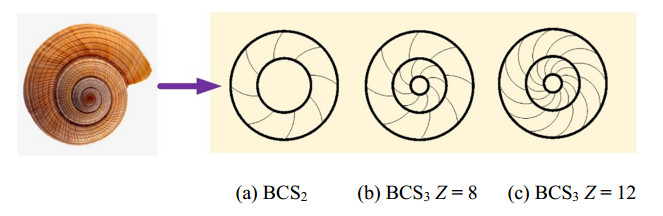
Citation: Haijun Hu, Xupu Yuan, Lihong Huang, Chuangxia Huang. Global dynamics of an SIRS model with demographics and transfer from infectious to susceptible on heterogeneous networks[J]. Mathematical Biosciences and Engineering, 2019, 16(5): 5729-5749. doi: 10.3934/mbe.2019286
[1] | Dongtao Wang, Ping Xu, Chengxing Yang, Shuguang Yao, Zhen Liu . Crashworthiness performance of gradient energy-absorbing structure for subway vehicles under quasi-static loading. Electronic Research Archive, 2023, 31(6): 3568-3593. doi: 10.3934/era.2023181 |
[2] | Chao Ma, Hong Fu, Pengcheng Lu, Hongpeng Lu . Multi-objective crashworthiness design optimization of a rollover protective structure by an improved constraint-handling technique. Electronic Research Archive, 2023, 31(7): 4278-4302. doi: 10.3934/era.2023218 |
[3] | Laiyu Liang, Huaiming Zhu, Dong Wei, Yaozhong Wu, Weijia Li . Energy absorption and multi-objective optimization of TPMS filled cylinder shell structures. Electronic Research Archive, 2023, 31(5): 2834-2854. doi: 10.3934/era.2023143 |
[4] | Kai Zheng, Zengshen Ye, Fanchao Wang, Xi Yang, Jianguo Wu . Custom software development of structural dimension optimization software for the buckling of stiffened plates. Electronic Research Archive, 2023, 31(2): 530-548. doi: 10.3934/era.2023026 |
[5] | Yuhai Zhong, Huashan Feng, Hongbo Wang, Runxiao Wang, Weiwei Yu . A bionic topology optimization method with an additional displacement constraint. Electronic Research Archive, 2023, 31(2): 754-769. doi: 10.3934/era.2023037 |
[6] | Xiang Xu, Chuanqiang Huang, Chongchong Li, Gang Zhao, Xiaojie Li, Chao Ma . Uncertain design optimization of automobile structures: A survey. Electronic Research Archive, 2023, 31(3): 1212-1239. doi: 10.3934/era.2023062 |
[7] | Furong Xie, Yunkai Gao, Ting Pan, De Gao, Lei Wang, Yanan Xu, Chi Wu . Novel lightweight connecting bracket design with multiple performance constraints based on optimization and verification process. Electronic Research Archive, 2023, 31(4): 2019-2047. doi: 10.3934/era.2023104 |
[8] | Wuhua Jiang, Yuexin Zhang, Jie Liu, Daisheng Zhang, Yajie Yan, Chuanzheng Song . Multi-objective optimization design for steel-aluminum lightweight body of pure electric bus based on RBF model and genetic algorithm. Electronic Research Archive, 2023, 31(4): 1982-1997. doi: 10.3934/era.2023102 |
[9] | Donghyeon Kim, Jinsung Kim . GPU-accelerated non-dominated sorting genetic algorithm III for maximizing protein production. Electronic Research Archive, 2024, 32(4): 2514-2540. doi: 10.3934/era.2024116 |
[10] | Mingtao Cui, Wennan Cui, Wang Li, Xiaobo Wang . A polygonal topology optimization method based on the alternating active-phase algorithm. Electronic Research Archive, 2024, 32(2): 1191-1226. doi: 10.3934/era.2024057 |
To strengthen the protection of passengers in traffic accidents, realize the lightweight and improve the impact energy absorption efficiency, thin-walled structures have been widely used as energy absorbers during the past two decades. For example, Wierzbicki and Abramowicz [1], Krolak et al. [2], Langseth et al. [3] and Xiang et al. [4] studied the energy absorption characteristics of single-walled columns under axial impact loading. It was found that the energy absorption capacity of the single-walled structure is still insufficient and prone to Euler buckling, which greatly reduces the energy absorption efficiency of thin-walled structures. Many teams have conducted academic research on multi-cell thin-walled structures through experiments [5,6,7,8], simulations [9,10,11,12], and theoretical analyses [13,14,15]. For example, Zhang et al. [16] analyzed the axial impact energy absorption of different square multi-cell aluminum tubes with the same mass and found that the multi-cell structure with more walls can effectively improve the energy absorption efficiency. Jusuf et al. [17] studied the impact resistance of single-walled, double-walled and multi-cell columns under dynamic axial loading by means of numerical analysis and experimental research. The results showed that adding internal ribs between the walls of double-walled columns is beneficial to improve the impact resistance of multi-cell columns. It was found that the multi-cell properties of thin-walled structures could improve their energy absorption. Moreover, the energy dissipation of thin-walled structures is mainly concentrated at the corners of thin-walled structures [1,18], and forming a 90° angle at corners can effectively improve the average crushing force and energy absorption [19]. Chen et al. [18] proposed simplified super folding element (SSFE) theory, and deduced the theoretical expressions of folding wavelength and mean crushing force of multi-cell columns.
Recently, some researchers found that there are many biological tissues in nature with outstanding mechanical properties and excellent crashworthiness, such as bamboo [20,21,22,23,24,25], beetle elytra [26,27,28], animal horns [29,30,31]. They used bio-inspired theory to optimize the design of thin-walled structures. At the same time, hierarchical designs inspired by bionic design [32,33,34,35,36,37,38,39] have been regarded as one of the effective design methods to improve the crashworthiness of thin-walled structures. For example, Zhang et al. [40,41] proposed a series of hierarchical designs of hexagonal and circular structures. The result revealed that hierarchical structures had higher energy absorption efficiency than single-walled structures. Yin et al. [42] compared the energy absorption of triangular hierarchical honeycomb and conventional honeycomb with the same thickness. The result showed that the hierarchical honeycomb has better axial crashworthiness. Wang et al. [43] investigated the crashworthiness of the vertex-based hierarchical square multi-cell tubes by means of numerical and theoretical analysis, and showed that the hierarchical design can make the deformation mode of multi-cell structures more stable. Zhang et al. [44] established a theoretical model and investigated the energy absorption of the hexagonal hierarchical structure, and found that hierarchical order is an important factor affecting the energy absorption efficiency.
It is found that the multi-cell characteristics of thin-walled structures can enhance their energy absorption performances. The plastic strain and energy dissipation of the thin-walled structure mainly focus on the corner of the structure. In other words, the more corners there are, the more stable the structure will be. How to properly distribute the corner elements in thin-walled structures? — Nature can give us the answer. In this study, novel thin-walled structures are inspired by the spiral hierarchy of the conch. A series of conch-like spiral multi-cell structures are formed by adding corner units of the multi-cell structure with regular lines. Based on the design of conch hierarchical structures, the bionic hierarchical energy-absorbing structures are developed. In addition, on the basis of the bionic conch design, a gradient design of gradual energy absorption was proposed to ensure the acceptable initial peak force and energy absorption efficiency. This design can reduce the initial peak force while ensuring the characteristics of efficient and gradual energy absorption, and effectively improve the safety performance of vehicles.
The novel bionic conch structures (BCS) are shown in Figure 1. The geometric cross section and geometric parameters of the bionic conch structures are shown in Figure 2(a), and the main geometric parameters including wall thickness T, inner ring diameter D1, middle ring diameter D2, the number of inner panels Z1, and the number of outer panels Z2. Figures 2(b) and 2(c) show the geometric cross-section of conventional thin-walled structures, in which Figure 2(b) shows the conventional single-cell tube (CT) and Figure 2(c) shows the conventional multi-cell tube (MCT). Among them, the outermost circle diameter of geometric sections of all thin-walled structures is 60 mm.
The established finite element model of BCS is shown in Figure 3(b). The length of the model is 160 mm, and the bottom is connected with a rigid wall. The thin-walled structure is impacted with a mass of 500 kg and the initial velocity is 10 m/s. The grid size is discretized by BT four-node shell elements of 1 mm × 1 mm. The impact block and rigid wall are defined as rigid bodies, and the rigid support adopts MAT-20 rigid material model in LS-DYNA. Automatic point-surface contact is adopted between the thin-walled structure and the rigid support, and MAT-24 material model is adopted for the thin-walled structure, which is made of aluminum alloy AL6061-O. The basic material parameters of Al6061-O are as follows: density ρ = 2.7×103 kg/m3, Young's modulus E = 71.7 GPa, initial yield stress σy = 98.9 MPa, ultimate stress σu = 169 MPa, and Poisson's ratio μ = 0.3. In this case, automatic single-sided contact is applied to the contact surface to ensure that contact penetration does not occur during compression.
The effective stress-strain curve of AL6061-O is shown in Figure 4. The static and dynamic friction coefficients of contact are 0.3 and 0.2, respectively. In addition, the strain rate effect is ignored in the numerical model because aluminum alloys are not sensitive to strain rate [45].
The main crashworthiness indicators include Energy Absorption (EA), Specific Energy Absorption (SEA), Peak Crushing Force (PCF), and Crushing Load Efficiency (CLE).
SEA is the most critical indicator of energy absorption efficiency, which represents the energy absorption rate of thin-walled structures per unit mass, and can be expressed as:
SEA=EAm | (1) |
where m represents the mass of the structure, EA represents the total energy absorption of the structure in the process of plastic deformation, and its equation is:
EA=∫δ0F(x)dx | (2) |
where δ represents the effective compression displacement. Generally, the effective compression displacement is 70% of the length of the model. F(x) is the instantaneous crushing force. The Peak Crushing Force (PCF) and Mean Crushing Force (MCF) of F(x) are closely related to the safety of passengers, because a high initial peak force will cause serious damage to passengers.
MCF can be expressed as:
MCF=EAδ=∫δ0F(x)dxδ | (3) |
In addition, CLE can evaluate the stability of load in the impact process, and can be expressed as:
CLE = MCFPCF×100% | (4) |
To verify the accuracy of the model, this paper compared the model built by the above method with the simulation results of references. The simulation models CS1 and CS2 established by Chen et al. [46] were highly consistent with the experimental results. Therefore, this paper used the same method to establish the finite element model for the corresponding simulation analysis. The comparison of the deformation modes with the reference and experimental results is shown in Figure 5. The test and simulation results are shown in Table 1, and the results show that the deformation modes, process curves, PCF and SEA results of the model established in this paper are in good consistency with the experimental results of the reference, and the error is less than 1%, which proves the accuracy of the model.
Indicators | Structure | Test | Reference [46] | This paper | Error (%) |
PCF/kN | CS1 | 29.65 | 30.14 | 30.06 | -0.27 |
CS2 | 29.58 | 29.39 | 29.16 | -0.78 | |
SEA/(kJ/kg) | CS1 | 9.74 | 9.29 | 9.22 | -0.73 |
CS2 | 9.55 | 9.35 | 9.34 | -0.13 |
To explore whether the hierarchical fractal of conch structures has obvious advantages in crashworthiness, simulations were carried out on four thin-walled structures of different masses. The relevant structural parameters of BCS2 are: D = 30 mm, Z = 8; The relevant structural parameters of BCS3 are: D1 = 10 mm, D2 = 30 mm, Z1 = Z2 = 8. The simulation results are shown in Table 2.
Structure | m/kg | T/mm | EA /kJ | PCF /kN | SEA/(kJ/kg) | CLE |
CT | 0.110 | 1.35 | 1.73 | 33.31 | 15.73 | 0.47 |
MCT | 0.110 | 0.59 | 1.85 | 32.91 | 16.82 | 0.51 |
BCS2 | 0.110 | 0.60 | 2.07 | 31.21 | 18.82 | 0.60 |
BCS3 | 0.110 | 0.45 | 2.26 | 29.89 | 20.55 | 0.69 |
CT | 0.145 | 1.80 | 2.70 | 46.45 | 18.62 | 0.53 |
MCT | 0.145 | 0.79 | 2.89 | 46.16 | 19.93 | 0.57 |
BCS2 | 0.145 | 0.80 | 3.23 | 44.46 | 22.28 | 0.66 |
BCS3 | 0.145 | 0.60 | 3.38 | 42.33 | 23.31 | 0.73 |
CT | 0.183 | 2.24 | 4.07 | 67.45 | 22.24 | 0.55 |
MCT | 0.183 | 0.98 | 3.99 | 60.72 | 21.80 | 0.60 |
BCS2 | 0.183 | 0.99 | 4.57 | 58.07 | 24.97 | 0.72 |
BCS3 | 0.183 | 0.76 | 4.80 | 56.67 | 26.23 | 0.77 |
CT | 0.213 | 2.65 | 5.23 | 81.05 | 24.55 | 0.59 |
MCT | 0.213 | 1.14 | 5.06 | 72.88 | 23.76 | 0.63 |
BCS2 | 0.213 | 1.15 | 5.62 | 68.55 | 26.38 | 0.75 |
BCS3 | 0.213 | 0.80 | 5.66 | 65.91 | 26.57 | 0.78 |
The comparison of SEA and CLE of four thin-walled structures (Figures 6 and 7) shows that BCS can absorb more energy and have higher energy absorption efficiency under the same mass conditions compared with other conventional structures. Moreover, with the increase of hierarchy order, the efficiency of BCS3 is further enhanced than that of BCS2, and CLE also shows a similar trend, indicating that BCS3 has better load stability. Among them, the SEA of BCS3 (m = 0.110 kg) increased by 30.6 and 22.2% respectively compared with CT and MCT of the same mass, and CLE increased by 46.8 and 35.3% respectively. Figure 7 shows the deformation modes of the four structures after compression. Obviously, the deformation mode of BCS3 is more orderly and the number of folded layers is more than that of other structures, which indicates that this structure has a more reasonable geometric layout and a higher material utilization rate. In addition, the growth rate of SEA and CLE gradually decreases with the increase of mass, so simply increasing the mass is not the optimum way to improve the crashworthiness of the structure.
Figure 8 shows the force-displacement curves of the four thin-walled structures (m = 0.110 kg). It can be seen from the figure that the MCF of BCS3 is significantly higher than that of the other three structures. From this curve, the amplitude of BCS3 is lower, and the energy absorption is more stable. At the same time, the local magnification shows that the PCF of BCS3 is also the lowest among the four structures. Therefore, the CLE is higher and the energy absorption efficiency is better. In summary, BCS3 has two important characteristics of high energy absorption structure.
In addition, it can be seen from Figure 9 (PCF of four thin-walled structures under four groups of the same mass conditions) that although the BCS brings a high absorption effect, it does not bring about a significant increase in PCF, but a certain decrease. The PCF of BCS3 (m = 0.213 kg) is 18.7 and 9.6% lower than that of CT and MCT of the same mass, respectively.
In conclusion, BCS is superior to the conventional thin-walled structures in energy absorption capacity, load stability and peak impact.
Since the conch structures have hierarchical gradients in the axial direction, a bionic conch axial gradient design is established in this paper (the height of the inner ring is 2 mm higher than the secondary outer ring, and the secondary outer ring is 2 mm higher than the outermost ring), as shown in Figure 10. This design can play the role of progressive collapse, effectively reduce the initial peak force, and further improve the impact efficiency of the structure.
Table 3 shows the comparison of crashworthiness between BCS3 initial design and graded gradient design. It can be seen that CLE is increased by 13.04% at most, which proves the effectiveness of the design.
Structure | m/kg | T/mm | EA/kJ | CLE | CLE increase rate | EA decrease rate |
BCS3 | 0.110 | 0.45 | 2.26 | 0.69 | - | - |
0.145 | 0.60 | 3.38 | 0.73 | - | - | |
0.183 | 0.76 | 4.80 | 0.77 | - | - | |
0.213 | 0.80 | 5.66 | 0.78 | - | - | |
Graded gradient design | 0.110 | 0.45 | 2.20 | 0.78 | 13.04% | 2.65% |
0.145 | 0.60 | 3.35 | 0.80 | 9.59% | 0.89% | |
0.183 | 0.76 | 4.68 | 0.81 | 5.19% | 2.50% | |
0.213 | 0.80 | 5.24 | 0.84 | 7.69% | 7.42% |
From the above summary, it can be concluded that BCS can effectively improve the crashworthiness of the structure, and the energy absorption efficiency is further improved with the increase of the conch hierarchy. Further research shows that the crashworthiness of thin-walled structures is closely related to the geometric topological parameters of the section. The geometric parameters of BCS3, including wall thickness T, inner ring diameter D1, middle ring diameter D2, the number of inner panels Z1 and the number of outer panels Z2, all have different degrees of influence on its energy absorption characteristics. To improve the energy absorption capacity of the structure as much as possible under the condition of limited mass, this section will analyze the influence of main geometric parameters on crashworthiness.
To study the influence of inner ring diameter D1 and middle ring diameter D2 on the crashworthiness of BCS, the corresponding simulation analysis was carried out. By changing D1 and D2, the distribution of inner and outer diameters can be adjusted, thereby affecting the folding mode of the structure. Unreasonable structure distribution may lead to structural instability, global buckling, and low material utilization rate, which should be adjusted within a reasonable range.
Firstly, by controlling the variables, set D2 to 30 mm and the range of D1 to {5, 10, 15, 20} mm. To exclude the influence of the number of inner and outer panels and wall thickness, three levels of Z1 = Z2 = Z = {4, 8, 12} (T = 0.8 mm) were set respectively. Table 4 lists the structural crashworthiness parameters and indicators corresponding to changing D1.
D1 /mm | Z1 | Z2 | EA /kJ | PCF /kN | SEA /(kJ/kg) | CLE |
5 | 4 | 4 | 3.36 | 44.83 | 21.77 | 0.68 |
10 | 4 | 4 | 3.43 | 45.60 | 21.74 | 0.68 |
15 | 4 | 4 | 3.38 | 45.76 | 20.96 | 0.67 |
20 | 4 | 4 | 3.84 | 50.08 | 22.93 | 0.70 |
5 | 8 | 8 | 5.18 | 61.19 | 24.95 | 0.77 |
10 | 8 | 8 | 5.24 | 56.46 | 25.06 | 0.84 |
15 | 8 | 8 | 5.26 | 63.44 | 24.87 | 0.75 |
20 | 8 | 8 | 5.57 | 60.93 | 25.58 | 0.83 |
5 | 12 | 12 | 7.19 | 78.49 | 27.56 | 0.83 |
10 | 12 | 12 | 7.06 | 71.65 | 26.72 | 0.90 |
15 | 12 | 12 | 7.08 | 71.53 | 27.10 | 0.90 |
20 | 12 | 12 | 7.54 | 74.22 | 28.13 | 0.92 |
It can be seen from Figure 11 that the SEA of BCS increases significantly with the gradual increase of Z. This is because with the increase of Z, the number of corner elements of the BCS structure will increase, thus increasing the energy absorption concentration area, and SEA has an obvious growth trend. However, with the increase of D1, SEA first decreased and then increased, but the trend was not obvious and fluctuated around ± 1(kJ/kg). In addition, Figure 12 shows the change curve of CLE with D1 and Z, and the same conclusion can be drawn. With the increase of D1, SEA and CLE of BCS showed an upward trend, but it was not obvious.
Next, set D1 to 10mm and the range of D2 to {20, 30, 40, 50}mm using the same control variables method. To exclude the influence of the number of inner and outer panels and wall thickness, Z1 = Z2 = Z = {4, 8, 12} (T = 0.8 mm) were set respectively. Table 5 lists the structural crashworthiness parameters and indicators corresponding to changing D2.
D2 /mm | Z1 | Z2 | EA /kJ | PCF /kN | SEA /(kJ/kg) | CLE |
20 | 4 | 4 | 3.24 | 43.26 | 22.71 | 0.68 |
30 | 4 | 4 | 3.43 | 45.60 | 21.74 | 0.68 |
40 | 4 | 4 | 3.77 | 46.70 | 21.75 | 0.73 |
50 | 4 | 4 | 3.66 | 47.12 | 20.48 | 0.71 |
20 | 8 | 8 | 5.06 | 57.55 | 26.70 | 0.80 |
30 | 8 | 8 | 5.24 | 60.46 | 25.06 | 0.79 |
40 | 8 | 8 | 5.75 | 65.81 | 25.04 | 0.79 |
50 | 8 | 8 | 5.25 | 62.57 | 22.89 | 0.76 |
20 | 12 | 12 | 6.84 | 75.41 | 29.27 | 0.82 |
30 | 12 | 12 | 7.06 | 81.65 | 26.72 | 0.79 |
40 | 12 | 12 | 7.51 | 84.52 | 26.26 | 0.81 |
50 | 12 | 12 | 7.02 | 84.23 | 25.25 | 0.76 |
It can be seen from Figures 13 and 14 that the SEA of BCS gradually decreases with the increase of D2, while CLE did not show the same trend. There was no significant difference between the CLE at Z = 8 and the CLE at Z = 12, indicating that increasing Z is not the only factor to improve the load stability of the structure. In addition, D2 has a greater impact on the crashworthiness of BCS than D1, so it can be expected that D2 will have a greater weight than D1 in the following structural optimization process.
It is known that the crashworthiness of thin-walled structures is closely related to the distribution of mechanical materials, and the type and number of geometric corner elements also play an important role in the energy absorption capacity of structures. Changing the number of inner panels Z1 and the number of outer panels Z2 can change the morphology of the corner elements and effectively increase the number of corner elements. Therefore, it is of great significance to analyze the parameters of Z1 and Z2.
First of all, to exclude the influence of ring diameters, Z1 {4, 6, 8, 10, 12} and Z2 {4, 6, 8, 10, 12} were set respectively on the basis of D1 = 10 mm and D2 = 30 mm to explore the influence of Z1 and Z2 changes. The corresponding results of crashworthiness indicators are listed in Table 6 (T = 0.8 mm).
Z1 | Z2 | EA /kJ | PCF /kN | SEA /(kJ/kg) | CLE |
4 | 4 | 3.43 | 45.60 | 21.74 | 0.68 |
4 | 6 | 4.01 | 52.22 | 23.04 | 0.70 |
4 | 8 | 4.35 | 54.44 | 22.81 | 0.73 |
4 | 10 | 4.88 | 60.00 | 23.55 | 0.74 |
4 | 12 | 5.31 | 65.40 | 23.73 | 0.74 |
6 | 4 | 3.78 | 49.08 | 22.68 | 0.70 |
6 | 6 | 4.28 | 54.74 | 23.34 | 0.74 |
6 | 8 | 4.71 | 57.56 | 23.57 | 0.74 |
6 | 10 | 5.34 | 64.39 | 24.70 | 0.75 |
6 | 12 | 5.68 | 68.70 | 24.38 | 0.75 |
8 | 4 | 4.28 | 49.84 | 24.37 | 0.78 |
8 | 6 | 4.70 | 56.50 | 24.44 | 0.76 |
8 | 8 | 5.24 | 60.46 | 25.06 | 0.84 |
8 | 10 | 5.75 | 65.74 | 25.53 | 0.80 |
8 | 12 | 6.18 | 71.02 | 25.55 | 0.79 |
10 | 4 | 4.69 | 54.94 | 25.36 | 0.78 |
10 | 6 | 5.13 | 60.98 | 25.46 | 0.76 |
10 | 8 | 5.53 | 63.99 | 25.36 | 0.79 |
10 | 10 | 6.09 | 68.02 | 25.99 | 0.81 |
10 | 12 | 6.62 | 74.83 | 26.37 | 0.80 |
12 | 4 | 5.21 | 56.61 | 26.88 | 0.84 |
12 | 6 | 5.69 | 62.69 | 27.01 | 0.82 |
12 | 8 | 6.15 | 66.00 | 27.09 | 0.85 |
12 | 10 | 6.61 | 70.97 | 27.15 | 0.85 |
12 | 12 | 7.06 | 76.65 | 26.72 | 0.84 |
Figure 15 shows the changing trends of the SEA of BCS with Z1 and Z2. It can be seen from the figure that as Z1 increases by one gradient, the SEA of BCS increases by 1.2–7.4% compared with the previous gradient, with an average increase of 4.1%. With the increase of Z2, the SEA of BCS increased by -1.6–5.9% compared with the previous gradient under the same Z1 condition, with an average increase of 1.22%. It can be seen that Z1 can improve the crashworthiness of BCS more effectively than Z2. In addition, when Z1 = Z2 = 12, an obvious inflection point appears in Figure 15. The deformation modes are shown in Figure 16 With the increase of Z2 (Z2 ≤ 10), the folding modes of BCS become more and more regular, and the number of folds increases. When Z2 = 12, the folding mode presents irregular buckling compared to Z2 = 10. In this deformation mode, the effective folding area and the number of effective folding layers are reduced, so the SEA is significantly decreased.
The change of CLE has the same trend. With the increase of Z1 and Z2, the CLE gradually increased, and with the increase of Z2, the increase of BCS was more obvious in the early stage, but the growth in the later stage was relatively gentle.
Based on the studies of a large number of scholars [13,14,15,18,41,44], it can be clearly concluded that the wall thickness T can effectively improve the total energy absorption of the structure, but it will increase the PCF of the structure. Therefore, this paper also verifies this conclusion by several groups of simulations with different T (Table 7).
D1 /mm | D2 /mm | Z1 | Z2 | T | PCF /kN | SEA /(kJ/kg) | CLE |
10 | 20 | 8 | 8 | 0.6 | 41.04 | 23.12 | 0.73 |
10 | 20 | 8 | 8 | 0.8 | 57.55 | 26.70 | 0.80 |
10 | 20 | 8 | 8 | 1 | 77.45 | 29.96 | 0.83 |
10 | 30 | 8 | 8 | 0.6 | 37.84 | 21.37 | 0.80 |
10 | 30 | 8 | 8 | 0.8 | 56.46 | 25.06 | 0.84 |
10 | 30 | 8 | 8 | 1 | 79.03 | 27.00 | 0.81 |
10 | 40 | 8 | 8 | 0.6 | 44.09 | 21.17 | 0.75 |
10 | 40 | 8 | 8 | 0.8 | 65.81 | 25.04 | 0.79 |
10 | 40 | 8 | 8 | 1 | 89.03 | 26.58 | 0.78 |
10 | 50 | 8 | 8 | 0.6 | 43.84 | 19.91 | 0.71 |
10 | 50 | 8 | 8 | 0.8 | 62.57 | 22.89 | 0.76 |
10 | 50 | 8 | 8 | 1 | 82.63 | 26.57 | 0.84 |
To further study the influence of five important geometric parameters D1, D2, Z1, Z2 and T on the crashworthiness of BCS, the significance parameters of PCF and SEA were analyzed by stepwise regression. Step regression is based on the principle of least square method. From one independent variable to all independent variables, each step analyzes whether the model is improved, so as to explore independent variables with significant contributions. After deleting the interaction parameters with less influence, the analysis results are shown in Tables 8 and 9.
PCF | p value | t ratio |
T | < 0.0001* | 23.27 |
Z2 | < 0.0001* | 17.06 |
Z1 | < 0.0001* | 10.17 |
D2 | < 0.0001* | 5.34 |
D2*Z1 | 0.02425 | 2.32 |
D1 | 0.80903 | 0.24 |
SEA | p value | t ratio |
T | < 0.0001* | 18.81 |
Z1 | < 0.0001* | 18.81 |
D2 | < 0.0001* | -11.36 |
Z2 | < 0.0001* | 6.15 |
Z1*Z2 | 0.00012 | -4.17 |
D2*D2 | 0.00553 | 2.90 |
D1*D1 | 0.03324 | 2.19 |
D2*Z1 | 0.05482 | -1.97 |
D1 | 0.86509 | -0.17 |
When the probability value p < 0.05, it indicates that the parameter has a significant effect on PCF and SEA. The absolute value of t ratio can be used to compare the significance of parameters. Therefore, the order of influence of geometric parameters on PCF is T > Z2 > Z1 > D2 >> D1. The p value of D1 > 0.05 indicates that D1 has no significant effect on PCF. For SEA, the order is as follows: Z1 ≈ T > D2 > Z2 >> D1. The effect of D1 on SEA is also not significant.
Based on the validation of the parameter study and significance analysis, the following conclusions can be drawn:
1) With the increase of each geometric parameter, the PCF of BCS gradually increases. The order of influence degree of geometric parameters is: T > Z2 > Z1 > D2 >> D1.
2) The SEA of BCS gradually increases with the increase of other geometric parameters except D2. Therefore, reducing D2 can effectively improve SEA (t ratio = -11.36) and decrease PCF (t ratio = 5.34). The order of influence degree of geometric parameters is: T ≈ Z1 > D2 > Z2 >> D1.
3) D1 has no significant effect on SEA (t ratio = -0.17) and PCF (t ratio = 0.24), which can be ignored and fixed at 10 mm in the subsequent optimization analysis.
4) Increasing Z2 can increase SEA (t ratio = 6.15), but PCF (t ratio = 17.06) also increases significantly. Therefore, increasing Z2 cannot effectively improve the energy absorption efficiency of BCS.
As an important energy-absorbing component of the front bumper, BCS aims to absorb as much energy as possible per unit mass. At the same time, the PCF of the structure is also a key indicator of vehicle safety. It is generally required to be limited within a safe range to reduce the impact acceleration and ensure the safety of passengers. Therefore, this section will further optimize the geometric parameter configuration of BCS through a multi-objective optimization design.
According to the conclusion of the previous section, the influence of D1 is negligible and is set to 10 mm. The minimum value of D2 is set to 15mm to ensure manufacturability. Therefore, the multi-objective optimization model expression of BCS can be described as:
{Min{−SEA(T,D2,Z1,Z2),PCF(T,D2,Z1,Z2)}s.t.0.6mm≤T≤1.2mm15mm≤D2≤55mm4≤Z1≤124≤Z2≤12 | (5) |
Establishing a high precision approximate model is an effective means for optimal design. According to the collected data, by fitting different approximate models, the relationship surface between variables and responses can be derived, which can effectively reduce the number of experiments and improve the optimization efficiency. There are many kinds of approximation models, such as: RSM (Response Surface Method) [47], RBF (Radial Basis Function) [48] and KRG (Kriging approximation model) [49]. Different approximation models have their own advantages and disadvantages. Therefore, this paper establishes RSM, KRG and RBF approximation models respectively, and verifies their respective accuracy.
The advantage of RSM model is that it can fit a small number of data results with algebraic formulas, and the calculation is simple and efficient. It has good robustness, strong practicability and wide application range. The mathematical expression of the third-order RSM model is as follows:
y=β0+β1x1+β2x2+⋯+βMxM#+βM+1x21+βM+2x22+⋯+β2Mx22M+β2M+1x31+β2M+2x32+⋯+β3Mx33M+∑i≠jβijxixj | (6) |
The minimum number of sample points to be input by the model is: (M + 1) (M + 2) /2 + M, where M is the number of independent variables.
KRG model is an unbiased optimal estimation method of interval variables using spatial local interpolation. Its mathematical expression is as follows:
˜y(x0)=N∑i=1λiy(xi) | (7) |
where x0 represents the point to be estimated, xi represents the i-th observation point around x0, and y(xi) represents the corresponding observation value. λi represents the undetermined weighting coefficient of the corresponding observed value.
The advantage of RBF model is that it has strong nonlinear function fitting ability and strong generalization ability. Occasional noise points have little influence on the whole approximate model. Its mathematical expression is as follows:
ˆf(x)=N∑i=1φiϕ[r(xi,x)] | (8) |
where xi represents the vector of design variables at the i-th design point. φi is the linear combination coefficient of m neurons. 𝜙 can be represented as:
ϕ[r(xi,x)]=√∥xi−x∥2+c2 | (9) |
where‖xi ― x‖is the Euclidean distance and c is taken as 1 in this paper.
To evaluate the accuracy of different approximate models, three kinds of approximate models can be evaluated by R2 and RMSE (Root Mean Square Error). Where R2 is expressed as:
R2=N∑i=1(fi−−f)2−N∑i=1(fi−f'i)2N∑i=1(fi−−f)2 | (10) |
where fi and f'i represent the sample data and the approximate data corresponding to point i respectively, −f represents the mean value of the sample data, and N represents the number of sample points. The closer R2 is to 1, the more accurate the response surface.
The expression of RMSE is:
RMSE=√N∑i=1(fi−f'i)2N | (11) |
The RMSE result values less than 0.2 are considered acceptable accuracy. Therefore, according to the simulation data, RSM, RBF and KRG response surfaces were established respectively, and R2 and RMSE corresponding to the three approximation models are listed in Table 10.
RSM | KRG | RBF | ||
PCF | R2 | 0.968 | 0.930 | 0.933 |
RMSE | 0.061 | 0.083 | 0.081 | |
SEA | R2 | 0.976 | 0.874 | 0.935 |
RMSE | 0.052 | 0.099 | 0.073 |
According to the data, R2 of the third-order RSM is closer to 1, and RMSE is closer to 0, so the accuracy order of approximation models is RSM > RBF > KRG. In addition, the accuracy of RSM was verified by 10 random samplings, as shown in Table 11. The errors were all less than 10%, and the accuracy was acceptable.
D1 | D2 | Z1 | Z2 | T | SEA-RSM | SEA- initial | error-SEA | PCF-RSM | PCF- initial | error-PCF |
10 | 30 | 4 | 12 | 0.8 | 24.10 | 23.73 | 1.56% | 67.38 | 65.40 | 3.03% |
10 | 30 | 10 | 10 | 0.8 | 26.13 | 25.99 | 0.54% | 67.28 | 68.02 | -1.09% |
10 | 40 | 8 | 8 | 0.6 | 21.14 | 21.17 | -0.14% | 45.88 | 44.09 | 4.06% |
10 | 30 | 8 | 8 | 0.6 | 21.35 | 21.37 | -0.09% | 41.36 | 37.84 | 9.30% |
10 | 50 | 4 | 4 | 0.8 | 20.78 | 20.48 | 1.46% | 44.18 | 47.12 | -6.24% |
10 | 30 | 8 | 4 | 0.8 | 24.01 | 24.37 | -1.48% | 51.14 | 49.84 | 2.61% |
10 | 30 | 4 | 4 | 0.8 | 21.59 | 21.74 | -0.69% | 45.12 | 45.60 | -1.05% |
10 | 40 | 8 | 8 | 0.8 | 24.54 | 25.04 | -2.00% | 65.78 | 65.81 | -0.05% |
10 | 30 | 12 | 4 | 0.8 | 26.65 | 26.88 | -0.86% | 58.07 | 56.61 | 2.58% |
10 | 30 | 10 | 8 | 0.8 | 26.01 | 25.36 | 2.56% | 63.01 | 63.99 | -1.53% |
After obtaining an accurate and reliable approximation model, the second step is to optimize and discussion based on RSM. Multi-objective particle swarm optimization (MOPSO) was used to obtain the corresponding optimization results.
MOPSO is a population intelligence algorithm, which is designed by simulating the predatory behavior of birds. The process of finding the optimal design point is much like the foraging activity of these creatures. PSO is a population-based search process, in which individuals (called particles) continuously change positions (called states) within the search area. In other words, the birds communicate their location throughout the search process to let other birds know where the food comes from. Eventually, the whole bird flock can gather around the food source, which is what we call the optimal solution, and the problem converges.
Figure 18 shows the Pareto diagram of SEA and PCF. According to the safety requirements, the PCF value was limited to 80 kN to obtain the optimum configuration after BCS optimization (D1 = 10 mm, D2 = 15 mm, Z1 = 12, Z2 = 4, T = 1.03 mm). As shown in Table 12, the finite element analysis was carried out based on the corresponding optimization results and compared with the optimization results. The results show that the error between the predicted and actual values of the optimum structural PCF and SEA obtained by the RSM approximation model is less than 5%, with high accuracy and acceptability.
Indicator | Actual | Predict | Error |
SEA/(kJ/kg) | 33.60 | 34.42 | -2.38% |
PCF/kN | 79.12 | 80.13 | -1.26% |
In this paper, a series of novel bionic conch structures (BCS) are proposed to find a more excellent crashworthiness design scheme in this study. The comparative analysis of crashworthiness, the study of parameter effects, and the multi-objective optimization design have been carried out successively. The following conclusions can be drawn:
1) By comparing and analyzing the energy absorption characteristics of bionic conch structures, conventional single-cell tubes, and multi-cell tubes under axial loading, the results show that the bionic conch structures have higher energy absorption efficiency than the other two structures with the same mass. Among them, the SEA of BCS3 (m = 0.110 kg) is increased by 30.6 and 22.2% compared with CT and MCT of the same mass, and CLE is increased by 46.8 and 35.3%. The PCF of BCS3 (m = 0.213 kg) is 18.7 and 9.6% lower than that of CT and MCT of the same mass, respectively.
2) Through parameter design and factor significance analysis, the contribution of key parameters of BCS (wall thickness, inner and outer ring diameter, number of inner and outer panels) to crashworthiness was analyzed. The results show that the order of influence of geometric parameters on PCF is T > Z2 > Z1 > D2 >> D1; The order of influence on SEA is Z1 > T > D2 > Z2 >> D1.
3) Through the multi-objective optimization design, the approximation models (RSM, KRG, and RBF) and the multi-objective particle swarm optimization method were combined to find the optimum geometric configuration, and further optimize the crashworthiness of BCS to obtain the optimum configuration (D1 = 10 mm, D2 = 15 mm, Z1 = 12, Z2 = 4, T = 1.03 mm).
The research results of this paper have positive significance for the development of new lightweight thin-walled energy-absorbing structures.
This work was supported by the Natural Science Foundation of Fujian Province (Grant No. 2021J011168), the Education Research Project for Young and Middle-aged Teachers of Fujian Province (Grant No. JAT200688), the Research Project for Young and Middle-aged Teachers of Ningde Normal University (Grant No. 2022ZQ109 and Grant No. 2022ZQ102) and the Collaborative Innovation Center of Ningde Normal University (Grant No. 2022ZX02).
The authors declare there is no conflict of interest.
[1] | WHO Ebola Response Team, Ebola virus disease in west Africa-the first 9 months of the epidemic and forward projections, N. Engl. J. Med., 371 (2014), 1481–1495. |
[2] | S. Watts, SARS: a case study in emerging infections, Soc. Hist. Med., 18 (2005), 498–500. |
[3] | R. Xu and Z. Ma, Stability of a delayed SIRS epidemic model with a nonlinear incidence rate, Chaos Solitons Fractals, 41 (2009), 2319–2325. |
[4] | J. M. Epstein, Modelling to contain pandemics, Nature, 460 (2009), 687–689. |
[5] | J. Chen, An SIRS epidemic model, Appl. Math. J. Chinese Univ., 19 (2004), 101–108. |
[6] | T. Li, F. Zhang, H. Liu, et al., Threshold dynamics of an SIRS model with nonlinear incidence rate and transfer from infectious to susceptible, Appl. Math. Lett., 70 (2017), 52–57. |
[7] | C. Huang, H. Zhang, J. Cao, et al., Stability and Hopf bifurcation of a delayed prey-predator model with disease in the predator, Int. J. Bifurcat. Chaos, (2019), in press. |
[8] | M. Martcheva, Introduction to Mathematical Epidemiology, Springer, New York, 2015. |
[9] | W. O. Kermack and A.G. McKendrick, A contribution to the mathematical theory of epidemics, Proc. R. Soc. Lond. Ser. A Math. Phys. Eng. Sci., 115 (1927), 700–721. |
[10] | W. O. Kermack and A. G. McKendrick, Contributions to the mathematical theory of epidemics. II. The problem of endemicity, Proc. R. Soc. Lond. A, 138 (1932), 55–83. |
[11] | W. O. Kermack and A. G. McKendrick, Contributions to the mathematical theory of epidemics. III. Further studies of the problem of endemicity, Proc. R. Soc. Lond. A, 141 (1933), 94–122. |
[12] | H. W. Hethcote, Qualitative analyses of communicable disease models, Math. Biosci., 28 (1976), 335–356. |
[13] | S. Riley, C. Fraser and C. A. Donnelly, Transmission dynamics of the etiological agent of SARS in Hong Kong: impact of public health interventions, Science, 300 (2003), 1961–1966. |
[14] | M. Small and C. K. Tse, Small world and scale free model of transmission of SARS, Int. J. Bifurcat. Chaos, 15 (2005), 1745–1755. |
[15] | G. Zhu, G. Chen and X. Fu, Effects of active links on epidemic transmission over social networks, Phys. A, 468 (2017), 614–621. |
[16] | M. E. J. Newman, The structure and function of complex networks, SIAM Rev., 45 (2003), 167–256. |
[17] | X. Chu, Z. Zhang, J. Guan, et al., Epidemic spreading with nonlinear infectivity in weighted scale-free networks, Phys. A, 390 (2011), 471–481. |
[18] | H. Han, A. Ma and Z. Huang, An improved SIRS epidemic model on complex network, Int. Conf. Comput. Intell. Softw. Eng. IEEE, (2009), 1–5. |
[19] | R. Olinky and L. Stone, Unexpected epidemic thresholds in heterogeneous networks: The role of disease transmission, Phy. Rev. E, 70 (2004), 030902. |
[20] | R. Pastor-Satorras and A. Vespignani, Epidemic spreading in scale-free networks, Phys. Rev. Lett., 86 (2001), 3200–3213. |
[21] | L. Wang and G. Dai, Global stability of virus spreading in complex heterogeneous networks, SIAM J. Appl. Math., 68 (2008), 1495–1502. |
[22] | R. Yang, B. Wang, J. Ren, et al., Epidemic spreading on heterogeneous networks with identical infectivity, Phys. Lett. A, 364 (2007), 189–193. |
[23] | H. Zhang and X. Fu, Spreading of epidemics on scale-free networks with nonlinear infectivity, Nonlinear Anal., 70 (2009), 3273–327. |
[24] | X. Zhang, J. Wu, P. Zhao, et al., Epidemic spreading on a complex network with partial immu-nization, Soft Comput. 22 (2017), 1–9. |
[25] | C. H. Li, C. C. Tsai and S. Y. Yang, Analysis of epidemic spreading of an SIRS model in complex heterogeneous networks, Commun. Nonlinear Sci. Numer. Simul., 19 (2014), 1042–1054. |
[26] | C. Huang, J. Cao, F. Wen, et al., Stability analysis of SIR model with distributed delay on complex networks, PloS One, 11 (2016), e0158813. |
[27] | J. Huo and H. Y. Zhao, Dynamical analysis of a fractional SIR model with birth and death on heterogeneous complex networks, Phys. A, 448 (2016), 41–56. |
[28] | Z. Jin, G. Sun and H. Zhu, Epidemic models for complex networks with demographics, Math. Biosci. Eng., 11 (2014), 1295–1317. |
[29] | J. Liu, Y. Tang and Z. R. Yang, The spread of disease with birth and death on networks, J. Stat. Mech. Theory Exp., 8 (2004), P08008. |
[30] | Y. Wang, J. Cao, A. Alsaedi, et al., The spreading dynamics of sexually transmitted diseases with birth and death on heterogeneous networks, J. Stat. Mech. Theor. Exp., 2 (2017), 023502. |
[31] | P. Van den Driessche and J. Watmough, Reproduction numbers and sub-threshold endemic equi-libria for compartmental models of disease transmission, Math. Biosci., 180 (2002), 29–48. |
[32] | H. Yang, The basic reproduction number obtained from Jacobian and next generation matrices-A case study of dengue transmission modelling, Biosystems, 126 (2014), 52–75. |
[33] | F. Chen, On a nonlinear nonautonomous predator-prey model with diffusion and distributed delay, J. Comput. Appl. Math., 180 (2005), 33–49. |
[34] | H. R. Thieme, Persistence under relaxed point-dissipativity (with application to an endemic model), SIAM J. Math. Anal., 24 (1993), 407–435. |
[35] | H. L. Smith and P. De Leenheer, Virus dynamics: A global analysis, SIAM J. Appl. Math., 63 (2003), 1313–1327. |
[36] | J. P. LaSalle, The stability of dynamical systems, SIAM, Philadelphia, 1976. |
[37] | R. Cohen, S. Havlin and D. Ben-Avraham, Effecient immunization strategies for computer net-works and populations, Phys. Rev. Lett., 91 (2003), 247901. |
[38] | X. Fu, M. Small, D. M. Walker, et al., Epidemic dynamics on scale-free networks with piecewise linear infectivity and immunization, Phys. Rev. E, 77 (2008), 036113. |
[39] | F. Nian and X. Wang, Efficient immunization strategies on complex networks, J. Theor. Biol., 264 (2010), 77–83. |
[40] | R. Pastor-satorras and A.Vespignani, Immunization of complex networks, Phys. Rev. E, 65 (2002), 036104. |
[41] | D. S. Callaway, M. E. J. Newman, S. H. Strogatz, et al., Network robustness and fragility: perco-lation on random graphs, Phys. Rev. Lett., 85 (2000), 5468–5471. |
[42] | A. L. Barabasi and R. Albert, Emergence of scaling in random networks, Science, 286 (1999), 509–512. |
1. | Xiang Xu, Chuanqiang Huang, Chongchong Li, Gang Zhao, Xiaojie Li, Chao Ma, Uncertain design optimization of automobile structures: A survey, 2023, 31, 2688-1594, 1212, 10.3934/era.2023062 | |
2. | Xiaolin Deng, Yuwen Chen, Jiale Huang, Crashworthiness analysis of hexagonal hierarchical gradient tubes with axial variable thickness inspired by tree fractal structure, 2024, 31, 1537-6494, 5398, 10.1080/15376494.2023.2215778 | |
3. | Yuwen Chen, Xiaolin Deng, Huilan Huang, Hailong Ran, Chengming Wang, Crashworthiness of bionic tree-shaped hexagonal hierarchical gradient structures under oblique crushing conditions, 2024, 31, 1537-6494, 6923, 10.1080/15376494.2023.2240328 | |
4. | Lili Liu, Yurong Wang, Jianyong Zhao, Zhihao Cai, Ce Guo, Longhai Li, Multiscale Characterization and Biomimetic Design of Porcupine Quills for Enhanced Mechanical Performance, 2024, 17, 1996-1944, 1949, 10.3390/ma17091949 | |
5. | Xiaolin Deng, Fumo Yang, Libo Cao, Jiale Huang, Multi-objective optimization for a novel sandwich corrugated square tubes, 2023, 74, 11100168, 611, 10.1016/j.aej.2023.05.069 | |
6. | Yansong Liu, Meng Zou, Yingchun Qi, Lining Chen, Zhaoyang Wang, Jiafeng Song, Lianbin He, Bionic design of thin-walled bilinear tubes with excellent crashworthiness inspired by glass sponge structures, 2024, 19, 1748-3182, 046018, 10.1088/1748-3190/ad580a | |
7. | Hailong Ran, Huilan Huang, Xiaolin Deng, Crashworthiness of double-gradient hierarchical multi-cell hexagonal tubes under multi-load impacts, 2024, 25, 2083-3318, 10.1007/s43452-024-01087-1 |
Indicators | Structure | Test | Reference [46] | This paper | Error (%) |
PCF/kN | CS1 | 29.65 | 30.14 | 30.06 | -0.27 |
CS2 | 29.58 | 29.39 | 29.16 | -0.78 | |
SEA/(kJ/kg) | CS1 | 9.74 | 9.29 | 9.22 | -0.73 |
CS2 | 9.55 | 9.35 | 9.34 | -0.13 |
Structure | m/kg | T/mm | EA /kJ | PCF /kN | SEA/(kJ/kg) | CLE |
CT | 0.110 | 1.35 | 1.73 | 33.31 | 15.73 | 0.47 |
MCT | 0.110 | 0.59 | 1.85 | 32.91 | 16.82 | 0.51 |
BCS2 | 0.110 | 0.60 | 2.07 | 31.21 | 18.82 | 0.60 |
BCS3 | 0.110 | 0.45 | 2.26 | 29.89 | 20.55 | 0.69 |
CT | 0.145 | 1.80 | 2.70 | 46.45 | 18.62 | 0.53 |
MCT | 0.145 | 0.79 | 2.89 | 46.16 | 19.93 | 0.57 |
BCS2 | 0.145 | 0.80 | 3.23 | 44.46 | 22.28 | 0.66 |
BCS3 | 0.145 | 0.60 | 3.38 | 42.33 | 23.31 | 0.73 |
CT | 0.183 | 2.24 | 4.07 | 67.45 | 22.24 | 0.55 |
MCT | 0.183 | 0.98 | 3.99 | 60.72 | 21.80 | 0.60 |
BCS2 | 0.183 | 0.99 | 4.57 | 58.07 | 24.97 | 0.72 |
BCS3 | 0.183 | 0.76 | 4.80 | 56.67 | 26.23 | 0.77 |
CT | 0.213 | 2.65 | 5.23 | 81.05 | 24.55 | 0.59 |
MCT | 0.213 | 1.14 | 5.06 | 72.88 | 23.76 | 0.63 |
BCS2 | 0.213 | 1.15 | 5.62 | 68.55 | 26.38 | 0.75 |
BCS3 | 0.213 | 0.80 | 5.66 | 65.91 | 26.57 | 0.78 |
Structure | m/kg | T/mm | EA/kJ | CLE | CLE increase rate | EA decrease rate |
BCS3 | 0.110 | 0.45 | 2.26 | 0.69 | - | - |
0.145 | 0.60 | 3.38 | 0.73 | - | - | |
0.183 | 0.76 | 4.80 | 0.77 | - | - | |
0.213 | 0.80 | 5.66 | 0.78 | - | - | |
Graded gradient design | 0.110 | 0.45 | 2.20 | 0.78 | 13.04% | 2.65% |
0.145 | 0.60 | 3.35 | 0.80 | 9.59% | 0.89% | |
0.183 | 0.76 | 4.68 | 0.81 | 5.19% | 2.50% | |
0.213 | 0.80 | 5.24 | 0.84 | 7.69% | 7.42% |
D1 /mm | Z1 | Z2 | EA /kJ | PCF /kN | SEA /(kJ/kg) | CLE |
5 | 4 | 4 | 3.36 | 44.83 | 21.77 | 0.68 |
10 | 4 | 4 | 3.43 | 45.60 | 21.74 | 0.68 |
15 | 4 | 4 | 3.38 | 45.76 | 20.96 | 0.67 |
20 | 4 | 4 | 3.84 | 50.08 | 22.93 | 0.70 |
5 | 8 | 8 | 5.18 | 61.19 | 24.95 | 0.77 |
10 | 8 | 8 | 5.24 | 56.46 | 25.06 | 0.84 |
15 | 8 | 8 | 5.26 | 63.44 | 24.87 | 0.75 |
20 | 8 | 8 | 5.57 | 60.93 | 25.58 | 0.83 |
5 | 12 | 12 | 7.19 | 78.49 | 27.56 | 0.83 |
10 | 12 | 12 | 7.06 | 71.65 | 26.72 | 0.90 |
15 | 12 | 12 | 7.08 | 71.53 | 27.10 | 0.90 |
20 | 12 | 12 | 7.54 | 74.22 | 28.13 | 0.92 |
D2 /mm | Z1 | Z2 | EA /kJ | PCF /kN | SEA /(kJ/kg) | CLE |
20 | 4 | 4 | 3.24 | 43.26 | 22.71 | 0.68 |
30 | 4 | 4 | 3.43 | 45.60 | 21.74 | 0.68 |
40 | 4 | 4 | 3.77 | 46.70 | 21.75 | 0.73 |
50 | 4 | 4 | 3.66 | 47.12 | 20.48 | 0.71 |
20 | 8 | 8 | 5.06 | 57.55 | 26.70 | 0.80 |
30 | 8 | 8 | 5.24 | 60.46 | 25.06 | 0.79 |
40 | 8 | 8 | 5.75 | 65.81 | 25.04 | 0.79 |
50 | 8 | 8 | 5.25 | 62.57 | 22.89 | 0.76 |
20 | 12 | 12 | 6.84 | 75.41 | 29.27 | 0.82 |
30 | 12 | 12 | 7.06 | 81.65 | 26.72 | 0.79 |
40 | 12 | 12 | 7.51 | 84.52 | 26.26 | 0.81 |
50 | 12 | 12 | 7.02 | 84.23 | 25.25 | 0.76 |
Z1 | Z2 | EA /kJ | PCF /kN | SEA /(kJ/kg) | CLE |
4 | 4 | 3.43 | 45.60 | 21.74 | 0.68 |
4 | 6 | 4.01 | 52.22 | 23.04 | 0.70 |
4 | 8 | 4.35 | 54.44 | 22.81 | 0.73 |
4 | 10 | 4.88 | 60.00 | 23.55 | 0.74 |
4 | 12 | 5.31 | 65.40 | 23.73 | 0.74 |
6 | 4 | 3.78 | 49.08 | 22.68 | 0.70 |
6 | 6 | 4.28 | 54.74 | 23.34 | 0.74 |
6 | 8 | 4.71 | 57.56 | 23.57 | 0.74 |
6 | 10 | 5.34 | 64.39 | 24.70 | 0.75 |
6 | 12 | 5.68 | 68.70 | 24.38 | 0.75 |
8 | 4 | 4.28 | 49.84 | 24.37 | 0.78 |
8 | 6 | 4.70 | 56.50 | 24.44 | 0.76 |
8 | 8 | 5.24 | 60.46 | 25.06 | 0.84 |
8 | 10 | 5.75 | 65.74 | 25.53 | 0.80 |
8 | 12 | 6.18 | 71.02 | 25.55 | 0.79 |
10 | 4 | 4.69 | 54.94 | 25.36 | 0.78 |
10 | 6 | 5.13 | 60.98 | 25.46 | 0.76 |
10 | 8 | 5.53 | 63.99 | 25.36 | 0.79 |
10 | 10 | 6.09 | 68.02 | 25.99 | 0.81 |
10 | 12 | 6.62 | 74.83 | 26.37 | 0.80 |
12 | 4 | 5.21 | 56.61 | 26.88 | 0.84 |
12 | 6 | 5.69 | 62.69 | 27.01 | 0.82 |
12 | 8 | 6.15 | 66.00 | 27.09 | 0.85 |
12 | 10 | 6.61 | 70.97 | 27.15 | 0.85 |
12 | 12 | 7.06 | 76.65 | 26.72 | 0.84 |
D1 /mm | D2 /mm | Z1 | Z2 | T | PCF /kN | SEA /(kJ/kg) | CLE |
10 | 20 | 8 | 8 | 0.6 | 41.04 | 23.12 | 0.73 |
10 | 20 | 8 | 8 | 0.8 | 57.55 | 26.70 | 0.80 |
10 | 20 | 8 | 8 | 1 | 77.45 | 29.96 | 0.83 |
10 | 30 | 8 | 8 | 0.6 | 37.84 | 21.37 | 0.80 |
10 | 30 | 8 | 8 | 0.8 | 56.46 | 25.06 | 0.84 |
10 | 30 | 8 | 8 | 1 | 79.03 | 27.00 | 0.81 |
10 | 40 | 8 | 8 | 0.6 | 44.09 | 21.17 | 0.75 |
10 | 40 | 8 | 8 | 0.8 | 65.81 | 25.04 | 0.79 |
10 | 40 | 8 | 8 | 1 | 89.03 | 26.58 | 0.78 |
10 | 50 | 8 | 8 | 0.6 | 43.84 | 19.91 | 0.71 |
10 | 50 | 8 | 8 | 0.8 | 62.57 | 22.89 | 0.76 |
10 | 50 | 8 | 8 | 1 | 82.63 | 26.57 | 0.84 |
PCF | p value | t ratio |
T | < 0.0001* | 23.27 |
Z2 | < 0.0001* | 17.06 |
Z1 | < 0.0001* | 10.17 |
D2 | < 0.0001* | 5.34 |
D2*Z1 | 0.02425 | 2.32 |
D1 | 0.80903 | 0.24 |
SEA | p value | t ratio |
T | < 0.0001* | 18.81 |
Z1 | < 0.0001* | 18.81 |
D2 | < 0.0001* | -11.36 |
Z2 | < 0.0001* | 6.15 |
Z1*Z2 | 0.00012 | -4.17 |
D2*D2 | 0.00553 | 2.90 |
D1*D1 | 0.03324 | 2.19 |
D2*Z1 | 0.05482 | -1.97 |
D1 | 0.86509 | -0.17 |
RSM | KRG | RBF | ||
PCF | R2 | 0.968 | 0.930 | 0.933 |
RMSE | 0.061 | 0.083 | 0.081 | |
SEA | R2 | 0.976 | 0.874 | 0.935 |
RMSE | 0.052 | 0.099 | 0.073 |
D1 | D2 | Z1 | Z2 | T | SEA-RSM | SEA- initial | error-SEA | PCF-RSM | PCF- initial | error-PCF |
10 | 30 | 4 | 12 | 0.8 | 24.10 | 23.73 | 1.56% | 67.38 | 65.40 | 3.03% |
10 | 30 | 10 | 10 | 0.8 | 26.13 | 25.99 | 0.54% | 67.28 | 68.02 | -1.09% |
10 | 40 | 8 | 8 | 0.6 | 21.14 | 21.17 | -0.14% | 45.88 | 44.09 | 4.06% |
10 | 30 | 8 | 8 | 0.6 | 21.35 | 21.37 | -0.09% | 41.36 | 37.84 | 9.30% |
10 | 50 | 4 | 4 | 0.8 | 20.78 | 20.48 | 1.46% | 44.18 | 47.12 | -6.24% |
10 | 30 | 8 | 4 | 0.8 | 24.01 | 24.37 | -1.48% | 51.14 | 49.84 | 2.61% |
10 | 30 | 4 | 4 | 0.8 | 21.59 | 21.74 | -0.69% | 45.12 | 45.60 | -1.05% |
10 | 40 | 8 | 8 | 0.8 | 24.54 | 25.04 | -2.00% | 65.78 | 65.81 | -0.05% |
10 | 30 | 12 | 4 | 0.8 | 26.65 | 26.88 | -0.86% | 58.07 | 56.61 | 2.58% |
10 | 30 | 10 | 8 | 0.8 | 26.01 | 25.36 | 2.56% | 63.01 | 63.99 | -1.53% |
Indicator | Actual | Predict | Error |
SEA/(kJ/kg) | 33.60 | 34.42 | -2.38% |
PCF/kN | 79.12 | 80.13 | -1.26% |
Indicators | Structure | Test | Reference [46] | This paper | Error (%) |
PCF/kN | CS1 | 29.65 | 30.14 | 30.06 | -0.27 |
CS2 | 29.58 | 29.39 | 29.16 | -0.78 | |
SEA/(kJ/kg) | CS1 | 9.74 | 9.29 | 9.22 | -0.73 |
CS2 | 9.55 | 9.35 | 9.34 | -0.13 |
Structure | m/kg | T/mm | EA /kJ | PCF /kN | SEA/(kJ/kg) | CLE |
CT | 0.110 | 1.35 | 1.73 | 33.31 | 15.73 | 0.47 |
MCT | 0.110 | 0.59 | 1.85 | 32.91 | 16.82 | 0.51 |
BCS2 | 0.110 | 0.60 | 2.07 | 31.21 | 18.82 | 0.60 |
BCS3 | 0.110 | 0.45 | 2.26 | 29.89 | 20.55 | 0.69 |
CT | 0.145 | 1.80 | 2.70 | 46.45 | 18.62 | 0.53 |
MCT | 0.145 | 0.79 | 2.89 | 46.16 | 19.93 | 0.57 |
BCS2 | 0.145 | 0.80 | 3.23 | 44.46 | 22.28 | 0.66 |
BCS3 | 0.145 | 0.60 | 3.38 | 42.33 | 23.31 | 0.73 |
CT | 0.183 | 2.24 | 4.07 | 67.45 | 22.24 | 0.55 |
MCT | 0.183 | 0.98 | 3.99 | 60.72 | 21.80 | 0.60 |
BCS2 | 0.183 | 0.99 | 4.57 | 58.07 | 24.97 | 0.72 |
BCS3 | 0.183 | 0.76 | 4.80 | 56.67 | 26.23 | 0.77 |
CT | 0.213 | 2.65 | 5.23 | 81.05 | 24.55 | 0.59 |
MCT | 0.213 | 1.14 | 5.06 | 72.88 | 23.76 | 0.63 |
BCS2 | 0.213 | 1.15 | 5.62 | 68.55 | 26.38 | 0.75 |
BCS3 | 0.213 | 0.80 | 5.66 | 65.91 | 26.57 | 0.78 |
Structure | m/kg | T/mm | EA/kJ | CLE | CLE increase rate | EA decrease rate |
BCS3 | 0.110 | 0.45 | 2.26 | 0.69 | - | - |
0.145 | 0.60 | 3.38 | 0.73 | - | - | |
0.183 | 0.76 | 4.80 | 0.77 | - | - | |
0.213 | 0.80 | 5.66 | 0.78 | - | - | |
Graded gradient design | 0.110 | 0.45 | 2.20 | 0.78 | 13.04% | 2.65% |
0.145 | 0.60 | 3.35 | 0.80 | 9.59% | 0.89% | |
0.183 | 0.76 | 4.68 | 0.81 | 5.19% | 2.50% | |
0.213 | 0.80 | 5.24 | 0.84 | 7.69% | 7.42% |
D1 /mm | Z1 | Z2 | EA /kJ | PCF /kN | SEA /(kJ/kg) | CLE |
5 | 4 | 4 | 3.36 | 44.83 | 21.77 | 0.68 |
10 | 4 | 4 | 3.43 | 45.60 | 21.74 | 0.68 |
15 | 4 | 4 | 3.38 | 45.76 | 20.96 | 0.67 |
20 | 4 | 4 | 3.84 | 50.08 | 22.93 | 0.70 |
5 | 8 | 8 | 5.18 | 61.19 | 24.95 | 0.77 |
10 | 8 | 8 | 5.24 | 56.46 | 25.06 | 0.84 |
15 | 8 | 8 | 5.26 | 63.44 | 24.87 | 0.75 |
20 | 8 | 8 | 5.57 | 60.93 | 25.58 | 0.83 |
5 | 12 | 12 | 7.19 | 78.49 | 27.56 | 0.83 |
10 | 12 | 12 | 7.06 | 71.65 | 26.72 | 0.90 |
15 | 12 | 12 | 7.08 | 71.53 | 27.10 | 0.90 |
20 | 12 | 12 | 7.54 | 74.22 | 28.13 | 0.92 |
D2 /mm | Z1 | Z2 | EA /kJ | PCF /kN | SEA /(kJ/kg) | CLE |
20 | 4 | 4 | 3.24 | 43.26 | 22.71 | 0.68 |
30 | 4 | 4 | 3.43 | 45.60 | 21.74 | 0.68 |
40 | 4 | 4 | 3.77 | 46.70 | 21.75 | 0.73 |
50 | 4 | 4 | 3.66 | 47.12 | 20.48 | 0.71 |
20 | 8 | 8 | 5.06 | 57.55 | 26.70 | 0.80 |
30 | 8 | 8 | 5.24 | 60.46 | 25.06 | 0.79 |
40 | 8 | 8 | 5.75 | 65.81 | 25.04 | 0.79 |
50 | 8 | 8 | 5.25 | 62.57 | 22.89 | 0.76 |
20 | 12 | 12 | 6.84 | 75.41 | 29.27 | 0.82 |
30 | 12 | 12 | 7.06 | 81.65 | 26.72 | 0.79 |
40 | 12 | 12 | 7.51 | 84.52 | 26.26 | 0.81 |
50 | 12 | 12 | 7.02 | 84.23 | 25.25 | 0.76 |
Z1 | Z2 | EA /kJ | PCF /kN | SEA /(kJ/kg) | CLE |
4 | 4 | 3.43 | 45.60 | 21.74 | 0.68 |
4 | 6 | 4.01 | 52.22 | 23.04 | 0.70 |
4 | 8 | 4.35 | 54.44 | 22.81 | 0.73 |
4 | 10 | 4.88 | 60.00 | 23.55 | 0.74 |
4 | 12 | 5.31 | 65.40 | 23.73 | 0.74 |
6 | 4 | 3.78 | 49.08 | 22.68 | 0.70 |
6 | 6 | 4.28 | 54.74 | 23.34 | 0.74 |
6 | 8 | 4.71 | 57.56 | 23.57 | 0.74 |
6 | 10 | 5.34 | 64.39 | 24.70 | 0.75 |
6 | 12 | 5.68 | 68.70 | 24.38 | 0.75 |
8 | 4 | 4.28 | 49.84 | 24.37 | 0.78 |
8 | 6 | 4.70 | 56.50 | 24.44 | 0.76 |
8 | 8 | 5.24 | 60.46 | 25.06 | 0.84 |
8 | 10 | 5.75 | 65.74 | 25.53 | 0.80 |
8 | 12 | 6.18 | 71.02 | 25.55 | 0.79 |
10 | 4 | 4.69 | 54.94 | 25.36 | 0.78 |
10 | 6 | 5.13 | 60.98 | 25.46 | 0.76 |
10 | 8 | 5.53 | 63.99 | 25.36 | 0.79 |
10 | 10 | 6.09 | 68.02 | 25.99 | 0.81 |
10 | 12 | 6.62 | 74.83 | 26.37 | 0.80 |
12 | 4 | 5.21 | 56.61 | 26.88 | 0.84 |
12 | 6 | 5.69 | 62.69 | 27.01 | 0.82 |
12 | 8 | 6.15 | 66.00 | 27.09 | 0.85 |
12 | 10 | 6.61 | 70.97 | 27.15 | 0.85 |
12 | 12 | 7.06 | 76.65 | 26.72 | 0.84 |
D1 /mm | D2 /mm | Z1 | Z2 | T | PCF /kN | SEA /(kJ/kg) | CLE |
10 | 20 | 8 | 8 | 0.6 | 41.04 | 23.12 | 0.73 |
10 | 20 | 8 | 8 | 0.8 | 57.55 | 26.70 | 0.80 |
10 | 20 | 8 | 8 | 1 | 77.45 | 29.96 | 0.83 |
10 | 30 | 8 | 8 | 0.6 | 37.84 | 21.37 | 0.80 |
10 | 30 | 8 | 8 | 0.8 | 56.46 | 25.06 | 0.84 |
10 | 30 | 8 | 8 | 1 | 79.03 | 27.00 | 0.81 |
10 | 40 | 8 | 8 | 0.6 | 44.09 | 21.17 | 0.75 |
10 | 40 | 8 | 8 | 0.8 | 65.81 | 25.04 | 0.79 |
10 | 40 | 8 | 8 | 1 | 89.03 | 26.58 | 0.78 |
10 | 50 | 8 | 8 | 0.6 | 43.84 | 19.91 | 0.71 |
10 | 50 | 8 | 8 | 0.8 | 62.57 | 22.89 | 0.76 |
10 | 50 | 8 | 8 | 1 | 82.63 | 26.57 | 0.84 |
PCF | p value | t ratio |
T | < 0.0001* | 23.27 |
Z2 | < 0.0001* | 17.06 |
Z1 | < 0.0001* | 10.17 |
D2 | < 0.0001* | 5.34 |
D2*Z1 | 0.02425 | 2.32 |
D1 | 0.80903 | 0.24 |
SEA | p value | t ratio |
T | < 0.0001* | 18.81 |
Z1 | < 0.0001* | 18.81 |
D2 | < 0.0001* | -11.36 |
Z2 | < 0.0001* | 6.15 |
Z1*Z2 | 0.00012 | -4.17 |
D2*D2 | 0.00553 | 2.90 |
D1*D1 | 0.03324 | 2.19 |
D2*Z1 | 0.05482 | -1.97 |
D1 | 0.86509 | -0.17 |
RSM | KRG | RBF | ||
PCF | R2 | 0.968 | 0.930 | 0.933 |
RMSE | 0.061 | 0.083 | 0.081 | |
SEA | R2 | 0.976 | 0.874 | 0.935 |
RMSE | 0.052 | 0.099 | 0.073 |
D1 | D2 | Z1 | Z2 | T | SEA-RSM | SEA- initial | error-SEA | PCF-RSM | PCF- initial | error-PCF |
10 | 30 | 4 | 12 | 0.8 | 24.10 | 23.73 | 1.56% | 67.38 | 65.40 | 3.03% |
10 | 30 | 10 | 10 | 0.8 | 26.13 | 25.99 | 0.54% | 67.28 | 68.02 | -1.09% |
10 | 40 | 8 | 8 | 0.6 | 21.14 | 21.17 | -0.14% | 45.88 | 44.09 | 4.06% |
10 | 30 | 8 | 8 | 0.6 | 21.35 | 21.37 | -0.09% | 41.36 | 37.84 | 9.30% |
10 | 50 | 4 | 4 | 0.8 | 20.78 | 20.48 | 1.46% | 44.18 | 47.12 | -6.24% |
10 | 30 | 8 | 4 | 0.8 | 24.01 | 24.37 | -1.48% | 51.14 | 49.84 | 2.61% |
10 | 30 | 4 | 4 | 0.8 | 21.59 | 21.74 | -0.69% | 45.12 | 45.60 | -1.05% |
10 | 40 | 8 | 8 | 0.8 | 24.54 | 25.04 | -2.00% | 65.78 | 65.81 | -0.05% |
10 | 30 | 12 | 4 | 0.8 | 26.65 | 26.88 | -0.86% | 58.07 | 56.61 | 2.58% |
10 | 30 | 10 | 8 | 0.8 | 26.01 | 25.36 | 2.56% | 63.01 | 63.99 | -1.53% |
Indicator | Actual | Predict | Error |
SEA/(kJ/kg) | 33.60 | 34.42 | -2.38% |
PCF/kN | 79.12 | 80.13 | -1.26% |