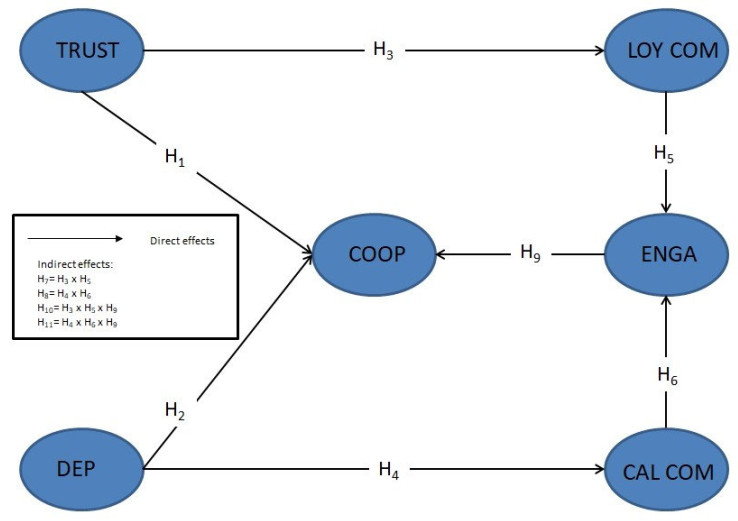
This research paper aims to demonstrate that relationships play a pivotal role in fostering inter-organizational cooperation, both at the corporate and individual levels, within the fast-moving consumer goods (FMCG) distribution industry. Despite the extensive body of literature on relationship management, its impact on such a unique and important channel as FMCG remains undetermined. To achieve this objective, we designed a questionnaire for a survey targeting Spanish FMCG distributors, from which we collected 204 responses. We analyzed this data using partial least squares structural equation modeling (PLS-SEM), an emerging methodology in business research that enables us to assess the model's explanatory power and predictive capacity in understanding the relationships between various constructs. Our findings indicate the efficacy of governing inter-organizational relationships to facilitate cooperation. Additionally, we uncover links between corporate and individual relationships that contribute significantly to cooperation. In terms of practical implications for businesses, our results emphasize the importance for managers to prioritize relationships as an integral aspect of efficient channel management. This pertains to relationships within the distribution channel among partners and also individual relationships among business managers. Notably, the existing academic literature has not yet established the effectiveness of relationships in governing channels within the FMCG marketing channel, nor has it established a direct connection between inter-organizational and individual relationships among business managers.
Citation: Fernando Gimeno-Arias, José Manuel Santos-Jaén, María del Carmen Valls Martínez, Manuel Sánchez-Pérez. From trust and dependence commitment to B2B engagement: An empirical analysis of inter-organizational cooperation in FMCG[J]. Electronic Research Archive, 2023, 31(12): 7511-7543. doi: 10.3934/era.2023379
[1] | Yanling Dong, Xiaolan Zhou . Advancements in AI-driven multilingual comprehension for social robot interactions: An extensive review. Electronic Research Archive, 2023, 31(11): 6600-6633. doi: 10.3934/era.2023334 |
[2] | Kun Han, Feng Jiang, Haiqi Zhu, Mengxuan Shao, Ruyu Yan . Learning cooperative strategies in StarCraft through role-based monotonic value function factorization. Electronic Research Archive, 2024, 32(2): 779-798. doi: 10.3934/era.2024037 |
[3] | Xiaoyang Xie, Shanghua Wen, Minglong Li, Yong Yang, Songru Zhang, Zhiwei Chen, Xiaoke Zhang, Hongyan Dui . Resilience evaluation and optimization for an air-ground cooperative network. Electronic Research Archive, 2024, 32(5): 3316-3333. doi: 10.3934/era.2024153 |
[4] | Hao Wen, Yantao Luo, Jianhua Huang, Yuhong Li . Stochastic travelling wave solution of the N-species cooperative systems with multiplicative noise. Electronic Research Archive, 2023, 31(8): 4406-4426. doi: 10.3934/era.2023225 |
[5] | Zhiqiang Yuan, Xi Deng, Tianzi Ding, Jing Liu, Qi Tan . Factors influencing secondary school teachers' usage behavior of dynamic mathematics software: A partial least squares structural equation modeling (PLS-SEM) method. Electronic Research Archive, 2023, 31(9): 5649-5684. doi: 10.3934/era.2023287 |
[6] | Xuemin Fan, Wenjie Zhang, Lu Xu . Global dynamics of a predator-prey model with prey-taxis and hunting cooperation. Electronic Research Archive, 2025, 33(3): 1610-1632. doi: 10.3934/era.2025076 |
[7] | Yujia Xiang, Yuqi Jiao, Xin Wang, Ruizhi Yang . Dynamics of a delayed diffusive predator-prey model with Allee effect and nonlocal competition in prey and hunting cooperation in predator. Electronic Research Archive, 2023, 31(4): 2120-2138. doi: 10.3934/era.2023109 |
[8] | Yue Ma, Zhongfei Li . Robust portfolio choice with limited attention. Electronic Research Archive, 2023, 31(7): 3666-3687. doi: 10.3934/era.2023186 |
[9] | Ming Wei, Shaopeng Zhang, Bo Sun . Airport passenger flow, urban development and nearby airport capacity dynamic correlation: 2006-2019 time-series data analysis for Tianjin city, China. Electronic Research Archive, 2022, 30(12): 4447-4468. doi: 10.3934/era.2022226 |
[10] | Tian Hou, Yi Wang, Xizhuang Xie . Instability and bifurcation of a cooperative system with periodic coefficients. Electronic Research Archive, 2021, 29(5): 3069-3079. doi: 10.3934/era.2021026 |
This research paper aims to demonstrate that relationships play a pivotal role in fostering inter-organizational cooperation, both at the corporate and individual levels, within the fast-moving consumer goods (FMCG) distribution industry. Despite the extensive body of literature on relationship management, its impact on such a unique and important channel as FMCG remains undetermined. To achieve this objective, we designed a questionnaire for a survey targeting Spanish FMCG distributors, from which we collected 204 responses. We analyzed this data using partial least squares structural equation modeling (PLS-SEM), an emerging methodology in business research that enables us to assess the model's explanatory power and predictive capacity in understanding the relationships between various constructs. Our findings indicate the efficacy of governing inter-organizational relationships to facilitate cooperation. Additionally, we uncover links between corporate and individual relationships that contribute significantly to cooperation. In terms of practical implications for businesses, our results emphasize the importance for managers to prioritize relationships as an integral aspect of efficient channel management. This pertains to relationships within the distribution channel among partners and also individual relationships among business managers. Notably, the existing academic literature has not yet established the effectiveness of relationships in governing channels within the FMCG marketing channel, nor has it established a direct connection between inter-organizational and individual relationships among business managers.
In the study of cooperation and exchange within the business field, marketing relationships have been a recurrent and widely discussed topic in recent decades, often referred to as the "relational paradigm" [1,2]. Under this paradigm, several influential theories have emerged, including relational norms theory, commitment-trust theory and network [3,4,5,6,7]. However, scholars should not assume that their work in this field is complete. Some aspects of exchange relationships, such as their functioning in specific industries or the connection between inter-organizational and individual relationships, offer room for further exploration [7,8].
While empirical research in this area is abundant, there is a notable limitation. Research with empirical evidence has often overlooked crucial economic sectors, including the marketing channels of FMCG. This distribution channel is a key industry in developed economies, as shown by the fact that some of the most important companies in the world have based their success in this sector, such as Wal-Mart, Amazon, and Costco. In Spain FMCG industry represents 20% of the gross domestic product. However, the FMCG distribution channel has not received attention from the point of view of the importance of its business relationships that favor efficiency [9]. This oversight may be due to some authors who have argued that, in this context, relationships take a backseat to transactional considerations such as, economic efficiency, transaction costs, integration, pricing, multi-channel strategies and omni-channel approaches [7,10,11]. The distribution of FMCG has not received attention through empirical studies of the relationships in the channel [5,12,21,22,23,24,13,14,15,16,17,18,19,20].
On the other hand, the study of marketing relationships has primarily concentrated on the inter-organizational aspect [5,6,8,25], often disregarding the significance of individual relationships within the business environment. Individual relationships have typically been limited to the context of the final consumer (B2C: business-to-consumer) [26,27]. However, few studies have accorded individual relationships a prominent role in industrial business management (B2B: business-to-business) [28,29,30,31]. In this regard, we consider that the examination of the relationship between inter-organizational and individual relationships remains an unexplored field.
Given this backdrop, we have identified certain gaps in the literature, namely the absence of empirical investigations into inter-organizational relationships within the FMCG distribution channel and the role played by individual relationships managed by business partner relationship managers. Our research makes two significant contributions: 1) We present an empirical study of inter-organizational relationships in the Spanish FMCG distribution channel, exploring the role of these relationships in effective channel management and their impact on cooperation among channel business partners. 2) We establish connections between inter-organizational and individual relationships, elucidating how the management of marketing channel relationships leads to the emergence of individual relationships that foster cooperation among business partners. Notably, higher-quality individual relationships promote increased levels of cooperation and long-term collaboration [26,29].
These gaps in the literature prompt us to formulate the following research questions: 1) Can efficiency in the FMCG distribution channel be achieved through the management of inter-organizational relationships? 2) Can distribution channel organizations translate their corporate commitment into individual engagement among business managers? 3) What impact do inter-organizational and individual relationships within the FMCG sector have on cooperation? Through the answers to these questions, we address an important issue that remains unanswered to date: the role of relationships in the FMCG marketing channel. Relationships have been shown to be effective in distribution channels, but the FMCG channel has not been explored. To address these questions, we collected data from a sample of 204 Spanish FMCG distribution companies. The primary respondent in the selected sample is the purchasing manager, given their comprehensive perspective on both inter-organizational relationships (through their involvement in agreements) and their perception of individual relationships with other channel agents.
Data analysis was conducted using a structural equation model based on PLS-SEM for confirmatory and predictive purposes. PLS-SEM, in contrast to ordinary least squares (OLS) regression, canonical correlation, or covariance-based structural equation modeling (SEM), is an emerging tool in business research that instills a high level of confidence in research outcomes [32]. The robustness of software tools like SmartPls facilitates complex model estimation with minimal prerequisites and has demonstrated its effectiveness in scientific domains such as business, economics, and other social sciences [33]. This technique encompasses a structural model (depicting dependency relationships between independent and dependent variables) and a measurement model (representing relationships between constructs and their indicators) [34]. As a variance-based technique, it offers greater modeling flexibility and does not necessitate stringent parametric assumptions [35].
The subsequent sections of this research work are organized as follows. First, we provide a review of the relevant literature to develop the proposed hypotheses. We then describe the research design, data collection methods, scales used in the research, data analysis procedures, and present the results. Finally, we conclude with a discussion of the findings and their implications at both the academic and managerial levels and outline some limitations of the study and offer recommendations for future research.
The relational paradigm has been a foundational area of focus for marketing scholars for decades and is essential for comprehending business-to-business exchanges [5,14,16,21,25,36,37,38,39,40,41,42,43]. Cooperation stands out as a crucial element within the relationships among organizations in the distribution channel. Cooperation does not merely imply the absence of conflict; it also entails organizational interdependence, prompting parties to collaborate towards shared objectives [5,12,25,44,45,46,47].
Cooperation, derived from the Latin "co" (together) and "operari" (to work), denotes situations in which parties collaborate to achieve mutual goals [39]. In the context of inter-organizational relationships, we define cooperation as the "inclination of a party to engage in concerted/joint action with another party to achieve individual or joint goals" [12]. Cooperation thus serves as a linchpin for the long-term success of relationships between business partners [8,11,47,48]. Given the pivotal role of cooperation in fruitful business relationships, we must ask which factors within the relationship foster cooperation among distribution channel stakeholders? In response to this research question, the existing literature consistently highlights trust and dependence as the antecedents of cooperation among business partners [5,7,8,12,16,22,45].
Trust represents the relationship state with the highest affective component due to its nature as a psychosocial construct [15]. Trust is conceptualized as "existing when one party has confidence in an exchange partner's reliability and integrity" [5]. The literature reveals that trust is associated with observable qualities in the relationship, such as consistency, competence, honesty, fairness, responsibility, service, and benevolence [22,49]. Consequently, trust instills the belief that the parties involved will take actions conducive to positive outcomes while refraining from engaging in unexpected actions that could yield negative consequences for the relationship [50]. Building trust in inter-organizational relationships is a time-consuming process that may be challenging in the presence of an unsatisfactory business relationship [19]. Therefore, satisfaction with the business partner is implicit in the concept of trust. Nevertheless, once trust is established, it contributes to relationship durability by reducing uncertainty between parties and facilitating collaborative efforts. In this context, "once trust is established, firms learn that coordinated, joint efforts will lead to outcomes that exceed what the firm would achieve if it acted solely in its own best interests" [39].
These considerations lead us to postulate a positive causal pathway from trust to cooperation [14,39,51]. This implies that greater trust in the business partner will lead to greater cooperation between them so that both will perform better together in the channel. We formulate this relationship in the following hypothesis:
H1: Trust has a positive impact on cooperation in inter-organizational relationships.
On the other hand, dependence is another element of the interfirm relationship that fosters cooperation between organizations [5,7,12,16,45]. Business relationships also encompass a dimension of necessity, exemplified by dependence. Its significance lies in situations where one party brings something unique and irreplaceable, or extremely challenging to replace, to the relationship [5]. This need leads to a "lock-in" situation in the event of a hypothetical change of relationship partner on the part of the dependent party [52]. Mutual dependence or interdependence between parties can also result in such a situation [13].
Dependence within a relationship is a concept widely explored in academic literature, often converging on the idea of irreplaceability [5,7,13,45]. Cai et al. define dependence as the extent to which the results obtained from the partnership are rare, important, or highly valued. Two key aspects are emphasized here: the significance of the business partner and the availability of alternatives. In essence, dependence underscores that a business partner provides valuable resources for which few alternative sources exist [49].
The concept of dependence is rooted in the relationship between necessary resources and reliance, imbuing it with a strong economic component. This stands in contrast to the affective aspects of relationships, which create less dependence [14]. The economic dimension is further enriched by one of the fundamental antecedents of dependence—the "cost of termination of the relationship." This cost encompasses the expected losses resulting from the termination of the relationship, including the scarcity of comparable alternative partners, expenses related to dissolving the relationship, and the "costs of change" [5]. The notion of costs of change is a common assumption in relationship marketing literature, defined as the costs arising from the search for and initiation of an alternative relationship. Depending on whether these costs are manageable or not, they may lead to varying levels of dependence on the existing relationship [53].
The need for certain agents in the supply or distribution of others frequently leads to dependence within the relationship. This dependence typically revolves around three essential aspects: 1) the consideration of the number of viable alternatives available, 2) the extent to which distribution relies exclusively on the supply or intermediation of a particular agent, and 3) the potential for substitution without compromising competitive positioning in the market [53]. A lack of flexibility can reinforce the relationship by preserving the participation of the dependent agent and intensifying their interest in it. Consequently, "the buyer's anticipation of high switching costs gives rise to the buyer's interest in maintaining a quality relationship" [14].
In light of these considerations, it is apparent that a high-quality relationship necessitates cooperation among its constituents, leading us to posit the following hypothesis:
H2: Dependence has a positive effect on cooperation in inter-organizational relationships.
In the field of inter-organizational relationships, particularly within marketing channels, commitment has been a subject of extensive academic study [5,8,17,20,36]. This is primarily due to its role as the most advanced stage of a relationship, fostering stronger and deeper connections between organizations [14]. Its conceptualization originated in social psychology, where it initially focused on understanding the causes and maintenance of various types of relationships, including personal, employer-employee, and individual-community relationships. It was subsequently expanded to the business context to examine the reasons for continued engagement in business activities and the process of building cooperative marketing relationships [21].
Achieving a state of commitment in a business relationship involves progressing through various phases that gradually establish and solidify this commitment within the relationship [5]. This implies that both parties have invested efforts and made sacrifices to provide the relationship with stability and a long-term orientation, prioritizing actions that align with a longer-term vision over short-term gains [3,54]. This sustained effort over time establishes commitment as a barrier or deterrent to decisions that could jeopardize the relationship [14,19].
The significance of commitment extends across diverse areas, including service marketing, relationships among marketing channel members, industrial markets, and strategic alliances [3]. This diversity has led to its treatment in the literature from multiple perspectives, encompassing elements such as the desire to maintain the relationship [5], willingness to make short-term sacrifices [25], readiness to invest in the relationship [14], a sense of identification and affection [54] and internalization of the norms and values of the relationship partner [55].
Within marketing channels and the relationships among channel members, the presence of commitment plays a pivotal role in the channel's sustainability [3,25]. It yields outcomes that enhance effectiveness, productivity and efficiency in the distribution of goods and services [5,54]. Commitment among channel members fosters solidarity and cohesion [14,16], motivating them to resist the allure of short-term alternatives. Empirical evidence, as demonstrated by Weitz & Jap, supports the perception that commitment in a relationship generates associated benefits, including improved communication between business partners, achievement of objectives, and reduced opportunistic behavior.
In this context, commitment in business relationships is defined as "an exchange partner believing that an ongoing relationship with another is so important as to warrant maximum efforts at maintaining it; that is, the committed party believes the relationship is worth working on to ensure that it endures indefinitely" [5]. This definition encompasses not only a "belief" but also an "intention" between organizations to sustain their relationship [14]. Furthermore, these beliefs and intentions create an environment conducive to the parties perceiving their relationship as contributing to the achievement of their goals, free from concerns about unexpected behaviors [19].
Commitment, as a concept, is characterized by its dual dimension: affective or loyalty commitment and calculative commitment [15,17,19,44]. The former is also commonly referred to as emotional commitment, while the latter is sometimes termed functional or economic commitment, as noted by Morgan and Hunt (1994). Affective commitment is the dimension most frequently discussed in the literature (e.g., Anderson & Weitz, 1992; Dwyer et al., ), and it pertains to the "desire" to maintain a relationship because of the satisfaction derived from conducting business with a specific partner. This form of commitment is rooted in emotions such as affection, emotional attachment, and social bonding [13,54]. It encompasses the desire to nurture and strengthen a relationship with another party due to the familiarity, friendship, and personal trust cultivated through interpersonal interactions over time [21].
Conversely, calculative commitment views commitment as a "need" to maintain a relationship, arising from the costs associated with its termination or a lack of viable alternatives for conducting business [13]. This dimension of commitment involves a weighing of incentives against costs when considering the continuation of a relationship [21]. While some research suggests that affective commitment plays a more significant role in fostering and sustaining enduring relationships compared to financially motivated commitment [54], evidence also indicates that affective commitment alone may not compensate for a failure to meet the performance objectives of the organizations involved [21].
The fundamental distinction between affective and calculative commitment lies in their motivations. Affective commitment arises from emotional connections and serves as a motivation to collaboratively resolve issues or conflicts. In contrast, commitment driven by economic considerations may wane if a more advantageous alternative emerges, leading parties to question the relinquishment of individual benefits [17]. Consequently, commitment rooted in loyalty (affective) entails a subordination of individual interests to those of the relationship [5,8,15], whereas commitment based on economic motives may raise doubts regarding the parties' willingness to forgo personal gains [13,17,21].
In light of this framework, we establish trust as an antecedent of emotional commitment and dependence as an antecedent of calculative commitment. These connections have been extensively explored in academic literature [5,8,13,14,15,17,19,21]. Therefore, we propose the following hypotheses:
H3: Trust positively influences affective commitment in inter-organizational relationships.
H4: Dependence positively influences calculative commitment in inter-organizational relationships.
Once the establishment of commitment within organizations has been elucidated [5,25,36,56], it becomes imperative to determine whether the commitment of the organizations comprising the distribution channel exerts an influence on the individual engagement of the employees responsible for the company's operations [28,29]. The marketing literature has predominantly examined the concept of engagement within the context of "customer engagement in B2C settings, "customer engagement in B2C settings, while B2B settings have largely been neglected" [26]. Nevertheless, the purchasing processes in B2B environments share similar motivational drivers with B2C scenarios [30]. Consequently, it is essential to recognize that "engagement is both an individual- and a collective-level phenomenon" [31]. In the context of our analysis, "B2B Engagement involves a complex network of heterogeneous members who interact at organizational and individual levels" [29].
Unlike consumers, B2B buyers procure products to fulfill the requirements of their respective customers [57]. Moreover, B2B purchasing processes entail the participation of multiple functions and individuals in decision-making. Consequently, the analysis of engagement in business markets necessitates a multi-level perspective [26,27] because it encompasses cognitive, affective, and behavioral investments in firm interactions [58]. It manifests as a more rational form of engagement compared to that found in consumer markets [30]. Several factors contributing to the likelihood of engagement occurrence are rooted in fundamental concepts from the inter-organizational relationship marketing literature, including trust, dependence, affective commitment, and calculative commitment [26,28,59]. Consequently, the engagement within a B2B context is a psychological state emerging under specific conditions, such as organizational commitment [27,29,60,61]. However, it also has individual elements [62,63,64]. This leads to the formulation of the following hypotheses:
H5: Affective commitment positively influences B2B engagement.
H6: Calculative commitment positively influences B2B engagement.
Furthermore, the sequence of hypotheses H3 and H5, on one hand, and hypotheses H4 and H6, on the other, reveals mediating effects between trust and B2B engagement, as well as between dependence and B2B engagement. These mediating relationships are captured in the following hypotheses:
H7: Trust indirectly exerts a positive effect on B2B engagement through its influence on affective commitment.
H8: Dependence indirectly exerts a positive effect on B2B engagement through its influence on calculative commitment.
A substantial body of literature has effectively established the connection between classical inter-organizational relationships and inter-firm cooperation [5,7,12,13,14,15,16,45,49]. However, the relationship between individual engagement in a B2B environment and cooperation has received comparatively less attention. Nonetheless, research has revealed that it is feasible to foster inter-organizational cooperation through the individual engagement of employees engaged in firm relations, as B2B engagement "affects long-term business collaboration" [29], "impacts customer satisfaction, purchase/usage intentions, and loyalty" [28], and higher levels of engagement correlate with higher levels of cooperation [26,27]. These findings lead us to propose the following hypothesis:
H9: B2B engagement positively influences cooperation in inter-organizational relationships.
Furthermore, the sequence of hypotheses H3, H5 and H7 suggests that cooperation can be achieved not only directly through trust (H1) but also through a mediated pathway involving affective commitment and B2B engagement. Consequently, we propose the following hypothesis:
H10: Trust indirectly exerts a positive effect on cooperation in inter-organizational relationships through a sequential influence of affective commitment and B2B engagement.
Similarly, the sequence of hypotheses H4, H6, and H8 indicates that cooperation can be achieved not only directly through dependence (H2) but also through a mediated pathway involving calculative commitment and B2B engagement. Therefore, we propose the following hypothesis:
H11: Dependence indirectly exerts a positive effect on cooperation in inter-organizational relationships through a sequential influence of calculative commitment and B2B engagement.
Figure 1 illustrates the proposed model and the underlying assumptions.
The proposed hypotheses were empirically examined using a sample of spanish fast-moving consumer goods (FMCG) distribution companies operating in sectors such as beverages, food, cleaning, and personal care. Spain hosts a substantial number of these distribution companies, totaling 25,100, which contribute significantly to the country's gross domestic product (GDP), accounting for more than 20% of its economic output. The target distributors in the survey included both wholesale and retail establishments, or a combination of both. In the context of this sector, determining the roles played by both institutional and individual relationships is often challenging, and addressing this gap in the literature is one of the contributions of our research.
To collect data on relationships within the FMCG marketing channel, we developed a questionnaire specifically designed for purchasing or procurement managers of distribution companies. Key informants were identified through profiles on the professional social network LinkedIn. Upon contacting them, we explained the purpose of the research and assured them of the confidentiality of their responses. We then sent them a self-administered online questionnaire requesting responses to various questions concerning their relationships with suppliers, which could be either manufacturers or other distributors of FMCG products. After sending out 1,200 questionnaires, we received 204 valid responses. Six questionnaires were discarded for incomplete answers.
Before distributing the survey, we conducted a pre-test involving ten purchasing managers to gather their opinions regarding the clarity and comprehensibility of the survey's concepts. The positive feedback received indicated that the surveyed concepts were suitable for research purposes and for understanding relationships in the distribution channel, suggesting face validity [65]. Although the data were obtained from a single source, we assessed multicollinearity concerns and ruled out issues related to common method bias [66] (Appendix Ⅰ). Additionally, we conducted an ANOVA test based on the response phase criterion, and the absence of significant differences suggested a low likelihood of non-response bias [67]. Similarly, it has been shown that there are no statistical differences by gender of the respondent (see Appendix Ⅱ). Furthermore, we maintained complete anonymity in the questionnaire to mitigate potential social desirability bias. Table 1 presents the characteristics of the obtained sample:
MEASURE | CATEGORY | N | PERCENT |
Gender of respondent | Male | 171 | 83.82% |
Female | 33 | 16.18% | |
Firm Size* | Small (<50 employees) | 87 | 42.65% |
Medium (50−250 employees) | 28 | 13.73% | |
Large (>250 employees) | 89 | 43.62% | |
Distributor type | Wholesale | 95 | 46.57% |
Retail | 53 | 25.98% | |
Both | 56 | 27.45% | |
*Note: (*) European Union (EU) classification |
The research constructs were assessed using a Likert-type scale ranging from 1 to 7, where 1 = "Strongly disagree, " and 7 = "Strongly agree." These concepts have been extensively explored in the literature, and we employed scales that have demonstrated their reliability and validity in previous studies within the field of marketing channels. The following constructs were examined: TRUST, DEPENDENCE, COOPERATION, AFFECTIVE COMMITMENT, CALCULATIVE COMMITMENT, and B2B ENGAGEMENT. For further details regarding the scales used, please refer to Table 2:
TRUST (Sharma, 2020; Suh et al., 2019) [22,50] | |
TRUST 1 | We feel we can trust this supplier completely |
TRUST 2 | We have full confidence in the information provided to us by this supplier |
TRUST 3 | We have complete confidence in this supplier's motives |
TRUST 4 | The supplier keeps the promises it makes to our firms |
TRUST 5 | When it comes to things that are important to us, we can depend on the supplier's support |
DEPENDENCE (Cai et al., 2017; Du & Zhang, 2017; Vázquez-Casielles et al., 2017) [13,49,68] | |
DEP 1 | Our company is quite dependent on this supplier |
DEP 2 | If this relationship ended, our company would face a significant loss |
DEP 3 | The supplier provides vital resources our company would find difficult to obtain elsewhere |
DEP 4 | It would be very difficult to replace the sales and profits generated by this manufacturer's products |
DEP 5 | If our relationship was discontinued with the manufacturer, it would be difficult for us to find an alternative supplier |
COOPERATION (Gaski & Ray, 2004; Gimeno-Arias & Santos-Jaén, 2022; Xue et al., 2018) [11,45,47] | |
COOP 1 | We share information with our supplier |
COOP 2 | We participate in joint goal setting and forecasting with our supplier |
COOP 3 | This supplier is generally willing to cooperate with us |
COOP 4 | This supplier provides adequate help in selling his products |
COOP 5 | We have a good working relationship with this supplier |
COOP 6 | We view the success of the supplier as our own success |
AFFECTIVE COMMITMENT (Gilliland & Bello, 2002; Sharma et al., 2015) [17,21] | |
LOY_COM 1 | Our company believes that this supplier acts in our best interest |
LOY_COM 2 | We have positive feelings towards this supplier |
LOY_COM 3 | Our loyalty to this supplier is among the primary reasons for our continued collaboration |
LOY_COM 4 | We wish to maintain our association with this supplier due to our strong loyalty |
LOY_COM 5 | We intend to continue working with this supplier because we feel that they are "part of the family" |
CALCULATIVE COMMITMENT (Du & Zhang, 2017; Sharma et al., 2015) [13,21] | |
CAL_COM 1 | We currently have no alternatives to this relationship |
CAL_COM 2 | It would be very difficult for us to find a replacement for this supplier |
CAL_COM 3 | Changing from this supplier to another would cost us too much |
CAL_COM 4 | We want to maintain the relationship with this supplier because it is difficult to find similar suppliers |
CAL_COM 5 | We need to maintain the relationship with this supplier because ending it would result in significant losses. |
B2B ENGAGEMENT (Kumar & Pansari, 2016) [27] | |
ENGA 1 | I love talking about my experience with this supplier |
ENGA 2 | I feel a sense of belonging towards this supplier |
ENGA 3 | When referring to this supplier, I usually say "we" rather than "they" |
ENGA 4 | This supplier holds significant personal significance for us |
Our research model incorporates six constructs, each with distinct relationships to the indicators they encompass. As per Sarstedt et al. [69], these variables are categorized as composites, specifically classified as Mode A composites due to the substantial correlation among the indicators [70]. To analyze the interrelationships among these variables, we utilized PLS-SEM. PLS-SEM can accommodate intricate relationships involving mediating and moderating effects without necessitating specific data distribution assumptions [71]. It employs a non-parametric bootstrap procedure to assess the significance of the model's parameters [72,73,74]. Furthermore, PLS-SEM is widely recognized as a suitable technique for examining complex and multiple relationships [75,76,77], making it the optimal choice for our proposed model.
To evaluate the hypotheses within our model, we employed the SmartPLS 4.0.9.5 software program [78] and conducted bootstrapping with 10,000 samples, following the recommendation of Streukens and Leroi-Werelds [79], conducted bootstrapping with 10,000 samples. Additionally, we used the G*Power 3.1.9.7 software program [80] to calculate statistical power. Based on the presence of a path leading to the final dependent variable, a significance level of 5%, an average effect size of 0.15, and a statistical power of 95%, a priori analysis determined that a minimum sample of 89 observations was necessary [81]. Given that our sample size comprises 204 observations, we have an adequate sample size to validate the obtained results.
SmartPLS is a software tool used for conducting structural equation modeling (SEM). SEM is a statistical technique employed to analyze relationships between latent and observed variables. SmartPLS is particularly known for its focus on partial least squares (PLS) to estimate model parameters. SmartPLS is known for its PLS-SEM approach and is often preferred when working with complex models or when data does not meet normality assumptions. The flexibility of PLS-SEM and its ability to handle non-normally distributed data make it an appealing choice when working with small samples [79,80].
As for the basic operation of this software, regarding data input, data is entered into SmartPLS in the form of a data matrix containing information about latent and observed variables. Next, the latent variables are created with the items, and the nomogram is drawn. Latent variables are unobservable constructs measured indirectly through observed variables.
With the drawing of the model, the theoretical model is created. A theoretical model describing the relationships between latent and observed variables is specified. This model is represented through a path diagram.
The next step is the estimation of the parameters. SmartPLS uses the PLS method to estimate the model parameters. Unlike other SEM approaches, PLS does not require the normal distribution of data and is robust even with small samples.
Once parameters are estimated, SmartPLS evaluates the fit of the model. This involves reviewing statistics such as the coefficient of determination (R²), factor loadings, t-values, etc.
The next step is to test the validity of the measurement model or internal model. Its verification will depend on whether the variables created are reflective or formative. If they are reflective, the reliability and validity of the items are analyzed, evaluating item loadings, Cronbach's Alpha, mean extracted variance, and HTMT (Heterotrait-Monotrait Ratio of Correlations), among others. In the case of the use of formative variables, the significance of the weights and loadings will be checked, and the existence of multicollinearity problems will be ruled out.
Once the internal model has been validated, the results of the structural model will be interpreted by bootstrapping 10,000 subsamples. Results are generated to interpret relationships between variables and assess the significance of estimated effects. This involves reviewing statistics such as the coefficient of determination (R²) and the effect size (f2).
The PLS algorithm consists of four phases. In the initial phase, the structural model weights and scores of latent variables are determined through a four-step iterative procedure [82,83,84]:
Initial external estimation of the constructs' scores by linearly combining their observed variables:
ηk,n:=∑lkδlk⋅ψlk,n | (1) |
where
Calculation of internal weights for constructs using the factor weighting approach, determined based on the direction of correlations among constructs:
ωk,h={cov(ηk,ηh), if ηk and ηh are neighbors0, otherwise | (2) |
Calculation of construct scores from within, accomplished by linearly combining their associated variables using the internal weights determined in the previous phase:
ˉηk:=∑hβk,h⋅ηh | (3) |
Estimation of external weights, taking into account that all constructs in our model are considered in reflective form:
zlk,n=δlk⋅ˉηk,n+elk,n | (4) |
with
The process is iterative and concludes either when the weights in Step 4 exhibit minimal change between successive iterations, or when the maximum iteration limit is reached.
Utilizing the construct scores derived during Stage 1 and employing a sequence of ordinary least squares regressions in Stages 2 and 3, we establish the external weights and loadings of indicators, path coefficients, as well as various parameters including direct and indirect effects, the R2 coefficient for endogenous constructs, and diverse model evaluation measures.
Finally, during Stage 4, we employ the non-parametric bootstrapping method (a resampling technique with replacement) to assess the statistical significance of the model's parameters.
To assess the reflective constructs, we must compare the reliability of each of its indicators, along with the reliability of the construct itself, and its convergent and discriminant validity. Composite reliability [82], with an optimal range of 0.7 to 0.95, represents the minimal threshold for the internal consistency reliability of the reflective construct. This metric is calculated as follows:
ρC=(L∑l=1pl)2(L∑l=1pl)2+L∑l=1var(el) | (5) |
where
α=L⋅ˉm1+(L−1)⋅ˉm′ | (6) |
where
ρA:=(δ′δ)2⋅δ′(M−diag(S))δδ′(δδ′−diag(δδ′))δ′ | (7) |
where
Finally, the average variance extracted (AVE) quantifies the degree of convergent validity [86], and is considered satisfactory when its value is greater than 0.5, indicating that the latent variable explains over 50% of the variance in its indicators:
AVE=L∑l=1p2lL | (8) |
The results were obtained through a three-phase approach: firstly, the evaluation of the measurement model, followed by the assessment of the structural model; and finally, the analysis of the predictive power.
By analyzing the measurement model, we assessed the reliability and validity of both the indicators and the latent variables. Reliability ensures consistent outcomes from the measurements, while validity confirms that the indicators of a construct indeed assess the intended construct and not an alternative one [70].
To begin the measurement model analysis, we examined the reliability of the items used to construct the variables within the model. The results presented in Table 3 indicated that the standardized factor loadings of the indicators exceeded the minimum threshold of 0.7, indicating satisfactory item reliability [87]. Only one of the indicators did not surpass this minimum threshold; however, it was retained in the model as its value exceeded 0.4, and its inclusion was supported by theoretical rationale [70].
Indicator | Mean | SD | Loading | t-student* | Indicator | Mean | SD | Loading | t-student* | |
CAL COM | DEP | |||||||||
CAL COM1 | 3.438 | 1.774 | 0.843 | 23.777 | DEP1 | 4.300 | 1.490 | 0.778 | 22.804 | |
CAL COM2 | 3.627 | 1.793 | 0.930 | 80.461 | DEP2 | 3.970 | 1.740 | 0.918 | 78.527 | |
CAL COM3 | 3.824 | 1.852 | 0.935 | 93.530 | DEP3 | 3.794 | 1.803 | 0.891 | 36.583 | |
CAL COM4 | 3.926 | 1.774 | 0.911 | 53.192 | DEP4 | 3.897 | 1.676 | 0.904 | 58.519 | |
CAL COM5 | 3.665 | 1.846 | 0.909 | 46.168 | DEP5 | 3.716 | 1.809 | 0.844 | 31.230 | |
TRUST | ENGA | |||||||||
CONF1 | 5.338 | 1.298 | 0.929 | 84.323 | ENGA1 | 4.862 | 1.537 | 0.817 | 28.227 | |
CONF2 | 5.404 | 1.334 | 0.916 | 56.846 | ENGA2 | 4.198 | 1.782 | 0.904 | 50.228 | |
CONF3 | 5.182 | 1.376 | 0.912 | 64.906 | ENGA3 | 4.778 | 1.725 | 0.477 | 6.542 | |
CONF4 | 5.588 | 1.211 | 0.854 | 37.282 | ENGA4 | 4.044 | 1.981 | 0.875 | 37.506 | |
CONF5 | 5.696 | 1.195 | 0.851 | 30.836 | ENGA5 | 4.308 | 1.893 | 0.885 | 37.980 | |
COOP | LOY COM | |||||||||
COOP1 | 5.456 | 1.377 | 0.843 | 36.006 | LOY COM1 | 5.162 | 1.256 | 0.802 | 28.622 | |
COOP2 | 5.127 | 1.591 | 0.808 | 28.233 | LOY COM2 | 5.417 | 1.212 | 0.830 | 36.773 | |
COOP3 | 5.794 | 1.320 | 0.846 | 28.382 | LOY COM3 | 4.917 | 1.478 | 0.845 | 30.462 | |
COOP4 | 5.534 | 1.373 | 0.842 | 25.834 | LOY COM4 | 4.824 | 1.471 | 0.876 | 44.212 | |
COOP5 | 5.887 | 1.205 | 0.883 | 51.894 | LOY COM5 | 4.455 | 1.715 | 0.816 | 31.411 | |
*Note: Significance and standard deviations (SD) performed by 10,000 repetitions bootstrapping procedure ∗∗∗: All loadings are significant at a 0.001 level. |
The analysis of the measurement model also includes an assessment of the reliability and validity of the latent variables. Following the approach outlined by Dijkstra and Henseler [85], we utilized Cronbach's alpha, composite reliability, and the Dijkstra-Henseler rho ratio to evaluate the reliability of the variables. The results presented in Table 4 confirm that the variables meet the reliability criterion, exceeding the predefined minimum threshold of 0.7 [87].
Composites | α | ρA | ρC | AVE |
CAL COM | 0.945 | 0.951 | 0.958 | 0.821 |
TRUST | 0.875 | 0.893 | 0.909 | 0.632 |
COOP | 0.918 | 0.922 | 0.939 | 0.754 |
DEP | 0.893 | 0.894 | 0.926 | 0.758 |
ENGA | 0.891 | 0.893 | 0.920 | 0.696 |
LOY COM | 0.936 | 0.938 | 0.952 | 0.798 |
*Note: α: Chronbach's alpha; ρA: Dijkstra–Henseler's composite reliability; ρC: Jöreskog's composite reliability; AVE: average variance extracted. |
Furthermore, the convergent validity of the variables was examined by assessing the average variance extracted (AVE), which also surpasses the established minimum of 0.5 [87]. This demonstrates that the variables in the model adequately explain over 50% of the variance in their respective indicators.
In the subsequent phase of the measurement model analysis, we confirmed discriminant validity using two established criteria: the Fornell-Larcker criterion by Fornell & Larcker [88] and the Heterotrait-Monotrait (HTMT) ratio values as proposed by Henseler [89]. The objective is to ensure that each of the constructs is distinct from the others.
According to the Fornell-Larcker criterion, the correlations between any two variables should be less than the square root of the average variance extracted (AVE) for each respective variable. The results presented in Table 5 validate that all variables within the model meet the Fornell-Larcker criterion, indicating discriminant validity.
Ⅰ | Ⅱ | Ⅲ | Ⅳ | Ⅴ | Ⅵ | ||
Ⅰ | CAL COM | 0.906 | |||||
Ⅱ | COOP | 0.043 | 0.795 | ||||
Ⅲ | DEP | 0.828 | 0.175 | 0.868 | |||
Ⅳ | ENGA | 0.398 | 0.448 | 0.429 | 0.871 | ||
Ⅴ | LOY COM | 0.253 | 0.689 | 0.344 | 0.633 | 0.834 | |
Ⅵ | TRUST | 0.107 | 0.780 | 0.208 | 0.396 | 0.722 | 0.893 |
*Note: Fornell–Larcker criterion: square root of AVE in diagonal (bold) and construct correlations below the diagonal. |
Additionally, the HTMT ratio values, as proposed by Henseler, were examined. These values should be less than 0.85 to confirm discriminant validity. The results confirmed that all HTMT ratios in our model were below this threshold, further supporting the discriminant validity of the constructs.
Overall, the measurement model analysis confirms the reliability and validity of the indicators and latent variables and establishes discriminant validity among the constructs.
With respect to HTMT, the results in Table 6 show that all the values obtained are below the maximum recommended value of 0.85 [87].
Ⅰ | Ⅱ | Ⅲ | Ⅳ | Ⅴ | ||
Ⅰ | CAL COM | |||||
Ⅱ | COOP | 0.102 | ||||
Ⅲ | DEP | 0.784 | 0.212 | |||
Ⅳ | ENGA | 0.428 | 0.542 | 0.473 | ||
Ⅴ | LOY COM | 0.278 | 0.775 | 0.388 | 0.713 | |
Ⅵ | TRUST | 0.117 | 0.843 | 0.228 | 0.430 | 0.770 |
Finally, the standardized root mean square residual (SRMR), used to assess the goodness of fit in this study, was examined in both saturated and estimated models to ensure it remained below the threshold of 0.08. These results indicate that the model aligns well with the data requirements [90].
Based on the results obtained, the validity and reliability of the model are confirmed.
Before scrutinizing the established relationships within the model, we conducted an evaluation of potential multicollinearity by examining the variance inflation factor (VIF), as presented in Table 7. The findings revealed VIF values ranging from 1.000 to 1.394, which remain below the preferred threshold of 3 [87]. Consequently, it can be inferred that the model is devoid of multicollinearity concerns.
CAL_COM | COOP | ENGA | LOY_COM | TRUST | |
CAL_COM | 1.068 | 1.189 | |||
COOP | |||||
DEP | 1.000 | 1.228 | |||
ENGA | 1.394 | ||||
LOY_COM | 1.068 | 1.000 | |||
TRUST | 1.189 | 1.000 |
Next, we conducted a percentile bootstrapping test with 10,000 subsamples, employing a one-tailed test with a significance level of 5% to evaluate the size, direction, and significance of the paths derived from the relationships established as hypotheses in the proposed model. Table 8 and Figure 2, for reference, present the t-values and corresponding confidence intervals derived from this analysis. The findings indicate that ten of the eleven established relationships are significant and supported.
CI | ||||||||
Path | SD | T-value | 5% | 95% | f2 | H | Supported | |
Direct effects | ||||||||
TRUST - > COOP | 0.717 | 0.050 | 14.286*** | 0.629 | 0.794 | 1.177 | H1 | YES |
DEP - > COOP | −0.054 | 0.053 | 1.007ns | −0.142 | 0.033 | 0.006 | H2 | NO |
TRUST - > LOY COM | 0.722 | 0.034 | 20.946*** | 0.663 | 0.776 | 1.091 | H3 | YES |
DEP - > CAL COM | 0.828 | 0.026 | 31.289*** | 0.782 | 0.870 | 2.188 | H4 | YES |
LOY_COM - > ENGA | 0.569 | 0.057 | 9.962*** | 0.474 | 0.661 | 0.561 | H5 | YES |
CAL_COM - > ENGA | 0.254 | 0.061 | 4.163*** | 0.152 | 0.354 | 0.112 | H6 | YES |
ENGA - > COOP | 0.187 | 0.065 | 2.872*** | 0.078 | 0.291 | 0.068 | H9 | YES |
Indirect effects | ||||||||
Individual indirect effects | VAF | |||||||
TRUST - > LOY COM - > ENGA | 0.411 | 0.046 | 9.021*** | 0.336 | 0.487 | 100% | H7 | YES |
DEP - > CAL COM - > ENGA | 0.210 | 0.052 | 4.079*** | 0.126 | 0.296 | 100% | H8 | YES |
TRUST - > LOY COM - > ENGA - > COOP | 0.077 | 0.030 | 2.597** | 0.030 | 0.127 | 10% | H10 | YES |
DEP - > CAL COM - > ENGA - > COOP | 0.039 | 0.015 | 2.657** | 0.016 | 0.064 | 100% | H11 | YES |
CAL COM - > ENGA - > COOP | 0.047 | 0.017 | 2.715** | 0.019 | 0.077 | 100% | ||
LOY COM - > ENGA - > COOP | 0.106 | 0.042 | 2.555** | 0.041 | 0.178 | 100% | ||
Indirect effects | ||||||||
CAL COM - > COOP | 0.047 | 0.017 | 2.715** | 0.019 | 0.077 | 100% | ||
DEP - > COOP | 0.039 | 0.015 | 2.657** | 0.016 | 0.064 | 100% | ||
DEP - > ENGA | 0.210 | 0.052 | 4.079*** | 0.126 | 0.296 | 100% | ||
LOY COM - > COOP | 0.106 | 0.042 | 2.555** | 0.041 | 0.178 | 100% | ||
TRUST - > COOP | 0.077 | 0.030 | 2.597** | 0.030 | 0.127 | 10% | ||
TRUST - > ENGA | 0.411 | 0.046 | 9.021*** | 0.336 | 0.487 | 100% | ||
Total effects | ||||||||
CAL_COM - > COOP | 0.047 | 0.017 | 2.715** | 0.019 | 0.077 | |||
DEP - > COOP | -0.014 | 0.050 | 0.288ns | -0.099 | 0.067 | |||
DEP - > ENGA | 0.210 | 0.052 | 4.079*** | 0.126 | 0.296 | |||
LOY_COM - > COOP | 0.106 | 0.042 | 2.555** | 0.041 | 0.178 | |||
TRUST - > COOP | 0.793 | 0.038 | 20.750*** | 0.726 | 0.851 | |||
TRUST - > ENGA | 0.411 | 0.046 | 9.021*** | 0.336 | 0.487 | |||
*Note: R2 [95% CI in brackets]: CAL COM: 0.685 [0.610; 0.756]; COOP: 0.627 [0.542; 0.715]; ENGA: 0.455 [0.367; 0.556]; LOY COM: 0.519 [0.437; 0.601]. f2: size effect index; 95PCI:95% percentile Confidence Interval; Significance, standard deviations, 95% bias-corrected CIs were performed by 10,000 repetitions bootstrapping procedure; *: p < 0.05; **: p < 0.01; ***: p < 0.001. Only total effects that differ from direct effects are showed. |
Regarding the direct effects, the findings demonstrate that TRUST positively and significantly influences COOP and LOY COM (β = 0.717*** and β = 0.722***, respectively), confirming H1 and H3. However, the effect of DEP on COOP is not significant (β = −0.054 ns), leading to the rejection of H2. Conversely, the effect of DEP on CAL COM is positive and significant (β = 0.828***), resulting in the acceptance of H4. Similarly, the influence received by ENGA from both LOY COM and CAL COM is positive and significant (β = 0.569*** and β = 0.254***, respectively), supporting H5 and H6. Finally, ENGA significantly and positively influences COOP (β = 0.187***), confirming H9.
The results corresponding to the indirect effects indicate that LOY COM mediates the relationship between TRUST and ENGA (β = 0.411***). Since there is no prior direct effect, mediation is complete, and H7 is accepted. Similarly, CAL COM has been shown to mediate the relationship between DEP and ENGA in a full-fledged manner (β = 0.411***), supporting H8. As a consequence of the two mediations described above, two other sequential mediations have emerged. On the one hand, the relationship between TRUST and COOP is measured by LOY COM and ENGA sequentially (β = 0.077**). On the other hand, the relationship between DEP and COOP is sequentially measured by CAL COM and ENGA (β = 0.039**). Therefore, H10 and H11 are accepted.
The R2 results of the endogenous variables suggest that the model possesses a satisfactory capacity to elucidate the data. The results show that the model explains 68.50% of the variance in CAL COM, 62.70% in COOP, 45.50% in ENGA and 51.90% in LOY COM. It is important to note that R2 values above 0.33 are considered moderate, and values above 0.60 are considered substantial [81,91].
Lastly, f2 is employed as an indicator of effect size [81]. It quantifies the extent to which an exogenous construct contributes to explaining a particular endogenous construct in relation to R2. Values of f2 greater than 0.35 demonstrate a large effect [91]. This occurs in all established relationships that have been accepted, except in the relationship between ENGA and COOP, where the effect is weak.
In summary, Figure 2 illustrates the standardized path coefficients and R2 values, encapsulating the results of the study.
Given that R2 solely evaluates a model's explanatory capability and not its predictive prowess for new instances beyond the sample [92], the predictive performance of our model has been evaluated with data other than those of the sample obtained. In this context, predictive validity, which pertains to out-of-sample prediction, demonstrates that a specific set of measures for a variable can forecast a designated outcome variable [93]. This was evaluated using cross-validation with holdout samples [94], achieved by implementing the PLSpredict algorithm available in SmartPLS [95].
Initially, a k-fold cross-validation was carried out, with k set at 6 subgroups, to ensure adherence to the minimum holdout sample size of N = 30 [96], with ten repetitions of this process. Subsequently, a PLS predict analysis was conducted within the model [97].
The results displayed in Table 9 indicate that in both instances - at the construct and indicator levels - all Q2 values are greater than 0. As a result, the model presents a satisfactory predictive performance [98]. Similarly, we obtained positive values for the root mean squared error (RMSE) and the mean absolute error (MAE) in the constructs, showing good predictability [99,100]. Furthermore, a comparable conclusion emerged at the indicator levels when comparing the outcomes of RMSE or MAE from the PLS-SEM with those of the linear regression model (LM). Due to the symmetry of the items, only RMSE has been compared. As seen in Table 9, the value of Q2 predict is positive in all items. Moreover, as shown in ANNEX 3, except for those that form the LOY COM construct, RMSE with PLS is lower than with LM, demonstrating the predictive capability of the model [87].
CONSTRUCT PREDICTION SUMMARY | |||
Construct | Q2predict | RMSE | MAE |
CAL_COM | 0.683 | 0.569 | 0.434 |
COOP | 0.598 | 0.643 | 0.493 |
ENGA | 0.244 | 0.879 | 0.723 |
LOY_COM | 0.518 | 0.703 | 0.563 |
The results obtained provide empirical support for the research questions posed. Firstly, it is noteworthy that there is limited evidence regarding the effectiveness of relationship governance in sectors such as FMCG distribution. The literature has either questioned or overlooked the significant role of relationships [7,10,11]. However, our findings indicate that relationship governance does contribute to fostering high-quality business cooperation in the FMCG sector, aligning with established relational literature in different contexts [5,7,12,13,14,15,45].
Furthermore, our research has established a profile of how relationships play a crucial role in promoting cooperation among organizations. Cooperation is not solely established directly through trust [5,8]. Instead, it also results from organizational commitment to the business partner and individual engagement with decision-makers [26,29]. While we did not find direct evidence supporting the notion that dependence leads to cooperation (H2 was rejected), the absence of the effect of dependence on cooperation is due to the fact that cooperation implies voluntariness. Dependence implies an imbalance of power and one agent is forced by another. In this situation, voluntary cooperation, which the forced agent may not identify as cooperation, vanishes (Witek-Hajduk & Zaborek, 2020). On the other hand, our results indicate that dependence can promote calculative commitment. This organizational commitment, in turn, fosters individual B2B engagement, ultimately leading to business cooperation [27,28].
Marketing literature has traditionally focused on transaction-level efficiency in FMCG distribution environments, leaving a gap in understanding relationship efficiency in these contexts. Our research contributes to bridging this gap by revealing that distribution companies in the FMCG channel establish cooperation based on factors such as trust between the channel's stakeholders. While our findings did not show a direct impact of dependence on cooperation, we established its influence through mediating effects such as the creation of calculated commitment stemming from dependence. This calculated commitment, in turn, significantly affects the individual engagement of purchasing managers in their relationships with suppliers.
Moreover, our research addresses a notable distinction made in academic literature between inter-organizational relationships and individual relationships within B2B environments. We establish a connection between organizational commitment (both affective and calculated) and the individual engagement developed by buyers, which we term B2B engagement. This individual engagement can be inferred from the profile of inter-organizational relationships, including trust-affective commitment and dependence-calculated commitment. Additionally, our work contributes to the literature by demonstrating that business cooperation arises not only from organizational relationships but also from individual engagement in B2B contexts. In this context, purchasing managers, who function as customers, exhibit engagement behaviors similar to those observed in B2C settings, such as satisfaction, purchase intent, and loyalty.
Promoting business cooperation is a critical aspect of effective business management, particularly within the distribution channel. Cooperation among business partners or agents in the distribution channel is not solely determined by the governance of inter-organizational relationships; it also stems from the individual commitment of purchasing managers within distribution companies. Therefore, it is prudent for business managers to establish a comprehensive profile of inter-organizational relationships, which can serve as a guiding framework for the individual relationships maintained by those involved in business management.
By doing so, businesses can leverage multiple strategies to foster cooperation, leading to enhanced organizational performance both in collaboration with their partners and within their internal operations. The extensive body of literature on efficient distribution channel management underscores the significance of addressing business dynamics at both the transactional and relational levels.
Every research endeavor must engage in self-reflection and acknowledge its limitations. In our study, we validated the relationship model within the context of FMCG distribution. However, it's important to recognize that relationships are influential in various distribution environments, where their efficiency may differ. Therefore, one limitation of our study is its context-specific validation. To address this, future research could broaden its scope to encompass different distribution channels, especially in today's rapidly evolving landscape with the significant growth of e-commerce. This expansion would contribute to a more comprehensive understanding and generalization of the relationship model in the FMCG distribution channel.
Another limitation is the geographical scope of our study. While our research provides valuable insights within its chosen geographical context, extending the investigation to other regions or markets could enhance the applicability and generalizability of the relationship model. This would enable a more thorough understanding of how relationships function within the FMCG distribution channel across diverse settings. In addition, the study in countries with other levels of development (e.g., developing economies) would provide a deeper understanding of one of the most important industries in the world.
Finally, we would like to emphasize that not only the FMCG distribution industry has received little attention from the literature. There are distribution channels where we are not aware that the effectiveness of relationships in distribution channel management has been addressed. In this regard, we would like to highlight those of household consumer technology or textile products. In the channels mentioned, the relationship between organizational commitment and individual engagement is particularly interesting.
The authors declare that they have not used artificial intelligence tools in the creation of this article.
We would like to thank you for following the instructions above very closely in advance.
The authors declare that there is no conflict of interest.
Bartlett's Test of Sphericity | ||
χ2 | df | p |
5485 | 435 | < .001 |
KMO Measure of Sampling Adequacy | ||
MSA | ||
Overall | 0.926 | |
TRUST1 | 0.926 | |
TRUST2 | 0.915 | |
TRUST3 | 0.933 | |
TRUST4 | 0.939 | |
TRUST5 | 0.956 | |
DEPE1 | 0.914 | |
DEPE2 | 0.907 | |
DEPE3 | 0.951 | |
DEPE4 | 0.943 | |
DEPE5 | 0.941 | |
COOP1 | 0.936 | |
COOP2 | 0.961 | |
COOP3 | 0.936 | |
COOP4 | 0.951 | |
COOP5 | 0.938 | |
COOP6 | 0.902 | |
LOY_COM1 | 0.946 | |
LOY_COM2 | 0.948 | |
LOY_COM3 | 0.863 | |
LOY_COM4 | 0.876 | |
LOY_COM5 | 0.932 |
Bartlett's Test of Sphericity | ||
χ2 | df | p |
CAL_COM1 | 0.899 | |
CAL_COM2 | 0.907 | |
CAL_COM3 | 0.942 | |
CAL_COM4 | 0.910 | |
CAL_COM5 | 0.900 | |
ENGA1 | 0.925 | |
ENGA2 | 0.924 | |
ENGA3 | 0.921 | |
ENGA4 | 0.922 |
Initial Eigenvalues | |||
Component | Eigenvalue | % of Variance | Cumulative % |
1 | 11.3641 | 37.880 | 37.9 |
2 | 6.8304 | 22.768 | 60.6 |
3 | 2.7527 | 9.176 | 69.8 |
4 | 1.1413 | 3.804 | 73.6 |
5 | 0.9395 | 3.132 | 76.8 |
6 | 0.7349 | 2.450 | 79.2 |
7 | 0.5651 | 1.884 | 81.1 |
8 | 0.5423 | 1.808 | 82.9 |
9 | 0.4342 | 1.447 | 84.3 |
10 | 0.4270 | 1.423 | 85.8 |
11 | 0.3975 | 1.325 | 87.1 |
12 | 0.3742 | 1.247 | 88.3 |
13 | 0.3484 | 1.161 | 89.5 |
14 | 0.3228 | 1.076 | 90.6 |
15 | 0.2934 | 0.978 | 91.6 |
16 | 0.2699 | 0.900 | 92.5 |
17 | 0.2623 | 0.874 | 93.3 |
18 | 0.2341 | 0.780 | 94.1 |
19 | 0.2221 | 0.740 | 94.9 |
20 | 0.2103 | 0.701 | 95.6 |
21 | 0.2033 | 0.678 | 96.2 |
22 | 0.1892 | 0.631 | 96.9 |
23 | 0.1597 | 0.532 | 97.4 |
24 | 0.1388 | 0.463 | 97.9 |
25 | 0.1321 | 0.440 | 98.3 |
26 | 0.1284 | 0.428 | 98.7 |
27 | 0.1082 | 0.361 | 99.1 |
28 | 0.1011 | 0.337 | 99.4 |
29 | 0.0899 | 0.300 | 99.7 |
30 | 0.0826 | 0.275 | 100.0 |
t-value | DF | p | ||
COOP1 | –0.0872 | 202 | 0.931 | |
COOP2 | –0.9342 | 202 | 0.351 | |
COOP3 | –1.9145 | ᵃ | 202 | 0.057 |
COOP4 | –0.5812 | 202 | 0.562 | |
COOP5 | –1.3219 | ᵃ | 202 | 0.188 |
TRUST1 | –1.3942 | ᵃ | 202 | 0.165 |
TRUST2 | –0.9529 | ᵃ | 201 | 0.342 |
TRUST3 | –0.8446 | ᵃ | 201 | 0.399 |
TRUST4 | –0.4036 | 202 | 0.687 | |
TRUST5 | –1.7356 | ᵃ | 202 | 0.084 |
DEP1 | 2.1116 | 201 | 0.036 | |
DEP2 | 1.5257 | 201 | 0.129 | |
DEP3 | 1.6130 | 202 | 0.108 | |
DEP4 | 1.7877 | 201 | 0.075 | |
DEP5 | 1.5789 | 202 | 0.116 | |
COM_AF1 | –2.0705 | ᵃ | 202 | 0.040 |
COM_AF2 | –0.9646 | 202 | 0.336 | |
COM_AF3 | 0.6913 | 202 | 0.490 | |
COM_AF4 | 1.5656 | 202 | 0.119 | |
COM_AF5 | 1.9051 | 200 | 0.058 | |
COM_EC1 | 2.5842 | 201 | 0.010 | |
COM_EC2 | 2.1232 | 202 | 0.035 | |
COM_EC3 | 3.1196 | 202 | 0.002 | |
COM_EC4 | 2.2717 | 202 | 0.024 | |
COM_EC5 | 1.7581 | 201 | 0.080 | |
ENGA1 | 1.0283 | 201 | 0.305 | |
ENGA2 | 1.1652 | 200 | 0.245 | |
ENGA3 | 0.8423 | 201 | 0.401 | |
ENGA4 | 1.6134 | 201 | 0.108 | |
ENGA5 | 1.8234 | 199 | 0.070 |
t-value | DF | p | ||
COOP1 | 1.6666 | 202 | 0.097 | |
COOP2 | 1.3381 | 202 | 0.182 | |
COOP3 | 1.9101 | ᵃ | 202 | 0.058 |
COOP4 | 1.3328 | ᵃ | 202 | 0.184 |
COOP5 | 0.6725 | 202 | 0.502 | |
COOP6 | –0.8988 | 201 | 0.370 | |
TRUST1 | 0.7535 | 202 | 0.452 | |
TRUST2 | 0.1883 | 201 | 0.851 | |
TRUST3 | 0.5513 | 201 | 0.582 | |
TRUST4 | 0.6898 | 202 | 0.491 | |
TRUST5 | 0.1537 | 202 | 0.878 | |
DEP1 | –0.0106 | 201 | 0.992 | |
DEP2 | –0.4337 | 201 | 0.665 | |
DEP3 | 0.4415 | 202 | 0.659 | |
DEP4 | 0.6301 | 201 | 0.529 | |
DEP5 | 0.7980 | 202 | 0.426 | |
COM_AF1 | 0.8056 | 202 | 0.421 | |
COM_AF2 | 1.0568 | ᵃ | 202 | 0.292 |
COM_AF3 | 2.3686 | ᵃ | 202 | 0.019 |
COM_AF4 | 2.5038 | ᵃ | 202 | 0.013 |
COM_AF5 | 2.3194 | 200 | 0.021 | |
COM_EC1 | –0.3202 | 201 | 0.749 | |
COM_EC2 | 0.0745 | 202 | 0.941 | |
COM_EC3 | –0.1863 | 202 | 0.852 | |
COM_EC4 | 0.4881 | 202 | 0.626 | |
COM_EC5 | –0.6967 | 201 | 0.487 | |
ENGA1 | 0.3217 | 201 | 0.748 | |
ENGA2 | 0.7979 | 200 | 0.426 | |
ENGA3 | 0.5233 | 201 | 0.601 | |
ENGA4 | 0.5911 | 199 | 0.555 |
INDICATOR PREDICTION SUMMARY | ||||
PLS | LM | PLS-LM | ||
Indicator | Q2predict | RMSE | ||
CAL_COM1 | 0.408 | 1.372 | 1.418 | –0.046 |
CAL_COM2 | 0.618 | 1.113 | 1.117 | –0.004 |
CAL_COM3 | 0.628 | 1.135 | 1.186 | –0.051 |
CAL_COM4 | 0.548 | 1.199 | 1.234 | –0.035 |
CAL_COM5 | 0.585 | 1.195 | 1.212 | –0.017 |
COOP1 | 0.369 | 1.098 | 1.095 | 0.003 |
COOP2 | 0.346 | 1.294 | 1.341 | –0.047 |
COOP3 | 0.401 | 1.027 | 1.027 | 0.000 |
COOP4 | 0.447 | 1.027 | 1.056 | –0.029 |
COOP5 | 0.576 | 0.789 | 0.791 | –0.002 |
ENGA3 | 0.105 | 1.641 | 1.651 | –0.010 |
ENGA1 | 0.239 | 1.347 | 1.395 | –0.048 |
ENGA2 | 0.201 | 1.601 | 1.609 | –0.008 |
ENGA4 | 0.126 | 1.864 | 1.895 | –0.031 |
ENGA5 | 0.160 | 1.744 | 1.741 | 0.003 |
LOY_COM1 | 0.607 | 0.792 | 0.755 | 0.037 |
LOY_COM2 | 0.563 | 0.804 | 0.784 | 0.020 |
LOY_COM3 | 0.135 | 1.381 | 1.352 | 0.029 |
LOY_COM4 | 0.199 | 1.325 | 1.323 | 0.002 |
LOY_COM5 | 0.174 | 1.567 | 1.551 | 0.016 |
[1] |
R. S. Achrol, Changes in the theory of interorganizational relations in marketing: Toward a Network Paradigm, J. Acad. Mark. Sci., 25 (1996), 56–71. https://doi.org/10.1177/0092070397251006 doi: 10.1177/0092070397251006
![]() |
[2] |
R. S. Achrol, P. Kotler, Marketing in the network economy, J. Mark., 63 (1999), 146–163. https://doi.org/10.1177/00222429990634s114 doi: 10.1177/00222429990634s114
![]() |
[3] |
E. Anderson, B. Weitz, The use of pledges to build and sustain commitment in distribution channels, J. Mark. Res., 29 (1992), 18–34. https://doi.org/10.1177/002224379202900103 doi: 10.1177/002224379202900103
![]() |
[4] |
A. Kumar, J. B. Heide, K. H. Wathne, Performance implications of mismatched governance regimes across external and internal relationships, J. Mark., 75 (2011), 1–17. https://doi.org/10.1509/jm.75.2.1 doi: 10.1509/jm.75.2.1
![]() |
[5] |
R. M. Morgan, S. D. Hunt, The commitment-trust theory of relationship marketing, J. Mark., 58 (1994), 20–38. https://doi.org/10.1177/002224299405800302 doi: 10.1177/002224299405800302
![]() |
[6] |
R. W. Palmatier, Interfirm relational drivers of customer value, J. Mark., 72 (2008), 76–89. https://doi.org/10.1509/jmkg.72.4.076 doi: 10.1509/jmkg.72.4.076
![]() |
[7] |
G. F. Watson, S. Worm, R. W. Palmatier, S. Ganesan, The evolution of marketing channels: Trends and research directions, J. Retail., 91 (2015), 546–568. https://doi.org/10.1016/j.jretai.2015.04.002 doi: 10.1016/j.jretai.2015.04.002
![]() |
[8] |
J. R. Brown, J. L. Crosno, P. Y. Tong, Is the theory of trust and commitment in marketing relationships incomplete?, Ind. Mark. Manag., 77 (2019), 155–169. https://doi.org/10.1016/j.indmarman.2018.10.005 doi: 10.1016/j.indmarman.2018.10.005
![]() |
[9] |
I. V. Kozlenkova, G. T. M. Hult, D. J. Lund, J. A. Mena, P. Kekec, The role of marketing channels in supply chain management, J. Retail., 91 (2015), 586–609. https://doi.org/10.1016/j.jretai.2015.03.003 doi: 10.1016/j.jretai.2015.03.003
![]() |
[10] |
G. L. Frazier, Organizing and managing channels of distribution, J. Acad. Mark. Sci., 27 (1999), 226–240. https://doi.org/10.1177/0092070399272007 doi: 10.1177/0092070399272007
![]() |
[11] |
F. Gimeno-Arias, J. M. Santos-Jaén, Using PLS-SEM for assessing negative impact and cooperation as antecedents of gray market in FMCG supply chains: An analysis on Spanish wholesale distributors, Int. J. Phys. Distrib. Logist. Manag., 53 (2023), 718–742. https://doi.org/10.1108/IJPDLM-02-2022-0038 doi: 10.1108/IJPDLM-02-2022-0038
![]() |
[12] |
S. S. Andaleeb, An experimental investigation of satisfaction and commitment in marketing channels: The role of trust and dependence, J. Retail., 72 (1996), 77–93. https://doi.org/10.1016/S0022-4359(96)90006-8 doi: 10.1016/S0022-4359(96)90006-8
![]() |
[13] |
N. Sharma, L. C. Young, I. Wilkinson, The nature and role of different types of commitment in inter-firm relationship cooperation, J. Bus. Ind. Mark., 30 (2015), 45–59. https://doi.org/10.1108/JBIM-11-2012-0202 doi: 10.1108/JBIM-11-2012-0202
![]() |
[14] |
T. Suh, J. C. Jung, G. M. Zank, R. J. Arend, Twofold relationship dynamics of supplier's knowledge sharing, J. Bus. Ind. Mark., 34 (2019), 862–874. https://doi.org/10.1108/JBIM-10-2017-0241 doi: 10.1108/JBIM-10-2017-0241
![]() |
[15] |
A. S. Vinhas, R. Gibbs, Exclusive dealing without territory exclusivity in multichannel systems: Managing channel conflict and driving commitment, Mark. Lett., 29 (2018), 291–305. https://doi.org/10.1007/s11002-018-9462-9 doi: 10.1007/s11002-018-9462-9
![]() |
[16] |
L. Wang, C. Zhang, J. Li, D. Huo, X. Fan, The influence of unilateral supplier transaction-specific investments on international buyer opportunism: empirical findings from local suppliers in China, Int. Mark. Rev., 37 (2020), 213–239. https://doi.org/10.1108/IMR-05-2018-0177 doi: 10.1108/IMR-05-2018-0177
![]() |
[17] | M. Du, J. Zhang, Encouraging customer participation by leveraging interdependence structure and relationship commitments: empirical evidence from chinese industrial firms, Int. J. Bus. Inf., 12 (2017), 433–477. |
[18] |
F. R. Dwyer, P. H. Schurr, S. Oh, Developing buyer-seller relationships, J. Mark., 51 (1987), 11–27. https://doi.org/10.1177/002224298705100202 doi: 10.1177/002224298705100202
![]() |
[19] |
C. Ferro, C. Padin, G. Svensson, J. Payan, Trust and commitment as mediators between economic and non-economic satisfaction in manufacturer-supplier relationships, J. Bus. Ind. Mark., 31 (2016), 13–23. https://doi.org/10.1108/JBIM-07-2013-0154 doi: 10.1108/JBIM-07-2013-0154
![]() |
[20] |
I. Geyskens, J. B. Steenkamp, L. K. Scheer, N. Kumar, The effects of trust and interdependence on relationship commitment: A trans-Atlantic study, Int. J. Res. Mark., 13 (1996), 303–317. https://doi.org/10.1016/S0167-8116(96)00006-7 doi: 10.1016/S0167-8116(96)00006-7
![]() |
[21] |
D. I. Gilliland, D. C. Bello, Two sides to attitudinal commitment: The effect of calculative and loyalty commitment on enforcement mechanisms in distribution channels, J. Acad. Mark. Sci., 30 (2002), 24–43. https://doi.org/10.1177/03079450094306 doi: 10.1177/03079450094306
![]() |
[22] |
N. Kumar, L. K. Scheer, J. B. Steenkamp, The effects of perceived interdependence on dealer attitudes, J. Mark. Res., 32 (1995), 348–356. https://doi.org/10.1177/002224379503200309 doi: 10.1177/002224379503200309
![]() |
[23] |
M. Mpinganjira, M. Roberts-Lombard, G. Svensson, Validating the relationship between trust, commitment, economic and non-economic satisfaction in South African buyer-supplier relationships, J. Bus. Ind. Mark., 32 (2017), 421–431. https://doi.org/10.1108/JBIM-04-2015-0073 doi: 10.1108/JBIM-04-2015-0073
![]() |
[24] |
N. Ponder, B. B. Holloway, J. D. Hansen, The mediating effects of customers' intimacy perceptions on the trust-commitment relationship, J. Serv. Mark., 30 (2016), 75–87. https://doi.org/10.1108/JSM-04-2014-0117 doi: 10.1108/JSM-04-2014-0117
![]() |
[25] |
W. J. Johnston, A. N. H. Le, J. M. S. Cheng, A meta-analytic review of influence strategies in marketing channel relationships, J. Acad. Mark. Sci., 46 (2018), 674–702. https://doi.org/10.1007/s11747-017-0564-3 doi: 10.1007/s11747-017-0564-3
![]() |
[26] |
W. J. Reinartz, M. Berkmann, From customer to partner engagement: a conceptualization and typology of engagement in B2B, Customer Engagement Mark., (2018), 243–268. https://doi.org/10.1007/978-3-319-61985-9_11 doi: 10.1007/978-3-319-61985-9_11
![]() |
[27] |
V. Kumar, A. Pansari, Competitive advantage through engagement, J. Mark. Res., 53 (2016), 497–514. https://doi.org/10.1509/jmr.15.0044 doi: 10.1509/jmr.15.0044
![]() |
[28] |
S. Sands, C. Campbell, C. Ferraro, K. Plangger, Buffering B2B service failure: The role of customer engagement, Ind. Mark. Manag., 103 (2022), 47–60. https://doi.org/10.1016/j.indmarman.2022.03.007 doi: 10.1016/j.indmarman.2022.03.007
![]() |
[29] |
S. D. Vivek, V. Dalela, M. S. Ahmed, A framework for partner engagement: Episodes in the life of interorganizational partnerships, J. Mark. Theory Pract., 30 (2022), 476–493. https://doi.org/10.1080/10696679.2021.191639898 doi: 10.1080/10696679.2021.1916398
![]() |
[30] |
M. W. Nyadzayo, R. Casidy, P. Thaichon, B2B purchase engagement: Examining the key drivers and outcomes in professional services, Ind. Mark. Manag., 85 (2020), 197–208. https://doi.org/10.1016/j.indmarman.2019.11.007 doi: 10.1016/j.indmarman.2019.11.007
![]() |
[31] |
M. Kleinaltenkamp, I. O. Karpen, C. Plewa, E. Jaakkola, J. Conduit, Collective engagement in organizational settings, Ind. Mark. Manag., 80 (2019), 11–23. https://doi.org/10.1016/j.indmarman.2019.02.009 doi: 10.1016/j.indmarman.2019.02.009
![]() |
[32] |
J. F. Hair, M. Sarstedt, L. Hopkins, V. G. Kuppelwieser, Partial least squares structural equation modeling (PLS-SEM): An emerging tool in business research, Eur. Bus. Rev., 26 (2014), 106–121. https://doi.org/10.1108/EBR-10-2013-0128 doi: 10.1108/EBR-10-2013-0128
![]() |
[33] |
J. M. Santos-Jaén, A. León-Gómez, M. del C. Valls Martínez, F. Gimeno-Arias, The effect of public healthcare expenditure on the reduction in mortality rates caused by unhealthy habits among the population, Healthcare, 10 (2022), 2253. https://doi.org/10.3390/healthcare10112253 doi: 10.3390/healthcare10112253
![]() |
[34] |
M. Martínez Ávila, E. Fierro Moreno, Aplicación de la técnica PLS-SEM en la gestión del conocimiento: un enfoque técnico práctico / application of the PLS-SEM technique in knowledge management: A practical technical approach, RIDE Rev. Iberoam. para la Investig. y el Desarro. Educ., 8 (2018), 130–164. https://doi.org/10.23913/ride.v8i16.336 doi: 10.23913/ride.v8i16.336
![]() |
[35] |
P. B. Lowry, J. Gaskin, Partial least squares (PLS) structural equation modeling (SEM) for building and testing behavioral causal theory: When to choose it and how to use it, IEEE Trans. Prof. Commun., 57 (2014), 123–146. https://doi.org/10.1109/TPC.2014.2312452 doi: 10.1109/TPC.2014.2312452
![]() |
[36] |
R. W. Palmatier, R. P. Dant, D. Grewal, K. R. Evans, Factors influencing the effectiveness of relationship marketing: A meta-analysis, J. Mark., 70 (2006), 136–153. https://doi.org/10.1509/jmkg.70.4.136 doi: 10.1509/jmkg.70.4.136
![]() |
[37] |
A. I. Rokkan, J. B. Heide, K. H. Wathne, Specific investments in marketing relationships: expropriation and bonding effects, J. Mark. Res., 40 (2003), 210–224. https://doi.org/10.1509/jmkr.40.2.210.19223 doi: 10.1509/jmkr.40.2.210.19223
![]() |
[38] |
L. W. Stern, T. Reve, Distribution channels as political economies: A framework for comparative analysis, J. Mark., 44 (1980), 52–64. https://doi.org/10.1177/002224298004400306 doi: 10.1177/002224298004400306
![]() |
[39] |
J. C. Anderson, J. A. Narus, A model of distributor firm and manufacturer firm working partnerships, J. Mark., 54 (1990), 42–58. https://doi.org/10.1177/002224299005400103 doi: 10.1177/002224299005400103
![]() |
[40] |
E. Garbarino, M. S. Johnson, The different roles of satisfaction, trust, and commitment in customer relationships, J. Mark., 63 (1999), 70–87. https://doi.org/10.1177/002224299906300205 doi: 10.1177/002224299906300205
![]() |
[41] |
I. Geyskens, J. B. Steenkamp, N. Kumar, A meta-analysis of satisfaction in marketing channel relationships, J. Mark. Res., 36 (1999), 223–238. https://doi.org/10.1177/002224379903600207 doi: 10.1177/002224379903600207
![]() |
[42] | I. R. Macneil, Contracts: Adjustment of long-term economic relations under classical, neoclassical, and relational contract law, Nw. UL Rev., 72 (1977), 854. |
[43] |
D. Narayandas, V. K. Rangan, Building and sustaining buyer–seller relationships in mature industrial markets, J. Mark., 68 (2004), 63–77. https://doi.org/10.1509/jmkg.68.3.63.34772 doi: 10.1509/jmkg.68.3.63.34772
![]() |
[44] |
N. M. Høgevold, G. Svensson, C. Otero-Neira, Validating action and social alignment constituents of collaboration in business relationships, Mark. Intell. Plan., 37 (2019), 721–740. https://doi.org/10.1108/MIP-12-2018-0577 doi: 10.1108/MIP-12-2018-0577
![]() |
[45] |
J. Xue, S. Lu, B. Shi, H. Zheng, Trust, guanxi, and cooperation: A study on partner opportunism in Chinese joint-venture manufacturing, J. Bus. Ind. Mark., 33 (2018), 95–106. https://doi.org/10.1108/JBIM-07-2016-0159 doi: 10.1108/JBIM-07-2016-0159
![]() |
[46] |
Y. Zhou, X. Zhang, G. Zhuang, N. Zhou, Relational norms and collaborative activities: Roles in reducing opportunism in marketing channels, Ind. Mark. Manag., 46 (2015), 147–159. https://doi.org/10.1016/j.indmarman.2015.01.014 doi: 10.1016/j.indmarman.2015.01.014
![]() |
[47] |
J. F. Gaski, N. M. Ray, Alienation in the distribution channel, Int. J. Phys. Distrib. Logist. Manag., 34 (2004), 158–200. https://doi.org/10.1108/09600030410526941 doi: 10.1108/09600030410526941
![]() |
[48] |
J. Crosno, R. Dahlstrom, Y. Liu, P. Y. Tong, Effectiveness of contracts in marketing exchange relationships: A meta-analytic review, Ind. Mark. Manag., 92 (2021), 122–139. https://doi.org/10.1016/j.indmarman.2020.11.007 doi: 10.1016/j.indmarman.2020.11.007
![]() |
[49] |
R. Vázquez-Casielles, V. Iglesias, C. Varela-Neira, Manufacturer–distributor relationships: Role of relationship-specific investment and dependence types, J. Bus. Ind. Mark., 32 (2017), 1245–1260. https://doi.org/10.1108/JBIM-10-2016-0244 doi: 10.1108/JBIM-10-2016-0244
![]() |
[50] | N. Sharma, An examination of customer relationship value in high vs low technology industries, Acad. Mark. Stud. J., 24 (2020), 1–24. |
[51] |
J. C. Anderson, J. A. Narus, A model of the distributor's perspective of distributor-manufacturer working relationships, J. Mark., 48 (1984), 62–74. https://doi.org/10.1177/002224298404800407 doi: 10.1177/002224298404800407
![]() |
[52] |
K. H. Wathne, J. B. Heide, Opportunism in interfirm relationships: Forms, outcomes, and solutions, J. Mark., 64 (2000), 36–51. https://doi.org/10.1509/jmkg.64.4.36.18070 doi: 10.1509/jmkg.64.4.36.18070
![]() |
[53] |
J. B. Heide, G. John, The role of dependence balancing in safeguarding transaction-specific assets in conventional channels, J. Mark., 52 (1988), 20-35. https://doi.org/10.1177/002224298805200103 doi: 10.1177/002224298805200103
![]() |
[54] |
S. Ganesan, S. P. Brown, B. J. Mariadoss, H. Ho, Buffering and amplifying effects of relationship commitment in business-to-business relationships, J. Mark. Res., 47 (2010), 361–373. https://doi.org/10.1509/jmkr.47.2.361 doi: 10.1509/jmkr.47.2.361
![]() |
[55] |
J. R. Brown, R. F. Lusch, C. Y. Nicholson, Power and relationship commitment: their impact on marketing channel member performance, J. Retail., 71 (1995), 363–392. https://doi.org/10.1016/0022-4359(95)90019-5 doi: 10.1016/0022-4359(95)90019-5
![]() |
[56] | A. T. Coughlan, E. Anderson, L. W. Stern, A. El-Ansary, Marketing Channels, Prentice Hall, 2006. |
[57] |
R. Grewal, G. L. Lilien, S. Bharadwaj, P. Jindal, U. Kayande, R. F. Lusch, et al., Business-to-business buying: Challenges and opportunities, Cust. Needs Solut., 2 (2015), 193–208. https://doi.org/10.1007/s40547-015-0040-5 doi: 10.1007/s40547-015-0040-5
![]() |
[58] | S. D. Vivek, V. Dalela, S. E. Beatty, Partner engagement: A perspective on B2B engagement, Customer Engagement, Routledge (2015), 53–66. |
[59] |
R. W. Palmatier, R. P. Dant, D. Grewal, A comparative longitudinal analysis of theoretical perspectives of interorganizational relationship performance, J. Mark., 71 (2007), 172–194. https://doi.org/10.1509/jmkg.71.4.172 doi: 10.1509/jmkg.71.4.172
![]() |
[60] |
A. Pansari, V. Kumar, Customer engagement: the construct, antecedents, and consequences, J. Acad. Mark. Sci., 45 (2017), 294–311. https://doi.org/10.1007/s11747-016-0485-6 doi: 10.1007/s11747-016-0485-6
![]() |
[61] |
S. Vatavwala, B. Kumar, A. Sharma, A. Billore, A. Sadh, Customer disengagement in business-to-business markets: A framework for analysis, Ind. Mark. Manag., 105 (2022), 114–130. https://doi.org/10.1016/j.indmarman.2022.05.018 doi: 10.1016/j.indmarman.2022.05.018
![]() |
[62] |
J. Barnes, Y. Liao, The effect of individual, network, and collaborative competencies on the supply chain management system, Int. J Prod. Econ., 140 (2012), 888–899. https://doi.org/10.1016/j.ijpe.2012.07.010 doi: 10.1016/j.ijpe.2012.07.010
![]() |
[63] |
E. W. Choi, Ö. Özer, Y. Zheng, Network trust and trust behaviors among executives in supply chain interactions, Manag. Sci., 66 (2020), 5823–5849. https://doi.org/10.1287/mnsc.2019.3499 doi: 10.1287/mnsc.2019.3499
![]() |
[64] |
S. Ambulkar, J. V. Blackhurst, D. E. Cantor, Supply chain risk mitigation competency: An individual-level knowledge-based perspective, Int. J Prod. Res., 54 (2016), 1398–1411. https://doi.org/10.1080/00207543.2015.1070972 doi: 10.1080/00207543.2015.1070972
![]() |
[65] |
D. M. Hardesty, W. O. Bearden, The use of expert judges in scale development: Implications for improving face validity of measures of unobservable constructs, J. Bus. Res., 57 (2004), 98–107. https://doi.org/10.1016/S0148-2963(01)00295-8 doi: 10.1016/S0148-2963(01)00295-8
![]() |
[66] |
P. M. Podsakoff, S. B. MacKenzie, J. Y. Lee, N. P. Podsakoff, Common method biases in behavioral research: A critical review of the literature and recommended remedies, J. Appl. Psychol., 88 (2003), 879–903. https://doi.org/10.1037/0021-9010.88.5.879 doi: 10.1037/0021-9010.88.5.879
![]() |
[67] |
J. S. Armstrong, T. S. Overton, Estimating nonresponse bias in mail surveys, J. Mark. Res., 14 (1977), 396–402. https://doi.org/10.1177/002224377701400320 doi: 10.1177/002224377701400320
![]() |
[68] |
S. Cai, M. Jun, Z. Yang, The effects of boundary Spanners' personal relationships on interfirm collaboration and conflict: A study of the role of Guanxi in China, J. Supply Chain Manag., 53 (2017), 19–40. https://doi.org/10.1111/jscm.12132 doi: 10.1111/jscm.12132
![]() |
[69] |
M. Sarstedt, J. F. Hair, C. M. Ringle, K. O. Thiele, S. P. Gudergan, Estimation issues with PLS and CBSEM: Where the bias lies!, J. Bus. Res., 69 (2016), 3998–4010. https://doi.org/10.1016/j.jbusres.2016.06.007 doi: 10.1016/j.jbusres.2016.06.007
![]() |
[70] |
A. Leguina, A primer on partial least squares structural equation modeling (PLS-SEM), Int. J. Res. Method Educ., 38 (2015), 220–221. https://doi.org/10.1080/1743727X.2015.1005806 doi: 10.1080/1743727X.2015.1005806
![]() |
[71] |
J. F. Hair, C. M. Ringle, M. Sarstedt, PLS-SEM: Indeed a silver bullet, J. Mark. Theory Pract., 19 (2011), 139–152. https://doi.org/10.2753/MTP1069-6679190202 doi: 10.2753/MTP1069-6679190202
![]() |
[72] | A. C. Davison, D. V. Hinkley, Bootstrap Methods And Their Application, Cambridge University Press, UK, 1977. |
[73] |
B. Efron, R. Tibshirani, Bootstrap methods for standard errors, confidence intervals, and other measures of statistical accuracy, Stat. Sci., 1 (1986), 54–75. https://doi.org/10.1214/ss/1177013815 doi: 10.1214/ss/1177013815
![]() |
[74] |
D. Kushary, Bootstrap methods and their application, Technometrics, 42 (2000), 216–217. https://doi.org/10.1080/00401706.2000.10486018 doi: 10.1080/00401706.2000.10486018
![]() |
[75] |
J. F. Hair Jr, M. Sarstedt, Factors versus composites: Guidelines for choosing the right structural equation modeling method, Proj. Manag. J., 50 (2019), 619–624. https://doi.org/10.1177/8756972819882132 doi: 10.1177/8756972819882132
![]() |
[76] |
D. Ruiz-Palomo, J. Diéguez-Soto, A. Duréndez, J. A. C Santos, Family management and firm performance in family SMEs: The mediating roles of management control systems and technological innovation, Sustainability, 11 (2019), 3805. https://doi.org/10.3390/su11143805 doi: 10.3390/su11143805
![]() |
[77] |
M. Sarstedt, C. M. Ringle, J. H. Cheah, H. Ting, O. I. Moisescu, L. Radomir, Structural model robustness checks in PLS-SEM, Tour. Econ., 26 (2020), 531–554. https://doi.org/10.1177/1354816618823921 doi: 10.1177/1354816618823921
![]() |
[78] | C. M. Ringle, S. Wende, J. M. Baker, Smart-Pls, SmartPLS 4. Oststeinbek: SmartPLS, (2022). Available from: https://www.smartpls.com. |
[79] |
S. Streukens, S. Leroi-Werelds, Bootstrapping and PLS-SEM: A step-by-step guide to get more out of your bootstrap results, Eur. Manag. J., 34 (2016), 618–632. https://doi.org/10.1016/j.emj.2016.06.003 doi: 10.1016/j.emj.2016.06.003
![]() |
[80] |
S. Mayr, E. Erdfelder, A. Buchner, F. Faul, A short tutorial of GPower, Tutor. Quant. Methods Psychol., 3 (2007), 51–59. https://doi.org/10.20982/tqmp.03.2.p051 doi: 10.20982/tqmp.03.2.p051
![]() |
[81] | J. Cohen, Statistical Power Analysis for the Behavioral Sciences, Erbaum Press, Hillsdale, NJ, USA, 2013. https://doi.org/10.20982/tqmp.03.2.p051 |
[82] | M. Sarstedt, C. M. Ringle, J. F. Hair, Partial least squares structural equation modeling, in Handbook of Market Research, Cham, Springer (2022), 587–632. https://doi.org/10.1007/978-3-319-57413-4_15 |
[83] |
J. Henseler, G. Hubona, P. A. Ray, Using PLS path modeling in new technology research: Updated guidelines, Ind. Manag. Data Syst., 116 (2016), 2–20. https://doi.org/10.1108/IMDS-09-2015-0382 doi: 10.1108/IMDS-09-2015-0382
![]() |
[84] |
J. Henseler, On the convergence of the partial least squares path modeling algorithm, Comput. Stat., 25 (2010), 107–120. https://doi.org/10.1007/s00180-009-0164-x doi: 10.1007/s00180-009-0164-x
![]() |
[85] |
T. K. Dijkstra, J. Henseler, Consistent partial least squares path modeling, MIS Q. Manag. Inf. Syst., 39 (2015), 297–316. https://doi.org/10.25300/MISQ/2015/39.2.02 doi: 10.25300/MISQ/2015/39.2.02
![]() |
[86] |
J. Henseler, C. M. Ringle, M. Sarstedt, A new criterion for assessing discriminant validity in variance-based structural equation modeling, J. Acad. Mark. Sci., 43 (2015), 115–135. https://doi.org/10.1007/s11747-014-0403-8 doi: 10.1007/s11747-014-0403-8
![]() |
[87] |
J. F. Hair, J. J. Risher, M. Sarstedt, C. M. Ringle, When to use and how to report the results of PLS-SEM, Eur. Bus. Rev., 31 (2019), 2–24. https://doi.org/10.1108/EBR-11-2018-0203 doi: 10.1108/EBR-11-2018-0203
![]() |
[88] |
C. Fornell, D. F. Larcker, Evaluating structural equation models with unobservable variables and measurement error, J. Mark. Res., 18 (1981), 39–50. https://doi.org/10.1177/002224378101800104 doi: 10.1177/002224378101800104
![]() |
[89] | J. Henseler, Some inconvenient truths about PLS path modeling, 9th Int. Conf. PLS Relat. Methods, (2016), 74–76. |
[90] |
L. T. Hu, P. M. Bentler, Fit indices in covariance structure modeling: Sensitivity to underparameterized model misspecification, Psychol. Methods, 3 (1998), 424–453. https://doi.org/10.1037/1082-989X.3.4.424 doi: 10.1037/1082-989X.3.4.424
![]() |
[91] | W. W. Chin, How to write up and report pls analyses, in Handbook of Partial Least Squares, Springer, (2010), 655–690. https://doi.org/10.1007/978-3-540-32827-8_29 |
[92] |
G. Shmueli, To explain or to predict?, Stat. Sci., 25 (2010), 289–310. https://doi.org/10.1214/10-STS330 doi: 10.1214/10-STS330
![]() |
[93] |
D. Straub, D. Gefen, Validation guidelines for is positivist research, Commun. Assoc. Inf. Syst., 13 (2004), 380–427. https://doi.org/10.17705/1CAIS.01324 doi: 10.17705/1CAIS.01324
![]() |
[94] |
J. Evermann, M. Tate, Assessing the predictive performance of structural equation model estimators, J. Bus. Res., 69 (2016), 4565–4582. https://doi.org/10.1016/j.jbusres.2016.03.050 doi: 10.1016/j.jbusres.2016.03.050
![]() |
[95] |
G. Shmueli, M. Sarstedt, J. F. Hair, J. Cheah, H. Ting, S. Vaithilingam, et al., Predictive model assessment in PLS-SEM: guidelines for using PLSpredict, Eur. J. Mark., 53 (2019), 2322–2347. https://doi.org/10.1108/EJM-02-2019-0189 doi: 10.1108/EJM-02-2019-0189
![]() |
[96] |
J. F. Hair, M. C. Howard, C. Nitzl, Assessing measurement model quality in PLS-SEM using confirmatory composite analysis, J. Bus. Res., 109 (2020), 101–110. https://doi.org/10.1016/j.jbusres.2019.11.069 doi: 10.1016/j.jbusres.2019.11.069
![]() |
[97] |
A. Calvo-Mora, A. Blanco-Oliver, J. L. Roldán, R. Periáñez-Cristóbal, TQM factors and organisational results in the EFQM excellence model framework: an explanatory and predictive analysis, Ind. Manag. Data Syst., 120 (2020), 2297–2317. https://doi.org/10.1108/IMDS-12-2019-0701 doi: 10.1108/IMDS-12-2019-0701
![]() |
[98] |
C. M. Felipe, J. L. Roldán, A. L. Leal-Rodríguez, Impact of organizational culture values on organizational agility, Sustainability, 9 (2017), 2354. https://doi.org/10.3390/su9122354 doi: 10.3390/su9122354
![]() |
[99] |
A. G. Woodside, Moving beyond multiple regression analysis to algorithms: Calling for adoption of a paradigm shift from symmetric to asymmetric thinking in data analysis and crafting theory, J. Bus. Res., 66 (2013), 463–472. https://doi.org/10.1016/j.jbusres.2012.12.021 doi: 10.1016/j.jbusres.2012.12.021
![]() |
[100] |
A. Martín-Navarro, F. Velicia-Martín, J. A. Medina-Garrido, R. G. Rodrigues, Causal propensity as an antecedent of entrepreneurial intentions, Int. Entrep. Manag. J., 19 (2023), 501–522. https://doi.org/10.1007/s11365-022-00826-1 doi: 10.1007/s11365-022-00826-1
![]() |
1. | Carlos Ferro-Soto, Carmen Padin, Mornay Roberts-Lombard, Göran Svensson, Nils Høgevold, Economic and non-economic satisfaction as interlocking constructs in B2B sales relationships, 2024, 55, 2078-5976, 10.4102/sajbm.v55i1.3956 | |
2. | Eithel F. Bonilla-Chaves, Pedro R. Palos-Sánchez, José A. Folgado-Fernández, Jorge A. Marino-Romero, The effect of innovation performance on the adoption of human resources analytics in business organizations, 2024, 32, 2688-1594, 1126, 10.3934/era.2024054 |
MEASURE | CATEGORY | N | PERCENT |
Gender of respondent | Male | 171 | 83.82% |
Female | 33 | 16.18% | |
Firm Size* | Small (<50 employees) | 87 | 42.65% |
Medium (50−250 employees) | 28 | 13.73% | |
Large (>250 employees) | 89 | 43.62% | |
Distributor type | Wholesale | 95 | 46.57% |
Retail | 53 | 25.98% | |
Both | 56 | 27.45% | |
*Note: (*) European Union (EU) classification |
TRUST (Sharma, 2020; Suh et al., 2019) [22,50] | |
TRUST 1 | We feel we can trust this supplier completely |
TRUST 2 | We have full confidence in the information provided to us by this supplier |
TRUST 3 | We have complete confidence in this supplier's motives |
TRUST 4 | The supplier keeps the promises it makes to our firms |
TRUST 5 | When it comes to things that are important to us, we can depend on the supplier's support |
DEPENDENCE (Cai et al., 2017; Du & Zhang, 2017; Vázquez-Casielles et al., 2017) [13,49,68] | |
DEP 1 | Our company is quite dependent on this supplier |
DEP 2 | If this relationship ended, our company would face a significant loss |
DEP 3 | The supplier provides vital resources our company would find difficult to obtain elsewhere |
DEP 4 | It would be very difficult to replace the sales and profits generated by this manufacturer's products |
DEP 5 | If our relationship was discontinued with the manufacturer, it would be difficult for us to find an alternative supplier |
COOPERATION (Gaski & Ray, 2004; Gimeno-Arias & Santos-Jaén, 2022; Xue et al., 2018) [11,45,47] | |
COOP 1 | We share information with our supplier |
COOP 2 | We participate in joint goal setting and forecasting with our supplier |
COOP 3 | This supplier is generally willing to cooperate with us |
COOP 4 | This supplier provides adequate help in selling his products |
COOP 5 | We have a good working relationship with this supplier |
COOP 6 | We view the success of the supplier as our own success |
AFFECTIVE COMMITMENT (Gilliland & Bello, 2002; Sharma et al., 2015) [17,21] | |
LOY_COM 1 | Our company believes that this supplier acts in our best interest |
LOY_COM 2 | We have positive feelings towards this supplier |
LOY_COM 3 | Our loyalty to this supplier is among the primary reasons for our continued collaboration |
LOY_COM 4 | We wish to maintain our association with this supplier due to our strong loyalty |
LOY_COM 5 | We intend to continue working with this supplier because we feel that they are "part of the family" |
CALCULATIVE COMMITMENT (Du & Zhang, 2017; Sharma et al., 2015) [13,21] | |
CAL_COM 1 | We currently have no alternatives to this relationship |
CAL_COM 2 | It would be very difficult for us to find a replacement for this supplier |
CAL_COM 3 | Changing from this supplier to another would cost us too much |
CAL_COM 4 | We want to maintain the relationship with this supplier because it is difficult to find similar suppliers |
CAL_COM 5 | We need to maintain the relationship with this supplier because ending it would result in significant losses. |
B2B ENGAGEMENT (Kumar & Pansari, 2016) [27] | |
ENGA 1 | I love talking about my experience with this supplier |
ENGA 2 | I feel a sense of belonging towards this supplier |
ENGA 3 | When referring to this supplier, I usually say "we" rather than "they" |
ENGA 4 | This supplier holds significant personal significance for us |
Indicator | Mean | SD | Loading | t-student* | Indicator | Mean | SD | Loading | t-student* | |
CAL COM | DEP | |||||||||
CAL COM1 | 3.438 | 1.774 | 0.843 | 23.777 | DEP1 | 4.300 | 1.490 | 0.778 | 22.804 | |
CAL COM2 | 3.627 | 1.793 | 0.930 | 80.461 | DEP2 | 3.970 | 1.740 | 0.918 | 78.527 | |
CAL COM3 | 3.824 | 1.852 | 0.935 | 93.530 | DEP3 | 3.794 | 1.803 | 0.891 | 36.583 | |
CAL COM4 | 3.926 | 1.774 | 0.911 | 53.192 | DEP4 | 3.897 | 1.676 | 0.904 | 58.519 | |
CAL COM5 | 3.665 | 1.846 | 0.909 | 46.168 | DEP5 | 3.716 | 1.809 | 0.844 | 31.230 | |
TRUST | ENGA | |||||||||
CONF1 | 5.338 | 1.298 | 0.929 | 84.323 | ENGA1 | 4.862 | 1.537 | 0.817 | 28.227 | |
CONF2 | 5.404 | 1.334 | 0.916 | 56.846 | ENGA2 | 4.198 | 1.782 | 0.904 | 50.228 | |
CONF3 | 5.182 | 1.376 | 0.912 | 64.906 | ENGA3 | 4.778 | 1.725 | 0.477 | 6.542 | |
CONF4 | 5.588 | 1.211 | 0.854 | 37.282 | ENGA4 | 4.044 | 1.981 | 0.875 | 37.506 | |
CONF5 | 5.696 | 1.195 | 0.851 | 30.836 | ENGA5 | 4.308 | 1.893 | 0.885 | 37.980 | |
COOP | LOY COM | |||||||||
COOP1 | 5.456 | 1.377 | 0.843 | 36.006 | LOY COM1 | 5.162 | 1.256 | 0.802 | 28.622 | |
COOP2 | 5.127 | 1.591 | 0.808 | 28.233 | LOY COM2 | 5.417 | 1.212 | 0.830 | 36.773 | |
COOP3 | 5.794 | 1.320 | 0.846 | 28.382 | LOY COM3 | 4.917 | 1.478 | 0.845 | 30.462 | |
COOP4 | 5.534 | 1.373 | 0.842 | 25.834 | LOY COM4 | 4.824 | 1.471 | 0.876 | 44.212 | |
COOP5 | 5.887 | 1.205 | 0.883 | 51.894 | LOY COM5 | 4.455 | 1.715 | 0.816 | 31.411 | |
*Note: Significance and standard deviations (SD) performed by 10,000 repetitions bootstrapping procedure ∗∗∗: All loadings are significant at a 0.001 level. |
Composites | α | ρA | ρC | AVE |
CAL COM | 0.945 | 0.951 | 0.958 | 0.821 |
TRUST | 0.875 | 0.893 | 0.909 | 0.632 |
COOP | 0.918 | 0.922 | 0.939 | 0.754 |
DEP | 0.893 | 0.894 | 0.926 | 0.758 |
ENGA | 0.891 | 0.893 | 0.920 | 0.696 |
LOY COM | 0.936 | 0.938 | 0.952 | 0.798 |
*Note: α: Chronbach's alpha; ρA: Dijkstra–Henseler's composite reliability; ρC: Jöreskog's composite reliability; AVE: average variance extracted. |
Ⅰ | Ⅱ | Ⅲ | Ⅳ | Ⅴ | Ⅵ | ||
Ⅰ | CAL COM | 0.906 | |||||
Ⅱ | COOP | 0.043 | 0.795 | ||||
Ⅲ | DEP | 0.828 | 0.175 | 0.868 | |||
Ⅳ | ENGA | 0.398 | 0.448 | 0.429 | 0.871 | ||
Ⅴ | LOY COM | 0.253 | 0.689 | 0.344 | 0.633 | 0.834 | |
Ⅵ | TRUST | 0.107 | 0.780 | 0.208 | 0.396 | 0.722 | 0.893 |
*Note: Fornell–Larcker criterion: square root of AVE in diagonal (bold) and construct correlations below the diagonal. |
Ⅰ | Ⅱ | Ⅲ | Ⅳ | Ⅴ | ||
Ⅰ | CAL COM | |||||
Ⅱ | COOP | 0.102 | ||||
Ⅲ | DEP | 0.784 | 0.212 | |||
Ⅳ | ENGA | 0.428 | 0.542 | 0.473 | ||
Ⅴ | LOY COM | 0.278 | 0.775 | 0.388 | 0.713 | |
Ⅵ | TRUST | 0.117 | 0.843 | 0.228 | 0.430 | 0.770 |
CAL_COM | COOP | ENGA | LOY_COM | TRUST | |
CAL_COM | 1.068 | 1.189 | |||
COOP | |||||
DEP | 1.000 | 1.228 | |||
ENGA | 1.394 | ||||
LOY_COM | 1.068 | 1.000 | |||
TRUST | 1.189 | 1.000 |
CI | ||||||||
Path | SD | T-value | 5% | 95% | f2 | H | Supported | |
Direct effects | ||||||||
TRUST - > COOP | 0.717 | 0.050 | 14.286*** | 0.629 | 0.794 | 1.177 | H1 | YES |
DEP - > COOP | −0.054 | 0.053 | 1.007ns | −0.142 | 0.033 | 0.006 | H2 | NO |
TRUST - > LOY COM | 0.722 | 0.034 | 20.946*** | 0.663 | 0.776 | 1.091 | H3 | YES |
DEP - > CAL COM | 0.828 | 0.026 | 31.289*** | 0.782 | 0.870 | 2.188 | H4 | YES |
LOY_COM - > ENGA | 0.569 | 0.057 | 9.962*** | 0.474 | 0.661 | 0.561 | H5 | YES |
CAL_COM - > ENGA | 0.254 | 0.061 | 4.163*** | 0.152 | 0.354 | 0.112 | H6 | YES |
ENGA - > COOP | 0.187 | 0.065 | 2.872*** | 0.078 | 0.291 | 0.068 | H9 | YES |
Indirect effects | ||||||||
Individual indirect effects | VAF | |||||||
TRUST - > LOY COM - > ENGA | 0.411 | 0.046 | 9.021*** | 0.336 | 0.487 | 100% | H7 | YES |
DEP - > CAL COM - > ENGA | 0.210 | 0.052 | 4.079*** | 0.126 | 0.296 | 100% | H8 | YES |
TRUST - > LOY COM - > ENGA - > COOP | 0.077 | 0.030 | 2.597** | 0.030 | 0.127 | 10% | H10 | YES |
DEP - > CAL COM - > ENGA - > COOP | 0.039 | 0.015 | 2.657** | 0.016 | 0.064 | 100% | H11 | YES |
CAL COM - > ENGA - > COOP | 0.047 | 0.017 | 2.715** | 0.019 | 0.077 | 100% | ||
LOY COM - > ENGA - > COOP | 0.106 | 0.042 | 2.555** | 0.041 | 0.178 | 100% | ||
Indirect effects | ||||||||
CAL COM - > COOP | 0.047 | 0.017 | 2.715** | 0.019 | 0.077 | 100% | ||
DEP - > COOP | 0.039 | 0.015 | 2.657** | 0.016 | 0.064 | 100% | ||
DEP - > ENGA | 0.210 | 0.052 | 4.079*** | 0.126 | 0.296 | 100% | ||
LOY COM - > COOP | 0.106 | 0.042 | 2.555** | 0.041 | 0.178 | 100% | ||
TRUST - > COOP | 0.077 | 0.030 | 2.597** | 0.030 | 0.127 | 10% | ||
TRUST - > ENGA | 0.411 | 0.046 | 9.021*** | 0.336 | 0.487 | 100% | ||
Total effects | ||||||||
CAL_COM - > COOP | 0.047 | 0.017 | 2.715** | 0.019 | 0.077 | |||
DEP - > COOP | -0.014 | 0.050 | 0.288ns | -0.099 | 0.067 | |||
DEP - > ENGA | 0.210 | 0.052 | 4.079*** | 0.126 | 0.296 | |||
LOY_COM - > COOP | 0.106 | 0.042 | 2.555** | 0.041 | 0.178 | |||
TRUST - > COOP | 0.793 | 0.038 | 20.750*** | 0.726 | 0.851 | |||
TRUST - > ENGA | 0.411 | 0.046 | 9.021*** | 0.336 | 0.487 | |||
*Note: R2 [95% CI in brackets]: CAL COM: 0.685 [0.610; 0.756]; COOP: 0.627 [0.542; 0.715]; ENGA: 0.455 [0.367; 0.556]; LOY COM: 0.519 [0.437; 0.601]. f2: size effect index; 95PCI:95% percentile Confidence Interval; Significance, standard deviations, 95% bias-corrected CIs were performed by 10,000 repetitions bootstrapping procedure; *: p < 0.05; **: p < 0.01; ***: p < 0.001. Only total effects that differ from direct effects are showed. |
CONSTRUCT PREDICTION SUMMARY | |||
Construct | Q2predict | RMSE | MAE |
CAL_COM | 0.683 | 0.569 | 0.434 |
COOP | 0.598 | 0.643 | 0.493 |
ENGA | 0.244 | 0.879 | 0.723 |
LOY_COM | 0.518 | 0.703 | 0.563 |
t-value | DF | p | ||
COOP1 | –0.0872 | 202 | 0.931 | |
COOP2 | –0.9342 | 202 | 0.351 | |
COOP3 | –1.9145 | ᵃ | 202 | 0.057 |
COOP4 | –0.5812 | 202 | 0.562 | |
COOP5 | –1.3219 | ᵃ | 202 | 0.188 |
TRUST1 | –1.3942 | ᵃ | 202 | 0.165 |
TRUST2 | –0.9529 | ᵃ | 201 | 0.342 |
TRUST3 | –0.8446 | ᵃ | 201 | 0.399 |
TRUST4 | –0.4036 | 202 | 0.687 | |
TRUST5 | –1.7356 | ᵃ | 202 | 0.084 |
DEP1 | 2.1116 | 201 | 0.036 | |
DEP2 | 1.5257 | 201 | 0.129 | |
DEP3 | 1.6130 | 202 | 0.108 | |
DEP4 | 1.7877 | 201 | 0.075 | |
DEP5 | 1.5789 | 202 | 0.116 | |
COM_AF1 | –2.0705 | ᵃ | 202 | 0.040 |
COM_AF2 | –0.9646 | 202 | 0.336 | |
COM_AF3 | 0.6913 | 202 | 0.490 | |
COM_AF4 | 1.5656 | 202 | 0.119 | |
COM_AF5 | 1.9051 | 200 | 0.058 | |
COM_EC1 | 2.5842 | 201 | 0.010 | |
COM_EC2 | 2.1232 | 202 | 0.035 | |
COM_EC3 | 3.1196 | 202 | 0.002 | |
COM_EC4 | 2.2717 | 202 | 0.024 | |
COM_EC5 | 1.7581 | 201 | 0.080 | |
ENGA1 | 1.0283 | 201 | 0.305 | |
ENGA2 | 1.1652 | 200 | 0.245 | |
ENGA3 | 0.8423 | 201 | 0.401 | |
ENGA4 | 1.6134 | 201 | 0.108 | |
ENGA5 | 1.8234 | 199 | 0.070 |
t-value | DF | p | ||
COOP1 | 1.6666 | 202 | 0.097 | |
COOP2 | 1.3381 | 202 | 0.182 | |
COOP3 | 1.9101 | ᵃ | 202 | 0.058 |
COOP4 | 1.3328 | ᵃ | 202 | 0.184 |
COOP5 | 0.6725 | 202 | 0.502 | |
COOP6 | –0.8988 | 201 | 0.370 | |
TRUST1 | 0.7535 | 202 | 0.452 | |
TRUST2 | 0.1883 | 201 | 0.851 | |
TRUST3 | 0.5513 | 201 | 0.582 | |
TRUST4 | 0.6898 | 202 | 0.491 | |
TRUST5 | 0.1537 | 202 | 0.878 | |
DEP1 | –0.0106 | 201 | 0.992 | |
DEP2 | –0.4337 | 201 | 0.665 | |
DEP3 | 0.4415 | 202 | 0.659 | |
DEP4 | 0.6301 | 201 | 0.529 | |
DEP5 | 0.7980 | 202 | 0.426 | |
COM_AF1 | 0.8056 | 202 | 0.421 | |
COM_AF2 | 1.0568 | ᵃ | 202 | 0.292 |
COM_AF3 | 2.3686 | ᵃ | 202 | 0.019 |
COM_AF4 | 2.5038 | ᵃ | 202 | 0.013 |
COM_AF5 | 2.3194 | 200 | 0.021 | |
COM_EC1 | –0.3202 | 201 | 0.749 | |
COM_EC2 | 0.0745 | 202 | 0.941 | |
COM_EC3 | –0.1863 | 202 | 0.852 | |
COM_EC4 | 0.4881 | 202 | 0.626 | |
COM_EC5 | –0.6967 | 201 | 0.487 | |
ENGA1 | 0.3217 | 201 | 0.748 | |
ENGA2 | 0.7979 | 200 | 0.426 | |
ENGA3 | 0.5233 | 201 | 0.601 | |
ENGA4 | 0.5911 | 199 | 0.555 |
MEASURE | CATEGORY | N | PERCENT |
Gender of respondent | Male | 171 | 83.82% |
Female | 33 | 16.18% | |
Firm Size* | Small (<50 employees) | 87 | 42.65% |
Medium (50−250 employees) | 28 | 13.73% | |
Large (>250 employees) | 89 | 43.62% | |
Distributor type | Wholesale | 95 | 46.57% |
Retail | 53 | 25.98% | |
Both | 56 | 27.45% | |
*Note: (*) European Union (EU) classification |
TRUST (Sharma, 2020; Suh et al., 2019) [22,50] | |
TRUST 1 | We feel we can trust this supplier completely |
TRUST 2 | We have full confidence in the information provided to us by this supplier |
TRUST 3 | We have complete confidence in this supplier's motives |
TRUST 4 | The supplier keeps the promises it makes to our firms |
TRUST 5 | When it comes to things that are important to us, we can depend on the supplier's support |
DEPENDENCE (Cai et al., 2017; Du & Zhang, 2017; Vázquez-Casielles et al., 2017) [13,49,68] | |
DEP 1 | Our company is quite dependent on this supplier |
DEP 2 | If this relationship ended, our company would face a significant loss |
DEP 3 | The supplier provides vital resources our company would find difficult to obtain elsewhere |
DEP 4 | It would be very difficult to replace the sales and profits generated by this manufacturer's products |
DEP 5 | If our relationship was discontinued with the manufacturer, it would be difficult for us to find an alternative supplier |
COOPERATION (Gaski & Ray, 2004; Gimeno-Arias & Santos-Jaén, 2022; Xue et al., 2018) [11,45,47] | |
COOP 1 | We share information with our supplier |
COOP 2 | We participate in joint goal setting and forecasting with our supplier |
COOP 3 | This supplier is generally willing to cooperate with us |
COOP 4 | This supplier provides adequate help in selling his products |
COOP 5 | We have a good working relationship with this supplier |
COOP 6 | We view the success of the supplier as our own success |
AFFECTIVE COMMITMENT (Gilliland & Bello, 2002; Sharma et al., 2015) [17,21] | |
LOY_COM 1 | Our company believes that this supplier acts in our best interest |
LOY_COM 2 | We have positive feelings towards this supplier |
LOY_COM 3 | Our loyalty to this supplier is among the primary reasons for our continued collaboration |
LOY_COM 4 | We wish to maintain our association with this supplier due to our strong loyalty |
LOY_COM 5 | We intend to continue working with this supplier because we feel that they are "part of the family" |
CALCULATIVE COMMITMENT (Du & Zhang, 2017; Sharma et al., 2015) [13,21] | |
CAL_COM 1 | We currently have no alternatives to this relationship |
CAL_COM 2 | It would be very difficult for us to find a replacement for this supplier |
CAL_COM 3 | Changing from this supplier to another would cost us too much |
CAL_COM 4 | We want to maintain the relationship with this supplier because it is difficult to find similar suppliers |
CAL_COM 5 | We need to maintain the relationship with this supplier because ending it would result in significant losses. |
B2B ENGAGEMENT (Kumar & Pansari, 2016) [27] | |
ENGA 1 | I love talking about my experience with this supplier |
ENGA 2 | I feel a sense of belonging towards this supplier |
ENGA 3 | When referring to this supplier, I usually say "we" rather than "they" |
ENGA 4 | This supplier holds significant personal significance for us |
Indicator | Mean | SD | Loading | t-student* | Indicator | Mean | SD | Loading | t-student* | |
CAL COM | DEP | |||||||||
CAL COM1 | 3.438 | 1.774 | 0.843 | 23.777 | DEP1 | 4.300 | 1.490 | 0.778 | 22.804 | |
CAL COM2 | 3.627 | 1.793 | 0.930 | 80.461 | DEP2 | 3.970 | 1.740 | 0.918 | 78.527 | |
CAL COM3 | 3.824 | 1.852 | 0.935 | 93.530 | DEP3 | 3.794 | 1.803 | 0.891 | 36.583 | |
CAL COM4 | 3.926 | 1.774 | 0.911 | 53.192 | DEP4 | 3.897 | 1.676 | 0.904 | 58.519 | |
CAL COM5 | 3.665 | 1.846 | 0.909 | 46.168 | DEP5 | 3.716 | 1.809 | 0.844 | 31.230 | |
TRUST | ENGA | |||||||||
CONF1 | 5.338 | 1.298 | 0.929 | 84.323 | ENGA1 | 4.862 | 1.537 | 0.817 | 28.227 | |
CONF2 | 5.404 | 1.334 | 0.916 | 56.846 | ENGA2 | 4.198 | 1.782 | 0.904 | 50.228 | |
CONF3 | 5.182 | 1.376 | 0.912 | 64.906 | ENGA3 | 4.778 | 1.725 | 0.477 | 6.542 | |
CONF4 | 5.588 | 1.211 | 0.854 | 37.282 | ENGA4 | 4.044 | 1.981 | 0.875 | 37.506 | |
CONF5 | 5.696 | 1.195 | 0.851 | 30.836 | ENGA5 | 4.308 | 1.893 | 0.885 | 37.980 | |
COOP | LOY COM | |||||||||
COOP1 | 5.456 | 1.377 | 0.843 | 36.006 | LOY COM1 | 5.162 | 1.256 | 0.802 | 28.622 | |
COOP2 | 5.127 | 1.591 | 0.808 | 28.233 | LOY COM2 | 5.417 | 1.212 | 0.830 | 36.773 | |
COOP3 | 5.794 | 1.320 | 0.846 | 28.382 | LOY COM3 | 4.917 | 1.478 | 0.845 | 30.462 | |
COOP4 | 5.534 | 1.373 | 0.842 | 25.834 | LOY COM4 | 4.824 | 1.471 | 0.876 | 44.212 | |
COOP5 | 5.887 | 1.205 | 0.883 | 51.894 | LOY COM5 | 4.455 | 1.715 | 0.816 | 31.411 | |
*Note: Significance and standard deviations (SD) performed by 10,000 repetitions bootstrapping procedure ∗∗∗: All loadings are significant at a 0.001 level. |
Composites | α | ρA | ρC | AVE |
CAL COM | 0.945 | 0.951 | 0.958 | 0.821 |
TRUST | 0.875 | 0.893 | 0.909 | 0.632 |
COOP | 0.918 | 0.922 | 0.939 | 0.754 |
DEP | 0.893 | 0.894 | 0.926 | 0.758 |
ENGA | 0.891 | 0.893 | 0.920 | 0.696 |
LOY COM | 0.936 | 0.938 | 0.952 | 0.798 |
*Note: α: Chronbach's alpha; ρA: Dijkstra–Henseler's composite reliability; ρC: Jöreskog's composite reliability; AVE: average variance extracted. |
Ⅰ | Ⅱ | Ⅲ | Ⅳ | Ⅴ | Ⅵ | ||
Ⅰ | CAL COM | 0.906 | |||||
Ⅱ | COOP | 0.043 | 0.795 | ||||
Ⅲ | DEP | 0.828 | 0.175 | 0.868 | |||
Ⅳ | ENGA | 0.398 | 0.448 | 0.429 | 0.871 | ||
Ⅴ | LOY COM | 0.253 | 0.689 | 0.344 | 0.633 | 0.834 | |
Ⅵ | TRUST | 0.107 | 0.780 | 0.208 | 0.396 | 0.722 | 0.893 |
*Note: Fornell–Larcker criterion: square root of AVE in diagonal (bold) and construct correlations below the diagonal. |
Ⅰ | Ⅱ | Ⅲ | Ⅳ | Ⅴ | ||
Ⅰ | CAL COM | |||||
Ⅱ | COOP | 0.102 | ||||
Ⅲ | DEP | 0.784 | 0.212 | |||
Ⅳ | ENGA | 0.428 | 0.542 | 0.473 | ||
Ⅴ | LOY COM | 0.278 | 0.775 | 0.388 | 0.713 | |
Ⅵ | TRUST | 0.117 | 0.843 | 0.228 | 0.430 | 0.770 |
CAL_COM | COOP | ENGA | LOY_COM | TRUST | |
CAL_COM | 1.068 | 1.189 | |||
COOP | |||||
DEP | 1.000 | 1.228 | |||
ENGA | 1.394 | ||||
LOY_COM | 1.068 | 1.000 | |||
TRUST | 1.189 | 1.000 |
CI | ||||||||
Path | SD | T-value | 5% | 95% | f2 | H | Supported | |
Direct effects | ||||||||
TRUST - > COOP | 0.717 | 0.050 | 14.286*** | 0.629 | 0.794 | 1.177 | H1 | YES |
DEP - > COOP | −0.054 | 0.053 | 1.007ns | −0.142 | 0.033 | 0.006 | H2 | NO |
TRUST - > LOY COM | 0.722 | 0.034 | 20.946*** | 0.663 | 0.776 | 1.091 | H3 | YES |
DEP - > CAL COM | 0.828 | 0.026 | 31.289*** | 0.782 | 0.870 | 2.188 | H4 | YES |
LOY_COM - > ENGA | 0.569 | 0.057 | 9.962*** | 0.474 | 0.661 | 0.561 | H5 | YES |
CAL_COM - > ENGA | 0.254 | 0.061 | 4.163*** | 0.152 | 0.354 | 0.112 | H6 | YES |
ENGA - > COOP | 0.187 | 0.065 | 2.872*** | 0.078 | 0.291 | 0.068 | H9 | YES |
Indirect effects | ||||||||
Individual indirect effects | VAF | |||||||
TRUST - > LOY COM - > ENGA | 0.411 | 0.046 | 9.021*** | 0.336 | 0.487 | 100% | H7 | YES |
DEP - > CAL COM - > ENGA | 0.210 | 0.052 | 4.079*** | 0.126 | 0.296 | 100% | H8 | YES |
TRUST - > LOY COM - > ENGA - > COOP | 0.077 | 0.030 | 2.597** | 0.030 | 0.127 | 10% | H10 | YES |
DEP - > CAL COM - > ENGA - > COOP | 0.039 | 0.015 | 2.657** | 0.016 | 0.064 | 100% | H11 | YES |
CAL COM - > ENGA - > COOP | 0.047 | 0.017 | 2.715** | 0.019 | 0.077 | 100% | ||
LOY COM - > ENGA - > COOP | 0.106 | 0.042 | 2.555** | 0.041 | 0.178 | 100% | ||
Indirect effects | ||||||||
CAL COM - > COOP | 0.047 | 0.017 | 2.715** | 0.019 | 0.077 | 100% | ||
DEP - > COOP | 0.039 | 0.015 | 2.657** | 0.016 | 0.064 | 100% | ||
DEP - > ENGA | 0.210 | 0.052 | 4.079*** | 0.126 | 0.296 | 100% | ||
LOY COM - > COOP | 0.106 | 0.042 | 2.555** | 0.041 | 0.178 | 100% | ||
TRUST - > COOP | 0.077 | 0.030 | 2.597** | 0.030 | 0.127 | 10% | ||
TRUST - > ENGA | 0.411 | 0.046 | 9.021*** | 0.336 | 0.487 | 100% | ||
Total effects | ||||||||
CAL_COM - > COOP | 0.047 | 0.017 | 2.715** | 0.019 | 0.077 | |||
DEP - > COOP | -0.014 | 0.050 | 0.288ns | -0.099 | 0.067 | |||
DEP - > ENGA | 0.210 | 0.052 | 4.079*** | 0.126 | 0.296 | |||
LOY_COM - > COOP | 0.106 | 0.042 | 2.555** | 0.041 | 0.178 | |||
TRUST - > COOP | 0.793 | 0.038 | 20.750*** | 0.726 | 0.851 | |||
TRUST - > ENGA | 0.411 | 0.046 | 9.021*** | 0.336 | 0.487 | |||
*Note: R2 [95% CI in brackets]: CAL COM: 0.685 [0.610; 0.756]; COOP: 0.627 [0.542; 0.715]; ENGA: 0.455 [0.367; 0.556]; LOY COM: 0.519 [0.437; 0.601]. f2: size effect index; 95PCI:95% percentile Confidence Interval; Significance, standard deviations, 95% bias-corrected CIs were performed by 10,000 repetitions bootstrapping procedure; *: p < 0.05; **: p < 0.01; ***: p < 0.001. Only total effects that differ from direct effects are showed. |
CONSTRUCT PREDICTION SUMMARY | |||
Construct | Q2predict | RMSE | MAE |
CAL_COM | 0.683 | 0.569 | 0.434 |
COOP | 0.598 | 0.643 | 0.493 |
ENGA | 0.244 | 0.879 | 0.723 |
LOY_COM | 0.518 | 0.703 | 0.563 |
t-value | DF | p | ||
COOP1 | –0.0872 | 202 | 0.931 | |
COOP2 | –0.9342 | 202 | 0.351 | |
COOP3 | –1.9145 | ᵃ | 202 | 0.057 |
COOP4 | –0.5812 | 202 | 0.562 | |
COOP5 | –1.3219 | ᵃ | 202 | 0.188 |
TRUST1 | –1.3942 | ᵃ | 202 | 0.165 |
TRUST2 | –0.9529 | ᵃ | 201 | 0.342 |
TRUST3 | –0.8446 | ᵃ | 201 | 0.399 |
TRUST4 | –0.4036 | 202 | 0.687 | |
TRUST5 | –1.7356 | ᵃ | 202 | 0.084 |
DEP1 | 2.1116 | 201 | 0.036 | |
DEP2 | 1.5257 | 201 | 0.129 | |
DEP3 | 1.6130 | 202 | 0.108 | |
DEP4 | 1.7877 | 201 | 0.075 | |
DEP5 | 1.5789 | 202 | 0.116 | |
COM_AF1 | –2.0705 | ᵃ | 202 | 0.040 |
COM_AF2 | –0.9646 | 202 | 0.336 | |
COM_AF3 | 0.6913 | 202 | 0.490 | |
COM_AF4 | 1.5656 | 202 | 0.119 | |
COM_AF5 | 1.9051 | 200 | 0.058 | |
COM_EC1 | 2.5842 | 201 | 0.010 | |
COM_EC2 | 2.1232 | 202 | 0.035 | |
COM_EC3 | 3.1196 | 202 | 0.002 | |
COM_EC4 | 2.2717 | 202 | 0.024 | |
COM_EC5 | 1.7581 | 201 | 0.080 | |
ENGA1 | 1.0283 | 201 | 0.305 | |
ENGA2 | 1.1652 | 200 | 0.245 | |
ENGA3 | 0.8423 | 201 | 0.401 | |
ENGA4 | 1.6134 | 201 | 0.108 | |
ENGA5 | 1.8234 | 199 | 0.070 |
t-value | DF | p | ||
COOP1 | 1.6666 | 202 | 0.097 | |
COOP2 | 1.3381 | 202 | 0.182 | |
COOP3 | 1.9101 | ᵃ | 202 | 0.058 |
COOP4 | 1.3328 | ᵃ | 202 | 0.184 |
COOP5 | 0.6725 | 202 | 0.502 | |
COOP6 | –0.8988 | 201 | 0.370 | |
TRUST1 | 0.7535 | 202 | 0.452 | |
TRUST2 | 0.1883 | 201 | 0.851 | |
TRUST3 | 0.5513 | 201 | 0.582 | |
TRUST4 | 0.6898 | 202 | 0.491 | |
TRUST5 | 0.1537 | 202 | 0.878 | |
DEP1 | –0.0106 | 201 | 0.992 | |
DEP2 | –0.4337 | 201 | 0.665 | |
DEP3 | 0.4415 | 202 | 0.659 | |
DEP4 | 0.6301 | 201 | 0.529 | |
DEP5 | 0.7980 | 202 | 0.426 | |
COM_AF1 | 0.8056 | 202 | 0.421 | |
COM_AF2 | 1.0568 | ᵃ | 202 | 0.292 |
COM_AF3 | 2.3686 | ᵃ | 202 | 0.019 |
COM_AF4 | 2.5038 | ᵃ | 202 | 0.013 |
COM_AF5 | 2.3194 | 200 | 0.021 | |
COM_EC1 | –0.3202 | 201 | 0.749 | |
COM_EC2 | 0.0745 | 202 | 0.941 | |
COM_EC3 | –0.1863 | 202 | 0.852 | |
COM_EC4 | 0.4881 | 202 | 0.626 | |
COM_EC5 | –0.6967 | 201 | 0.487 | |
ENGA1 | 0.3217 | 201 | 0.748 | |
ENGA2 | 0.7979 | 200 | 0.426 | |
ENGA3 | 0.5233 | 201 | 0.601 | |
ENGA4 | 0.5911 | 199 | 0.555 |