier (NCTE) are plotted in Figure 7. From the results, it can be seen that both two classifiers have similar convergence speed, and the final ensemble classifier outperforms the two classifiers at most time. These results validate that the ensemble of these two classifiers can promote the performance than the individual single classifiers.
The number of the classifiers is also an important hyper-parameter for NCTE. In this subsection, the effect of the number of classifiers on the final results is analyzed. The number of classifiers in the experiments are set to be 2, 3, 5, 7, 9, 11, 13, and 15. The NCTE with the large number of classifiers are discussed as well, and the number of classifiers are 20, 30 and 50. The baseline method is using the NCTE with only one number of the classifiers.
The results in this experiment are presented in Table 4 and Figure 8. The best λ of cross validation, Acccv, Acc and the training time are presented in Table 4. For each results, only the best λ value and Acccv is presented. From the results, it can be seen that the best number of the classifiers is 13. And the Acccv is 98.73% while the performance of this version of NCTE in the testing dataset Acc is also the best among these methods, and it is as high as 98.72%.
Number of classifiers | 1 (Baseline) | 2 | 3 | 5 | 7 | 9 |
λ value | - | 0.4 | 0.8 | 0.5 | 0.4 | 1.0 |
Acccv | 98.41% | 98.62% | 98.65% | 98.64% | 98.68% | 98.70% |
Acc | 98.38% | 98.62% | 98.64% | 98.63% | 98.67% | 98.66% |
Time | 261.31 | 429.27 | 608.82 | 930.67 | 1320.73 | 1670.69 |
Number of classifiers | 11 | 13 | 15 | 20 | 30 | 50 |
λ value | 0.8 | 0.4 | 0.1 | 0.2 | 0.2 | 0 |
Acccv | 98.69% | 98.73% | 98.71% | 98.69% | 98.69% | 98.69% |
Acc | 98.67% | 98.72% | 98.69% | 98.67% | 98.67% | 98.68% |
Time | 1932.04 | 2389.01 | 2626.02 | 3447.68 | 4706.05 | 8082.40 |
On the other side, the training time increases sharply along with the number of the classifiers, as shown in Figure 8. From the Figure 8, it can be seen that the number of the classifiers should be keep in a proper size. A large number of classifiers don't help to increase the final accuracy while it would increase the computation resource largely. However, taking the baseline into consideration, the Acc of baseline is only 98.41%, all NCTE variants are better than this result.
In this subsection, the NCTL is compared with traditional bagging method and the ResNet-50. The bagging is select as the k-fold bagging [1,50]. The ResNet-50 are random initialized and there are used to show the effect of TL. The comparison results are shown in TABLE 5. It should be noted that the bagging method is also based on TL, and it replace the ensemble method from NCL to Bagging. The ResNet-50 uses the same data-preprocessing process with NCTL, but it trained from the raw data without TL.
Methods | Mean Accuracy |
NCTE | 98.73 |
Bagging | 98.62 |
ResNet-50 | 72.31 |
From the results, it can be seen that the accuracy of Bagging is 98.62%, which is inferior to NCTL slightly. The results of ResNet-50 is 72.31%. The results show that the NCTL has better performance than the random initialized ResNet-50. These results show that transfer learning using the pre-trained ResNet-50 could provide better results than to train a new random initialized ResNet-50.
In order to validate the performance of the proposed NCTE, the version of NCTE with 13 classifiers are compared with other published methods. The comparison of NCTE with traditional machine learning methods [49] are presented in Table 5, and the comparison of NCTE with deep learning methods are presented in Table 6.
Methods | Mean Accuracy |
NCTE | 98.73 |
Ensemble | 98.3 |
CART | 98.3 |
RF | 98.3 |
BT | 83.3 |
SVM-PSO | 75.8 |
KNN | 62.5 |
ELM | 60.8 |
NN | 44.2 |
In Table 6, the comparison methods are classification and regression trees (CART), random forests (RF), Boosted Trees (BT), neural networks (NN), support vector machines with parameters optimally tuned using particle swarm optimization (SVM-PSO), extreme learning machine (ELM), k-nearest neighbors (KNN) and their ensemble algorithms using majority voting (Ensemble). The details of these methods can be found in [49], and here their results are directly taken from [49]. From the results, it can be seen that NCTE has achieved a good result, and it outperforms all these traditional machine learning methods.
Table 7 presents the comparison of NCTE with other deep learning methods. These deep learning methods are deep inception net with atrous convolution (ACDIN), Convolution Neural Networks with Training Interference (TICNN), Deep Convolutional Neural Networks with Wide First-layer Kernels (WDCNN), AlexNet, ResNet and convolutional neural network based on a capsule network with an inception block (ICN). Their results can be found in [51] and [52]. The results show that the prediction accuracy of ACDIN, TICNN, WDCNN, AlexNet, ResNet and ICN are 94.5%, 54.09%, 54.55%, 79.92%, 77.52% and 82.05% respectively. These results validate the performance of NCTE.
Methods | Mean Accuracy |
NCTE | 98.73 |
ACDIN 51 | 94.5 |
TICNN 51 | 54.09 |
WDCNN 51 | 54.55 |
AlexNet 52 | 79.92 |
ResNet 52 | 77.52 |
ICN 52 | 82.05 |
This research presents a new negative correlation ensemble transfer learning for fault diagnosis based on convolutional neural network (NCTE). The main contribution of this paper are as following: 1) On the structure aspect, the transfer learning is applied for fault diagnosis to build a deeper structure than traditional DL method for fault diagnosis; 2) On the training method aspect, the transfer learning is trained using negative correlation learning (NCL), and several softmax classifiers are added and trained cooperatively based on the transfer learning.3) The hyper-parameter of NCTE are determined by cross validation, and it could help to obtain a more reliable fault classifier. The proposed NCTE is conducted on the KAT Bearing Dataset, and the results show that NCTE has achieved good results compared with other machine learning and deep learning methods. However, the time consumption of NCTE increases sharply with the increase of the number of softmax classifiers. So it is better to keep the number of the classifiers in a proper size.
The limitations of the proposed method may include as followings: Firstly, the time consumption of NCTE increases sharply with the increase of the number of softmax classifiers. Secondly, the imbalance of the fault data and normal data in fault diagnosis is ignored in this research. Based on these limitations, the future researches can be done in the following ways. Firstly, an improve version of NCTE can be investigated to reduce the time consumption. Secondly, the imbalance data handle techniques can be combined with NCTE.
This work was supported in part by the Natural Science Foundation of China (NSFC) under Grants 51805192, National Natural Science Foundation for Distinguished Young Scholars of China under Grant No.51825502, China Postdoctoral Science Foundation under Grant 2017M622414, Guangdong Science and Technology Planning Program under Grant 2015A020214003 and Supported by Program for HUST Academic Frontier Youth Team under Grant 2017QYTD04.
The authors declare that there is no conflict of interests regarding the publication of this paper.
[1] | B. Sun, M. Wei, W. Wu, B. B. Jing. A novel group decision making method for airport operational risk management, Math. Biosci. Eng., 17 (2020), 2402-2417. |
[2] | S. L. Wu, P. C. Chen, K. Y. Chang, C. C. Huang, Robust gain-scheduled control for vertical/short take-off and landing aircraft in hovering with time-varying mass and moment of inertia, Proc. Inst. Mech. Eng. Part G., 222 (2008), 473-482. |
[3] | H. Nazini, T. Sasikala, Simulating aircraft landing and takeoff scheduling in distributed framework environment using Hadoop file system, Cluster. Comput., 22 (2019), 13463-13471. |
[4] | Y. Ding, J. Valasek, Aircraft landing scheduling optimization for single runway noncontrolled airports: Static Case, J. Guid. Control Dynam., 30 (2007), 252-255. |
[5] | M. Ahmed, S Alam, M. Barlow, A cooperative co-evolutionary optimization model for best-fit aircraft sequence and feasible runway configuration in a multi-runway airport, Aerospace, 5 (2018), 345-353. |
[6] | L. Bianco, P. Dell'Olmo, S. Giordani, Scheduling models for air trafic control in terminal areas, J. Scheduling, 9 (2006), 180-197. |
[7] | D. Briskorn, R. Stolletz, A dynamic programming approach for the aircraft landing problem with aircraft classes, Eur. J. Oper. Res., 43 (2015), 61-69. |
[8] | R. G. Dear, The dynamic scheduling of aircraft in the near terminal area, MIT Libraries, (1976). |
[9] | H. N. Psaraftis, A dynamic programming approach for sequencing identical groups of jobs, Oper. Res., 28 (1980), 1347-1359. |
[10] | V. J. Hansen, Genetic search methods in air traffic control, Comput. Oper. Res., 31 (2004), 445- 459. |
[11] | H. Pinol, J. E. Beasley, Scatter search and bionomic algorithms for the aircraft landing problem, Eur. J. Oper. Res., 171 (2006), 439-462. |
[12] | A. Salehipour, L. M. Naeni, H. Kazemipoor, Scheduling aircraft landings by applying a variable neighborhood descent algorithm: runway-dependent landing time case, J. Appl. Oper. Res., 1 (2009), 39-49. |
[13] | Y. H. Liu, A genetic local search algorithm with a threshold accepting mechanism for solving the runway dependent aircraft landing problem, Optim. Lett., 5 (2011), 229-245. |
[14] | G. Bencheikh, J. Boukachour, A. E. H. Alaoui, Improved ant colony algorithm to solve the aircraft landing problem, Int. J. Comput. Theory Eng., 3 (2011), 224-233. |
[15] | A. Salehipour, M. Modarres, L. Moslemi Naeni, An efficient hybrid meta-heuristic for aircraft landing problem, Comput. Oper. Res., 40 (2013), 207-213. |
[16] | A. Faye, Solving the aircraft landing problem with time discretization approach, Eur. J. Oper. Res., 242 (2015), 1028-1038. |
[17] | A. T. Ernst, M. Krishnamoorthy, R. H. Storer, Heuristic and exact algorithms for scheduling aircraft landings, Networks, 34 (1999), 229-241. |
[18] | J. E. Beasley, M. Krishnamoorthy, Y. M. Sharaiha, D. Abramson, Scheduling aircraft landings - The static case, Transport. Sci., 34 (2000), 180-197. |
[19] | H. N. Psaraftis, A dynamic programming approach for sequencing groups of identical jobs, Oper. Res., 28 (1980), 1347-1359. |
[20] | C. S. Venkatakrishnan, A. Barnett, A. M. Odoni, Landings at Logan airport: Describing and increasing airport capacity, Transport. Sci., 27 (1993), 211-227. |
[21] | F. Farhadi, A. Ghoniem, M. Al-Salem, Runway capacity management - an empiricalstudy with application to Doha international airport, Transp. Res. Part E: Logist. Transp. Rev., 68 (2014), 53-63. |
[22] | F. Furini, M. P. Kidd, C. A. Persiani, P. Toth, State space reduced dynamic programming for the aircraft sequencing problem with constrained position shifting, Int. Symp. Comb. Optim. (ISCO), 2014 (2014), 267-279. |
[23] | A. Ghoniem, F. Farhadi, M. Reihaneh, An accelerated branch-and-price algorithm for multiple-runway aircraft sequencing problems, Eur. J. Oper. Res., 246 (2015), 34-43. |
[24] | A. Ghoniem, F. Farhadi, A column generation approach for aircraft sequencing problems: A computational study, J. Oper. Res. Soc., 66 (2015), 1717-1729. |
[25] | H. Balakrishnan, B. Chandran, Algorithms for scheduling runway operations under constrained position shifting, Oper. Res., 58 (2010), 1650-1665. |
[26] | D. Harikiopoulo, N. Neogi, Polynomial-time feasibility condition for multiclass aircraft sequencing on a single-runway airport, IEEE Trans. Intell. Transp. Syst., 12 (2011), 2-14. |
[27] | A. Lieder. R. Stolletz, Scheduling aircraft take-offs and landings on interdependent and heterogeneous runways, Transport. Res. E-Log., 88 (2016), 67-188. |
[28] | M. Samà, A. D'Ariano, F. Corman, D. Pacciarelli, Coordination of scheduling decisions in the management of airport airspace and taxiway operations. Transport. Res. Pro., 23 (2017), 246- 262. |
[29] | J. Jemai M. Zekri K. Mellouli, An NSGA-II algorithm for the green vehicle routing problem, Evo. Comput. Com. Opt., 2012 (2012), 37-48. |
[30] | M. Wei, T. Liu, B. Sun, B. B. Jing, Optimal integrated model for feeder transit route design and frequency-setting problem with stop selection, J. Adv. Transport., 2020 (20200. |
[31] | A. Slowik, H. Kwasnicka, Nature inspired methods and their industry Applications-Swarm intelligence algorithms, IEEE T. Ind. Inform., 14 (2018), 1004-1015. |
[32] | M. A. Dulebenets, A comprehensive evaluation of weak and strong mutation mechanisms in evolutionary algorithms for truck scheduling at cross-docking terminals, IEEE Access, 6 (2018). |
[33] | X. Zhao, C. Wang, J. Su, J. Wang, Research and application based on the swarm intelligence algorithm and artificial intelligence for wind farm decision system, Renew. Energ., 134 (2019), 681-697. |
[34] | L. Brezonik, I. Fister, V. Podgorelec, Swarm intelligence algorithms for feature selection: A review, Appl. Sci., 8 (2018), 1521. |
[35] | M. A. Dulebenets, A delayed start parallel evolutionary algorithm for just-in-time truck scheduling at a cross-docking facility, Int. J. Prod. Econ., 212 (2019), 236-258. |
[36] | H. Anandakumar, K. Umamaheswari, A bio-inspired swarm intelligence technique for social aware cognitive radio handovers, Comput. Electr. Eng., 71 (2018), 925-937. |
[37] | T. Li, G. Kou, Y. Peng, Y. Shi, Classifying with adaptive hyper-spheres: An incremental classifier based on competitive learning, IEEE Trans. Syst. Man Cybern. Syst., 50 (2020), 1218-1229. |
[38] | G. Kou, C. S. Lin, A cosine maximization method for the priority vector derivation in AHP, Eur. J. Oper. Res., 235 (2014), 225-232. |
1. | Chuan Li, Shaohui Zhang, Yi Qin, Edgar Estupinan, A systematic review of deep transfer learning for machinery fault diagnosis, 2020, 407, 09252312, 121, 10.1016/j.neucom.2020.04.045 | |
2. | Changhe Zhang, Li Kong, Qi Xu, Kaibo Zhou, Hao Pan, Fault diagnosis of key components in the rotating machinery based on Fourier transform multi-filter decomposition and optimized LightGBM, 2021, 32, 0957-0233, 015004, 10.1088/1361-6501/aba93b | |
3. | Hao Sheng, Zhongsheng Chen, Yemei Xia, Jing He, 2020, Review of Artificial Intelligence-based Bearing Vibration Monitoring, 978-1-7281-5181-6, 58, 10.1109/PHM-Jinan48558.2020.00018 | |
4. | Wentao Luo, Jianfu Zhang, Pingfa Feng, Dingwen Yu, Zhijun Wu, A concise peephole model based transfer learning method for small sample temporal feature-based data-driven quality analysis, 2020, 195, 09507051, 105665, 10.1016/j.knosys.2020.105665 | |
5. | Jinyang Jiao, Ming Zhao, Jing Lin, Kaixuan Liang, A comprehensive review on convolutional neural network in machine fault diagnosis, 2020, 417, 09252312, 36, 10.1016/j.neucom.2020.07.088 | |
6. | Gui-Rong You, Yeou-Ren Shiue, Wei-Chang Yeh, Xi-Li Chen, Chih-Ming Chen, A Weighted Ensemble Learning Algorithm Based on Diversity Using a Novel Particle Swarm Optimization Approach, 2020, 13, 1999-4893, 255, 10.3390/a13100255 | |
7. | Ruqiang Yan, Fei Shen, Chuang Sun, Xuefeng Chen, Knowledge Transfer for Rotary Machine Fault Diagnosis, 2020, 20, 1530-437X, 8374, 10.1109/JSEN.2019.2949057 | |
8. | Gandi Satyanarayana, P. Appala Naidu, Venkata Subbaiah Desanamukula, Kadupukotla Satish kumar, B. Chinna Rao, A mass correlation based deep learning approach using deep Convolutional neural network to classify the brain tumor, 2023, 81, 17468094, 104395, 10.1016/j.bspc.2022.104395 | |
9. | Asefeh Asemi, Andrea Ko, Adeleh Asemi, Infoecology of the deep learning and smart manufacturing: thematic and concept interactions, 2022, 40, 0737-8831, 994, 10.1108/LHT-08-2021-0252 | |
10. | Jin Kyu Oh, Jun Young Lee, Sung-Jong Eun, Jong Mok Park, New Trends in Innovative Technologies Applying Artificial Intelligence to Urinary Diseases, 2022, 26, 2093-6931, 268, 10.5213/inj.2244280.140 | |
11. | Xin Pei, Shaohui Su, Linbei Jiang, Changyong Chu, Lei Gong, Yiming Yuan, Research on Rolling Bearing Fault Diagnosis Method Based on Generative Adversarial and Transfer Learning, 2022, 10, 2227-9717, 1443, 10.3390/pr10081443 | |
12. | Zhiping Song, 2022, Mathematical Modeling Method based on Neural Network and Computer Multi-Dimensional Space, 978-1-7281-8115-8, 1080, 10.1109/ICETCI55101.2022.9832088 | |
13. | Fei Xia, Xiaojun Xie, Zongqin Wang, Shichao Jin, Ke Yan, Zhiwei Ji, A Novel Computational Framework for Precision Diagnosis and Subtype Discovery of Plant With Lesion, 2022, 12, 1664-462X, 10.3389/fpls.2021.789630 | |
14. | Zhang Zhihao, Wang Zumin, 2022, Research on rolling bearing fault diagnosis method based on hybrid deep learning network model, 978-1-6654-5417-9, 406, 10.1109/iThings-GreenCom-CPSCom-SmartData-Cybermatics55523.2022.00091 | |
15. | Lifa Fang, Yanqiang Wu, Yuhua Li, Hongen Guo, Hua Zhang, Xiaoyu Wang, Rui Xi, Jialin Hou, Using Channel and Network Layer Pruning Based on Deep Learning for Real-Time Detection of Ginger Images, 2021, 11, 2077-0472, 1190, 10.3390/agriculture11121190 | |
16. | Marcel Braig, Peter Zeiler, Using Data From Similar Systems for Data-Driven Condition Diagnosis and Prognosis of Engineering Systems: A Review and an Outline of Future Research Challenges, 2023, 11, 2169-3536, 1506, 10.1109/ACCESS.2022.3233220 | |
17. | Cinzia Giannetti, Aniekan Essien, Towards scalable and reusable predictive models for cyber twins in manufacturing systems, 2022, 33, 0956-5515, 441, 10.1007/s10845-021-01804-0 | |
18. | Aniekan Emmanuel Essien, Ilias Petrounias, 2022, chapter 7, 9781799898153, 84, 10.4018/978-1-7998-9815-3.ch007 | |
19. | Chenhui Qian, Junjun Zhu, Yehu Shen, Quansheng Jiang, Qingkui Zhang, Deep Transfer Learning in Mechanical Intelligent Fault Diagnosis: Application and Challenge, 2022, 54, 1370-4621, 2509, 10.1007/s11063-021-10719-z | |
20. | Eui-Sun Kim, Sung-Jong Eun, Seunghyun Youn, The Current State of Artificial Intelligence Application in Urology, 2023, 27, 2093-6931, 227, 10.5213/inj.2346336.168 | |
21. | Yanhua Guo, Ningbo Wang, Shuangquan Shao, Congqi Huang, Zhentao Zhang, Xiaoqiong Li, Youdong Wang, A review on hybrid physics and data-driven modeling methods applied in air source heat pump systems for energy efficiency improvement, 2024, 204, 13640321, 114804, 10.1016/j.rser.2024.114804 | |
22. | Weijie Shen, Maohua Xiao, Zhenyu Wang, Xinmin Song, Rolling Bearing Fault Diagnosis Based on Support Vector Machine Optimized by Improved Grey Wolf Algorithm, 2023, 23, 1424-8220, 6645, 10.3390/s23146645 | |
23. | Iqbal Misbah, C.K.M. LEE, K.L. KEUNG, Fault diagnosis in rotating machines based on transfer learning: Literature review, 2024, 283, 09507051, 111158, 10.1016/j.knosys.2023.111158 | |
24. | Yaochun Wu, Shaohua Du, Guijun Wu, Xiaobo Guo, Jie Wu, Rongzheng Zhao, Chi Ma, Minimum maximum regularized multiscale convolutional neural network and its application in intelligent fault diagnosis of rotary machines, 2025, 00190578, 10.1016/j.isatra.2025.01.044 |
Healthy (Class 1) | Outer ring damage (Class 2) | Inner ring damage (Class 3) |
K001 | KA04 | KI04 |
K002 | KA15 | KI14 |
K003 | KA16 | KI16 |
K004 | KA22 | KI18 |
K005 | KA30 | KI21 |
No. | Rotational speed | Load torque | Radial force |
0 | 1500 | 0.7 | 1000 |
1 | 900 | 0.7 | 1000 |
2 | 1500 | 0.1 | 1000 |
3 | 1500 | 0.7 | 400 |
λ | 0 | 0.1 | 0.2 | 0.3 | 0.4 | 0.5 |
max | 98.67% | 98.62% | 98.71% | 98.68% | 98.68% | 98.66% |
mean | 98.52% | 98.56% | 98.49% | 98.52% | 98.62% | 98.55% |
min | 98.14% | 98.46% | 98.13% | 98.21% | 98.59% | 98.44% |
std | 0.0022 | 0.0006 | 0.0024 | 0.0018 | 0.0004 | 0.0009 |
λ | 0.6 | 0.7 | 0.8 | 0.9 | 1.0 | |
max | 98.68% | 98.63% | 98.64% | 98.64% | 98.67% | |
mean | 98.60% | 98.52% | 98.47% | 98.29% | 98.56% | |
min | 98.50% | 98.27% | 97.98% | 97.76% | 98.48% | |
std | 0.0008 | 0.0016 | 0.0028 | 0.0039 | 0.0007 |
Number of classifiers | 1 (Baseline) | 2 | 3 | 5 | 7 | 9 |
λ value | - | 0.4 | 0.8 | 0.5 | 0.4 | 1.0 |
Acccv | 98.41% | 98.62% | 98.65% | 98.64% | 98.68% | 98.70% |
Acc | 98.38% | 98.62% | 98.64% | 98.63% | 98.67% | 98.66% |
Time | 261.31 | 429.27 | 608.82 | 930.67 | 1320.73 | 1670.69 |
Number of classifiers | 11 | 13 | 15 | 20 | 30 | 50 |
λ value | 0.8 | 0.4 | 0.1 | 0.2 | 0.2 | 0 |
Acccv | 98.69% | 98.73% | 98.71% | 98.69% | 98.69% | 98.69% |
Acc | 98.67% | 98.72% | 98.69% | 98.67% | 98.67% | 98.68% |
Time | 1932.04 | 2389.01 | 2626.02 | 3447.68 | 4706.05 | 8082.40 |
Methods | Mean Accuracy |
NCTE | 98.73 |
Bagging | 98.62 |
ResNet-50 | 72.31 |
Methods | Mean Accuracy |
NCTE | 98.73 |
Ensemble | 98.3 |
CART | 98.3 |
RF | 98.3 |
BT | 83.3 |
SVM-PSO | 75.8 |
KNN | 62.5 |
ELM | 60.8 |
NN | 44.2 |
Methods | Mean Accuracy |
NCTE | 98.73 |
ACDIN 51 | 94.5 |
TICNN 51 | 54.09 |
WDCNN 51 | 54.55 |
AlexNet 52 | 79.92 |
ResNet 52 | 77.52 |
ICN 52 | 82.05 |
Healthy (Class 1) | Outer ring damage (Class 2) | Inner ring damage (Class 3) |
K001 | KA04 | KI04 |
K002 | KA15 | KI14 |
K003 | KA16 | KI16 |
K004 | KA22 | KI18 |
K005 | KA30 | KI21 |
No. | Rotational speed | Load torque | Radial force |
0 | 1500 | 0.7 | 1000 |
1 | 900 | 0.7 | 1000 |
2 | 1500 | 0.1 | 1000 |
3 | 1500 | 0.7 | 400 |
λ | 0 | 0.1 | 0.2 | 0.3 | 0.4 | 0.5 |
max | 98.67% | 98.62% | 98.71% | 98.68% | 98.68% | 98.66% |
mean | 98.52% | 98.56% | 98.49% | 98.52% | 98.62% | 98.55% |
min | 98.14% | 98.46% | 98.13% | 98.21% | 98.59% | 98.44% |
std | 0.0022 | 0.0006 | 0.0024 | 0.0018 | 0.0004 | 0.0009 |
λ | 0.6 | 0.7 | 0.8 | 0.9 | 1.0 | |
max | 98.68% | 98.63% | 98.64% | 98.64% | 98.67% | |
mean | 98.60% | 98.52% | 98.47% | 98.29% | 98.56% | |
min | 98.50% | 98.27% | 97.98% | 97.76% | 98.48% | |
std | 0.0008 | 0.0016 | 0.0028 | 0.0039 | 0.0007 |
Number of classifiers | 1 (Baseline) | 2 | 3 | 5 | 7 | 9 |
λ value | - | 0.4 | 0.8 | 0.5 | 0.4 | 1.0 |
Acccv | 98.41% | 98.62% | 98.65% | 98.64% | 98.68% | 98.70% |
Acc | 98.38% | 98.62% | 98.64% | 98.63% | 98.67% | 98.66% |
Time | 261.31 | 429.27 | 608.82 | 930.67 | 1320.73 | 1670.69 |
Number of classifiers | 11 | 13 | 15 | 20 | 30 | 50 |
λ value | 0.8 | 0.4 | 0.1 | 0.2 | 0.2 | 0 |
Acccv | 98.69% | 98.73% | 98.71% | 98.69% | 98.69% | 98.69% |
Acc | 98.67% | 98.72% | 98.69% | 98.67% | 98.67% | 98.68% |
Time | 1932.04 | 2389.01 | 2626.02 | 3447.68 | 4706.05 | 8082.40 |
Methods | Mean Accuracy |
NCTE | 98.73 |
Bagging | 98.62 |
ResNet-50 | 72.31 |
Methods | Mean Accuracy |
NCTE | 98.73 |
Ensemble | 98.3 |
CART | 98.3 |
RF | 98.3 |
BT | 83.3 |
SVM-PSO | 75.8 |
KNN | 62.5 |
ELM | 60.8 |
NN | 44.2 |
Methods | Mean Accuracy |
NCTE | 98.73 |
ACDIN 51 | 94.5 |
TICNN 51 | 54.09 |
WDCNN 51 | 54.55 |
AlexNet 52 | 79.92 |
ResNet 52 | 77.52 |
ICN 52 | 82.05 |