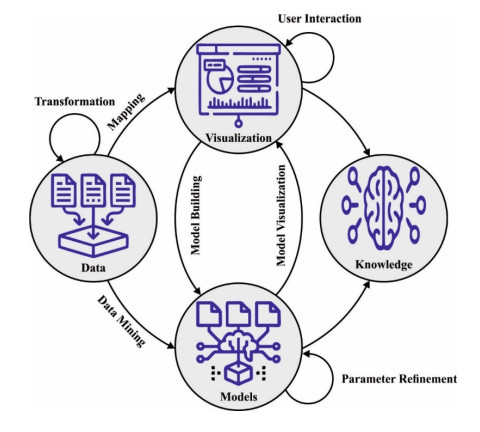
Customer churn prediction (CCP) is among the greatest challenges faced in the telecommunication sector. With progress in the fields of machine learning (ML) and artificial intelligence (AI), the possibility of CCP has dramatically increased. Therefore, this study presents an artificial intelligence with Jaya optimization algorithm based churn prediction for data exploration (AIJOA-CPDE) technique for human-computer interaction (HCI) application. The major aim of the AIJOA-CPDE technique is the determination of churned and non-churned customers. In the AIJOA-CPDE technique, an initial stage of feature selection using the JOA named the JOA-FS technique is presented to choose feature subsets. For churn prediction, the AIJOA-CPDE technique employs a bidirectional long short-term memory (BDLSTM) model. Lastly, the chicken swarm optimization (CSO) algorithm is enforced as a hyperparameter optimizer of the BDLSTM model. A detailed experimental validation of the AIJOA-CPDE technique ensured its superior performance over other existing approaches.
Citation: Ilyоs Abdullaev, Natalia Prodanova, Mohammed Altaf Ahmed, E. Laxmi Lydia, Bhanu Shrestha, Gyanendra Prasad Joshi, Woong Cho. Leveraging metaheuristics with artificial intelligence for customer churn prediction in telecom industries[J]. Electronic Research Archive, 2023, 31(8): 4443-4458. doi: 10.3934/era.2023227
[1] | Dong-hyeon Kim, Se-woon Choe, Sung-Uk Zhang . Recognition of adherent polychaetes on oysters and scallops using Microsoft Azure Custom Vision. Electronic Research Archive, 2023, 31(3): 1691-1709. doi: 10.3934/era.2023088 |
[2] | Meizhen Deng, Yimeng Liu, Ling Chen . AI-driven innovation in ethnic clothing design: an intersection of machine learning and cultural heritage. Electronic Research Archive, 2023, 31(9): 5793-5814. doi: 10.3934/era.2023295 |
[3] | Zhizhou Zhang, Zhenglei Wei, Bowen Nie, Yang Li . Discontinuous maneuver trajectory prediction based on HOA-GRU method for the UAVs. Electronic Research Archive, 2022, 30(8): 3111-3129. doi: 10.3934/era.2022158 |
[4] | Mengke Lu, Shang Gao, Xibei Yang, Hualong Yu . Improving performance of decision threshold moving-based strategies by integrating density-based clustering technique. Electronic Research Archive, 2023, 31(5): 2501-2518. doi: 10.3934/era.2023127 |
[5] | Ruyu Yan, Jiafei Jin, Kun Han . Reinforcement learning for deep portfolio optimization. Electronic Research Archive, 2024, 32(9): 5176-5200. doi: 10.3934/era.2024239 |
[6] | Ju Wang, Leifeng Zhang, Sanqiang Yang, Shaoning Lian, Peng Wang, Lei Yu, Zhenyu Yang . Optimized LSTM based on improved whale algorithm for surface subsidence deformation prediction. Electronic Research Archive, 2023, 31(6): 3435-3452. doi: 10.3934/era.2023174 |
[7] | Shangce Gao, Rong-Long Wang, Dongbao Jia, Ting Jin . Special Issue: Artificial intelligence and computational intelligence. Electronic Research Archive, 2023, 31(12): 7556-7558. doi: 10.3934/era.2023381 |
[8] | Kai Huang, Chang Jiang, Pei Li, Ali Shan, Jian Wan, Wenhu Qin . A systematic framework for urban smart transportation towards traffic management and parking. Electronic Research Archive, 2022, 30(11): 4191-4208. doi: 10.3934/era.2022212 |
[9] | Hui Yao, Yaning Fan, Xinyue Wei, Yanhao Liu, Dandan Cao, Zhanping You . Research and optimization of YOLO-based method for automatic pavement defect detection. Electronic Research Archive, 2024, 32(3): 1708-1730. doi: 10.3934/era.2024078 |
[10] | Shizhen Huang, Enhao Tang, Shun Li, Xiangzhan Ping, Ruiqi Chen . Hardware-friendly compression and hardware acceleration for transformer: A survey. Electronic Research Archive, 2022, 30(10): 3755-3785. doi: 10.3934/era.2022192 |
Customer churn prediction (CCP) is among the greatest challenges faced in the telecommunication sector. With progress in the fields of machine learning (ML) and artificial intelligence (AI), the possibility of CCP has dramatically increased. Therefore, this study presents an artificial intelligence with Jaya optimization algorithm based churn prediction for data exploration (AIJOA-CPDE) technique for human-computer interaction (HCI) application. The major aim of the AIJOA-CPDE technique is the determination of churned and non-churned customers. In the AIJOA-CPDE technique, an initial stage of feature selection using the JOA named the JOA-FS technique is presented to choose feature subsets. For churn prediction, the AIJOA-CPDE technique employs a bidirectional long short-term memory (BDLSTM) model. Lastly, the chicken swarm optimization (CSO) algorithm is enforced as a hyperparameter optimizer of the BDLSTM model. A detailed experimental validation of the AIJOA-CPDE technique ensured its superior performance over other existing approaches.
With an abundance of prototype and in-field industrial applications, machine learning (ML) is a rapidly developing technology [1]. Some of the areas that have not covered the potential of the technologies are knowledge acquisition, robotics, manufacturing, execution and control, computer vision, design, medicine, scheduling and planning. Studies utilizing new networks and media have found a niche for ML in the retrieval of information and navigation [2,3]. Human-computer interaction (HCI) signifies a vast area of research and applications. HCI has addressed various areas, such as computer-supported cooperative work or user interface design along with the tasks relevant to optimization, user modeling and system adaptation to meet the demand of the user [4,5]. HCI professionals and researchers are involved in several fields, including but not limited to manufacturing, aviation, and aerospace. HCI concerns the role of individuals in complicated mechanisms, the model of equipment and facilities for human use and the progression of environments for safety and comfort [6]. Figure 1 exhibits the process of visual analysis.
The progressions of the telecommunication sector and globalization have exponentially increased the number of operators in the market, which increases competition [7,8]. In this competitive period, it is required that profits should be maximized periodically, and thus many approaches were framed such as up-selling the existing consumer, expanding the retention time of existing consumers and getting new consumers [9]. The important objective of customer churn prediction (CCP) is to assist in accomplishing strategies for retaining the customer. In addition to increasing competition in marketplaces for offering services, the risk of customer churn is also increasing exponentially [10,11]. So, creating tactics for tracking loyal consumers (non-churners) turns out to be a necessity. The customer churn methods try to detect early churn signs and also forecast the customers who leave voluntarily [12]. Therefore, several companies have understood that their existing customer database was the most valuable asset, and churn prediction was an advantageous tool for predicting consumers at risk. Nowadays, HCI techniques, which are mostly dominated by graphical user interfaces, face the limitations of inadequate capture of emotions, slow processing speeds and low accuracy [13]. To overcome the difficulties in these communications, it is required to make breakthroughs in intent understanding, vision, context perception, speech, etc. Deep learning (DL) can find valuable association rules using big data analytics for learning the human cognitive process and building new complicated techniques that lead to accurate and efficient predictions and judgments, thereby making human-computer communications more natural and quicker [14]. Hence, there comes a demand for empirical, theoretical, systems and design research on artificial intelligence and evolutionary computation techniques [15].
This study presents an artificial intelligence with Jaya optimization algorithm based churn prediction for data exploration (AIJOA-CPDE) technique for HCI application. In the AIJOA-CPDE technique, an initial stage of feature selection using the JOA named the JOA-FS technique is presented to choose feature subsets. For churn prediction, the AIJOA-CPDE technique employs a bidirectional long short-term memory (BDLSTM) model. Lastly, the chicken swarm optimization (CSO) algorithm is enforced as a hyperparameter optimizer of the BDLSTM model. A detailed experimental validation of the AIJOA-CPDE technique ensured its superior performance over other existing approaches.
Al-Shourbaji et al. [16] devised a new FS technique, ACO-RSA, which incorporates two metaheuristic approaches, the reptile search algorithm (RSA) and ant colony optimization (ACO). In their study, the incorporation of ACO and RSA was used for selecting a crucial subset of features for churn prediction. Hegde and Mundada [17] aimed to accurately forecast the attrition rate in the banking field with an enhanced deep feed forward neural network (FFNN). In the presented technique, the predictive ML algorithm was used through an optimized deep FFNN which had five hidden layers in it. The Adam optimizer was used for training the model to accomplish the optimum performance. The Banking Churn dataset was fed as input to Deep FFNN. The same dataset was passed as input to other ML algorithms, namely, artificial neural network (ANN), decision tree (DT), logistic regression (LR), and Gaussian Naïve Bayes, for performing the comparison study.
In [18], ML and deep learning (DL) algorithms were implemented for predicting telecom customer churn. Ubiquitous models including support vector machines (SVMs) and random forest (RF) classifiers were compared with comparatively new architectures such as XGBoost and DNN to categorize whether a customer will churn or not. Further, the effectiveness of this model was explored by a grid search. Vakeel et al. [19] introduced an efficient ML technique that involves a boosting algorithm to recognize Gaussian mixture and customer churn (CC) models for clustering the churned customers. Also, the presented method uses the Light Gradient Boosting (LightGB) mechanism, which outperforms XGBoost and AdaBoost for churn prediction by a factor of 15x.
Tariq et al. [20] aimed to assist an e-business in predicting the churned users through ML. The study focused on performing decision-making and monitoring customer behavior consequently. The proposed technique made use of 2D-CNN, a DL algorithm. The presented method was a layered structure that included 2D-CNN layer, data load layer and pre-processing layer. Furthermore, Apache Spark parallel and distributed architectures have been implemented for data processing in the parallel environment. Mohammad et al. [21] aimed to recognize the factors that influencecustomer churn, developed an efficient churn prediction method and provided a better analysis of data visualization outcomes. The presented technique was used for the analysis of churn prediction, which covers multiple stages: evaluation of the classifiers, data pre-processing, analysis, enforcement of ML algorithm and selection of the best one for prediction. Data preprocessing involved three main actions: feature selection, data cleaning and data transformation. ML classifiers selected were RF, LR and ANN.
In the present study, we have established a novel AIJOA-CPDE approach for automated churn prediction in HCI applications. The goal of the AIJOA-CPDE technique exists in the accurate identification of churned or non-churned customers. In the AIJOA-CPDE technique, three subprocesses are used, namely, feature subset election, BDLSTM classification and CSO-based parameter tuning. Figure 2 defines the workflow of the AIJOA-CPDE system.
In this work, the JOA-FS approach was used to produce a set of features to enhance the classification results. Rao [22] established the JOA deal with constraint and unconstraint optimized techniques. It is significantly easy to implement this approach because it has only one phase. Jaya means "victory" in Sanskrit. This method exploits a population-oriented metaheuristic that contains evolutionary and SI features. It was identified in the behavior of the "survival of fittest" conception. "The search method of the proposed method aim is to get closer to success by finding the best global solution and evade failure by avoiding the worst choice [22]." In this work, properties of swarm‐based intelligence and evolutionary algorithms were incorporated.
Consider that the objective function ψ(x) must be increased or decreased according to the problems. Assume that n is the number of candidate solutions, and d is the number of design parameters (population size, k=1,2…,n) for t iterations. Assume the superior candidate obtains the best value of ψ(x) (ψ(x)best) of all the candidate solutions, and the worst candidate acquires the worst value of ψ(x) (ψ(x)worst) of all the candidate solutions [23]. Once the values of thejth variable for the kth candidate at iteration t are X(j,k,t), the value is upgraded as follows:
X'(j,k,t)=X(j,k,t)+q(1,j,t)(X(j,best,t)−|x(j,k,t)|)−q(2,j,t)(X(j,worst,t)−|x(j,k,t)|). | (1) |
From the expression, the value of the jth parameter for the best candidate represents X(j,best,t) while the value of the jth parameter for the worst candidate characterizes X(j,worst,t). X'(j,k,t) shows the upgraded values of X(j,k,t), and q(1,j,t) and q(2,j,t) indicate random numbers within [0, 1] for parameter j at iteration t. The term q(1,j,t)(X(j,best,t)−|x(j,k,t)|) demonstrates the solution's tendency to get nearer to the best solution, whereas the term " q(2,j,t)(X(j,worst,t)−|x(j,k,t)|) " demonstrates the solution's tendency to avoid the worst. Once X'(j,k,t) generates a better function value, it is accepted. In each iteration, every acceptable function value was utilized and kept as the input for the forthcoming iteration.
The fitness function (FF) of JOA considers the classifier accuracy and number of selected features. It reduces the size set of chosen features and maximizes the classifier performances. Thereby, the subsequent FF is exploited for estimating individual solutions, as follows.
Fitness=α∗ErrorRate+(1−α)∗#SF#All_F | (2) |
In Eq (2), theErrorRate identifies the classifier error rate applying the feature that is chosen. The error rate was calculated as a percentage of inaccurate categories for counting classifications made, specified as values among [0, 1]. #SF was chosen as feature count, and #All_F is the overall amount of features in actual data. α was applied for controlling consequence subset length and classification quality. In the presented method, α is fixed as 0.9.
For the churn prediction process, the BDLSTM model was used. LSTM is a variant of recurrent neural networks (RNN) that was presented [24]. In every t iteration, xt represents the input, and ht symbolizes the output of the LSTM cell, and the cell output state of the preceding time step can be represented as Ct−1 are integrated with LSTM cells for updating network parameters in the training procedure. The gates mechanism is presented for controlling the ceU state of LSTM by permitting data to pass through alternatively. Forget, input and output gates are represented by ft, it, and 0t, correspondingly. The value of the cell input state and gate can be computed as follows:
it=σg(Wixt+Uiht−1+bi) | (3) |
ft=σg(Wfxt+Ufht−1+bf) | (4) |
ot=σg(Woxt+Uoht−1+bo) | (5) |
Ct=tanh(Wcxt+Uoht−1+bc) | (6) |
From the equation, Wf, Wi, Wc and Wo represent the weight matrices between inputs of the hidden layer, forget gate, input gate and input cell state and the output gate. Uf,Ui, Uc and Uo signify weight matrices between the previous cell output state, forget gate, input gate and input cell state and the output gate. bf, bi, bc and bo denote the bias vectors. BDLSTM is a kind of LSTM variation. In BDLSTM, sequential data is processed with backward and forward LSTM layers, and those 2 hidden layers are closely linked with the same output layer. Based on Eqs (3) to (6), at every iteration t, the cell output state Ct and LSTM layer output ht were formulated as follows:
Ct=ft∗Ct−1+ Ct∗it | (7) |
ht=0t∗tanh(Ct) | (8) |
BDLSTM has been efficaciously used in the fields of speech recognition, trajectory prediction, natural language processing, biomedical event analysis and so on. It has been proved that BDLSTM outperforms traditional LSTM in some fields, such as frame automatic speech recognition and understanding and wise phoneme classification. Figure 3 represents the structure of LSTM.
To scale up the predictive results of the BDLSTM method, the CSO technique was implemented as a hyperparameter optimizer of the BDLSTM method. In CSO, there exist numerous groups, and each group encompasses some hens, chicks and dominant roosters [25]. Hens, chicks and roosters in the groups are determined according to their fitness values. Chicks are chickens with poor fitness values. The rooster (group head) is a chicken that has a better fitness value. Mostly, chickens will be hens, and it randomly selects which group to stay in. Mother-child associations among the hens and the chicks are carried out at random. The dominance and mother‐child relations in the group remain the same and update (G) time step. The chicken movements can be expressed as follows:
1) The rooster location update is shown below:
Xt+1i,j=Xti,j∗(1+randn(0,σ2)) | (9) |
where
σ2={1if fi≤fkexp(fk−fi|fi+ϵ|)Otherwise |
From the expression, k∈[1,Nr], k≠i and Nr denote the number of selected roosters. Xi,j indicates the position of i - th rooster in the jth parameter at t and t+1 iterations, randn (O,σ2) produces Gaussian random values having mean 0 and variance σ2, ϵ signifies an arbitrarily small constant, and fi demonstrates fitness values for corresponding rooster i.
2) The hen location updating is given in the following:
Xt+1i,j=Xti,j+S1randn(Xtr1,j−Xti,j)+S2randn(Xtr2,j−Xti,j) | (10) |
where
S1=exp(fi−fr1|fi|+ϵ) | (11) |
and
S1=exp(fr2−fi). | (12) |
Now, r1, r2∈[1,…,N],r1≠r2 denote the index of the rooster, r2 denotes swarming chicken, eitherrooster or hen and the uniform random number is generated through randn.
3) The chick location updating is expressed as follows:
Xt+1i,j=Xti,j+FL(Xtm,j−Xti,j),FL∈[0,2] | (13) |
In Eq (13), Xtm,j denotes the position of the ith chick's mother.
The CSO derives a fitness function (FF) for accomplishing improved classifier outcomes. It specifies the positive value to characterize the better accuracy of the candidate solution. The reduction classifier error amount is considered as the FF in the following.
fitness(xi)=ClassifierErrorRate(xi)=number of misclassified samplesTotal number of samples∗100 | (14) |
The AIJOA-CPDE procedure was tested by employing the churn dataset [26]. It involves 3333 samples with 21 features and two classes. The JOA-FS technique has chosen 12 features out of available 21 features. For experimental validation, the training (TR) and testing (TS) datasets are split in two ways as 90:10 (90% TR samples and 10% TS samples) and 80:20 (80% TR samples and 20% TS samples). Figure 4 demonstrates the churn prediction results of the AIJOA-CPDE model through the confusion matrix under 90:10 and 80:20 of TR/TS datasets.
The outcomes indicated that the AIJOA-CPDE procedure has precisely recognized churn and non-churn customers. As an example, with 90% of the TR dataset, the AIJOA-CPDE model has identified 356 as churn and 2546 as non-churn. Meanwhile, with 10% of the TS database, the AIJOA-CPDE procedure has identified 36 as churn and 288 as non-churn. Moreover, with 80% of the TR dataset, the AIJOA-CPDE method has identified 316 as churn and 2268 as non-churn.
In Table 1, a brief set of CCP outcomes of the AIJOA-CPDE model with 90:10 and 80:20 of TR/TS datasets is shown. With 90% of the TR dataset, the AIJOA-CPDE procedure has attained an average accubal of 90.20%, precn of 96.64%, recal of 90.20%, Fscore of 93.07% and AUCscore of 90.20%. Meanwhile, with 10% of the TS database, the AIJOA-CPDE approach has acquired an average accubal of 91.35%, precn of 94.97%, recal of 91.35%, Fscore of 93.05% and AUCscore of 91.35%. Eventually, with 80% of the TR dataset, the AIJOA-CPDE approach has achieved an average accubal of 90.17%, precn of 97.45%, recal of 90.17%, Fscore of 93.37% and AUCscore of 90.17%. Finally, with 20% of the TS dataset, the AIJOA-CPDE method has reached an average accubal of 90.02%, precn of 97.81%, recal of 90.02%, Fscore of 93.43% and AUCscore of 90.02%.
Class | Accuracybal | Precision | Recall | F-Score | AUC Score |
Training Phase (90%) | |||||
Churn | 80.91 | 96.48 | 80.91 | 88.01 | 90.20 |
Non-Churn | 99.49 | 96.81 | 99.49 | 98.13 | 90.20 |
Average | 90.20 | 96.64 | 90.20 | 93.07 | 90.20 |
Testing Phase (10%) | |||||
Churn | 83.72 | 92.31 | 83.72 | 87.80 | 91.35 |
Non-Churn | 98.97 | 97.63 | 98.97 | 98.29 | 91.35 |
Average | 91.35 | 94.97 | 91.35 | 93.05 | 91.35 |
Training Phase (80%) | |||||
Churn | 80.61 | 98.14 | 80.61 | 88.52 | 90.17 |
Non-Churn | 99.74 | 96.76 | 99.74 | 98.22 | 90.17 |
Average | 90.17 | 97.45 | 90.17 | 93.37 | 90.17 |
Testing Phase (20%) | |||||
Churn | 80.22 | 98.65 | 80.22 | 88.48 | 90.02 |
Non-Churn | 99.83 | 96.96 | 99.83 | 98.37 | 90.02 |
Average | 90.02 | 97.81 | 90.02 | 93.43 | 90.02 |
Figure 5 demonstrates the churn prediction results of the AIJOA-CPDE model by means of a confusion matrix under 70:30 and 60:40 of TR/TS databases. The outcomes show the AIJOA-CPDE algorithm has accurately recognized churn and non-churn customers. As an example, with 60% of the TR dataset, the AIJOA-CPDE model has recognized 262 as churn and 1978 as non-churn. In the meantime, with 10% of the TS database, the AIJOA-CPDE methodology has identified 121 as churn and 834 as non-churn. Additionally, with 60% of the TR database, the AIJOA-CPDE approach has detected 232 as churn and 1713 as non-churn.
In Table 2, a brief set of CCP outcomes of the AIJOA-CPDE model with 70:30 and 60:40 of TR/TS datasets is shown. With 70% of the TR dataset, the AIJOA-CPDE method has attained an average accubal of 89.36%, precn of 93.58%, recal of 89.36%, Fscore of 91.32% and AUCscore of 89.36%. In the meantime, with 30% of the TS dataset, the AIJOA-CPDE procedure has obtained an average accubal of 88.19%, precn of 94.17%, recal of 88.19%, Fscore of 90.85% and an AUCscore of 88.19%. In parallel, with 60% of the TR dataset, the AIJOA-CPDE method has achieved an average accubal of 91.74%, precn of 96.68%, recal of 91.74%, Fscore of 94.01% and AUCscore of 91.74%. Lastly, with 40% of the TS dataset, the AIJOA-CPDE procedure has gained an average accubal of 91.41%, precn of 97.63%, recal of 91.41%, Fscore of 94.20% and AUCscore of 91.41%.
Class | Accubal | Precn | Recal | Fscore | AUCscore |
Training Phase (70%) | |||||
Churn | 80.12 | 90.34 | 80.12 | 84.93 | 89.36 |
Non-Churn | 98.60 | 96.82 | 98.60 | 97.70 | 89.36 |
Average | 89.36 | 93.58 | 89.36 | 91.32 | 89.36 |
Testing Phase (30%) | |||||
Churn | 77.56 | 92.37 | 77.56 | 84.32 | 88.19 |
Non-Churn | 98.82 | 95.97 | 98.82 | 97.37 | 88.19 |
Average | 88.19 | 94.17 | 88.19 | 90.85 | 88.19 |
Training Phase (60%) | |||||
Churn | 84.06 | 95.87 | 84.06 | 89.58 | 91.74 |
Non-Churn | 99.42 | 97.50 | 99.42 | 98.45 | 91.74 |
Average | 91.74 | 96.68 | 91.74 | 94.01 | 91.74 |
Testing Phase (40%) | |||||
Churn | 83.09 | 98.29 | 83.09 | 90.05 | 91.41 |
Non-Churn | 99.73 | 96.98 | 99.73 | 98.34 | 91.41 |
Average | 91.41 | 97.63 | 91.41 | 94.20 | 91.41 |
The TACC and VACC of the AIJOA-CPDE algorithm are examined on CCP achievement in Figure 6. The results exhibited that the AIJOA-CPDE method has demonstrated improved accomplishment with improved values of TACC and VACC. Particularly, the AIJOA-CPDE technique has attained the greatest TACC results.
The TLS and VLS of the AIJOA-CPDE method are verified on CCP performance in Figure 7. The figure showed that the AIJOA-CPDE procedure has revealed improved accomplishment with the least values of TLS and VLS. Apparently, the AIJOA-CPDE method has resulted in decreased VLS results.
A precision-recall examination of the AIJOA-CPDE procedure under the test dataset is shown in Figure 8. The figure depicted the AIJOA-CPDE approach has improved values precision-recall values under all classes.
An elaborated ROC research of the AIJOA-CPDE method under the test dataset is shown in Figure 9. The figure exhibited the AIJOA-CPDE method has exposed its capability to categorize different classes.
A comparative analysis of the AIJOA-CPDE technique with current procedures on churn prediction is reported in Table 3 [27,28,29]. A brief analysis of the AIJOA-CPDE method with current methods in terms of accuy is depicted. Based on accuy, the outcomes exhibited that the DT method has reduced accuy by 76.78%. Then, the LR, SVM, SGD, and RMSProp models have reported slightly improved accuy of 80.65, 84.41, 84.54 and 87.48% respectively. Although the ISMOTE-OWELM models have reached reasonable outcomes with anaccuy of 90.59%, the AIJOA-CPDE model has shown a maximum accuy of 91.41%.
Methods | Accuy | Precn | Recal | Fscore | AUCscore |
AIJOA-CPDE | 91.41 | 97.63 | 91.41 | 94.20 | 91.41 |
Logistic Regression [27] | 80.65 | 79.44 | 80.56 | 79.17 | 82.30 |
Decision Tree [27] | 76.78 | 56.90 | 75.81 | 65.09 | 78.37 |
ISMOTE-OWELM [28] | 90.59 | 91.78 | 89.51 | 89.77 | 89.96 |
SVM [28] | 84.41 | 84.66 | 84.11 | 85.71 | 84.10 |
SGD-NN [29] | 84.54 | 86.22 | 85.92 | 84.43 | 84.91 |
RMSProp-NN [29] | 87.48 | 85.29 | 85.30 | 85.20 | 86.38 |
Although the ISMOTE-OWELM approaches have reached reasonable outcomes with a recal of 89.51%, the AIJOA-CPDE technique has shown maximum recal of 91.41%. Although the ISMOTE-OWELM techniques have reached reasonable outcomes with aFscore of 89.77%, the AIJOA-CPDE model has shown a maximum Fscore of 94.20%. In addition, the AIJOA-CPDE technique has shown a maximum AUCscore of 91.41%. Thus, the AIJOA-CPDE method can be employed for an accurate churn prediction process.
In this study, we have advanced a novel AIJOA-CPDE technique for automated churn prediction in HCI applications. The goal of the AIJOA-CPDE technique exists in the accurate identification of churned or non-churned customers. In the AIJOA-CPDE technique, an early stage of feature selection through JOA named the JOA-FS technique is presented to choose feature subsets. To identify churn customers, the AIJOA-CPDE technique employed the BDLSTM model. Lastly, the CSO algorithm is implemented as a hyperparameter optimizer of the BDLSTM method. A detailed experimental validation of the AIJOA-CPDE technique ensured its superior performance over other existing approaches. In the future, the outlier detection process can be employed to boost the prediction performance of the AIJOA-CPDE technique.
The authors declare they have not used Artificial Intelligence (AI) tools in the creation of this article.
This work was supported by the Ulsan City & Electronics and Telecommunications Research Institute (ETRI) grant funded by the Ulsan City [23AS1600, the development of intelligentization technology for the main industry for manufacturing innovation and Human-mobile-space autonomous collaboration intelligence technology development in industrial sites].
The authors declare that they have no conflict of interest. The manuscript was written through contributions of all authors. All authors have given approval to the final version of the manuscript.
[1] |
R. A. de L. Lemos, T. C. Silva, B. M. Tabak, Propension to customer churn in a financial institution: a machine learning approach, Neural Comput. Appl., 34 (2022), 11751–11768. https://doi.org/10.1007/s00521-022-07067-x doi: 10.1007/s00521-022-07067-x
![]() |
[2] | Y. K. Saheed, M. A. Hambali, Customer churn prediction in telecom sector with machine learning and information gain filter feature selection algorithms, in 2021 International Conference on Data Analytics for Business and Industry (ICDABI), (2021), 208–213. https://doi.org/10.1109/ICDABI53623.2021.9655792 |
[3] |
M. T. Quasim, A. Sulaiman, A. Shaikh, M. Younus, Blockchain in churn prediction based telecommunication system on climatic weather application, Sustainable Comput. Inf. Syst., 35 (2022), 100705. https://doi.org/10.1016/j.suscom.2022.100705 doi: 10.1016/j.suscom.2022.100705
![]() |
[4] | J. Dias, P. Godinho, P. Torres, Machine learning for customer churn prediction in retail banking, in Computational Science and Its Applications – ICCSA 2020, (2020), 576–589. https://doi.org/10.1007/978-3-030-58808-3_42 |
[5] |
E. Domingos, B. Ojeme, O. Daramola, Experimental analysis of hyperparameters for deep learning-based churn prediction in the banking sector, Computation, 9 (2021), 34. https://doi.org/10.3390/computation9030034 doi: 10.3390/computation9030034
![]() |
[6] |
R. Sudharsan, E. N. Ganesh, A Swish RNN based customer churn prediction for the telecom industry with a novel feature selection strategy, Connect. Sci., 34 (2022), 1855–1876. https://doi.org/10.1080/09540091.2022.2083584 doi: 10.1080/09540091.2022.2083584
![]() |
[7] |
D. AL-Najjar, N. Al-Rousan, H. AL-Najjar, Machine learning to develop credit card customer churn prediction, J. Theor. Appl. Electron. Commer. Res., 17 (2022), 1529–1542. https://doi.org/10.3390/jtaer17040077 doi: 10.3390/jtaer17040077
![]() |
[8] |
C. W. Lin, T. P. Hong, K. T. Yang, S. L. Wang, The GA-based algorithms for optimizing hiding sensitive itemsets through transaction deletion, Appl. Intell., 42 (2015), 210–230. https://doi.org/10.1007/s10489-014-0590-5 doi: 10.1007/s10489-014-0590-5
![]() |
[9] |
J. C. W. Lin, L. Yang, P. Fournier-Viger, J. M. T. Wu, T. P. Hong, L. S. L. Wang, et al., Mining high-utility itemsets based on particle swarm optimization, Eng. Appl. Artif. Intell., 55 (2016), 320–330. https://doi.org/10.1016/j.engappai.2016.07.006 doi: 10.1016/j.engappai.2016.07.006
![]() |
[10] |
X. Xiahou, Y. Harada, B2C E-commerce customer churn prediction based on K-means and SVM, J. Theor. Appl. Electron. Commer. Res., 17 (2022), 458–475. https://doi.org/10.3390/jtaer17020024 doi: 10.3390/jtaer17020024
![]() |
[11] | A. Kolomiiets, O. Mezentseva, K. Kolesnikova, Customer churn prediction in the software by subscription models it business using machine learning methods, in 2021 1nd International Workshop on Information Technologies: Theoretical and Applied Problems, 2021. Available from: http://star.informatik.rwth-aachen.de/Publications/CEUR-WS/Vol-3039/paper49.pdf. |
[12] |
Seema, G. Gupta, Development of fading channel patch based convolutional neural network models for customer churn prediction, Int. J. Syst. Assur. Eng. Manage., 2022 (2022), 1–21. https://doi.org/10.1007/s13198-022-01759-2 doi: 10.1007/s13198-022-01759-2
![]() |
[13] | K. A. Amuda, A. B. Adeyemo, Customers churn prediction in financial institution using artificial neural network, preprint, arXiv: 1912.11346. |
[14] |
M. E. Kara, S. Ü. O. Fırat, A. Ghadge, A data mining-based framework for supply chain risk management, Comput. Ind. Eng., 139 (2020), 105570. https://doi.org/10.1016/j.cie.2018.12.017 doi: 10.1016/j.cie.2018.12.017
![]() |
[15] |
A. D. Caigny, K. Coussement, K. W. D. Bock, S. Lessmann, Incorporating textual information in customer churn prediction models based on a convolutional neural network, Int. J. Forecasting, 36 (2020), 1563–1578. https://doi.org/10.1016/j.ijforecast.2019.03.029 doi: 10.1016/j.ijforecast.2019.03.029
![]() |
[16] |
I. Al-Shourbaji, N. Helian, Y. Sun, S. Alshathri, M. A. Elaziz, Boosting ant colony optimization with reptile search algorithm for churn prediction, Mathematics, 10 (2022), 1031. https://doi.org/10.3390/math10071031 doi: 10.3390/math10071031
![]() |
[17] |
S. Hegde, M. R. Mundada, Optimized deep neural network based predictive model for customer attrition analysis in the banking sector, Recent Pat. Eng., 14 (2020), 412–421. https://doi.org/10.2174/1872212113666190211130117 doi: 10.2174/1872212113666190211130117
![]() |
[18] | P. Bhuse, A. Gandhi, P. Meswani, R. Muni, N. Katre, Machine learning based telecom-customer churn prediction, in 2020 3rd International Conference on Intelligent Sustainable Systems (ICISS), (2020), 1297–1301. https://doi.org/10.1109/ICISS49785.2020.9315951 |
[19] | A. Vakeel, N. R. Vantari, S. N. Reddy, R. Muthyapu, A. Chavan, Machine learning models for predicting and clustering customer churn based on boosting algorithms and gaussian mixture model, in 2022 International Conference for Advancement in Technology (ICONAT), (2022), 1–5. https://doi.org/10.1109/ICONAT53423.2022.9725957 |
[20] |
M. U. Tariq, M. Babar, M. Poulin, A. S. Khattak, Distributed model for customer churn prediction using convolutional neural network, J. Modell. Manage., 17 (2021) 853–863. https://doi.org/10.1108/JM2-01-2021-0032 doi: 10.1108/JM2-01-2021-0032
![]() |
[21] | N. I. Mohammad, S. A. Ismail, M. N. Kama, O. M. Yusop, A. Azmi, Customer churn prediction in telecommunication industry using machine learning classifiers, in ICVISP 2019: Proceedings of the 3rd International Conference on Vision, Image and Signal Processing, (2019), 1–7. https://doi.org/10.1145/3387168.3387219 |
[22] |
R. V. Rao, Jaya: a simple and new optimization algorithm for solving constrained and unconstrained optimization problems, Int. J. Ind. Eng. Comput., 7 (2016), 19–34. https://doi.org/10.5267/j.ijiec.2015.8.004 doi: 10.5267/j.ijiec.2015.8.004
![]() |
[23] |
H. Migallón, A. Jimeno-Morenilla, H. Rico, J. L. Sánchez-Romero, A. Belazi, Multi-level parallel chaotic Jaya optimization algorithms for solving constrained engineering design problems, J. Supercomput., 77 (2021), 12280–12319. https://doi.org/10.1007/s11227-021-03737-0 doi: 10.1007/s11227-021-03737-0
![]() |
[24] |
F. Zhang, A hybrid structured deep neural network with Word2Vec for construction accident causes classification, Int. J. Construct. Manage., 22 (2022), 1120–1140. https://doi.org/10.1080/15623599.2019.1683692 doi: 10.1080/15623599.2019.1683692
![]() |
[25] |
Y. Ci, H. Wu, Y. Sun, L. Wu, A prediction model with wavelet neural network optimized by the chicken swarm optimization for on-ramps metering of the urban expressway, J. Intell. Transp. Syst., 26 (2022), 356–365. https://doi.org/10.1080/15472450.2021.1890070 doi: 10.1080/15472450.2021.1890070
![]() |
[26] | I. Brandusoiu, G. Toderean, H. Beleiu, Methods for churn prediction in the pre-paid mobile telecommunications industry, in 2016 International Conference on Communications (COMM), (2016), 97–100. https://doi.org/10.1109/ICComm.2016.7528311 |
[27] |
P. Lalwani, M. K. Mishra, J. S. Chadha, P. Sethi, Customer churn prediction system: a machine learning approach, Computing, 104 (2022), 271–294. https://doi.org/10.1007/s00607-021-00908-y doi: 10.1007/s00607-021-00908-y
![]() |
[28] |
I. V. Pustokhina, D. A. Pustokhin, P. T. Nguyen, M. Elhoseny, K. Shankar, Multi-objective rain optimization algorithm with WELM model for customer churn prediction in telecommunication sector, Complex Intell. Syst., 2021. https://doi.org/10.1007/s40747-021-00353-6 doi: 10.1007/s40747-021-00353-6
![]() |
[29] |
A. Dalli, Impact of hyperparameters on Deep Learning model for customer churn prediction in telecommunication sector, Math. Probl. Eng., 2022 (2022), 4720539. https://doi.org/10.1155/2022/4720539 doi: 10.1155/2022/4720539
![]() |
1. | Dilshod Hudayberganov, Tukhtabek Rakhimov, Akmal Rakhimbaev, Ilmira Yusupova, Nilufar Sapaeva, I. Abdullayev, V. Kukhar, E. Akhmetshin, D. Bekjanov, A. Carballo-Penela, Some areas of development of foreign cooperation in the higher education system, 2023, 449, 2267-1242, 07001, 10.1051/e3sconf/202344907001 | |
2. | Hanan Abdullah Mengash, Nuha Alruwais, Fadoua Kouki, Chinu Singla, Elmouez Samir Abd Elhameed, Ahmed Mahmud, Archimedes Optimization Algorithm-Based Feature Selection with Hybrid Deep-Learning-Based Churn Prediction in Telecom Industries, 2023, 9, 2313-7673, 1, 10.3390/biomimetics9010001 | |
3. | Leena Jenefa, Dr.Karuppasamy Ramanathan, Namita Mishra, Vishnu Vishnu, M. Jayakumar, S. Ashok, 2024, chapter 6, 9798369322840, 127, 10.4018/979-8-3693-2284-0.ch006 | |
4. | Maria Eflova, Yulia Vinogradova, Aleksandr Vitushkin, I. Abdullayev, V. Kukhar, E. Akhmetshin, D. Bekjanov, A. Carballo-Penela, The impact of artificial intelligence on the development of modern society, 2023, 449, 2267-1242, 07005, 10.1051/e3sconf/202344907005 | |
5. | Kermen Naminova, Anna Auslender, Nurulla Fayzullayev, Ochir Andriyanov, Nodirbek Shamurotov, I. Abdullayev, V. Kukhar, E. Akhmetshin, D. Bekjanov, A. Carballo-Penela, Financing investment activities trends in the Russian Federation, 2023, 449, 2267-1242, 04005, 10.1051/e3sconf/202344904005 | |
6. | Alibek Rajabov, Ergash Ibadullaev, Bahtiyor Egamov, Xurshid Zaripov, Jaxongir Qosimov, I. Abdullayev, V. Kukhar, E. Akhmetshin, D. Bekjanov, A. Carballo-Penela, Empirical analysis of determining the influence of the level of innovative development on the economy of world countries, 2023, 449, 2267-1242, 02001, 10.1051/e3sconf/202344902001 | |
7. | Kaveh Faraji Googerdchi, Shahrokh Asadi, Seyed Mohammadbagher Jafari, Sajid Anwar, Customer churn modeling in telecommunication using a novel multi-objective evolutionary clustering-based ensemble learning, 2024, 19, 1932-6203, e0303881, 10.1371/journal.pone.0303881 | |
8. | Kacem Salmi, Aziz Hmioui, 2024, Tourist Experiences in Smart Cities, 979-8-3503-7400-1, 1, 10.1109/MSCC62288.2024.10697017 | |
9. | Bunyod Matyusupov, Elbek Khodjaniyazov, Manzura Masharipova, Firuz Gurbanov, V. Trukhachev, V. Khavinson, A. Zhuravlev, S. Belopukhov, R. Migunov, V. Kukhar, The concepts of Smart cities, Smart Tourism Destination and Smart Tourism Cities and their interrelationship, 2024, 82, 2117-4458, 06015, 10.1051/bioconf/20248206015 | |
10. | Dilshod Hudayberganov, Farxod Abdullaev, Tukhtabek Rakhimov, Maksuda Aminova, Sarvar Norboyev, I. Abdullayev, V. Kukhar, E. Akhmetshin, D. Bekjanov, A. Carballo-Penela, Possibilities of organizing smart markets in the region, 2023, 449, 2267-1242, 04001, 10.1051/e3sconf/202344904001 | |
11. | Eduard Osadchy, Irina Kosorukova, Kuvvat Khamraev, 2024, Chapter 18, 978-981-97-7879-9, 203, 10.1007/978-981-97-7880-5_18 | |
12. | S. Arockia Panimalar, A. Krishnakumar, S. Senthil Kumar, Intensified customer churn Prediction: Connectivity with weighted Multi-Layer Perceptron and Enhanced Multipath Back Propagation, 2024, 09574174, 125993, 10.1016/j.eswa.2024.125993 | |
13. | Ildar Begishev, Elena Kirillova, Saphiya Mukhametgaliyeva, E. Laxmi Lydia, 2024, Chapter 15, 978-981-97-4891-4, 177, 10.1007/978-981-97-4892-1_15 | |
14. | Prathibha R J, Anupama N, Sudarshan A S, Spellbound Kannada: Harnessing Conditional Generative Adversarial Networks for Transformative Word Suggestion Systems in Kannada Language Processing, 2025, 11, 2414-1399, 10.4108/eetiot.7792 |
Class | Accuracybal | Precision | Recall | F-Score | AUC Score |
Training Phase (90%) | |||||
Churn | 80.91 | 96.48 | 80.91 | 88.01 | 90.20 |
Non-Churn | 99.49 | 96.81 | 99.49 | 98.13 | 90.20 |
Average | 90.20 | 96.64 | 90.20 | 93.07 | 90.20 |
Testing Phase (10%) | |||||
Churn | 83.72 | 92.31 | 83.72 | 87.80 | 91.35 |
Non-Churn | 98.97 | 97.63 | 98.97 | 98.29 | 91.35 |
Average | 91.35 | 94.97 | 91.35 | 93.05 | 91.35 |
Training Phase (80%) | |||||
Churn | 80.61 | 98.14 | 80.61 | 88.52 | 90.17 |
Non-Churn | 99.74 | 96.76 | 99.74 | 98.22 | 90.17 |
Average | 90.17 | 97.45 | 90.17 | 93.37 | 90.17 |
Testing Phase (20%) | |||||
Churn | 80.22 | 98.65 | 80.22 | 88.48 | 90.02 |
Non-Churn | 99.83 | 96.96 | 99.83 | 98.37 | 90.02 |
Average | 90.02 | 97.81 | 90.02 | 93.43 | 90.02 |
Class | Accubal | Precn | Recal | Fscore | AUCscore |
Training Phase (70%) | |||||
Churn | 80.12 | 90.34 | 80.12 | 84.93 | 89.36 |
Non-Churn | 98.60 | 96.82 | 98.60 | 97.70 | 89.36 |
Average | 89.36 | 93.58 | 89.36 | 91.32 | 89.36 |
Testing Phase (30%) | |||||
Churn | 77.56 | 92.37 | 77.56 | 84.32 | 88.19 |
Non-Churn | 98.82 | 95.97 | 98.82 | 97.37 | 88.19 |
Average | 88.19 | 94.17 | 88.19 | 90.85 | 88.19 |
Training Phase (60%) | |||||
Churn | 84.06 | 95.87 | 84.06 | 89.58 | 91.74 |
Non-Churn | 99.42 | 97.50 | 99.42 | 98.45 | 91.74 |
Average | 91.74 | 96.68 | 91.74 | 94.01 | 91.74 |
Testing Phase (40%) | |||||
Churn | 83.09 | 98.29 | 83.09 | 90.05 | 91.41 |
Non-Churn | 99.73 | 96.98 | 99.73 | 98.34 | 91.41 |
Average | 91.41 | 97.63 | 91.41 | 94.20 | 91.41 |
Methods | Accuy | Precn | Recal | Fscore | AUCscore |
AIJOA-CPDE | 91.41 | 97.63 | 91.41 | 94.20 | 91.41 |
Logistic Regression [27] | 80.65 | 79.44 | 80.56 | 79.17 | 82.30 |
Decision Tree [27] | 76.78 | 56.90 | 75.81 | 65.09 | 78.37 |
ISMOTE-OWELM [28] | 90.59 | 91.78 | 89.51 | 89.77 | 89.96 |
SVM [28] | 84.41 | 84.66 | 84.11 | 85.71 | 84.10 |
SGD-NN [29] | 84.54 | 86.22 | 85.92 | 84.43 | 84.91 |
RMSProp-NN [29] | 87.48 | 85.29 | 85.30 | 85.20 | 86.38 |
Class | Accuracybal | Precision | Recall | F-Score | AUC Score |
Training Phase (90%) | |||||
Churn | 80.91 | 96.48 | 80.91 | 88.01 | 90.20 |
Non-Churn | 99.49 | 96.81 | 99.49 | 98.13 | 90.20 |
Average | 90.20 | 96.64 | 90.20 | 93.07 | 90.20 |
Testing Phase (10%) | |||||
Churn | 83.72 | 92.31 | 83.72 | 87.80 | 91.35 |
Non-Churn | 98.97 | 97.63 | 98.97 | 98.29 | 91.35 |
Average | 91.35 | 94.97 | 91.35 | 93.05 | 91.35 |
Training Phase (80%) | |||||
Churn | 80.61 | 98.14 | 80.61 | 88.52 | 90.17 |
Non-Churn | 99.74 | 96.76 | 99.74 | 98.22 | 90.17 |
Average | 90.17 | 97.45 | 90.17 | 93.37 | 90.17 |
Testing Phase (20%) | |||||
Churn | 80.22 | 98.65 | 80.22 | 88.48 | 90.02 |
Non-Churn | 99.83 | 96.96 | 99.83 | 98.37 | 90.02 |
Average | 90.02 | 97.81 | 90.02 | 93.43 | 90.02 |
Class | Accubal | Precn | Recal | Fscore | AUCscore |
Training Phase (70%) | |||||
Churn | 80.12 | 90.34 | 80.12 | 84.93 | 89.36 |
Non-Churn | 98.60 | 96.82 | 98.60 | 97.70 | 89.36 |
Average | 89.36 | 93.58 | 89.36 | 91.32 | 89.36 |
Testing Phase (30%) | |||||
Churn | 77.56 | 92.37 | 77.56 | 84.32 | 88.19 |
Non-Churn | 98.82 | 95.97 | 98.82 | 97.37 | 88.19 |
Average | 88.19 | 94.17 | 88.19 | 90.85 | 88.19 |
Training Phase (60%) | |||||
Churn | 84.06 | 95.87 | 84.06 | 89.58 | 91.74 |
Non-Churn | 99.42 | 97.50 | 99.42 | 98.45 | 91.74 |
Average | 91.74 | 96.68 | 91.74 | 94.01 | 91.74 |
Testing Phase (40%) | |||||
Churn | 83.09 | 98.29 | 83.09 | 90.05 | 91.41 |
Non-Churn | 99.73 | 96.98 | 99.73 | 98.34 | 91.41 |
Average | 91.41 | 97.63 | 91.41 | 94.20 | 91.41 |
Methods | Accuy | Precn | Recal | Fscore | AUCscore |
AIJOA-CPDE | 91.41 | 97.63 | 91.41 | 94.20 | 91.41 |
Logistic Regression [27] | 80.65 | 79.44 | 80.56 | 79.17 | 82.30 |
Decision Tree [27] | 76.78 | 56.90 | 75.81 | 65.09 | 78.37 |
ISMOTE-OWELM [28] | 90.59 | 91.78 | 89.51 | 89.77 | 89.96 |
SVM [28] | 84.41 | 84.66 | 84.11 | 85.71 | 84.10 |
SGD-NN [29] | 84.54 | 86.22 | 85.92 | 84.43 | 84.91 |
RMSProp-NN [29] | 87.48 | 85.29 | 85.30 | 85.20 | 86.38 |