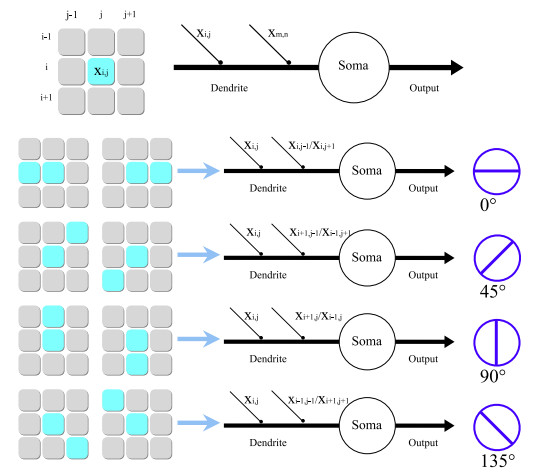
As the most studied sensory system, the visual system plays an important role in our understanding of brain functions. Biological researchers have divided the nerve cells in the retina into dozens of visual channels carrying various characteristics based on visual features. Although orientation-selective cells have been identified in the retinas of various animals, the specific neural circuits of such cells have been controversial. In this study, a new simple and efficient orientation detection model based on the perceptron is proposed to restore the neural circuitry of orientation-selective cells in the retina. The performance of this model is experimentally compared with that of the convolutional neural network for image orientation recognition, and the results verify that the proposed model offers very good orientation detection. The proposed perceptron-based orientation detection model provides a new perspective to explain the neural circuits of orientation-selective cells.
Citation: Fenggang Yuan, Cheng Tang, Zheng Tang, Yuki Todo. A model of amacrine cells for orientation detection[J]. Electronic Research Archive, 2023, 31(4): 1998-2018. doi: 10.3934/era.2023103
[1] | Han-Cheng Dan, Yongcheng Long, Hui Yao, Songlin Li, Yanhao Liu, Quanfeng Zhou . Investigation on the fractal characteristic of asphalt pavement texture roughness incorporating 3D reconstruction technology. Electronic Research Archive, 2023, 31(4): 2337-2357. doi: 10.3934/era.2023119 |
[2] | Abul Bashar . Employing combined spatial and frequency domain image features for machine learning-based malware detection. Electronic Research Archive, 2024, 32(7): 4255-4290. doi: 10.3934/era.2024192 |
[3] | Hao Yang, Peihan Wang, Fang Han, Qingyun Wang . An interpretable mechanism for grating-induced cross-inhibition and gamma oscillation based on a visual cortical neuronal network model. Electronic Research Archive, 2024, 32(4): 2936-2954. doi: 10.3934/era.2024134 |
[4] | Eray Önler . Feature fusion based artificial neural network model for disease detection of bean leaves. Electronic Research Archive, 2023, 31(5): 2409-2427. doi: 10.3934/era.2023122 |
[5] | Xue Yu . Orientable vertex imprimitive complete maps. Electronic Research Archive, 2024, 32(4): 2466-2477. doi: 10.3934/era.2024113 |
[6] | Yogesh Kumar Rathore, Rekh Ram Janghel, Chetan Swarup, Saroj Kumar Pandey, Ankit Kumar, Kamred Udham Singh, Teekam Singh . Detection of rice plant disease from RGB and grayscale images using an LW17 deep learning model. Electronic Research Archive, 2023, 31(5): 2813-2833. doi: 10.3934/era.2023142 |
[7] | Chao Chen, Hua Kong, Bin Wu . Edge detection of remote sensing image based on Grünwald-Letnikov fractional difference and Otsu threshold. Electronic Research Archive, 2023, 31(3): 1287-1302. doi: 10.3934/era.2023066 |
[8] | Chunyan An, Wei Bai, Donglei Zhang . Meet-in-the-middle differential fault analysis on Midori. Electronic Research Archive, 2023, 31(11): 6820-6832. doi: 10.3934/era.2023344 |
[9] | Chetan Swarup, Kamred Udham Singh, Ankit Kumar, Saroj Kumar Pandey, Neeraj varshney, Teekam Singh . Brain tumor detection using CNN, AlexNet & GoogLeNet ensembling learning approaches. Electronic Research Archive, 2023, 31(5): 2900-2924. doi: 10.3934/era.2023146 |
[10] | Yuhang Liu, Jun Chen, Yuchen Wang, Wei Wang . Interpretable machine learning models for detecting fine-grained transport modes by multi-source data. Electronic Research Archive, 2023, 31(11): 6844-6865. doi: 10.3934/era.2023346 |
As the most studied sensory system, the visual system plays an important role in our understanding of brain functions. Biological researchers have divided the nerve cells in the retina into dozens of visual channels carrying various characteristics based on visual features. Although orientation-selective cells have been identified in the retinas of various animals, the specific neural circuits of such cells have been controversial. In this study, a new simple and efficient orientation detection model based on the perceptron is proposed to restore the neural circuitry of orientation-selective cells in the retina. The performance of this model is experimentally compared with that of the convolutional neural network for image orientation recognition, and the results verify that the proposed model offers very good orientation detection. The proposed perceptron-based orientation detection model provides a new perspective to explain the neural circuits of orientation-selective cells.
The visual system is a component of the nervous system, which, as one of the most basic human sensory systems, gives humans the ability to visualize and perceive [1]. More than one-third of the human cerebral cortex is related to the visual system, and for humans, the vast majority of external information is obtained through vision. Thus, the visual system contributes to human cognition, decision-making, emotional behavior and other behaviors [2]. The complete visual system consists of the eye (especially the retina), the optic nerve, the optic cross, the visual tract, the lateral geniculate body, the visual cortex and the visual association cortex [3]. These structures are divided into the anterior and posterior visual pathways from the lateral geniculate body. The eye, as the first station in the anterior visual pathway, is responsible for the initial processing of visual information [4]. Light is refracted by the eye and then projected onto the retina. Photoreceptor cells in the retina convert light signals into electrical signals, transmit them to bipolar cells and ganglion cells and finally transmit action potential signals to the brain via the optic nerve [5]. Amacrine cells and horizontal cells are involved in lateral information transmission in the retina, thus forming various complex visual receptive fields, which are usually sensitive to certain specific features of visual information, such as color, size, distance and orientation [6]. Hence, visual information is usually initially processed in the retina into channels carrying various specific features [7]. Retinal cells that carry similar visual information features are classified under the same visual channel. To date, more than 30 visual channels have been verified by genetics and anatomy [8]. In the present study, we concentrate on the visual channels associated with orientation features.
Orientation-selective cells were first identified in the pigeon retina by Maturana and Frenk in 1963 [9], and Levick demonstrated similar orientation selectivity in the rabbit retina in 1967 [10]. Subsequent research was reported on the orientation selectivity of retinal cells in cats [11,12,13,14], turtles [15], mice [16,17,18], goldfish [19,20] and zebrafish [21,22]. The congruence between the functional roles of amacrine cells and retinal ganglion cells in orientation selection circuits was established by Paride Antinucci in 2013 [23]. The study of the cell-adhesion molecule Tenm3 further demonstrated that AC is a critical component of orientation selection in retinal ganglion cells [24]. In a study of rabbit retinal cells, researchers found that orientation-selective amacrine cells (OSACs) have radially symmetrical dendrites, and the receptive field of OSACs can be approximated as a circle [25,26]. A common feature evident in these vertebrate visual models is that an orientation-selective amacrine cell becomes an essential element in the visual circuitry for tuning neurons, and it is always present in the initial reception and transmission of visual information. In these models, the orientation tuning function exhibited by the amacrine cells tends to be determined more by their own morphological features than by the inhibition of the superficial cells. That is, the dendritic orientation of the amacrine cell itself determines the implementation of orientation-selective functions in initial visual information processing to a greater extent. OSACs are sensitive to orientation information stimuli that are consistent with the direction of dendrite growth and insensitive to orientation information stimuli that are inconsistent with the direction of dendrite growth; hence, OSACs have two types of expressions for orientation information stimuli: ON response and OFF response [27]. In essence, OSACs are activated only by stimuli from a specific orientation and do not respond to stimuli from other orientations.
There is great potential value in employing the biological properties and mechanisms of OSACs in the field of engineering. Accordingly, in this study, a perceptron-based orientation detection model (PODM) is proposed, and the effectiveness of the model for the orientation detection of objects in images is experimentally verified. As a single neuron is sensitive only to stimuli in a specific orientation, four neurons are inserted into the mechanism to detect information in four orientations. These neurons receive information in the receptive field and are activated by the corresponding information, in the same manner as OSACs receive information from photoreceptor cells and are sensitive to information in a specific orientation. This indicates that the PODM is highly compatible with the biological properties of the cell. The global orientation selection is dependent on the aggregation of neuronal activation in all receptive fields. To ensure the validity of the mechanism's orientation detection, the object features (e.g., color, location and shape) in the experimental dataset are randomly generated. The experimental results confirm that the PODM is always efficient in detecting the orientation of objects, regardless of changes in features such as the color, position and shape of the objects. Based on the experimental results, it is likely that more angles of orientation recognition can be achieved by adding more neurons to the model or by interacting information between a limited number of neurons. This may inspire new ideas to unravel the mysteries of the functioning of the visual system.
The perceptron was invented by Frank Rosenblatt in 1957 [28]. As a type of artificial neural network (ANN), it was designed from its inception to mimic the working mechanism of nerve cells. The state of a nerve cell depends on the strength of the information received from other nerve cells. When the strength of the information exceeds a certain threshold, the nerve cell is activated and generates action potentials, which are then transmitted to other neurons via synapses [29]. Corresponding underlying concepts in the perceptron include weight ω corresponding to synapses, bias b corresponding to thresholds and activation functions corresponding to cell bodies. The equation of the perceptron is shown below:
f(x)={1ifω⋅x+b>00else, | (2.1) |
where x is the input received by the current neuron. OSACs usually receive input from multiple photoreceptors to determine the orientation of the object comprehensively, rather than relying on a single photoreceptor [30]. In this work, the neuron is set to receive the grayscale values of two adjacent points to determine whether the neuron corresponding to the orientation where these two points are located is activated or not. When the grayscale values of the two adjacent points are equal, the object is considered to be in the orientation corresponding to the two points, and the corresponding neuron is activated. Considering that the human eye has a limited grayscale recognition rate, and the colors of real objects are not exactly the same, a threshold needs to be added to the mechanism to determine whether the grayscale values are the same (approximately) [31]. Since human individuals differ, the ability of the human eye to recognize the minimum grayscale difference varies. For our model, the threshold value, as a user-set parameter, is both a switch for the model to work properly and a fault tolerance for the model detection. The smaller that the threshold is set, the better the model will recognize the smallest grayscale difference, and the more it will be affected by the background color change; the larger that the threshold is set, the weaker the model will be at recognizing the smallest grayscale, and it is likely to lose the detection ability. So, we need to give the threshold a suitable value to give the model a certain error tolerance. During our experiment, we found that the model can maintain a more ideal working state when the threshold is set to 3, and thus we define the threshold as 3. When the difference between two points is less than or equal to the threshold value, the two points are considered to have the same (approximate) grayscale value, and the neurons of the corresponding orientation are activated; otherwise, the two points are regarded as having different grayscale values, and the neurons of the corresponding orientation are not activated. In the receptive field of neuronal cells, the central point is selected as the reference point, the grayscale values of eight adjacent points are compared with the reference point, and the neuronal cells of the corresponding orientation are activated if the difference in grayscale values is less than the threshold. Based on the aforementioned basic principles, we propose an equation to adapt our mechanism as shown below:
Response={ONif|x∗−xi|<thresholdOFFelse, | (2.2) |
where x∗ represents the grayscale value of the central reference point of the perceptual field, and the xi represents the grayscale value of the points adjacent to the reference point.
In this study, four neurons are set up in the mechanism to detect the four orientations of 0°, 45°, 90° and 135°, as shown in Figure 1. We define the photoreceptor cell in the center of the receptive field as the reference point with coordinates (i, j), and Xi,j represents the signal received from the photoreceptor cell by the amacrine cell. In such a receptive field, the horizontally oriented neuron (0 degrees) is activated when the signal received from the photoreceptor cell located at (i, j+1) or (i, j-1) is close to the signal at the reference point. A vertically oriented neuron (90 degrees) is activated when Xi+1,j or Xi−1,j is close to Xi,j. The neuron corresponding to 135 degrees is activated when the Xi+1,j+1 or Xi−1,j−1 is similar to Xi,j. The neuron corresponding to 45 degrees is activated when Xi−1,j+1 or Xi+1,j−1 is approaching Xi,j.
Neuronal cells based on this mechanism would be able to be activated by an object as small as 1*2 pixels. A simple demonstration is given in Figure 2. In the retina, photoreceptor cells receive light signals that are converted into electrical signals and then transmit information to cells in the posterior layer in turn. Two of the photoreceptor cells receive light signals, which are reflected in the current receptive field as the central point with the same signal as its horizontal neighbor, and activate the neuron in the amacrine cell layer in the corresponding horizontal orientation (0°).
When processing a complete image, it is necessary for the neurons to globally detect the image to detect the orientation of objects in the image. Therefore, the sliding window scanning mechanism from the convolutional neural network (CNN) [32] is utilized in this study. That is, the perceptual field slides sequentially from the beginning of the image to the next position in a fixed step, scanning over the whole image line by line to read the information of the whole image as an input to the model. The neurons corresponding to the four orientations are activated during the scanning process, the frequencies of activation are recorded and summarized at the same time, and the orientation detection results of the model are output after substitution into the activation function calculation. The equation of the activation function is shown below:
f(x)=eXi∑ni=1Xi | (2.3) |
where Xi represents the activation frequencies, and n equals 4, meaning that a total of four orientation-selective neurons are employed here. It is worth noting that the output here is the probability of selecting each orientation, and the sum of the probabilities of the four orientations being selected is 1. The final detection result of the model is taken from the one with the highest probability among the four orientations, i.e., the orientation with the highest activation frequency is considered to be the final detection result of the model. A simplified diagram of the model is shown in Figure 3. An example of the specific sliding window scanning mechanism is shown in Figure 4. Figure 4(a) shows an object which is sized at 1*3 and in the orientation of 135°, placed on an image with a gradient grayscale background. Figure 4(b) shows the whole process of receiving this image by the model. A receptive field of size 3*3 slides across the entire image from left to right line by line. In each receptive field, the neurons in the corresponding orientation are activated and recorded separately (activation is shown in highlighted blue). Figure 4(c) summarizes the result of the scan, and the direction with the highest number of neuron activations is considered to be the orientation of the object. It can be seen that the global detection result obtained by the four neurons matches the actual orientation of the object. Unlike the sliding window scanning in the CNN, the proposed neuron needs minimal information interaction to read the features of the whole picture completely, and thus using a 3*3 receptive field to scan the whole picture will cause unnecessary waste of computational resources. Therefore, the size of the receptive field is collapsed from 3*3 to 2*3, and the activation level of neurons in the corresponding orientation is weighted accordingly, as shown in Figure 5. The experimental results verify that this improvement saves approximately one-third of the computational resources while ensuring detection accuracy.
A series of experiments were conducted to evaluate the effectiveness of the suggested model. In the first subsection, the composition of the experimental dataset and the method it generates are described. In the second subsection, the mechanism proposed above is utilized to detect the orientation of each object in the dataset to validate the feasibility of the mechanism. In the third subsection, the PODM is compared with CNN to verify the robustness of the mechanism by detecting simulated realistic images and the detection accuracy when subjected to the same level of interference. The fourth subsection then compares the performances of the mechanism when different sizes of perceptual fields are applied.
All datasets were randomly generated and according to the following guidelines: Each dataset contains 2500 images, each image has a resolution of 100*100 pixels, the background color of the image is a randomly generated grayscale color, an object is placed on each image, and the orientation and color of the object are randomly generated. Figure 6 displays a partial sample of the dataset. The experiments are classified only according to the size of the object pixel values to check the accuracy and reliability of the model when dealing with objects of different sizes. Further experiments are applied to examine the ability of the proposed mechanism to cope with different backgrounds by replacing the filled form of the image background.
It is well known that a complete image is a combination of grayscale maps of multiple color channels. In this set of experiments, the validity of the proposed mechanism is verified in the monochromatic grayscale images. In this dataset, the background of the image and the color of the object in the image are each randomly generated monochromatic grayscale colors. The sizes of the objects in the images are divided into four categories: 50,100,500 and 1000 pixel values. The percentage of the object occupying the image ranges from 0.5 to 10%, which allows the tolerance of the proposed mechanism to object size to be fully investigated. Some samples from the monochrome grayscale image dataset are shown in Figure 6. It can be seen that the background of the image is a randomly generated monochrome grayscale, and the position of the object in the image, as well as its color, is random, while the aspect ratio (shape) of the object also has different variations. Figure 7 shows the detection result of the mechanism for one of the images in which the object has a size of approximately 500 pixels and an orientation of 135°. The activation intensity of neurons in the 135° orientation reaches 924, which is significantly higher than the activation intensity in the other three directions. The mechanism detection results point to 135°, which is consistent with the actual orientation of the object. It indicates that the proposed mechanism successfully detects the orientation of the object in that image. Table 1 presents the results obtained by the mechanism in this dataset. The mechanism had an accurate detection of the orientation of each object with a success rate of 100%, which demonstrates that the mechanism is always able to efficiently detect the orientation of the object in the images, regardless of the object's size, orientation, shape or color. Thus, the results indicate the mechanism has a stable recognition rate and good robustness in detecting the orientation of objects in monochrome grayscale images. Also, since monochrome grayscale images of multiple channels can be superimposed to form color images, the mechanism is likely also capable of detecting the orientation of objects in color images.
Object Size | Angle | 0° | 45° | 90° | 135° | Total |
50 | Number of images | 646 | 608 | 627 | 619 | 2500 |
Predicted number | 646 | 608 | 627 | 619 | 2500 | |
Accuracy rate | 100% | 100% | 100% | 100% | 100% | |
100 | Number of images | 641 | 589 | 644 | 626 | 2500 |
Predicted number | 641 | 589 | 644 | 626 | 2500 | |
Accuracy rate | 100% | 100% | 100% | 100% | 100% | |
500 | Number of images | 634 | 646 | 585 | 635 | 2500 |
Predicted number | 634 | 646 | 585 | 635 | 2500 | |
Accuracy rate | 100% | 100% | 100% | 100% | 100% | |
1000 | Number of images | 600 | 623 | 643 | 634 | 2500 |
Predicted number | 600 | 623 | 643 | 634 | 2500 | |
Accuracy rate | 100% | 100% | 100% | 100% | 100% |
The effectiveness of the mechanism in monochromatic grayscale images was verified in the previous set of experiments. However, ambient light in the real world often appears as gradient colors because the intensity of light is usually affected by many factors, such as temperature, humidity, light source proximity and irradiation angle. Therefore, in this set of experiments, the background of the images is replaced with a gradient grayscale color. It should be noted that to restore the background under various lighting conditions to the extent possible, the gradient direction of the grayscale background is random, and the grayscale value of the background is also random. An example is given in Figure 8. The background of this sample image is a grayscale color that fades to white (grayscale values become larger) from the upper left to the lower right, and there is an object of approximately 100 pixels in size and 45° orientation in the image. The detection result on the right side of the image shows that the activation intensity of neurons in the 45° direction reaches 336, which is stronger than the activation in other directions. Thus, the detection result of the mechanism is 45°, which matches with the actual orientation of the object. The results of this set of experiments are shown in Table 2, and it is clearly observed that the detection accuracy remains at 100% in all orientations. This indicates that even if the background is changed to a random gradient grayscale color, the proposed mechanism still recognizes the object independent of the background grayscale, object size, location, etc. It further supports the robustness and feasibility of the mechanism.
Object Size | Angle | 0° | 45° | 90° | 135° | Total |
50 | Number of images | 580 | 647 | 653 | 620 | 2500 |
Predicted number | 580 | 647 | 653 | 620 | 2500 | |
Accuracy rate | 100% | 100% | 100% | 100% | 100% | |
100 | Number of images | 611 | 635 | 648 | 606 | 2500 |
Predicted number | 611 | 635 | 648 | 606 | 2500 | |
Accuracy rate | 100% | 100% | 100% | 100% | 100% | |
500 | Number of images | 602 | 602 | 670 | 626 | 2500 |
Predicted numbers | 602 | 602 | 670 | 626 | 2500 | |
Accuracy rate | 100% | 100% | 100% | 100% | 100% | |
1000 | Number of images | 642 | 616 | 591 | 651 | 2500 |
Predicted number | 642 | 616 | 591 | 651 | 2500 | |
Accuracy rate | 100% | 100% | 100% | 100% | 100% |
Considering the excellent performance of CNNs in image processing, it is informative to compare the performance of the PODM with the CNN. The images for this set of experiments are still set to have gradient grayscale backgrounds, while, to increase the difficulty, different levels of salt-and-pepper noise are added to all of the images to test noise resistance. Salt-and-pepper noise, also known as impulse noise, is often observed in images. It is a random appearance of white or black dots, either as black pixels in bright areas or white pixels in dark areas (or both). The cause of such noise in an image is usually a sudden and strong disturbance of the transmitted signal. A partial failure of the sensor produces black dots (pepper) on the image, whereas an oversaturation of the sensor produces white dots (salt)[33]. Since the size of the largest object in the dataset in this study is about 1000 pixels, which occupies only 10% of the image size, a noise value is added in the range of 1–10% of the image size so that the maximum noise value is controlled to be no more than the size of the object. Two example sets of images with added noise are presented in Figure 10. The original image without noise is placed on the left, while the examples on the right present the actual image when the added noise is incremented from 1 to 10%. The added pepper noise is evenly and randomly distributed over the image, while the ratio of pepper noise to salt noise is 1:1. Figure 10(a) shows a horizontally placed object of size 50 pixels. When excessive noise is added, the shape of the object becomes greatly disturbed, offering a severe test for the detection mechanism. Figure 10(b) shows an object with an orientation of 135° and a size of 1000 pixels. Both sets of images are added with equal noise values, and it is obvious to the naked eye that the shapes of large objects are easier to distinguish compared with small objects. The detection results made by PODM for the orientation of the objects on these images are also similar to the perception of human eye observation, which to some extent indicates that PODM is built in accordance with the logic of human eye work.
The CNN is a feedforward neural network that includes convolutional computation and has a deep structure [34]. The "neocognitron" neural network proposed by Fukushima in 1980 is considered to be the inspiration for the CNN [35]. Alex formally proposed the first CNN called "time delay network" in 1987, which is mainly applied to the field of sound recognition [36]. In 1988, Wei Zhang proposed the first two-dimensional CNN [37]. Recently, the application area of CNNs has been extended to include portrait recognition [38] and gesture recognition [39]. An input layer, hidden layers and an output layer are the three components of a CNN. The hidden layer usually contains n convolutional and pooling layers and a fully connected layer. CNN usually consumes a large number of computational resources in the training process, and the more convolutional layers there are, the more computational resources are required. Therefore, to ensure the fairness of the experimental comparison, we allocate the computational resources of both methods using the time required to implement both methods on the same platform as a uniform metric (with an error of no more than 10%). In this experiment, only two convolutional layers are set, the size of the convolutional kernel is 3*3, the step size is 1, and the ratio of training set to test set is 7:3. A simplified diagram of the CNN for the present experiment is displayed in Figure 9. With such acceptable computational resource consumption, each set of experiments runs 30 times, and the results derived from the experiments will eventually be tested by hypothesis testing to verify whether the results are significantly different, as shown in Tables 3–6. These tables give the mean and standard deviation (SD) differences of the detection accuracies obtained from the two methods running 30 times each in the four sets of control experiments when adding different levels of noise to the images. Higher accuracy rates are bolded in the table. It is clear that PODM outperforms CNN in most instances at accurately detecting the orientation of an object. The detection accuracies of PODM and CNN are only comparable in images with large objects and high noise values. We employed a P-test to examine whether the accuracies of the two methods are fundamentally different. The P-test is a statistical method that is applied to examine the validity of a generally accepted hypothesis about the aggregate. The smaller the p-value is, the more evidence there is that the null hypothesis should be rejected, and the alternative hypothesis is more plausible [40]. In this set of experiments, the p-value is less than 0.05, which implies that there is a significant difference between the detection results of PODM and CNN. In other words, the detection results of PODM are significantly better than those of CNN. As can be seen from the results in the tables, all of the p-values are less than 0.05, which means there is a significant difference between the results obtained by the two methods. This indicates the PODM is always capable of achieving better detection results than those obtained by the CNN regardless of object size.
Noise | PODM | CNN | ||||
Mean(%) | ± | SD | Mean(%) | ± | SD | |
1% | 99.99 | ± | 0.0149 | 67.83 | ± | 0.1596 |
2% | 99.40 | ± | 0.1228 | 52.71 | ± | 0.1674 |
3% | 97.16 | ± | 0.3379 | 45.52 | ± | 0.1447 |
4% | 92.95 | ± | 0.4359 | 41.59 | ± | 0.1298 |
5% | 87.69 | ± | 0.7140 | 41.61 | ± | 0.1813 |
6% | 81.84 | ± | 0.5256 | 35.97 | ± | 0.1021 |
7% | 76.59 | ± | 0.7738 | 33.96 | ± | 0.1017 |
8% | 70.99 | ± | 0.7243 | 31.58 | ± | 0.1052 |
9% | 66.31 | ± | 0.8904 | 30.90 | ± | 0.0748 |
10% | 62.28 | ± | 0.7972 | 32.30 | ± | 0.0889 |
P-value | - | 8.64E-07 |
Noise | PODM | CNN | ||||
Mean(%) | ± | SD | Mean(%) | ± | SD | |
1% | 99.98 | ± | 0.0267 | 93.03 | ± | 0.0236 |
2% | 99.54 | ± | 0.1267 | 90.87 | ± | 0.0399 |
3% | 98.60 | ± | 0.2016 | 85.30 | ± | 0.1019 |
4% | 96.99 | ± | 0.2789 | 84.34 | ± | 0.1290 |
5% | 95.34 | ± | 0.3437 | 79.01 | ± | 0.1528 |
6% | 93.11 | ± | 0.4067 | 77.87 | ± | 0.1796 |
7% | 90.43 | ± | 0.4366 | 78.39 | ± | 0.1530 |
8% | 87.91 | ± | 0.6577 | 76.80 | ± | 0.1522 |
9% | 84.91 | ± | 0.5372 | 72.51 | ± | 0.1787 |
10% | 82.16 | ± | 0.7730 | 75.30 | ± | 0.1598 |
P-value | - | 9.53E-04 |
Noise | PODM | CNN | ||||
Mean(%) | ± | SD | Mean(%) | ± | SD | |
1% | 100.00 | ± | 0.0100 | 97.58 | ± | 0.0067 |
2% | 99.94 | ± | 0.0503 | 97.17 | ± | 0.0068 |
3% | 99.69 | ± | 0.1204 | 96.93 | ± | 0.0072 |
4% | 99.34 | ± | 0.1709 | 95.96 | ± | 0.0121 |
5% | 98.91 | ± | 0.1891 | 96.08 | ± | 0.0092 |
6% | 98.42 | ± | 0.2377 | 95.80 | ± | 0.0078 |
7% | 97.81 | ± | 0.3068 | 95.25 | ± | 0.0084 |
8% | 97.19 | ± | 0.3345 | 95.26 | ± | 0.0063 |
9% | 96.52 | ± | 0.2720 | 94.83 | ± | 0.0100 |
10% | 95.74 | ± | 0.3695 | 94.67 | ± | 0.0077 |
P-value | - | 5.13E-04 |
Noise | PODM | CNN | ||||
Mean(%) | ± | SD | Mean(%) | ± | SD | |
1% | 99.99 | ± | 0.0072 | 96.71 | ± | 0.0065 |
2% | 99.81 | ± | 2.8279 | 96.49 | ± | 0.0055 |
3% | 99.43 | ± | 0.7075 | 96.29 | ± | 0.0070 |
4% | 98.85 | ± | 0.1964 | 96.13 | ± | 0.0064 |
5% | 98.16 | ± | 0.1602 | 95.85 | ± | 0.0101 |
6% | 97.41 | ± | 0.2816 | 95.66 | ± | 0.0058 |
7% | 96.71 | ± | 0.3358 | 95.90 | ± | 0.0075 |
8% | 95.70 | ± | 0.3497 | 95.43 | ± | 0.0115 |
9% | 94.91 | ± | 0.3863 | 95.93 | ± | 0.0087 |
10% | 93.77 | ± | 0.3876 | 95.58 | ± | 0.0096 |
P-value | - | 4.76E-02 |
The results are also shown in the form of a line graph in Figure 11 for a more in-depth visual analysis of the differences between the two methods. It is obvious that the CNN responds more obviously to the effect of noise, and the obtained results are usually accompanied by certain volatility. This also indicates that the PODM has better noise tolerance than the CNN. Furthermore, in the recognition of small objects, especially when the noise value is larger than that of small objects, the recognition accuracy of the CNN decreases sharply, whereas the proposed PODM maintains acceptable accuracy. The results of this set of experiments reveal that the PODM has many advantages over the CNN, such as higher accuracy in detecting the orientation of objects (especially for small objects), better noise tolerance, less sensitivity to the influence of external factors and better robustness.
In this subsection, a comparison between the original PODM with a 3*3 receptive field and the evolved PODM with a 2*3 receptive field is performed. Gradient grayscale images make up this dataset for the comparative experiment. The size of the detected objects in the images is still divided into four categories, and the images are also added with different levels of noise. The results are shown in Tables 7–10. As can be seen from these tables, the detection accuracies achieved by both models with the same noise impact are very close, regardless of how the size of the object varies. The p-values obtained from the statistical hypothesis tests are all greater than 0.05, which indicates there is no difference in the final results between the two mechanisms with different receptive fields. Unlike the differences between the objects compared in the previous section, neither mechanism in this group imposes a high computational load on the computer, so the length of time taken is the only criterion for comparing the two mechanisms. The time recorded at the bottom of the table clearly shows that the mechanism with a perceptual field of 3*3 takes a significantly longer time. Furthermore, the reduced perceptual field of the mechanism does not reduce the accuracy of recognition. In other words, the improved PODM reduces the reuse rate of information while still maintaining the efficiency and robustness of orientation detection. This indicates that it is necessary and beneficial for the model to change the shape of the receptive field from 3*3 to 2*3.
Receptive field | 2*3 | 3*3 | ||||
Noise | Mean(%) | ± | SD | Mean(%) | ± | SD |
1% | 99.98 | ± | 0.0246 | 100.00 | ± | 0.0147 |
2% | 99.17 | ± | 0.1496 | 99.36 | ± | 0.1210 |
3% | 96.42 | ± | 0.3577 | 96.96 | ± | 0.3363 |
4% | 92.11 | ± | 0.6329 | 92.80 | ± | 0.4348 |
5% | 86.64 | ± | 0.5642 | 88.00 | ± | 0.7038 |
6% | 80.74 | ± | 0.9089 | 81.68 | ± | 0.5230 |
7% | 75.04 | ± | 0.8508 | 77.48 | ± | 0.7652 |
8% | 70.24 | ± | 0.8117 | 71.56 | ± | 0.7256 |
9% | 65.31 | ± | 0.8048 | 66.24 | ± | 0.8806 |
10% | 61.68 | ± | 0.9211 | 62.28 | ± | 0.7914 |
P-value | - | 8.84E-01 | ||||
Time cost | 2193.02 s | 3378.61 s |
Receptive field | 2*3 | 3*3 | ||||
Noise | Mean(%) | ± | SD | Mean(%) | ± | SD |
1% | 99.95 | ± | 0.0377 | 99.96 | ± | 0.0266 |
2% | 99.39 | ± | 0.1232 | 99.72 | ± | 0.1251 |
3% | 98.23 | ± | 0.2248 | 98.68 | ± | 0.1983 |
4% | 96.71 | ± | 0.3103 | 97.00 | ± | 0.2752 |
5% | 94.97 | ± | 0.3515 | 95.08 | ± | 0.3446 |
6% | 92.70 | ± | 0.4397 | 92.88 | ± | 0.4001 |
7% | 90.19 | ± | 0.4157 | 90.44 | ± | 0.4311 |
8% | 87.31 | ± | 0.6059 | 87.44 | ± | 0.6692 |
9% | 84.57 | ± | 0.5317 | 84.36 | ± | 0.5287 |
10% | 81.49 | ± | 0.7801 | 82.12 | ± | 0.7805 |
P-value | - | 9.40E-01 | ||||
Time cost | 2195.05 s | 3312.37 s |
Receptive field | 2*3 | 3*3 | ||||
Noise | Mean(%) | ± | SD | Mean(%) | ± | SD |
1% | 99.99 | ± | 0.0149 | 100.00 | ± | 0.0098 |
2% | 99.91 | ± | 0.0572 | 99.84 | ± | 0.0507 |
3% | 99.65 | ± | 0.1274 | 99.72 | ± | 0.1222 |
4% | 99.27 | ± | 0.1609 | 99.40 | ± | 0.1699 |
5% | 98.78 | ± | 0.2088 | 98.84 | ± | 0.1891 |
6% | 98.32 | ± | 0.2011 | 98.20 | ± | 0.2344 |
7% | 97.63 | ± | 0.1843 | 97.64 | ± | 0.3094 |
8% | 97.04 | ± | 0.2397 | 97.56 | ± | 0.3291 |
9% | 96.41 | ± | 0.3563 | 96.56 | ± | 0.2679 |
10% | 95.69 | ± | 0.3067 | 95.72 | ± | 0.3724 |
P-value | - | 9.06E-01 | ||||
Time cost | 2214.19 s | 3379.97 s |
Receptive field | 2*3 | 3*3 | ||||
Noise | Mean(%) | ± | SD | Mean(%) | ± | SD |
1% | 99.99 | ± | 0.0181 | 100.00 | ± | 0.0071 |
2% | 99.81 | ± | 0.0696 | 99.84 | ± | 2.7832 |
3% | 99.43 | ± | 0.1429 | 99.56 | ± | 0.6968 |
4% | 98.85 | ± | 0.1799 | 99.00 | ± | 0.1933 |
5% | 98.16 | ± | 0.2300 | 98.24 | ± | 0.1582 |
6% | 97.41 | ± | 0.3432 | 97.32 | ± | 0.2833 |
7% | 96.71 | ± | 0.3401 | 96.36 | ± | 0.3413 |
8% | 95.70 | ± | 0.3142 | 95.60 | ± | 0.3461 |
9% | 94.91 | ± | 0.3150 | 95.08 | ± | 0.3803 |
10% | 93.77 | ± | 0.3777 | 94.00 | ± | 0.3815 |
P-value | - | 9.84E-01 | ||||
Time cost | 2335.16 s | 3583.72 s |
A set of experiments on the orientation detection of natural objects is added in this section to further validate the confidence level of the PODM. This dataset contains 50 images of size 100*100 with objects such as pens of various colors, contrails of airplanes, elongated stars, elongated drops of water, etc. The orientation of these objects in the images also varies, and there are various light disturbances in the background of the images because of the shooting angle. This imposes some demands on the ability of the PODM to detect the orientation. Meanwhile, to further evaluate the performance of the model, we conducted a downsampling operation on all the images in this dataset, and the size of the images after the operation became 50*50. The image downsampling operation usually reduces the image quality while decreasing the image size, so it can perfectly reproduce the actual quality reduction process of the image delivery. Some of the examples are shown in Figure 12, and the detection results on both the original images and downsampled images are displayed in Table 11. It can be seen that the PODM still achieves 100% correctness in dealing with these practical problems, which proves that the proposed model is also effective in dealing with practical problems and that the stability of getting correct results can be trusted.
Angle | 0° | 45° | 90° | 135° | Total |
Original images | 16 | 10 | 13 | 11 | 50 |
Predicted number | 16 | 10 | 13 | 11 | 50 |
Accuracy rate | 100% | 100% | 100% | 100% | 100% |
Downsampled images | 16 | 10 | 13 | 11 | 50 |
Predicted number | 16 | 10 | 13 | 11 | 50 |
Accuracy rate | 100% | 100% | 100% | 100% | 100% |
The motivation of this study was to propose a perceptron-based orientation detection mechanism (PODM) inspired by the working mechanism of amacrine cells and to verify the effectiveness of the mechanism through a series of experiments. As color images are usually superimposed by multiple channels of grayscale images, it is reasonable to believe that the successful orientation detection of the classical monochrome grayscale image means the mechanism will also be effective for object orientation detection in color images. Images with gradient grayscale backgrounds can restore the state of actual objects to some extent, and the successful detection of object orientation in such images verifies that the mechanism is also competent for actual object orientation recognition. To further corroborate the effectiveness of the mechanism, it is compared with CNN, the state-of-the-art method in image recognition processing. Different levels of noise are added to the dataset to compare the accuracy of both methods for object orientation recognition under the same interference conditions. The results also confirm that the PODM is superior to the CNN in terms of accuracy, noise tolerance and robustness. Because each feature of the object in the dataset is randomly generated, it can be concluded that the orientation detection of the object by the PODM is always efficient and consistent, regardless of the color, size or shape of the object. The detection results of PODM for noise-affected images closely resemble the perception of the human eye, and we are confident that this model can account for the functioning of the human visual system. In other words, it can give biologists a fresh perspective when conducting research on the visual system.
The authors declare there is no conflict of interest.
[1] | D. Milner, M. Goodale, The Visual Brain in Action, OUP Oxford, 2006. https://doi.org/10.1093/acprof:oso/9780198524724.001.0001 |
[2] | S. T. Fiske, S. E. Taylor, Social Cognition, Mcgraw-Hill Book Company, 1991. |
[3] | M. J. Tovée, An Introduction to the Visual System, Cambridge University Press, 1996. |
[4] |
D. C. Burr, M. C. Morrone, J. Ross, Selective suppression of the magnocellular visual pathway during saccadic eye movements, Nature, 371 (1994), 511–513. https://doi.org/10.1038/371511a0 doi: 10.1038/371511a0
![]() |
[5] |
T. Soldatos, D. Karakitsos, K. Chatzimichail, M. Papathanasiou, A. Gouliamos, A. Karabinis, Optic nerve sonography in the diagnostic evaluation of adult brain injury, Crit. Care, 12 (2008), R67. https://doi.org/10.1186/cc6897 doi: 10.1186/cc6897
![]() |
[6] |
A. Kaneko, Receptive field organization of bipolar and amacrine cells in the goldfish retina, J. Physiol., 235 (1973), 133–153. https://doi.org/10.1113/jphysiol.1973.sp010381 doi: 10.1113/jphysiol.1973.sp010381
![]() |
[7] |
D. I. Vaney, B. Sivyer, W. R. Taylor, Direction selectivity in the retina: symmetry and asymmetry in structure and function, Nat. Rev. Neurosci., 13 (2012), 194–208. https://doi.org/10.1038/nrn3165 doi: 10.1038/nrn3165
![]() |
[8] |
T. Baden, P. Berens, K. Franke, M. R. Rosón, M. Bethge, T. Euler, The functional diversity of retinal ganglion cells in the mouse, Nature, 529 (2016), 345–350. https://doi.org/10.1038/nature16468 doi: 10.1038/nature16468
![]() |
[9] |
H. R. Maturana, S. Frenk, Directional movement and horizontal edge detectors in the pigeon retina, Science, 142 (1963), 977–979. https://doi.org/10.1126/science.142.3594.977 doi: 10.1126/science.142.3594.977
![]() |
[10] |
W. R. Levick, Receptive fields and trigger features of ganglion cells in the visual streak of the rabbit's retina, J. Physiol., 188 (1967), 285–307. https://doi.org/10.1113/jphysiol.1967.sp008140 doi: 10.1113/jphysiol.1967.sp008140
![]() |
[11] |
D. H. Hubel, T. N. Wiesel, Receptive fields, binocular interaction and functional architecture in the cat's visual cortex, J. Physiol., 160 (1962), 106–154. https://doi.org/10.1113/jphysiol.1962.sp006837 doi: 10.1113/jphysiol.1962.sp006837
![]() |
[12] |
W. R. Levick, L. N. Thibos, Orientation bias of cat retinal ganglion cells, Nature, 286 (1980), 389–390. https://doi.org/10.1038/286389a0 doi: 10.1038/286389a0
![]() |
[13] |
W. R. Levick, L. N. Thibos, Analysis of orientation bias in cat retina, J. Physiol., 329 (1982), 243–261. https://doi.org/10.1113/jphysiol.1982.sp014301 doi: 10.1113/jphysiol.1982.sp014301
![]() |
[14] |
L. N. Thibos, W. R. Levick, Orientation bias of brisk-transient y-cells of the cat retina for drifting and alternating gratings, Exp. Brain Res., 58 (1985), 1–10. https://doi.org/10.1007/BF00238948 doi: 10.1007/BF00238948
![]() |
[15] |
E. Sernagor, N. M. Grzywacz, Emergence of complex receptive field properties of ganglion cells in the developing turtle retina, J. Neurophysiol., 73 (1995), 1355–1364. https://doi.org/10.1152/jn.1995.73.4.1355 doi: 10.1152/jn.1995.73.4.1355
![]() |
[16] |
J. H. Marshel, A. P. Kaye, I. Nauhaus, E. M. Callaway, Anterior-posterior direction opponency in the superficial mouse lateral geniculate nucleus, Neuron, 76 (2012), 713–720. https://doi.org/10.1016/j.neuron.2012.09.021 doi: 10.1016/j.neuron.2012.09.021
![]() |
[17] |
D. M. Piscopo, R. N. El-Danaf, A. D. Huberman, C. M. Niell, Diverse visual features encoded in mouse lateral geniculate nucleus, J. Neurophysiol., 33 (2013), 4642–4656. https://doi.org/10.1523/JNEUROSCI.5187-12.2013 doi: 10.1523/JNEUROSCI.5187-12.2013
![]() |
[18] |
B. Scholl, A. Y. Y. Tan, J. Corey, N. J. Priebe, Emergence of orientation selectivity in the mammalian visual pathway, J. Neurophysiol., 33 (2013), 10616–10624. https://doi.org/10.1523/JNEUROSCI.0404-13.2013 doi: 10.1523/JNEUROSCI.0404-13.2013
![]() |
[19] |
I. Damjanović, E. Maximova, V. Maximov, On the organization of receptive fields of orientation-selective units recorded in the fish tectum, J. Integr. Neurosci., 8 (2009), 323–344. https://doi.org/10.1142/S0219635209002174 doi: 10.1142/S0219635209002174
![]() |
[20] |
J. Johnston, H. Ding, S. H. Seibel, F. Esposti, L. Lagnado, Rapid mapping of visual receptive fields by filtered back projection: application to multi-neuronal electrophysiology and imaging, J. Neurophysiol., 592 (2014), 4839–4854. https://doi.org/10.1113/jphysiol.2014.276642 doi: 10.1113/jphysiol.2014.276642
![]() |
[21] |
N. Nikolaou, A. S. Lowe, A. S. Walker, F. Abbas, P. R. Hunter, I. D. Thompson, et al., Parametric functional maps of visual inputs to the tectum, Neuron, 76 (2012), 317–324. https://doi.org/10.1016/j.neuron.2012.08.040 doi: 10.1016/j.neuron.2012.08.040
![]() |
[22] |
A. S. Lowe, N. Nikolaou, P. R. Hunter, I. D. Thompson, M. P. Meyer, A systems-based dissection of retinal inputs to the zebrafish tectum reveals different rules for different functional classes during development, J. Neurophysiol., 33 (2013), 13946–13956. https://doi.org/10.1523/JNEUROSCI.1866-13.2013 doi: 10.1523/JNEUROSCI.1866-13.2013
![]() |
[23] |
P. Antinucci, N. Nikolaou, M. P. Meyer, R. Hindges, Teneurin-3 specifies morphological and functional connectivity of retinal ganglion cells in the vertebrate visual system, Cell Rep., 5 (2013), 582–592. https://doi.org/10.1016/j.celrep.2013.09.045 doi: 10.1016/j.celrep.2013.09.045
![]() |
[24] |
P. Antinucci, O. Suleyman, C. Monfries, R. Hindges, Neural mechanisms generating orientation selectivity in the retina, Curr. Biol., 26 (2016), 1802–1815. https://doi.org/10.1016/j.cub.2016.05.035 doi: 10.1016/j.cub.2016.05.035
![]() |
[25] |
S. A. Bloomfield, Two types of orientation-sensitive responses of amacrine cells in the mammalian retina, Nature, 350 (1991), 347–350. https://doi.org/10.1038/350347a0 doi: 10.1038/350347a0
![]() |
[26] |
S. A. Bloomfield, Orientation-sensitive amacrine and ganglion cells in the rabbit retina, J. Neurophysiol., 71 (1994), 1672–1691. https://doi.org/10.1152/jn.1994.71.5.1672 doi: 10.1152/jn.1994.71.5.1672
![]() |
[27] |
R. Nelson, E. V. F. Jr, H. Kolb, Intracellular staining reveals different levels of stratification for on-and off-center ganglion cells in cat retina, J. Neurophysiol., 41 (1978), 472–483. https://doi.org/10.1152/jn.1978.41.2.472 doi: 10.1152/jn.1978.41.2.472
![]() |
[28] | F. Rosenblatt, The Perceptron, A perceiving and Recognizing Automaton Project Para, Cornell Aeronautical Laboratory, 1957. |
[29] |
J. R. Huguenard, Low-threshold calcium currents in central nervous system neurons, Annu. Rev. Physiol., 58 (1996), 329–348. https://doi.org/10.1146/annurev.ph.58.030196.001553 doi: 10.1146/annurev.ph.58.030196.001553
![]() |
[30] |
A. Borst, T. Euler, Seeing things in motion: models, circuits, and mechanisms, Neuron, 71 (2011), 974–994. https://doi.org/10.1016/j.neuron.2011.08.031 doi: 10.1016/j.neuron.2011.08.031
![]() |
[31] | A. B. Watson, G. Y. Yang, J. A. Solomon, J. D. Villasenor, Visual thresholds for wavelet quantization error, in Hum. Vision Electron. Imaging, 2657 (1996), 382–392. https://doi.org/10.1117/12.238735 |
[32] |
S. Lawrence, C. L. Giles, A. C. Tsoi, A. D. Back, Face recognition: a convolutional neural-network approach, IEEE Trans. Neural Networks, 8 (1997), 98–113. https://doi.org/10.1109/72.554195 doi: 10.1109/72.554195
![]() |
[33] |
F. A. Gerritsen, P. W. Verbeek, Implementation of cellular-logic operators using 33 convolution and table lookup hardware, Comput. Vision Graphics Image Process., 27 (1984), 115–123. https://doi.org/10.1016/0734-189X(84)90086-0 doi: 10.1016/0734-189X(84)90086-0
![]() |
[34] | M. Liang, X. Hu, Recurrent convolutional neural network for object recognition, in Proceedings of the IEEE Conference on Computer Vision and Pattern Recognition (CVPR), (2015), 3367–3375. Available from: https://openaccess.thecvf.com/content_cvpr_2015/html/Liang_Recurrent_Convolutional_Neural_2015_CVPR_paper.html. |
[35] | K. Fukushima, S. Miyake, Neocognitron: a self-organizing neural network model for a mechanism of visual pattern recognition, in Competition and Cooperation in Neural Nets, (1982), 267–285. https://doi.org/10.1007/978-3-642-46466-9_18 |
[36] |
A. Waibel, Modular construction of time-delay neural networks for speech recognition, Neural Comput., 1 (1989), 39–46. https://doi.org/10.1162/neco.1989.1.1.39 doi: 10.1162/neco.1989.1.1.39
![]() |
[37] | W. Zhang, J. Tanida, K. Itoh, Y. Ichioka, Shift-invariant pattern recognition neural network and its optical architecture, in Proceedings of Annual Conference of the Japan Society of Applied Physics, (1988), 2147–2151. |
[38] |
C. Garcia, M. Delakis, Convolutional face finder: a neural architecture for fast and robust face detection, IEEE Trans. Pattern Anal. Mach. Intell., 26 (2004), 1408–1423. https://doi.org/10.1109/TPAMI.2004.97 doi: 10.1109/TPAMI.2004.97
![]() |
[39] | J. Platt, S. Nowlan, A convolutional neural network hand tracker, Proc. Adv. Neural Inf. Process. Syst., 1995 (1995), 901–908. Available from: https://www.microsoft.com/en-us/research/wp-content/uploads/2016/02/cnnHand.pdf. |
[40] |
S. Greenland, S. J. Senn, K. J. Rothman, J. B. Carlin, C. Poole, S. N. Goodman, et al., Statistical tests, p values, confidence intervals, and power: a guide to misinterpretations, Eur. J. Epidemiol., 31 (2016), 337–350. https://doi.org/10.1007/s10654-016-0149-3 doi: 10.1007/s10654-016-0149-3
![]() |
1. | Shangce Gao, Rong-Long Wang, Dongbao Jia, Ting Jin, Special Issue: Artificial intelligence and computational intelligence, 2023, 31, 2688-1594, 7556, 10.3934/era.2023381 |
Object Size | Angle | 0° | 45° | 90° | 135° | Total |
50 | Number of images | 646 | 608 | 627 | 619 | 2500 |
Predicted number | 646 | 608 | 627 | 619 | 2500 | |
Accuracy rate | 100% | 100% | 100% | 100% | 100% | |
100 | Number of images | 641 | 589 | 644 | 626 | 2500 |
Predicted number | 641 | 589 | 644 | 626 | 2500 | |
Accuracy rate | 100% | 100% | 100% | 100% | 100% | |
500 | Number of images | 634 | 646 | 585 | 635 | 2500 |
Predicted number | 634 | 646 | 585 | 635 | 2500 | |
Accuracy rate | 100% | 100% | 100% | 100% | 100% | |
1000 | Number of images | 600 | 623 | 643 | 634 | 2500 |
Predicted number | 600 | 623 | 643 | 634 | 2500 | |
Accuracy rate | 100% | 100% | 100% | 100% | 100% |
Object Size | Angle | 0° | 45° | 90° | 135° | Total |
50 | Number of images | 580 | 647 | 653 | 620 | 2500 |
Predicted number | 580 | 647 | 653 | 620 | 2500 | |
Accuracy rate | 100% | 100% | 100% | 100% | 100% | |
100 | Number of images | 611 | 635 | 648 | 606 | 2500 |
Predicted number | 611 | 635 | 648 | 606 | 2500 | |
Accuracy rate | 100% | 100% | 100% | 100% | 100% | |
500 | Number of images | 602 | 602 | 670 | 626 | 2500 |
Predicted numbers | 602 | 602 | 670 | 626 | 2500 | |
Accuracy rate | 100% | 100% | 100% | 100% | 100% | |
1000 | Number of images | 642 | 616 | 591 | 651 | 2500 |
Predicted number | 642 | 616 | 591 | 651 | 2500 | |
Accuracy rate | 100% | 100% | 100% | 100% | 100% |
Noise | PODM | CNN | ||||
Mean(%) | ± | SD | Mean(%) | ± | SD | |
1% | 99.99 | ± | 0.0149 | 67.83 | ± | 0.1596 |
2% | 99.40 | ± | 0.1228 | 52.71 | ± | 0.1674 |
3% | 97.16 | ± | 0.3379 | 45.52 | ± | 0.1447 |
4% | 92.95 | ± | 0.4359 | 41.59 | ± | 0.1298 |
5% | 87.69 | ± | 0.7140 | 41.61 | ± | 0.1813 |
6% | 81.84 | ± | 0.5256 | 35.97 | ± | 0.1021 |
7% | 76.59 | ± | 0.7738 | 33.96 | ± | 0.1017 |
8% | 70.99 | ± | 0.7243 | 31.58 | ± | 0.1052 |
9% | 66.31 | ± | 0.8904 | 30.90 | ± | 0.0748 |
10% | 62.28 | ± | 0.7972 | 32.30 | ± | 0.0889 |
P-value | - | 8.64E-07 |
Noise | PODM | CNN | ||||
Mean(%) | ± | SD | Mean(%) | ± | SD | |
1% | 99.98 | ± | 0.0267 | 93.03 | ± | 0.0236 |
2% | 99.54 | ± | 0.1267 | 90.87 | ± | 0.0399 |
3% | 98.60 | ± | 0.2016 | 85.30 | ± | 0.1019 |
4% | 96.99 | ± | 0.2789 | 84.34 | ± | 0.1290 |
5% | 95.34 | ± | 0.3437 | 79.01 | ± | 0.1528 |
6% | 93.11 | ± | 0.4067 | 77.87 | ± | 0.1796 |
7% | 90.43 | ± | 0.4366 | 78.39 | ± | 0.1530 |
8% | 87.91 | ± | 0.6577 | 76.80 | ± | 0.1522 |
9% | 84.91 | ± | 0.5372 | 72.51 | ± | 0.1787 |
10% | 82.16 | ± | 0.7730 | 75.30 | ± | 0.1598 |
P-value | - | 9.53E-04 |
Noise | PODM | CNN | ||||
Mean(%) | ± | SD | Mean(%) | ± | SD | |
1% | 100.00 | ± | 0.0100 | 97.58 | ± | 0.0067 |
2% | 99.94 | ± | 0.0503 | 97.17 | ± | 0.0068 |
3% | 99.69 | ± | 0.1204 | 96.93 | ± | 0.0072 |
4% | 99.34 | ± | 0.1709 | 95.96 | ± | 0.0121 |
5% | 98.91 | ± | 0.1891 | 96.08 | ± | 0.0092 |
6% | 98.42 | ± | 0.2377 | 95.80 | ± | 0.0078 |
7% | 97.81 | ± | 0.3068 | 95.25 | ± | 0.0084 |
8% | 97.19 | ± | 0.3345 | 95.26 | ± | 0.0063 |
9% | 96.52 | ± | 0.2720 | 94.83 | ± | 0.0100 |
10% | 95.74 | ± | 0.3695 | 94.67 | ± | 0.0077 |
P-value | - | 5.13E-04 |
Noise | PODM | CNN | ||||
Mean(%) | ± | SD | Mean(%) | ± | SD | |
1% | 99.99 | ± | 0.0072 | 96.71 | ± | 0.0065 |
2% | 99.81 | ± | 2.8279 | 96.49 | ± | 0.0055 |
3% | 99.43 | ± | 0.7075 | 96.29 | ± | 0.0070 |
4% | 98.85 | ± | 0.1964 | 96.13 | ± | 0.0064 |
5% | 98.16 | ± | 0.1602 | 95.85 | ± | 0.0101 |
6% | 97.41 | ± | 0.2816 | 95.66 | ± | 0.0058 |
7% | 96.71 | ± | 0.3358 | 95.90 | ± | 0.0075 |
8% | 95.70 | ± | 0.3497 | 95.43 | ± | 0.0115 |
9% | 94.91 | ± | 0.3863 | 95.93 | ± | 0.0087 |
10% | 93.77 | ± | 0.3876 | 95.58 | ± | 0.0096 |
P-value | - | 4.76E-02 |
Receptive field | 2*3 | 3*3 | ||||
Noise | Mean(%) | ± | SD | Mean(%) | ± | SD |
1% | 99.98 | ± | 0.0246 | 100.00 | ± | 0.0147 |
2% | 99.17 | ± | 0.1496 | 99.36 | ± | 0.1210 |
3% | 96.42 | ± | 0.3577 | 96.96 | ± | 0.3363 |
4% | 92.11 | ± | 0.6329 | 92.80 | ± | 0.4348 |
5% | 86.64 | ± | 0.5642 | 88.00 | ± | 0.7038 |
6% | 80.74 | ± | 0.9089 | 81.68 | ± | 0.5230 |
7% | 75.04 | ± | 0.8508 | 77.48 | ± | 0.7652 |
8% | 70.24 | ± | 0.8117 | 71.56 | ± | 0.7256 |
9% | 65.31 | ± | 0.8048 | 66.24 | ± | 0.8806 |
10% | 61.68 | ± | 0.9211 | 62.28 | ± | 0.7914 |
P-value | - | 8.84E-01 | ||||
Time cost | 2193.02 s | 3378.61 s |
Receptive field | 2*3 | 3*3 | ||||
Noise | Mean(%) | ± | SD | Mean(%) | ± | SD |
1% | 99.95 | ± | 0.0377 | 99.96 | ± | 0.0266 |
2% | 99.39 | ± | 0.1232 | 99.72 | ± | 0.1251 |
3% | 98.23 | ± | 0.2248 | 98.68 | ± | 0.1983 |
4% | 96.71 | ± | 0.3103 | 97.00 | ± | 0.2752 |
5% | 94.97 | ± | 0.3515 | 95.08 | ± | 0.3446 |
6% | 92.70 | ± | 0.4397 | 92.88 | ± | 0.4001 |
7% | 90.19 | ± | 0.4157 | 90.44 | ± | 0.4311 |
8% | 87.31 | ± | 0.6059 | 87.44 | ± | 0.6692 |
9% | 84.57 | ± | 0.5317 | 84.36 | ± | 0.5287 |
10% | 81.49 | ± | 0.7801 | 82.12 | ± | 0.7805 |
P-value | - | 9.40E-01 | ||||
Time cost | 2195.05 s | 3312.37 s |
Receptive field | 2*3 | 3*3 | ||||
Noise | Mean(%) | ± | SD | Mean(%) | ± | SD |
1% | 99.99 | ± | 0.0149 | 100.00 | ± | 0.0098 |
2% | 99.91 | ± | 0.0572 | 99.84 | ± | 0.0507 |
3% | 99.65 | ± | 0.1274 | 99.72 | ± | 0.1222 |
4% | 99.27 | ± | 0.1609 | 99.40 | ± | 0.1699 |
5% | 98.78 | ± | 0.2088 | 98.84 | ± | 0.1891 |
6% | 98.32 | ± | 0.2011 | 98.20 | ± | 0.2344 |
7% | 97.63 | ± | 0.1843 | 97.64 | ± | 0.3094 |
8% | 97.04 | ± | 0.2397 | 97.56 | ± | 0.3291 |
9% | 96.41 | ± | 0.3563 | 96.56 | ± | 0.2679 |
10% | 95.69 | ± | 0.3067 | 95.72 | ± | 0.3724 |
P-value | - | 9.06E-01 | ||||
Time cost | 2214.19 s | 3379.97 s |
Receptive field | 2*3 | 3*3 | ||||
Noise | Mean(%) | ± | SD | Mean(%) | ± | SD |
1% | 99.99 | ± | 0.0181 | 100.00 | ± | 0.0071 |
2% | 99.81 | ± | 0.0696 | 99.84 | ± | 2.7832 |
3% | 99.43 | ± | 0.1429 | 99.56 | ± | 0.6968 |
4% | 98.85 | ± | 0.1799 | 99.00 | ± | 0.1933 |
5% | 98.16 | ± | 0.2300 | 98.24 | ± | 0.1582 |
6% | 97.41 | ± | 0.3432 | 97.32 | ± | 0.2833 |
7% | 96.71 | ± | 0.3401 | 96.36 | ± | 0.3413 |
8% | 95.70 | ± | 0.3142 | 95.60 | ± | 0.3461 |
9% | 94.91 | ± | 0.3150 | 95.08 | ± | 0.3803 |
10% | 93.77 | ± | 0.3777 | 94.00 | ± | 0.3815 |
P-value | - | 9.84E-01 | ||||
Time cost | 2335.16 s | 3583.72 s |
Angle | 0° | 45° | 90° | 135° | Total |
Original images | 16 | 10 | 13 | 11 | 50 |
Predicted number | 16 | 10 | 13 | 11 | 50 |
Accuracy rate | 100% | 100% | 100% | 100% | 100% |
Downsampled images | 16 | 10 | 13 | 11 | 50 |
Predicted number | 16 | 10 | 13 | 11 | 50 |
Accuracy rate | 100% | 100% | 100% | 100% | 100% |
Object Size | Angle | 0° | 45° | 90° | 135° | Total |
50 | Number of images | 646 | 608 | 627 | 619 | 2500 |
Predicted number | 646 | 608 | 627 | 619 | 2500 | |
Accuracy rate | 100% | 100% | 100% | 100% | 100% | |
100 | Number of images | 641 | 589 | 644 | 626 | 2500 |
Predicted number | 641 | 589 | 644 | 626 | 2500 | |
Accuracy rate | 100% | 100% | 100% | 100% | 100% | |
500 | Number of images | 634 | 646 | 585 | 635 | 2500 |
Predicted number | 634 | 646 | 585 | 635 | 2500 | |
Accuracy rate | 100% | 100% | 100% | 100% | 100% | |
1000 | Number of images | 600 | 623 | 643 | 634 | 2500 |
Predicted number | 600 | 623 | 643 | 634 | 2500 | |
Accuracy rate | 100% | 100% | 100% | 100% | 100% |
Object Size | Angle | 0° | 45° | 90° | 135° | Total |
50 | Number of images | 580 | 647 | 653 | 620 | 2500 |
Predicted number | 580 | 647 | 653 | 620 | 2500 | |
Accuracy rate | 100% | 100% | 100% | 100% | 100% | |
100 | Number of images | 611 | 635 | 648 | 606 | 2500 |
Predicted number | 611 | 635 | 648 | 606 | 2500 | |
Accuracy rate | 100% | 100% | 100% | 100% | 100% | |
500 | Number of images | 602 | 602 | 670 | 626 | 2500 |
Predicted numbers | 602 | 602 | 670 | 626 | 2500 | |
Accuracy rate | 100% | 100% | 100% | 100% | 100% | |
1000 | Number of images | 642 | 616 | 591 | 651 | 2500 |
Predicted number | 642 | 616 | 591 | 651 | 2500 | |
Accuracy rate | 100% | 100% | 100% | 100% | 100% |
Noise | PODM | CNN | ||||
Mean(%) | ± | SD | Mean(%) | ± | SD | |
1% | 99.99 | ± | 0.0149 | 67.83 | ± | 0.1596 |
2% | 99.40 | ± | 0.1228 | 52.71 | ± | 0.1674 |
3% | 97.16 | ± | 0.3379 | 45.52 | ± | 0.1447 |
4% | 92.95 | ± | 0.4359 | 41.59 | ± | 0.1298 |
5% | 87.69 | ± | 0.7140 | 41.61 | ± | 0.1813 |
6% | 81.84 | ± | 0.5256 | 35.97 | ± | 0.1021 |
7% | 76.59 | ± | 0.7738 | 33.96 | ± | 0.1017 |
8% | 70.99 | ± | 0.7243 | 31.58 | ± | 0.1052 |
9% | 66.31 | ± | 0.8904 | 30.90 | ± | 0.0748 |
10% | 62.28 | ± | 0.7972 | 32.30 | ± | 0.0889 |
P-value | - | 8.64E-07 |
Noise | PODM | CNN | ||||
Mean(%) | ± | SD | Mean(%) | ± | SD | |
1% | 99.98 | ± | 0.0267 | 93.03 | ± | 0.0236 |
2% | 99.54 | ± | 0.1267 | 90.87 | ± | 0.0399 |
3% | 98.60 | ± | 0.2016 | 85.30 | ± | 0.1019 |
4% | 96.99 | ± | 0.2789 | 84.34 | ± | 0.1290 |
5% | 95.34 | ± | 0.3437 | 79.01 | ± | 0.1528 |
6% | 93.11 | ± | 0.4067 | 77.87 | ± | 0.1796 |
7% | 90.43 | ± | 0.4366 | 78.39 | ± | 0.1530 |
8% | 87.91 | ± | 0.6577 | 76.80 | ± | 0.1522 |
9% | 84.91 | ± | 0.5372 | 72.51 | ± | 0.1787 |
10% | 82.16 | ± | 0.7730 | 75.30 | ± | 0.1598 |
P-value | - | 9.53E-04 |
Noise | PODM | CNN | ||||
Mean(%) | ± | SD | Mean(%) | ± | SD | |
1% | 100.00 | ± | 0.0100 | 97.58 | ± | 0.0067 |
2% | 99.94 | ± | 0.0503 | 97.17 | ± | 0.0068 |
3% | 99.69 | ± | 0.1204 | 96.93 | ± | 0.0072 |
4% | 99.34 | ± | 0.1709 | 95.96 | ± | 0.0121 |
5% | 98.91 | ± | 0.1891 | 96.08 | ± | 0.0092 |
6% | 98.42 | ± | 0.2377 | 95.80 | ± | 0.0078 |
7% | 97.81 | ± | 0.3068 | 95.25 | ± | 0.0084 |
8% | 97.19 | ± | 0.3345 | 95.26 | ± | 0.0063 |
9% | 96.52 | ± | 0.2720 | 94.83 | ± | 0.0100 |
10% | 95.74 | ± | 0.3695 | 94.67 | ± | 0.0077 |
P-value | - | 5.13E-04 |
Noise | PODM | CNN | ||||
Mean(%) | ± | SD | Mean(%) | ± | SD | |
1% | 99.99 | ± | 0.0072 | 96.71 | ± | 0.0065 |
2% | 99.81 | ± | 2.8279 | 96.49 | ± | 0.0055 |
3% | 99.43 | ± | 0.7075 | 96.29 | ± | 0.0070 |
4% | 98.85 | ± | 0.1964 | 96.13 | ± | 0.0064 |
5% | 98.16 | ± | 0.1602 | 95.85 | ± | 0.0101 |
6% | 97.41 | ± | 0.2816 | 95.66 | ± | 0.0058 |
7% | 96.71 | ± | 0.3358 | 95.90 | ± | 0.0075 |
8% | 95.70 | ± | 0.3497 | 95.43 | ± | 0.0115 |
9% | 94.91 | ± | 0.3863 | 95.93 | ± | 0.0087 |
10% | 93.77 | ± | 0.3876 | 95.58 | ± | 0.0096 |
P-value | - | 4.76E-02 |
Receptive field | 2*3 | 3*3 | ||||
Noise | Mean(%) | ± | SD | Mean(%) | ± | SD |
1% | 99.98 | ± | 0.0246 | 100.00 | ± | 0.0147 |
2% | 99.17 | ± | 0.1496 | 99.36 | ± | 0.1210 |
3% | 96.42 | ± | 0.3577 | 96.96 | ± | 0.3363 |
4% | 92.11 | ± | 0.6329 | 92.80 | ± | 0.4348 |
5% | 86.64 | ± | 0.5642 | 88.00 | ± | 0.7038 |
6% | 80.74 | ± | 0.9089 | 81.68 | ± | 0.5230 |
7% | 75.04 | ± | 0.8508 | 77.48 | ± | 0.7652 |
8% | 70.24 | ± | 0.8117 | 71.56 | ± | 0.7256 |
9% | 65.31 | ± | 0.8048 | 66.24 | ± | 0.8806 |
10% | 61.68 | ± | 0.9211 | 62.28 | ± | 0.7914 |
P-value | - | 8.84E-01 | ||||
Time cost | 2193.02 s | 3378.61 s |
Receptive field | 2*3 | 3*3 | ||||
Noise | Mean(%) | ± | SD | Mean(%) | ± | SD |
1% | 99.95 | ± | 0.0377 | 99.96 | ± | 0.0266 |
2% | 99.39 | ± | 0.1232 | 99.72 | ± | 0.1251 |
3% | 98.23 | ± | 0.2248 | 98.68 | ± | 0.1983 |
4% | 96.71 | ± | 0.3103 | 97.00 | ± | 0.2752 |
5% | 94.97 | ± | 0.3515 | 95.08 | ± | 0.3446 |
6% | 92.70 | ± | 0.4397 | 92.88 | ± | 0.4001 |
7% | 90.19 | ± | 0.4157 | 90.44 | ± | 0.4311 |
8% | 87.31 | ± | 0.6059 | 87.44 | ± | 0.6692 |
9% | 84.57 | ± | 0.5317 | 84.36 | ± | 0.5287 |
10% | 81.49 | ± | 0.7801 | 82.12 | ± | 0.7805 |
P-value | - | 9.40E-01 | ||||
Time cost | 2195.05 s | 3312.37 s |
Receptive field | 2*3 | 3*3 | ||||
Noise | Mean(%) | ± | SD | Mean(%) | ± | SD |
1% | 99.99 | ± | 0.0149 | 100.00 | ± | 0.0098 |
2% | 99.91 | ± | 0.0572 | 99.84 | ± | 0.0507 |
3% | 99.65 | ± | 0.1274 | 99.72 | ± | 0.1222 |
4% | 99.27 | ± | 0.1609 | 99.40 | ± | 0.1699 |
5% | 98.78 | ± | 0.2088 | 98.84 | ± | 0.1891 |
6% | 98.32 | ± | 0.2011 | 98.20 | ± | 0.2344 |
7% | 97.63 | ± | 0.1843 | 97.64 | ± | 0.3094 |
8% | 97.04 | ± | 0.2397 | 97.56 | ± | 0.3291 |
9% | 96.41 | ± | 0.3563 | 96.56 | ± | 0.2679 |
10% | 95.69 | ± | 0.3067 | 95.72 | ± | 0.3724 |
P-value | - | 9.06E-01 | ||||
Time cost | 2214.19 s | 3379.97 s |
Receptive field | 2*3 | 3*3 | ||||
Noise | Mean(%) | ± | SD | Mean(%) | ± | SD |
1% | 99.99 | ± | 0.0181 | 100.00 | ± | 0.0071 |
2% | 99.81 | ± | 0.0696 | 99.84 | ± | 2.7832 |
3% | 99.43 | ± | 0.1429 | 99.56 | ± | 0.6968 |
4% | 98.85 | ± | 0.1799 | 99.00 | ± | 0.1933 |
5% | 98.16 | ± | 0.2300 | 98.24 | ± | 0.1582 |
6% | 97.41 | ± | 0.3432 | 97.32 | ± | 0.2833 |
7% | 96.71 | ± | 0.3401 | 96.36 | ± | 0.3413 |
8% | 95.70 | ± | 0.3142 | 95.60 | ± | 0.3461 |
9% | 94.91 | ± | 0.3150 | 95.08 | ± | 0.3803 |
10% | 93.77 | ± | 0.3777 | 94.00 | ± | 0.3815 |
P-value | - | 9.84E-01 | ||||
Time cost | 2335.16 s | 3583.72 s |
Angle | 0° | 45° | 90° | 135° | Total |
Original images | 16 | 10 | 13 | 11 | 50 |
Predicted number | 16 | 10 | 13 | 11 | 50 |
Accuracy rate | 100% | 100% | 100% | 100% | 100% |
Downsampled images | 16 | 10 | 13 | 11 | 50 |
Predicted number | 16 | 10 | 13 | 11 | 50 |
Accuracy rate | 100% | 100% | 100% | 100% | 100% |