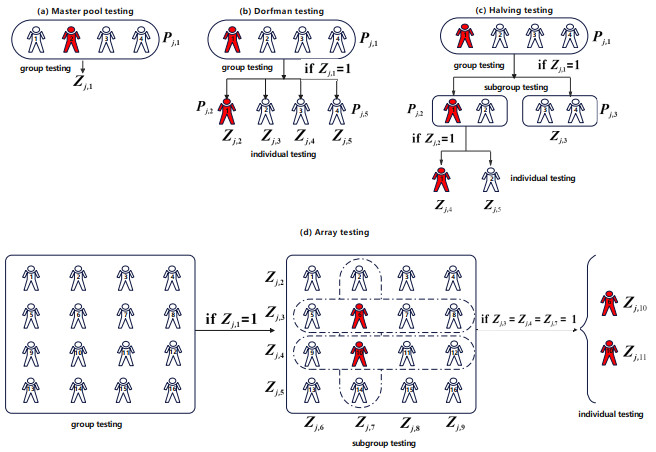
In many applications, the investigation of traveling wave solutions is essential in obtaining an accurate description of the dynamical behavior of most physical phenomena. The exact solutions to nonlinear equations can provide more physical descriptions and insightful details for many problems of practical interest. This paper focuses on investigating the solitary wave solutions of the generalized Zakharov equations (GZEs) by using four integration algorithms, namely, the modified (g′/g2)-expansion method, the modified (g′)-expansion method, the generalized simple (w/g)-expansion method, and the addendum to Kudryashov's method. The GZEs have been widely used to describe the propagation of Langmuir waves in the field of plasma physics. The efficiency and simplicity of these methods are evaluated based on their application to GZEs, which have yielded multiple new optical solitary wave solutions in the form of rational, trigonometric, and hyperbolic functions. By using a suitable wave transformation, the coupled nonlinear partial differential equations are converted into ordinary differential equations. The derived optical solutions are graphically depicted in 2D and 3D plots for some specific parameter values. The traveling wave solutions discovered in the current study constitute just one example of the desired solutions that may enable the exploration of the physical properties of many complex systems and could also contribute greatly to improving our understanding of many interesting natural phenomena that arise in different applications, including plasma physics, fluid mechanics, protein chemistry, wave propagation, and optical fibers.
Citation: Hammad Alotaibi. Solitary waves of the generalized Zakharov equations via integration algorithms[J]. AIMS Mathematics, 2024, 9(5): 12650-12677. doi: 10.3934/math.2024619
[1] | Kannat Na Bangchang . Application of Bayesian variable selection in logistic regression model. AIMS Mathematics, 2024, 9(5): 13336-13345. doi: 10.3934/math.2024650 |
[2] | Yin Xu, Ning Wang . Variable selection and estimation for accelerated failure time model via seamless-L0 penalty. AIMS Mathematics, 2023, 8(1): 1195-1207. doi: 10.3934/math.2023060 |
[3] | Lijie Zhou, Liucang Wu, Bin Yang . Estimation and diagnostic for single-index partially functional linear regression model with p-order autoregressive skew-normal errors. AIMS Mathematics, 2025, 10(3): 7022-7066. doi: 10.3934/math.2025321 |
[4] | Qingqing Jiang, Guangming Deng . Ultra-high-dimensional feature screening of binary categorical response data based on Jensen-Shannon divergence. AIMS Mathematics, 2024, 9(2): 2874-2907. doi: 10.3934/math.2024142 |
[5] | Zouaoui Chikr Elmezouar, Fatimah Alshahrani, Ibrahim M. Almanjahie, Salim Bouzebda, Zoulikha Kaid, Ali Laksaci . Strong consistency rate in functional single index expectile model for spatial data. AIMS Mathematics, 2024, 9(3): 5550-5581. doi: 10.3934/math.2024269 |
[6] | Hanji He, Meini Li, Guangming Deng . Group feature screening for ultrahigh-dimensional data missing at random. AIMS Mathematics, 2024, 9(2): 4032-4056. doi: 10.3934/math.2024197 |
[7] | Hsin-Lun Li . Leader–follower dynamics: stability and consensus in a socially structured population. AIMS Mathematics, 2025, 10(2): 3652-3671. doi: 10.3934/math.2025169 |
[8] | Chenlu Zheng, Jianping Zhu . Promote sign consistency in cure rate model with Weibull lifetime. AIMS Mathematics, 2022, 7(2): 3186-3202. doi: 10.3934/math.2022176 |
[9] | Jeong-Kweon Seo, Byeong-Chun Shin . Reduced-order modeling using the frequency-domain method for parabolic partial differential equations. AIMS Mathematics, 2023, 8(7): 15255-15268. doi: 10.3934/math.2023779 |
[10] | Dingyu Wang, Chunming Ye . Single machine and group scheduling with random learning rates. AIMS Mathematics, 2023, 8(8): 19427-19441. doi: 10.3934/math.2023991 |
In many applications, the investigation of traveling wave solutions is essential in obtaining an accurate description of the dynamical behavior of most physical phenomena. The exact solutions to nonlinear equations can provide more physical descriptions and insightful details for many problems of practical interest. This paper focuses on investigating the solitary wave solutions of the generalized Zakharov equations (GZEs) by using four integration algorithms, namely, the modified (g′/g2)-expansion method, the modified (g′)-expansion method, the generalized simple (w/g)-expansion method, and the addendum to Kudryashov's method. The GZEs have been widely used to describe the propagation of Langmuir waves in the field of plasma physics. The efficiency and simplicity of these methods are evaluated based on their application to GZEs, which have yielded multiple new optical solitary wave solutions in the form of rational, trigonometric, and hyperbolic functions. By using a suitable wave transformation, the coupled nonlinear partial differential equations are converted into ordinary differential equations. The derived optical solutions are graphically depicted in 2D and 3D plots for some specific parameter values. The traveling wave solutions discovered in the current study constitute just one example of the desired solutions that may enable the exploration of the physical properties of many complex systems and could also contribute greatly to improving our understanding of many interesting natural phenomena that arise in different applications, including plasma physics, fluid mechanics, protein chemistry, wave propagation, and optical fibers.
Group testing, or pooled testing, was first introduced by Dorfman [1] to identify syphilis infections among U.S. Army personnel during World War II. This approach involves combining specimens (e.g., blood, plasma, urine, swabs) from multiple individuals and conducting a single test to check for infection. According to Dorfman's procedure, if the combined sample tests negative, all individuals in this sample can be confirmed disease-free. Conversely, a positive result necessitates further testing to identify the affected individuals. This strategy gained prominence during the COVID-19 pandemic [2,3,4] and has been applied to detect various infectious diseases, including HIV [5,6], chlamydia and gonorrhea [7], influenza [8], and the Zika virus [9]. The primary motivation for pooled testing lies in its economic efficiency; for instance, the State Hygienic Laboratory at the University of Iowa saved approximately fanxiexian_myfh3.1 million over five years by employing a modified Dorfman protocol for testing chlamydia and gonorrhea among residents of Iowa [10,11].
Despite its cost-effectiveness, group testing poses significant challenges for statistical analysis due to the absence of individual response data [12]. However, advancements in digital technology have provided access to rich covariate information, including demographic data, electronic health records, genomic data, lifestyle data, physiological monitoring data, imaging data, and environmental variables [13]. Integrating these covariates into various statistical models for group testing has been shown to enhance accuracy and robustness, as evidenced by studies from Mokalled et al. [14], Huang and Warasi [15], Haber et al. [16]. This integration leads to improved estimations of individual risk probabilities, thereby reducing the number of tests required and overall costs.
In managing covariates, single-index models offer advantages, such as less restrictive assumptions, good interpretability, and adaptability to high-dimensional data [17]. For high-dimensional single-index models, Radchenko [18] proposed a novel estimation method based on L1 regularization, extending it to generalized linear models. Elmezouar et al. [19] developed a functional single index expectile model with a nonparametric estimator to address spatial dependency in financial data, showing strong consistency and practical applicability. Chen and Samworth [20] explored generalized additive models, deriving non-parametric estimators for each additive component by maximizing the likelihood function, and adapted this approach to generalized additive index models. Kereta et al. [21] employed a k-nearest neighbor estimator, enhanced by geodesic metrics, to extend local linear regression for single-index models. However, research on generalized semi-parametric single-index models in high-dimensional contexts remains limited, particularly in group testing applications, which are still underexplored.
Most current integrations of covariate information with group testing are developed based on parametric regression models. For example, Wang et al. [22] introduced a comprehensive binary regression framework, while McMahan et al. [11] developed a Bayesian regression framework. Gregory et al. [23] adopted an adaptive elastic net method, which remains effective as data dimensionality increases. Ko et al. [24] compared commonly used group testing procedures with group lasso regarding true positive selection in high-dimensional genomic data analysis. Furthermore, nonparametric regression methods have gained traction for applying covariates in group testing. Delaigle and Hall [25] proposed a nonparametric method for estimating conditional probabilities and testing specificity and sensitivity, addressing the unique dilution effects and complex data structures inherent in group testing. Self et al. [26] introduced a Bayesian generalized additive regression method to tackle dilution effects further, while Yuan et al. [12] developed a semiparametric monotone regression model using the expectation-maximization (EM) algorithm to navigate the complexities of group testing data. Zuo et al. [27] proposed a more flexible generalized nonparametric additive model, utilizing B-splines and group lasso methods for model estimation in high-dimensional data.
This article proposes a generalized single-index group testing model aimed at enhancing flexibility in addressing various nonlinear models and facilitating the selection of important variables. Given the absence of individual disease testing results in group testing data, the EM algorithm is employed to perform the necessary calculations for the model. B-spline functions are utilized to approximate the nonlinear unknown smooth functions, with model parameters estimated by maximizing the likelihood function. In modern group testing, a substantial amount of individual covariate information is typically collected during sample testing. Consequently, a penalty term is incorporated into the likelihood function, promoting the construction of a sparse model and enabling effective variable selection. We apply the method to four group testing strategies: master pool, Dorfman, halving, and array. The method is evaluated using both simulated and real data.
The remaining sections are organized as follows. Section 2 introduces our model with B-spline approximation, detailing the corresponding algorithm employing the EM algorithm. Section 3 elaborates on the E-step in the EM algorithm, facilitating the acceleration of our algorithm's convergence. Sections 4 and 5 present comprehensive simulations and real data application, demonstrating the method's robust performance. Finally, we conclude our findings and provide some discussion in Section 6.
Consider a dataset comprising n individuals. For each i∈{1,2,…,n}, let the true disease status of the i-th individual be denoted by ˜Yi∈{0,1}, where ˜Yi=1 indicates disease presence, and ˜Yi=0 indicates absence. Additionally, the dataset includes covariate information for each individual, represented as Xi=(Xi1,…,Xiqn)T∈Rqn, where Rqn denotes a qn-dimensional real vector space. We assume the number of covariates qn is high-dimensional.
Let the risk probability for the i-th individual be defined as pi=Pr(˜Yi=1∣Xi), where i∈{1,2,…,n}. In many cases, the influence of covariates may be nonlinear; imposing linearity can result in inaccurate estimations. This study explores nonlinear scenarios, assuming pi follows a flexible logistic single-index model, expressed as
Pr(˜Yi=1|Xi)=exp[g(X⊤iβ)]1+exp[g(X⊤iβ)], | (2.1) |
where β=(β1,β2,…,βqn)⊤∈Rqn represents the unknown parameters, and g(⋅) is an unknown smooth function capturing the relationship between covariates and risk probabilities.
In semiparametric single-index models, the true parameters are generally considered non-identifiable without imposed constraints. To ensure the identifiability of β, we impose a classical constraint: β1=√1−‖β−1‖22, where β−1=(β2,β3,…,βqn)⊤∈Rqn−1, and ‖⋅‖2 denotes the L2-norm. Note that both the function g(⋅) and the coefficient β in the single-index model are unknown. The L2-norm constraint ‖β‖2=1 is crucial for the identifiability of β as shown by Carroll et al. [28], Zhu et al. [29], Lin et al. [30], Cui et al. [31], and Guo et al. [32]. We assume that the true parameter β∗ is sparse, defining the true model as M∗={j∈{1,2,…,qn}:β∗j≠0}.
For i∈{1,2,…,n}, ˜Yi follows a Binomial distribution with parameter pi, denoted as ˜Yi∼Binom(1,pi). In traditional single-index model studies, the true status ˜Y={˜Yi,i=1,2,…,n}, is directly observable. However, in group testing, ˜Y is unobservable [33]. This paper investigates parameter estimation and statistical inference of single-index models based on group testing data. Moreover, if a group test result is positive, further testing is required to identify infected individuals. These results may depend on shared characteristics, leading to correlations within group test outcomes, complicating the modeling.
In group testing, we partition n individuals into J groups, denoted as P1,1,P2,1,…,PJ,1. Here, Pj,1 represents the initial index set of individuals for the j-th group, ensuring ∪Jj=1Pj,1={1,2,…,n}. For j∈{1,2,…,J}, if any testing result for Pj,1 is positive, further testing may be warranted. Define Zj={Zj,l,l=1,2,…,Lj} as the set of testing outcomes for the j-th group, where Lj denotes the total number of tests conducted within j-th group. Each Zj,l∈{0,1}, where Zj,l=0 indicates a negative result and Zj,l=1 indicates a positive result. If Zj,1=0, then Lj=1; otherwise, Lj≥1. Let Pj={Pj,l,l=1,2,…,Lj}, where Pj,l corresponds to the individuals associated with Zj,l. Define ˜Zj={˜Zj,l,l=1,2,…,Lj} as the true status corresponding to Zj. The true statuses of individuals determine the group's true status, defined as ˜Zj,l=I(∑i∈Pj,l˜Yi), where I(⋅) denotes the indicator function.
In practical applications, measurement error of the test kits exists. We define Se=Pr(Zj,l=1∣˜Zj,l=1) as sensitivity, representing the probability of correctly identifying positive samples, and Sp=Pr(Zj,l=0∣˜Zj,l=0) as specificity, denoting the probability of correctly identifying negative samples, where l∈{1,2,…,Lj} and j∈{1,2,…,J}. According to the definitions of Se and Sp, given the true status ˜Zj,l, the group's testing results satisfy Zj,l|˜Zj,l∼Binom(1,Se˜Zj,l(1−Sp)1−˜Zj,l).
Our approach is based on two widely accepted fundamental assumptions in group testing. The first assumption is that Se and Sp are independent of group size, supported by various studies [34,35,36,37]. The second assumption posits that, given the true statuses of individuals in the j-th group {˜Yi,i∈Pj,1}, the group's true statuses ˜Zj are mutually independent, as supported by previous research [23,34,35].
We apply our method to four group testing methods: master pool testing, Dorfman testing, halving testing, and array testing. Figure 1 illustrates the process of four testing methods: (a) Master pool testing, where a group of individuals (e.g., Pj,1 consisting of individuals 1, 2, 3, and 4) is tested as a whole to obtain the group testing result Zj,1; (b) Dorfman testing, where initially the same group testing as in master pool testing is conducted, and if the result of the master pool testing is positive (Zj,1=1), each individual in the group is then tested separately to obtain individual testing results Zj,2, Zj,3, Zj,4, and Zj,5; (c) Halving testing, where the entire group (e.g., Pj,1) is tested as a whole, and if the result is positive (Zj,1=1), the group is divided into two subgroups (e.g., Pj,2 and Pj,3) for subgroup testing, and if the result of subgroup testing is positive (e.g., Zj,2=1), individuals in the positive subgroup are then tested individually; and (d) Array testing, where multiple individuals (e.g., 16 individuals) are arranged in an array for group testing to obtain multiple group testing results such as Zj,1, and if a specific group testing result is positive (e.g., Zj,1=1), further subgroup testing is performed (e.g., obtaining results Zj,2, Zj,3), and if the group testing results for both the row and column where an individual is located are positive (e.g., Zj,3=Zj,4=Zj,7=1), the individuals (e.g., 6-th individual and 10-th individual) are then tested.
Due to the nature of group testing, the true status of individuals, denoted as ˜Y, remains unknown. Our objective is to estimate M∗, β∗, and g(⋅) based on observed data Z={Zj,j=1,2,…,J} and covariate information X=(X1,X2,…,Xn)T∈Rn×qn to ascertain individual risk probabilities. The likelihood function based on the observed data Z is defined as
P(Z|X)=∑˜Y∈{0,1}nP(Z|˜Y)P(˜Y|X), | (2.2) |
where
P(Z∣˜Y)=J∏j=1Lj∏l=1P(Zj,l∣˜YPj,l), |
and ˜YPj,l={˜Yi,i∈Pj,l} represents the set of true statuses for individuals in Pj,l. Furthermore, the conditional probability P(Zj,l∣˜YPj,l) is expressed as
P(Zj,l∣˜YPj,l)={S˜Zj,le(1−Sp)1−˜Zj,l}Zj,l{(1−Se)˜Zj,lS1−˜Zj,lp}1−Zj,l. |
The likelihood function for the true disease status ˜Y can be written as
P(˜Y|X)=n∏i=1p˜Yii(1−pi)1−˜Yi. |
Combining this with the logistic single-index model defined in (2.1), we obtain the log-likelihood function for ˜Y:
lnP(˜Y|X)=n∑i=1{˜Yig(X⊤iβ)−ln(1+exp[g(X⊤iβ)])}. | (2.3) |
Since the smooth function g(⋅) is unknown, we approximate it using B-spline functions. Let the support interval of g(⋅) be [a,b]. We partition [a,b] at points a=d0<d1<…<dN<b=dN+1 into several segments, referred to as knots or internal nodes. This division generates subintervals Ik=[dk,dk+1) for 0≤k≤N−1 and IN=[dN,dN+1], ensuring that
max0≤k≤N|dk−dk+1|min0≤k≤N|dk−dk+1|≤M, |
where M∈(0,∞). The B-spline basis functions of order q are denoted as Φ(⋅)=(ϕ1(⋅),ϕ2(⋅),…,ϕS(⋅))⊤∈RS, with S=N+q. Thus, g(⋅) can be approximated as
g(⋅)≈S∑s=1ϕs(⋅)γs, |
where γs are the spline coefficients to be estimated [38]. Denote γ=(γ1,γ2,…,γS)⊤∈RS. We approximate g(XTiβ) as
g(XTiβ)=Φ⊤(X⊤iβ)γ, |
where Φ(X⊤iβ)=(ϕ1(X⊤iβ),ϕ2(X⊤iβ),…,ϕS(X⊤iβ))⊤. Therefore, we approximate pi by using a spline function, and denote the spline approximation of pi as piB, which is defined as follows:
piB=exp[Φ⊤(X⊤iβ)γ]1+exp[Φ⊤(X⊤iβ)γ]. | (2.4) |
In the following, we use the spline approximation piB of pi to construct the log-likelihood function and the objective function in the subsequent EM algorithm. Thus, the log-likelihood function (2.3) for ˜Y can be reformulated as
lnPB(˜Y|X)=n∑i=1{˜YiΦ⊤(X⊤iβ)γ−ln(1+exp[Φ⊤(X⊤iβ)γ])}. |
Furthermore, the target likelihood function (2.2) can be represented as
PB(Z|X)=∑˜Y∈{0,1}nP(Z|˜Y)PB(˜Y|X). |
By employing spline approximation, we transform the estimation problem of β∗−1 and g(⋅) into estimating β∗−1 and γ.
For high-dimensional group testing data, we aim to estimate β∗−1 using the penalized approach within a single-index model framework. The penalized log-likelihood function is defined as follows:
lnPB(Z|X)−qn∑j=2Pλ(βj), | (2.5) |
where Pλ(⋅) is the penalty function and λ is a tuning parameter. We consider three common penalty functions: LASSO [39], SCAD [40], and MCP [41]. Specifically, for LASSO, Pλ(x)=λ|x|. For SCAD, it is defined as
Pλ(x)={λ|x|if |x|≤λ,−x2+2δλ|x|−λ22(δ−1)if λ<|x|≤δλ,(δ+1)λ22if |x|>δλ, |
where δ>2. In MCP, the penalty function is given by
Pλ(x)={λ|x|−x22δif |x|≤δλ,12δλ2if |x|>δλ, |
with δ>1. The following section will detail the parameter estimation process.
The penalized log-likelihood function (2.5) lacks the individual latent status ˜Y. The complete data penalized log-likelihood function can be expressed as
lnPB(Z,˜Y|X)−qn∑j=2Pλ(βj)=lnP(Z|˜Y)+lnPB(˜Y|X)−qn∑j=2Pλ(βj). | (2.6) |
Notably, lnP(Z|˜Y) depends solely on known parameters Se and Sp, allowing us to disregard it in computations. The presence of the latent variable ˜Y complicates direct maximization of the complete data penalized log-likelihood function (2.6). Therefore, we employ the EM algorithm, comprising two steps: the Expectation (E) step, and the Maximization (M) step.
In the E step, given the observed data Z and the parameters from the t-th iteration (β(t)−1,γ(t)), calculate the following function:
S(t)(β−1,γ)=E{n∑i=1{˜YiΦ⊤(X⊤iβ)γ−ln(1+exp[Φ⊤(X⊤iβ)γ])}|Z,β(t)−1,γ(t)}−qn∑j=2Pλ(βj)=n∑i=1{w(t)iΦ⊤(X⊤iβ)γ−ln(1+exp[Φ⊤(X⊤iβ)γ])}−qn∑j=2Pλ(βj), | (2.7) |
where w(t)i=E[˜Yi|Z,γ(t),β(t)−1],i=1,2,…,n. The calculation of the w(t)i varies among the four grouping testing methods, which will be discussed in Section 3.
In the M step, we update β(t+1)−1 and γ(t+1), respectively. Initially, we update γ(t+1) by maximizing:
S(t)(β(t)−1,γ)=n∑i=1{w(t)iΦ⊤(X⊤iβ(t))γ−ln(1+exp[Φ⊤(X⊤iβ(t))γ])}−qn∑j=2Pλ(β(t)j). | (2.8) |
Subsequently, we maximize S(t)(β−1,γ(t+1)) to update the parameters β(t+1)−1:
S(t)(β−1,γ(t+1))=n∑i=1{w(t)iΦ⊤(X⊤iβ)γ(t+1)−ln(1+exp[Φ⊤(X⊤iβ)γ(t+1)])}−qn∑j=2Pλ(βj). | (2.9) |
Given that β−1 appears in each B-spline basis function ϕ(X⊤iβ), direct iteration presents challenges. Let ˜g(t)(XTiβ)=Φ⊤(XTiβ)γ(t+1). We apply the approach by Guo et al. [42], approximating ˜g(t)(XTiβ) via a first-order Taylor expansion
˜g(t)(XTiβ)≈˜g(t)(XTiβ(t))+˜g(t)′(XTiβ(t))XTiJ(β(t))(β−1−β(t)−1), |
where J(β)=∂β/∂β−1=(−β−1/√1−‖β−1‖22,Iqn−1)⊤ represents the Jacobian matrix of size qn×(qn−1) and Iqn−1 denotes the (qn−1)-dimensional identity matrix. This approximation is incorporated into S(t)(β−1,γ(t+1)) to maximize the expression and update β(t+1)−1. Therefore, we approximate S(t)(β−1,γ(t+1)) by ˜S(t)(β−1,γ(t+1)) as follows:
˜S(t)(β−1,γ(t+1))=n∑i=1{w(t)i˜g(t)(X⊤iβ)−ln(1+exp[˜g(t)(X⊤iβ)])}−qn∑j=2Pλ(βj)=n∑i=1{w(t)i[˜g(t)(XTiβ(t))+˜g(t)′(XTiβ(t))XTiJ(β(t))(β−1−β(t)−1)]−ln(1+exp[˜g(t)(XTiβ(t))+˜g(t)′(XTiβ(t))XTiJ(β(t))(β−1−β(t)−1)])}−qn∑j=2Pλ(βj). | (2.10) |
We employ stochastic gradient descent [43] and coordinate descent [44] to update γ and β, respectively. Let ˆγ and ˆβ−1 denote the estimated parameters, and ˆM={j∈{1,2,…,qn}:ˆβj≠0} represent the estimated model. Furthermore, ˆγ and ˆβ−1 can be used to calculate individual risk probabilities and guide subsequent testing strategies. In summary, the EM algorithm offers a structured approach to handle the latent variable ˜Y and estimate model parameters. The detailed steps of this method are summarized in Algorithm 1.
Algorithm 1: Regularized single-index model for group testing. |
Input: Z, X, tmax and initialization (β(0)−1,γ(0)). For: t=0,1,2,…,tmax ● Step 1 (E-step): In the E step, given the parameters (β(t)−1,γ(t)) and Z, calculate the conditional expectation S(t)(β−1,γ) in (2.7). ● Step 2 (M-step): Update the iterative parameters β(t+1)−1 and γ(t+1) in two substeps: 1. Update γ(t+1) by maximizing S(t)(β(t)−1,γ) in (2.8). 2. Update β(t+1)−1 by maximizing ˜S(t)(β−1,γ(t+1)) in (2.10). End for: Repeat steps 1 and 2 until parameters converge or reach the maximum number of iterations tmax. Output: The estimates ˆβ−1 and ˆγ. |
Implementing Algorithm 1 requires deriving formulas to calculate the conditional expectations of individuals' true statuses. These expressions are essential for the effective application of the EM algorithm in various testing scenarios. Common group testing methods include master pool testing, Dorfman testing, halving testing, and array testing. We have derived the conditional expectation formula of these methods under our methodological framework, which will facilitate our other calculations.
For master pool testing, samples are divided into J distinct groups, with each sample assigned to only one group, and each group undergoes a single test without subsequent testing. When the i-th individual is assigned to the j-th group, consider two cases for w(t)i:
While Zj=0,
w(t)i=P(˜Yi=1,Zj=0)P(Zj=0)=P(Zj=0|˜Yi=1)P(˜Yi=1)P(Zj=0). |
Due to
P(Zj=1)=P(Zj=1|˜Zj=1)P(˜Zj=1)+P(Zj=1|˜Zj=0)P(˜Zj=0)=Se[1−∏i∈Pj(1−p(t)iB)]+(1−Sp)∏i∈Pj(1−p(t)iB)=Se+(1−Se−Sp)∏i∈Pj(1−p(t)iB), |
let Δj=Se+(1−Se−Sp)∏i∈Pj(1−p(t)iB), where piB is an approximate result of pi in (2.4). Therefore,
P(Zj=0)=1−[Se+(1−Se−Sp)∏i∈Pj(1−p(t)iB)]=1−Δj. |
Then,
w(t)i=(1−Se)⋅p(t)iB1−[Se+(1−Se−Sp)∏i∈Pj(1−p(t)iB)]=(1−Se)⋅p(t)iB(1−Δj). |
While Zj=1,
w(t)i=P(˜Yi=1|Zj=1)=p(Zj=1|˜Yi=1)P(˜Yi=1)P(Zj=1)=Se⋅p(t)iBSe+(1−Se−Sp)∏i∈Pj(1−p(t)iB)=Se⋅p(t)iBΔj. |
In conclusion,
w(t)i={P(˜Yi=1|Zj=0)=(1−Se)⋅p(t)iB/(1−Δj),ifZj=0,P(˜Yi=1|Zj=1)=Se⋅p(t)iB/Δj,ifZj=1. |
We apply our method to four group testing algorithms: master pool testing, Dorfman testing, halving testing, and array testing. For other algorithms, detailed expressions can be found in Appendix C. Using these expressions, we apply the EM algorithm to estimate the model parameters.
In this section, we assess the performance of the proposed method using simulated datasets. The generation of covariates follows the approach described by Guo et al. [42]. Specifically, covariates X∈Rn×qn are drawn from a truncated multivariate normal distribution. We first generate covariates from N(0,Σ), where Σ∈Rqn×qn and Σij=0.5|i−j| for 1≤i,j≤qn. These covariates are then truncated to the range (−2,2) to obtain X. We consider logistic single-index models to describe pi=Pr(˜Yi=1∣Xi), with the function g(X⊤iβ) in the model (2.1) defined as follows,
Example 4.1. We set n=500 and β∗=(3√15.25,2.5√15.25,0,…,0)⊤. We consider two scenarios: qn=50 and qn=100. The model is described as follows:
g(X⊤iβ∗)=exp(X⊤iβ∗)−7. |
Under this setting, the disease prevalence is approximately 8.93%.
Example 4.2. We set n=1000 and β∗=(1√3,1√3,1√3,0,…,0)⊤. We consider two scenarios: qn=100 and qn=500. The model is described as follows:
g(X⊤iβ∗)=X⊤iβ∗(1−X⊤iβ∗)+exp(X⊤iβ∗)−6. |
In this example, the disease prevalence is approximately 11.41%.
Example 4.3. We set qn=50 and β∗=(9√181,8√181,6√181,0,…,0)⊤. We consider two scenarios: n=500 and n=1000. The model is described as follows:
g(X⊤iβ∗)=X⊤iβ∗(1−X⊤iβ∗)+0.5⋅sin(πX⊤iβ∗2)−6. |
In this example, the disease prevalence is approximately 9.42%.
Example 4.4. We set qn=100 and β∗=(0.5,0.5,0.5,0.5,0,…,0)⊤. Two scenarios are considered: n=750 and n=1000. The model is described as follows:
g(X⊤iβ∗)=X⊤iβ∗(1−X⊤iβ∗)+exp(X⊤iβ∗)+0.1⋅sin(πX⊤iβ∗2)−6. |
In this scenario, the disease prevalence is approximately 10.32%.
In our simulation study, we employed four group testing algorithms: master pool testing (MPT), Dorfman testing (DT), halving testing (HT), and array testing (AT) to evaluate the model. For MPT, DT, and HT, the group size was set to 4, while in AT, individuals were arranged in a 4×4 array. Both sensitivity and specificity were fixed at Se=Sp=0.98. Based on the methodologies of Fan and Li [40] and Zhang [41], we set δ values of 3.7 and 2 for SCAD and MCP, respectively. Each scenario was simulated B=100 times, where ˆβ[b] denotes the estimated β∗ in the b-th simulation, with b∈{1,2,…,B}.
Following the approach of Guan et al. [45], we measured the estimation accuracy of ˆβj (j=1,2,3,4) using the mean squared error (MSE), defined as
MSE=1BB∑b=1(β∗j−ˆβ[b]j)2,j=1,2,3,4. |
We utilized average mean squared error (AMSE) to assess the accuracy of ˆβ, consistent with methods employed by Wang and Yang [46]:
AMSE=1BqnB∑b=1‖β∗−ˆβ[b]‖22. |
Average mean absolute error (AMAE) was used to evaluate the estimation performance of g(⋅) and individual risk probabilities pi [42]. The AMAE for g(⋅) is defined as
AMAEg=1BnB∑b=1n∑i=1|g(X⊤iβ∗)−g(X⊤iˆβ[b])|, |
while the AMAE for ˆp[b]i=eg(X⊤iˆβ[b])1+eg(X⊤iˆβ[b]) is defined as
AMAEp=1BnB∑b=1n∑i=1|p∗i−ˆp[b]i|, |
where p∗i=g(X⊤iβ∗)1+eg(X⊤iβ∗).
To evaluate variable selection performance, we employed true positive rate (TPR) and false positive rate (FPR). The FPR represents the proportion of false positives among identified predictors, while the TPR indicates the proportion of true positives among relevant predictors. Table 1 shows the results of variable selection. TPR and FPR are defined as follows:
TPR=TPTP+FN,FPR=FPFP+TN. |
Metric | Implication |
True positive (TP) | Actual positive and predicted positive |
False positive (FP) | Actual negative and predicted positive |
False negative (FN) | Actual positive and predicted negative |
True negative (TN) | Actual negative and predicted negative |
The simulation results are summarized in Tables 2 to 5. As shown in the tables, the TPR was approximately 97%, with a very low FPR. The result shows that the probability that M∗ is contained in ˆM is very close to 1. This demonstrates the notable performance of our model in variable selection. The AMAE for g(⋅) and pi was approximately 0.5 and 0.01, respectively. This shows that we have accurately captured the form of the unknown smooth function g(⋅) and are able to precisely predict the individual risk probability. The AMSE for the model parameters β was around 10−4, while the AMSE for significant variables βj was approximately 10−3. This demonstrates the accuracy of our model in parameter estimation.
AMAE | AMSE | MSE | ||||||||
Model | Setting | Test | Penalty | TPR | FPR | g(⋅) | Prob | β | β1 | β2 |
Example 4.1 (n=500) | qn=50 | MPT | MCP | 0.980 | 0.061 | 0.325 | 0.011 | 0.0003 | 0.0022 | 0.0015 |
HT | 0.985 | 0.003 | 0.413 | 0.007 | 0.0002 | 0.0068 | 0.0025 | |||
DT | 0.968 | 0.062 | 0.295 | 0.011 | 0.0003 | 0.0001 | 0.0004 | |||
AT | 0.987 | 0.035 | 0.388 | 0.009 | 0.0004 | 0.0019 | 0.0009 | |||
MPT | SCAD | 0.967 | 0.060 | 0.508 | 0.014 | 0.0003 | 0.0012 | 0.0021 | ||
HT | 0.988 | 0.001 | 0.479 | 0.008 | 0.0001 | 0.0021 | 0.0023 | |||
DT | 0.980 | 0.051 | 0.511 | 0.012 | 0.0003 | 0.0005 | 0.0004 | |||
AT | 0.974 | 0.063 | 0.432 | 0.009 | 0.0003 | 0.0035 | 0.0024 | |||
MPT | LASSO | 0.964 | 0.060 | 0.337 | 0.011 | 0.0003 | 0.0038 | 0.0051 | ||
HT | 0.986 | 0.003 | 0.436 | 0.006 | 0.0001 | 0.0003 | 0.0002 | |||
DT | 1.000 | 0.029 | 0.522 | 0.013 | 0.0001 | 0.0004 | 0.0003 | |||
AT | 0.981 | 0.034 | 0.320 | 0.007 | 0.0001 | 0.0006 | 0.0008 | |||
qn=100 | MPT | MCP | 0.985 | 0.010 | 0.511 | 0.009 | 0.0001 | 0.0004 | 0.0004 | |
HT | 0.973 | 0.038 | 0.374 | 0.009 | 0.0002 | 0.0022 | 0.0033 | |||
DT | 0.986 | 0.023 | 0.338 | 0.010 | 0.0001 | 0.0004 | 0.0001 | |||
AT | 0.982 | 0.023 | 0.470 | 0.005 | 0.0001 | 0.0004 | 0.0005 | |||
MPT | SCAD | 0.987 | 0.031 | 0.265 | 0.013 | 0.0002 | 0.0002 | 0.0003 | ||
HT | 0.988 | 0.038 | 0.458 | 0.015 | 0.0005 | 0.0017 | 0.0001 | |||
DT | 0.978 | 0.051 | 0.451 | 0.011 | 0.0001 | 0.0008 | 0.0004 | |||
AT | 0.985 | 0.010 | 0.422 | 0.009 | 0.0001 | 0.0058 | 0.0047 | |||
MPT | LASSO | 0.987 | 0.026 | 0.478 | 0.010 | 0.0001 | 0.0008 | 0.0012 | ||
HT | 0.966 | 0.044 | 0.364 | 0.011 | 0.0003 | 0.0029 | 0.0052 | |||
DT | 0.984 | 0.031 | 0.503 | 0.012 | 0.0001 | 0.0001 | 0.0003 | |||
AT | 0.987 | 0.031 | 0.401 | 0.008 | 0.0001 | 0.0016 | 0.0014 |
AMAE | AMSE | MSE | |||||||||
Model | Setting | Test | Penalty | TPR | FPR | g(⋅) | Prob | β | β1 | β2 | β3 |
Example 4.2 (n=1000) | qn=100 | MPT | MCP | 0.980 | 0.001 | 0.569 | 0.011 | 0.0001 | 0.0006 | 0.0025 | 0.0073 |
HT | 0.974 | 0.001 | 0.626 | 0.012 | 0.0003 | 0.0059 | 0.0167 | 0.0054 | |||
DT | 0.971 | 0.027 | 0.601 | 0.012 | 0.0002 | 0.0035 | 0.0022 | 0.0019 | |||
AT | 0.986 | 0.010 | 0.582 | 0.011 | 0.0001 | 0.0059 | 0.0011 | 0.0032 | |||
MPT | SCAD | 0.970 | 0.019 | 0.551 | 0.010 | ∗ | 0.0014 | 0.0006 | 0.0011 | ||
HT | 0.964 | 0.029 | 0.588 | 0.011 | 0.0001 | 0.0021 | 0.0041 | 0.0001 | |||
DT | 0.972 | 0.021 | 0.572 | 0.011 | 0.0001 | 0.0037 | 0.0002 | 0.0034 | |||
AT | 0.971 | 0.021 | 0.575 | 0.011 | 0.0001 | 0.0057 | 0.0005 | 0.0042 | |||
MPT | LASSO | 0.974 | 0.048 | 0.553 | 0.010 | 0.0001 | 0.0000 | 0.0002 | 0.0003 | ||
HT | 0.972 | 0.056 | 0.601 | 0.010 | 0.0001 | 0.0003 | 0.0001 | 0.0006 | |||
DT | 0.982 | 0.021 | 0.574 | 0.010 | 0.0001 | 0.0035 | 0.0001 | 0.0042 | |||
AT | 0.986 | 0.010 | 0.584 | 0.011 | 0.0001 | 0.0041 | 0.0002 | 0.0056 | |||
qn=500 | MPT | MCP | 0.964 | 0.011 | 0.562 | 0.013 | 0.0001 | 0.0005 | 0.0015 | 0.0042 | |
HT | 0.972 | 0.010 | 0.670 | 0.018 | 0.0001 | 0.0056 | 0.0001 | 0.0115 | |||
DT | 0.987 | 0.011 | 0.567 | 0.012 | ∗ | 0.0044 | 0.0003 | 0.0058 | |||
AT | 0.986 | 0.020 | 0.669 | 0.015 | 0.0001 | 0.0022 | 0.0108 | 0.0012 | |||
MPT | SCAD | 0.965 | 0.014 | 0.515 | 0.010 | 0.0001 | 0.0003 | 0.0055 | 0.0045 | ||
HT | 0.968 | 0.018 | 0.547 | 0.015 | 0.0001 | 0.0023 | 0.0112 | 0.0069 | |||
DT | 0.989 | 0.007 | 0.534 | 0.011 | 0.0001 | 0.0048 | 0.0001 | 0.0047 | |||
AT | 0.985 | 0.005 | 0.608 | 0.010 | ∗ | 0.0042 | 0.0021 | 0.0007 | |||
MPT | LASSO | 0.978 | 0.006 | 0.536 | 0.012 | 0.0001 | 0.0013 | 0.0132 | 0.0104 | ||
HT | 0.970 | 0.002 | 0.644 | 0.015 | 0.0001 | 0.0000 | 0.0092 | 0.0126 | |||
DT | 0.987 | 0.005 | 0.545 | 0.012 | ∗ | 0.0015 | 0.0007 | 0.0019 | |||
AT | 0.981 | 0.002 | 0.526 | 0.012 | ∗ | 0.0011 | 0.0093 | 0.0045 | |||
Symbol ∗ indicates value smaller than 0.0001. |
AMAE | AMSE | MSE | |||||||||
Model | Setting | Test | Penalty | TPR | FPR | g(⋅) | Prob | β | β1 | β2 | β3 |
Example 4.3 (qn=50) | n=500 | MPT | MCP | 0.951 | 0.103 | 0.466 | 0.019 | 0.0003 | 0.0003 | 0.0009 | 0.0011 |
HT | 0.966 | 0.091 | 0.571 | 0.021 | 0.0005 | 0.0007 | 0.0036 | 0.0045 | |||
DT | 0.982 | 0.043 | 0.360 | 0.006 | 0.0001 | 0.0002 | 0.0001 | 0.0001 | |||
AT | 0.981 | 0.021 | 0.464 | 0.012 | 0.0001 | 0.0005 | 0.0009 | 0.0006 | |||
MPT | SCAD | 0.957 | 0.139 | 0.527 | 0.023 | 0.0005 | 0.0001 | 0.0031 | 0.0098 | ||
HT | 0.968 | 0.082 | 0.433 | 0.020 | 0.0004 | 0.0006 | 0.0001 | 0.0003 | |||
DT | 0.954 | 0.140 | 0.411 | 0.013 | 0.0002 | 0.0011 | 0.0018 | 0.0012 | |||
AT | 0.972 | 0.064 | 0.793 | 0.018 | 0.0002 | 0.0038 | 0.0021 | 0.0004 | |||
MPT | LASSO | 0.981 | 0.024 | 0.604 | 0.021 | 0.0003 | 0.0042 | 0.0014 | 0.0019 | ||
HT | 0.983 | 0.021 | 0.432 | 0.026 | 0.0001 | 0.0017 | 0.0005 | 0.0016 | |||
DT | 0.971 | 0.094 | 0.470 | 0.013 | 0.0002 | 0.0004 | 0.0014 | 0.0023 | |||
AT | 0.980 | 0.061 | 0.447 | 0.013 | 0.0002 | 0.0002 | 0.0004 | 0.0015 | |||
n=1000 | MPT | MCP | 0.988 | 0.040 | 0.358 | 0.015 | 0.0002 | 0.0011 | 0.0024 | 0.0042 | |
HT | 0.984 | 0.021 | 0.399 | 0.017 | 0.0006 | 0.0008 | 0.0009 | 0.0013 | |||
DT | 0.989 | 0.000 | 0.583 | 0.014 | 0.0001 | 0.0001 | 0.0019 | 0.0024 | |||
AT | 0.985 | 0.009 | 0.405 | 0.013 | 0.0001 | 0.0017 | 0.0041 | 0.0012 | |||
MPT | SCAD | 0.989 | 0.043 | 0.537 | 0.016 | 0.0002 | 0.0025 | 0.0004 | 0.0038 | ||
HT | 0.987 | 0.003 | 0.512 | 0.012 | 0.0001 | 0.0012 | 0.0032 | 0.0031 | |||
DT | 0.986 | 0.003 | 0.515 | 0.012 | 0.0001 | 0.0001 | 0.0002 | 0.0004 | |||
AT | 1.000 | 0.000 | 0.410 | 0.013 | 0.0001 | 0.0013 | 0.0022 | 0.0013 | |||
MPT | LASSO | 0.988 | 0.004 | 0.441 | 0.011 | 0.0002 | 0.0029 | 0.0012 | 0.0021 | ||
HT | 0.982 | 0.007 | 0.326 | 0.007 | 0.0001 | 0.0002 | 0.0004 | 0.0002 | |||
DT | 0.987 | 0.008 | 0.489 | 0.013 | 0.0001 | 0.0008 | 0.0001 | 0.0032 | |||
AT | 0.977 | 0.043 | 0.283 | 0.007 | 0.0001 | 0.0012 | 0.0024 | 0.0034 |
AMAE | AMSE | MSE | ||||||||||
Model | Setting | Test | Penalty | TPR | FPR | g(⋅) | Prob | β | β1 | β2 | β3 | β4 |
Example 4.4 (qn=100) | n=750 | MPT | MCP | 0.979 | 0.053 | 0.744 | 0.019 | 0.0004 | 0.0028 | 0.0015 | 0.0036 | 0.0076 |
HT | 0.959 | 0.100 | 0.970 | 0.027 | 0.0011 | 0.0024 | 0.0005 | 0.0022 | 0.0018 | |||
DT | 0.986 | 0.035 | 0.611 | 0.011 | 0.0001 | 0.0001 | 0.0025 | 0.0034 | 0.0016 | |||
AT | 0.984 | 0.043 | 0.789 | 0.013 | 0.0002 | 0.0012 | 0.0051 | 0.0026 | 0.0012 | |||
MPT | SCAD | 0.966 | 0.059 | 0.723 | 0.014 | 0.0003 | 0.0030 | 0.0078 | 0.0081 | 0.0001 | ||
HT | 0.978 | 0.069 | 0.576 | 0.014 | 0.0002 | 0.0004 | 0.0083 | 0.0052 | 0.0002 | |||
DT | 0.989 | 0.063 | 0.698 | 0.013 | 0.0003 | 0.0034 | 0.0155 | 0.0011 | 0.0051 | |||
AT | 0.981 | 0.052 | 0.671 | 0.022 | 0.0005 | 0.0078 | 0.0023 | 0.0085 | 0.0073 | |||
MPT | LASSO | 0.977 | 0.072 | 0.620 | 0.014 | 0.0003 | 0.0047 | 0.0041 | 0.0141 | 0.0002 | ||
HT | 0.964 | 0.069 | 0.680 | 0.015 | 0.0003 | 0.0018 | 0.0071 | 0.0073 | 0.0007 | |||
DT | 0.986 | 0.041 | 0.581 | 0.016 | 0.0003 | 0.0034 | 0.0090 | 0.0014 | 0.0005 | |||
AT | 0.984 | 0.065 | 0.679 | 0.016 | 0.0003 | 0.0001 | 0.0095 | 0.0065 | 0.0003 | |||
n=1000 | MPT | MCP | 0.967 | 0.029 | 0.706 | 0.015 | 0.0002 | 0.0068 | 0.0097 | 0.0022 | 0.0015 | |
HT | 0.986 | 0.001 | 0.818 | 0.012 | 0.0001 | 0.0035 | 0.0061 | 0.0007 | 0.0001 | |||
DT | 0.987 | 0.032 | 0.872 | 0.012 | 0.0002 | 0.0007 | 0.0074 | 0.0017 | 0.0016 | |||
AT | 0.988 | 0.037 | 0.800 | 0.027 | 0.0002 | 0.0013 | 0.0061 | 0.0002 | 0.0025 | |||
MPT | SCAD | 0.961 | 0.059 | 0.724 | 0.015 | 0.0002 | 0.0081 | 0.0087 | 0.0030 | 0.0006 | ||
HT | 0.974 | 0.010 | 0.779 | 0.013 | 0.0001 | 0.0036 | 0.0066 | 0.0012 | 0.0001 | |||
DT | 0.983 | 0.071 | 0.405 | 0.010 | 0.0001 | 0.0013 | 0.0059 | 0.0008 | 0.0001 | |||
AT | 0.981 | 0.041 | 0.422 | 0.010 | 0.0001 | 0.0003 | 0.0009 | 0.0020 | 0.0011 | |||
MPT | LASSO | 0.977 | 0.029 | 0.819 | 0.017 | 0.0004 | 0.0057 | 0.0012 | 0.0083 | 0.0079 | ||
HT | 0.951 | 0.004 | 0.545 | 0.043 | 0.0001 | 0.0093 | 0.0004 | 0.0004 | 0.0025 | |||
DT | 0.985 | 0.021 | 0.408 | 0.009 | 0.0001 | 0.0002 | 0.0011 | 0.0026 | 0.0007 | |||
AT | 0.989 | 0.008 | 0.581 | 0.010 | 0.0001 | 0.0042 | 0.0003 | 0.0004 | 0.0008 |
We set up two different sample sizes (n) or covariate scenarios (qn) for each example. Results of Examples 4.1 and 4.2 suggest that our method maintains robust estimation performance as dimensionality increases in small sample scenarios. Furthermore, results of Examples 4.3 and 4.4 demonstrate that estimation accuracy improves with increased sample size. Figure 2 illustrates the estimation performance of g(⋅) and individual risk probabilities pi, confirming our method's efficacy in estimating unknown functions and risk probabilities.
Moreover, we aim to evaluate our method's performance under different group sizes. Using Example 4.4, we investigated group sizes of 2, 4, 6, and 8 with the Dorfman algorithm and LASSO penalty function. Results are presented in Table 6, reporting the means of ˆβj for j=1,2,3,4. The simulation results indicate that our method consistently delivers strong estimation performance across various group sizes. At the same time, we set up comparative experiments with different Se and Sp, and the simulation results are shown in Tables 8 to 11 in Appendix A. As shown in these tables, our model maintains a certain level of stability, ensuring that M∗ is still contained within ˆM.
AMAE | MEAN | |||||||||
Model | Setting | Group Size | TPR | FPR | g(⋅) | Prob | β1 | β2 | β3 | β4 |
Example 4 (qn=100) | n=750 | 2 | 0.970 | 0.015 | 0.611 | 0.011 | 0.452 | 0.465 | 0.478 | 0.460 |
4 | 0.965 | 0.020 | 0.581 | 0.016 | 0.445 | 0.405 | 0.464 | 0.477 | ||
6 | 0.986 | 0.041 | 0.627 | 0.009 | 0.519 | 0.497 | 0.487 | 0.495 | ||
8 | 0.973 | 0.020 | 0.594 | 0.012 | 0.471 | 0.467 | 0.484 | 0.477 | ||
n=1000 | 2 | 0.974 | 0.014 | 0.447 | 0.009 | 0.468 | 0.484 | 0.473 | 0.485 | |
4 | 0.964 | 0.018 | 0.408 | 0.009 | 0.489 | 0.468 | 0.450 | 0.474 | ||
6 | 0.985 | 0.021 | 0.440 | 0.011 | 0.486 | 0.478 | 0.443 | 0.471 | ||
8 | 0.974 | 0.010 | 0.466 | 0.009 | 0.494 | 0.494 | 0.447 | 0.437 |
In this section, we validate the effectiveness of our method using the diabetes dataset from the National Health and Nutrition Examination Survey (NHANES) conducted between 1999 and 2004. NHANES is a probability-based cross-sectional survey representing the U.S. population, collecting demographic, health history, and behavioral information through household interviews. Participants were also invited to equip mobile examination centers for detailed physical, psychological, and laboratory assessments. The dataset is accessible at https://wwwn.cdc.gov/Nchs/Nhanes/.
The dataset comprises n=5515 records and 17 variables, categorizing individuals as diabetic or non-diabetic. Covariates include age (X1), waist circumference (X2), BMI (X3), height (X4), weight (X5), smoking age (X6), alcohol use (X7), leg length (X8), total cholesterol (X9), hypertension (X10), education level (X11), household income (X12), family history (X13), physical activity (X14), gender (X15), and race (X16). Notably, nominal variables from X10 to X16 are transformed using one-hot encoding, resulting in qn=47 covariates per individual. The first nine variables are continuous, while the remainder are binary. A detailed explanation of the variables as well as the content of the questionnaire can be found at https://wwwn.cdc.gov/Nchs/Nhanes/search/default.aspx. For convenience, the nominal variables are explained in Table 12. in Appendix B.
For i∈{1,2,…,n}, we define ˜Yi=1 for diabetes and ˜Yi=0 for non-diabetes. Individual covariate information is represented as Xi=(Xi1,Xi2,…,Xiqn)⊤. We construct the following single-index model for the probability of diabetes risk for the i-th individual:
Pr(˜Yi=1|Xi)=exp[g(X⊤iβ)]1+exp[g(X⊤iβ)], |
where the smooth function g(⋅) is unknown, and our objective is to estimate the coefficients β.
To verify the accuracy of our method, we compare the results with those obtained from two other methods. The first method is penalized logistic regression (PLR), which uses the true individual status, ˜Yi. This method is implemented using the R package "glmnet". The second method is the adaptive elastic net for group testing (aenetgt) data, as introduced by Gregory et al. [23]. This approach utilizes group testing data and employs a penalized Expectation-Maximization (EM) algorithm to fit an adaptive elastic net logistic regression model. The R package "aenetgt" is used for implementation. We generate Dorfman group testing data with a group size of 6, setting both sensitivity and specificity at Se=Sp=0.98.
To ensure comparability, we adhere to the standardization techniques referenced in Cui et al. [31]. First, we center the covariates to facilitate the comparison of relative effects across different explanatory variables. Second, we normalize the PLR and aenetgt coefficients by dividing them by their L2-norm, as follows:
ˆβnormPLR=ˆβPLR‖ˆβPLR‖2,ˆβnormaenet=ˆβaenet‖ˆβaenet‖2, |
thereby obtaining coefficients with unit norm. This enables a comparison of regression coefficients from PLR, aenetgt, and the single-index group testing model.
The estimated coefficients from the three models are summarized in Table 7, and the parameter estimation of our method is denoted as ˆβour. In this study, the estimated coefficients for age, ˆβnormPLR and ˆβour, are 0.280 and 0.307, respectively, indicating that the risk of diabetes increases with age, consistent with the findings of Turi et al. [47]. However, the coefficient ˆβnormaenet is close to zero. For waist circumference, the coefficients ˆβnormPLR, ˆβour, and ˆβnormaenet are 0.178, 0.194, and 0.271, respectively, suggesting a positive association between waist circumference and diabetes risk, which is supported by Bai et al. [48] and Snijder et al. [49]. In addition, all three methods also identified leg length [50], hypertension [51], race [52], family history [53], and sex [54] as variables associated with diabetes. These covariates are widely recognized as being related to diabetes in the biomedical field [55].
Variable | ˆβnormPLR | ˆβour | ˆβnormaenet | Variable | ˆβnormPLR | ˆβour | ˆβnormaenet | Variable | ˆβnormPLR | ˆβour | ˆβnormaenet |
age | 0.280 | 0.307 | -0.085 | Family history | Household income | ||||||
waist circumference | 0.178 | 0.194 | 0.271 | family history1 | 0.000 | 0.000 | 0.000 | household income1 | 0.000 | 0.000 | 0.000 |
BMI | 0.000 | 0.000 | 0.000 | family history2 | -0.492 | -0.567 | -0.466 | household income2 | 0.024 | 0.000 | 0.000 |
height | 0.000 | 0.000 | 0.000 | family history9 | 0.000 | 0.000 | 0.000 | household income3 | 0.000 | 0.000 | 0.000 |
weight | 0.000 | 0.000 | 0.000 | Physical activity | household income4 | 0.000 | -0.069 | 0.000 | |||
smoking age | 0.000 | 0.007 | 0.000 | physical activity1 | 0.000 | 0.056 | 0.000 | household income5 | 0.000 | 0.000 | 0.000 |
alcohol use | 0.009 | 0.013 | 0.000 | physical activity2 | -0.086 | -0.018 | 0.000 | household income6 | 0.000 | 0.000 | 0.000 |
leg length | -0.048 | -0.100 | -0.043 | physical activity3 | -0.134 | -0.039 | 0.000 | household income7 | 0.000 | 0.000 | 0.000 |
total cholesterol | 0.000 | 0.000 | 0.000 | physical activity4 | -0.088 | 0.000 | 0.000 | household income8 | 0.001 | 0.065 | 0.000 |
Hypertension | physical activity9 | 0.000 | 0.000 | 0.000 | household income9 | 0.000 | 0.000 | 0.000 | |||
hypertension1 | 0.000 | 0.000 | 0.000 | Sex | household income10 | 0.000 | 0.000 | 0.000 | |||
hypertension2 | -0.350 | -0.372 | -0.641 | sex1 | -0.010 | 0.000 | 0.000 | household income11 | 0.000 | 0.000 | 0.000 |
Education | sex2 | -0.237 | -0.225 | -0.424 | household income12 | 0.000 | 0.000 | 0.000 | |||
education1 | 0.000 | 0.000 | 0.000 | race | household income13 | 0.000 | 0.000 | 0.000 | |||
education2 | 0.000 | 0.000 | 0.000 | race1 | 0.000 | 0.000 | 0.000 | household income77 | 0.000 | 0.231 | 0.000 |
education3 | 0.000 | 0.000 | 0.000 | race2 | -0.019 | -0.073 | 0.000 | household income99 | 0.000 | 0.000 | 0.000 |
education4 | 0.000 | 0.000 | 0.000 | race3 | -0.399 | -0.380 | -0.330 | ||||
education5 | -0.014 | -0.052 | 0.000 | race4 | 0.000 | 0.000 | 0.000 | ||||
education7 | -0.523 | -0.335 | 0.000 | race5 | 0.000 | 0.124 | 0.000 |
We found that the covariable physical activity is associated with diabetes, but the aenetgt method failed to identify this association. The results of a study by Yu et al. [55], which used the same dataset as ours, are consistent with this finding. In addition, we found that education level was also a covariable associated with diabetes (ˆβnormPLR and ˆβour are -0.523 and -0.335). Evidence for this association can also be found in the study by Aldossari et al. [56], and in this dataset, the probability that these participants will not develop diabetes is 100%. We also identified that household income is associated with diabetes, which is consistent with the study by Yen et al. [57]. In this dataset, the probability of developing diabetes for those who refused to answer about their household income is 60%. Furthermore, our model yields results similar to those obtained by the PLR method, which uses individual observations (˜Y), suggesting that our method is able to extract information from group observations.
This study presents a group testing framework based on a logistic regression single-index model for disease screening in low-prevalence environments. By employing B-splines to estimate unknown functions and incorporating penalty functions, our approach achieves high flexibility in capturing the relationships between covariates and individual risk probabilities while accurately identifying important variables. To address potential computational challenges in individual disease status estimation, we implemented an iterative EM algorithm for model estimation. Our simulation experiments demonstrate the proposed method's performance in high-dimensional covariate contexts with limited sample sizes, while application to real data confirms its efficacy. Our framework offers a unified approach for various group testing methods, showcasing its practical application value.
Despite these promising outcomes, our study acknowledges several limitations. First, our model assumes that sensitivity and specificity of testing are independent of group size, which may not always hold in practical applications. Second, data quality and variations in the testing population can impact the model's applicability. Therefore, exploring how to integrate prior information to enhance model accuracy and practical value remains a critical research direction. Furthermore, the potential high dimensionality of individual covariates poses significant challenges, necessitating the development of models capable of handling ultra-high-dimensional data.
Future research could explore the following directions. Firstly, examining model performance under varying group testing configurations, such as changes in testing errors and group sizes, could yield valuable insights. Secondly, investigating methods to incorporate additional prior knowledge to improve estimation accuracy is a worthwhile endeavor. Additionally, considering computational efficiency, developing faster algorithms for processing large-scale datasets will be a key focus for future work.
Changfu Yang: Methodolog, formal analysis, writing-original draft; Wenxin Zhou: Methodology, formal analysis; Wenjun Xiong: Conceptualization, methodology, writing-original draft, funding acquisition; Junjian Zhang: Conceptualization, methodology, writing-review and editing, funding acquisition; Juan Ding: Conceptualization, formal analysis, writing-review and editin, funding acquisition. All authors have read and approved the final version of the manuscript for publication.
The authors declare that they have not used Artificial Intelligence (AI) tools in the creation of this article.
This research was supported by the National Natural Science Foundation of China (Grant Nos. 12361055, 11801102), Guangxi Natural Science Foundation (2021GXNSFAA220054), and the Fundamental Research Funds for the Central Universities (B240201095).
The authors declare that there are no conflicts of interest regarding the publication of this paper.
In this part, we tested the performance of four examples at different sensitivity and specificity, using the Dofman algorithm and the LASSO penalty function. The simulation results are shown in Tables 8 to 11.
AMAE | AMSE | MSE | |||||||
Model | Setting | (Se,Sp) | TPR | FPR | g(⋅) | Prob | β | β1 | β2 |
Example 4.1 (qn=50) | n=500 | (0.98, 0.98) | 1.000 | 0.029 | 0.522 | 0.013 | 0.0001 | 0.0004 | 0.0003 |
(0.95, 0.95) | 0.987 | 0.020 | 0.474 | 0.011 | 0.0001 | 0.0003 | 0.0003 | ||
(0.90, 0.90) | 0.982 | 0.036 | 0.532 | 0.011 | 0.0001 | 0.0006 | 0.0007 | ||
(0.85, 0.85) | 0.984 | 0.040 | 0.578 | 0.016 | 0.0003 | 0.0001 | 0.0002 |
AMAE | AMSE | MSE | ||||||||
Model | Setting | (Se,Sp) | TPR | FPR | g(⋅) | Prob | β | β1 | β2 | β3 |
Example 4.2 (qn=100) | n=1000 | (0.98, 0.98) | 0.982 | 0.021 | 0.574 | 0.010 | 0.0001 | 0.0035 | 0.0001 | 0.0042 |
(0.95, 0.95) | 0.975 | 0.030 | 0.612 | 0.011 | 0.0001 | 0.0047 | 0.0001 | 0.0069 | ||
(0.90, 0.90) | 0.978 | 0.020 | 0.556 | 0.012 | 0.0001 | 0.0023 | 0.0002 | 0.0049 | ||
(0.85, 0.85) | 0.965 | 0.020 | 0.717 | 0.016 | 0.0004 | 0.0158 | 0.0002 | 0.0212 |
AMAE | AMSE | MSE | ||||||||
Model | Setting | (Se,Sp) | TPR | FPR | g(⋅) | Prob | β | β1 | β2 | β3 |
Example 4.3 (qn=50) | n=1000 | (0.98, 0.98) | 0.987 | 0.008 | 0.489 | 0.013 | 0.0001 | 0.0008 | 0.0001 | 0.0032 |
(0.95, 0.95) | 0.971 | 0.064 | 0.404 | 0.011 | 0.0003 | 0.0005 | 0.0033 | 0.0085 | ||
(0.90, 0.90) | 0.963 | 0.048 | 0.465 | 0.011 | 0.0001 | 0.0002 | 0.0012 | 0.0055 | ||
(0.85, 0.85) | 0.966 | 0.018 | 0.377 | 0.015 | 0.0004 | 0.0016 | 0.0023 | 0.0007 |
AMAE | AMSE | MSE | |||||||||
Model | Setting | (Se,Sp) | TPR | FPR | g(⋅) | Prob | β | β1 | β2 | β3 | β4 |
Example 4.4 (qn=100) | n=750 | (0.98, 0.98) | 0.986 | 0.041 | 0.581 | 0.016 | 0.0003 | 0.0034 | 0.0090 | 0.0014 | 0.0005 |
(0.95, 0.95) | 0.981 | 0.026 | 0.534 | 0.018 | 0.0001 | 0.0016 | 0.0045 | 0.0018 | 0.0005 | ||
(0.90, 0.90) | 0.974 | 0.018 | 0.546 | 0.016 | 0.0002 | 0.0004 | 0.0024 | 0.0014 | 0.0028 | ||
(0.85, 0.85) | 0.976 | 0.024 | 0.539 | 0.011 | 0.0002 | 0.0047 | 0.0085 | 0.0004 | 0.0039 |
Variable | Implication | Variable | Implication |
Hypertension circumstance | Family history of diabetes | ||
hypertension1 | Have a history of hypertension | family history1 | Blood relatives with diabetes |
hypertension2 | No history of hypertension | family history2 | Blood relatives do not have diabetes |
Education level | family history9 | Not known if any blood relatives have diabetes | |
education1 | Less Than 9th Grade | Physical activity | |
education2 | 9 - 11th Grade (Includes 12th grade with no diploma) | physical activity1 | Sit during the day and do not walk about very much |
education3 | High School Grad/GED or Equivalent | physical activity2 | Stand or walk about a lot during the day, but do not have to carry or lift things very often |
education4 | Some College or AA degree | physical activity3 | Lift light load or has to climb stairs or hills often |
education5 | College Graduate or above | physical activity4 | Do heavy work or carry heavy loads |
education7 | Refuse to answer about the level of education | physical activity9 | Don't know physical activity level |
Household income | Sex | ||
household income1 | 0 to 4,999 fanxiexian_myfh | sex1 | Male |
household income2 | 5,000 to 9,999 fanxiexian_myfh | sex2 | Female |
household income3 | 10,000 to 14,999 fanxiexian_myfh | Race/Ethnicity | |
household income4 | 15,000 to 19,999 fanxiexian_myfh | race1 | Mexican American |
household income5 | 20,000 to 24,999 fanxiexian_myfh | race2 | Other Hispanic |
household income6 | 25,000 to 34,999 fanxiexian_myfh | race3 | Non - Hispanic White |
household income7 | 35,000 to 44,999 fanxiexian_myfh | race4 | Non - Hispanic Black |
household income8 | 45,000 to 54,999 fanxiexian_myfh | race5 | Other Race - Including Multi - Racial |
household income9 | 55,000 to 64,999 fanxiexian_myfh | ||
household income10 | 65,000 to 74,999 fanxiexian_myfh | ||
household income11 | 75,000 and Over fanxiexian_myfh | ||
household income12 | Over 20,000 fanxiexian_myfh | ||
household income13 | Under 20,000 fanxiexian_myfh | ||
household income77 | Refusal to answer about household income | ||
household income99 | Don't know household income |
In this part, we derive the conditional expectation formulas for Dorfman testing, halving testing, and array testing within the framework of our method. Before proceeding, it is necessary to clarify some notations. Let Pj∖{i} represent the set of individuals in Pj excluding the i-th individual, and |Pj| denotes the number of individuals in Pj. Let Yi represent the test result of the i-th individual and YPj,l={Yi,i∈Pj,l} represent the set of testing results of individuals in Pj,l.
If the initial group testing result is negative, no re-testing is performed. However, if Zj,1=1, each individual in the group needs to undergo a separate re-testing.
1) When Zj,1=0, the result is the same as the master poor testing:
w(t)i,0=P(˜Yi=1,Zj,1=0)P(Zj,1=0)=(1−Se)⋅p(t)iB1−[Se+(1−Se−Sp)∏i∈Pj(1−p(t)iB)]. |
2) When Zj,1=1, each individual in the group must undergo a separate re-test. In total, the group has undergone |Pj|+1 tests.
w(t)i,1=P(˜Yi=1,Zj,1,YPj)P(Zj,1,YPj)=P(˜Yi=1)P(Zj,1,YPj|˜Yi=1)P(Zj,1,YPj). |
The denominator is
P(Zj,1,YPj)=∑˜YPjP(Zj,1,YPj|˜YPj)P(˜YPj)=∑˜YPjP(Zj,1|˜Zj,1)∏i∈PjP(Yi|˜Yi)P(˜Yi)=∑˜YPj[S˜Zj,1e(1−Sp)1−˜Zj,1]∏i∈Pj[SYie(1−Se)(1−Yi)]˜Yi×[(1−Sp)YiS(1−Yi)p](1−˜Yi)[p(t)iB]˜Yi[1−p(t)iB]1−˜Yi=∑˜YPj[S˜Zj,1e(1−Sp)1−˜Zj,1]∏i∈Pj[SYie(1−Se)(1−Yi)p(t)iB]˜Yi×[(1−Sp)YiS(1−Yi)p(1−p(t)iB)](1−˜Yi). |
Thus, the numerator is
P(˜Yi=1,Zj,1,YPj)=P(Zj,1,YPj|˜Yi=1)P(˜Yi=1)=∑˜YPj∖iP(Zj,1,YPj|˜Yi=1,˜YPj∖i)P(˜YPj∖i)P(˜Yi=1)=∑˜YPj∖iP(Zj,1|˜Zj,1=1)P(Yi|˜Yi=1)∏i∈Pj∖{i}P(Yi|˜Yi)P(˜Yi)P(˜Yi=1)=∑˜YPj∖iSeSYie(1−Se)(1−Yi)∏i∈Pj∖{i}[SYie(1−Se)(1−Yi)]˜Yi×[(1−Sp)YiS(1−Yi)p](1−˜Yi)[p(t)iB]˜Yi[1−p(t)iB]1−˜Yip(t)iB=∑˜YPj∖iS1+Yie(1−Se)(1−Yi)p(t)iB×∏i∈Pj∖{i}[SYie(1−Se)(1−Yi)p(t)iB]˜Yi×[(1−Sp)YiS(1−Yi)p(1−p(t)iB)](1−˜Yi). |
Therefore, the final expression is
w(t)i=Zj,1w(t)i,1+(1−Zj,1)w(t)i,0. |
Assume that the maximum number of partitions required during testing is two. Let the test result of the first testing be Zj,1. At this time, the set of all unpartitioned individuals is Pj,1, which contains |Pj| individuals. After the first partition, the partitioning method is to divide into two equal parts, with the two subsets of individuals being Pj,2 and Pj,3, respectively. The responses of the second testing are Zj,2 and Zj,3. There are five types of testing results in halving testing.
1) When Zj,1=0:
Only one testing is performed, and the process is the same as master pool testing. Since the result of one testing is negative, no further partitioning and testing are performed. At this time,
w(t)i=P(˜Yi=1|Zj,1=0)=P(˜Yi=1,Zj,1=0)P(Zj,1=0)=P(˜Yi=1)P(Zj,1=0|˜Yi=1)P(Zj,1=0)=p(t)iB(1−Se)1−[Se+(1−Se−Sp)∏i∈Pj(1−p(t)iB)]. |
2) When Zj,1=1,Zj,2=0,Zj,3=0:
That is, the result of the first testing is Zj,1=1. Subsequently, the first partition is performed, dividing into two equal parts Pj,2 and Pj,3. Then, testings are performed on the two sets respectively, with the testing results being Zj,2=Zj,3=0. At this time,
w(t)i=P(˜Yi=1|Zj,1=1,Zj,2=0,Zj,3=0)=P(Zj,1=1,Zj,2=0,Zj,3=0|˜Yi=1)P(˜Yi=1)P(Zj,1=1,Zj,2=0,Zj,3=0). |
The denominator is
P(Zj,1=1,Zj,2=0,Zj,3=0)=∑˜YPj,1P(Zj,1=1,Zj,2=0,Zj,3=0|˜YPj,1)P(˜YPj,2)P(˜YPj,3)=∑˜YPj,1P(Zj,1=1|˜YPj,1)P(Zj,2=0|˜YPj,2)P(Zj,3=0|˜YPj,3)P(˜YPj,2)P(˜YPj,3)=∑˜YPj,1[S˜Zj,1e(1−Sp)1−˜Zj,1][(1−Se)˜Zj,2S1−˜Zj,2p]∏i∈Pj,2[p(t)iB]˜Yi[1−p(t)iB]1−˜Yi×[(1−Se)˜Zj,3S1−˜Zj,3p]∏i∈Pj,3[p(t)iB]˜Yi[1−p(t)iB]1−˜Yi=∑˜YPj,1[S˜Zj,1e(1−Sp)1−˜Zj,1]3∏u=2(1−Se)˜Zj,uS1−˜Zj,up∏i∈Pj[p(t)iB]˜Yi[1−p(t)iB]1−˜Yi. |
Since the placement of the i-th individual in the sets Pj,2 and Pj,3 is symmetric, assume that i-th individual is placed in the set Pj,2. Then, the numerator is
P(Zj,1=1,Zj,2=0,Zj,3=0,˜Yi=1)=P(Zj,1=1,Zj,2=0,Zj,3=0|˜Yi=1)P(˜Yi=1)=∑˜YPj∖iP(Zj,1=1,Zj,2=0,Zj,3=0|˜Yi=1,˜YPj,2)×P(˜YPj,2∖i)P(˜YPj,3)P(˜Yi=1)=∑˜YPj∖iP(Zj,1=1|˜Zj,1=1)P(Zj,2=0|˜Zj,2=1)P(Zj,3=0|˜Zj,3)×P(˜YPj,2∖i)P(˜YPj,3)P(˜Yi=1)=∑˜YPj∖iSe(1−Se)∏i∈Pj,2∖{i}[p(t)iB]˜Yi[1−p(t)iB]1−˜Yi(1−Se)˜Zj,3S1−˜Zj,3p∏i∈Pj,3[p(t)iB]˜Yi[1−p(t)iB]1−˜Yip(t)iB=∑˜YPj∖iSe(1−Se)1+˜Zj,3S1−˜Zj,3pp(t)iB∏i∈Pj∖{i}[p(t)iB]˜Yi[1−p(t)iB]1−˜Yi. |
3) When Zj,1=1,Zj,2=0,Zj,3=1:
At this time, the second partitions are performed. The first partition divides all individuals into two sets, Pj,2 and Pj,3, with testing results Zj,2=0 and Zj,3=1, respectively. Individual testings are performed separately on the individuals in Pj,3, and the set of testing results is YPj,3. At this time,
w(t)i=P(˜Yi=1|Zj,1=1,Zj,2=0,Zj,3=1,YPj,3)=P(Zj,1=1,Zj,2=0,Zj,3=1,YPj,3|˜Yi=1)P(˜Yi=1)P(Zj,1=1,Zj,2=0,Zj,3=1,YPj,3). |
The denominator is
P(Zj,1=1,Zj,2=0,Zj,3=1,YPj,3)=∑˜YPjP(Zj,1=1,Zj,2=0,Zj,3=1,YPj,3|˜YPj,2,˜YPj,3)P(˜YPj,2)P(˜YPj,3)=∑˜YPjP(Zj,1=1|˜YPj)P(Zj,2=0|˜YPj,2)P(Zj,3=1|˜YPj,3)×P(˜YPj,3)P(˜YPj,2)P(YPj,3|˜YPj,3)=∑˜YPjP(Zj,1=1|˜Zj,1)P(Zj,2=0|˜Zj,2)P(Zj,3=1|˜Zj,3)×∏i∈Pj,3P(Yi|˜Yi)P(˜YPj,2)P(˜YPj,3)=∑˜YPj[S˜Zj,1e(1−Sp)1−˜Zj,1][(1−Se)˜Zj,2S1−˜Zj,2p][S˜Zj,3e(1−Sp)1−˜Zj,3]×∏i∈Pj,2[p(t)iB]˜Yi[1−p(t)iB]1−˜Yi∏i∈Pj,3[p(t)iB]˜Yi[1−p(t)iB]1−˜Yi×∏i∈Pj,3[SYie(1−Se)1−Yi]˜Yi[(1−Sp)YiS1−Yip]1−˜Yi=∑˜YPj[S˜Zj,1+˜Zj,3e(1−Sp)2−˜Zj,1−˜Zj,3][(1−Se)˜Zj,2S1−˜Zj,2p]×∏i∈Pj[p(t)iB]˜Yi[1−p(t)iB]1−˜Yi∏i∈Pj,3[SYie(1−Se)1−Yi]˜Yi[(1−Sp)YiS1−Yip]1−˜Yi. |
Since an i-th individual may belong to either set Pj,2 or Pj,3, the numerator is discussed accordingly.
(a) Assume that i-th individual belongs to set Pj,2. Then, the numerator is
\begin{align*} &P(\tilde{Y}_i = 1, Z_{j, 1} = 1, Z_{j, 2} = 0, Z_{j, 3} = 1, \mathcal{Y}_{\mathcal{P}_{j, 3}})\\ = &\sum\limits_{\tilde{\mathcal{Y}}_{\mathcal{P}_{j} \setminus i}}P(Z_{j, 1} = 1, Z_{j, 2} = 0, Z_{j, 3} = 1, \mathcal{Y}_{\mathcal{P}_{j, 3}}|\tilde{Y}_i = 1, \tilde{\mathcal{Y}}_{\mathcal{P}_{j, 2} \setminus i}, \tilde{\mathcal{Y}}_{\mathcal{P}_{j, 3}}) \\ &\times P(\tilde{\mathcal{Y}}_{\mathcal{P}_{j, 2} \setminus i}, \tilde{\mathcal{Y}}_{\mathcal{P}_{j, 3}})P(\tilde{Y}_i = 1)\\ = &\sum\limits_{\tilde{\mathcal{Y}}_{\mathcal{P}_{j} \setminus i}}P(Z_{j, 1} = 1|\tilde{Z}_{j, 1} = 1)P(Z_{j, 2} = 0|\tilde{Z}_{j, 2} = 1)P(Z_{j, 3} = 1|\tilde{Z}_{j, 3})\\ &\times P(\mathcal{Y}_{\mathcal{P}_{j, 3}}|\tilde{\mathcal{Y}}_{\mathcal{P}_{j, 3}})P(\tilde{\mathcal{Y}}_{\mathcal{P}_{j, 2} \setminus i})P(\tilde{\mathcal{Y}}_{\mathcal{P}_{j, 3}})P(\tilde{Y}_i = 1)\\ = &\sum\limits_{\tilde{\mathcal{Y}}_{\mathcal{P}_{j} \setminus i}}S_e(1-S_e) S_{e}^{\tilde{Z}_{j, 3}}(1-S_p)^{1-\tilde{Z}_{j, 3}} \prod\limits_{i\in \mathcal{P}_{j, 2} \setminus \{i\}} \left [p_{iB}^{(t)}\right ]^{\tilde{Y}_{i}} \left [1-p_{iB}^{(t)}\right ]^{1-\tilde{Y}_{i}}\\ &\times \prod\limits_{i\in \mathcal{P}_{j, 3}}\left [p_{iB}^{(t)}\right ]^{\tilde{Y}_{i}}\left [1-p_{iB}^{(t)}\right ]^{1-\tilde{Y}_{i}}p_{iB}^{(t)}\\ & \times \prod\limits_{i\in \mathcal{P}_{j, 3}} \left [S_e^{Y_{i}}(1-S_e)^{1-{Y_{i}}}\right ]^{\tilde{Y}_{i}} \left [(1-S_p)^{Y_{i}}S_p^{1-Y_{i}}\right ]^{1-{\tilde{Y}_{i}}} . \end{align*} |
(b) Assume that i -th individual belongs to set \mathcal{P}_{j, 3} . Then, the numerator is
\begin{align*} & P(\tilde{Y}_i = 1, Z_{j, 1} = 1, Z_{j, 2} = 0, Z_{j, 3} = 1, \mathcal{Y}_{\mathcal{P}_{j, 3}} ) \\ = & \sum\limits_{\tilde{\mathcal{Y}}_{\mathcal{P}_{j} \setminus i}} P(Z_{j, 1} = 1, Z_{j, 2} = 0, Z_{j, 3} = 1, \mathcal{Y}_{\mathcal{P}_{j, 3}} | \tilde{Y}_i = 1, \tilde{\mathcal{Y}}_{\mathcal{P}_{j, 2}}, \tilde{\mathcal{Y}}_{\mathcal{P}_{j, 3} \setminus i} ) \\ &\times P(\tilde{\mathcal{Y}}_{\mathcal{P}_{j, 2}}, \tilde{\mathcal{Y}}_{\mathcal{P}_{j, 3} \setminus i}) P(\tilde{Y}_i = 1) \\ = & \sum\limits_{\tilde{\mathcal{Y}}_{\mathcal{P}_{j} \setminus i}} P(Z_{j, 1} = 1 | \tilde{Z}_{j, 1} = 1) P(Z_{j, 2} = 0 | \tilde{Z}_{j, 2}) P(Z_{j, 3} = 1 | \tilde{Z}_{j, 3} = 1) \\ &\times P(\mathcal{Y}_{\mathcal{P}_{j, 3} \setminus i} | \tilde{\mathcal{Y}}_{\mathcal{P}_{j, 3} \setminus i}) P(Y_i | \tilde{Y}_i = 1) P(\tilde{\mathcal{Y}}_{\mathcal{P}_{j, 2}}) P(\tilde{\mathcal{Y}}_{\mathcal{P}_{j, 3} \ \setminus i}) P(\tilde{Y}_i = 1) \\ = & \sum\limits_{\tilde{\mathcal{Y}}_{\mathcal{P}_{j} \setminus i}} S_e^2 (1-S_e)^{\tilde{Z}_{j, 2}} S_p^{1-\tilde{Z}_{j, 2}} p_{iB}^{(t)} S_e^{Y_i} (1-S_e)^{1-Y_i} \\ & \times \prod\limits_{i\in \mathcal{P}_{j, 3} \setminus \{i\}} \left[ S_e^{Y_{i}}(1-S_e)^{1-{Y_{i}}} \right]^{\tilde{Y}_{i}} \left[ (1-S_p)^{Y_{i}} S_p^{1-Y_{i}} \right]^{1-{\tilde{Y}_{i}}} \\ & \times \prod\limits_{i\in \mathcal{P}_j \setminus \{i\}} \left[ p_{iB}^{(t)} \right]^{\tilde{Y}_{i}} \left[ 1-p_{iB}^{(t)} \right]^{1-\tilde{Y}_{i}} . \end{align*} |
4) When Z_{j, 1} = 1, Z_{j, 2} = 1 , and Z_{j, 3} = 0 , the process is the same as when Z_{j, 1} = 1, Z_{j, 2} = 0 , and Z_{j, 3} = 1 , and the numerator needs to be discussed accordingly. At this time,
\begin{align*} {w}^{(t)}_i & = P(\tilde{Y}_i = 1 | Z_{j, 1} = 1, Z_{j, 2} = 1, Z_{j, 3} = 0, \mathcal{Y}_{\mathcal{P}_{j, 2}} ) \\ & = \frac{ P( Z_{j, 1} = 1, Z_{j, 2} = 1, Z_{j, 3} = 0, \mathcal{Y}_{\mathcal{P}_{j, 2}} | \tilde{Y}_i = 1 ) P(\tilde{Y}_i = 1) } { P( Z_{j, 1} = 1, Z_{j, 2} = 1, Z_{j, 3} = 0, \mathcal{Y}_{\mathcal{P}_{j, 2}} ) }. \end{align*} |
First, the denominator is
\begin{align*} &P(Z_{j, 1} = 1, Z_{j, 2} = 1, Z_{j, 3} = 0, \mathcal{Y}_{\mathcal{P}_{j, 2}})\\ = &\sum\limits_{\tilde{\mathcal{Y}}} P(Z_{j, 1} = 1, Z_{j, 2} = 1, Z_{j, 3} = 0, \mathcal{Y}_{\mathcal{P}_{j, 2}} | \tilde{\mathcal{Y}}_{\mathcal{P}_{j, 2}}, \tilde{\mathcal{Y}}_{\mathcal{P}_{j, 3}} ) P(\tilde{\mathcal{Y}}_{\mathcal{P}_{j, 2}}) P(\tilde{\mathcal{Y}}_{\mathcal{P}_{j, 3}})\\ = &\sum\limits_{\tilde{\mathcal{Y}}} P(Z_{j, 1} = 1 | \tilde{Z}_{j, 1} ) P(Z_{j, 2} = 1 | \tilde{Z}_{j, 2} ) P(Z_{j, 3} = 0 | \tilde{Z}_{j, 3} ) \\ & \times \prod\limits_{i\in \mathcal{P}_{j, 2}} P(Y_{i} | \tilde{Y}_{i} ) P(\tilde{\mathcal{Y}}_{\mathcal{P}_{j, 2}}) P(\tilde{\mathcal{Y}}_{\mathcal{P}_{j, 3}})\\ = &\sum\limits_{\tilde{\mathcal{Y}}} S_e^{\tilde{Z}_{j, 1}+\tilde{Z}_{j, 2}} (1-S_p)^{2-\tilde{Z}_{j, 1}-\tilde{Z}_{j, 2}} (1-S_e)^{\tilde{Z}_{j, 3}} S_p^{1-\tilde{Z}_{j, 3}} \\ & \times \prod\limits_{i\in {\mathcal{P}_j}} [ p_{iB}^{(t)} ]^{\tilde{Y}_{i}} [ 1-p_{iB}^{(t)} ]^{1-\tilde{Y}_{i}} \prod\limits_{i\in \mathcal{P}_{j, 2}} [ S_e^{Y_{i}} (1-S_e)^{1-Y_{i}} ]^{\tilde{Y}_{i}} [ (1-S_p)^{Y_{i}} S_p^{1-Y_{i}} ]^{1-\tilde{Y}_{i}} . \end{align*} |
Next, the numerator is discussed.
(a) Assume that i -th individual belongs to set \mathcal{P}_{j, 2} . Then, the numerator is
\begin{align*} &P(\tilde{Y}_i = 1, Z_{j, 1} = 1, Z_{j, 2} = 1, Z_{j, 3} = 0, \mathcal{Y}_{\mathcal{P}_{j, 2}})\\ = &\sum\limits_{\tilde{\mathcal{Y}}_{\mathcal{P}_{j} \setminus i}} P(Z_{j, 1} = 1, Z_{j, 2} = 1, Z_{j, 3} = 0, \mathcal{Y}_{\mathcal{P}_{j, 2}} | \tilde{Y}_i = 1, \tilde{\mathcal{Y}}_{\mathcal{P}_{j, 2} \setminus i}, \tilde{\mathcal{Y}}_{\mathcal{P}_{j, 3}} ) P(\tilde{\mathcal{Y}}_{\mathcal{P}_{j, 2} \setminus i}, \tilde{\mathcal{Y}}_{\mathcal{P}_{j, 3}}) P(\tilde{Y}_i = 1)\\ = &\sum\limits_{\tilde{\mathcal{Y}}_{\mathcal{P}_{j} \setminus i}} P(Z_{j, 1} = 1 | \tilde{Z}_{j, 1} = 1) P(Z_{j, 2} = 1 | \tilde{Z}_{j, 2} = 1) P(Z_{j, 3} = 0 | \tilde{Z}_{j, 3} )\\ &\times P(\mathcal{Y}_{\mathcal{P}_{j, 2} \setminus i} | \tilde{\mathcal{Y}}_{\mathcal{P}_{j, 2} \setminus i}) P(Y_i | \tilde{Y}_i = 1) P(\tilde{\mathcal{Y}}_{\mathcal{P}_{j, 2} \setminus i}) P(\tilde{\mathcal{Y}}_{\mathcal{P}_{j, 3}}) P(\tilde{Y}_i = 1)\\ = &\sum\limits_{\tilde{\mathcal{Y}}_{\mathcal{P}_{j} \setminus i}} S_e^2 (1-S_e)^{\tilde{Z}_{j, 3}} S_p^{1-\tilde{Z}_{j, 3}} S_e^{Y_i} (1-S_e)^{1-Y_i} p_{iB}^{(t)}\\ &\times \prod\limits_{i\in \mathcal{P}_{j, 2} \setminus \{i\}} [ S_e^{Y_{i}}(1-S_e)^{1-{Y_{i}}} ]^{\tilde{Y}_{i}} [ (1-S_p)^{Y_{i}} S_p^{1-Y_{i}} ]^{1-{\tilde{Y}_{i}}} \times \prod\limits_{i\in \mathcal{P}_j \setminus \{i\}} [ p_{iB}^{(t)} ]^{\tilde{Y}_{i}} [ 1-p_{iB}^{(t)} ]^{1-\tilde{Y}_{i}}. \end{align*} |
(b) Assume that i -th individual belongs to set \mathcal{P}_{j, 3} . Then, the numerator is
\begin{align*} &P(\tilde{Y}_i = 1, Z_{j, 1} = 1, Z_{j, 2} = 1, Z_{j, 3} = 0, \mathcal{Y}_{\mathcal{P}_{j, 2}})\\ = &P(Z_{j, 1} = 1, Z_{j, 2} = 1, Z_{j, 3} = 0, \mathcal{Y}_{\mathcal{P}_{j, 2}} | \tilde{Y}_i = 1) P(\tilde{Y}_i = 1)\\ = &\sum\limits_{\tilde{\mathcal{Y}}_{\mathcal{P}_{j} \setminus i}} P(Z_{j, 1} = 1, Z_{j, 2} = 1, Z_{j, 3} = 0, \mathcal{Y}_{\mathcal{P}_{j, 2}} | \tilde{Y}_i = 1, \tilde{\mathcal{Y}}_{\mathcal{P}_{j, 2}}, \tilde{\mathcal{Y}}_{\mathcal{P}_{j, 3} \setminus i} ) P(\tilde{\mathcal{Y}}_{\mathcal{P}_{j, 2}}, \tilde{\mathcal{Y}}_{\mathcal{P}_{j, 3} \setminus i}) P(\tilde{Y}_i = 1)\\ = &\sum\limits_{\tilde{\mathcal{Y}}_{\mathcal{P}_{j} \setminus i}} P(Z_{j, 1} = 1 | \tilde{Z}_{j, 1} = 1) P(Z_{j, 2} = 1 | \tilde{Z}_{j, 2} ) P(Z_{j, 3} = 0 | \tilde{Z}_{j, 3} = 1 )\\ & \times P(\mathcal{Y}_{\mathcal{P}_{j, 2}} | \tilde{\mathcal{Y}}_{\mathcal{P}_{j, 2}}) P(\tilde{\mathcal{Y}}_{\mathcal{P}_{j, 2}}) P(\mathcal{Y}_{\mathcal{P}_{j, 3} \setminus i} | \tilde{\mathcal{Y}}_{\mathcal{P}_{j, 3} \setminus i}) P(\tilde{\mathcal{Y}}_{\mathcal{P}_{j, 3} \setminus i}) P(\tilde{Y}_i = 1)\\ = &\sum\limits_{\tilde{\mathcal{Y}}_{\mathcal{P}_{j} \setminus i}} S_e(1-S_e) S_e^{\tilde{Z}_{j, 2}}(1-S_p)^{1-\tilde{Z}_{j, 2}} p_{iB}^{(t)} \times \prod\limits_{i\in \mathcal{P}_{j, 2}} [ S_e^{Y_{i}}(1-S_e)^{1-{Y_{i}}} ]^{\tilde{Y}_{i}}\\ & \times [(1-S_p)^{Y_{i}}S_p^{1-Y_{i}}]^{1-{\tilde{Y}_{i}}} \prod\limits_{i\in \mathcal{P}_j\setminus \{i\}} [ p_{iB}^{(t)} ]^{\tilde{Y}_{i}} [ 1-p_{iB}^{(t)} ]^{1-\tilde{Y}_{i}}. \end{align*} |
5) When Z_{j, 1} = 1, Z_{j, 2} = 1 , and Z_{j, 3} = 1 , two similar partitions are performed as above, and individual retests are conducted separately for all individuals in \mathcal{P}_j . At this time, \mathcal{Y}_{\mathcal{P}_{j}} = \mathcal{Y}_{\mathcal{P}_{j, 2}} \cup \mathcal{Y}_{\mathcal{P}_{j, 3}} , and we have
\begin{align*} {w}^{(t)}_i = & P(\tilde{Y}_i = 1 | Z_{j, 1} = 1, Z_{j, 2} = 1, Z_{j, 3} = 1, \mathcal{Y}_{\mathcal{P}_{j, 2}}, \mathcal{Y}_{\mathcal{P}_{j, 3}} )\\ = &\frac{ P(Z_{j, 1} = 1, Z_{j, 2} = 1, Z_{j, 3} = 1, \mathcal{Y}_{\mathcal{P}_{j}} | \tilde{Y}_i = 1 ) P(\tilde{Y}_i = 1) } { P(Z_{j, 1} = 1, Z_{j, 2} = 1, Z_{j, 3} = 1, \mathcal{Y}_{\mathcal{P}_{j}} ) }. \end{align*} |
The denominator is
\begin{align*} &P(Z_{j, 1} = 1, Z_{j, 2} = 1, Z_{j, 3} = 1, \mathcal{Y}_{\mathcal{P}_{j}})\\ = &\sum\limits_{\tilde{\mathcal{Y}}_{\mathcal{P}_{j}}} P(Z_{j, 1} = 1, Z_{j, 2} = 1, Z_{j, 3} = 1, \mathcal{Y}_{\mathcal{P}_{j}}| \tilde{\mathcal{Y}}_{\mathcal{P}_{j}}) P(\tilde{\mathcal{Y}}_{\mathcal{P}_{j}})\\ = &\sum\limits_{\tilde{\mathcal{Y}}_{\mathcal{P}_{j}}} P(Z_{j, 1} = 1 | \tilde{Z}_{j, 1}) P(Z_{j, 2} = 1 | \tilde{Z}_{j, 2}) P(Z_{j, 3} = 1 | \tilde{Z}_{j, 3}) \prod\limits_{i\in \mathcal{P}_j} P(Y_{i} | \tilde{Y}_{i}) P(\tilde{Y}_{i})\\ = &\sum\limits_{\tilde{\mathcal{Y}}_{\mathcal{P}_{j}}} S_e^{\tilde{Z}_{j, 1}} (1-S_p)^{1-\tilde{Z}_{j, 1}} \prod\limits_{u = 2}^{3} S_e^{\tilde{Z}_{j, u}} (1-S_p)^{1-\tilde{Z}_{j, u }}\\ &\times \prod\limits_{i\in \mathcal{P}_j} [ S_e^{Y_{i}} (1-S_e)^{1-{Y_{i}}} p_{iB}^{(t)} ]^{\tilde{Y}_{i}} [ (1-S_p)^{Y_{i}} S_p^{1-Y_{i}} (1-p_{iB}^{(t)}) ]^{1-{\tilde{Y}_{i}}}. \end{align*} |
The results of i -th individual belonging to either set \mathcal{P}_{j, 2} or \mathcal{P}_{j, 3} are symmetric. Assume that i -th individual belongs to set \mathcal{P}_{j, 2} . Then, the numerator is
\begin{align*} &P(Z_{j, 1} = 1, Z_{j, 2} = 1, Z_{j, 3} = 1, \mathcal{Y}_{\mathcal{P}_{j}}, \tilde{Y}_i = 1)\\ = &\sum\limits_{\tilde{\mathcal{Y}}_{\mathcal{P}_{j} \setminus i}} P(Z_{j, 1} = 1, Z_{j, 2} = 1, Z_{j, 3} = 1, \mathcal{Y}_{\mathcal{P}_{j}} | \tilde{Y}_i = 1, \tilde{\mathcal{Y}}_{\mathcal{P}_{j, 2} \setminus i}, \tilde{\mathcal{Y}}_{\mathcal{P}_{j, 3}} ) P(\tilde{\mathcal{Y}}_{\mathcal{P}_{j, 2} \setminus i}, \tilde{\mathcal{Y}}_{\mathcal{P}_{j, 3}})P(\tilde{Y}_i = 1)\\ = &\sum\limits_{\tilde{\mathcal{Y}}_{\mathcal{P}_{j} \setminus i}} P(Z_{j, 1} = 1 | \tilde{Z}_{j, 1} = 1)P(Z_{j, 2} = 1 | \tilde{Z}_{j, 2} = 1)P(Z_{j, 3} = 1 | \tilde{Z}_{j, 3})\\ & \times P(Y_i | \tilde{Y}_i = 1)P( \mathcal{Y}_{\mathcal{P}_{j} \setminus i} | \tilde{\mathcal{Y}}_{\mathcal{P}_{j} \setminus i}) P(\tilde{\mathcal{Y}}_{\mathcal{P}_{j} \setminus i})P(\tilde{Y}_i = 1)\\ = &\sum\limits_{\tilde{\mathcal{Y}}_{\mathcal{P}_{j} \setminus i}} S_e^2 S_e^{\tilde{Z}_{j, 3}} (1-S_p)^{1-\tilde{Z}_{j, 3}} p_{iB}^{(t)} S_e^{Y_i} (1-S_e)^{1-Y_i}\\ &\times \prod\limits_{i \in \mathcal{P}_j\setminus \{i\}} \bigg[ S_e^{Y_{i}} (1-S_e)^{1-{Y_{i}}} p_{iB}^{(t)} \bigg]^{\tilde{Y}_{i}} \bigg[ (1-S_p)^{Y_{i}} S_p^{1-Y_{i}} (1-p_{iB}^{(t)}) \bigg]^{1-\tilde{Y}_{i}}. \end{align*} |
For convenience, assume that the set of all individuals is G , and all individuals can be arranged into an R \times C array, that is, G = \left\{(r, c), r \in R, c \in C\right\}. Define \mathcal{R} = (R_1, R_2, \cdots, R_R) and \mathcal{C} = (C_1, C_2, \cdots, C_C) as the collections of row and column testing results, respectively. Let R = \max R_{r} and C = \max C_{c} . Furthermore, define \tilde{R}_r = \max_c \tilde{Y}_{rc} and \tilde{C}_c = \max_r \tilde{Y}_{rc} as the true result sets for rows and columns, respectively. Let Y_{rc} denote the testing result of the individual in the r -th row and c -th column of the array, and \tilde{Y}_{rc} represents the true disease status of the individual in the r -th row and c -th column of the array. Let
\begin{align*} Q = & \left\{(s, t) \mid R_s = 1, C_t = 1, 1 \leq s \leq R, 1 \leq t \leq C, \right. \\ & \left. \text{or } R_s = 1, C_1 = \cdots = C_C = 0, 1 \leq s \leq R, \right. \\ & \left. \text{or } R_1 = \cdots = R_R = 0, C_t = 1, 1 \leq t \leq C \right\}. \end{align*} |
\mathcal{Y}_Q represents the collection of responses from all potentially positive individuals, and \tilde{\mathcal{Y}}_Q denotes the true disease statuses of all potentially positive individuals. Let \mathcal{Z}_G = (R, C) denote the group testing responses. Since (r, c) \in G , define
\begin{equation*} \tilde{\mathcal{Y}}_{G \setminus (r, c)} = \left\{\tilde{Y}_{r' c'}, r' \in R \setminus \{r\}, c' \in C \setminus \{c\}\right\}. \end{equation*} |
Then,
\begin{equation*} {w}^{(t)}_{rc} = P(\tilde{Y}_{rc} = 1 \mid \mathcal{Z}_G, \mathcal{Y}_Q) = \frac{P(\tilde{Y}_{rc} = 1, \mathcal{Z}_G, \mathcal{Y}_Q)}{P(\mathcal{Z}_G, \mathcal{Y}_Q)}. \end{equation*} |
1) When \mathcal{Z}_G = (0, 0) , there is no need to retest individuals within the group. At this time,
\begin{equation*} {w}^{(t)}_{rc} = P\big(\tilde{Y}_{rc} = 1 \mid \mathcal{Z}_G = (0, 0)\big) = \frac{P\big(\tilde{Y}_{rc} = 1, \mathcal{Z}_G = (0, 0)\big)}{P\big(\mathcal{Z}_G = (0, 0)\big)}. \end{equation*} |
The denominator is
\begin{align*} P\big(\mathcal{Z}_G = (0, 0)\big) = &\sum\limits_{\tilde{\mathcal{Y}}_G}P\big(\mathcal{Z}_G = (0, 0) | \tilde{\mathcal{Y}}_G\big)P(\tilde{\mathcal{Y}}_G)\\ = &\sum\limits_{\tilde{\mathcal{Y}}_G}P(\mathcal{R} = 0 | \tilde{\mathcal{Y}}_G)P(\mathcal{C} = 0 | \tilde{\mathcal{Y}}_G)P(\tilde{\mathcal{Y}}_G)\\ = &\sum\limits_{\tilde{\mathcal{Y}}_G} \bigg[ \prod\limits_{r' = 1}^{R} P\Big(R_{r'} = 0 | \tilde{Y}_{r'1}, \tilde{Y}_{r'2}, \cdots, \tilde{Y}_{r'C} \Big) \bigg] \bigg[ \prod\limits_{c' = 1}^{C} P\Big(C_{c'} = 0 | \tilde{Y}_{1c'}, \tilde{Y}_{2c'}, \cdots, \tilde{Y}_{Rc'} \Big) \bigg] \\ & \times \prod\limits_{r' \in R} \prod\limits_{c' \in C} {p_{r'c'B}^{(t)}}^{\tilde{Y}_{r'c'}} \Big(1-{p_{r'c'B}^{(t)}}\Big)^{1-{\tilde{Y}_{r'c'}}}\\ = &\sum\limits_{\tilde{\mathcal{Y}}_G} \prod\limits_{r' = 1}^{R} \bigg[(1-S_e)^{\tilde{R}_{r'}} S_p^{1-\tilde{R}_{r'}} \bigg] \prod\limits_{c' = 1}^{C} \bigg[(1-S_e)^{\tilde{C}_{c'}} S_p^{1-\tilde{C}_{c'}} \bigg] \prod\limits_{(r', c') \in G} \bigg\{ {p_{r'c'B}^{(t)}}^{\tilde{Y}_{r'c'}} \Big(1-{p_{r'c'B}^{(t)}}\Big)^{1-{\tilde{Y}_{r'c'}}} \bigg\}\\ = &\sum\limits_{\tilde{\mathcal{Y}}_G} \prod\limits_{r' = 1}^{R} \prod\limits_{c' = 1}^{C} \bigg[ (1-S_e)^{\tilde{R}_{r'}+\tilde{C}_{c'}} S_p^{2-\tilde{R}_{r'}-\tilde{C}_{c'}} \bigg] \prod\limits_{(r', c') \in G} \bigg\{ {p_{r'c'B}^{(t)}}^{\tilde{Y}_{r'c'}} \Big(1-{p_{r'c'B}^{(t)}}\Big)^{1-{\tilde{Y}_{r'c'}}} \bigg\}. \end{align*} |
The numerator is
\begin{align*} P\big(\mathcal{Z}_G = (0, 0), \tilde{Y}_{rc} = 1\big) & = P\big(\mathcal{Z}_G = (0, 0) | \tilde{Y}_{rc} = 1\big) P(\tilde{Y}_{rc} = 1)\\ = &\sum\limits_{\tilde{\mathcal{Y}}_{G \setminus (r, c)}} P(\mathcal{R} = 0, \mathcal{C} = 0 | \tilde{\mathcal{Y}}_{G \setminus (r, c)}, \tilde{Y}_{rc} = 1) P(\tilde{\mathcal{Y}}_{G \setminus (r, c)})\\ = &\sum\limits_{\tilde{\mathcal{Y}}_{G \setminus (r, c)}} P(R_r = 0 | \tilde{R}_r = 1) \bigg [\prod\limits_{r'\in R\setminus \{r\}} P(R_{r'} = 0 | \tilde{Y}_{r'1}, \tilde{Y}_{r'2}, \cdots, \tilde{Y}_{r'C}) \bigg ]\\ & \times P(C_c = 0 | \tilde{C}_c = 1) \bigg [\prod\limits_{c'\in C\setminus \{c\}} P(C_{c'} = 0 | \tilde{Y}_{1c'}, \cdots, \tilde{Y}_{Rc'}) \bigg ]\\ & \times \prod\limits_{r' \in R\setminus \{r\}} \prod\limits_{c' \in C\setminus \{c\}} {p_{r'c'B}^{(t)}}^{\tilde{Y}_{r'c'}} (1-{p_{r'c'B}^{(t)}})^{1-{\tilde{Y}_{r'c'}}} p_{rcB}^{(t)}\\ = &\sum\limits_{\tilde{\mathcal{Y}}_{G \setminus (r, c)}} (1-S_e)^2 \prod\limits_{r'\in R \setminus \{r\}} (1-S_e)^{\tilde{R}_{r'}} S_p^{1-\tilde{R}_{r'}} \prod\limits_{c'\in C \setminus \{c\}} (1-S_e)^{\tilde{C}_{c'}} S_p^{1-\tilde{C}_{c'}}\\ & \times \prod\limits_{(r', c') \in G} {p_{r'c'B}^{(t)}}^{\tilde{Y}_{r'c'}} (1-{p_{r'c'B}^{(t)}})^{1-{\tilde{Y}_{r'c'}}} p_{rcB}^{(t)}\\ = &\sum\limits_{\tilde{\mathcal{Y}}_{G \setminus (r, c)}} \prod\limits_{r' \in R\setminus \{r\}} \prod\limits_{c' \in C\setminus \{c\}} (1-S_e)^{2+\tilde{R}_i+\tilde{C}_c} S_p^{2-\tilde{R}_i-\tilde{C}_c}\\ & \times \prod\limits_{(r', c') \in G\setminus(r, c)} {p_{r'c'B}^{(t)}}^{\tilde{Y}_{r'c'}} (1-{p_{r'c'B}^{(t)}})^{1-{\tilde{Y}_{r'c'}}} p_{rcB}^{(t)}. \end{align*} |
2) When \mathcal{Z}_G \neq (0, 0) , \mathcal{Z}_G = (R, C) has multiple scenarios, specifically \mathcal{Z}_G = (R, C) = (1, 0) , (R, C) = (0, 1) , and (R, C) = (1, 1) . Therefore, when \mathcal{Z}_G \neq (0, 0) , the following classifications can be discussed:
(a) When (R, C) = (1, 0) ,
\begin{equation*} {w}^{(t)}_{rc} = P\big(\tilde{Y}_{rc} = 1 \mid \mathcal{Z}_G = (1, 0)\big) = \frac{P\big(\tilde{Y}_{rc} = 1, \mathcal{Z}_G = (1, 0)\big)}{P\big(\mathcal{Z}_G = (1, 0)\big)}. \end{equation*} |
The denominator is
\begin{align*} P\big(\mathcal{Z}_G = (1, 0)\big) = & \sum\limits_{\tilde{\mathcal{Y}}_G} P(\mathcal{R} \neq 0, \mathcal{C} = 0, \mathcal{Y}_Q | \tilde{\mathcal{Y}}_G) P(\tilde{\mathcal{Y}}_G) \\ = & \sum\limits_{\tilde{\mathcal{Y}}_G} \bigg[ \prod\limits_{r' = 1}^{R} P\Big(R_{r'} | \tilde{Y}_{r'1}, \tilde{Y}_{r'2}, \dots, \tilde{Y}_{r'C}\Big) \bigg] \bigg[ \prod\limits_{c' = 1}^{C} P\Big(C_{c'} = 0 | \tilde{Y}_{1c'}, \tilde{Y}_{2c'}, \dots, \tilde{Y}_{Rc'}\Big) \bigg] \\ &\times \bigg[ \prod\limits_{(s, t) \in \mathcal{Q}} P\Big(Y_{st} | \tilde{Y}_{st}\Big) \bigg] \bigg[ \prod\limits_{r' \in R} \prod\limits_{c' \in C} {p_{r'c'B}^{(t)}}^{\tilde{Y}_{r'c'}} \Big(1 - {p_{r'c'B}^{(t)}}\Big)^{1 - \tilde{Y}_{r'c'}} \bigg] \\ = & \sum\limits_{\tilde{\mathcal{Y}}_G} \prod\limits_{r' = 1}^{R} \bigg[ S_e^{R_{r'}} (1 - S_e)^{1 - R_{r'}} \bigg]^{\tilde{R}_{r'c}} \bigg[ (1 - S_p)^{R_{r'}} S_p^{1 - R_{r'}} \bigg]^{1 - \tilde{R}_{r'}} \\ & \times \prod\limits_{c' = 1}^{C} \bigg[ (1 - S_e)^{1 - C_{c'}} \bigg]^{\tilde{C}_{c'}} \bigg[ S_p^{1 - C_{c'}} \bigg]^{1 - \tilde{C}_{c'}} \times \prod\limits_{(s, t) \in Q} \bigg[ S_e^{Y_{st}} (1 - S_e)^{1 - Y_{st}} \bigg]^{\tilde{Y}_{st}} \bigg[ (1 - S_p)^{Y_{st}} S_p^{1 - Y_{st}} \bigg]^{1 - \tilde{Y}_{st}} \\ &\times \prod\limits_{(r', c') \in G} {p_{r'c'B}^{(t)}}^{\tilde{Y}_{r'c'}} \Big(1 - {p_{r'c'B}^{(t)}}\Big)^{1 - \tilde{Y}_{r'c'}}. \end{align*} |
At this point, the numerator requires further discussion:
(i) If (r, c) \in Q and R_r = 1 and C_r = 0 , then
\begin{align*} &P\big(\tilde{Y}_{rc} = 1, \mathcal{Z}_G = (1, 0)\big)\\ = &\sum\limits_{\tilde{\mathcal{Y}}_{G \setminus (r, c)}}P\big(\mathcal{Z}_G = \big(1, 0\big), \mathcal{Y}_Q\big|\tilde{Y}_{rc} = 1, \tilde{\mathcal{Y}}_{G \setminus (r, c)}\big)P\big(\tilde{Y}_{rc} = 1, \tilde{\mathcal{Y}}_{G \setminus (r, c)}\big) \\ = &\sum\limits_{\tilde{\mathcal{Y}}_{G \setminus (r, c)}}P\big(\mathcal{R}\neq0\big|\tilde{Y}_{rc} = 1, \tilde{\mathcal{Y}}_{G \setminus (r, c)}\big)P\big(\mathcal{C} = 0\big|\tilde{Y}_{rc} = 1, \tilde{\mathcal{Y}}_{G \setminus (r, c)}\big) \\ & \times P\big(\mathcal{Y}_Q\big|\tilde{Y}_{rc} = 1, \tilde{\mathcal{Y}}_{Q \setminus (r, c)}\big)P\big(\tilde{Y}_{rc} = 1\big)P\big(\tilde{\mathcal{Y}}_{G \setminus (r, c)}\big) \\ = &\sum\limits_{\tilde{\mathcal{Y}}_{G \setminus (r, c)}}P\Big(R_r = 1|\tilde{R}_r = 1\Big) \bigg[\prod\limits_{r'\in R\setminus \{r\}} P\Big(R_{r'}|\tilde{Y}_{r'1}, \tilde{Y}_{r'2}, \cdots, \tilde{Y}_{r'C}\Big)\bigg] \\ & \times P\Big(C_c = 0|\tilde{C}_c = 1\Big)\bigg[\prod\limits_{c'\in C\setminus \{c\}}P\Big(C_{c'} = 0|\tilde{Y}_{1c'}, \cdots, \tilde{Y}_{Rc'}\Big)\bigg]P\Big(Y_{rc}|\tilde{Y}_{rc} = 1\Big) \\ & \times \bigg[\prod\limits_{(s, t)\in Q\setminus {(r, c)}}P\Big(Y_{st}|\tilde{Y}_{st}\Big)\bigg] p_{rcB}^{(t)} \times \bigg[\prod\limits_{r'\in R\setminus \{r\}}\prod\limits_{c'\in C\setminus \{c\}} {p_{r'c'B}^{(t)}}^{\tilde{Y}_{r'c'}} \Big(1-{p_{r'c'B}^{(t)}}\Big)^{1-{\tilde{Y}_{r'c'}}}\bigg] \\ = &\sum\limits_{\tilde{\mathcal{Y}}_{G \setminus (r, c)}}S_e^{1+Y_{rc}}\Big(1-S_e\Big)^{2-Y_{rc}}p_{rcB}^{(t)}\\ & \times \prod\limits_{r' \in R\setminus \{r\}}\bigg[S_e^{R_{r'}}\Big(1-S_e\Big)^{1-{R_{r'}}}\bigg]^{\tilde{R}_{r'c}} \Big[(1-S_p)^{R_{r'}}S_p^{1-R_{r'}}\Big]^{1-\tilde{R}_{r'}} \\ & \times \prod\limits_{c' \in C\setminus \{c\}}\bigg[(1-S_e)^{1-{C_{c'}}}\bigg]^{\tilde{C}_{c'}} \Big[S_p^{1-C_{c'}}\Big]^{1-\tilde{C}_{c'}} \\ & \times \prod\limits_{(s, t)\in Q\setminus\{(r, c)\}} \bigg[S_e^{Y_{st}}\Big(1-S_e\Big)^{1-Y_{st}}\bigg]^{\tilde{Y}_{st}} \bigg[(1-S_p)^{Y_{st}}S_p^{1-Y_{st}}\bigg]^{1-\tilde{Y}_{st}} \\ & \times \prod\limits_{(r', c')\in G\setminus \{(r, c)\}} {p_{r'c'B}^{(t)}}^{\tilde{Y}_{r'c'}} \Big(1-{p_{r'c'B}^{(t)}}\Big)^{1-{\tilde{Y}_{r'c'}}} . \end{align*} |
(ii) If (r, c) \notin Q , then \mathcal{R} = 0 , \mathcal{C} \neq 0 , but R_r = 0 and C_r = 0 :
\begin{align*} &P\big(\tilde{Y}_{rc} = 1, \mathcal{Z}_G = (1, 0)\big)\\ = &\sum\limits_{\tilde{\mathcal{Y}}_{G \setminus (r, c)}}P\bigg(\mathcal{R}, \mathcal{C}, \mathcal{Y}_Q|\tilde{Y}_{rc} = 1, \tilde{\mathcal{Y}}_{G \setminus (r, c)}\bigg)P\big(\tilde{Y}_{rc} = 1, \tilde{\mathcal{Y}}_{G \setminus (r, c)}\big)\\ = &\sum\limits_{\tilde{\mathcal{Y}}_{G \setminus (r, c)}}P\bigg(\mathcal{R}|\tilde{Y}_{rc} = 1, \tilde{\mathcal{Y}}_{G \setminus (r, c)}\bigg) P\bigg(\mathcal{C}|\tilde{Y}_{rc} = 1, \tilde{\mathcal{Y}}_{G \setminus (r, c)}\bigg) P\big(\mathcal{Y}_Q\big|\tilde{\mathcal{Y}}_Q\big)P\big(\tilde{Y}_{rc} = 1\big)P\big(\tilde{\mathcal{Y}}_{G \setminus (r, c)}\big)\\ = &\sum\limits_{\tilde{\mathcal{Y}}_{G \setminus (r, c)}}P\Big(R_r = 0|\tilde{R}_r = 1\Big) \bigg[\prod\limits_{r'\in R\setminus \{r\}} P\Big(R_{r'}|\tilde{Y}_{r'1}, \tilde{Y}_{r'2}, \cdots, \tilde{Y}_{r'C}\Big)\bigg]\\ & \times P\Big(C_c = 0|\tilde{C}_c = 1\Big) \bigg[\prod\limits_{c'\in C\setminus \{c\}}P\Big(C_{c'} = 0|\tilde{Y}_{1c'}, \cdots, \tilde{Y}_{Rc'}\Big)\bigg]\\ & \times \bigg[\prod\limits_{(s, t)\in Q}P\Big(Y_{st}|\tilde{Y}_{st}\Big)\bigg] p_{rcB}^{(t)} \prod\limits_{(r', c')\in G\setminus \{(r, c)\}} {p_{r'c'B}^{(t)}}^{\tilde{Y}_{r'c'}} \Big(1-{p_{r'c'B}^{(t)}}\Big)^{1-{\tilde{Y}_{r'c'}}}\\ = &\sum\limits_{\tilde{\mathcal{Y}}_{G \setminus (r, c)}}S_e^{Y_{rc}}\Big(1-S_e\Big)^{3-Y_{rc}}p_{rcB}^{(t)}\\ & \times \prod\limits_{r' \in R\setminus \{r\}} \bigg[S_e^{R_{r'}}\big(1-S_e\big)^{1-{R_{r'}}}\bigg]^{\tilde{R}_{r'c}} \Big[(1-S_p)^{R_{r'}}S_p^{1-R_{r'}}\Big]^{1-\tilde{R}_{r'c}}\\ & \times \prod\limits_{c' \in C\setminus \{c\}} \bigg[(1-S_e)^{1-{C_{c'}}}\bigg]^{\tilde{C}_{c'}} \Big[S_p^{1-C_{c'}}\Big]^{1-\tilde{C}_{c'}}\\ & \times \prod\limits_{(s, t)\in Q} \bigg[S_e^{Y_{st}}\big(1-S_e\big)^{1-Y_{st}}\bigg]^{\tilde{Y}_{st}} \Big[(1-S_p)^{Y_{st}}S_p^{1-Y_{st}}\Big]^{1-\tilde{Y}_{st}}\\ & \times \prod\limits_{(r', c')\in G\setminus \{(r, c)\}} \bigg[{p_{r'c'B}^{(t)}}^{\tilde{Y}_{r'c'}} \Big(1-{p_{r'c'B}^{(t)}}\Big)^{1-{\tilde{Y}_{r'c'}}}\bigg]. \end{align*} |
(b) When (R, C) = (0, 1) , the denominator is
\begin{align*} P\big(\mathcal{Z}_G = (0, 1)\big) = &\sum\limits_{\tilde{\mathcal{Y}}_G}P(\mathcal{R} = 0, \mathcal{C}\neq0, \mathcal{Y}_Q|\tilde{\mathcal{Y}}_G)P\big(\tilde{\mathcal{Y}}_G\big)\\ = &\sum\limits_{\tilde{\mathcal{Y}}_G} \bigg[ \prod\limits_{r' = 1}^{R} P(R_r' = 0|\tilde{Y}_{r'1}, \tilde{Y}_{r'2}, \cdots, \tilde{Y}_{r'C}) \bigg] \\ & \times \bigg[ \prod\limits_{c' = 1}^{C} P(C_{c'}|\tilde{Y}_{1c'}, \tilde{Y}_{2c'}, \cdots, \tilde{Y}_{Rc'}) \bigg] \\ & \times \prod\limits_{(s, t)\in \mathcal{Q}} P\big(Y_{st}\big|\tilde{Y}_{st}\big) \prod\limits_{r' \in R}\prod\limits_{c' \in C} {p_{r'c'B}^{(t)}}^{\tilde{Y}_{r'c'}} (1-{p_{r'c'B}^{(t)}})^{1-{\tilde{Y}_{r'c'}}} \\ = &\sum\limits_{\tilde{\mathcal{Y}}_G} \prod\limits_{r' = 1}^{R} \bigg[ \big(1-S_e\big)^{1-R_{r'}} \bigg]^{\tilde{R}_{r'}} \Big[ S_p^{1-R_{r'}} \Big]^{1-\tilde{R}_{r'}} \\ & \times \prod\limits_{c' = 1}^{C} \bigg[ S_e^{C_{c'}}\big(1-S_e\big)^{1-C_{c'}} \bigg]^{\tilde{C}_{c'}} \Big[ (1-S_p)^{C_{c'}}S_p^{1-C_{c'}} \Big]^{1-\tilde{C}_{c'}} \\ & \times \prod\limits_{(s, t)\in Q} \bigg[ S_e^{Y_{st}}\big(1-S_e\big)^{1-Y_{st}} \bigg]^{\tilde{Y}_{st}} \Big[ (1-S_p)^{Y_{st}}S_p^{1-Y_{st}} \Big]^{1-\tilde{Y}_{st}} \\ & \times \prod\limits_{(r', c') \in G} \bigg[ {p_{r'c'B}^{(t)}}^{\tilde{Y}_{r'c'}} (1-{p_{r'c'B}^{(t)}})^{1-{\tilde{Y}_{r'c'}}} \bigg]. \end{align*} |
The numerator requires further discussion:
(ⅰ) If (r, c) \in Q and R_r = 0 and C_c = 1 , then
\begin{align*} &P\big(\tilde{Y}_{rc} = 1, \mathcal{Z}_G = (0, 1)\big) = P(\tilde{Y}_{rc} = 1, \mathcal{R}, \mathcal{C}, \tilde{\mathcal{Y}}_Q)\\ = &\sum\limits_{\tilde{\mathcal{Y}}_{G \setminus (r, c)}}P(\mathcal{R}, \mathcal{C}, \mathcal{Y}_Q|\tilde{Y}_{rc} = 1, \tilde{\mathcal{Y}}_{G \setminus (r, c)})P\big(\tilde{Y}_{rc} = 1, \tilde{\mathcal{Y}}_{G \setminus (r, c)}\big)\\ = &\sum\limits_{\tilde{\mathcal{Y}}_{G \setminus (r, c)}}S_e^{Y_{rc}}(3-S_e)^{1-Y_{rc}}p_{rcB}^{(t)} \prod\limits_{r' \in R\setminus \{r\}} \bigg[\big(1-S_e\big)^{1-{R_{r'}}}\bigg]^{\tilde{R}_{r'c}} \Big[S_p^{1-R_{r'}}\Big]^{1-\tilde{R}_{r'c}}\\ & \times \prod\limits_{c' \in C\setminus \{c\}} \bigg[S_e^{C_{c'}}\big(1-S_e\big)^{1-{C_{c'}}}\bigg]^{\tilde{C}_{c'}} \Big[(1-S_p)^{C_{c'}}S_p^{1-C_{c'}}\Big]^{1-\tilde{C}_{c'}}\\ & \times \prod\limits_{(s, t)\in Q} \bigg[S_e^{Y_{st}}\big(1-S_e\big)^{1-Y_{st}}\bigg]^{\tilde{Y}_{st}} \Big[(1-S_p)^{Y_{st}}S_p^{1-Y_{st}}\Big]^{1-\tilde{Y}_{st}}\\ & \times \prod\limits_{(r', c')\in G\setminus \{(r, c)\}} \bigg[{p_{r'c'B}^{(t)}}^{\tilde{Y}_{r'c'}} (1-{p_{r'c'B}^{(t)}})^{1-{\tilde{Y}_{r'c'}}}\bigg]. \end{align*} |
(ⅱ) If (r, c) \notin Q , then \mathcal{R} = 0 , \mathcal{C} \neq 0 , but R_r = 0 and C_r = 0 :
\begin{align*} P\big(\tilde{Y}_{rc} = 1, \mathcal{Z}_G = (0, 1)\big) = &P(\tilde{Y}_{rc} = 1, \mathcal{R}, \mathcal{C}, \mathcal{Y}_Q)\\ = &\sum\limits_{\tilde{\mathcal{Y}}_{G \setminus (r, c)}}P(\mathcal{R}, \mathcal{C}, \mathcal{Y}_Q|\tilde{Y}_{rc} = 1, \tilde{\mathcal{Y}}_{G \setminus (r, c)})P(\tilde{Y}_{rc} = 1, \tilde{\mathcal{Y}}_{G \setminus (r, c)})\\ = &\sum\limits_{\tilde{\mathcal{Y}}_{G \setminus (r, c)}}S_e^{Y_{rc}}(3-S_e)^{1-Y_{rc}}p_{rcB}^{(t)} \prod\limits_{r' \in R\setminus \big\{r\big\}} \bigg[ \big(1-S_e\big)^{1-{R_{r'}}} \bigg]^{\tilde{R}_{r'c}} \bigg[ S_p^{1-R_{r'}} \bigg]^{1-\tilde{R}_{r'c}}\\ & \times \prod\limits_{c' \in C\setminus \big\{c\big\}} \bigg[ S_e^{C_{c'}}\big(1-S_e\big)^{1-{C_{c'}}} \bigg]^{\tilde{C}_{c'}} \bigg[ \big(1-S_p\big)^{C_{c'}}S_p^{1-C_{c'}} \bigg]^{1-\tilde{C}_{c'}}\\ & \times \prod\limits_{(s, t)\in Q} \bigg[ S_e^{Y_{st}}\big(1-S_e\big)^{1-Y_{st}} \bigg]^{\tilde{Y}_{st}} \bigg[ \big(1-S_p\big)^{Y_{st}}S_p^{1-Y_{st}} \bigg]^{1-\tilde{Y}_{st}}\\ & \times \prod\limits_{(r', c')\in G\setminus \big\{(r, c)\big\}} ( {p_{r'c'B}^{(t)}}^{\tilde{Y}_{r'c'}} \big(1-{p_{r'c'B}^{(t)}}\big)^{1-{\tilde{Y}_{r'c'}}} ). \end{align*} |
(c) When (R, C) = (1, 1) , the denominator is
\begin{align*} P\big(\mathcal{Z}_G = (1, 1)\big) = &\sum\limits_{\tilde{\mathcal{Y}}}P(\mathcal{R}, \mathcal{C}, \mathcal{Y}_Q | \tilde{\mathcal{Y}})P(\tilde{\mathcal{Y}})\\ = &\sum\limits_{\tilde{\mathcal{Y}}} \bigg[\prod\limits_{r' = 1}^{R} P(R_r' | \tilde{Y}_{r'1}, \tilde{Y}_{r'2}, \cdots, \tilde{Y}_{r'C} ) \bigg] \bigg[\prod\limits_{c' = 1}^{C} P(C_{c'} | \tilde{Y}_{1c'}, \tilde{Y}_{2c'}, \cdots, \tilde{Y}_{Rc'} ) \bigg] \\ & \times \prod\limits_{(s, t)\in \mathcal{Q}} P(Y_{st} | \tilde{Y}_{st} ) \prod\limits_{r' \in R} \prod\limits_{c' \in C} {p_{r'c'B}^{(t)}}^{\tilde{Y}_{r'c'}} (1-{p_{r'c'B}^{(t)}})^{1-{\tilde{Y}_{r'c'}}}\\ = &\sum\limits_{\tilde{\mathcal{Y}}} \prod\limits_{r' = 1}^{R} \bigg[ S_e^{R_{r'}}(1-S_e)^{1-{R_{r'}}} \bigg]^{\tilde{R}_{r'c}} \bigg[ (1-S_p)^{R_{r'}}S_p^{1-R_{r'}} \bigg]^{1-\tilde{R}_{r'}}\\ & \times \prod\limits_{c' = 1}^{C} \bigg[ S_e^{C_{c'}}(1-S_e)^{1-C_{c'}} \bigg]^{\tilde{C}_{c'}} \bigg[ (1-S_p)^{C_{c'}}S_p^{1-C_{c'}} \bigg]^{1-\tilde{C}_{c'}}\\ & \times \prod\limits_{(s, t)\in Q} \bigg[ S_e^{Y_{st}}(1-S_e)^{1-Y_{st}} \bigg]^{\tilde{Y}_{st}} \bigg[ (1-S_p)^{Y_{st}}S_p^{1-Y_{st}} \bigg]^{1-\tilde{Y}_{st}}\\ & \times \prod\limits_{(r', c') \in G} \bigg\{ {p_{r'c'B}^{(t)}}^{\tilde{Y}_{r'c'}} (1-{p_{r'c'B}^{(t)}})^{1-{\tilde{Y}_{r'c'}}} \bigg\}. \end{align*} |
For the numerator, we provide the following derivations:
(ⅰ) If (r, c) \in Q and R_r = 1 and C_c = 1 , then
\begin{align*} &P\big(\tilde{Y}_{rc} = 1, \mathcal{Z}_G = (1, 1)\big) = P(\tilde{Y}_{rc} = 1, \mathcal{R}, \mathcal{C}, \mathcal{Y}_Q)\\ = &\sum\limits_{\tilde{\mathcal{Y}}_{G \setminus (r, c)}}P(\mathcal{R}, \mathcal{C}, \mathcal{Y}_Q | \tilde{Y}_{rc} = 1, \tilde{\mathcal{Y}}_{G \setminus (r, c)})P(\tilde{Y}_{rc} = 1, \tilde{\mathcal{Y}}_{G \setminus (r, c)})\\ = &\sum\limits_{\tilde{\mathcal{Y}}_{G \setminus (r, c)}}S_e^{2+Y_{rc}}(1-S_e)^{1-Y_{rc}} \cdot p_{rcB}^{(t)}\\ & \times \prod\limits_{r' \in R\setminus \{r\}}\bigg[ S_e^{R_{r'}}(1-S_e)^{1-{R_{r'}}} \bigg]^{\tilde{R}_{r'c}} \bigg[ (1-S_p)^{R_{r'}}S_p^{1-R_{r'}} \bigg]^{1-\tilde{R}_{r'c}}\\ & \times \prod\limits_{c' \in C\setminus \{c\}}\bigg[ S_e^{C_{c'}}(1-S_e)^{1-{C_{c'}}} \bigg]^{\tilde{C}_{c'}} \bigg[ (1-S_p)^{C_{c'}}S_p^{1-C_{c'}} \bigg]^{1-\tilde{C}_{c'}}\\ & \times \prod\limits_{(s, t)\in Q\setminus \{(r, c)\}} \bigg[ S_e^{Y_{st}}(1-S_e)^{1-Y_{st}} \bigg]^{\tilde{Y}_{st}} \bigg[ (1-S_p)^{Y_{st}}S_p^{1-Y_{st}} \bigg]^{1-\tilde{Y}_{st}}\\ & \times \prod\limits_{(r', c')\in G\setminus \{(r, c)\}} \bigg\{ {p_{r'c'B}^{(t)}}^{\tilde{Y}_{r'c'}} ( 1-{p_{r'c'B}^{(t)}} )^{1-{\tilde{Y}_{r'c'}}} \bigg\}. \end{align*} |
(ⅱ) If (r, c) \notin Q , then \mathcal{R} \neq 0 , \mathcal{C} \neq 0 , but R_r = 0 and C_r = 0 :
\begin{align*} &P\big(\tilde{Y}_{rc} = 1, \mathcal{Z}_G = (1, 1)\big) = P(\tilde{Y}_{rc} = 1, \mathcal{R}, \mathcal{C}, \mathcal{Y}_Q)\\ = &\sum\limits_{\tilde{\mathcal{Y}}_{G \setminus (r, c)}}P(\mathcal{R}, \mathcal{C}, \mathcal{Y}_Q|\tilde{Y}_{rc} = 1, \tilde{\mathcal{Y}}_{G \setminus (r, c)})P(\tilde{Y}_{rc} = 1, \tilde{\mathcal{Y}}_{G \setminus (r, c)})\\ = &\sum\limits_{\tilde{\mathcal{Y}}_{G \setminus (r, c)}}(1-S_e)^2S_e^{Y_{rc}}(1-S_e)^{1-Y_{rc}}p_{rcB}^{(t)}\\ & \times \prod\limits_{r' \in R\setminus \{r\}}\bigg [S_e^{R_{r'}}(1-S_e)^{1-{R_{r'}}}\bigg ]^{\tilde{R}_{r'c}} \bigg [(1-S_p)^{R_{r'}}S_p^{1-R_{r'}}\bigg ]^{1-\tilde{R}_{r'c}}\\ & \times \prod\limits_{c' \in C\setminus \{c\}}\bigg [S_e^{C_{c'}}(1-S_e)^{1-{C_{c'}}}\bigg ]^{\tilde{C}_{c'}} \bigg [(1-S_p)^{C_{c'}}S_p^{1-C_{c'}}\bigg ]^{1-\tilde{C}_{c'}}\\ & \times \prod\limits_{(s, t)\in Q} \bigg [S_e^{Y_{st}}(1-S_e)^{1-Y_{st}}\bigg ]^{\tilde{Y}_{st}}\bigg [(1-S_p)^{Y_{st}}S_p^{1-Y_{st}}\bigg ]^{1-\tilde{Y}_{st}}\\ & \times \prod\limits_{(r', c')\in G\setminus \{(r, c)\}} \bigg\{ {p_{r'c'B}^{(t)}}^{\tilde{Y}_{r'c'}} (1-{p_{r'c'B}^{(t)}})^{1-{\tilde{Y}_{r'c'}}} \bigg\}. \end{align*} |
[1] | A. Wazwaz, Partial differential equations and solitary waves theorem, Berlin: Springer, 2009. https://doi.org/10.1007/978-3-642-00251-9 |
[2] |
R. Grimshaw, The solitary wave in water of variable depth, J. Fluid Mech., 42 (1970), 639–656. https://doi.org/10.1017/S0022112070001520 doi: 10.1017/S0022112070001520
![]() |
[3] | D. Baleanu, A. Machado, A. Luo, Fractional dynamics and control, New York: Springer, 2012. https://doi.org/10.1007/978-1-4614-0457-6 |
[4] | B. Boudjehem, D. Boudjehem, Parameter tuning of a fractional-order PI controller using the ITAE criteria, In: Fractional dynamics control, New York: Springer, 2012, 49–57. https://doi.org/10.1007/978-1-4614-0457-6_4 |
[5] | H. Alotaibi, Developing multiscale methodologies for computational fluid mechanics, Ph. D Thesis, University of Adelaide, 2017. https://doi.org/10.25909/5ba30242307d5 |
[6] |
A. Zhou, X. Li, Zakharov equations for viscous flow and their use in the blood clot formation, Pramana, 89 (2017), 82. https://doi.org/10.1007/s12043-017-1478-9 doi: 10.1007/s12043-017-1478-9
![]() |
[7] |
A. Wazwaz, New solitary wave solutions to the modified forms of Degasperis-Procesi and Camassa-Holm equations, Appl. Math. Comput., 186 (2007), 130–141. https://doi.org/10.1016/j.amc.2006.07.092 doi: 10.1016/j.amc.2006.07.092
![]() |
[8] | K. Khan, M. Akbar, Application of (\exp (-\phi (\xi)))-expansion method to find the exact solutions of modified Benjamin-Bona-Mahony equation, World Appl. Sci. J., 10 (2013), 1373–1377. |
[9] |
L. Wu, S. Chen, C. Pang, Traveling wave solution for generalized Drinfeld-Sokolov equations, Appl. Math. Model., 33 (2009), 4126–4130. https://doi.org/10.1016/j.apm.2009.02.013 doi: 10.1016/j.apm.2009.02.013
![]() |
[10] |
F. Zhang, J. Qi, W. Yuan, Further results about traveling wave exact solutions of the Drinfeld-Sokolov equations, J. Appl. Math., 2013 (2013), 523732. https://doi.org/10.1155/2013/523732 doi: 10.1155/2013/523732
![]() |
[11] |
M. Abdou, The extended tanh-method and its applications for solving nonlinear physical models, Appl. Math. Comput., 190 (2007), 988–996. https://doi.org/10.1016/j.amc.2007.01.070 doi: 10.1016/j.amc.2007.01.070
![]() |
[12] |
A. Wazwaz, A sine-cosine method for handling nonlinear wave equations, Math. Comput. Model., 40 (2004), 499–508. https://doi.org/10.1016/j.mcm.2003.12.010 doi: 10.1016/j.mcm.2003.12.010
![]() |
[13] |
Sirendaoreji, New exact traveling wave solutions for the Kawahara and modified Kawahara equations, Chaos Soliton. Fract., 19 (2004), 147–150. https://doi.org/10.1016/S0960-0779(03)00102-4 doi: 10.1016/S0960-0779(03)00102-4
![]() |
[14] |
M. Abd-el-Malek, A. Amin, New exact solutions for solving the initial-value-problem of the KdV-KP equation via the Lie group method, Appl. Math. Comput., 261 (2015), 408–418. https://doi.org/10.1016/j.amc.2015.03.117 doi: 10.1016/j.amc.2015.03.117
![]() |
[15] | M. Akbar, N. Ali, New solitary and periodic solutions of nonlinear evolution equation by exp-function method, World Appl. Sci. J., 17 (2012), 1603–1610. |
[16] |
J. He, X. Wu, Exp-function method for nonlinear wave equations, Chaos Soliton. Fract., 30 (2006), 700–708. https://doi.org/10.1016/j.chaos.2006.03.020 doi: 10.1016/j.chaos.2006.03.020
![]() |
[17] |
S. Abbasbandy, Numerical solutions of nonlinear Klein-Gordon equation by variational iteration method, Int. J. Numer. Meth. Eng., 70 (2007), 876–881. https://doi.org/10.1002/nme.1924 doi: 10.1002/nme.1924
![]() |
[18] |
M. Kaplan, A. Bekir, A. Akbulut, A generalized Kudryashov method to some nonlinear evolution equations in mathematical physics, Nonlinear Dyn., 85 (2016), 2843–2850. https://doi.org/10.1007/s11071-016-2867-1 doi: 10.1007/s11071-016-2867-1
![]() |
[19] |
H. Alotaibi, Traveling wave solutions to the nonlinear evolution equation using expansion method and Addendum to Kudryashov's method, Symmetry, 13 (2021), 2126. https://doi.org/10.3390/sym13112126 doi: 10.3390/sym13112126
![]() |
[20] |
H. Alotaibi, Explore optical solitary wave solutions of the KP equation by recent approaches, Crystals, 12 (2022), 159. https://doi.org/10.3390/cryst12020159 doi: 10.3390/cryst12020159
![]() |
[21] |
K. Gepreel, Exact soliton solutions for nonlinear perturbed Schrödinger equations with nonlinear optical media, Appl. Sci., 10 (2020), 8929. https://doi.org/10.3390/app10248929 doi: 10.3390/app10248929
![]() |
[22] |
B. Zhong, J. Jiang, Y. Feng, New exact solutions of fractional Boussinesq-like equations, Commun. Optim. Theory, 2020 (2020), 21. https://doi.org/10.23952/cot.2020.21 doi: 10.23952/cot.2020.21
![]() |
[23] |
E. Zayed, K. Gepreel, M. El-Horbaty, A. Biswas, Y. Yıldırım, H. Alshehri, Highly dispersive optical solitons with complex Ginzburg-Landau equation having six nonlinear forms, Mathematics, 9 (2021), 3270. https://doi.org/10.3390/math9243270 doi: 10.3390/math9243270
![]() |
[24] |
J. Xu, E. Fan, Y. Chen, Long-time asymptotic for the derivative nonlinear Schrödinger equation with step-like initial value, Math. Phys. Anal. Geom., 16 (2013), 253–288. https://doi.org/10.1007/s11040-013-9132-3 doi: 10.1007/s11040-013-9132-3
![]() |
[25] |
L. Xu, D. Wang, X. Wen, Y. Jiang, Exotic localized vector waves in a two-component nonlinear wave system, J. Nonlinear Sci., 30 (2020), 537–564. https://doi.org/10.1007/s00332-019-09581-0 doi: 10.1007/s00332-019-09581-0
![]() |
[26] |
C. Charlier, J. Lenells, D. Wang, The "good" Boussinesq equation: long-time asymptotics, Anal. PDE, 16 (2023), 1351–1388. https://doi.org/10.2140/apde.2023.16.1351 doi: 10.2140/apde.2023.16.1351
![]() |
[27] |
D. Bilman, R. Buckingham, D. Wang, Far-field asymptotics for multiple-pole solitons in the large-order limit, J. Differ. Equations, 297 (2021), 320–369. https://doi.org/10.1016/j.jde.2021.06.016 doi: 10.1016/j.jde.2021.06.016
![]() |
[28] | V. Zakharov, Collapse of Langmuir waves, Sov. Phys. JETP, 35 (1972), 908–914. |
[29] |
M. Goldman, Strong turbulence of plasma waves, Rev. Mod. Phys., 56 (1984), 709. https://doi.org/10.1103/RevModPhys.56.709 doi: 10.1103/RevModPhys.56.709
![]() |
[30] |
Y. Shang, Y. Huang, W. Yuan, The extended hyperbolic functions method and new exact solutions to the Zakharov equations, Appl. Math. Comput., 200 (2008), 110–122. https://doi.org/10.1016/j.amc.2007.10.059 doi: 10.1016/j.amc.2007.10.059
![]() |
[31] |
D. Huang, H. Zhang, Extended hyperbolic function method and new exact solitary wave solutions of Zakharov equations (Chinese), Acta Phys. Sin., 53 (2004), 2434–2438. https://doi.org/10.7498/aps.53.2434 doi: 10.7498/aps.53.2434
![]() |
[32] | S. Liu, Z. Fu, S. Liu, Q. Zhao, The envelope periodic solutions to nonlinear wave equations with Jacobi elliptic function (Chinese), Acta Phys. Sin., 51 (2002), 718–722. |
[33] |
G. Wu, M. Zhang, L. Shi, W. Zhang, J. Han, An extended expansion method for Jacobi elliptic functions and new exact periodic solutions of Zakharov equations (Chinese), Acta Phys. Sin., 56 (2007), 5054–5059. https://doi.org/10.7498/aps.56.5054 doi: 10.7498/aps.56.5054
![]() |
[34] |
C. Zhao, Z. Sheng, Explicit traveling wave solutions for Zakharov equations (Chinese), Acta Phys. Sin., 53 (2004), 1629–1634. https://doi.org/10.7498/aps.53.1629 doi: 10.7498/aps.53.1629
![]() |
[35] |
E. Zayed, M. Abdelaziz, Exact solutions for the generalized Zakharov-Kuznetsov equation with variable coefficients using the generalized (G' / G)-expansion method, AIP Conf. Proc., 1281 (2010), 2216–2219. https://doi.org/10.1063/1.3498415 doi: 10.1063/1.3498415
![]() |
[36] |
Y. Yıldırım, A. Biswas, M. Ekici, O. Gonzalez-Gaxiola, S. Khan, H. Triki, et al., Optical solitons with Kudryashov's model by a range of integration norms, Chinese J. Phys., 66 (2020), 660–672. https://doi.org/10.1016/j.cjph.2020.06.005 doi: 10.1016/j.cjph.2020.06.005
![]() |
[37] |
Y. Gurefe, E. Misirli, Y. Pandir, A. Sonmezoglu, M. Ekici, New exact solutions of the Davey-Stewartson equation with power-law nonlinearity, Bull. Malays. Math. Sci. Soc., 38 (2015), 1223–1234. https://doi.org/10.1007/s40840-014-0075-z doi: 10.1007/s40840-014-0075-z
![]() |
[38] |
H. Zhang, New exact traveling wave solutions of the generalized Zakharov equations, Rep. Math. Phys., 60 (2007), 97–106. https://doi.org/10.1016/S0034-4877(07)80101-7 doi: 10.1016/S0034-4877(07)80101-7
![]() |
[39] |
J. Pava, C. Brango, Orbital stability for the periodic Zakharov system, Nonlinearity, 24 (2011), 2913. https://doi.org/10.1088/0951-7715/24/10/013 doi: 10.1088/0951-7715/24/10/013
![]() |
[40] |
A. Borhanifar, M. Kabir, L. Maryam Vahdat, New periodic and soliton wave solutions for the generalized Zakharov system and (2+ 1)-dimensional Nizhnik-Novikov-Veselov system, Chaos Soliton. Fract., 42 (2009), 1646–1654. https://doi.org/10.1016/j.chaos.2009.03.064 doi: 10.1016/j.chaos.2009.03.064
![]() |
[41] |
M. Wang, X. Li, Extended F-expansion method and periodic wave solutions for the generalized Zakharov equations, Phys. Lett. A, 343 (2005), 48–54. https://doi.org/10.1016/j.physleta.2005.05.085 doi: 10.1016/j.physleta.2005.05.085
![]() |
[42] |
S. Abbasbandy, E. Babolian, M. Ashtiani, Numerical solution of the generalized Zakharov equation by homotopy analysis method, Commun. Nonlinear Sci., 14 (2009), 4114–4121. https://doi.org/10.1016/j.cnsns.2009.03.001 doi: 10.1016/j.cnsns.2009.03.001
![]() |
[43] |
J. He, Variational principles for some nonlinear partial differential equations with variable coefficients, Chaos Soliton. Fract., 19 (2004), 847–851. https://doi.org/10.1016/S0960-0779(03)00265-0 doi: 10.1016/S0960-0779(03)00265-0
![]() |
[44] |
J. He, Erratum to: variational principle for two-dimensional incompressible inviscid flow, Phys. Lett. A, 372 (2008), 5858–5859. https://doi.org/10.1016/j.physleta.2008.07.043 doi: 10.1016/j.physleta.2008.07.043
![]() |
[45] |
Y. Khan, N. Faraz, A. Yildirim, New soliton solutions of the generalized Zakharov equations using He's variational approach, Appl. Math. Lett., 24 (2011), 965–968. https://doi.org/10.1016/j.aml.2011.01.006 doi: 10.1016/j.aml.2011.01.006
![]() |
[46] |
P. Veeresha, D. Prakasha, Solution for fractional generalized Zakharov equations with Mittag-Leffler function, Results in Engineering, 5 (2020), 100085. https://doi.org/10.1016/j.rineng.2019.100085 doi: 10.1016/j.rineng.2019.100085
![]() |
[47] |
M. Wang, X. Li, J. Zhang, The (G' / G)-expansion method and traveling wave solutions of nonlinear evolution equations in mathematical physics, Phys. Lett. A, 372 (2008), 417–423. https://doi.org/10.1016/j.physleta.2007.07.051 doi: 10.1016/j.physleta.2007.07.051
![]() |
[48] |
W. Li, H. Chen, G. Zhang, The (w/g) -expansion method and its application to Vakhnenko equation, Chinese Phys. B, 18 (2009), 400. https://doi.org/10.1088/1674-1056/18/2/004 doi: 10.1088/1674-1056/18/2/004
![]() |
[49] |
M. Golman, Langmuir wave solitons and spatial collapse in plasma physics, Physica D, 18 (1986), 67–76. https://doi.org/10.1016/0167-2789(86)90163-6 doi: 10.1016/0167-2789(86)90163-6
![]() |
[50] |
E. Zayed, M. Alngar, A. Biswas, A. Kara, M. Ekici, A. Alzahrani, et al., Cubic-quartic optical solitons and conservation laws with Kudryashov's sextic power-law of refractive index, Optik, 227 (2021), 166059. https://doi.org/10.1016/j.ijleo.2020.166059 doi: 10.1016/j.ijleo.2020.166059
![]() |
[51] |
M. Attia, A. Elhanbaly, M. Abdou, New exact solutions for isothermal magne to static atmosphere equations, WJST, 12 (2014), 961–973. https://doi.org/10.14456/WJST.2015.42 doi: 10.14456/WJST.2015.42
![]() |
[52] |
M. Wang, X. Li, Extended F-expansion method and periodic wave solutions for the generalized Zakharov equations, Phys. Lett. A, 343 (2005), 48–54. https://doi.org/10.1016/j.physleta.2005.05.085 doi: 10.1016/j.physleta.2005.05.085
![]() |
[53] |
K. Gepreel, Exact solutions for nonlinear integral member of Kadomtsev-Petviashvili hierarchy differential equations using the modified (w/g)-expansion method, Comput. Math. Appl., 72 (2016), 2072–2083. https://doi.org/10.1016/j.camwa.2016.08.005 doi: 10.1016/j.camwa.2016.08.005
![]() |
[54] |
H. Abdusalam, On an improved complex \tanh-function method, Int. J. Nonlin. Sci. Num., 6 (2005), 99–106. https://doi.org/10.1515/IJNSNS.2005.6.2.99 doi: 10.1515/IJNSNS.2005.6.2.99
![]() |
[55] |
E. Zayed, H. Zedan, K. Gepreel, Group analysis and modified extended Tanh-function to find the invariant solutions and soliton solutions for nonlinear Euler equations, Int. J. Nonlin. Sci. Num., 5 (2004), 221–234. https://doi.org/10.1515/IJNSNS.2004.5.3.221 doi: 10.1515/IJNSNS.2004.5.3.221
![]() |
[56] |
S. Ege, E. Misirli, Extended Kudryashov method for fractional nonlinear differential equations, Mathematical Sciences and Applications E-Notes, 6 (2018), 19–28. https://doi.org/10.36753/mathenot.421751 doi: 10.36753/mathenot.421751
![]() |
[57] |
E. Zayed, R. Shohib, M. Alngar, New extended generalized Kudryashov method for solving three nonlinear partial differential equations, Nonlinear Anal.-Model., 25 (2020), 598–617. https://doi.org/10.15388/namc.2020.25.17203 doi: 10.15388/namc.2020.25.17203
![]() |
Metric | Implication |
True positive (TP) | Actual positive and predicted positive |
False positive (FP) | Actual negative and predicted positive |
False negative (FN) | Actual positive and predicted negative |
True negative (TN) | Actual negative and predicted negative |
AMAE | AMSE | MSE | ||||||||
Model | Setting | Test | Penalty | TPR | FPR | g(\cdot) | Prob | \boldsymbol{\beta} | \beta_1 | \beta_2 |
Example 4.1 (n=500) | q_n =50 | MPT | MCP | 0.980 | 0.061 | 0.325 | 0.011 | 0.0003 | 0.0022 | 0.0015 |
HT | 0.985 | 0.003 | 0.413 | 0.007 | 0.0002 | 0.0068 | 0.0025 | |||
DT | 0.968 | 0.062 | 0.295 | 0.011 | 0.0003 | 0.0001 | 0.0004 | |||
AT | 0.987 | 0.035 | 0.388 | 0.009 | 0.0004 | 0.0019 | 0.0009 | |||
MPT | SCAD | 0.967 | 0.060 | 0.508 | 0.014 | 0.0003 | 0.0012 | 0.0021 | ||
HT | 0.988 | 0.001 | 0.479 | 0.008 | 0.0001 | 0.0021 | 0.0023 | |||
DT | 0.980 | 0.051 | 0.511 | 0.012 | 0.0003 | 0.0005 | 0.0004 | |||
AT | 0.974 | 0.063 | 0.432 | 0.009 | 0.0003 | 0.0035 | 0.0024 | |||
MPT | LASSO | 0.964 | 0.060 | 0.337 | 0.011 | 0.0003 | 0.0038 | 0.0051 | ||
HT | 0.986 | 0.003 | 0.436 | 0.006 | 0.0001 | 0.0003 | 0.0002 | |||
DT | 1.000 | 0.029 | 0.522 | 0.013 | 0.0001 | 0.0004 | 0.0003 | |||
AT | 0.981 | 0.034 | 0.320 | 0.007 | 0.0001 | 0.0006 | 0.0008 | |||
q_n =100 | MPT | MCP | 0.985 | 0.010 | 0.511 | 0.009 | 0.0001 | 0.0004 | 0.0004 | |
HT | 0.973 | 0.038 | 0.374 | 0.009 | 0.0002 | 0.0022 | 0.0033 | |||
DT | 0.986 | 0.023 | 0.338 | 0.010 | 0.0001 | 0.0004 | 0.0001 | |||
AT | 0.982 | 0.023 | 0.470 | 0.005 | 0.0001 | 0.0004 | 0.0005 | |||
MPT | SCAD | 0.987 | 0.031 | 0.265 | 0.013 | 0.0002 | 0.0002 | 0.0003 | ||
HT | 0.988 | 0.038 | 0.458 | 0.015 | 0.0005 | 0.0017 | 0.0001 | |||
DT | 0.978 | 0.051 | 0.451 | 0.011 | 0.0001 | 0.0008 | 0.0004 | |||
AT | 0.985 | 0.010 | 0.422 | 0.009 | 0.0001 | 0.0058 | 0.0047 | |||
MPT | LASSO | 0.987 | 0.026 | 0.478 | 0.010 | 0.0001 | 0.0008 | 0.0012 | ||
HT | 0.966 | 0.044 | 0.364 | 0.011 | 0.0003 | 0.0029 | 0.0052 | |||
DT | 0.984 | 0.031 | 0.503 | 0.012 | 0.0001 | 0.0001 | 0.0003 | |||
AT | 0.987 | 0.031 | 0.401 | 0.008 | 0.0001 | 0.0016 | 0.0014 |
AMAE | AMSE | MSE | |||||||||
Model | Setting | Test | Penalty | TPR | FPR | g(\cdot) | Prob | \boldsymbol{\beta} | \beta_1 | \beta_2 | \beta_3 |
Example 4.2 (n=1000) | q_n =100 | MPT | MCP | 0.980 | 0.001 | 0.569 | 0.011 | 0.0001 | 0.0006 | 0.0025 | 0.0073 |
HT | 0.974 | 0.001 | 0.626 | 0.012 | 0.0003 | 0.0059 | 0.0167 | 0.0054 | |||
DT | 0.971 | 0.027 | 0.601 | 0.012 | 0.0002 | 0.0035 | 0.0022 | 0.0019 | |||
AT | 0.986 | 0.010 | 0.582 | 0.011 | 0.0001 | 0.0059 | 0.0011 | 0.0032 | |||
MPT | SCAD | 0.970 | 0.019 | 0.551 | 0.010 | \ast | 0.0014 | 0.0006 | 0.0011 | ||
HT | 0.964 | 0.029 | 0.588 | 0.011 | 0.0001 | 0.0021 | 0.0041 | 0.0001 | |||
DT | 0.972 | 0.021 | 0.572 | 0.011 | 0.0001 | 0.0037 | 0.0002 | 0.0034 | |||
AT | 0.971 | 0.021 | 0.575 | 0.011 | 0.0001 | 0.0057 | 0.0005 | 0.0042 | |||
MPT | LASSO | 0.974 | 0.048 | 0.553 | 0.010 | 0.0001 | 0.0000 | 0.0002 | 0.0003 | ||
HT | 0.972 | 0.056 | 0.601 | 0.010 | 0.0001 | 0.0003 | 0.0001 | 0.0006 | |||
DT | 0.982 | 0.021 | 0.574 | 0.010 | 0.0001 | 0.0035 | 0.0001 | 0.0042 | |||
AT | 0.986 | 0.010 | 0.584 | 0.011 | 0.0001 | 0.0041 | 0.0002 | 0.0056 | |||
q_n =500 | MPT | MCP | 0.964 | 0.011 | 0.562 | 0.013 | 0.0001 | 0.0005 | 0.0015 | 0.0042 | |
HT | 0.972 | 0.010 | 0.670 | 0.018 | 0.0001 | 0.0056 | 0.0001 | 0.0115 | |||
DT | 0.987 | 0.011 | 0.567 | 0.012 | \ast | 0.0044 | 0.0003 | 0.0058 | |||
AT | 0.986 | 0.020 | 0.669 | 0.015 | 0.0001 | 0.0022 | 0.0108 | 0.0012 | |||
MPT | SCAD | 0.965 | 0.014 | 0.515 | 0.010 | 0.0001 | 0.0003 | 0.0055 | 0.0045 | ||
HT | 0.968 | 0.018 | 0.547 | 0.015 | 0.0001 | 0.0023 | 0.0112 | 0.0069 | |||
DT | 0.989 | 0.007 | 0.534 | 0.011 | 0.0001 | 0.0048 | 0.0001 | 0.0047 | |||
AT | 0.985 | 0.005 | 0.608 | 0.010 | \ast | 0.0042 | 0.0021 | 0.0007 | |||
MPT | LASSO | 0.978 | 0.006 | 0.536 | 0.012 | 0.0001 | 0.0013 | 0.0132 | 0.0104 | ||
HT | 0.970 | 0.002 | 0.644 | 0.015 | 0.0001 | 0.0000 | 0.0092 | 0.0126 | |||
DT | 0.987 | 0.005 | 0.545 | 0.012 | \ast | 0.0015 | 0.0007 | 0.0019 | |||
AT | 0.981 | 0.002 | 0.526 | 0.012 | \ast | 0.0011 | 0.0093 | 0.0045 | |||
Symbol \ast indicates value smaller than 0.0001. |
AMAE | AMSE | MSE | |||||||||
Model | Setting | Test | Penalty | TPR | FPR | g(\cdot) | Prob | \boldsymbol{\beta} | \beta_1 | \beta_2 | \beta_3 |
Example 4.3 ( q_n =50) | n=500 | MPT | MCP | 0.951 | 0.103 | 0.466 | 0.019 | 0.0003 | 0.0003 | 0.0009 | 0.0011 |
HT | 0.966 | 0.091 | 0.571 | 0.021 | 0.0005 | 0.0007 | 0.0036 | 0.0045 | |||
DT | 0.982 | 0.043 | 0.360 | 0.006 | 0.0001 | 0.0002 | 0.0001 | 0.0001 | |||
AT | 0.981 | 0.021 | 0.464 | 0.012 | 0.0001 | 0.0005 | 0.0009 | 0.0006 | |||
MPT | SCAD | 0.957 | 0.139 | 0.527 | 0.023 | 0.0005 | 0.0001 | 0.0031 | 0.0098 | ||
HT | 0.968 | 0.082 | 0.433 | 0.020 | 0.0004 | 0.0006 | 0.0001 | 0.0003 | |||
DT | 0.954 | 0.140 | 0.411 | 0.013 | 0.0002 | 0.0011 | 0.0018 | 0.0012 | |||
AT | 0.972 | 0.064 | 0.793 | 0.018 | 0.0002 | 0.0038 | 0.0021 | 0.0004 | |||
MPT | LASSO | 0.981 | 0.024 | 0.604 | 0.021 | 0.0003 | 0.0042 | 0.0014 | 0.0019 | ||
HT | 0.983 | 0.021 | 0.432 | 0.026 | 0.0001 | 0.0017 | 0.0005 | 0.0016 | |||
DT | 0.971 | 0.094 | 0.470 | 0.013 | 0.0002 | 0.0004 | 0.0014 | 0.0023 | |||
AT | 0.980 | 0.061 | 0.447 | 0.013 | 0.0002 | 0.0002 | 0.0004 | 0.0015 | |||
n=1000 | MPT | MCP | 0.988 | 0.040 | 0.358 | 0.015 | 0.0002 | 0.0011 | 0.0024 | 0.0042 | |
HT | 0.984 | 0.021 | 0.399 | 0.017 | 0.0006 | 0.0008 | 0.0009 | 0.0013 | |||
DT | 0.989 | 0.000 | 0.583 | 0.014 | 0.0001 | 0.0001 | 0.0019 | 0.0024 | |||
AT | 0.985 | 0.009 | 0.405 | 0.013 | 0.0001 | 0.0017 | 0.0041 | 0.0012 | |||
MPT | SCAD | 0.989 | 0.043 | 0.537 | 0.016 | 0.0002 | 0.0025 | 0.0004 | 0.0038 | ||
HT | 0.987 | 0.003 | 0.512 | 0.012 | 0.0001 | 0.0012 | 0.0032 | 0.0031 | |||
DT | 0.986 | 0.003 | 0.515 | 0.012 | 0.0001 | 0.0001 | 0.0002 | 0.0004 | |||
AT | 1.000 | 0.000 | 0.410 | 0.013 | 0.0001 | 0.0013 | 0.0022 | 0.0013 | |||
MPT | LASSO | 0.988 | 0.004 | 0.441 | 0.011 | 0.0002 | 0.0029 | 0.0012 | 0.0021 | ||
HT | 0.982 | 0.007 | 0.326 | 0.007 | 0.0001 | 0.0002 | 0.0004 | 0.0002 | |||
DT | 0.987 | 0.008 | 0.489 | 0.013 | 0.0001 | 0.0008 | 0.0001 | 0.0032 | |||
AT | 0.977 | 0.043 | 0.283 | 0.007 | 0.0001 | 0.0012 | 0.0024 | 0.0034 |
AMAE | AMSE | MSE | ||||||||||
Model | Setting | Test | Penalty | TPR | FPR | g(\cdot) | Prob | \boldsymbol{\beta} | \beta_1 | \beta_2 | \beta_3 | \beta_4 |
Example 4.4 ( q_n =100) | n=750 | MPT | MCP | 0.979 | 0.053 | 0.744 | 0.019 | 0.0004 | 0.0028 | 0.0015 | 0.0036 | 0.0076 |
HT | 0.959 | 0.100 | 0.970 | 0.027 | 0.0011 | 0.0024 | 0.0005 | 0.0022 | 0.0018 | |||
DT | 0.986 | 0.035 | 0.611 | 0.011 | 0.0001 | 0.0001 | 0.0025 | 0.0034 | 0.0016 | |||
AT | 0.984 | 0.043 | 0.789 | 0.013 | 0.0002 | 0.0012 | 0.0051 | 0.0026 | 0.0012 | |||
MPT | SCAD | 0.966 | 0.059 | 0.723 | 0.014 | 0.0003 | 0.0030 | 0.0078 | 0.0081 | 0.0001 | ||
HT | 0.978 | 0.069 | 0.576 | 0.014 | 0.0002 | 0.0004 | 0.0083 | 0.0052 | 0.0002 | |||
DT | 0.989 | 0.063 | 0.698 | 0.013 | 0.0003 | 0.0034 | 0.0155 | 0.0011 | 0.0051 | |||
AT | 0.981 | 0.052 | 0.671 | 0.022 | 0.0005 | 0.0078 | 0.0023 | 0.0085 | 0.0073 | |||
MPT | LASSO | 0.977 | 0.072 | 0.620 | 0.014 | 0.0003 | 0.0047 | 0.0041 | 0.0141 | 0.0002 | ||
HT | 0.964 | 0.069 | 0.680 | 0.015 | 0.0003 | 0.0018 | 0.0071 | 0.0073 | 0.0007 | |||
DT | 0.986 | 0.041 | 0.581 | 0.016 | 0.0003 | 0.0034 | 0.0090 | 0.0014 | 0.0005 | |||
AT | 0.984 | 0.065 | 0.679 | 0.016 | 0.0003 | 0.0001 | 0.0095 | 0.0065 | 0.0003 | |||
n=1000 | MPT | MCP | 0.967 | 0.029 | 0.706 | 0.015 | 0.0002 | 0.0068 | 0.0097 | 0.0022 | 0.0015 | |
HT | 0.986 | 0.001 | 0.818 | 0.012 | 0.0001 | 0.0035 | 0.0061 | 0.0007 | 0.0001 | |||
DT | 0.987 | 0.032 | 0.872 | 0.012 | 0.0002 | 0.0007 | 0.0074 | 0.0017 | 0.0016 | |||
AT | 0.988 | 0.037 | 0.800 | 0.027 | 0.0002 | 0.0013 | 0.0061 | 0.0002 | 0.0025 | |||
MPT | SCAD | 0.961 | 0.059 | 0.724 | 0.015 | 0.0002 | 0.0081 | 0.0087 | 0.0030 | 0.0006 | ||
HT | 0.974 | 0.010 | 0.779 | 0.013 | 0.0001 | 0.0036 | 0.0066 | 0.0012 | 0.0001 | |||
DT | 0.983 | 0.071 | 0.405 | 0.010 | 0.0001 | 0.0013 | 0.0059 | 0.0008 | 0.0001 | |||
AT | 0.981 | 0.041 | 0.422 | 0.010 | 0.0001 | 0.0003 | 0.0009 | 0.0020 | 0.0011 | |||
MPT | LASSO | 0.977 | 0.029 | 0.819 | 0.017 | 0.0004 | 0.0057 | 0.0012 | 0.0083 | 0.0079 | ||
HT | 0.951 | 0.004 | 0.545 | 0.043 | 0.0001 | 0.0093 | 0.0004 | 0.0004 | 0.0025 | |||
DT | 0.985 | 0.021 | 0.408 | 0.009 | 0.0001 | 0.0002 | 0.0011 | 0.0026 | 0.0007 | |||
AT | 0.989 | 0.008 | 0.581 | 0.010 | 0.0001 | 0.0042 | 0.0003 | 0.0004 | 0.0008 |
AMAE | MEAN | |||||||||
Model | Setting | Group Size | TPR | FPR | g(\cdot) | Prob | \beta_1 | \beta_2 | \beta_3 | \beta_4 |
Example 4 ( q_n =100) | n=750 | 2 | 0.970 | 0.015 | 0.611 | 0.011 | 0.452 | 0.465 | 0.478 | 0.460 |
4 | 0.965 | 0.020 | 0.581 | 0.016 | 0.445 | 0.405 | 0.464 | 0.477 | ||
6 | 0.986 | 0.041 | 0.627 | 0.009 | 0.519 | 0.497 | 0.487 | 0.495 | ||
8 | 0.973 | 0.020 | 0.594 | 0.012 | 0.471 | 0.467 | 0.484 | 0.477 | ||
n=1000 | 2 | 0.974 | 0.014 | 0.447 | 0.009 | 0.468 | 0.484 | 0.473 | 0.485 | |
4 | 0.964 | 0.018 | 0.408 | 0.009 | 0.489 | 0.468 | 0.450 | 0.474 | ||
6 | 0.985 | 0.021 | 0.440 | 0.011 | 0.486 | 0.478 | 0.443 | 0.471 | ||
8 | 0.974 | 0.010 | 0.466 | 0.009 | 0.494 | 0.494 | 0.447 | 0.437 |
Variable | \hat{\beta}^{norm}_{PLR} | \hat{\beta}_{our} | \hat{\beta}^{norm}_{aenet} | Variable | \hat{\beta}^{norm}_{PLR} | \hat{\beta}_{our} | \hat{\beta}^{norm}_{aenet} | Variable | \hat{\beta}^{norm}_{PLR} | \hat{\beta}_{our} | \hat{\beta}^{norm}_{aenet} |
age | 0.280 | 0.307 | -0.085 | Family history | Household income | ||||||
waist circumference | 0.178 | 0.194 | 0.271 | family history1 | 0.000 | 0.000 | 0.000 | household income1 | 0.000 | 0.000 | 0.000 |
BMI | 0.000 | 0.000 | 0.000 | family history2 | -0.492 | -0.567 | -0.466 | household income2 | 0.024 | 0.000 | 0.000 |
height | 0.000 | 0.000 | 0.000 | family history9 | 0.000 | 0.000 | 0.000 | household income3 | 0.000 | 0.000 | 0.000 |
weight | 0.000 | 0.000 | 0.000 | Physical activity | household income4 | 0.000 | -0.069 | 0.000 | |||
smoking age | 0.000 | 0.007 | 0.000 | physical activity1 | 0.000 | 0.056 | 0.000 | household income5 | 0.000 | 0.000 | 0.000 |
alcohol use | 0.009 | 0.013 | 0.000 | physical activity2 | -0.086 | -0.018 | 0.000 | household income6 | 0.000 | 0.000 | 0.000 |
leg length | -0.048 | -0.100 | -0.043 | physical activity3 | -0.134 | -0.039 | 0.000 | household income7 | 0.000 | 0.000 | 0.000 |
total cholesterol | 0.000 | 0.000 | 0.000 | physical activity4 | -0.088 | 0.000 | 0.000 | household income8 | 0.001 | 0.065 | 0.000 |
Hypertension | physical activity9 | 0.000 | 0.000 | 0.000 | household income9 | 0.000 | 0.000 | 0.000 | |||
hypertension1 | 0.000 | 0.000 | 0.000 | Sex | household income10 | 0.000 | 0.000 | 0.000 | |||
hypertension2 | -0.350 | -0.372 | -0.641 | sex1 | -0.010 | 0.000 | 0.000 | household income11 | 0.000 | 0.000 | 0.000 |
Education | sex2 | -0.237 | -0.225 | -0.424 | household income12 | 0.000 | 0.000 | 0.000 | |||
education1 | 0.000 | 0.000 | 0.000 | race | household income13 | 0.000 | 0.000 | 0.000 | |||
education2 | 0.000 | 0.000 | 0.000 | race1 | 0.000 | 0.000 | 0.000 | household income77 | 0.000 | 0.231 | 0.000 |
education3 | 0.000 | 0.000 | 0.000 | race2 | -0.019 | -0.073 | 0.000 | household income99 | 0.000 | 0.000 | 0.000 |
education4 | 0.000 | 0.000 | 0.000 | race3 | -0.399 | -0.380 | -0.330 | ||||
education5 | -0.014 | -0.052 | 0.000 | race4 | 0.000 | 0.000 | 0.000 | ||||
education7 | -0.523 | -0.335 | 0.000 | race5 | 0.000 | 0.124 | 0.000 |
AMAE | AMSE | MSE | |||||||
Model | Setting | (S_e, S_p) | TPR | FPR | g(\cdot) | Prob | \boldsymbol{\beta} | \beta_1 | \beta_2 |
Example 4.1 ( q_n=50 ) | n=500 | (0.98, 0.98) | 1.000 | 0.029 | 0.522 | 0.013 | 0.0001 | 0.0004 | 0.0003 |
(0.95, 0.95) | 0.987 | 0.020 | 0.474 | 0.011 | 0.0001 | 0.0003 | 0.0003 | ||
(0.90, 0.90) | 0.982 | 0.036 | 0.532 | 0.011 | 0.0001 | 0.0006 | 0.0007 | ||
(0.85, 0.85) | 0.984 | 0.040 | 0.578 | 0.016 | 0.0003 | 0.0001 | 0.0002 |
AMAE | AMSE | MSE | ||||||||
Model | Setting | (S_e, S_p) | TPR | FPR | g(\cdot) | Prob | \boldsymbol{\beta} | \beta_1 | \beta_2 | \beta_3 |
Example 4.2 ( q_n=100 ) | n=1000 | (0.98, 0.98) | 0.982 | 0.021 | 0.574 | 0.010 | 0.0001 | 0.0035 | 0.0001 | 0.0042 |
(0.95, 0.95) | 0.975 | 0.030 | 0.612 | 0.011 | 0.0001 | 0.0047 | 0.0001 | 0.0069 | ||
(0.90, 0.90) | 0.978 | 0.020 | 0.556 | 0.012 | 0.0001 | 0.0023 | 0.0002 | 0.0049 | ||
(0.85, 0.85) | 0.965 | 0.020 | 0.717 | 0.016 | 0.0004 | 0.0158 | 0.0002 | 0.0212 |
AMAE | AMSE | MSE | ||||||||
Model | Setting | (S_e, S_p) | TPR | FPR | g(\cdot) | Prob | \boldsymbol{\beta} | \beta_1 | \beta_2 | \beta_3 |
Example 4.3 ( q_n=50 ) | n=1000 | (0.98, 0.98) | 0.987 | 0.008 | 0.489 | 0.013 | 0.0001 | 0.0008 | 0.0001 | 0.0032 |
(0.95, 0.95) | 0.971 | 0.064 | 0.404 | 0.011 | 0.0003 | 0.0005 | 0.0033 | 0.0085 | ||
(0.90, 0.90) | 0.963 | 0.048 | 0.465 | 0.011 | 0.0001 | 0.0002 | 0.0012 | 0.0055 | ||
(0.85, 0.85) | 0.966 | 0.018 | 0.377 | 0.015 | 0.0004 | 0.0016 | 0.0023 | 0.0007 |
AMAE | AMSE | MSE | |||||||||
Model | Setting | (S_e, S_p) | TPR | FPR | g(\cdot) | Prob | \boldsymbol{\beta} | \beta_1 | \beta_2 | \beta_3 | \beta_4 |
Example 4.4 ( q_n=100 ) | n=750 | (0.98, 0.98) | 0.986 | 0.041 | 0.581 | 0.016 | 0.0003 | 0.0034 | 0.0090 | 0.0014 | 0.0005 |
(0.95, 0.95) | 0.981 | 0.026 | 0.534 | 0.018 | 0.0001 | 0.0016 | 0.0045 | 0.0018 | 0.0005 | ||
(0.90, 0.90) | 0.974 | 0.018 | 0.546 | 0.016 | 0.0002 | 0.0004 | 0.0024 | 0.0014 | 0.0028 | ||
(0.85, 0.85) | 0.976 | 0.024 | 0.539 | 0.011 | 0.0002 | 0.0047 | 0.0085 | 0.0004 | 0.0039 |
Variable | Implication | Variable | Implication |
Hypertension circumstance | Family history of diabetes | ||
hypertension1 | Have a history of hypertension | family history1 | Blood relatives with diabetes |
hypertension2 | No history of hypertension | family history2 | Blood relatives do not have diabetes |
Education level | family history9 | Not known if any blood relatives have diabetes | |
education1 | Less Than 9th Grade | Physical activity | |
education2 | 9 - 11th Grade (Includes 12th grade with no diploma) | physical activity1 | Sit during the day and do not walk about very much |
education3 | High School Grad/GED or Equivalent | physical activity2 | Stand or walk about a lot during the day, but do not have to carry or lift things very often |
education4 | Some College or AA degree | physical activity3 | Lift light load or has to climb stairs or hills often |
education5 | College Graduate or above | physical activity4 | Do heavy work or carry heavy loads |
education7 | Refuse to answer about the level of education | physical activity9 | Don't know physical activity level |
Household income | Sex | ||
household income1 | 0 to 4,999 fanxiexian_myfh | sex1 | Male |
household income2 | 5,000 to 9,999 fanxiexian_myfh | sex2 | Female |
household income3 | 10,000 to 14,999 fanxiexian_myfh | Race/Ethnicity | |
household income4 | 15,000 to 19,999 fanxiexian_myfh | race1 | Mexican American |
household income5 | 20,000 to 24,999 fanxiexian_myfh | race2 | Other Hispanic |
household income6 | 25,000 to 34,999 fanxiexian_myfh | race3 | Non - Hispanic White |
household income7 | 35,000 to 44,999 fanxiexian_myfh | race4 | Non - Hispanic Black |
household income8 | 45,000 to 54,999 fanxiexian_myfh | race5 | Other Race - Including Multi - Racial |
household income9 | 55,000 to 64,999 fanxiexian_myfh | ||
household income10 | 65,000 to 74,999 fanxiexian_myfh | ||
household income11 | 75,000 and Over fanxiexian_myfh | ||
household income12 | Over 20,000 fanxiexian_myfh | ||
household income13 | Under 20,000 fanxiexian_myfh | ||
household income77 | Refusal to answer about household income | ||
household income99 | Don't know household income |
Metric | Implication |
True positive (TP) | Actual positive and predicted positive |
False positive (FP) | Actual negative and predicted positive |
False negative (FN) | Actual positive and predicted negative |
True negative (TN) | Actual negative and predicted negative |
AMAE | AMSE | MSE | ||||||||
Model | Setting | Test | Penalty | TPR | FPR | g(\cdot) | Prob | \boldsymbol{\beta} | \beta_1 | \beta_2 |
Example 4.1 (n=500) | q_n =50 | MPT | MCP | 0.980 | 0.061 | 0.325 | 0.011 | 0.0003 | 0.0022 | 0.0015 |
HT | 0.985 | 0.003 | 0.413 | 0.007 | 0.0002 | 0.0068 | 0.0025 | |||
DT | 0.968 | 0.062 | 0.295 | 0.011 | 0.0003 | 0.0001 | 0.0004 | |||
AT | 0.987 | 0.035 | 0.388 | 0.009 | 0.0004 | 0.0019 | 0.0009 | |||
MPT | SCAD | 0.967 | 0.060 | 0.508 | 0.014 | 0.0003 | 0.0012 | 0.0021 | ||
HT | 0.988 | 0.001 | 0.479 | 0.008 | 0.0001 | 0.0021 | 0.0023 | |||
DT | 0.980 | 0.051 | 0.511 | 0.012 | 0.0003 | 0.0005 | 0.0004 | |||
AT | 0.974 | 0.063 | 0.432 | 0.009 | 0.0003 | 0.0035 | 0.0024 | |||
MPT | LASSO | 0.964 | 0.060 | 0.337 | 0.011 | 0.0003 | 0.0038 | 0.0051 | ||
HT | 0.986 | 0.003 | 0.436 | 0.006 | 0.0001 | 0.0003 | 0.0002 | |||
DT | 1.000 | 0.029 | 0.522 | 0.013 | 0.0001 | 0.0004 | 0.0003 | |||
AT | 0.981 | 0.034 | 0.320 | 0.007 | 0.0001 | 0.0006 | 0.0008 | |||
q_n =100 | MPT | MCP | 0.985 | 0.010 | 0.511 | 0.009 | 0.0001 | 0.0004 | 0.0004 | |
HT | 0.973 | 0.038 | 0.374 | 0.009 | 0.0002 | 0.0022 | 0.0033 | |||
DT | 0.986 | 0.023 | 0.338 | 0.010 | 0.0001 | 0.0004 | 0.0001 | |||
AT | 0.982 | 0.023 | 0.470 | 0.005 | 0.0001 | 0.0004 | 0.0005 | |||
MPT | SCAD | 0.987 | 0.031 | 0.265 | 0.013 | 0.0002 | 0.0002 | 0.0003 | ||
HT | 0.988 | 0.038 | 0.458 | 0.015 | 0.0005 | 0.0017 | 0.0001 | |||
DT | 0.978 | 0.051 | 0.451 | 0.011 | 0.0001 | 0.0008 | 0.0004 | |||
AT | 0.985 | 0.010 | 0.422 | 0.009 | 0.0001 | 0.0058 | 0.0047 | |||
MPT | LASSO | 0.987 | 0.026 | 0.478 | 0.010 | 0.0001 | 0.0008 | 0.0012 | ||
HT | 0.966 | 0.044 | 0.364 | 0.011 | 0.0003 | 0.0029 | 0.0052 | |||
DT | 0.984 | 0.031 | 0.503 | 0.012 | 0.0001 | 0.0001 | 0.0003 | |||
AT | 0.987 | 0.031 | 0.401 | 0.008 | 0.0001 | 0.0016 | 0.0014 |
AMAE | AMSE | MSE | |||||||||
Model | Setting | Test | Penalty | TPR | FPR | g(\cdot) | Prob | \boldsymbol{\beta} | \beta_1 | \beta_2 | \beta_3 |
Example 4.2 (n=1000) | q_n =100 | MPT | MCP | 0.980 | 0.001 | 0.569 | 0.011 | 0.0001 | 0.0006 | 0.0025 | 0.0073 |
HT | 0.974 | 0.001 | 0.626 | 0.012 | 0.0003 | 0.0059 | 0.0167 | 0.0054 | |||
DT | 0.971 | 0.027 | 0.601 | 0.012 | 0.0002 | 0.0035 | 0.0022 | 0.0019 | |||
AT | 0.986 | 0.010 | 0.582 | 0.011 | 0.0001 | 0.0059 | 0.0011 | 0.0032 | |||
MPT | SCAD | 0.970 | 0.019 | 0.551 | 0.010 | \ast | 0.0014 | 0.0006 | 0.0011 | ||
HT | 0.964 | 0.029 | 0.588 | 0.011 | 0.0001 | 0.0021 | 0.0041 | 0.0001 | |||
DT | 0.972 | 0.021 | 0.572 | 0.011 | 0.0001 | 0.0037 | 0.0002 | 0.0034 | |||
AT | 0.971 | 0.021 | 0.575 | 0.011 | 0.0001 | 0.0057 | 0.0005 | 0.0042 | |||
MPT | LASSO | 0.974 | 0.048 | 0.553 | 0.010 | 0.0001 | 0.0000 | 0.0002 | 0.0003 | ||
HT | 0.972 | 0.056 | 0.601 | 0.010 | 0.0001 | 0.0003 | 0.0001 | 0.0006 | |||
DT | 0.982 | 0.021 | 0.574 | 0.010 | 0.0001 | 0.0035 | 0.0001 | 0.0042 | |||
AT | 0.986 | 0.010 | 0.584 | 0.011 | 0.0001 | 0.0041 | 0.0002 | 0.0056 | |||
q_n =500 | MPT | MCP | 0.964 | 0.011 | 0.562 | 0.013 | 0.0001 | 0.0005 | 0.0015 | 0.0042 | |
HT | 0.972 | 0.010 | 0.670 | 0.018 | 0.0001 | 0.0056 | 0.0001 | 0.0115 | |||
DT | 0.987 | 0.011 | 0.567 | 0.012 | \ast | 0.0044 | 0.0003 | 0.0058 | |||
AT | 0.986 | 0.020 | 0.669 | 0.015 | 0.0001 | 0.0022 | 0.0108 | 0.0012 | |||
MPT | SCAD | 0.965 | 0.014 | 0.515 | 0.010 | 0.0001 | 0.0003 | 0.0055 | 0.0045 | ||
HT | 0.968 | 0.018 | 0.547 | 0.015 | 0.0001 | 0.0023 | 0.0112 | 0.0069 | |||
DT | 0.989 | 0.007 | 0.534 | 0.011 | 0.0001 | 0.0048 | 0.0001 | 0.0047 | |||
AT | 0.985 | 0.005 | 0.608 | 0.010 | \ast | 0.0042 | 0.0021 | 0.0007 | |||
MPT | LASSO | 0.978 | 0.006 | 0.536 | 0.012 | 0.0001 | 0.0013 | 0.0132 | 0.0104 | ||
HT | 0.970 | 0.002 | 0.644 | 0.015 | 0.0001 | 0.0000 | 0.0092 | 0.0126 | |||
DT | 0.987 | 0.005 | 0.545 | 0.012 | \ast | 0.0015 | 0.0007 | 0.0019 | |||
AT | 0.981 | 0.002 | 0.526 | 0.012 | \ast | 0.0011 | 0.0093 | 0.0045 | |||
Symbol \ast indicates value smaller than 0.0001. |
AMAE | AMSE | MSE | |||||||||
Model | Setting | Test | Penalty | TPR | FPR | g(\cdot) | Prob | \boldsymbol{\beta} | \beta_1 | \beta_2 | \beta_3 |
Example 4.3 ( q_n =50) | n=500 | MPT | MCP | 0.951 | 0.103 | 0.466 | 0.019 | 0.0003 | 0.0003 | 0.0009 | 0.0011 |
HT | 0.966 | 0.091 | 0.571 | 0.021 | 0.0005 | 0.0007 | 0.0036 | 0.0045 | |||
DT | 0.982 | 0.043 | 0.360 | 0.006 | 0.0001 | 0.0002 | 0.0001 | 0.0001 | |||
AT | 0.981 | 0.021 | 0.464 | 0.012 | 0.0001 | 0.0005 | 0.0009 | 0.0006 | |||
MPT | SCAD | 0.957 | 0.139 | 0.527 | 0.023 | 0.0005 | 0.0001 | 0.0031 | 0.0098 | ||
HT | 0.968 | 0.082 | 0.433 | 0.020 | 0.0004 | 0.0006 | 0.0001 | 0.0003 | |||
DT | 0.954 | 0.140 | 0.411 | 0.013 | 0.0002 | 0.0011 | 0.0018 | 0.0012 | |||
AT | 0.972 | 0.064 | 0.793 | 0.018 | 0.0002 | 0.0038 | 0.0021 | 0.0004 | |||
MPT | LASSO | 0.981 | 0.024 | 0.604 | 0.021 | 0.0003 | 0.0042 | 0.0014 | 0.0019 | ||
HT | 0.983 | 0.021 | 0.432 | 0.026 | 0.0001 | 0.0017 | 0.0005 | 0.0016 | |||
DT | 0.971 | 0.094 | 0.470 | 0.013 | 0.0002 | 0.0004 | 0.0014 | 0.0023 | |||
AT | 0.980 | 0.061 | 0.447 | 0.013 | 0.0002 | 0.0002 | 0.0004 | 0.0015 | |||
n=1000 | MPT | MCP | 0.988 | 0.040 | 0.358 | 0.015 | 0.0002 | 0.0011 | 0.0024 | 0.0042 | |
HT | 0.984 | 0.021 | 0.399 | 0.017 | 0.0006 | 0.0008 | 0.0009 | 0.0013 | |||
DT | 0.989 | 0.000 | 0.583 | 0.014 | 0.0001 | 0.0001 | 0.0019 | 0.0024 | |||
AT | 0.985 | 0.009 | 0.405 | 0.013 | 0.0001 | 0.0017 | 0.0041 | 0.0012 | |||
MPT | SCAD | 0.989 | 0.043 | 0.537 | 0.016 | 0.0002 | 0.0025 | 0.0004 | 0.0038 | ||
HT | 0.987 | 0.003 | 0.512 | 0.012 | 0.0001 | 0.0012 | 0.0032 | 0.0031 | |||
DT | 0.986 | 0.003 | 0.515 | 0.012 | 0.0001 | 0.0001 | 0.0002 | 0.0004 | |||
AT | 1.000 | 0.000 | 0.410 | 0.013 | 0.0001 | 0.0013 | 0.0022 | 0.0013 | |||
MPT | LASSO | 0.988 | 0.004 | 0.441 | 0.011 | 0.0002 | 0.0029 | 0.0012 | 0.0021 | ||
HT | 0.982 | 0.007 | 0.326 | 0.007 | 0.0001 | 0.0002 | 0.0004 | 0.0002 | |||
DT | 0.987 | 0.008 | 0.489 | 0.013 | 0.0001 | 0.0008 | 0.0001 | 0.0032 | |||
AT | 0.977 | 0.043 | 0.283 | 0.007 | 0.0001 | 0.0012 | 0.0024 | 0.0034 |
AMAE | AMSE | MSE | ||||||||||
Model | Setting | Test | Penalty | TPR | FPR | g(\cdot) | Prob | \boldsymbol{\beta} | \beta_1 | \beta_2 | \beta_3 | \beta_4 |
Example 4.4 ( q_n =100) | n=750 | MPT | MCP | 0.979 | 0.053 | 0.744 | 0.019 | 0.0004 | 0.0028 | 0.0015 | 0.0036 | 0.0076 |
HT | 0.959 | 0.100 | 0.970 | 0.027 | 0.0011 | 0.0024 | 0.0005 | 0.0022 | 0.0018 | |||
DT | 0.986 | 0.035 | 0.611 | 0.011 | 0.0001 | 0.0001 | 0.0025 | 0.0034 | 0.0016 | |||
AT | 0.984 | 0.043 | 0.789 | 0.013 | 0.0002 | 0.0012 | 0.0051 | 0.0026 | 0.0012 | |||
MPT | SCAD | 0.966 | 0.059 | 0.723 | 0.014 | 0.0003 | 0.0030 | 0.0078 | 0.0081 | 0.0001 | ||
HT | 0.978 | 0.069 | 0.576 | 0.014 | 0.0002 | 0.0004 | 0.0083 | 0.0052 | 0.0002 | |||
DT | 0.989 | 0.063 | 0.698 | 0.013 | 0.0003 | 0.0034 | 0.0155 | 0.0011 | 0.0051 | |||
AT | 0.981 | 0.052 | 0.671 | 0.022 | 0.0005 | 0.0078 | 0.0023 | 0.0085 | 0.0073 | |||
MPT | LASSO | 0.977 | 0.072 | 0.620 | 0.014 | 0.0003 | 0.0047 | 0.0041 | 0.0141 | 0.0002 | ||
HT | 0.964 | 0.069 | 0.680 | 0.015 | 0.0003 | 0.0018 | 0.0071 | 0.0073 | 0.0007 | |||
DT | 0.986 | 0.041 | 0.581 | 0.016 | 0.0003 | 0.0034 | 0.0090 | 0.0014 | 0.0005 | |||
AT | 0.984 | 0.065 | 0.679 | 0.016 | 0.0003 | 0.0001 | 0.0095 | 0.0065 | 0.0003 | |||
n=1000 | MPT | MCP | 0.967 | 0.029 | 0.706 | 0.015 | 0.0002 | 0.0068 | 0.0097 | 0.0022 | 0.0015 | |
HT | 0.986 | 0.001 | 0.818 | 0.012 | 0.0001 | 0.0035 | 0.0061 | 0.0007 | 0.0001 | |||
DT | 0.987 | 0.032 | 0.872 | 0.012 | 0.0002 | 0.0007 | 0.0074 | 0.0017 | 0.0016 | |||
AT | 0.988 | 0.037 | 0.800 | 0.027 | 0.0002 | 0.0013 | 0.0061 | 0.0002 | 0.0025 | |||
MPT | SCAD | 0.961 | 0.059 | 0.724 | 0.015 | 0.0002 | 0.0081 | 0.0087 | 0.0030 | 0.0006 | ||
HT | 0.974 | 0.010 | 0.779 | 0.013 | 0.0001 | 0.0036 | 0.0066 | 0.0012 | 0.0001 | |||
DT | 0.983 | 0.071 | 0.405 | 0.010 | 0.0001 | 0.0013 | 0.0059 | 0.0008 | 0.0001 | |||
AT | 0.981 | 0.041 | 0.422 | 0.010 | 0.0001 | 0.0003 | 0.0009 | 0.0020 | 0.0011 | |||
MPT | LASSO | 0.977 | 0.029 | 0.819 | 0.017 | 0.0004 | 0.0057 | 0.0012 | 0.0083 | 0.0079 | ||
HT | 0.951 | 0.004 | 0.545 | 0.043 | 0.0001 | 0.0093 | 0.0004 | 0.0004 | 0.0025 | |||
DT | 0.985 | 0.021 | 0.408 | 0.009 | 0.0001 | 0.0002 | 0.0011 | 0.0026 | 0.0007 | |||
AT | 0.989 | 0.008 | 0.581 | 0.010 | 0.0001 | 0.0042 | 0.0003 | 0.0004 | 0.0008 |
AMAE | MEAN | |||||||||
Model | Setting | Group Size | TPR | FPR | g(\cdot) | Prob | \beta_1 | \beta_2 | \beta_3 | \beta_4 |
Example 4 ( q_n =100) | n=750 | 2 | 0.970 | 0.015 | 0.611 | 0.011 | 0.452 | 0.465 | 0.478 | 0.460 |
4 | 0.965 | 0.020 | 0.581 | 0.016 | 0.445 | 0.405 | 0.464 | 0.477 | ||
6 | 0.986 | 0.041 | 0.627 | 0.009 | 0.519 | 0.497 | 0.487 | 0.495 | ||
8 | 0.973 | 0.020 | 0.594 | 0.012 | 0.471 | 0.467 | 0.484 | 0.477 | ||
n=1000 | 2 | 0.974 | 0.014 | 0.447 | 0.009 | 0.468 | 0.484 | 0.473 | 0.485 | |
4 | 0.964 | 0.018 | 0.408 | 0.009 | 0.489 | 0.468 | 0.450 | 0.474 | ||
6 | 0.985 | 0.021 | 0.440 | 0.011 | 0.486 | 0.478 | 0.443 | 0.471 | ||
8 | 0.974 | 0.010 | 0.466 | 0.009 | 0.494 | 0.494 | 0.447 | 0.437 |
Variable | \hat{\beta}^{norm}_{PLR} | \hat{\beta}_{our} | \hat{\beta}^{norm}_{aenet} | Variable | \hat{\beta}^{norm}_{PLR} | \hat{\beta}_{our} | \hat{\beta}^{norm}_{aenet} | Variable | \hat{\beta}^{norm}_{PLR} | \hat{\beta}_{our} | \hat{\beta}^{norm}_{aenet} |
age | 0.280 | 0.307 | -0.085 | Family history | Household income | ||||||
waist circumference | 0.178 | 0.194 | 0.271 | family history1 | 0.000 | 0.000 | 0.000 | household income1 | 0.000 | 0.000 | 0.000 |
BMI | 0.000 | 0.000 | 0.000 | family history2 | -0.492 | -0.567 | -0.466 | household income2 | 0.024 | 0.000 | 0.000 |
height | 0.000 | 0.000 | 0.000 | family history9 | 0.000 | 0.000 | 0.000 | household income3 | 0.000 | 0.000 | 0.000 |
weight | 0.000 | 0.000 | 0.000 | Physical activity | household income4 | 0.000 | -0.069 | 0.000 | |||
smoking age | 0.000 | 0.007 | 0.000 | physical activity1 | 0.000 | 0.056 | 0.000 | household income5 | 0.000 | 0.000 | 0.000 |
alcohol use | 0.009 | 0.013 | 0.000 | physical activity2 | -0.086 | -0.018 | 0.000 | household income6 | 0.000 | 0.000 | 0.000 |
leg length | -0.048 | -0.100 | -0.043 | physical activity3 | -0.134 | -0.039 | 0.000 | household income7 | 0.000 | 0.000 | 0.000 |
total cholesterol | 0.000 | 0.000 | 0.000 | physical activity4 | -0.088 | 0.000 | 0.000 | household income8 | 0.001 | 0.065 | 0.000 |
Hypertension | physical activity9 | 0.000 | 0.000 | 0.000 | household income9 | 0.000 | 0.000 | 0.000 | |||
hypertension1 | 0.000 | 0.000 | 0.000 | Sex | household income10 | 0.000 | 0.000 | 0.000 | |||
hypertension2 | -0.350 | -0.372 | -0.641 | sex1 | -0.010 | 0.000 | 0.000 | household income11 | 0.000 | 0.000 | 0.000 |
Education | sex2 | -0.237 | -0.225 | -0.424 | household income12 | 0.000 | 0.000 | 0.000 | |||
education1 | 0.000 | 0.000 | 0.000 | race | household income13 | 0.000 | 0.000 | 0.000 | |||
education2 | 0.000 | 0.000 | 0.000 | race1 | 0.000 | 0.000 | 0.000 | household income77 | 0.000 | 0.231 | 0.000 |
education3 | 0.000 | 0.000 | 0.000 | race2 | -0.019 | -0.073 | 0.000 | household income99 | 0.000 | 0.000 | 0.000 |
education4 | 0.000 | 0.000 | 0.000 | race3 | -0.399 | -0.380 | -0.330 | ||||
education5 | -0.014 | -0.052 | 0.000 | race4 | 0.000 | 0.000 | 0.000 | ||||
education7 | -0.523 | -0.335 | 0.000 | race5 | 0.000 | 0.124 | 0.000 |
AMAE | AMSE | MSE | |||||||
Model | Setting | (S_e, S_p) | TPR | FPR | g(\cdot) | Prob | \boldsymbol{\beta} | \beta_1 | \beta_2 |
Example 4.1 ( q_n=50 ) | n=500 | (0.98, 0.98) | 1.000 | 0.029 | 0.522 | 0.013 | 0.0001 | 0.0004 | 0.0003 |
(0.95, 0.95) | 0.987 | 0.020 | 0.474 | 0.011 | 0.0001 | 0.0003 | 0.0003 | ||
(0.90, 0.90) | 0.982 | 0.036 | 0.532 | 0.011 | 0.0001 | 0.0006 | 0.0007 | ||
(0.85, 0.85) | 0.984 | 0.040 | 0.578 | 0.016 | 0.0003 | 0.0001 | 0.0002 |
AMAE | AMSE | MSE | ||||||||
Model | Setting | (S_e, S_p) | TPR | FPR | g(\cdot) | Prob | \boldsymbol{\beta} | \beta_1 | \beta_2 | \beta_3 |
Example 4.2 ( q_n=100 ) | n=1000 | (0.98, 0.98) | 0.982 | 0.021 | 0.574 | 0.010 | 0.0001 | 0.0035 | 0.0001 | 0.0042 |
(0.95, 0.95) | 0.975 | 0.030 | 0.612 | 0.011 | 0.0001 | 0.0047 | 0.0001 | 0.0069 | ||
(0.90, 0.90) | 0.978 | 0.020 | 0.556 | 0.012 | 0.0001 | 0.0023 | 0.0002 | 0.0049 | ||
(0.85, 0.85) | 0.965 | 0.020 | 0.717 | 0.016 | 0.0004 | 0.0158 | 0.0002 | 0.0212 |
AMAE | AMSE | MSE | ||||||||
Model | Setting | (S_e, S_p) | TPR | FPR | g(\cdot) | Prob | \boldsymbol{\beta} | \beta_1 | \beta_2 | \beta_3 |
Example 4.3 ( q_n=50 ) | n=1000 | (0.98, 0.98) | 0.987 | 0.008 | 0.489 | 0.013 | 0.0001 | 0.0008 | 0.0001 | 0.0032 |
(0.95, 0.95) | 0.971 | 0.064 | 0.404 | 0.011 | 0.0003 | 0.0005 | 0.0033 | 0.0085 | ||
(0.90, 0.90) | 0.963 | 0.048 | 0.465 | 0.011 | 0.0001 | 0.0002 | 0.0012 | 0.0055 | ||
(0.85, 0.85) | 0.966 | 0.018 | 0.377 | 0.015 | 0.0004 | 0.0016 | 0.0023 | 0.0007 |
AMAE | AMSE | MSE | |||||||||
Model | Setting | (S_e, S_p) | TPR | FPR | g(\cdot) | Prob | \boldsymbol{\beta} | \beta_1 | \beta_2 | \beta_3 | \beta_4 |
Example 4.4 ( q_n=100 ) | n=750 | (0.98, 0.98) | 0.986 | 0.041 | 0.581 | 0.016 | 0.0003 | 0.0034 | 0.0090 | 0.0014 | 0.0005 |
(0.95, 0.95) | 0.981 | 0.026 | 0.534 | 0.018 | 0.0001 | 0.0016 | 0.0045 | 0.0018 | 0.0005 | ||
(0.90, 0.90) | 0.974 | 0.018 | 0.546 | 0.016 | 0.0002 | 0.0004 | 0.0024 | 0.0014 | 0.0028 | ||
(0.85, 0.85) | 0.976 | 0.024 | 0.539 | 0.011 | 0.0002 | 0.0047 | 0.0085 | 0.0004 | 0.0039 |
Variable | Implication | Variable | Implication |
Hypertension circumstance | Family history of diabetes | ||
hypertension1 | Have a history of hypertension | family history1 | Blood relatives with diabetes |
hypertension2 | No history of hypertension | family history2 | Blood relatives do not have diabetes |
Education level | family history9 | Not known if any blood relatives have diabetes | |
education1 | Less Than 9th Grade | Physical activity | |
education2 | 9 - 11th Grade (Includes 12th grade with no diploma) | physical activity1 | Sit during the day and do not walk about very much |
education3 | High School Grad/GED or Equivalent | physical activity2 | Stand or walk about a lot during the day, but do not have to carry or lift things very often |
education4 | Some College or AA degree | physical activity3 | Lift light load or has to climb stairs or hills often |
education5 | College Graduate or above | physical activity4 | Do heavy work or carry heavy loads |
education7 | Refuse to answer about the level of education | physical activity9 | Don't know physical activity level |
Household income | Sex | ||
household income1 | 0 to 4,999 fanxiexian_myfh | sex1 | Male |
household income2 | 5,000 to 9,999 fanxiexian_myfh | sex2 | Female |
household income3 | 10,000 to 14,999 fanxiexian_myfh | Race/Ethnicity | |
household income4 | 15,000 to 19,999 fanxiexian_myfh | race1 | Mexican American |
household income5 | 20,000 to 24,999 fanxiexian_myfh | race2 | Other Hispanic |
household income6 | 25,000 to 34,999 fanxiexian_myfh | race3 | Non - Hispanic White |
household income7 | 35,000 to 44,999 fanxiexian_myfh | race4 | Non - Hispanic Black |
household income8 | 45,000 to 54,999 fanxiexian_myfh | race5 | Other Race - Including Multi - Racial |
household income9 | 55,000 to 64,999 fanxiexian_myfh | ||
household income10 | 65,000 to 74,999 fanxiexian_myfh | ||
household income11 | 75,000 and Over fanxiexian_myfh | ||
household income12 | Over 20,000 fanxiexian_myfh | ||
household income13 | Under 20,000 fanxiexian_myfh | ||
household income77 | Refusal to answer about household income | ||
household income99 | Don't know household income |