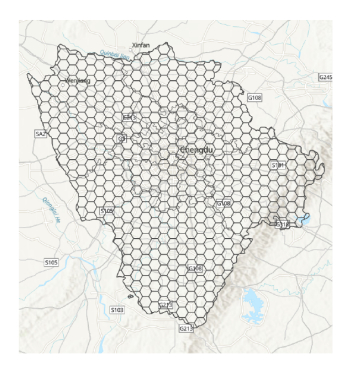
In the past few decades, many researchers have focused their research interests on nanocomposite hydrogel fibers (NHFs). These practitioners have developed and optimized techniques for preparing nanofiber membranes such as the template method, microfluidic spinning, electrospinning, wet spinning and three-dimensional printing (3D printing). NHFs have important applications in wearable monitoring, diagnosis and nursing due to their various excellent properties (such as high-water content, porous morphology, flexibility, braiding and rich active functional groups). In this paper, the latest progress of NHFs in pose monitoring, continuous monitoring of physiological indicators, diagnosis, wearables, nursing, drug delivery and dressings are reviewed. This paper also aims to review their key operational parameters, advantages and disadvantages of NHFs in the above fields, including sensitivity, working range and other special properties. Specifically, NHFs can be used for continuous monitoring of biological postures (such as gestures) or physiological indicators (such as blood sugar) in vitro and in vivo. NHFs also can be used for long-term monitoring of related indicators in the wearable field. NHFs can be used in tissue engineering and drug delivery. Finally, we look forward to the development prospects, challenges and opportunities of the next generation of NHFs. We confirm that the emergence of NHFs in the field of diagnosis and treatment has opened up a new vision for human health. Researchers have optimized the template method, microfluidic spinning, electrospinning, wet spinning and 3D printing.
Citation: Zhenguo Yu, Dong Wang, Zhentan Lu. Nanocomposite hydrogel fibers in the field of diagnosis and treatment[J]. AIMS Materials Science, 2023, 10(6): 1004-1033. doi: 10.3934/matersci.2023054
[1] | Hongqiang Zhu . A graph neural network-enhanced knowledge graph framework for intelligent analysis of policing cases. Mathematical Biosciences and Engineering, 2023, 20(7): 11585-11604. doi: 10.3934/mbe.2023514 |
[2] | Xiaowen Jia, Jingxia Chen, Kexin Liu, Qian Wang, Jialing He . Multimodal depression detection based on an attention graph convolution and transformer. Mathematical Biosciences and Engineering, 2025, 22(3): 652-676. doi: 10.3934/mbe.2025024 |
[3] | Xin Liu, Chen Zhao, Bin Zheng, Qinwei Guo, Yuanyuan Yu, Dezheng Zhang, Aziguli Wulamu . Spatiotemporal and kinematic characteristics augmentation using Dual-GAN for ankle instability detection. Mathematical Biosciences and Engineering, 2022, 19(10): 10037-10059. doi: 10.3934/mbe.2022469 |
[4] | Saranya Muniyappan, Arockia Xavier Annie Rayan, Geetha Thekkumpurath Varrieth . DTiGNN: Learning drug-target embedding from a heterogeneous biological network based on a two-level attention-based graph neural network. Mathematical Biosciences and Engineering, 2023, 20(5): 9530-9571. doi: 10.3934/mbe.2023419 |
[5] | Shuo Zhang, Yonghao Ren, Jing Wang, Bo Song, Runzhi Li, Yuming Xu . GSTCNet: Gated spatio-temporal correlation network for stroke mortality prediction. Mathematical Biosciences and Engineering, 2022, 19(10): 9966-9982. doi: 10.3934/mbe.2022465 |
[6] | Yijia Wang, Na Xie, Zhe Wang, Shuzhen Ding, Xijian Hu, Kai Wang . Spatio-temporal distribution characteristics of the risk of viral hepatitis B incidence based on INLA in 14 prefectures of Xinjiang from 2004 to 2019. Mathematical Biosciences and Engineering, 2023, 20(6): 10678-10693. doi: 10.3934/mbe.2023473 |
[7] | Suqi Zhang, Wenfeng Wang, Ningning Li, Ningjing Zhang . Multi-behavioral recommendation model based on dual neural networks and contrast learning. Mathematical Biosciences and Engineering, 2023, 20(11): 19209-19231. doi: 10.3934/mbe.2023849 |
[8] | Lu Yuan, Yuming Ma, Yihui Liu . Protein secondary structure prediction based on Wasserstein generative adversarial networks and temporal convolutional networks with convolutional block attention modules. Mathematical Biosciences and Engineering, 2023, 20(2): 2203-2218. doi: 10.3934/mbe.2023102 |
[9] | Kunli Zhang, Bin Hu, Feijie Zhou, Yu Song, Xu Zhao, Xiyang Huang . Graph-based structural knowledge-aware network for diagnosis assistant. Mathematical Biosciences and Engineering, 2022, 19(10): 10533-10549. doi: 10.3934/mbe.2022492 |
[10] | Hanyu Zhao, Chao Che, Bo Jin, Xiaopeng Wei . A viral protein identifying framework based on temporal convolutional network. Mathematical Biosciences and Engineering, 2019, 16(3): 1709-1717. doi: 10.3934/mbe.2019081 |
In the past few decades, many researchers have focused their research interests on nanocomposite hydrogel fibers (NHFs). These practitioners have developed and optimized techniques for preparing nanofiber membranes such as the template method, microfluidic spinning, electrospinning, wet spinning and three-dimensional printing (3D printing). NHFs have important applications in wearable monitoring, diagnosis and nursing due to their various excellent properties (such as high-water content, porous morphology, flexibility, braiding and rich active functional groups). In this paper, the latest progress of NHFs in pose monitoring, continuous monitoring of physiological indicators, diagnosis, wearables, nursing, drug delivery and dressings are reviewed. This paper also aims to review their key operational parameters, advantages and disadvantages of NHFs in the above fields, including sensitivity, working range and other special properties. Specifically, NHFs can be used for continuous monitoring of biological postures (such as gestures) or physiological indicators (such as blood sugar) in vitro and in vivo. NHFs also can be used for long-term monitoring of related indicators in the wearable field. NHFs can be used in tissue engineering and drug delivery. Finally, we look forward to the development prospects, challenges and opportunities of the next generation of NHFs. We confirm that the emergence of NHFs in the field of diagnosis and treatment has opened up a new vision for human health. Researchers have optimized the template method, microfluidic spinning, electrospinning, wet spinning and 3D printing.
With the rapid development of companies such as Didi, Uber, and Grab in the global ride-hailing service sector, ride-hailing has become one of the primary modes of transportation for people. According to statistics, there are currently 322 ride-hailing platform companies in China[1], and the number of users has reached 472 million[2]. In addition, the number of ride-hailing drivers has reached a high of 5,976,000 as of July 2023, an increase of 1,376,000 compared to last year[3]. In July, there were 821 million order information records. These data clearly show that the ride-hailing industry is in a stage of rapid growth and booming development. However, under the trend of expanding the ride-hailing market, balancing the distribution of supply and demand is still an urgent problem for ride-hailing platforms [4,5,6]. This is mainly in two aspects: From a passenger's perspective, due to the uncertainty of passenger travel and the aggregation of passengers, there may be longer waiting times for vehicles during peak hours or in specific areas. From a driver's perspective, drivers often offer services in areas where they believe there is more passenger demand. However, this can lead to oversupply in some areas and undersupply in others[7]. In the face of numerous driver and user demands, it has become crucial for ride-hailing platforms to fully utilize their existing operational data for effective demand forecasting and scheduling. This can enhance service quality, improve user experience, and increase vehicle utilization[8,9].
The prediction of ride-hailing demand shares many similarities with traditional taxi and traffic flow forecasting. Previous research in the field of transportation has laid the foundation for predicting ride-hailing demand. As early as 1978, Yang et al.[10] considered factors such as the number of taxis, taxi fares, and disposable income as endogenous variables in their study aimed at improving service levels. In 1972, Douglas [11] indicated that a reasonable number of taxis and pricing could enhance the service quality for passengers. With the widespread application of Global Positioning System (GPS) in taxis, a foundation was laid for research based on GPS data. In 2010, Bazzani et al.[12] utilized GPS data to analyze complex social systems. Asmundsdottir et al.[13] through the analysis of taxi GPS data, extracted travel characteristics of taxi passengers. However, predicting the demand for ride-hailing orders is a complex task. Its complexity is not only dependent on GPS data [14], but is also influenced by various factors such as time [15,16], space [17,18,19], and the environment [20,21]. This can be regarded as a complex spatiotemporal data prediction problem. Currently, spatiotemporal data prediction encompasses various aspects such as taxi demand [7], traffic flow [16], shared bicycle demand [22], etc. These share similarities with ride-hailing demand, demonstrating continuous spatial distribution and interconnectedness between areas. To address spatial distribution challenges, it is essential to partition them into grids [23,24,25] or structures based on road networks [26], thereby transforming spatial issues into graph model processing. To investigate the impact of region partitioning methods, Davis et al.[27] analyzed the impact of different spatial partitioning strategies on taxi demand prediction and proposed an efficient hybrid surface subdivision algorithm. In addition, external environmental factors such as weather conditions, holidays, and the distribution of Points of Interest (POI) have a significant impact on the demand for ride-hailing services. For instance, during rainy or extremely hot weather, individuals may be more inclined to use ride-hailing services, leading to an increase in demand. Similarly, during holiday periods, people might prefer choosing ride-hailing as their mode of transportation for travel or social activities, resulting in a potential surge in demand. Furthermore, individuals tend to seek ride-hailing services more frequently in commercial areas, tourist attractions, or event centers. Taking into account these factors, a comprehensive analysis of external spatiotemporal elements enhances our understanding and prediction of fluctuations in ride-hailing demand. References [7,24] confirm that studies considering time and weather conditions are promising. In such a complex and dynamic predictive environment, designing an accurate prediction model is crucial for enhancing the quality of ride-hailing services.
Researchers in the field of transportation have already accumulated rich and in-depth achievements, covering aspects such as traffic flow prediction, taxi order prediction, and ride-hailing order prediction. We can categorize the research methods into the following three types:
Prediction models based on statistical methods. Ride-hailing demand prediction is similar to other transportation prediction and can be viewed as a time series prediction problem[28]. Representative models in this field of application include the historical average model (HA)[29], the differential autoregressive moving average model (ARIMA) [30], and its variants. Williams et al. [31] proposed and demonstrated in 2003 that the Seasonal Autoregressive Integrated Moving Average (SARIMA) model is capable of capturing the seasonality in time series data. Moreira-Matias et al. [32] validated the feasibility of the ARIMA model in predicting taxi passenger demand using GPS trajectory data from Porto. Singh et al. [33] demonstrated the superiority of the ARIMA model by predicting the performance of virtual machines. However, these traditional models impose strict linear assumptions, insufficiently consider spatiotemporal correlations and the influence of external factors, and are incapable of handling nonlinear features. Therefore, their predictive performance is suboptimal when influenced by external factors.
Prediction models based on traditional machine learning. In recent years, machine learning methods have gradually become the primary methods for demand prediction[34,35,36], and they can achieve higher prediction accuracy and more sophisticated data modeling. For example, Yang and Gonzales [37] mined the factors of taxi demand from the number of cab users and socio-economic and employment data in New York. They used a multiple linear regression model to analyze passenger flow prediction in a particular area and verified its validity. Jiang et al. [38] proposed a least-squares support vector machine (LS-SVM) based method for ride-hailing short-term prediction and demonstrated its excellent performance. Peñalvo et al.[39] proposed a machine learning framework for predicting the fluctuation of stock prices. Lippi et al. [40] constructed a Support Vector Regression (SVR) model with seasonal identification capability to extract the seasonality of traffic flow. Castro-Neto et al. [41] proposed the Online-SVR (OL-SVR) prediction model, considering both typical and atypical conditions, thereby enhancing predictive capabilities under atypical conditions. However, when machine learning is utilized for complex data prediction, challenges such as poor predictive accuracy and overfitting may arise, representing limitations inherent in machine learning.
Deep learning-based prediction modeling. With the rapid development of deep learning methods in various fields such as computer vision [42,43], natural language processing [44], and recommendation systems [45], the application scope continues to expand. Traffic prediction [46,47] is a crucial domain where deep learning methods excel in capturing the nonlinearity and dynamic trends of data for modeling [48,49,50]. Demand prediction for ride-hailing is a typical time-series prediction problem. In the early stages, researchers commonly utilized Recurrent Neural Networks (RNN) for time-series data prediction. However, RNN faces challenges such as vanishing and exploding gradients, limiting its ability to capture long-term dependencies. Conversely, variants of RNN, such as Long Short-Term Memory (LSTM) and Gated Recurrent Unit (GRU), demonstrate certain advantages in capturing temporal dependencies and are frequently utilized for extracting time-dependent features in time-series prediction data [15,16]. Dogan [51] demonstrated that expanding the dataset of traffic flow can enhance the predictive performance of LSTMs. Kouziokas [52] optimized unidirectional LSTM and proposed Bidirectional LSTM (Bi-LSTM) to improve prediction accuracy. Dai et al. [53] proved in their research on traffic flow prediction that GRU outperforms LSTM in terms of performance. Additionally, spatial relationships are also a crucial factor that needs to be fully considered in this research field, contributing to extracting the spatial variations of transportation systems. Huang et al. [54] utilized a Convolutional Neural Network (CNN) model for regional partitioning to predict the demand for ride-hailing trips. However, CNN, when dealing with regional connectivity graphs, represents the regional network in the form of a two-dimensional image, limiting its applicability in non-Euclidean topology regional networks. Therefore, in recent years, many researchers have addressed the limitations of regional topology structures by employing Graph Convolutional Networks (GCNs) for processing [18]. Compared to CNNs, GCNs are better suited for capturing the spatial dependencies of regional networks [55]. Hence, Geng et al.[56] in their study of non-Euclidean regional structures, utilized GCN as a graph convolutional module and proposed the Spatiotemporal Multi-Graph Convolutional Network (ST-MGCN) model for demand prediction.
To better adapt to various complex environments and fully leverage the advantages of different algorithms in extracting spatiotemporal correlations, researchers are gradually and widely applying composite models [46,57,58]. In 2009, Tsai et al. [59] demonstrated the superiority of composite models through Parallel Ensemble Neural Networks (PENN). Li and Zhu [60] enhanced traffic flow prediction performance by integrating graph modules and gated convolutional modules. Ke et al. [8], considering the temporal, spatial, and exogenous dependencies of ride-hailing demand, proposed a Fusion Convolutional Long Short-Term Memory Network (FCL-Net) by combining Cov-LSTM, LSTM, and CNN, showing strong adaptability in predictions. In 2018, Li et al. [47] proposed a model called the Diffusion Convolutional Recurrent Neural Network (DCRNN) to address the complex spatial characteristics of road networks and the non-linear temporal dynamics of road condition changes. The model utilizes bidirectional random walks in the graph structure to capture spatial dependencies and employs a predetermined sampling encoder-decoder architecture to capture temporal dependencies. Zhao et al. [61] introduced a Time Graph Convolutional Network (T-GCN) model, which combines GCN with GRU. This model takes advantage of GCN for spatial information extraction and GRU for capturing dynamic temporal relationships to predict traffic flow, producing predictions close to real dataset values.
According to the above analysis, despite the current capability of many studies in extracting spatiotemporal relationships for traffic prediction, there is still a deficiency in capturing the impact of external spatiotemporal factors. On one hand, a majority of studies either neglect external spatiotemporal factors or insufficiently extract key information during the extraction process. On the other hand, the use of a single model is often susceptible to the influence of data complexity, resulting in suboptimal predictive accuracy. To address these issues, this study proposes a Spatiotemporal Information-Enhanced Graph Convolutional Network model (EST-GCN) that effectively tackles both of these challenges. Our main contributions to the work are as follows:
(1) The paper introduces an innovative model for predicting ride-hailing demand, named EST-GCN. It utilizes correlation analysis to extract essential information from external factors and integrates it with a spatiotemporal graph convolutional model. This is designed to accurately capture the spatiotemporal features of ride-hailing demand and the influence of external spatiotemporal factors.
(2) The EST-GCN model can adapt to the effects of weather conditions, date attributes, and the distribution of POIs, enabling more accurate prediction of ride-hailing demand in different environments.
(3) We evaluated the model using actual operational data, and the experimental results show that the EST-GCN model outperforms the baseline method in prediction and has vital portability.
Definition 1: Spatial Gridding
Based on the latitude and longitude of the city, the size and shape of each hexagon are determined to partition the entire area. As illustrated in Figure 1, the city is partitioned into a spatial hexagonal grid of P×Q specifications, with each spatial grid referring to an area Sij(i∈1...P,j∈1...Q).
Definition 2: Demand Characterization Matrix X
The demand for ride-hailing refers to the users' need for ride-hailing services during a specific period, typically measured using the number of orders placed. In this paper, xt represents the demand of the tth moment.
Definition 3:Areas Network G
We approximate the spatial grid as a transportation network and utilize the graph structure G=(V,E) to represent the connectivity between different areas network. V=(v1,v2,...,vn) denotes the set of spatial area grids, n the number of grids, E=(e1,e2,...,em) the set of edges denoting the connectivity between two areas, and m the number of edges. Then, the adjacency matrix A is used to represent the connectivity of the areas network.
Definition 4: External attribute matrix H
We form factors such as time periodicity, POIs, weather, and date attributes into a feature matrix H=h1,h2,...,hc, where c is the category number of external spatiotemporal factors. The time-varying information for the class j of factors is represented as Hj=j1,j2,...,jt, while for factors that do not vary with time, jt remains a fixed value.
Ride-hailing demand prediction is a spatiotemporal data prediction problem that varies continuously over time in different areas. This type of problem requires extractive modeling of temporal and spatial relationships[26,62]. Figure 2 illustrates the spatiotemporal correlation of ride-hailing demands. In the spatial dimension Figure 2(a), the neighborhoods of different areas form a network graph, and each vertex state of the graph represents the ride-hailing demand of the area, and in the temporal dimension Figure 2(b), the ride-hailing of the different areas is constantly changing with time. In conclusion, the correlation of ride-hailing demands shows strong dynamics in both spatial and temporal dimensions.
Building upon the exploration of spatiotemporal features, this paper further incorporates external spatiotemporal factors into the model, thereby enhancing the model's ability to perceive the impact of external factors.
To summarize, the ride-hailing demand prediction problem can be understood as predicting the most probable demand result in the following T time steps given the topological network G, the demand feature matrix X, and the external attribute matrix H, combined with the given n historical demand measurement values. The mapping relationship for this problem can be defined and represented as
f(Xt−n:t|H,G)→X(t+1):(t+T) | (2.1) |
To comprehensively account for the external spatiotemporal factors affecting ride-hailing demand, we divide these into two main categories: dynamic factors that change over time, and static factors that do not change with short-term fluctuations in time.
(1) Static Factors
Static factors impact demand that does not change over a short-term time horizon. For example, POI distribution information and date attributes do not vary from area to area over short time scales. Still, the characteristics they imply have the potential to be able to influence the movement and aggregation of people within an area. As shown in Figure 3, we can observe a difference in the number of POIs and demand between Area 1 and Area 2. In Area 1, the number of POIs is higher, and the order is higher during the time of day when the activity occurs. Within a week, the demand on weekends is significantly higher than on weekdays. These analyses indicate that the distribution of POI and date attributes has an impact on ride-hailing demand.
(2) Dynamic Factors
Dynamic factors change over time and can impact ride-hailing demand. For example, weather conditions can significantly affect travel, which directly affects the demand for ride-hailing. Figure 4 shows the variation of ride-hailing demand in the same area under different weather conditions. Specifically, during the rainy period, the demand surges and deviates far from the order quantity during regular hours. The analysis shows that weather has an enormous impact on ride-hailing demand.
This method integrates the features of ride-hailing demand in the area with external factor features. It employs correlation analysis to extract the main features, utilizes a GRU layer to capture temporal features, and incorporates a GCN layer to extract spatial features, enhancing the accuracy of ride-hailing predictions. We present the framework of our work in Figure 5, comprising four main components: data preprocessing, integration of external attributes, modeling spatiotemporal dependencies, and prediction.
In the data preprocessing phase, we conducted cleaning and feature engineering on the original dataset. For the extraction of spatiotemporal features, we employed the Pearson correlation coefficient to analyze the correlation. Features with correlations greater than the threshold α were selected for model training. Subsequently, we performed encoding and fusion processing on the selected external features and the ride-hailing demand data.
To effectively model spatiotemporal dependencies, we have chosen a combination of GRU and GCN models. These two models are used to extract the temporal and spatial features of ride-hailing demand data, enhancing the overall prediction accuracy. The GRU model is responsible for capturing temporal changes, while the GCN model focuses on modeling the spatial relationships between different locations in the transportation network. Through this combination, we expect to comprehensively consider spatiotemporal factors and improve the accurate prediction of ride-hailing demand.
This study conducts experiments by analyzing the correlation between external factors and short-term demand for ride-hailing. It extracts highly correlated attributes to mitigate the impact of the specificity of numerical values on experimental results.
(1) Feature extraction
We use the Pearson correlation coefficient to characterize the strength of linear correlation, denoted as r, between two attributes. We calculate the value of r using Eq (3.1) and select features with an r value greater than the threshold α for experimentation.
r=∑ni=1(xi−ˉx)(yi−ˉy)√∑ni=1(xi−ˉx)2√∑ni=1(yi−ˉy)2 | (3.1) |
where ˉx and ˉy are the means of the samples within the two feature sets, respectively, and r takes the value [−1,1].
Given that strongly correlated features typically yield more information about the relationships between data, the selection of such features can provide data with higher information content, thereby enhancing the reliability and predictive capability of experiments.
(2) Static factors extraction and enhance
Since the values of the static factors do not change over time, we use correlation analysis to extract the p static factors that are different in time but have a strong correlation to form the matrix S. Specifically, the matrix after fusing the static factors at time t is
Cts=[Xt,S],Cts∈Rn×(p+l) | (3.2) |
(3) Dynamic factors extraction and enhance
Considering that the dynamics factors will be affected with time, we use the method of correlation analysis to extract m+1 time slices with strong correlation from the continuous time series, i.e., we select Dt−m,tw=[Dt−mw,Dt−m−1w,…,Dtw] as the dynamic factors Dw for each submatrix.
Finally, through the incorporation of relevant attribute enhancement units, we create an enhancement matrix containing all external spatiotemporal factors and demand characteristic information at time t. This enhancement matrix minimizes the loss of feature information during model training, thereby enhancing the model's perceptiveness to various factors.
Ct=[Xt,S,Dt−m,t1,Dt−m,t2,…,Dt−m,tw] | (3.3) |
where Ct∈Rn×(p+l+w×(m+1)).
The demand for ride-hailing orders exhibits connectivity and fluidity between neighboring areas, resulting in mutual influence. In the transportation field, GCN is currently widely used [18], which can handle non-Euclidean spatial data and is very suitable for transportation data analysis and prediction tasks[9]. Therefore, we utilize GCNs to model the spatial relationships between different areas in the transportation network. Through graph convolution, GCNs can learn the connectivity patterns between different areas and the impact of external spatial factors, thereby enhancing model understanding and prediction of spatial features. The GCN model can be represented as
Ol+1=σ(˜D−12˜A˜D−12O(l)W(l)) | (3.4) |
where σ is the activation function, ˜A the adjacency matrix, ˜D the corresponding degree matrix, Wl the weight matrix of the lth convolutional layer, and O(l) the convolutional output of the lth layer. The architecture of the GCN model is shown in Figure 6.
In this study, we will use a 2-layer GCN model for training. The model can be represented as
f(X|H,G)=σ(ˆAReLu(ˆAXW0)W1) | (3.5) |
where ˆA=˜D−12˜A˜D−12.
Time dependency is also a vital issue in the prediction of demand. Currently, RNNs are a widely used method for processing time series data. However, during the backpropagation process, issues such as gradient vanishing or exploding can be encountered[63]. LSTM[64] and GRU[65] are two variants of RNNs, and they solve this problem nicely by introducing gating mechanisms. GRU replaces the forgetting gate and the input gate with an update gate on top of LSTM, which results in a smaller number of parameters and lower computational complexity, thus improving the training speed of GRU. So, we choose the GRU model to obtain the time dependence of the demand.
As shown in Figure 7, GRU consists of a combination of a reset gate and an update gate: rt denotes the reset gate, which determines how the candidate's hidden state at the current time step selectively ignores the information of the previous time step; ut denotes the update gate, which controls the degree of updating of the hidden state in the previous time step at the current time step; respective ct denotes the candidate hidden state of the current time step, which contains the intermediate state between the current input and the information of the previous time step; σ and tanh refer to the sigmoid and tanh activation functions; Ct denotes the characteristic information of the demand at the moment of t; and ht is the output state of the moment of t.
In the ride-hailing demand prediction model, GRU effectively captures the temporal dependencies in the time series data, such as hourly, daily, and weekly patterns, through its gating mechanism. This capability enables the model to capture the dynamic relationship between demand and external factors during training.
This section introduces the formation process of the EST-GCN unit.
As shown in Figure 8, taking the input at time t as an example, we represent the attributes related to dynamic factors as a continuum Dt−m,...,Dt−1,Dt, which includes time periodicity and weather conditions. Meanwhile, p attributes related to the target variable are extracted from static factors, denoted as s1,...,sp−1,sp. These static factors include POI information and date attributes. Subsequently, one-hot encoding is applied to these attribute values, transforming descriptive variables into continuous variable values, thereby reducing training errors.
Integrate external attributes with the continuously relevant historical demand quantities Xt−m,...,Xt−2,Xt−1,Xt required at time t to obtain the related attribute enhancement unit Ct, and subsequently, we incrementally input the fused feature unit into the GRU to capture the temporal dependencies of ride-hailing demand features. The output of the GRU further serves as the input for the GCN, utilizing graph convolution operations to learn the spatial correlations of ride-hailing demand across different areas. The objective of this process is to systematically capture spatiotemporal features through the training of GRU and GCN. Ultimately, we attain accurate prediction results, integrating considerations of ride-hailing demand features in both temporal and spatial dimensions.
The specific calculation process is shown below.
ut=σ(Wu[Ct,ht−1]+bu) | (3.6) |
rt=σ(Wr[Ct,ht−1]+br) | (3.7) |
ct=tanh(Wc[Ct,(rt∗ht−1)]+bc) | (3.8) |
ht=ut∗ht−1+(1−ut)∗ct | (3.9) |
^xt=gc[A,Yt] | (3.10) |
where gc denotes the graph convolution process, and W and b represent the weights and biases in the training process, respectively.
During the training process, the goal is to minimize the error between the actual regional demand and the prediction. We add the L2 regularization to adjust the loss function, which helps to avoid the overfitting problem. The loss function of the model can be expressed as
Loss=||Xt−^Xt||+λn∑i=1(Xt−^Xt)2 | (3.11) |
where Xt is the actual demand, ^Xt is the prediction demand, and λ is the hyperparameter.
To validate the effectiveness of the EST-GCN model, we opted for a real dataset from Chengdu's ride-hailing operations. This dataset covers two complete temporal cycles extensively and features detailed field content, making it well-suited for experimentation.
● MeiC Taxi: This dataset contains information on ride-hailing in Chengdu from June 3rd to June 17th, 2023, covering two weeks, to mine the impact of cyclicality on future demand. We count demand at five-minute intervals, i.e., we record demand every five minutes, totaling 730,000 pieces of total demand data.
● Areas: Each hexagonal area of the division is 0.7373 square kilometers. The 169 crucial areas within the Chengdu city bypass are selected, and each area is regarded as a vertex of the graph, constituting a adjacency matrix.
● Weather: This data was obtained from the Weather Query API (https://lbs.amap.com/api/webservice/guide/api/weatherinfo/), which obtains real-time weather conditions in the study areas every five minutes. The weather data contains weather conditions from June 3rd to June 17th, 2023.
● POIs: This dataset is the POI distribution information within the selected study area obtained through the API (https://lbs.amap.com/api/webservice/guide/api/search). When selecting POIs, we chose six indicators based on travel demand and study purpose: life, healthcare, tourism, transportation, residential, and companies and enterprises.
● Time Attribute: This dataset contains weekday, non-workday, and holiday attributes from June 3rd to June 17th, 2023, in Chengdu.
In this paper, we compare the proposed EST-GCN with the widely used temporal prediction baseline models:
● HA [29]: Predicting future demand based on the average demand from a past period;
● Autoregressive Integral Moving Average Model (ARIMA)[66]: Analyzing trends, seasonality, and randomness in demand data to predict future demand;
● SVR [67]: Mapping input demand features to continuous output values;
● GCN[18]: Learning graph network information through convolution operations to predict demand;
● Gated Recycling Unit Model (GRU)[16]: Predicting demand by learning the temporal dependencies of demand using temporal convolution;
● Spatio-Temporal Graph Convolutional Model (ST-GCN)[61]: An extension to GCN, specialized for processing graph data with a temporal dimension;
● Spatio-Temporal Attention Network (ST-GAT)[46]: Combines graph neural networks and attention mechanisms for learning representations and relationships of nodes in spatiotemporal graph data.
● Coupled Layer-wise Graph Convolution (CCRNN)[68]: A GCN with a layered coupling mechanism.
We trained using the same hyperparameters in the original paper for the above baseline model.
To validate the EST-GCN model's capability in perceiving external spatiotemporal factors, we have selected the following four criteria for evaluation:
1. Root Mean Squared Error (RMSE). To measure the deviation between the predicted demand values and the actual values; a smaller value indicates higher accuracy.
RMSE=√1TNT∑t=1N∑i=1(xti−^xti)2 | (4.1) |
2. Mean Absolute Error (MAE). Calculate the mean of the absolute error between the predicted demand values and the actual values; a smaller value indicates higher accuracy.
MAE=1TNT∑t=1N∑i=1|xti−^xti| | (4.2) |
3. Coefficient of Determination (R2). The model's explanatory power regarding the variability of actual values, with a range from 0 to 1; a value closer to 1 indicates a better model fit.
R2=1−T∑t=1N∑i=1(xti−^xti)2T∑t=1N∑i=1(xti−ˉx)2 | (4.3) |
4. Explained variance score (var). To measure the average deviation squared between the actual data and its mean; a larger value indicates a higher degree of data dispersion.
var=1NN∑i=1(xti−¯xti)2 | (4.4) |
where xti and ^xti denote the tth time period real demand and prediction demand in the ith area.
During the model training, the EST-GCN model requires setting parameters, including the training set ratio, learning rate, number of training epochs, and batch size. In the spatiotemporal dependency extraction stage, we construct a stacked pattern with two layers of GRU and GCN. The GRU model is configured with 32 hidden states, and the GCN model is configured with 64 hidden units. We perform grid search to select the optimal parameters.
In our experiments, to assess the impact of the number of training sessions on the model's performance, the results of each training session are recorded, as shown in the training results in Figure 9, where the horizontal axis represents the number of training sessions. The vertical axis represents the changes of different metrics. Figure 9(a) shows the trend of RMSE and MAE as the number of training times increases. Figure 9(b) shows the variation of R2 and Var for different training times. The prediction results are better when the training number is set to 70.
This experiment tested the performance of EST-GCN with other baseline methods in 15, 30, and 45-minute prediction tasks, and the performance comparison is shown in Table 1, where * denotes a negative number, which indicates that the model is less effective in prediction. It can be seen that our EST-GCN model outperforms other baseline models in the prediction performance of almost all evaluation indicators, demonstrating the effectiveness of external factors in predicting ride-hailing.
Model | T(15min/30min/45min) | |||
RMSE | MAE | R2 | Var | |
HA | 9.44 | 5.76 | 0.65 | 0.65 |
SVR | 7.88/9.12/11.32 | 4.54/5.66/6.94 | 0.81/0.81/0.80 | 0.81/0.81/0.80 |
ARIMA | 8.77/9.71/10.56 | 6.39/6.82/7.02 | ∗ | 0.0012/0.0035/0.0033 |
GRU | 6.35/6.52/6.87 | 4.12/4.43/4.75 | 0.83/0.81/0.80 | 0.83/0.81/0.80 |
GCN | 7.32/7.65/8.56 | 5.22/5.68/6.33 | 0.65/0.65/0.65 | 0.65/0.65/0.65 |
ST-GCN | 6.15/6.29/6.55 | 3.85/3.90/4.01 | 0.85/0.84/0.83 | 0.85/0.84/0.83 |
ST-GAT | 6.09/6.21/6.52 | 3.78/3.89/3.96 | 0.86/0.84/0.83 | 0.86/0.84/0.83 |
CCRNN | 6.01/6.15/6.50 | 3.75/3.85/3.95 | 0.86/0.84/0.83 | 0.86/0.84/0.83 |
EST-GCN | 5.93/6.10/6.39 | 3.72/3.81/3.93 | 0.86/0.84/0.83 | 0.86/0.84/0.83 |
(1) Excellent Prediction Performance. Methods based on deep learning neural networks have achieved remarkable predictive accuracy by modeling spatiotemporal features. In comparison to HA, SVR, and ARIMA models, the EST-GCN model consistently exhibits the best RMSE performance across different time horizons, reducing RMSE errors by 37.2, 24.7, and 32.4%, respectively. Compared to GRU and GCN models, the EST-GCN model, leveraging the strengths of both, reduces RMSE errors by 6.6 and 19%, respectively. While ensemble models like ST-GCN, ST-GAT, and CCRNN demonstrate exceptional performance in transportation domain predictions, they do not account for the influence of external spatiotemporal factors. EST-GCN, by integrating features of external spatiotemporal factors, reduces RMSE errors by 3.5, 2.6, and 1.3%, respectively, compared to the ensemble models.
(2) Effective External Spatiotemporal Factors. To validate the impact of external spatiotemporal factors on ride-hailing demand, we compared the EST-GCN model with the ST-GAT and CCRNN models. As shown in Figure 10, taking a 15-minute ridesharing demand prediction as an example, compared to models that do not consider external spatiotemporal factors, the RMSE errors were reduced by 2.6 and 1.3%, respectively.
(3) Predictive Capability across Different Time Horizons. For various prediction ranges (15 minutes, 30 minutes, and 45 minutes), EST-GCN demonstrates superior performance. In the 15-minute prediction range, the EST-GCN model reduces RMSE errors by 2.6 and 1.3% compared to the ST-GAT and CCRNN models, respectively. Within the 30-minute prediction range, the EST-GCN model exhibits RMSE errors 1.7 and 1.0% lower than those of the ST-GAT and CCRNN models, respectively. In the 45-minute prediction range, the EST-GCN model achieves RMSE errors 2.0 and 1.6% lower than those of the ST-GAT and CCRNN models, respectively.
These results have had a significant impact on the application of EST-GCN in predicting ride-hailing demand. First, EST-GCN demonstrates outstanding predictive performance across different time horizons, indicating its reliability in addressing short-term and long-term demand variations. This provides ride-hailing platforms with more flexible and accurate demand predictions, contributing to the optimization of resource allocation and improvement of service efficiency. Second, EST-GCN, by effectively capturing external spatiotemporal factors, better adapts to the complex changes in ride-hailing demand. This underscores the model's sensitivity to environmental and external factors, enabling it to maintain robustness when dealing with dynamic urban changes and special events. This is crucial for ride-hailing platforms to offer reliable services in complex urban environments.
In this experiment, to verify the degree of influence of external correlation factors in ride-hailing demand prediction, an ablation experiment is set up to compare the interpretation. The external spatiotemporal factors in the experiment include weather condition information, relevant POI information, and date attribute information. The experimental results are shown in Table 2.
Model | Attributes | RMSE | MAE | R2 | Var |
EST-GCN | Weather | 5.96 | 3.74 | 0.85 | 0.85 |
POIs | 5.99 | 3.77 | 0.84 | 0.84 | |
Date | 6.02 | 3.79 | 0.84 | 0.84 | |
Weather+POIs | 5.94 | 3.73 | 0.85 | 0.85 | |
Weather+Date | 5.94 | 3.73 | 0.85 | 0.85 | |
POIs+Date | 5.96 | 3.74 | 0.85 | 0.85 | |
Weather+POIs+Date | 5.93 | 3.72 | 0.86 | 0.86 | |
STGCN | None | 6.15 | 3.85 | 0.85 | 0.85 |
The experimental comparison shows that the model works best when introducing a single factor with weather condition information, indicating that weather conditions affect demand more than date attributes and POIs. In addition, when multiple external factors are introduced, the model's performance is better than the performance of the model when only a single external factor is introduced. Specifically, with the addition of single-factor information, the RMSE errors of the EST-GCN model are reduced by 3.0, 2.6, and 2.1%, respectively, compared to the ST-GCN model. Considering multiple external factor information, including weather conditions with POI information (Weather+POIs), weather with date attributes (Weather+Date), POI information with date attributes (POIs+Date), and weather conditions with POI information and date attributes (Weather+POIs+Date), the EST-GCN model reduced the RMSE error by 3.4, 3.4, 3.0, and 3.5%, respectively, compared to the ST-GCN model.
In summary, the experimental results show that external spatiotemporal factors are effective in improving the accuracy of the demand prediction task. Both a single factor and a combination of factors can significantly improve the performance of prediction models.
In this study, we conducted portability experiments on the EST-GCN model to verify its generalization ability. We chose four different geographic regions, as shown in Figure 11, with (a)–(d) as the experimental areas. These experiments aimed to evaluate the prediction ability of the EST-GCN model in new and unseen geographic areas. We select a dataset and parameters consistent with the model training. To ensure portability, we apply a transfer learning strategy by training the model in one area and then transferring it to another. This facilitates the model in converging more rapidly in the new area. In the model design, we also incorporate data standardization and adaptive adjacency matrix to enhance its adaptability to diverse environments and datasets.
The prediction result indicators are shown in Table 3. According to the data in the table, we can notice that the prediction errors of the EST-GCN model are very similar in the four experimental areas. The result proves that the EST-GCN model has a strong generalization ability in dealing with the spatially heterogeneous features of ride-hailing demand, which implies good adaptability and transferability in different areas.
Experimental area | RMSE | MAE |
Original study area (a) | 5.9312 | 3.7235 |
Experimental area (b) | 5.9335 | 3.7240 |
Experimental area (c) | 5.9302 | 3.7231 |
Experimental area (d) | 5.9289 | 3.7203 |
(1) Prediction capacity analysis.
To comprehensively evaluate the model's prediction ability, we choose the commercial areas in Chengdu City where the dataset is concentrated in human flow and visualize the actual demand values of the test set with the prediction results of the EST-GCN model. The results of the demand prediction for the next 15, 30, and 45 minutes are shown in Figure 12. The above graph shows the prediction results from June 15th, 2023 to June 17th, 2023. From the visualization results, we can draw the following conclusions:
1. For different prediction ranges, we find that the predictions of the EST-GCN model are consistent with the overall trend of the actual values, but the predictions are poor at the local extremes. This phenomenon is hypothesized to be due to the presence of unexpected events and the randomness of crowd movement, in addition to the external correlates considered in this study, which lead to unpredictable fluctuations in demand.
2. Short-term prediction is closer to the actual value. Because the EST-GCN model is more likely to capture short-term trends, as the prediction range increases the influence of external relevant factors may become complex and unstable, leading to relatively poorer prediction of the model in long-term prediction.
(2) Effect of external spatial and temporal factors. To deeply analyze the effect of external spatiotemporal factors, we chose data from the commercial area with the concentrated human flow in the ride-hailing dataset of Chengdu City to conduct the ablation experiment. The dataset contains information on different date attributes and weather conditions. We worked on demand prediction for different experimental conditions and visualized the prediction results as shown below Figures 13–15:
1. The external dynamic-related factor (weather) significantly improves the prediction effect of ride-hailing prediction at the peak and turning point. Especially in the early morning of June 17th, under heavy rainy weather conditions, the prediction effect of adding the weather condition information is shown in Figure 15, and its prediction value is closer to the actual value compared to adding the information of other factors.
2. External static factors, including date attributes and POI information, contribute significantly to the prediction accuracy of ride-hailing. In particular, the effect of date attributes is more significant during the transition between weekdays and days off.
3. The EST-GCN model can capture the various factors affecting ride-hailing more comprehensively by adding multiple external factors, including weather conditions, POI information, and date attributes, making the prediction model more flexible and adaptive, thus achieving better performance in the prediction task.
4. In different environments, the model's performance may excessively rely on external factors, potentially leading to a decrease in prediction accuracy in certain scenarios. For example, if the model relies heavily on POI information (such as popular events at specific locations), its predictive capability may be compromised in unconventional circumstances.
To address the problem that traditional ride-hailing prediction models do not comprehensively consider external spatiotemporal factors, we introduce the EST-GCN model for modeling the dependence of external spatiotemporal factors in ride-hailing prediction. We coded demand in each area with relevant external spatial and temporal factors to form area characterization units. Combining GCN and GRU models, spatiotemporal information is extracted from the feature units in different areas to explore the potential relationship between external spatiotemporal factors and demand. We conducted experiments on the Chengdu City operations dataset. The experimental results show that the spatiotemporal graph convolution model incorporating external factors can better adapt to changes in the external environment, and the overall prediction effect is better than that of the advanced baseline method, which proves the importance of external spatiotemporal factors in ride-hailing prediction. The model is essential for improving urban transportation systems' efficiency and intelligent scheduling. By accurately predicting ride-hailing demand and integrating external spatiotemporal factors, the model can assist ride-hailing companies in optimizing vehicle scheduling, improving operational efficiency, and reducing passenger wait times. Additionally, the model's insights into spatiotemporal dependencies can promote more effective urban traffic management, potentially reducing congestion and enhancing city resource allocation.
As future work, our planned research includes (1) considering using more external spatiotemporal information data to evaluate the model, (2) optimizing rules for dividing areas, and (3) applying this model to other cities to validate its applicability and effectiveness in different urban environments, thereby expanding the model's scope of application.
The authors declare they have not used Artificial Intelligence (AI) tools in the creation of this article.
This work was supported by the Scientific Research and Innovation Team Program of Sichuan University of Science and Technology under Grant SUSE652A006, the Key Research Base of Intelligent Tourism in Sichuan Province under Grant No.ZHZJ22-02 and No.ZHYR23-03, and the Graduate Innovation Fund of Sichuan University of Science and Engineering under Grant No.Y2023109 and the Key Laboratory of Philosophy and Social Sciences of Sichuan Province – Key Laboratory of Liquor Intelligent Management and Ecological Decision Optimization in the Upper Reaches of Yangtze River under Grant No.zdsy-12. This study was supported by the computational support provided by the High Performance Computing Center, School of Computer Science and Engineering, Sichuan University of Science and Engineering.
The authors declare there is no conflict of interest.
[1] |
Azeem MK, Islam A, Khan RU, et al. (2023) Eco-friendly three-dimensional hydrogels for sustainable agricultural applications: Current and future scenarios. Polym Adv Technol 34: 3046–3062. https://doi.org/10.1002/pat.6122 doi: 10.1002/pat.6122
![]() |
[2] |
Hou Y, Ma S, Hao J, et al. (2022) Construction and ion transport-related applications of the hydrogel-based membrane with 3D nanochannels. Polymers 14: 4037. https://doi.org/10.3390/polym14194037 doi: 10.3390/polym14194037
![]() |
[3] |
Matole V, Digge P (2022) A brief review on hydrogel. RJTCS 13: 99–100. https://doi.org/10.52711/2321-5844.2022.00016 doi: 10.52711/2321-5844.2022.00016
![]() |
[4] |
Ghandforoushan P, Alehosseini M, Golafshan N, et al. (2023) Injectable hydrogels for cartilage and bone tissue regeneration: A review. Int J Biol Macromol 246: 125674. https://doi.org/10.1016/j.ijbiomac.2023.125674 doi: 10.1016/j.ijbiomac.2023.125674
![]() |
[5] |
Tang Y, Wang H, Liu S, et al. (2022) A review of protein hydrogels: Protein assembly mechanisms, properties, and biological applications. Colloid Surface B 220: 112973. https://doi.org/10.1016/j.colsurfb.2022.112973 doi: 10.1016/j.colsurfb.2022.112973
![]() |
[6] |
Almajidi YQ, Gupta J, Sheri FS, et al. (2023) Advances in chitosan-based hydrogels for pharmaceutical and biomedical applications: A comprehensive review. Int J Biol Macromol 253: 127278. https://doi.org/10.1016/j.ijbiomac.2023.127278 doi: 10.1016/j.ijbiomac.2023.127278
![]() |
[7] |
Mahfoudhi N, Boufi S (2016) Poly (acrylic acid-co-acrylamide)/cellulose nanofibrils nanocomposite hydrogels: Effects of CNFs content on the hydrogel properties. Cellulose 23: 3691–3701. https://doi.org/10.1007/s10570-016-1074-z doi: 10.1007/s10570-016-1074-z
![]() |
[8] |
Chen F, Zhou D, Wang J, et al. (2018) Rational fabrication of anti-freezing, non-drying tough organohydrogels by one-pot solvent displacement. Angew Chem Int Ed 57: 6568–6571. https://doi.org/10.1002/anie.201803366 doi: 10.1002/anie.201803366
![]() |
[9] |
Sun K, Cui S, Gao X, et al. (2023) Graphene oxide assisted triple network hydrogel electrolyte with high mechanical and temperature stability for self-healing supercapacitor. J Energy Storage 61: 106658. https://doi.org/10.1016/j.est.2023.106658 doi: 10.1016/j.est.2023.106658
![]() |
[10] |
Bin Asghar Abbasi B, Gigliotti M, Aloko S, et al. (2023) Designing strong, fast, high-performance hydrogel actuators. Chem Commun 59: 7141–7150. https://doi.org/10.1039/D3CC01545A doi: 10.1039/D3CC01545A
![]() |
[11] |
Wei Z, Gerecht S (2018) A self-healing hydrogel as an injectable instructive carrier for cellular morphogenesis. Biomaterials 185: 86–96. https://doi.org/10.1016/j.biomaterials.2018.09.003 doi: 10.1016/j.biomaterials.2018.09.003
![]() |
[12] |
Ran C, Wang J, He Y, et al. (2022) Recent advances in bioinspired hydrogels with environment-responsive characteristics for biomedical applications. Macromol Biosci 22: 2100474. https://doi.org/10.1002/mabi.202100474 doi: 10.1002/mabi.202100474
![]() |
[13] |
Sokolov P, Samokhvalov P, Sukhanova A, et al. (2023) Biosensors based on inorganic composite fluorescent hydrogels. Nanomaterials 13: 1748. https://doi.org/10.3390/nano13111748 doi: 10.3390/nano13111748
![]() |
[14] |
Su M, Ruan L, Dong X, et al. (2022) Current state of knowledge on intelligent-response biological and other macromolecular hydrogels in biomedical engineering: A review. Int J Biol Macromol 227: 472–492. https://doi.org/10.1016/j.ijbiomac.2022.12.148 doi: 10.1016/j.ijbiomac.2022.12.148
![]() |
[15] |
Li W, Liu J, Wei J, et al. (2023) Recent progress of conductive hydrogel fibers for flexible electronics: Fabrications, applications, and perspectives. Adv Funct Mater 33: 2213485. https://doi.org/10.1002/adfm.202213485 doi: 10.1002/adfm.202213485
![]() |
[16] |
Shuai L, Guo ZH, Zhang P, et al. (2020) Stretchable, self-healing, conductive hydrogel fibers for strain sensing and triboelectric energy-harvesting smart textiles. Nano Energy 78: 105389. https://doi.org/10.1016/j.nanoen.2020.105389 doi: 10.1016/j.nanoen.2020.105389
![]() |
[17] |
Wang XQ, Chan KH, Lu W, et al. (2022) Macromolecule conformational shaping for extreme mechanical programming of polymorphic hydrogel fibers. Nat Commun 13: 3369. https://doi.org/10.1038/s41467-022-31047-3 doi: 10.1038/s41467-022-31047-3
![]() |
[18] |
Štular D, Kruse M, Župunski V, et al. (2019) Smart stimuli-responsive polylactic acid-hydrogel fibers produced via electrospinning. Fiber Polym 20: 1857–1868. https://doi.org/10.1007/s12221-019-9157-8 doi: 10.1007/s12221-019-9157-8
![]() |
[19] |
Kim K, Choi JH, Shin M (2021) Mechanical stabilization of alginate hydrogel fiber and 3D constructs by mussel-inspired catechol modification. Polymers 13: 892. https://doi.org/10.3390/polym13060892 doi: 10.3390/polym13060892
![]() |
[20] |
Luo Y, Shoichet MS (2004) A photolabile hydrogel for guided three-dimensional cell growth and migration. Nat Mater 3: 249–253. https://doi.org/10.1038/nmat1092 doi: 10.1038/nmat1092
![]() |
[21] |
Du J, Ma Q, Wang B, et al. (2023) Hydrogel fibers for wearable sensors and soft actuators. iScience 26: 106796. https://doi.org/10.1016/j.isci.2023.106796 doi: 10.1016/j.isci.2023.106796
![]() |
[22] |
Sun W, Zhao X, Webb E, et al. (2023) Advances in metal-organic framework-based hydrogel materials: Preparation, properties and applications. J Mater Chem A 11: 2092–2127. https://doi.org/10.1039/D2TA08841J doi: 10.1039/D2TA08841J
![]() |
[23] |
Norahan MH, Pedroza-González SC, Sánchez-Salazar MG, et al. (2023) Structural and biological engineering of 3D hydrogels for wound healing. Bioact Mater 24: 197–235. https://doi.org/10.1016/j.bioactmat.2022.11.019 doi: 10.1016/j.bioactmat.2022.11.019
![]() |
[24] |
Li M, Chen X, Li X, et al. (2021) Wearable and robust polyimide hydrogel fiber textiles for strain sensors. ACS Appl Mater Interfaces 13: 43323–43332. https://doi.org/10.1021/acsami.1c10055 doi: 10.1021/acsami.1c10055
![]() |
[25] |
Yao B, Wang H, Zhou Q, et al. (2017) Ultrahigh-conductivity polymer hydrogels with arbitrary structures. Adv Mater 29: 1700974. https://doi.org/10.1002/adma.201700974 doi: 10.1002/adma.201700974
![]() |
[26] |
Rezapour Sarabi M, Jiang N, Ozturk E, et al. (2021) Biomedical optical fibers. Lab Chip 21: 627–640. https://doi.org/10.1039/D0LC01155J doi: 10.1039/D0LC01155J
![]() |
[27] |
Zaszczyńska A, Niemczyk-Soczynska B, Sajkiewicz P (2022) A comprehensive review of electrospun fibers, 3D-printed scaffolds, and hydrogels for cancer therapies. Polymers 14: 5278. https://doi.org/10.3390/polym14235278 doi: 10.3390/polym14235278
![]() |
[28] |
Mahaman YAR, Embaye KS, Huang F, et al. (2022) Biomarkers used in Alzheimer's disease diagnosis, treatment, and prevention. Ageing Res Rev 74: 101544. https://doi.org/10.1016/j.arr.2021.101544 doi: 10.1016/j.arr.2021.101544
![]() |
[29] |
Chen YH, Lou JG, Yang ZH, et al. (2022) Diagnosis, treatment, and prevention of severe acute hepatitis of unknown etiology in children. World J Pediatr 18: 538–544. https://doi.org/10.1007/s12519-022-00581-x doi: 10.1007/s12519-022-00581-x
![]() |
[30] |
Kher C, Kumar S (2022) The application of nanotechnology and nanomaterials in cancer diagnosis and treatment: A review. Cureus 14: 29059. https://doi.org/10.7759/cureus.29059 doi: 10.7759/cureus.29059
![]() |
[31] |
Xu W, Qing X, Liu S, et al. (2022) Hollow mesoporous manganese oxides: Application in cancer diagnosis and therapy. Small 18: 2106511. https://doi.org/10.1002/smll.202106511 doi: 10.1002/smll.202106511
![]() |
[32] |
Song J, Zhang Y, Chan SY, et al. (2021) Hydrogel-based flexible materials for diabetes diagnosis, treatment, and management. Npj Flex Electron 5: 26. https://doi.org/10.1038/s41528-021-00122-y doi: 10.1038/s41528-021-00122-y
![]() |
[33] |
Li Q, Cao Y, Wang P (2022) Recent advances in hydrogels for the diagnosis and treatment of dry eye disease. Gels 8: 816. https://doi.org/10.3390/gels8120816 doi: 10.3390/gels8120816
![]() |
[34] |
Zhu JQ, Wu H, Li ZL, et al. (2022) Responsive hydrogels based on triggered click reactions for liver cancer. Adv Mater 34: 2201651. https://doi.org/10.1002/adma.202201651 doi: 10.1002/adma.202201651
![]() |
[35] |
Ma Y, Huang J, Song S, et al. (2016) Cancer-targeted nanotheranostics: Recent advances and perspectives. Small 12: 4936–4954. https://doi.org/10.1002/smll.201600635 doi: 10.1002/smll.201600635
![]() |
[36] |
Tehrany PM, Rahmanian P, Rezaee A, et al. (2023) Multifunctional and theranostic hydrogels for wound healing acceleration: An emphasis on diabetic-related chronic wounds. Environ Res 238: 117087. https://doi.org/10.1016/j.envres.2023.117087 doi: 10.1016/j.envres.2023.117087
![]() |
[37] |
Gupta BD, Pathak A, Shrivastav AM (2022) Optical biomedical diagnostics using lab-on-fiber technology: A review. Photonics 9: 86. https://doi.org/10.3390/photonics9020086 doi: 10.3390/photonics9020086
![]() |
[38] |
Zhou C, Wu T, Xie X, et al. (2022) Advances and challenges in conductive hydrogels: From properties to applications. Eur Polym J 177: 111454. https://doi.org/10.1016/j.eurpolymj.2022.111454 doi: 10.1016/j.eurpolymj.2022.111454
![]() |
[39] |
Liu Y, Wang L, Mi Y, et al. (2022) Transparent stretchable hydrogel sensors: Materials, design and applications. J Mater Chem C 10: 13351–13371. https://doi.org/10.1039/D2TC01104B doi: 10.1039/D2TC01104B
![]() |
[40] |
Liu G, Chen X, Zhou W, et al. (2016) Preparation of a novel composite nanofiber gel‑encapsulated human placental extract through layer‑by‑layer self‑assembly. Exp Ther Med 11: 1447–1452. https://doi.org/10.3892/etm.2016.3084 doi: 10.3892/etm.2016.3084
![]() |
[41] |
Zhao Z, Fang R, Rong Q, et al. (2017) Bioinspired nanocomposite hydrogels with highly ordered structures. Adv Mater 29: 1703045. https://doi.org/10.1002/adma.201703045 doi: 10.1002/adma.201703045
![]() |
[42] |
Li J, Li S, Huang J, et al. (2022) Spider silk-inspired artificial fibers. Adv Sci 9: 2103965. https://doi.org/10.1002/advs.202103965 doi: 10.1002/advs.202103965
![]() |
[43] |
Zhang X, Wang X, Fan W, et al. (2022) Fabrication, property and application of calcium alginate fiber: A review. Polymers 14: 3227. https://doi.org/10.3390/polym14153227 doi: 10.3390/polym14153227
![]() |
[44] |
Yetisen AK, Jiang N, Fallahi A, et al. (2017) Glucose-sensitive hydrogel optical fibers functionalized with phenylboronic acid. Adv Mater 29: 1606380. https://doi.org/10.1002/adma.201606380 doi: 10.1002/adma.201606380
![]() |
[45] |
Choi M, Humar M, Kim S, et al. (2015) Step-index optical fiber made of biocompatible hydrogels. Adv Mater 27: 4081–4086. https://doi.org/10.1002/adma.201501603 doi: 10.1002/adma.201501603
![]() |
[46] |
Zhang S, Chen Y, Liu H, et al. (2020) Room-temperature-formed PEDOT:PSS hydrogels enable injectable, soft, and healable organic bioelectronics. Adv Mater 32: 1904752. https://doi.org/10.1002/adma.201904752 doi: 10.1002/adma.201904752
![]() |
[47] |
Li Y, Poon CT, Li M, et al. (2015) Chinese-noodle-inspired muscle myofiber fabrication. Adv Funct Mater 25: 5999–6008. https://doi.org/10.1002/adfm.201502018 doi: 10.1002/adfm.201502018
![]() |
[48] |
Dou Y, Wang ZP, He W, et al. (2019) Artificial spider silk from ion-doped and twisted core-sheath hydrogel fibres. Nat Commun 10: 5293. https://doi.org/10.1038/s41467-019-13257-4 doi: 10.1038/s41467-019-13257-4
![]() |
[49] |
Kim SH, Kim SH, Nair S, et al. (2005) Reactive electrospinning of cross-linked poly(2-hydroxyethyl methacrylate) nanofibers and elastic properties of individual hydrogel nanofibers in aqueous solutions. Macromolecules 38: 3719–3723. https://doi.org/10.1021/ma050308g doi: 10.1021/ma050308g
![]() |
[50] |
Jeong W, Kim J, Kim S, et al. (2004) Hydrodynamic microfabrication via "on the fly" photopolymerization of microscale fibers and tubes. Lab Chip 4: 576–580. https://doi.org/10.1039/B411249K doi: 10.1039/B411249K
![]() |
[51] |
Scotti A, Schulte MF, Lopez CG, et al. (2022) How softness matters in soft nanogels and nanogel assemblies. Chem Rev 122: 11675–11700. https://doi.org/10.1021/acs.chemrev.2c00035 doi: 10.1021/acs.chemrev.2c00035
![]() |
[52] |
Ko A, Liao C (2023) Hydrogel wound dressings for diabetic foot ulcer treatment: Status-quo, challenges, and future perspectives. BMEMat 1: e12037. https://doi.org/10.1002/bmm2.12037 doi: 10.1002/bmm2.12037
![]() |
[53] |
Li P, Jin Z, Peng L, et al. (2018) Stretchable all-gel-state fiber-shaped supercapacitors enabled by macromolecularly interconnected 3D graphene/nanostructured conductive polymer hydrogels. Adv Mater 30: 1800124. https://doi.org/10.1002/adma.201800124 doi: 10.1002/adma.201800124
![]() |
[54] |
Perazzo A, Nunes JK, Guido S, et al. (2017) Flow-induced gelation of microfiber suspensions. P Natl Acad Sci USA 114: E8557–E8564. https://doi.org/10.1073/pnas.1710927114 doi: 10.1073/pnas.1710927114
![]() |
[55] |
Kim J, Kang T, Kim H, et al. (2019) Preparation of PVA/PAA nanofibers containing thiol-modified silica particles by electrospinning as an eco-friendly Cu (Ⅱ) adsorbent. J Ind Eng Chem 77: 273–279. https://doi.org/10.1016/j.jiec.2019.04.048 doi: 10.1016/j.jiec.2019.04.048
![]() |
[56] |
Cui Q, Bell DJ, Rauer SB, et al. (2020) Wet-spinning of biocompatible core-shell polyelectrolyte complex fibers for tissue engineering. Adv Mater Interfaces 7: 2000849. https://doi.org/10.1002/admi.202000849 doi: 10.1002/admi.202000849
![]() |
[57] |
Jin Y, Liu C, Chai W, et al. (2017) Self-supporting nanoclay as internal scaffold material for direct printing of soft hydrogel composite structures in air. ACS Appl Mater Interfaces 9: 17456–17465. https://doi.org/10.1021/acsami.7b03613 doi: 10.1021/acsami.7b03613
![]() |
[58] |
Foudazi R, Zowada R, Manas-Zloczower I, et al. (2023) Porous hydrogels: Present challenges and future opportunities. Langmuir 39: 2092–2111. https://doi.org/10.1021/acs.langmuir.2c02253 doi: 10.1021/acs.langmuir.2c02253
![]() |
[59] |
Tamayol A, Najafabadi AH, Aliakbarian B, et al. (2015) Hydrogel templates for rapid manufacturing of bioactive fibers and 3D constructs. Adv Healthc Mater 4: 2146–2153. https://doi.org/10.1002/adhm.201500492 doi: 10.1002/adhm.201500492
![]() |
[60] |
Ding H, Wu Z, Wang H, et al. (2022) An ultrastretchable, high-performance, and crosstalk-free proximity and pressure bimodal sensor based on ionic hydrogel fibers for human-machine interfaces. Mater Horiz 9: 1935–1946. https://doi.org/10.1039/D2MH00281G doi: 10.1039/D2MH00281G
![]() |
[61] |
Yin T, Wu L, Wu T, et al. (2019) Ultrastretchable and conductive core/sheath hydrogel fibers with multifunctionality. J Polym Sci Part B: Polym Phys 57: 272–280. https://doi.org/10.1002/polb.24781 doi: 10.1002/polb.24781
![]() |
[62] |
Wang L, Zhong C, Ke D, et al. (2018) Ultrasoft and highly stretchable hydrogel optical fibers for in vivo optogenetic modulations. Adv Opt Mater 6: 1800427. https://doi.org/10.1002/adom.201800427 doi: 10.1002/adom.201800427
![]() |
[63] |
Cheng J, Jun Y, Qin J, et al. (2017) Electrospinning versus microfluidic spinning of functional fibers for biomedical applications. Biomaterials 114: 121–143. https://doi.org/10.1016/j.biomaterials.2016.10.040 doi: 10.1016/j.biomaterials.2016.10.040
![]() |
[64] |
Ahn SY, Mun CH, Lee SH (2015) Microfluidic spinning of fibrous alginate carrier having highly enhanced drug loading capability and delayed release profile. RSC Adv 5: 15172–15181. https://doi.org/10.1039/C4RA11438H doi: 10.1039/C4RA11438H
![]() |
[65] |
Zhou M, Gong J, Ma J (2019) Continuous fabrication of near-infrared light responsive bilayer hydrogel fibers based on microfluidic spinning. E-Polymers 19: 215–224. https://doi.org/10.1515/epoly-2019-0022 doi: 10.1515/epoly-2019-0022
![]() |
[66] |
Shi X, Ostrovidov S, Zhao Y, et al. (2015) Microfluidic spinning of cell-responsive grooved microfibers. Adv Funct Mater 25: 2250–2259. https://doi.org/10.1002/adfm.201404531 doi: 10.1002/adfm.201404531
![]() |
[67] | Kasper FK, Liao J, Kretlow JD, et al. (1979) Flow Perfusion Culture of Mesenchymal Stem Cells for Bone Tissue Engineering, Cambridge: Harvard Stem Cell Institute. https://doi.org/10.3824/stembook.1.18.1 |
[68] |
Gao Z, Xiao X, Carlo AD, et al. (2023) Advances in wearable strain sensors based on electrospun fibers. Adv Funct Mater 33: 2214265. https://doi.org/10.1002/adfm.202214265 doi: 10.1002/adfm.202214265
![]() |
[69] |
Chen C, Tang J, Gu Y, et al. (2019) Bioinspired hydrogel electrospun fibers for spinal cord regeneration. Adv Funct Mater 29: 1806899. https://doi.org/10.1002/adfm.201806899 doi: 10.1002/adfm.201806899
![]() |
[70] |
Im JS, Bai BC, In SJ, et al. (2010) Improved photodegradation properties and kinetic models of a solar-light-responsive photocatalyst when incorporated into electrospun hydrogel fibers. J Colloid Interf Sci 346: 216–221. https://doi.org/10.1016/j.jcis.2010.02.043 doi: 10.1016/j.jcis.2010.02.043
![]() |
[71] |
Han F, Zhang H, Zhao J, et al. (2012) In situ encapsulation of hydrogel in ultrafine fibers by suspension electrospinning. Polym Eng Sci 52: 2695–2704. https://doi.org/10.1002/pen.23227 doi: 10.1002/pen.23227
![]() |
[72] |
Mirabedini A, Foroughi J, Romeo T, et al. (2015) Development and characterization of novel hybrid hydrogel fibers. Macromol Mater Eng 300: 1217–1225. https://doi.org/10.1002/mame.201500152 doi: 10.1002/mame.201500152
![]() |
[73] |
Song JC, Chen S, Sun LJ, et al. (2020) Mechanically and electronically robust transparent organohydrogel fibers. Adv Mater 32: 1906994. https://doi.org/10.1002/adma.201906994 doi: 10.1002/adma.201906994
![]() |
[74] |
Nechyporchuk O, Yang Nilsson T, Ulmefors H, et al. (2020) Wet spinning of chitosan fibers: Effect of sodium dodecyl sulfate adsorption and enhanced dope temperature. ACS Appl Polym Mater 2: 3867–3875. https://doi.org/10.1021/acsapm.0c00562 doi: 10.1021/acsapm.0c00562
![]() |
[75] |
Konop AJ, Colby RH (1999) Polyelectrolyte charge effects on solution viscosity of poly(acrylic acid). Macromolecules 32: 2803–2805. https://doi.org/10.1021/ma9818174 doi: 10.1021/ma9818174
![]() |
[76] |
Li S, Biswas MC, Ford E (2022) Dual roles of sodium polyacrylate in alginate fiber wet-spinning: Modify the solution rheology and strengthen the fiber. Carbohyd Polym 297: 120001. https://doi.org/10.1016/j.carbpol.2022.120001 doi: 10.1016/j.carbpol.2022.120001
![]() |
[77] |
He Y, Zhang N, Gong Q, et al. (2012) Alginate/graphene oxide fibers with enhanced mechanical strength prepared by wet spinning. Carbohyd Polym 88: 1100–1108. https://doi.org/10.1016/j.carbpol.2012.01.071 doi: 10.1016/j.carbpol.2012.01.071
![]() |
[78] |
Zhang C, Xiao P, Zhang D, et al. (2023) Wet-spinning knittable hygroscopic organogel fibers toward moisture-capture-enabled multifunctional devices. Adv Fiber Mater 5: 588–602. https://doi.org/10.1007/s42765-022-00243-7 doi: 10.1007/s42765-022-00243-7
![]() |
[79] |
Sharma A, Ansari MZ, Cho C (2022) Ultrasensitive flexible wearable pressure/strain sensors: Parameters, materials, mechanisms and applications. Sensor Actuat A-Phys 347: 113934. https://doi.org/10.1016/j.sna.2022.113934 doi: 10.1016/j.sna.2022.113934
![]() |
[80] |
Nasiri S, Khosravani MR (2020) Progress and challenges in fabrication of wearable sensors for health monitoring. Sensor Actuat A-Phys 312: 112105. https://doi.org/10.1016/j.sna.2020.112105 doi: 10.1016/j.sna.2020.112105
![]() |
[81] |
Tian K, Bae J, Bakarich SE, et al. (2017) 3D printing of transparent and conductive heterogeneous hydrogel-elastomer systems. Adv Mater 29: 1604827. https://doi.org/10.1002/adma.201604827 doi: 10.1002/adma.201604827
![]() |
[82] |
Liu C, Wang Z, Wei X, et al. (2021) 3D printed hydrogel/PCL core/shell fiber scaffolds with NIR-triggered drug release for cancer therapy and wound healing. Acta Biomater 131: 314–325. https://doi.org/10.1016/j.actbio.2021.07.011 doi: 10.1016/j.actbio.2021.07.011
![]() |
[83] |
Zheng SY, Shen Y, Zhu F, et al. (2018) Programmed deformations of 3D-printed tough physical hydrogels with high response speed and large output force. Adv Funct Mater 28: 1803366. https://doi.org/10.1002/adfm.201803366 doi: 10.1002/adfm.201803366
![]() |
[84] |
Wu Y, Zhou S, Yi J, et al. (2022) Facile fabrication of flexible alginate/polyaniline/graphene hydrogel fibers for strain sensor. J Eng Fiber Fabr 17. https://doi.org/10.1177/15589250221114641 doi: 10.1177/15589250221114641
![]() |
[85] |
Guo J, Liu X, Jiang N, et al. (2016) Highly stretchable, strain sensing hydrogel optical fibers. Adv Mater 28: 10244–10249. https://doi.org/10.1002/adma.201603160 doi: 10.1002/adma.201603160
![]() |
[86] |
Shi W, Wang Z, Song H, et al. (2022) High-sensitivity and extreme environment-resistant sensors based on PEDOT:PSS@PVA hydrogel fibers for physiological monitoring. ACS Appl Mater Interfaces 14: 35114–35125. https://doi.org/10.1021/acsami.2c09556 doi: 10.1021/acsami.2c09556
![]() |
[87] |
Mian Z, Hermayer KL, Jenkins A (2019) Continuous glucose monitoring: Review of an innovation in diabetes management. Am J Med Sci 358: 332–339. https://doi.org/10.1016/j.amjms.2019.07.003 doi: 10.1016/j.amjms.2019.07.003
![]() |
[88] |
Xie Y, Lu L, Gao F, et al. (2021) Integration of artificial intelligence, blockchain, and wearable technology for chronic disease management: A new paradigm in smart healthcare. Curr Med Sci 41: 1123–1133. https://doi.org/10.1007/s11596-021-2485-0 doi: 10.1007/s11596-021-2485-0
![]() |
[89] |
Heo YJ, Shibata H, Okitsu T, et al. (2011) Long-term in vivo glucose monitoring using fluorescent hydrogel fibers. P Natl Acad Sci USA 108: 13399–13403. https://doi.org/10.1073/pnas.1104954108 doi: 10.1073/pnas.1104954108
![]() |
[90] | Heo YJ, Shibata H, Okitsu T, et al. (2011) Fluorescent hydrogel fibers for long-term in vivo glucose monitoring. 16th International Solid-State Sensors, Actuators and Microsystems Conference, Beijing, China, 2140–214. https://10.1109/TRANSDUCERS.2011.5969342 |
[91] |
Nunoi H, Xie P, Nakamura H, et al. (2022) Treatment with polyethylene glycol-conjugated fungal d-amino acid oxidase reduces lung inflammation in a mouse model of chronic granulomatous disease. Inflammation 45: 1668–1679. https://doi.org/10.1007/s10753-022-01650-z doi: 10.1007/s10753-022-01650-z
![]() |
[92] |
Besir Y, Karaagac E, Kurus M, et al. (2023) The effect of bovine serum albumin-glutaraldehyde and polyethylene glycol polymer on local tissue reaction and inflammation in rabbit carotid artery anastomosis. Vascular 31: 554–563. https://doi.org/10.1177/17085381221075484 doi: 10.1177/17085381221075484
![]() |
[93] |
Zdolsek J, Eaton JW, Tang L (2007) Histamine release and fibrinogen adsorption mediate acute inflammatory responses to biomaterial implants in humans. J Transl Med 5: 31. https://doi.org/10.1186/1479-5876-5-31 doi: 10.1186/1479-5876-5-31
![]() |
[94] |
Bosetti M, Zanardi L, Bracco P, et al. (2003) In vitro evaluation of the inflammatory activity of ultra-high molecular weight polyethylene. Biomaterials 24: 1419–1426. https://doi.org/10.1016/S0142-9612(02)00526-4 doi: 10.1016/S0142-9612(02)00526-4
![]() |
[95] |
Elsherif M, Hassan MU, Yetisen AK, et al. (2019) Hydrogel optical fibers for continuous glucose monitoring. Biosens Bioelectron 137: 25–32. https://doi.org/10.1016/j.bios.2019.05.002 doi: 10.1016/j.bios.2019.05.002
![]() |
[96] |
De Fazio R, De Vittorio M, Visconti P (2021) Innovative IoT solutions and wearable sensing systems for monitoring human biophysical parameters: A review. Electronics 10: 1660. https://doi.org/10.3390/electronics10141660 doi: 10.3390/electronics10141660
![]() |
[97] |
Seshadri DR, Li RT, Voos JE, et al. (2019) Wearable sensors for monitoring the physiological and biochemical profile of the athlete. NPJ Digit Med 2: 72. https://doi.org/10.1038/s41746-019-0150-9 doi: 10.1038/s41746-019-0150-9
![]() |
[98] |
Bandodkar AJ, Jeang WJ, Ghaffari R, et al. (2019) Wearable sensors for biochemical sweat analysis. Annu Rev Anal Chem 12: 1–22. https://doi.org/10.1146/annurev-anchem-061318-114910 doi: 10.1146/annurev-anchem-061318-114910
![]() |
[99] |
Hanlon M, Anderson R (2009) Real-time gait event detection using wearable sensors. Gait Posture 30: 523–527. https://doi.org/10.1016/j.gaitpost.2009.07.128 doi: 10.1016/j.gaitpost.2009.07.128
![]() |
[100] |
Prasanth H, Caban M, Keller U, et al. (2021) Wearable sensor-based real-time gait detection: A systematic review. Sensors 21: 2727. https://doi.org/10.3390/s21082727 doi: 10.3390/s21082727
![]() |
[101] |
Wan F, Ping H, Wang W, et al. (2021) Hydroxyapatite-reinforced alginate fibers with bioinspired dually aligned architectures. Carbohyd Polym 267: 118167. https://doi.org/10.1016/j.carbpol.2021.118167 doi: 10.1016/j.carbpol.2021.118167
![]() |
[102] |
Duan X, Yu J, Zhu Y, et al. (2020) Large-scale spinning approach to engineering knittable hydrogel fiber for soft robots. ACS Nano 14: 14929–14938. https://doi.org/10.1021/acsnano.0c04382 doi: 10.1021/acsnano.0c04382
![]() |
[103] |
Song J, Chen S, Sun L, et al. (2020) Mechanically and electronically robust transparent organohydrogel fibers. Adv Mater 32: 1906994. https://doi.org/10.1002/adma.201906994 doi: 10.1002/adma.201906994
![]() |
[104] |
Hou K, Li Y, Liu Y, et al. (2017) Continuous fabrication of cellulose nanocrystal/poly(ethylene glycol) diacrylate hydrogel fiber from nanocomposite dispersion: Rheology, preparation and characterization. Polymer 123: 55–64. https://doi.org/10.1016/j.polymer.2017.06.034 doi: 10.1016/j.polymer.2017.06.034
![]() |
[105] |
Dzenis Y (2004) Spinning continuous fibers for nanotechnology. Science 304: 1917–1919. https://www.science.org/doi/10.1126/science.1099074 doi: 10.1126/science.1099074
![]() |
[106] |
Zhao L, Xu T, Wang B, et al. (2023) Continuous fabrication of robust ionogel fibers for ultrastable sensors via dynamic reactive spinning. Chem Eng J 455: 140796. https://doi.org/10.1016/j.cej.2022.140796 doi: 10.1016/j.cej.2022.140796
![]() |
[107] |
Pei M, Zhu D, Yang J, et al. (2023) Multi-crosslinked flexible nanocomposite hydrogel fibers with excellent strength and knittability. Eur Polym J 182: 111737. https://doi.org/10.1016/j.eurpolymj.2022.111737 doi: 10.1016/j.eurpolymj.2022.111737
![]() |
[108] |
Hua J, Liu C, Fei B, et al. (2022) Self-healable and super-tough double-network hydrogel fibers from dynamic acylhydrazone bonding and supramolecular interactions. Gels 8: 101. https://doi.org/10.3390/gels8020101 doi: 10.3390/gels8020101
![]() |
[109] |
Wang C, Zhai S, Yuan Z, et al. (2020) Drying graphene hydrogel fibers for capacitive energy storage. Carbon 164: 100–110. https://doi.org/10.1016/j.carbon.2020.03.053 doi: 10.1016/j.carbon.2020.03.053
![]() |
[110] |
Meng F, Ding Y (2011) Sub-micrometer-thick all-solid-state supercapacitors with high power and energy densities. Adv Mater 23: 4098–4102. https://doi.org/10.1002/adma.201101678 doi: 10.1002/adma.201101678
![]() |
[111] |
Yang B, Hao C, Wen F, et al. (2017) Flexible black-phosphorus nanoflake/carbon nanotube composite paper for high-performance all-solid-state supercapacitors. ACS Appl Mater Interfaces 9: 44478–44484. https://doi.org/10.1021/acsami.7b13572 doi: 10.1021/acsami.7b13572
![]() |
[112] |
Zhang X, Chen Y, Yan J, et al. (2020) Janus-faced film with dual function of conductivity and pseudo-capacitance for flexible supercapacitors with ultrahigh energy density. Chem Eng J 388: 124197. https://doi.org/10.1016/j.cej.2020.124197 doi: 10.1016/j.cej.2020.124197
![]() |
[113] |
Han X, Xiao G, Wang Y, et al. (2020) Design and fabrication of conductive polymer hydrogels and their applications in flexible supercapacitors. J Mater Chem A 8: 23059–23095. https://doi.org/10.1039/D0TA07468C doi: 10.1039/D0TA07468C
![]() |
[114] |
Huang H, Han L, Fu X, et al. (2021) A powder self-healable hydrogel electrolyte for flexible hybrid supercapacitors with high energy density and sustainability. Small 17: 2006807. https://doi.org/10.1002/smll.202006807 doi: 10.1002/smll.202006807
![]() |
[115] |
Jia R, Li L, Ai Y, et al. (2018) Self-healable wire-shaped supercapacitors with two twisted NiCo2O4 coated polyvinyl alcohol hydrogel fibers. Sci China Mater 61: 254–262. https://doi.org/10.1007/s40843-017-9177-5 doi: 10.1007/s40843-017-9177-5
![]() |
[116] |
Xu T, Yang D, Zhang S, et al. (2021) Antifreezing and stretchable all-gel-state supercapacitor with enhanced capacitances established by graphene/PEDOT-polyvinyl alcohol hydrogel fibers with dual networks. Carbon 171: 201–210. https://doi.org/10.1016/j.carbon.2020.08.071 doi: 10.1016/j.carbon.2020.08.071
![]() |
[117] |
Cao X, Jiang C, Sun N, et al. (2021) Recent progress in multifunctional hydrogel-based supercapacitors. J Sci-Adv Mater Dev 6: 338–350. https://doi.org/10.1016/j.jsamd.2021.06.002 doi: 10.1016/j.jsamd.2021.06.002
![]() |
[118] |
Liu J, Jia Y, Jiang Q, et al. (2018) Highly conductive hydrogel polymer fibers toward promising wearable thermoelectric energy harvesting. ACS Appl Mater Interfaces 10: 44033–44040. https://doi.org/10.1021/acsami.8b15332 doi: 10.1021/acsami.8b15332
![]() |
[119] |
Zhou M, Al-Furjan MSH, Zou J, et al. (2018) A review on heat and mechanical energy harvesting from human-principles, prototypes and perspectives. Renew Sust Energ Rev 82: 3582–3609. https://doi.org/10.1016/j.rser.2017.10.102 doi: 10.1016/j.rser.2017.10.102
![]() |
[120] |
Huang L, Lin S, Xu Z, et al. (2020) Fiber-based energy conversion devices for human-body energy harvesting. Adv Mater 32: 1902034. https://doi.org/10.1002/adma.201902034 doi: 10.1002/adma.201902034
![]() |
[121] |
Peng L, Liu Y, Huang J, et al. (2018) Microfluidic fabrication of highly stretchable and fast electro-responsive graphene oxide/polyacrylamide/alginate hydrogel fibers. Eur Polym J 103: 335–341. https://doi.org/10.1016/j.eurpolymj.2018.04.019 doi: 10.1016/j.eurpolymj.2018.04.019
![]() |
[122] |
Wang Y, Ding Y, Guo X, et al. (2019) Conductive polymers for stretchable supercapacitors. Nano Res 12: 1978–1987. https://doi.org/10.1007/s12274-019-2296-9 doi: 10.1007/s12274-019-2296-9
![]() |
[123] |
Zhao X, Chen F, Li Y, et al. (2018) Bioinspired ultra-stretchable and anti-freezing conductive hydrogel fibers with ordered and reversible polymer chain alignment. Nat Commun 9: 3579. https://doi.org/10.1038/s41467-018-05904-z doi: 10.1038/s41467-018-05904-z
![]() |
[124] |
Bai J, Liu D, Tian X, et al. (2022) Tissue-like organic electrochemical transistors. J Mater Chem C 10: 13303–13311. https://doi.org/10.1039/D2TC01530G doi: 10.1039/D2TC01530G
![]() |
[125] |
Kayser LV, Lipomi DJ (2019) Stretchable conductive polymers and composites based on PEDOT and PEDOT:PSS. Adv Mater 31: 1806133. https://doi.org/10.1002/adma.201806133 doi: 10.1002/adma.201806133
![]() |
[126] |
Tseghai GB, Mengistie DA, Malengier B, et al. (2020) PEDOT:PSS-based conductive textiles and their applications. Sensors 20: 1881. https://doi.org/10.3390/s20071881 doi: 10.3390/s20071881
![]() |
[127] |
Zhao P, Zhang R, Tong Y, et al. (2020) Strain-discriminable pressure/proximity sensing of transparent stretchable electronic skin based on PEDOT:PSS/SWCNT electrodes. ACS Appl Mater Interfaces 12: 55083–55093. https://doi.org/10.1021/acsami.0c16546 doi: 10.1021/acsami.0c16546
![]() |
[128] |
Li P, Wang Y, Gupta U, et al. (2019) Transparent soft robots for effective camouflage. Adv Funct Mater 29: 1901908. https://doi.org/10.1002/adfm.201901908 doi: 10.1002/adfm.201901908
![]() |
[129] |
Li Y, Wang J, Wang Y, et al. (2021) Advanced electrospun hydrogel fibers for wound healing. Compos Part B-Eng 223: 109101. https://doi.org/10.1016/j.compositesb.2021.109101 doi: 10.1016/j.compositesb.2021.109101
![]() |
[130] |
Neibert K, Gopishetty V, Grigoryev A, et al. (2012) Wound-healing with mechanically robust and biodegradable hydrogel fibers loaded with silver nanoparticles. Adv Healthc Mater 1: 621–630. https://doi.org/10.1002/adhm.201200075 doi: 10.1002/adhm.201200075
![]() |
[131] |
Peng J, Cheng Q (2017) High-performance nanocomposites inspired by nature. Adv Mater 29: 1702959. https://doi.org/10.1002/adma.201702959 doi: 10.1002/adma.201702959
![]() |
[132] |
Xia LW, Xie R, Ju XJ, et al. (2013) Nano-structured smart hydrogels with rapid response and high elasticity. Nat Commun 4: 2226. https://doi.org/10.1038/ncomms3226 doi: 10.1038/ncomms3226
![]() |
[133] |
Lan Z, Kar R, Chwatko M, et al. (2023) High porosity PEG-based hydrogel foams with self-tuning moisture balance as chronic wound dressings. J Biomed Mater Res A 111: 465–477. https://doi.org/10.1002/jbm.a.37498 doi: 10.1002/jbm.a.37498
![]() |
[134] |
Farahani M, Shafiee A (2021) Wound healing: From passive to smart dressings. Adv Healthc Mater 10: 2100477. https://doi.org/10.1002/adhm.202100477 doi: 10.1002/adhm.202100477
![]() |
[135] |
Zhang Q, Qian C, Xiao W, et al. (2019) Development of a visible light, cross-linked GelMA hydrogel containing decellularized human amniotic particles as a soft tissue replacement for oral mucosa repair. RSC Adv 9: 18344–18352. https://doi.org/10.1039/C9RA03009C doi: 10.1039/C9RA03009C
![]() |
[136] |
Raho R, Paladini F, Lombardi FA, et al. (2015) In-situ photo-assisted deposition of silver particles on hydrogel fibers for antibacterial applications. Mater Sci Eng C 55: 42–49. https://doi.org/10.1016/j.msec.2015.05.050 doi: 10.1016/j.msec.2015.05.050
![]() |
[137] |
Ji Y, Ghosh K, Li B, et al. (2006) Dual-syringe reactive electrospinning of cross-linked hyaluronic acid hydrogel nanofibers for tissue engineering applications. Macromol Biosci 6: 811–817. https://doi.org/10.1002/mabi.200600132 doi: 10.1002/mabi.200600132
![]() |
[138] |
Sun X, Lang Q, Zhang H, et al. (2017) Electrospun photocrosslinkable hydrogel fibrous scaffolds for rapid in vivo vascularized skin flap regeneration. Adv Funct Mater 27: 1604617. https://doi.org/10.1002/adfm.201604617 doi: 10.1002/adfm.201604617
![]() |
[139] |
Liu W, Bi W, Sun Y, et al. (2020) Biomimetic organic-inorganic hybrid hydrogel electrospinning periosteum for accelerating bone regeneration. Mater Sci Eng C 110: 110670. https://doi.org/10.1016/j.msec.2020.110670 doi: 10.1016/j.msec.2020.110670
![]() |
[140] |
Wei X, Liu C, Wang Z, et al. (2020) 3D printed core-shell hydrogel fiber scaffolds with NIR-triggered drug release for localized therapy of breast cancer. Int J Pharm 580: 119219. https://doi.org/10.1016/j.ijpharm.2020.119219 doi: 10.1016/j.ijpharm.2020.119219
![]() |
[141] |
Tsourdi E, Barthel A, Rietzsch H, et al. (2013) Current aspects in the pathophysiology and treatment of chronic wounds in diabetes mellitus. Biomed Res Int 2013: 385641. https://doi.org/10.1155/2013/385641 doi: 10.1155/2013/385641
![]() |
[142] |
Chen H, Jia P, Kang H, et al. (2016) Upregulating hif-1α by hydrogel nanofibrous scaffolds for rapidly recruiting angiogenesis relative cells in diabetic wound. Adv Healthc Mater 5: 907–918. https://doi.org/10.1002/adhm.201501018 doi: 10.1002/adhm.201501018
![]() |
[143] |
Zhou P, Zhou L, Zhu C, et al. (2016) Nanogel-electrospinning for controlling the release of water-soluble drugs. J Mater Chem B 4: 2171–2178. https://doi.org/10.1039/C6TB00023A doi: 10.1039/C6TB00023A
![]() |
[144] |
Zhao X, Sun X, Yildirimer L, et al. (2017) Cell infiltrative hydrogel fibrous scaffolds for accelerated wound healing. Acta Biomater 49: 66–77. https://doi.org/10.1016/j.actbio.2016.11.017 doi: 10.1016/j.actbio.2016.11.017
![]() |
[145] |
Wang H, Xu Z, Zhao M, et al. (2021) Advances of hydrogel dressings in diabetic wounds. Biomater Sci 9: 1530–1546. https://doi.org/10.1039/D0BM01747G doi: 10.1039/D0BM01747G
![]() |
[146] |
Lei H, Zhu C, Fan D (2020) Optimization of human-like collagen composite polysaccharide hydrogel dressing preparation using response surface for burn repair. Carbohyd Polym 239: 116249. https://doi.org/10.1016/j.carbpol.2020.116249 doi: 10.1016/j.carbpol.2020.116249
![]() |
[147] |
Kesharwani P, Bisht A, Alexander A, et al. (2021) Biomedical applications of hydrogels in drug delivery system: An update. J Drug Deliv Sci Tec 66: 102914. https://doi.org/10.1016/j.jddst.2021.102914 doi: 10.1016/j.jddst.2021.102914
![]() |
[148] |
Kamoun EA, Kenawy ERS, Chen X (2017) A review on polymeric hydrogel membranes for wound dressing applications: PVA-based hydrogel dressings. J Adv Res 8: 217–233. https://doi.org/10.1016/j.jare.2017.01.005 doi: 10.1016/j.jare.2017.01.005
![]() |
[149] |
Ahmad F, Mushtaq B, Butt FA, et al. (2021) Synthesis and characterization of nonwoven cotton-reinforced cellulose hydrogel for wound dressings. Polymers 13: 4098. https://doi.org/10.3390/polym13234098 doi: 10.3390/polym13234098
![]() |
[150] |
Wang W, Zeng Z, Xiang L, et al. (2021) Injectable self-healing hydrogel via biological environment-adaptive supramolecular assembly for gastric perforation healing. ACS Nano 15: 9913–9923. https://doi.org/10.1021/acsnano.1c01199 doi: 10.1021/acsnano.1c01199
![]() |
[151] |
Yao Y, Zhang A, Yuan C, et al. (2021) Recent trends on burn wound care: Hydrogel dressings and scaffolds. Biomater Sci 9: 4523–4540. https://doi.org/10.1039/D1BM00411E doi: 10.1039/D1BM00411E
![]() |
[152] |
Jiang S, Deng J, Jin Y, et al. (2023) Breathable, antifreezing, mechanically skin-like hydrogel textile wound dressings with dual antibacterial mechanisms. Bioact Mater 21: 313–323. https://doi.org/10.1016/j.bioactmat.2022.08.014 doi: 10.1016/j.bioactmat.2022.08.014
![]() |
[153] |
Liu J, Zhang C, Miao D, et al. (2018) Preparation and characterization of carboxymethylcellulose hydrogel fibers. J Eng Fiber Fabr 13. https://doi.org/10.1177/155892501801300302 doi: 10.1177/155892501801300302
![]() |
[154] |
Dong Y, Zheng Y, Zhang K, et al. (2020) Electrospun nanofibrous materials for wound healing. Adv Fiber Mater 2: 212–227. https://doi.org/10.1007/s42765-020-00034-y doi: 10.1007/s42765-020-00034-y
![]() |
1. | Jong Hyuk Park, Editorial: Artificial Intelligence-based Security Applications and Services for Smart Cities, 2024, 21, 1551-0018, 7012, 10.3934/mbe.2024307 |
Model | T(15min/30min/45min) | |||
RMSE | MAE | R2 | Var | |
HA | 9.44 | 5.76 | 0.65 | 0.65 |
SVR | 7.88/9.12/11.32 | 4.54/5.66/6.94 | 0.81/0.81/0.80 | 0.81/0.81/0.80 |
ARIMA | 8.77/9.71/10.56 | 6.39/6.82/7.02 | ∗ | 0.0012/0.0035/0.0033 |
GRU | 6.35/6.52/6.87 | 4.12/4.43/4.75 | 0.83/0.81/0.80 | 0.83/0.81/0.80 |
GCN | 7.32/7.65/8.56 | 5.22/5.68/6.33 | 0.65/0.65/0.65 | 0.65/0.65/0.65 |
ST-GCN | 6.15/6.29/6.55 | 3.85/3.90/4.01 | 0.85/0.84/0.83 | 0.85/0.84/0.83 |
ST-GAT | 6.09/6.21/6.52 | 3.78/3.89/3.96 | 0.86/0.84/0.83 | 0.86/0.84/0.83 |
CCRNN | 6.01/6.15/6.50 | 3.75/3.85/3.95 | 0.86/0.84/0.83 | 0.86/0.84/0.83 |
EST-GCN | 5.93/6.10/6.39 | 3.72/3.81/3.93 | 0.86/0.84/0.83 | 0.86/0.84/0.83 |
Model | Attributes | RMSE | MAE | R2 | Var |
EST-GCN | Weather | 5.96 | 3.74 | 0.85 | 0.85 |
POIs | 5.99 | 3.77 | 0.84 | 0.84 | |
Date | 6.02 | 3.79 | 0.84 | 0.84 | |
Weather+POIs | 5.94 | 3.73 | 0.85 | 0.85 | |
Weather+Date | 5.94 | 3.73 | 0.85 | 0.85 | |
POIs+Date | 5.96 | 3.74 | 0.85 | 0.85 | |
Weather+POIs+Date | 5.93 | 3.72 | 0.86 | 0.86 | |
STGCN | None | 6.15 | 3.85 | 0.85 | 0.85 |
Experimental area | RMSE | MAE |
Original study area (a) | 5.9312 | 3.7235 |
Experimental area (b) | 5.9335 | 3.7240 |
Experimental area (c) | 5.9302 | 3.7231 |
Experimental area (d) | 5.9289 | 3.7203 |
Model | T(15min/30min/45min) | |||
RMSE | MAE | R2 | Var | |
HA | 9.44 | 5.76 | 0.65 | 0.65 |
SVR | 7.88/9.12/11.32 | 4.54/5.66/6.94 | 0.81/0.81/0.80 | 0.81/0.81/0.80 |
ARIMA | 8.77/9.71/10.56 | 6.39/6.82/7.02 | ∗ | 0.0012/0.0035/0.0033 |
GRU | 6.35/6.52/6.87 | 4.12/4.43/4.75 | 0.83/0.81/0.80 | 0.83/0.81/0.80 |
GCN | 7.32/7.65/8.56 | 5.22/5.68/6.33 | 0.65/0.65/0.65 | 0.65/0.65/0.65 |
ST-GCN | 6.15/6.29/6.55 | 3.85/3.90/4.01 | 0.85/0.84/0.83 | 0.85/0.84/0.83 |
ST-GAT | 6.09/6.21/6.52 | 3.78/3.89/3.96 | 0.86/0.84/0.83 | 0.86/0.84/0.83 |
CCRNN | 6.01/6.15/6.50 | 3.75/3.85/3.95 | 0.86/0.84/0.83 | 0.86/0.84/0.83 |
EST-GCN | 5.93/6.10/6.39 | 3.72/3.81/3.93 | 0.86/0.84/0.83 | 0.86/0.84/0.83 |
Model | Attributes | RMSE | MAE | R2 | Var |
EST-GCN | Weather | 5.96 | 3.74 | 0.85 | 0.85 |
POIs | 5.99 | 3.77 | 0.84 | 0.84 | |
Date | 6.02 | 3.79 | 0.84 | 0.84 | |
Weather+POIs | 5.94 | 3.73 | 0.85 | 0.85 | |
Weather+Date | 5.94 | 3.73 | 0.85 | 0.85 | |
POIs+Date | 5.96 | 3.74 | 0.85 | 0.85 | |
Weather+POIs+Date | 5.93 | 3.72 | 0.86 | 0.86 | |
STGCN | None | 6.15 | 3.85 | 0.85 | 0.85 |
Experimental area | RMSE | MAE |
Original study area (a) | 5.9312 | 3.7235 |
Experimental area (b) | 5.9335 | 3.7240 |
Experimental area (c) | 5.9302 | 3.7231 |
Experimental area (d) | 5.9289 | 3.7203 |