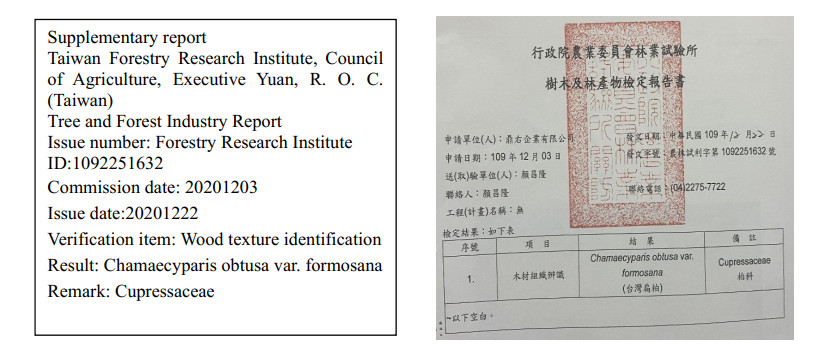
Citation: Bethan Morris, Lee Curtin, Andrea Hawkins-Daarud, Matthew E. Hubbard, Ruman Rahman, Stuart J. Smith, Dorothee Auer, Nhan L. Tran, Leland S. Hu, Jennifer M. Eschbacher, Kris A. Smith, Ashley Stokes, Kristin R. Swanson, Markus R. Owen. Identifying the spatial and temporal dynamics of molecularly-distinct glioblastoma sub-populations[J]. Mathematical Biosciences and Engineering, 2020, 17(5): 4905-4941. doi: 10.3934/mbe.2020267
[1] | Nosheen Aslam, Muhammad Sarfaraz Iqbal, Syed Makhdoom Hussain, Muhammad Rizwan, Qurat-Ul-Ain Naseer, Muhammad Afzal, Rizwan Muneer, Farzana Batool . Effects of chelating agents on heavy metals in Hepatitis C Virus (HCV) patients. Mathematical Biosciences and Engineering, 2019, 16(3): 1138-1149. doi: 10.3934/mbe.2019054 |
[2] | Jun Gao, Fei Wu, Yakufu Yasen, Wanqing Song, Lijia Ren . Generalized Cauchy process based on heavy-tailed distribution and grey relational analysis for reliability predicting of distribution systems. Mathematical Biosciences and Engineering, 2022, 19(7): 6620-6637. doi: 10.3934/mbe.2022311 |
[3] | Yuan Yang, Yuwei Ye, Min Liu, Ya Zheng, Guozhi Wu, Zhaofeng Chen, Yuping Wang, Qinghong Guo, Rui Ji, Yongning Zhou . Family with sequence similarity 153 member B as a potential prognostic biomarker of gastric cancer. Mathematical Biosciences and Engineering, 2022, 19(12): 12581-12600. doi: 10.3934/mbe.2022587 |
[4] | Zishuo Yan, Hai Qi, Yueheng Lan . The role of geometric features in a germinal center. Mathematical Biosciences and Engineering, 2022, 19(8): 8304-8333. doi: 10.3934/mbe.2022387 |
[5] | Yuan Yang, Lingshan Zhou, Xi Gou, Guozhi Wu, Ya Zheng, Min Liu, Zhaofeng Chen, Yuping Wang, Rui Ji, Qinghong Guo, Yongning Zhou . Comprehensive analysis to identify DNA damage response-related lncRNA pairs as a prognostic and therapeutic biomarker in gastric cancer. Mathematical Biosciences and Engineering, 2022, 19(1): 595-611. doi: 10.3934/mbe.2022026 |
[6] | Kaiyu Shen, Shuaiyi Ke, Binyu Chen, Tiantian Zhang, Hongtai Wang, Jianhui Lv, Wencang Gao . Identification and validation of biomarkers for epithelial-mesenchymal transition-related cells to estimate the prognosis and immune microenvironment in primary gastric cancer by the integrated analysis of single-cell and bulk RNA sequencing data. Mathematical Biosciences and Engineering, 2023, 20(8): 13798-13823. doi: 10.3934/mbe.2023614 |
[7] | Huu Dang Quoc . MEMINV: A hybrid efficient approximation method solving the multi skill-resource constrained project scheduling problem. Mathematical Biosciences and Engineering, 2023, 20(8): 15407-15430. doi: 10.3934/mbe.2023688 |
[8] | Fred Brauer . Some simple epidemic models. Mathematical Biosciences and Engineering, 2006, 3(1): 1-15. doi: 10.3934/mbe.2006.3.1 |
[9] | Kwabena Owusu-Agyemang, Zhen Qin, Appiah Benjamin, Hu Xiong, Zhiguang Qin . Guaranteed distributed machine learning: Privacy-preserving empirical risk minimization. Mathematical Biosciences and Engineering, 2021, 18(4): 4772-4796. doi: 10.3934/mbe.2021243 |
[10] | Shuyan Liu, Peilin Li, Yuanhao Tan, Geqi Ding, Bo Peng . A robust local pulse wave imaging method based on digital image processing techniques. Mathematical Biosciences and Engineering, 2023, 20(4): 6721-6734. doi: 10.3934/mbe.2023289 |
Red cypress, or chamaecyparis, is only grown in a few areas around the world. Located at the southernmost region of the cypress tree distribution, Taiwan is the only subtropical area with cypress. Taiwan cypress usually refers to Meniki (Chamaecyparis formosensis) and Hinoki (Chamaecyparis obtuse), and they are the coniferous species commonly seen at intermediate high altitude in Taiwan. They have very high economic value, and are used intensively for forestation in mountains of high attitude [1]. In the past half century, numerous researchers studied Meniki and Hinoki, exploring their habitats, physiological ecology, chemical elements, physical characteristics, and bioactivity [2].
Qualitative research was carried out for the steamed-water heat treatment on the outer bark of Hinoki (Chamaecyparis obtusa) to explore the possibility of using the residual forest biomass to produce valuable chemicals [3]. Most of Hinoki leaves contain essential oil, which is comprised of terpenoid glycoside. Raw terpenoid glycoside is extracted using traditional steamed hot water extraction on Hinoki leaves [4]. The ethanolic extracts on the bark of Hinoki can greatly inhibit fusarium and pseudomonas, and the inhibition is mainly caused by the volatile oil and non-volatile matter in the bark while neutral substances and acidic substances both have high activity towards pathogen [5]. The subject report highlighted that the cedar leaf oil of Hinoki can be used to stimulate olfactory sensation to significantly reduce the oxygenation concentration of hemoglobin in the right prefrontal cortex, as well as increase parasympathetic activities [6]. Academic studies revealed that touching Hinoki with palms is able to calm the activity on prefrontal cortex as well as increase the parasympathetic activities, and further cause physical relaxation [7]. According to the academic research, several chemical elements found on Hinoki contain terpenoids; some of them are considered good for antitumor, anti-malaria, and antimicrobial activity [8]. In terms of the application of Hinoki by dentists, the result shows that Hinoki is a strong antibacterial agent and able to carry out bactericidal action on porphyromonas gingivalis. Hinoki antibacterial agent does have a significant effect on the characteristics of the main outer membrane protein, but interferes with the antioxidant activities on bacteria [9].
Traditional extraction process of Taiwan cypress essential oil generates the by-product, hydrolat. Hydrolat is the condensate dissolved solution obtained from the distillation of logs, plants, or flowers. After the distillation during extraction process of distilling logs, plants, or flowers, it creates oil-water separation due to different density, known as hydrolat. Besides a small amount of essential oil, hydrolat contains all the water-soluble substances in logs, plants, or flowers. During the process of distillation, the oil elements that can be mixed with the odor escape to the condensation of distillation/condensation flow and are called water hydrolat along with oil-soluble oil. These hydrolats are commonly used to perfume, generic drug, food processing, aromatherapy, and traditional therapy [10]. The cypress hydrolat generated from traditional distillation is difficult to control its quality and lack of relevant hydrolat studies; thus, only essential oil is retained and the cypress hydrolat is discarded. When relevant pharmacological research and development application can be carried out, and the quality of hydrolat after distillation be controlled, there is a potential opportunity to develop high-value products.
Traditionally, Gas Chromatography-Mass Spectrometry (GC-MS) is the common approach to test the elements of hydrolat. GC-MS can identify the 35 main elements in Hinoki oil that accounts for 99.4% of the elements [11].
However, the traditional GC-MS testing methods are costly and time-consuming. The testing costs for all batches of cypress hydrolat are not economical for the industry in mass production. Hence, a rapid testing method for the quality of distilled cypress hydrolat with low cost and high efficiency is urgently needed by the industry to greatly reduce the testing time and cost, while maintaining the quality of cypress hydrolat.
In recent years, spectroscope has been wide used to collect the spectrum of an item and match it with data processing and machine learning in the fields of agriculture, food, drugs, and soil. Compared to the traditional testing technology, the spectrum of item matching with data processing and machine learning contains the advantages of non-destruction, immediacy, and low labor cost.
This study proposes a fast-testing technology on the quality of distilled cypress hydrolat based on spectrum matching, and provide the relevant experiment results.
The chemical elements of Hiba, Hinoki, Sawara, and Konotegashiwa can be used on the healing tissues of wounds on human body [1]. The ethanolic extracts on the bark of Hinoki can greatly inhibit fusarium and pseudomonas, and the inhibition is mainly caused by the volatile oil and non-volatile matter in the bark while neutral substances and acidic substances are both highly active towards pathogen [2]. Touching Hinoki with palms can calm the activity on prefrontal cortex, and at the same time, increase the parasympathetic activities and further cause physical relaxation [3]. The subject report highlighted that the cedar leaf oil of Hinoki can be used to stimulate olfactory sensation to significantly reduce the oxygenation concentration of hemoglobin in the right prefrontal cortex, as well as increase parasympathetic activities [4]. Hinoki essential oil can also be used in air purifiers to repel fruit flies and house flies, and the use of 70 μg/ ml of the essential oil has an effective period of about 5 hours [5]. This study evaluated the antibacterial activity of cypress essential oil against general infectious microorganisms and clinically resistant strains. The results revealed that the essential oil and non-volatile residue contain wide inhabitance activity towards the tester strain as well as antibacterial activity towards Methicillin-resistant Staphylococcus aureus (MRSA) and Vancomycin-Resistant Enterococcus (VRE) [6].
GC-MS is a method commonly used to identify analysis for different substances in the testing samples by combining the characteristics of gas chromatography and mass spectrometry [7]. It has been widely applied in the fields of environmental and biomedicine studies, as well as bioinformatics [8]. Previous studies examined the elements in the essential oil of Hinoki leaves and its seedlings leaves, and applied factor analysis and cluster analysis using the GC-MS [9].
Headspace solid-phase microextraction (static-HS) combining with GC-MS was used to analyze 23 leave samples from three species. The results indicated the static HS process greatly enhanced the speed of precision analysis of chemical fingerprints on a small number of samples. It is a fast and reliable tool for predicting characteristics of elements in the essential oil and the analysis of essential oil elements [10]. Essential oil from the upper part of Hinoki is obtained by hydro-distillation and analyzed by GC, GC-MS and 1C-NMR spectrum. The results showed that in the Hinoki oil, the main elements, such as perpinen-4 ol, SA olefin, camphor, citronellol, and γ- terpinene, account for 99.4% [11].
Hinoki residual forest biomass contains valuable chemicals, and GC-MS results also verified the content of chemical compounds [12]. Hinoki oil has great antibacterial and termite-repellent performance, without having strong bioactivity, and is a safe substance for the living environment [13]. Another study on Hinoki and terpenoids reported high performance in antibiosis, insect prevention, and antisepsis, as well as the effect of pressure relief and cancer prevention. Therefore, the terpenoids from the extracts can be used to help improving the public health through the humidifier [14].
Spectroscope captures signal on optical information and computerizes the signal to automatically display value of analysis, in order to identify the element contained in the specimens. Spectrometry time-of-flight secondary ion mass spectrometry (TOF-SIMS) is a technology with powerful functions and able to provide the chemical information on the surface of a solid sample without any chemical pre-treatment required. Compared with other technologies, the main advantage of TOF-SIMS is imaging analysis, which can directly observe the sub-micron spatial resolution of the chemical distribution on the sample surface [15]. There are many types of spectroscope, such as infrared spectroscopy and ultraviolet spectrophotometer, which are used in the visible wavelength. A previous study compared the Raman Spectroscopy and Pyrolysis-gaschromatography and mass spectrometr (Py-GC/MS) [16]. Thermogravimetric-Fourier Transform Infrared Spectroscopy (TG-FTIR) and Py-GC/MS were used to study the pyrolysis process of bagasse and mud on the mixed samples [17]. With the advantage of low cost, the near-infrared spectroscopy used in the microelectromechanical systems can also be used to perform estimate of organic carbon and total carbon in the soil. These findings are helpful in using less expensive equipment to carry out higher-efficient future spectrum prediction on the organic carbon and total carbon in the soil [18]. In order to satisfy the demand for more complicated analysis technology, the pharmaceutical industry has used the spectrum screening technology that can be deployed at site to check the medicines that contain the elements of adulterated drugs or drugs with alternative risks [19]. Double beam spectrophotometer was used to measure site reflectance, and several sets of Analytical Spectral Devices (ASD) visible- near infrared spectroscopy were required during the process. The testing result demonstrated the necessity of relative calibration among ASD spectroscopes [20]. The data presented by ASD visible- near infrared spectroscopy has better precision. In a further study, he standard deviation of the aerial measurements was six times smaller than the hand-held measurements. Low-altitude unmanned vehicle high spectrum data was used to carry out fast and large-scale monitoring on the heavy metal content in the soil [21]. The metabolism spectrum diagram of hydrolat points out the different potential usage as the functional elements of the products in food, beverage, and cosmetic industries based on a comparison of volatile components [22]. In the biotechnology pharmaceutical industry, near infrared spectrum\ is a powerful tool for pharmaceutical forensic science. It has been used to test the discrimination between false and true artesunate antimalarial drugs [23]. Near infrared spectrum has been used to develop qualitative and quantitative methods with non-invasive modes to monitor wet granulation process. The spectrum data has been used to establish qualitative multiple modes based on the principal component analysis. The qualitative mode only uses spectrum data to monitor different procedures in granulation process [24]. Reflectance spectroscopy was used to estimate the biochemical compound concentration (tea polyphenols and total amount of free amino acid) related to the mass of tea (Camellia sinensis (L.). Hyperspectral remote sensing is useful for lateral monitoring on chemical elements intea leaves [25]. As indicated in previous studies, an accurate and precise spectrum measure demonstrates the characteristics of the target material [26]. The first step of developing aflatoxin smart sorter is to confirm the critical wavelength of aflatoxin testing [27]. The reflection measurement carried out by ASD was used to predict the performance of chlorophyll on leaves [28]. The near-field optical instrument was used to obtain water quality parameter for monitoring and verifying optical satellite data [29]. According to the analysis results on the six type of hydrolats, the experimental results showed that better absorption value appears at 290~400 nm in Taiwan sandalwood hydrolat and Taiwan cypress hydrolat. Thus, they could be added to cosmetic products in the future for sun protection [30].
The monoterpenoids in the basic Chamaecyparis Obtusa oil were studied to confirm the presence of monoterpenoids in essential oils, by using gas chromatography, ultraviolet method and infrared absorption spectroscopy [31]. The spectrum of the neutral glycolipid content in the hydrolysis products revealed the existence of xylooligosaccharide, except for d-Xyl with degree of polymerization between 2 and 8. The Arabinoglucuronoxylans (AGX) from Chinese fir and H wood contains unsubstituted chain, and is consisted of at least 8 d-Xyl residues. Chemical equilibrium model and ultraviolet-visible spectrophotometer were used to estimate the copper adsorption amount on humic acid without further instrument analysis or copper adsorption isothermal experiment [32]. A mobile laser inductive fluorescence spectrometry laser was developed to monitor the pollen floating in the air. The fluorescence spectrometry was used to measure the fluorescence spectrum of pollen released at 355 nm, and the result revealed that a peak usually appeared at 460 nm in the range of 400–600 nm [33]. Spectrum analysis and texture analysis were also adopted to extract target forest species based on color spectrophotometry [34]. However, the apparent didecyldimethylammonium chloride (DDAC) concentration on Japanese larch and western hemlock higher than the actual value was solved at 477 nm. The method also provided accurate values [35]. Scholars clarified the structure on the new compounds through spectrum methods [36].
The process does not destroy the samples and the operator can be trained rapidly and easily. Each constituent of the sample has different reflections in different spectra, these data (or spectra) can be used for identifying the constituents [37]. Amelie et al. studied fake black pepper [38], and others used honey spectrum and machine learning for rapid sieving analysis of locally representative longan and lychee honey [39].
The high-accuracy spectral radiance metering system is established according to the spectral radiance characteristic of the deuterium lamp. The consistency of spectral distribution is better than ± 5% v/v within 165~300 nm [40]. The relative spectral distributions of three spectral radiance standard neon light sources were compared and the imported error was1.7% v/v [41]. The spectrum value was about 40 and different fragrance samples showed that they can be distinguished clearly [42]. The combination of Fourier transform infrared spectral technique and multivariate analysis can analyze wood samples without time-consuming sample preparation [43]. Two methods have been developed for quantifying melamine. The correlation coefficient (R^2) values obtained by univariate analysis using CN emission spectrum band and multivariate calibration NN model are 0.982 and 0.999 respectively [44].
The spectrographic technique of the micro spectrophotometer can be quickly and easily implemented [45]. The original theoretical basis of this study is the findings of scholars. The UV absorption capacity of the hydrolat was evaluated by using a UV-vis spectrophotometer. Taiwan cypress hydrolat had better absorption at 290~400 nm. Another study used the PLSR model of 350~400 nm band for rapidly filtering low concentration cypress hydrolat samples.
The experimental method can clarify the causality between variables in social science [46]. In this study, the product source of Chamaecyparis obtusa var. formosana was first confirmed, and the cypress hydrolat was distilled from the confirmed sample. The hydrolat was delivered to the notarial unit to measure the proportion of components by GC-MS. The cypress hydrolat of the same sample was diluted to 50% v/v, 25% v/v, and pure water 0% v/v.
The four samples were tested by a simple spectrophotometer and the data were acquired. The four groups of data were used for regression analysis analysis. The optimal correlation coefficient and R square wavelength interval were obtained, and then all band and optimum correlation coefficient and R square wavelength interval were used for regression statistics. The all band and optimum correlation coefficient and R square wavelength interval were compared for regression statistic of correlation.
This study used different spectral wavelengths 180~1100 nm for further discussion, in order to determine the obvious difference between wavelengths using a simple spectrophotometer. A low-cost and high-efficiency rapid sieving analysis technique was developed for managing the quality of distilled cypress hydrolat.
The experimental was based on the "fruit of the poisonous tree doctrine", meaning that if the source is contaminated, the collected samples should also be deemed contaminated, and thus discarded [47]. As there are numerous kinds of wood, identifying cypress wood has to be based experience and wood smell is risky to some extent. If the source is not confirmed, the samples could not be used for the experiment. Thus, to confirm the wood gene, the cypress wood sample was sent to the laboratory of the Forestry Bureau, and the formal report confirmed the cypress wood (Figure 1).
The measuring instrument used in this study was a simple micro spectrophotometer produced by Taiwan OTO Photonics:
1) Wavelength: 180–1100 nm
2) Entrance slit: 25 um wide slit
3) Spectral Resolution: 1.2 nm FWHM
4) A/D Resolution: 16 bits
5) Integration time: 0.21 ms ~ user define
6) Fiber Optics Connector: SMA905
7) Interface: USB2.0@480Mbps (High speed)
8) Power Supply: Default USB Power
9) Dimensions: 86(W) x 110(D) x 34.6(H) mm
10) CMOS Sensor SNR: 330: 1
The instrument serial number is Model SE1030-025-FUV (Table 1)
SpectraSmart Spectrum | |
Spectro-Module Serial Number : | OS361AC55012029 |
After the source of cypress wood was confirmed, the cypress wood was processed to obtain hydrolat. The single sample of cypress hydrolat was sent to Forestry Bureau to test the composition by GC-MS. The cypress hydrolat composition analysis was obtained (Figures 2 and 3). As seen, α-Terpineol-35.05% v/v, Borneol-19.92% v/v, Cornphor-8.55% v/v, Fenchyl Alcohol-6.92% v/v, Terpinen-4-ol-5.59% v/v, Dihydroterpineol-4.25% v/v, Fenchone-3.06% v/v.
The hydrolat was tested by spectrophotometer according to raw liquor 100, 50, 25 and 0% v/v. The absorbance data are shown in Figure 4.
The correlation coefficient represents the degree of linear correlation between two variables [48]. The determination coefficient (R2), also known as the multiple correlation coefficient is well established in classic regression analysis [49]. Many test units still use R2 or r as the discrimination standard of correction equation [50]. The value of the correlation coefficient R is ± 1. When R is 0, the variables are uncorrelated. When R is larger than 0, variables are positively correlated. When R is smaller than 0, the variables are negatively correlated. When R is equal to 1 or -1, the variables are correlated.
In this study, the value of R square is 0~1 and the R square of the regression model is equal to 0.7, and the interpretability of this regression model for prediction result is 70%. The R square is larger than 0.75, suggesting that the model has goodness-of–fit and high interpretability.
The 100/50/25/0% v/v cypress hydrolat sample was tested by Model SE1030-025-FUV simple micro spectrophotometer. The test wavelength interval is 180–1100 nm, and the absorbance data are shown in Figures 4 and 5. As seen, the wavelength of 180–350 nm has an excellent interval difference.
The obtained cypress hydrolat samples were arranged according to 0/25/50/100% v/v, and the R square was calculated by 0% v/v basic point The computing equation is as follows.
R2=SSReg/SSTotal | (1) |
SSReg is the amount of variation of the regression model. The SSReg computing equation is
SSReg=∑(⌢yi−ˉy)2 | (2) |
⌢yi is the prediction value of the regression model at point yi. ˉy is the average of all yi values.
SSTotal is the amount of variation of yi value and the SSTotal computing equation is
SSTotal=∑(yi−ˉy)2 | (3) |
The calculation results of cypress hydrolat 0/25/50/100% v/v ar.
The R correlation coefficient is 0.95, the R square value is 0.92, (Software calculation version: Microsoft Excel professional plus 2013). The regression significance value is 2.98E-78α < 0.05, the intercept P-value is 2.72E-55, the X1 variable 25% v/v P-value is 2.26E-45, the X2 variable 50% v/v P-value is 4.87E-21, the X3 variable 100% v/v P-value is 0.000671. The aforesaid dependent and independent variables are in a linear relationship. The result shows that there is a considerably fixed ratio between dependent and independent variables.
The regression equation is Y = a X + b
This study used cypress hydrolat 0% v/v as Y as a dependent variable, and 25, 50 and 100% v/v as independent variables. According to the 180–1100 nm regression statistic calculated by Excel 2013, the R correlation coefficient is 0.756864, the R square value is 0.572844, the regression significance value is 7.7E-169, the intercept P-value is 1.24E-18, the X1 variable 25% v/v P-value is 2.19E-50, the X2 variable 50% v/v P-value is 4.68E-11, the X3 variable 100% v/v P-value is 0.014315, as shown in Tables 2–4.
180~1100 nm Regression statistics | |
R related | 0.756864 |
R squared | 0.572844 |
Adjust R | 0.571446 |
Difference | 0.054024 |
Number measurements | 921 |
ANOVA Degree of freedom | SS | MS | F | Significant data | |
Regression | 3 | 3.589213 | 1.196404 | 409.9185 | 7.70E-169 |
Residuals | 917 | 2.676393 | 0.002919 | ||
sum | 920 | 6.2656.6 |
Coefficient | Difference | t statistics | P-value | |
Intercept | -0.01779 | 0.001976 | -9.00321 | 1.24E-18 |
X Variable 1 | 0.432843 | 0.027246 | 15.88665 | 2.19E-50 |
X Variable 2 | -0.23439 | 0.035188 | -6.66122 | 4.68E-11 |
X Variable 3 | 0.0371 | 0.015119 | 2.453937 | 1.43E-02 |
The R correlation coefficient is 0.756864, the R square is below 0.75, the regression significance value is 7.7E-169α < 0.05, the intercept P-value is 1.24E-18, the X1 variable 25% v/v P-value is 2.19E-50, the X2 variable 50% v/v P-value is 4.68E-11, the X3 variable 100% v/v P-value is 0.014315. The aforesaid dependent and independent variables are not in a linear relationship, as shown in Figure 10.
This study used cypress hydrolat 0% v/v as Y dependent variable, and 25, 50 and 100% v/v as independent variables. According to the 205–350 nm regression statistic calculated by Excel 2013, the R correlation coefficient value is 0.95998, the R square value is 0.921561, the regression significance value is 2.98E-78, the intercept P-value is 2.72E-55, the X1 variable 25% v/v P-value is 2.26E-45, the X2 variable 50% v/v P-value is 4.87E-21, the X3 variable 100% v/v P-value is 0.000671, as shown in Tables 5–7.
205~350 nm Regression statistics | |
R related | 0.95998 |
R squared | 0.921561 |
Adjust R | 919904 |
Difference | 0.013797 |
Number measurements | 146 |
ANOVA | Degree of freedom | SS | MS | F | Significant data |
Regression | 3 | 0.317571 | 0.105857 | 556.1108 | 2.98E-78 |
Residuals | 142 | 0.02703 | 0.00019 | ||
Sum | 145 | 0.344601 |
Coefficient | Difference | t statistics | P-value | |
Intercept | 0.057262 | 0.002227 | 25.71488 | 2.72E-55 |
X Variable 1 | 0.414892 | 0.019765 | 20.99164 | 2.26E-45 |
X Variable 2 | -0.19317 | 0.017379 | -11.1146 | 4.09E-18 |
X Variable 3 | -0.01967 | 0.005656 | -3.47794 | 0.000671 |
The R correlation coefficient is 0.95998, the R square value is 0.921561, the regression significance value is 2.98E-78α < 0.05, the intercept P-value is 2.72E-55, the X1 variable 25% v/v P-value is 2.26E-45, the X2 variable 50% v/v P-value is 4.87E-21, the X3 variable 100% v/v P-value is 0.000671. The aforesaid dependent variables and independent variables are in a linear relationship. The result shows that there is a relationship of a considerably fixed ratio between the dependent variable and independent variables, as shown in Figure 11.
This study compared a simple spectrometer with GC-MS in the analysis of the composition of pure dew. To discriminate the concentrations of cypress hydrolat samples, the cypress hydrolat was diluted with pure water to liquids of 100, 50, 25% v/v, and pure water 0% v/v according to raw liquor. The samples were tested by a simple spectrophotometer. The cypress hydrolat 0% v/v is Y, a dependent variable, 25, 50 and 100% v/v are independent variables. According to 205–350 nm regression statistic calculated by Excel 2013, the R correlation coefficient value is 0.95998, the R square value is 0.921561, the regression significance value is 2.98E-78, the intercept P-value is 2.72E-55, the X1 variable 25% v/v P-value is 2.26E-45, the X2 variable 50% v/v P-value is 4.87E-21, the X3 variable 100% v/v P-value is 0.000671. The aforesaid dependent variable and independent variables are in a linear relationship. The result shows a relationship of a considerably fixed ratio between the dependent variable and independent variables.
The experiment results of this study suggested that the absorbance of cypress hydrolat on wavelength between 205~350 nm spectrum have a linear relation to its concentration. This linear relation also suggests that a simple spectrophotometer could be sufficient to develop a low-cost, and high-efficiency rapid sieving analysis technique for managing the quality of distilled cypress hydrolat.
To obtain more accurate concentration data, some work and cost will be devoted to reproducibility tests and multi-batch tests in the future, and the quantitative prediction accuracy may be further increased.
This paper first appeared at the EIHP International Symposium in 2021 and is a revised version of the paper published at the Taiwan-Nantou County Conference in July 2021. The authors thank the organizer and Professor Yin for their guidance.
The authors declare no conflict of interest
[1] | R. Stupp, W. P. Mason, M. J. Van Den Bent, M. Weller, B. Fisher, M. J. B. Taphoorn, et al., Radiotherapy plus concomitant and adjuvant temozolomide for glioblastoma, N. Engl. J. Med., 352 (2005), 987-996. |
[2] | R. Stupp, M. E. Hegi, W. P. Mason, M. J. Van Den Bent, M. J. B. Taphoorn, R. C. Janzer, et al., Effects of radiotherapy with concomitant and adjuvant temozolomide versus radiotherapy alone on survival in glioblastoma in a randomised phase iii study: 5-year analysis of the eortc-ncic trial, Lancet Oncol., 10 (2009), 459-466. |
[3] | R. Bonavia, W. K. Cavenee, F. B. Furnari, Heterogeneity maintenance in glioblastoma: a social network, Cancer Res., 71 (2011), 4055-4060. |
[4] | Z. An, O. Aksoy, T. Zheng, Q.-W. Fan, W. A. Weiss, Epidermal growth factor receptor and egfrviii in glioblastoma: signaling pathways and targeted therapies, Oncogene, 37 (2018), 1561-1575. |
[5] | M. Nakada, D. Kita, T. Watanabe, Y. Hayashi, J.-i. Hamada, The mechanism of chemoresistance against tyrosine kinase inhibitors in malignant glioma, Brain Tumor Pathol., 31 (2014), 198-207. |
[6] |
N. J. Szerlip, A. Pedraza, D. Chakravarty, M. Azim, J. McGuire, Y. Fang, et al., Intratumoral heterogeneity of receptor tyrosine kinases egfr and pdgfra amplification in glioblastoma defines subpopulations with distinct growth factor response, Proc. Natl. Acad. Sci. U.S.A., 109 (2012), 3041-3046. doi: 10.1073/pnas.1114033109
![]() |
[7] | L. S. Hu, S. Ning, J. M. Eschbacher, L. C. Baxter, N. Gaw, S. Ranjbar, et al., Radiogenomics to characterize regional genetic heterogeneity in glioblastoma, Neuro-oncology, 19 (2017), 128-137. |
[8] | S. J. Smith, M. Diksin, S. Chhaya, S. Sairam, M. A. Estevez-Cebrero, R. Rahman, The invasive region of glioblastoma defined by 5ala guided surgery has an altered cancer stem cell marker profile compared to central tumour, Int.J. Mol. Sci., 18 (2017), 2452. |
[9] | A. Sottoriva, I. Spiteri, S. G. Piccirillo, A. Touloumis, V. P. Collins, J. C. Marioni, et al., Intratumor heterogeneity in human glioblastoma reflects cancer evolutionary dynamics, Proc. Natl. Acad. Sci. U.S.A., 110 (2013), 4009-4014. |
[10] | J. G. Lyons, E. Lobo, A. M. Martorana, M. R. Myerscough, Clonal diversity in carcinomas: its implications for tumour progression and the contribution made to it by epithelial-mesenchymal transitions, Clin. Exp. Metastasis, 25 (2008), 665-677. |
[11] |
C. Lopez-Gines, R. Gil-Benso, R. Ferrer-Luna, R. Benito, E. Serna, J. Gonzalez-Darder, et al., New pattern of egfr amplification in glioblastoma and the relationship of gene copy number with gene expression profile, Mod. Pathol., 23 (2010), 856-865. doi: 10.1038/modpathol.2010.62
![]() |
[12] | F. B. Furnari, T. F. Cloughesy, W. K. Cavenee, P. S. Mischel, Heterogeneity of epidermal growth factor receptor signalling networks in glioblastoma, Nat. Rev. Cancer, 15 (2015), 302. |
[13] | B. R. Voldborg, L. Damstrup, M. Spang-Thomsen, H. S. Poulsen, Epidermal growth factor receptor (egfr) and egfr mutations, function and possible role in clinical trials, Ann. Oncol., 8 (1997), 1197-1206. |
[14] | J. J. Parker, K. R. Dionne, R. Massarwa, M. Klaassen, N. K. Foreman, L. Niswander, et al., Gefitinib selectively inhibits tumor cell migration in egfr-amplified human glioblastoma, Neurooncology, 15 (2013), 1048-1057. |
[15] | K. M. Talasila, A. Soentgerath, P. Euskirchen, G. V. Rosland, J. Wang, P. C. Huszthy, et al., Egfr wild-type amplification and activation promote invasion and development of glioblastoma independent of angiogenesis, Acta Neuropathol., 125 (2013), 683-698. |
[16] | N. Shinojima, K. Tada, S. Shiraishi, T. Kamiryo, M. Kochi, H. Nakamura, et al., Prognostic value of epidermal growth factor receptor in patients with glioblastoma multiforme, Cancer Res., 63 (2003), 6962-6970. |
[17] | A. Alentorn, Y. Marie, C. Carpentier, B. Boisselier, M. Giry, M. Labussiere, et al., Prevalence, clinico-pathological value, and co-occurrence of pdgfra abnormalities in diffuse gliomas, Neurooncology, 14 (2012), 1393-1403. |
[18] | P. Blume-Jensen, T. Hunter, Oncogenic kinase signalling, Nature, 411 (2001), 355 |
[19] | Cancer Genome Atlas Research Network, Comprehensive genomic characterization defines human glioblastoma genes and core pathways, Nature, 455 (2008), 1061. |
[20] | M. M. Lino, A. Merlo, Pi3kinase signaling in glioblastoma, J. Neurooncol., 103 (2011), 417-427. |
[21] | M. Snuderl, L. Fazlollahi, L. P. Le, M. Nitta, B. H. Zhelyazkova, C. J. Davidson, et al., Mosaic amplification of multiple receptor tyrosine kinase genes in glioblastoma, Cancer Cell, 20 (2011), 810-817. |
[22] | F. Chen, L. Ding, Co-survival of the fittest few: mosaic amplification of receptor tyrosine kinases in glioblastoma, Genome Biol., 13 (2012), 141. |
[23] | M. J. Borad, M. D. Champion, J. B. Egan, W. S. Liang, R. Fonseca, A. H. Bryce, et al., Integrated genomic characterization reveals novel, therapeutically relevant drug targets in fgfr and egfr pathways in sporadic intrahepatic cholangiocarcinoma, PLoS Genet., 10, e1004135. |
[24] | D. W. Craig, J. A. O'Shaughnessy, J. A. Kiefer, J. Aldrich, S. Sinari, T. M. Moses, et al., Genome and transcriptome sequencing in prospective metastatic triple-negative breast cancer uncovers therapeutic vulnerabilities, Mol. Cancer Ther., 12 (2013), 104-116. |
[25] | R. Mehrian-Shai, M. Yalon, I. Moshe, I. Barshack, D. Nass, J. Jacob, et al., Identification of genomic aberrations in hemangioblastoma by droplet digital pcr and snp microarray highlights novel candidate genes and pathways for pathogenesis, BMC Genom., 17 (2016), 56. |
[26] | J. C. L. Alfonso, K. Talkenberger, M. Seifert, B. Klink, A. Hawkins-Daarud, K. R. Swanson, et al., The biology and mathematical modelling of glioma invasion: a review, J. R. Soc. Interface, 14 (2017), 20170490. |
[27] |
K. R. Swanson, H. L. P. Harpold, D. L. Peacock, R. Rockne, C. Pennington, L. Kilbride, et al., Velocity of radial expansion of contrast-enhancing gliomas and the effectiveness of radiotherapy in individual patients: a proof of principle, Clin. Oncol., 20 (2008), 301-308. doi: 10.1016/j.clon.2008.01.006
![]() |
[28] | K. R. Swanson, R. C. Rostomily, E. C. Alvord Jr, Confirmation of a theoretical model describing the relative contributions of net growth and dispersal in individual infiltrating gliomas, Can. J. Neurol. Sci., 30 (2003), 407-434. |
[29] | K. R. Swanson, R. C. Rostomily, E. C. Alvord Jr, A mathematical modelling tool for predicting survival of individual patients following resection of glioblastoma: a proof of principle, Br. J. Cancer, 98 (2008), 113-119. |
[30] | K. R. Swanson, E. C. Alvord, J. D. Murray, Virtual brain tumours (gliomas) enhance the reality of medical imaging and highlight inadequacies of current therapy, Br. J. Cancer, 86 (2002), 14-18. |
[31] | K. R. Swanson, E. C. Alvord Jr, J. D. Murray, A quantitative model for differential motility of gliomas in grey and white matter, Cell Prolif., 33 (2000), 317-329. |
[32] | K. R. Swanson, C. Bridge, J. D. Murray, E. C. Alvord Jr, Virtual and real brain tumors: using mathematical modeling to quantify glioma growth and invasion, J. Neurosci., 216 (2003), 1-10. |
[33] | A. L. Baldock, S. Ahn, R. Rockne, S. Johnston, M. Neal, D. Corwin, et al., Patient-specific metrics of invasiveness reveal significant prognostic benefit of resection in a predictable subset of gliomas, PLoS One, 9, e99057. |
[34] | P. R. Jackson, J. Juliano, A. Hawkins-Daarud, R. C. Rockne, K. R. Swanson, Patient-specific mathematical neuro-oncology: using a simple proliferation and invasion tumor model to inform clinical practice, Bull. Math. Biol., 77 (2015), 846-856. |
[35] | C. H. Wang, J. K. Rockhill, M. Mrugala, D. L. Peacock, A. Lai, K. Jusenius, et al., Prognostic significance of growth kinetics in newly diagnosed glioblastomas revealed by combining serial imaging with a novel biomathematical model, Cancer Res., 69 (2009), 9133-9140. |
[36] | K. J. Painter, T. Hillen, Volume-filling and quorum-sensing in models for chemosensitive movement, Can. Appl. Math. Quart, 10 (2002), 501-543. |
[37] | P. Gerlee, S. Nelander, The impact of phenotypic switching on glioblastoma growth and invasion, PLoS Comput. Biol., 8, e1002556. |
[38] | K. R. Swanson, R. C. Rockne, J. Claridge, M. A. Chaplain, E. C. Alvord, A. R. A. Anderson, Quantifying the role of angiogenesis in malignant progression of gliomas: in silico modeling integrates imaging and histology, Cancer Res., 71 (2011), 7366-7375. |
[39] | S. M. Blower, H. Dowlatabadi, Sensitivity and uncertainty analysis of complex models of disease transmission: an hiv model, as an example, International Statistical Review/Revue Internationale de Statistique, 229-243. |
[40] | A. Hawkins-Daarud, S. K. Johnston, K. R. Swanson, Quantifying uncertainty and robustness in a biomathematical model-based patient-specific response metric for glioblastoma, JCO Clin. Cancer Inform., 3 (2019), 1-8. |
[41] | S. Marino, I. B. Hogue, C. J. Ray, D. E. Kirschner, A methodology for performing global uncertainty and sensitivity analysis in systems biology, J. Theor. Biol., 254 (2008), 178-196. |
[42] | S. C. Massey, J. C. Urcuyo, B. M. Marin, J. N. Sarkaria, K. R. Swanson, Quantifying glioblastoma drug response dynamics incorporating resistance and blood brain barrier penetrance from experimental data, Front. Physiol., In Press. |
[43] | C. A. Smith, C. A. Yates, The auxiliary region method: a hybrid method for coupling pde-and brownian-based dynamics for reaction-diffusion systems, Royal Soc. Open Sci., 5 (2018), 180920. |
1. | Md. Shimul Bhuia, Raihan Chowdhury, Meher Afroz, Md. Showkot Akbor, Md. Sakib Al Hasan, Jannatul Ferdous, Rubel Hasan, Marcus Vinícius Oliveira Barros de Alencar, Mohammad S. Mubarak, Muhammad Torequl Islam, Therapeutic Efficacy Studies on the Monoterpenoid Hinokitiol in the Treatment of Different Types of Cancer, 2025, 1612-1872, 10.1002/cbdv.202401904 |
SpectraSmart Spectrum | |
Spectro-Module Serial Number : | OS361AC55012029 |
180~1100 nm Regression statistics | |
R related | 0.756864 |
R squared | 0.572844 |
Adjust R | 0.571446 |
Difference | 0.054024 |
Number measurements | 921 |
ANOVA Degree of freedom | SS | MS | F | Significant data | |
Regression | 3 | 3.589213 | 1.196404 | 409.9185 | 7.70E-169 |
Residuals | 917 | 2.676393 | 0.002919 | ||
sum | 920 | 6.2656.6 |
Coefficient | Difference | t statistics | P-value | |
Intercept | -0.01779 | 0.001976 | -9.00321 | 1.24E-18 |
X Variable 1 | 0.432843 | 0.027246 | 15.88665 | 2.19E-50 |
X Variable 2 | -0.23439 | 0.035188 | -6.66122 | 4.68E-11 |
X Variable 3 | 0.0371 | 0.015119 | 2.453937 | 1.43E-02 |
205~350 nm Regression statistics | |
R related | 0.95998 |
R squared | 0.921561 |
Adjust R | 919904 |
Difference | 0.013797 |
Number measurements | 146 |
ANOVA | Degree of freedom | SS | MS | F | Significant data |
Regression | 3 | 0.317571 | 0.105857 | 556.1108 | 2.98E-78 |
Residuals | 142 | 0.02703 | 0.00019 | ||
Sum | 145 | 0.344601 |
Coefficient | Difference | t statistics | P-value | |
Intercept | 0.057262 | 0.002227 | 25.71488 | 2.72E-55 |
X Variable 1 | 0.414892 | 0.019765 | 20.99164 | 2.26E-45 |
X Variable 2 | -0.19317 | 0.017379 | -11.1146 | 4.09E-18 |
X Variable 3 | -0.01967 | 0.005656 | -3.47794 | 0.000671 |
SpectraSmart Spectrum | |
Spectro-Module Serial Number : | OS361AC55012029 |
180~1100 nm Regression statistics | |
R related | 0.756864 |
R squared | 0.572844 |
Adjust R | 0.571446 |
Difference | 0.054024 |
Number measurements | 921 |
ANOVA Degree of freedom | SS | MS | F | Significant data | |
Regression | 3 | 3.589213 | 1.196404 | 409.9185 | 7.70E-169 |
Residuals | 917 | 2.676393 | 0.002919 | ||
sum | 920 | 6.2656.6 |
Coefficient | Difference | t statistics | P-value | |
Intercept | -0.01779 | 0.001976 | -9.00321 | 1.24E-18 |
X Variable 1 | 0.432843 | 0.027246 | 15.88665 | 2.19E-50 |
X Variable 2 | -0.23439 | 0.035188 | -6.66122 | 4.68E-11 |
X Variable 3 | 0.0371 | 0.015119 | 2.453937 | 1.43E-02 |
205~350 nm Regression statistics | |
R related | 0.95998 |
R squared | 0.921561 |
Adjust R | 919904 |
Difference | 0.013797 |
Number measurements | 146 |
ANOVA | Degree of freedom | SS | MS | F | Significant data |
Regression | 3 | 0.317571 | 0.105857 | 556.1108 | 2.98E-78 |
Residuals | 142 | 0.02703 | 0.00019 | ||
Sum | 145 | 0.344601 |
Coefficient | Difference | t statistics | P-value | |
Intercept | 0.057262 | 0.002227 | 25.71488 | 2.72E-55 |
X Variable 1 | 0.414892 | 0.019765 | 20.99164 | 2.26E-45 |
X Variable 2 | -0.19317 | 0.017379 | -11.1146 | 4.09E-18 |
X Variable 3 | -0.01967 | 0.005656 | -3.47794 | 0.000671 |