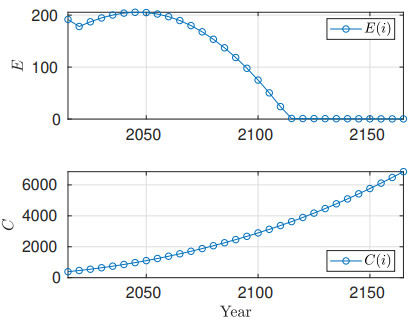
Citation: Philipp Braun, Timm Faulwasser, Lars Grüne, Christopher M. Kellett, Willi Semmler, Steven R. Weller. On the social cost of carbon and discounting in the DICE model[J]. AIMS Environmental Science, 2024, 11(3): 471-495. doi: 10.3934/environsci.2024024
[1] | Sergey Orlov, Elena Rovenskaya, Julia Puaschunder, Willi Semmler . Green bonds, transition to a low-carbon economy, and intertemporal welfare allocation: Evidence from an extended DICE model. AIMS Environmental Science, 2024, 11(4): 628-648. doi: 10.3934/environsci.2024031 |
[2] | Subhash Kumar, Ashok Kumar, Rekha Guchhait, Biswajit Sarkar . An environmental decision support system for manufacturer-retailer within a closed-loop supply chain management using remanufacturing. AIMS Environmental Science, 2023, 10(5): 644-676. doi: 10.3934/environsci.2023036 |
[3] | Serpil Guran . Options to feed plastic waste back into the manufacturing industry to achieve a circular carbon economy. AIMS Environmental Science, 2019, 6(5): 341-355. doi: 10.3934/environsci.2019.5.341 |
[4] | Izlawanie Muhammad . Carbon tax as the most appropriate carbon pricing mechanism for developing countries and strategies to design an effective policy. AIMS Environmental Science, 2022, 9(2): 161-184. doi: 10.3934/environsci.20220012 |
[5] | Benjamin M. Sleeter, Jinxun Liu, Colin Daniel, Leonardo Frid, Zhiliang Zhu . An integrated approach to modeling changes in land use, land cover, and disturbance and their impact on ecosystem carbon dynamics: a case study in the Sierra Nevada Mountains of California. AIMS Environmental Science, 2015, 2(3): 577-606. doi: 10.3934/environsci.2015.3.577 |
[6] | Afshin Meraj, Tina Shoa, Fereshteh Sadeghi Naieni Fard, Hassan Mina . A comprehensive bi-objective optimization model to design circular supply chain networks for sustainable electric vehicle batteries. AIMS Environmental Science, 2024, 11(2): 279-303. doi: 10.3934/environsci.2024013 |
[7] | Raj Kumar Bachar, Shaktipada Bhuniya, Santanu Kumar Ghosh, Biswajit Sarkar . Sustainable green production model considering variable demand, partial outsourcing, and rework. AIMS Environmental Science, 2022, 9(3): 325-353. doi: 10.3934/environsci.2022022 |
[8] | Soumya Kanti Hota, Santanu Kumar Ghosh, Biswajit Sarkar . Involvement of smart technologies in an advanced supply chain management to solve unreliability under distribution robust approach. AIMS Environmental Science, 2022, 9(4): 461-492. doi: 10.3934/environsci.2022028 |
[9] | Manfred Kircher . The bioeconomy needs economic, ecological and social sustainability. AIMS Environmental Science, 2022, 9(1): 33-50. doi: 10.3934/environsci.2022003 |
[10] | Kumari Anjali, YSC Khuman, Jaswant Sokhi . A Review of the interrelations of terrestrial carbon sequestration and urban forests. AIMS Environmental Science, 2020, 7(6): 464-485. doi: 10.3934/environsci.2020030 |
To quantify the damages from anthropogenic emissions of heat-trapping greenhouse gases, economists model the dynamics of climate–socio-economic interactions using integrated assessment models (IAMs) that incorporate mathematical models of phenomena from geophysical science, economics, and social sciences. A central role for IAMs is to estimate the social cost of carbon (SCC), defined as the dollar value of the economic damage caused by a one metric ton increase in carbon dioxide (CO2) emissions to the atmosphere. The SCC can then form the basis of a Pigouvian tax on greenhouse gas emissions, guide carbon prices in emissions trading schemes, inform cost-benefit analyses driving climate policy, or be used by investors looking to consider potential emissions costs in investment decisions. This wide range of uses highlights the importance of methods for estimating the SCC.
A critical and much debated issue in computing the SCC is the choice of discount rate. CO2 is a long-lived greenhouse gas (GHG), with CO2 emissions having a substantial impact on climate for thousands of years; e.g., 20–40% of a CO2 perturbation remains in the atmosphere even after equilibration with the ocean on a timescale of 2–20 centuries [1,2]. Comparing costs and benefits over such long time horizons necessitates a careful choice of discount rates and methodologies in order to provide a fair comparison of costs paid now—via emissions reductions—with economic benefits from curbed global warming in the far distant future.
Conceptually, the most common approach to computing the SCC using IAMs is to compute baseline emissions and economic consumption. These computations are then repeated with an increment of CO2 emissions in a chosen year, yielding reduced economic consumption into the future as the result of a climate damages function in the IAM. The results of these baseline and perturbed scenarios are then used to compute the SCC as the net present value (NPV) of the "damage stream" (i.e., pathway of lost consumption) (see, for example, [3,4]). This NPV approach is employed directly by two of the most widely used IAMs: PAGE (Policy Analysis of the Greenhouse Effect [5]) and FUND (Climate Framework for Uncertainty, Negotiation, and Distribution [6]). While descriptively this is what is intended with DICE (Dynamic Integrated model of Climate and Economy; see Figure 1 and associated discussion by Nordhaus W [4]), in practice the publicly available implementation of DICE uses a different method, based on shadow prices or Lagrange multipliers obtained as a result of solving an optimal control problem ([7]). Hence, a critical question, answered herein, is whether or not these different computational approaches are in fact equivalent.
The classical Ramsey formula for social discount rate is given by r=ρ+θg, where ρ is the pure rate of time preference (or "impatience factor"), θ is the elasticity of marginal utility of consumption, and g is the growth rate of per-capita consumption [8]. While the calculation of the SCC as the NPV of the damage stream due to CO2 pulse implies use of a discount rate, there is widespread disagreement in the literature regarding the appropriate discount rate to use in this context. For example, in some representative papers, the SCC is described as being computed as the NPV of a damage stream variously discounted at (a) the classical Ramsey formula (i.e., assuming a constant growth rate of consumption), (b) a time-varying Ramsey formula (reflecting the growth rate of time-varying consumption), (c) the pure rate of time preference, and (d) a fixed rate without any explicit reference to the Ramsey formula; see the studies by Marten AL, et al. [3,4,5,6,9,10,11,12,13,14,15,16], and also the study by Tol RSJ[17], which proposes exercises based on both (b) and (d). The report Economides 2018 provides a chapter on various discounting approaches in the economics of climate change. It is worth noting that in some cases it is unclear precisely how the discounting is being performed as it is described in words rather than by providing an explicit mathematical expression.
As briefly mentioned above, rather than computing the NPV of the damages stream, a conceptually distinct approach to computing the SCC relies on maximizing a social welfare function. As explicitly defined in the study of Newbold S C, et al. [19], "The [SCC] in a particular year is the decrease in aggregate consumption in that year that would change the current expected value of social welfare by the same amount as a one unit increase in carbon emissions in that year." Expressed formally, the SCC at time t is defined as
SCC(t)=−∂W∂E(t)∂WC(t) | (1.1) |
where a simple manipulation shows that this is the ratio of the marginal prices of consumption and emissions. In fact, this is the computation contained in the code implementing the DICE model and optimal control problem as presented in the study by D. Nordhaus W, et al. [7]. Here, W denotes social welfare, E denotes emissions, and C denotes consumption, whose definitions are made precise in Section 2.
The main contribution of this paper is to discuss and compare the three possible computational methods of the SCC indicated in the discussion above. In particular, we discuss a direct discretization of (1.1), an approach using the ratio of marginals obtained from solving an optimal control problem, and an NPV approach based on experimental CO2 emission pulses. For the last approach, there are several options for how to perform the required discounting. The three approaches are compared theoretically, in terms of their accuracy and their computational complexity, and numerically, based on simulations of the DICE model. In addition, the three approaches and their analysis lead us to the following question: Which (if any) of the above options for computing the SCC are consistent with each other? Importantly, neither FUND nor PAGE involve an optimal control problem, meaning the first two methods mentioned here are not relevant. Hence, answering this consistency question is critical for comparing results between IAMs based on optimal control methodologies with those based on scenario methodologies.
Our main mathematical result, which covers the third computation method, is summarized in Theorem 1. The result demonstrates that in order to obtain consistency between the NPV calculation and the SCC values calculated via optimal control approaches, it is necessary to use a time-varying discount rate in the NPV calculation. In particular, the time-varying component is inversely proportional to economic growth, leading to a declining discount rate [20,21,22]. Specifically, returning to the Ramsey formula r=ρ+θg, mentioned above, the quantity g is time-varying and given by the growth rate of consumption. The quantities ρ and θ are "exogenous" to the model and need to be selected by the modeler on the basis of data and/or economic expertise. Note that it has been argued that the use of declining rates is justified in the presence of significant uncertainty in future economic growth (see [23]). Here, we see that the use of a declining discount rate is required for consistency with outputs from calculations based on shadow prices obtained when solving optimal control problems.
This paper is organized as follows. In Section 2 we succinctly present the DICE model as described in the study by Kellett C M, et al. [24], with model parameters provided in Appendix E. In Section 3, we investigate three methods for computation of the SCC using the DICE model. Section 3.1 presents the calculation based on a discretization of the difference quotient of the two quantities in (1.1). In Section 3.2 we demonstrate how to rewrite the DICE optimal control problem so that emissions and consumption are decision variables, which allows us to obtain the marginals or dual variables directly as an output of solving the optimal control problem. This has the computational benefit of removing the welfare function as an intermediary in the SCC calculation. In Section 3.3 we present the main mathematical result of the paper (Theorem 1), which shows an equivalence between calculating the SCC as a ratio of the dual variables versus the NPV when the discounting approach is computed via the time-varying Ramsey formula accounting for a time-varying growth rate of consumption. Section 4 presents some numerical results and discussion, and we conclude in Section 5. Mathematical necessities and the proof of our main result are collected in Appendices.
Notation: The set N0={0,1,2,…} denotes the natural numbers including zero, while N={1,2,…} denotes the natural numbers without zero. The real numbers are denoted as R, and R≥0={x∈R| x≥0} denotes the real numbers bigger or equal to zero. For n∈N, the set Rn=R×⋯×R denotes the n-fold Cartesian product of the real numbers, and x∈Rn denotes an n-dimensional vector with x(i)∈R, i∈{1,…,n}. To simplify expressions and to define convergence rates we use the big O-notation, i.e., two functions f1,f2:R→R, f2(x)>0 for all x>0, satisfy f1(x)=O(f2(x)) if and only if there are C>0 and δ>0 such that |f1(x)|≤Cf2(x) for all 0<x<δ. For a continuously differentiable function f:Rn×Rm→R, (x,y)↦f(x,y), the gradient is denoted by ∇f(x,y). The gradient and the second derivative of f with respect to a subset of variables, that is with respect to x, for example, are denoted by ∇xf(x,y) and ∇2xxf(x,y), respectively.
For the computation of the social cost of carbon, we consider the Dynamic Integrated model of Climate and Economy (DICE). For a comprehensive introduction of the model and its parameters we refer to the studies [4,24]. Here, we only give a brief introduction of the dynamic equations and their interpretation. The presentation follows the notation introduced in [24]. We focus on the parameters defining the R-DICE2016 model, but the results derived in this paper also hold for the parameters defining the DICE-2013R model. Following the R-DICE2016 setting we denote time in years by t∈R≥0 so that
t=t0+Δ⋅i, | (2.1) |
with initial time t0=2015, a sample period Δ=5, and i∈N0. The DICE model is described through the dynamics
M(i+1)=ΦMM(i)+BM(σ(i)(1−μ(i))A(i)K(i)γL(i)1−γ+ELand(i)+EPulse(i)), | (CAR) |
T(i+1)=ΦTT(i)+BT(F2×log2(MAT(i)MAT,1750)+FEX(i)), | (CLI) |
K(i+1)=ΦKK(i)+Δ(11+a2TAT(i)a3)(1−θ1(i)μ(i)θ2)A(i)K(i)γL(i)1−γs(i), | (CAP) |
L(i+1)=L(i)(1+La1+L(i))ℓg, | (POP) |
A(i+1)=A(i)1−gAexp(−δAΔ(i−1)), | (TFP) |
σ(i+1)=σ(i)exp(−gσ(1−δσ)Δ(i−1)Δ), | (EI) |
modeling the carbon cycle (CAR), the climate (CLI), the capital (CAP), the population (POP), the total factor productivity (TFP), and the emissions intensity (EI). In (CAR), M=[MAT,MUP,MLO]⊤ is a three dimensional vector describing the average mass of carbon in the atmosphere, in the upper ocean, and in the deep or lower ocean. The climate dynamics (CLI) capture the evolution of the temperature T=[TAT,TLO]⊤ in the atmosphere (TAT) and in the lower ocean (TLO). The one dimensional state K represents the economic output in the economic dynamics (CAP). For the world-wide population L, the total factor productivity A, and the emissions intensity σ, the DICE model uses the Hassell model [25], a logistic-function model, and a monotonically decreasing function, respectively, to capture the dynamics.
The remaining time dependent quantities are described through the equations
θ1(i)=pb1000⋅θ2(1−δpb)i−1⋅σ(i), | (2.2) |
FEX(i)=f0+min{f1−f0,f1−f0tf(i−1)}, | (2.3) |
ELand(i)=EL0⋅(1−δEL)i−1, | (2.4) |
and denoted as the mitigation effort θ1, the effect of greenhouse gases FEX, and the emissions due to land use ELand.
Control inputs are the savings rate, s(i)∈[0,1] for all i∈N0, and the mitigation rate, μ(i)∈[0,1] for all i∈N0. An additional parameter, EPulse(i)∈R, for all i∈N0, is used for the calculation of the partial derivatives needed contained in the definition of the SCC, cf. equation (3.2), below. The additional parameter EPulse(i) has an impact on the total emissions
E(i)=σ(i)(1−μ(i))A(i)K(i)γL(i)1−γ+ELand(i)+EPulse(i) | (2.5) |
at time i∈N, which we can identify in (CAR). The parameter is not present in the baseline DICE model where EPulse(i)=0 for all i∈N0. We collect the states in the variable X, i.e.,
X(i)={T(i),M(i),K(i),L(i),A(i),σ(i)} |
and use X(0)=X0 to denote a fixed initial condition.
The control inputs are used to maximize the social welfare depending on the consumption and the utility at time i∈N0 as
C(i)=(11+aTAT(i)2)(1−θ1(i)μ(i)θ2)A(i)K(i)γL(i)1−γ(1−s(i))+CPulse(i) | (2.6) |
U(i)=L(i)((1000C(i)L(i))1−α−11−α). | (2.7) |
Here, CPulse(i)∈R, for all i∈N0, plays the same role as EPulse(i) in Equation (CAR), manipulating the consumption in a specific year. Then, for a fixed horizon, N∈N, the social welfare is defined as
W:=N−1∑i=0U(i)(1+ρ)Δi | (2.8) |
and the optimal control problem (2.9) which maximizes the social welfare and generates optimal pathways is defined as
W∗=maxs,μN−1∑i=0U(i)(1+ρ)ΔisubjecttoX(0)−X0=0(CAR)−(EI),∀ i=0,…,N−1,(2.2)−(2.4),∀ i=0,…,N−1,s(i),μ(i)∈[0,1]∀ i=0,…,N−1. | (2.9) |
The optimal control problem (2.9) constitutes a nonlinear program (NLP). We use the notation W∗ to denote the optimal value with respect to CPulse(j)=EPulse(j)=0, for all j∈{0,…,N−1}, i.e., W∗ denotes the nominal or baseline solution of (2.9). In the case that CPulse(j)≠0 or EPulse(j)≠0 for j∈{0,…,N−1} is considered in (2.9), the optimal solution is denoted by W∗[CPulse(j)] or W∗[EPulse(j)], respectively. Similarly, optimal pathways are denoted using ⋅∗. In this context, C∗(⋅), C∗[CPulse(j)](⋅), and C∗[EPulse(j)](⋅) denote the optimal consumption pathways obtained through (2.9) with CPulse(j)=EPulse(j)=0, CPulse(j)≠0, and EPulse(j)≠0, respectively (in all cases we set CPulse(i)=EPulse(i)=0 for all i≠j). Additionally, N∈N denotes a fixed horizon and s,μ∈RN define a short-hand notation for
s=[s(0)⋮s(N−1)]andμ=[μ(0)⋮μ(N−1)]. | (2.10) |
For completeness, the parameters used in R-DICE2016, and in the simulations presented in this paper, are reported in Appendix E.
In this section we take as a formal definition of the SCC at time j∈{0,…,N−1}, a scaled version of the change of the optimal consumption C∗(j) with respect to a change of the optimal emissions E∗(j). Accordingly, the SCC in US dollars per kilogram of emitted CO2 is defined as the change −∂C∗(j)∂E∗(j). However, instead of kilograms of emitted CO2, it is common to express the SCC in terms of tonnes of CO2, i.e., we define
SCC(j)=−1000∂C∗(j)∂E∗(j)[$tCO2], | (3.1) |
(see [24, Sec. 2.8]). Since E∗(j) is itself an outcome of the optimal control problem (2.9) rather than an independent variable on which C∗(j) would depend, it is not immediately clear how this partial derivative has to be interpreted. Its meaning is as follows: We consider EPulse(j)∈R∖{0} and EPulse(i):=0 for all i≠j. Then, we solve the optimal control problem (2.9) in order to obtain C∗[EPulse(j)](⋅). The partial derivative in (3.1) is then defined as
∂C∗(j)∂E∗(j):=limEPulse(j)→0EPulse(j)≠0C∗[EPulse(j)](j)−C∗(j)EPulse(j). | (3.2) |
In the same way, partial derivatives with respect to other quantities occurring in the optimization can be defined.
Under appropriate differentiability assumptions on E∗, C∗ and the optimal value function W∗, Equation (3.1) can be rewritten as
SCC(j)=−1000∂C∗(j)∂W∗⋅∂W∗∂E∗(j)=−1000∂W∗/∂E∗(j)∂W∗/∂C∗(j) | (3.3) |
used as the common definition in [4, Sec. 2.1], for example. Here, "[t]he numerator is the marginal impact of emissions at time j on welfare, while the denominator is the marginal welfare value of a unit of aggregate consumption in period j" [4, Section 2.1].
Since the optimal value function W∗ and the optimal total emissions E∗(j) and optimal consumptions C∗(j) are only implicitly known as the optimal solutions of the optimal control problems (2.9), a direct calculation of the social cost of carbon through (3.3) is not possible. Nevertheless, there are several efficient ways to approximate (3.3).
Using (3.2), we can approximate the quantity in (3.3) at a fixed time j∈{0,…,N−1} by performing three experiments to compute approximations
∂W∗∂E∗(j)≈W∗[EPulse(j)]−W∗EPulse(j)and∂W∗∂C∗(j)≈W∗[CPulse(j)]−W∗CPulse(j). | (3.4) |
To this end three optimal control problems can be solved.
1. A baseline solution of (2.9) that generates a welfare value W∗ corresponding to EPulse(j)=0 and CPulse(j)=0 for all j=0,…,N−1.
2. A second solution of (2.9) with a small non-zero perturbation on emissions at time j∈{0,…,N−1}; i.e., EPulse(j)≠0. This yields a second (optimal) welfare value W∗[EPulse(j)].
3. A third solution of (2.9) with a small non-zero perturbation on consumption at time j∈{0,…,N−1}, i.e., CPulse(j)≠0, that yields a third (optimal) welfare value W∗[CPulse(j)].
Then, the SCC in (3.3) can be approximated by
SCC(j)≈−1000CPulse(j)EPulse(j)⋅W∗[EPulse(j)]−W∗W∗[CPulse(j)]−W∗. | (3.5) |
Since (3.4) is a first order approximation of the derivative of W∗ with respect to E∗(j) and C∗(j), respectively, the right-hand side of (3.5) converges with order O(CPulse(j)+EPulse(j)) to (3.3) for CPulse(j)→0 and EPulse(j)→0 and under the assumption that the optimal control problem (2.9) can be solved with arbitrary precision. To calculate SCC(j) for all j∈{0,…,N−1} using the approximation (3.5), 2N+1 optimal control problems need to be solved.
Remark 1. The order of convergence follows from Taylor expansions of W∗ under the assumption that W∗ is twice continuously differentiable with respect to E and C. In particular, the derivation of the error term O(CPulse(j)+EPulse(j)) relies on
W∗[EPulse(j)]=W∗+∂W∗∂E∗(j)EPulse(j)+O(EPulse(j)2)W∗[CPulse(j)]=W∗+∂W∗∂C∗(j)CPulse(j)+O(CPulse(j)2) | (3.6) |
and is given in detail in Appendix D.
Most modern NLP solvers, including IPOPT [26] and CONOPT [27], for example, return the optimal Lagrange multipliers, i.e., the dual variables of all explicitly enforced equality and inequality constraints in addition to the optimal solution W∗, s∗, μ∗, are calculated. This can be used to compute the SCC directly via dual variables. All that is necessary to do is formulating (2.9) in a way such that C(i) and E(i) are decision variables of the NLP. Using (2.5) allows a reformulation of (CAR) in terms of
M(i+1)=ΦMM(i)+BME(i). | (3.7) |
Instead of (2.9), we can thus consider the modified optimal control problem
W∗=maxs,μ,C,EN−1∑i=0U(i)(1+ρ)ΔisubjecttoX(0)−X0=0(3.7),(CLI)−(EI),∀ i=0,…,N−1,(2.2) – (2.4),∀ i=0,…,N−1,(2.5) – (2.6),∀ i=0,…,N−1,s(i),μ(i)∈[0,1]∀ i=0,…,N−1, | (3.8) |
where
C=[C(0)⋮C(N−1)]andE=[E(0)⋮E(N−1)] |
are defined similar to (2.10). The optimal control problems (2.9) and (3.8) are identical in the sense that the optimal solutions W∗, s∗, and μ∗ coincide. However, in (3.8) we avoid the substitution of (2.6) and (2.5).
The main difference between (2.9) and (3.8) is that in the latter case, consumption and emissions are treated as pseudo decision variables defined via explicit equality constraints, while in the former case one substitutes C(i) and E(i) in the objective and the dynamics by the expressions given in (2.6) and (2.5). The implementation of (3.8) in an NLP solver such as IPOPT yields values for the optimal Lagrange multipliers of all constraints.
To make this more precise, note that we can rewrite (3.8) in the form of the parameter dependent optimization problem
f∗(p)=maxxf(x)subjecttohEi(x)=pEi,∀ i=0,…,N−1,hCi(x)=pCi,∀ i=0,…,N−1,k(x)=0,g(x)≥0, | (3.9) |
in the optimization variables x={s,μ,C,E}. Here,
f(x)=N−1∑i=0U(i)(1+ρ)Δi |
denotes the objective function in the variables s,μ,C,E, and
hEi(x)=E(i)−(σ(i)(1−μ(i))A(i)K(i)γL(i)1−γ+ELand(i)),hCi(x)=C(i)−((11+aTAT(i)2)(1−θ1(i)μ(i)θ2)A(i)K(i)γL(i)1−γ(1−s(i))) |
capture (2.5) and (2.6) for i=0,…,N−1. The pulses EPulse and CPulse are included through the parameter p=[pTE pTC]T,
pE=[pE0⋮pEN−1]andpC=[pC0⋮pCN−1], |
in the right-hand side, and the optimal value f∗(⋅) depends on the selection of p. The equality and inequality constraints x↦k(x) and x↦g(x) cover the remaining constraints of (3.8), i.e., X(0)=X0, (3.7), (CLI)–(EI), (2.2)–(2.4), s(i),μ(i)∈[0,1] for i∈{0,…,N−1} are encoded through appropriate equality and inequality constraints in the decision variable x.
The Lagrangian corresponding to (3.9) with Lagrange multipliers (λE,λC,δ,κ) is defined as
L(x,λE,λC,δ,κ;p)=f(x)−(N−1∑j=0λEi(pEi−hEi(x))+λCi(pCi−hCi(x)))−δTk(x)−κTg(x). | (3.10) |
If x∗ and (λ∗E,λ∗C,δ∗,κ∗) are an optimal solution-Lagrange multiplier pair (see [28, Sec. 5.1.3], for example), then the optimal value function satisfies
f∗(p)=L(x∗(p),λ∗E(p),λ∗C(p),δ∗(p),κ∗(p);p)=f(x∗(p)). | (3.11) |
Moreover, under appropriate conditions (second order sufficiency conditions [28, Prop. 3.3.2], for example), the partial derivatives of the optimal value function in a neighborhood around p=0 satisfy
∂f∗∂pEi(p)=∂L∂pEi(x∗(p),λ∗E(p),λ∗C(p),δ∗(p),κ∗(p);p)=−λ∗Ei(p),∂f∗∂pCi(p)=∂L∂pCi(x∗(p),λ∗E(p),λ∗C(p),δ∗(p),κ∗(p);p)=−λ∗Ci(p), | (3.12) |
for all i∈{0,…,N−1}, according to [28].
While in the optimal value function, necessarily, the terms containing Lagrange multipliers vanish, and only the objective function is important according to (3.11), in ∇pf∗(p) in (3.12), it turns out that the dependency of (x∗,λ∗E,λ∗C,δ∗,κ∗) on p can be ignored when computing the gradient through the Lagrangian (3.10). Thus, the Lagrange multipliers λE, λC are a measure of the sensitivity of the objective function with respect to changes in the right-hand side of the equality constraints. In economics, this sensitivity, i.e., the change in the objective function under a marginal change of a constraint, is also referred to as the shadow price (see [29,30], for example) and it provides an interpretation of how valuable a specific commodity is.
These observations finally allow us to return to (3.3). If we recall the connection between (3.8) and (3.9) we observe that
λ∗Ej=−∂W∗∂E∗(j),andλ∗Cj=−∂W∗∂C∗(j) | (3.13) |
is satisfied for all j∈{0,…,N−1}. Thus, according to (3.3), the SCC is given by
SCC(j)=−1000λ∗Ejλ∗Cj. | (3.14) |
Using (3.14), SCC(j) can be computed for all j∈{0,…,N−1} by solving only a single optimization problem (3.8). In addition, unlike (3.5), Equation (3.14) is not an approximation of (3.3) but an exact expression. However, the pulse experiments might be more intuitive and from an interpretation point of view easier to understand than the connection to the Lagrange multipliers.
In this section we present a third way to compute the SCC. This is based on the net present value, which, as described in the introduction, has been used in much of the climate economics research but not in a consistent manner. Here, we will show that a time-varying Ramsey discount factor, accounting for a time-varying growth rate of consumption, is required for consistency between calculating the SCC as a discounted damages stream and calculating the SCC as a ratio of marginal prices. The result relies on the assumption that (2.9) satisfies second order sufficiency conditions. The precise definition and a corresponding result are reported in Theorem 2 in Appendix A.
Theorem 1. Consider the optimal control problem (2.9) and assume that (2.9) satisfies second order sufficiency conditions. Then, the SCC at time j∈{0,…,N−1} is given by
SCC(j)=−1000N−1∑i=0∂C∗(i)∂E∗(j)δj,i, | (3.15) |
where the Ramsey discount factor between periods j and i∈{0,…,N−1} is defined as
δj,i:=1(1+ρ)Δ(i−j)⋅1(1+gj,i))α, | (3.16) |
and the growth rate of per capita consumption up to period i is defined as
gj,i:=C∗(i)/L(i)C∗(j)/L(j)−1. | (3.17) |
A proof of Theorem 1 is given in Appendix C. Based on Theorem 1, the SCC can again be computed using a finite difference approximation. While δj,i in (3.16) can be computed explicitly, the partial derivative ∂C∗(i)∂E∗(j) in (3.15) needs to be approximated. We can thus perform two experiments to approximate the SCC at time j∈{0,…,N−1} using Equation (3.15):
1. A baseline solution of (2.9) that generates a welfare value W∗ corresponding to EPulse(i)=0 and CPulse(i)=0 for all i=0,…,N−1 and additionally returns the nominal consumption pathway C∗(i) for i=0,…,N−1.
2. A second solution of (2.9) with a small non-zero perturbation on emissions at time j∈{0,…,N−1}, i.e., EPulse(j)≠0. This yields a second (optimal) welfare value W∗[EPulse(j)] and additionally a second (optimal) consumption pathway C∗[EPulse(j)](i) for i=0,…,N−1.
With the resulting pathways, we can calculate the discount rate δj,i and can approximate the partial derivatives, similar to (3.4), by (C∗[EPulse(j)](i)−C∗(i))/(EPulse(j)). The social cost of carbon can then be approximated by
SCC(j)≈−1000EPulse(j)N−1∑i=0(C∗[EPulse(j)](i)−C∗(i))δj,i. | (3.18) |
Remark 2. Using the Taylor expansion
C∗[EPulse(j)](i)=C∗(i)+∂C∗∂E∗(j)EPulse(j)+O(EPulse(j)2), | (3.19) |
i.e.,
∂C∗∂E∗(j)=C∗[EPulse(j)](i)−C∗(i)EPulse(j)+O(EPulse(j)), |
it follows that (3.18) converges with order O(EPulse(j)) to SCC(j), similar to the first order convergence of (3.5).
Similar to the method discussed in Section 3.1, the SCC calculation discussed in this section relies on pulse experiments and on first order approximations. In particular, while the method in Section 3.1 relies on a first order approximation of ∂W∗∂E∗(j) and ∂W∗∂C∗(j), the third method discussed here uses a first order approximation of ∂C∗∂E∗(j), respectively. However, as discussed in Remarks 1 and 2, the order of convergence of both methods is the same. The value of the formula in Theorem 1 is that the expression (3.15) (or (3.18), respectively, ) has a familiar interpretation as the net present value (NPV) of the stream of damages due to an emissions pulse at time j and is conventionally expressed in units of $/tCO2. Note, however, that in computing the NPV in (3.18), the discount factor δj,i is time-varying due to its dependence on the growth rate g(⋅) of per capita consumption; cf. (3.16). This marks a subtle, but potentially interesting point of departure between (3.18) and a large body of literature which applies the well-known, continuous-time Ramsey equation for the discount rate r=ρ+α⋅g for constant g, to the discrete-time setting (see [31], for example).
In this section we discuss three aspects in the calculation of the SCC using the DICE model. For the calculations we use the standard setting of the DICE-2016R model (see [24, Table 2] for the parameter selection) if not specified differently. For the numerical calculations we use the implementation [32]. While we use a horizon of N=100, consistent with the parameters in DICE-2016R, we only focus on the first time steps in the visualizations.
Before we focus on the calculation of the SCC, we recall one of its illustrative definitions and visualizations used in the literature and highlight the anticipatory response of solutions of the optimal control problem (2.9) when emission or consumption impulses are added at different time steps. In the study by Nordhaus W [4] the SCC is illustrated as "the economic cost caused by an additional ton of carbon dioxide emissions" which is usually illustrated by adding a pulse to the first time step in the future [4, Figure 1]. In particular, with respect to [4, Figure 1], if time step 1 indicates the initial conditions, an additional ton of carbon dioxide emissions is added at time step 2. Under these assumptions, baseline emission and consumption pathways are compared with perturbed emission and consumption pathways. The baseline emissions and consumption pathways using the DICE-2016R model are visualized in Figure 1.
By adding the additional tonne at the earliest possible time in the future, one might get the misleading impression that a perturbation EPulse(j), j∈{0,…,N−1}, only affects emissions and consumption at time steps k∈{j,…,N−1}. This is however not the case, as shown in Figure 2, where a pulse is added at time j=1, j=10, and j=20, respectively. In Figure 2 the differences between the baseline solution, i.e., EPulse(j)=CPulse(j)=0 for all j∈{0,…,N−1}, and perturbed solutions with CPulse(j)=1 for j=1, j=10, and j=20 are shown. A pulse at time j∈{0,…,N−1} has an impact on the whole pathways C[EPulse(j)](⋅) and E[EPulse(j)](⋅) due to the optimization involved in their computation. This also becomes clear from the definition of the partial derivative (3.2) used in the formula for the SCC: For computing this derivative, first the pulse is added, and then the optimization is performed, implying that the optimizer is aware of the pulse at time j when performing the optimization for the times i=0,…,j−1. In other words, if there is a change of emissions in the future, which importantly by assumption is known, then the change is anticipated and encoded in the optimal control problem (2.9). This explains the non-negligible differences in C[CPulse(20)](i) and C(i) and in E[CPulse(i)](20) and E(i), respectively, for i<20, for example.
As a next step, we show that all three methods discussed in Section 3 essentially lead to the same SCC value. Figure 3 compares the three methods with each other, where for the pulse experiments the values CPulse(j)=1 and CPulse(j)=1, j∈{0,…,N−1}, were used for the finite difference approximations (which corresponds to a one tonne impulse in the implementation). On the scale on the left of Figure 3, the SCC values are indistinguishable. However, the difference between the Lagrange multiplier method and the finite difference approximation (3.5) and the formula (3.18) derived in Theorem 1 appears to grow nonlinearly with j∈{0,…,N−1} as visualized in Figure 3 on the right.
In Figure 4 the errors in the SCC calculation with respect to EPulse and CPulse are shown. In particular, the impulses EPulse(j) and CPulse(j) are varied from 0.01 to 5 for different values of j∈{0,…,N−1}. As expected from Remarks 1 and 2, the error grows linearly with EPulse and CPulse, respectively.
Remark 3. While in theory the SCCs computed with the three methods coincide, the precise numerical values we obtain are sensitive with respect to EPulse and CPulse, the chosen optimization algorithms and the parameter selection of the optimization algorithm. Figure 5 shows the same results as Figure 4 with the only difference that the horizon N=100 has been replaced with N=150. In this case the parameter selection used in the IPOPT solver does not lead to reliable results for all impulse perturbations.
As a final visualization we show the change of the SCC with respect to the discount rate in Figure 6, i.e., ddρSCC(j) is calculated and shown in Figure 6. Here, ddρSCC(j) is approximated through a finite difference approximation
ddρSCC(j)≈1Δρ(SCC[ρ+Δρ](j)−SCC[ρ](j)), |
where SCC[ρ+Δρ](j) and SCC[ρ](j) are calculated using the Lagrange multiplier method presented in Section 3.2.
In this paper, we have investigated and compared three different methods for the computation of the SCC in integrated assessment models. While our discussion is focused on the DICE model, it is equally relevant for other models including PAGE and FUND, to obtain a characterization of the SCC that is consistent among the literature. While the first two methods, which rely on perturbations of a baseline model and on dual variables of an appropriately defined optimal control problem, respectively, are commonly known and used, the third method relies on a novel formula stated in Theorem 1. The novel formula allows a direct analysis of the impact of the discount factor in the calculation of the NPV and the SCC through Eq (3.16), which subsequently provides insights in SCC values stemming from different integrated assessment models. The consistency of the three methods is analyzed analytically through first order approximations of Taylor polynomials and through dual variables and shadow price interpretations. The theoretical findings are additionally confirmed through numerical simulations, while it is also highlighted that an appropriate parameter selection in the optimal control formulation and in the underlying solver is important. Future work will focus on the reliability of the three methods to compute the SCC with respect to the horizon N and the parameters of the DICE model as discussed in Remark 3.
The authors declare they have not used Artificial Intelligence (AI) tools in the creation of this article.
The authors declare no conflict of interest.
In this section we recapitulate optimality conditions for constrained optimization problems from Bertsekas DP' study [28], which are needed for the proof of the main Theorem 1 in Appendix C. In particular, for the convenience of the reader, we rewrite results for minimization problems in terms of maximization problems discussed in this paper. We consider optimization problems of the form
f∗=maxxf(x)subjecttoh(x)=0,g(x)≤0, | (A.1) |
where x∈Rn, f:Rn→R, h:Rn→Rm, and g:Rn→Rp for n,m,p∈N. With the Lagrange multipliers λ∈Rm and μ∈Rp≥0, the Lagrangian corresponding to (A.1) is defined as
L(x,λ,μ)=f(x)−λTh(x)−μTg(x). |
Additionally, for x∈Rn we define
A(x)={i∈{1,…,p} | gi(x)=0} |
as the corresponding active set with respect to the inequality constraints g.
Theorem 2 (Second order sufficiency conditions, [28, Prop. 3.3.2]). Consider the optimization problem (A.1) and assume that f, h, and g are twice continuously differentiable. Let x∗∈Rn, λ∗∈Rm, and μ∗∈Rm satisfy
∇xL(x∗,λ∗,μ∗)=0,h(x∗)=0,g(x∗)≤0,μ∗j≥0,∀j∈{1,…,p}μ∗j=0,∀j∈{1,…,p}∖A(x∗)yT∇2xxL(x∗,λ∗,μ∗)y<0 |
for all y≠0 such that
∇hi(x∗)Ty=0,∀ i=1,…,m,∇gi(x∗)Ty=0∀ i∈A(x∗). |
Finally, assume that
μ∗j>0∀j∈A(x∗). |
Then, x∗ is a strict local maximum of (A.1) and we say that (A.1) satisfies the second order sufficient condition at x∗.
Additionally we will need the following result for parameter dependent optimization problems where the parameter only appears in the objective function f:Rn×R→R.
Theorem 3 ([33, Corollary 3.4.2]). Consider the parameter dependent optimization problem
f∗(p)=maxxf(x,p)subjecttoh(x)=0g(x)≤0 | (A.2) |
with Lagrangian
L(x,λ,μ,p)=f(x,p)−λTh(x)−μTg(x), |
Lagrange multipliers λ, μ, and parameter p∈R in the objective function. Assume that the second order sufficiency conditions of Theorem 2 are satisfied. Then, in a neighborhood around p=0, it holds that
f∗(p)=L(x∗(p),λ∗(p),μ∗(p),p), | (A.3) |
∇pf∗(p)=∇pf(x∗(p),p), | (A.4) |
∇2pf∗(p)=∇2xf(x,p)∇px(p)+∇2pf(x(p),p). | (A.5) |
To prove Theorem 1 we will make use of results in [33] and the results summarized in Appendix A. Before we begin with the actual proof, we note that for
x=[sμ] |
the optimization problems (2.9) can be written in the general form
f∗(p)=maxxf(x,p)subjecttog(x)≤0, | (B.1) |
where the equality constraints are eliminated, and the box constraints are captured in the function g. Here, the parameter p represents the pulses EPulse(j) and CPulse(j), or equivalently, p represents a change in C(j) and E(j), for j∈{0,…,N−1}. Since we only consider the perturbation with respect to C or E and only with respect to one j∈{0,…,N−1} at a time, we assume that p is one dimensional. With these definitions we can use Theorem 3 (without the function h) in the following. Additionally we note that according to the definition of the functions involved in the optimal control problem (2.9), the functions f and g are twice continuously differentiable.
Proof of Theorem 1: We begin the proof by rewriting the partial derivatives
∂W∗∂C∗(j)and∂W∗∂E∗(j) |
for j∈{0,…,N−1}. To this end we define
ci=ci(C(i))=1000C(i)L(i), | (B.2) |
which is a scaling of C(i) through the known parameter L(i), i∈{0,…,N−1}. Thus, the utility U defined in (2.7) can be written as a function of ci,
U(ci)=L(i)c1−αi−11−α. | (B.3) |
For the derivative we obtain
∂U∂ci(ci)=L(i)c−αi, | (B.4) |
and with respect to the original variables, it holds that
∂U∂C(i)(ci)=∂U(ci)∂ci∂ci(C(i))∂C(i)=L(i)c−αi1000L(i)=1000c−αi. | (B.5) |
With Theorem 3, and in particular with (A.4) for p=EPulse(j), j∈{0,…,N−1}, it holds that
∂W∗∂E∗(j)=∂∂E∗(j)(N−1∑i=0(1−ρ)−ΔiU(c∗i)). |
Using the notation
γi=(1−ρ)−Δi |
to shorten the expressions and the equation derived in Equation (B.5), the right-hand side can be written in the form
∂W∗∂E∗(j)=N−1∑i=0γi∂U∂E∗(j)(c∗i)=N−1∑i=0γi∂U(c∗i)∂C(i)∂C∗(i)∂E(j)=N−1∑i=0γi1000(c∗i)−α∂C∗(i)∂E(j). | (B.6) |
Similarly, the application of Equation (A.3) in Theorem 3, where p represents a change in C(j), j∈{0,…,N−1}, leads to the expression
∂W∗∂C∗(j)=∂∂C∗(j)(N−1∑i=0γiU(c∗i))=N−1∑i=0γi∂U(c∗i)∂C∗(j)=N−1∑i=0γi∂U(c∗i)∂C∗(i)∂C∗(i)∂C∗(j)=N−1∑i=0γi1000(c∗i)−α∂C∗(i)∂C∗(j). | (B.7) |
As a next step, we derive Taylor expansions of the optimal value function W∗. In particular, for parameters pi representing a change in C∗(j), (i.e., a pulse CPulse(i)) for i∈{0,…,N−1}, we derive Taylor expansions in a neighborhood of pi=0. Using the definition of ci in Equation (B.2), the consumption (2.6) can be equivalently written as
ci=103L(i)(11+aTAT(i)2)(1−θ1(i)μ(i)θ2)A(i)K(i)γL(i)1−γ(1−s(i))+103L(i)CPulse(i). | (B.8) |
Moreover, with the definition cPulse(i)=103L(i)CPulse(i) and ci=˜ci+cPulse(i), Equation (B.8) can be written as
˜ci=L(i)103(11+aTAT(i)2)(1−θ1(i)μ(i)θ2)A(i)K(i)γL(i)1−γ(1−s(i)) | (B.9) |
and the utility function can be written as
U[cPulse(i)](˜ci)=L(i)(˜ci+cPulse(i))1−α−11−α. | (B.10) |
Thus, instead of the parameter CPulse(j) in the consumption (2.6), we can use a scaled parameter directly in the utility function (2.7), and the optimal value function satisfies
W∗[pi]=γiU(˜c∗i[pi]+pi)+N∑k=0k≠iγkU(˜c∗k[pi]) |
with pi=cPulse(i). According to Theorem 3 (using Equation (A.5)), the optimal value function is twice continuously differentiable with respect to the parameter pi, and using Theorem 3, Equation (A.4), the Taylor approximation is given by
W∗[pi]=W∗[0]+γiL(i)(˜c∗i[0])−αpi+Ri(pi) |
where
Ri(pi)=12d2dp2iW∗[ξ]p2i | (B.11) |
for ξ∈[0,pi]. For i,j∈{0,…,N−1}, we take specific perturbations pi≠0, pj≠0 with the property W∗[pi]=W∗[pj] so that the Taylor approximation satisfies
W∗[0]+γiL(i)(˜c∗i[0])−αpi+Ri(pi)=W∗[0]+γjL(j)(˜c∗j[0])−αpj+Rj(pj). | (B.12) |
A pj satisfying (B.12) can be found for each sufficiently small pi because the terms in front of pi and pj in (B.12) are not zero due to the assumption that (2.9) satisfies the second order sufficiency conditions. In fact, γjL(j)(˜c∗j[0])−α=0 implies that s∗(i)=1 or θ1(i)μ∗(i)θ2=1 (see Equation (B.9)). If s∗(i)=1, then μ∗(i)∈[0,1] can be selected arbitrarily, and if θ1(i)μ∗(i)θ2=1, then s∗(j)∈[0,1] can be selected arbitrarily, i.e., the optimal solution of (2.9) is not characterized through a strict local maximum, which contradicts Theorem 2. Under these assumptions stated above, pj is a function of pi, and limpi→0pj(pi)=0 (since W∗ is continuously differentiable). Rearranging terms yields
pjpi=γiL(i)(˜c∗i[0])−αγjL(j)(˜c∗j[0])−α+Ri(pi)−Rj(pj)piγjL(j)(˜c∗j[0])−α | (B.13) |
and allows us to investigate the limit pi→0, pi≠0, (i.e., pj(pi)→0). We observe that
limpi→0pj(pi)pi=limpi→0pj(pi)−pj(0)pi−0=dpjdpi(0), |
and since the remainder in Equation (B.11) satisfies Ri(pi)=O(p2i), we obtain
limpi→01piRi(pi)=0. |
To be able to neglect the second term in the right-hand side of Equation (B.13), it is left to show that 1piRj(pj(pi)) converges to zero for pi→0. With the definition of the remainder Rj(pj)=12d2dp2jW∗j[ξ]pj(pi)2 in Equation (B.11), the limit satisfies
limpi→0(1piRj(pj(pi)))=limpi→0(12d2dp2jW∗[ξ(pi)]pj(pi)pipj(pi))=12d2dp2jW∗[ξ(0)]dpjdpi(0)pj(0)=12d2dp2jW∗dpjdpi(0)⋅0=0. |
Consequently, using Equation (B.4) together with ˜ci=ci in the case pi=0, it holds that
limpi→0pjpi=γi−jL(i)(c∗i)−αL(j)(c∗j)−α=γi−j∂U∂ci(c∗i)∂U∂cj(c∗j). | (B.14) |
To return to the original variables C(i), we recall the coordinate transformation (B.2), and use the notation Pi=CPulse(j)=L(i)103pi.
With the above computations we are finally in the position to derive the partial derivative of C∗(i) with respect to C∗(j) for i,j∈{0,…,N−1}. In particular, using the chain rule and the definition of the derivative, it holds that
∂C∗(i)∂C∗(j)=∂W∗∂C∗(j)∂W∗∂C∗(i)=limPj→01Pj(W∗[Pj]−W∗j)limPi→01Pi(W∗[Pi]−W∗). |
Using the relation between pi and Pi, this expression can be equivalently written as
∂C∗(i)∂C∗(j)=limpj→0103L(j)pj(W∗[pj]−W∗)limpi→0103L(i)pi(W∗[pi]−W∗)=limpi→0L(i)piL(j)pj(W∗[pj]−W∗)(W∗[pi]−W∗). |
Finally, with the result derived in Equation (B.14), it holds that
∂C∗(i)∂C∗(j)=limpi→0L(i)piL(j)pj(pi)=γj−i(c∗j)−α(c∗i)−α | (B.15) |
where the second term is equal to one since W∗[pi]=W∗[pj(pi)] according the assumption made at the beginning of the derivation of (B.14) in (B.12).
To complete the proof we combine the results so far in the definition of SCC(j). With Equations (B.6) and (B.7), the SCC can be expressed as
SCC(j)=−1000∂W∗/∂E(j)∂W∗/∂C(j)=−1000∑N−1i=0γi1000(c∗i)−α∂C∗(i)∂E∗(j)∑N−1i=0γi1000(c∗i)−α∂C∗(i)∂C∗(j) |
and which becomes
SCC(j)=−1000∑N−1i=0γi(c∗i)−α∂C∗(i)∂E∗(j)∑N−1i=0γi(c∗i)−αγj−i(c∗j)−α(c∗i)−α |
through the identity (B.15). Finally, reformulating individual terms and recalling the definition of δji in Theorem 1 completes the proof:
SCC(j)=−1000∑N−1i=0γi(c∗i)−α∂C∗(i)∂E∗(j)∑N−1i=0γj(c∗j)−α=−1000N−1∑i=0γi−j(c∗i)−α(c∗j)−α∂C∗(i)∂E∗(j)=−1000N−1∑i=0∂C∗(i)∂E∗(j)δji. |
As a last result in this paper, we show that (3.5) converges with order O(CPulse(j)+EPulse(j)) to SCC(j). Using the notation introduced in (3.4) and (3.5), the SCC satisfies
SCC(j)=−1000∂W∗∂E∗(j)∂W∗∂C∗(j)=−1000W∗[EPulse(j)]−W∗EPulse(j)+ΦE(EPulse(j))W∗[CPulse(j)]−W∗CPulse(j)+ΦC(CPulse(j)) | (C.1) |
=−1000CPulse(j)EPulse(j)⋅W∗[EPulse(j)]−W∗W∗[CPulse(j)]−W∗−1000Φ(EPulse(j),CPulse(j)) | (C.2) |
for unknown functions ΦE, ΦC, and Φ. However, according to the Taylor expansions in (3.6), it holds that ΦE(EPulse(j))=O(EPulse(j)) and ΦC(CPulse(j))=O(CPulse(j)).
We investigate properties of the function Φ. To simplify the notation, we drop the index in the derivations, i.e., we investigate the equation
W∗[E]−W∗E+ΦE(E)W∗[C]−W∗C+ΦC(C)=CE⋅W∗[E]−W∗W∗[C]−W∗+Φ(E,C), |
or equivalently
CE(W∗[E]−W∗)+EΦE(E)(W∗[C]−W∗)+CΦC(C)=CE(W∗[E]−W∗)+EC(W∗[C]−W∗)Φ(E,C)W∗[C]−W∗. |
We further simplify the notation and define ΔW∗E=W∗[E]−W∗ and ΔW∗C=W∗[C]−W∗. Then, multiplying by the denominator leads to the equation
ΔW∗CΔW∗E+ΔW∗CEΦE(E)=(ΔW∗C+CΦC(C))ΔW∗E+EC(ΔW∗C+CΦC(C))ΔW∗CΦ(E,C)=ΔW∗CΔW∗E+CΦC(C)ΔW∗E+EC(ΔW∗C+CΦC(C))ΔW∗CΦ(E,C). |
After canceling and rearranging terms, we are left with the expression:
ΔW∗CEΦE(E)−CΦC(C)ΔW∗E=EC(ΔW∗C+CΦC(C))ΔW∗CΦ(E,C). |
Since W∗ is differentiable, i.e., the limits
∂W∗∂E∗(j)=limEPulse(j)→0EPulse(j)≠0W∗[EPulse(j)]−W∗EPulse(j),∂W∗∂C∗(j)=limCPulse(j)→0CPulse(j)≠0W∗[CPulse(j)]−W∗CPulse(j) | (C.3) |
exist, it holds that ΔW∗E=O(EPulse(j)) and ΔW∗C=O(CPulse(j)). Using these properties in the last equation simplifies the expression to
O(C)EO(E)+CO(C)O(E)=EC(O(C)+CO(C))O(C)Φ(E,C) |
or equivalently
O(CE2)+O(C2E)=(O(CE)+O(C2E))Φ(E,C)=O(CE)Φ(E,C). |
In particular, Φ satisfies Φ(E,C)=O(C+E), proving the assertion.
In this section we report the parameters used to define the R-DICE2016 model. The parameters in Table 1 are taken from [24, Appendix A], which additionally contains the parameters for R-DICE2013. Moreover, the initial conditions used to define X0 in (3.9) are defined as
[TAT(0)TLO(0)K(0)]=[0.850.0068223],[MAT(0)MUP(0)MLO(0)]=[8514601740],σ0=e0q0(1−μ0)=35.85105.5(1−0.03). |
Parameter | Value | Unit |
Δ | 5 | years |
t0 | 2015 | year |
N | 100 | time steps |
μ0 | 0.03 | |
Climate diffusion parameters | ||
ϕ11 | 0.8718 | |
ϕ12 | 0.0088 | |
ϕ21 | 0.025 | |
ϕ22 | 0.975 | |
Carbon cycle diffusion parameters | ||
ζ11 | 0.88 | |
ζ12 | 0.196 | |
ζ21 | 0.12 | |
ζ22 | 0.797 | |
ζ23 | 0.001465 | |
ζ32 | 0.007 | |
ζ33 | 0.99853488 | |
Other geophysical parameters | ||
η | 3.6813 | W/m2 |
ξ1 | 0.1005 | |
ξ2 | 12/44 | GtC/GtCO2 |
MAT,1750 | 588 | GtC |
f0 | 0.5 | W/m2 |
f1 | 1.0 | W/m2 |
tf | 17 | time steps |
Parameter | Value | Unit |
EL0 | 2.6 | GtCO2/yr |
δEL | 0.115 | |
Socioeconomic parameters | ||
γ | 0.3 | |
θ2 | 2.6 | n/a |
a2 | 0.00236 | |
a3 | 2 | n/a |
δK | 0.1 | |
α | 1.45 | |
ρ | 0.015 | |
L0 | 7403 | millions people |
La | 11500 | millions people |
ℓg | 0.134 | |
A0 | 5.115 | |
ga | 0.076 | |
δA | 0.005 | |
σ0 | 0.3503 | GtC / trillions 2010USD |
gσ | 0.0152 | |
δσ | 0.001 | |
pb | 550 | 2010USD/tCO2 |
δpb | 0.025 | |
scale1 | 0.030245527 | n/a |
scale2 | 10993.704 | 2010USD |
[1] |
Archer D, Eby M, Brovkin V, et al. (2020) Atmospheric lifetime of fossil fuel carbon dioxide. Annu Rev Earth Planet Sci 37: 117–134. https://doi.org/10.1146/annurev.earth.031208.100206 doi: 10.1146/annurev.earth.031208.100206
![]() |
[2] |
Eby M, Zickfeld K, Montenegro A, et al.(2009) Lifetime of anthropogenic climate change: Millennial time scales of potential CO2 and surface temperature perturbations. J Clim 22: 2501–2511. https://doi.org/10.1175/2008JCLI2554.1 doi: 10.1175/2008JCLI2554.1
![]() |
[3] |
Marten A L, Kopits E A, Griffiths C W, et al. (2015) Incremental CH4 and N2O mitigation benefits consistent with the US Government's SC-CO2 estimates. Clim Policy 15: 272–298. https://doi.org/10.1080/14693062.2014.912981 doi: 10.1080/14693062.2014.912981
![]() |
[4] |
Nordhaus W (2014) Estimates of the social cost of carbon: Concepts and results from the DICE-2013R model and alternative approaches. J Assoc Environ Resour Econ 1: 273–312. https://doi.org/10.1086/676035 doi: 10.1086/676035
![]() |
[5] | Hope C, Newbery D (2008) Calculating the social cost of carbon. In M. Grubb, T. Jamasb, and M. G. Pollitt, editors, Delivering a Low Carbon Electricity System: Technologies, Economics and Policy, chapter 2, 31–63. Cambridge University Press, Cambridge, 2008. |
[6] |
Anthoff D, Tol R S J, Yohe G W (2009) Discounting for climate change. Economics: the Open-Access, Open-Assessment e-Journal, 3: 1–24. https://doi.org/10.5018/economics-ejournal.ja.2009-24 doi: 10.5018/economics-ejournal.ja.2009-24
![]() |
[7] | D. Nordhaus W, Sztorc P (2013) DICE 2013R: Introduction and User's Manual, second edition, 31 October 2013. |
[8] |
Ramsey FP(1928) A mathematical theory of saving. Econ J 38: 543–559. https://doi.org/10.2307/2224098 doi: 10.2307/2224098
![]() |
[9] |
Anthoff D, Tol RSJ (2013) The uncertainty about the social cost of carbon: A decomposition analysis using FUND. Clim Change 117: 515–530. https://doi.org/10.1007/s10584-013-0706-7 doi: 10.1007/s10584-013-0706-7
![]() |
[10] |
Anthoff D, Tol RSJ, Yohe GW (2009) Risk aversion, time preference, and the social cost of carbon. Environ Res Lett 4: 1–7. https://doi.org/10.1088/1748-9326/4/2/024002 doi: 10.1088/1748-9326/4/2/024002
![]() |
[11] |
Hope C (2013) Critical issues for the calculation of the social cost of CO2: why the estimates from PAGE09 are higher than those from PAGE2002. Clim Change 117: 531–543. https://doi.org/10.1007/s10584-012-0633-z doi: 10.1007/s10584-012-0633-z
![]() |
[12] | Kögel T (2012) The rate of change of the social cost of carbon and the social planner's Hotelling rule. Economics: the Open-Access, Open-Assessment e-Journal 1–26. 2012-37. |
[13] | A. L. Marten. (2011) Transient temperature response modeling in IAMs: The effects of over simplification on the SCC. Economics: the Open-Access, Open-Assessment e-Journal 1–44. https://doi.org/10.5018/economics-ejournal.ja.2011-18 |
[14] |
Marten A L, Newbold S C (2012) Estimating the social cost of non-CO2 GHG emissions: Methane and nitrous oxide. Energy Policy 51: 957–972. https://doi.org/10.1016/j.enpol.2012.09.073 doi: 10.1016/j.enpol.2012.09.073
![]() |
[15] | Tol RSJ (2009) An analysis of mitigation as a response to climate change. Technical report, Copenhagen Consensus Center, 2009. |
[16] |
Moyer E J, Woolley M D, Matteson N J, et al. (2014) Climate impacts on economic growth as drivers of uncertainty in the social cost of carbon. J Leg Stud 43: 401–425. https://doi.org/10.1086/678140 doi: 10.1086/678140
![]() |
[17] | Tol RSJ (2014) Climate Economics: Economic Analysis of Climate, Climate Change, and Climate Policy. Edward Elgar, 2014. |
[18] | Economides G, Papandreou A, Sartzetakis E, et al. (2018) The Economics of Climate Change. Bank of Greece, 2018. |
[19] |
Newbold S C, Griffiths C, Moore C, et al. (2013) A rapid assessment model for understanding the social cost of carbon. Clim Change Econ 4: 1–40. https://doi.org/10.1142/S2010007813500012 doi: 10.1142/S2010007813500012
![]() |
[20] | Ploeg F (2020) Discounting and climate policy, 2020. CESifo Working Paper no. 8441, Available at SSRN: https://ssrn.com/abstract = 3657977+. |
[21] |
Arrow K, Cropper M, Gollier C, et al. (2013) Determining benefits and costs for future generations. Science 341: 349–350. https://doi.org/10.1126/science.1235665 doi: 10.1126/science.1235665
![]() |
[22] |
Groom B, Hepburn C, Koundouri P, et al. (2005) Declining discount rates: The long and the short of it. Environ Resour Econ 32: 445–493. https://doi.org/10.1007/s10640-005-4681-y doi: 10.1007/s10640-005-4681-y
![]() |
[23] |
Farber DA (2015) Gambling over growth: Economic uncertainty, discounting, and regulatory policy. J Leg Stud 44: S509–S528. https://doi.org/10.1086/676690 doi: 10.1086/676690
![]() |
[24] |
Kellett C M, Weller S R, Faulwasser T, et al. (2019) Feedback and optimal control in climate economics. Annu Rev Control 47: 7–20. https://doi.org/10.1016/j.arcontrol.2019.04.003 doi: 10.1016/j.arcontrol.2019.04.003
![]() |
[25] |
Hassell MP (1975) Density-dependence in single-species populations. Journal of Animal Ecology 44: 283–295. https://doi.org/10.2307/3863 doi: 10.2307/3863
![]() |
[26] |
Wächter A, Biegler LT (2006) On the implementation of an interior-point filter line-search algorithm for large-scale nonlinear programming. Mathematical programming 106: 25–57. https://doi.org/10.1007/s10107-004-0559-y doi: 10.1007/s10107-004-0559-y
![]() |
[27] | Drud AS (1994) CONOPT-a large-scale GRG code. ORSA Journal on computing 6: 207–216. |
[28] | Bertsekas DP(1999) Nonlinear Programming. Athena Scientific, 1999. https://doi.org/10.1287/ijoc.6.2.207 |
[29] | Grass D, Caulkins J P, Feichtinger G, et al. (2008) Optimal Control of Nonlinear Processes: With Applications in Drugs, Corruption, and Terror. Springer, 2008. https://doi.org/10.1007/978-3-540-77647-5 |
[30] | Seierstad A, Sydsaeter K (1986) Optimal Control Theory with Economic Applications. Elsevier North-Holland, 1986. |
[31] | Campbell J Y, Viceira L M (2002) Strategic Asset Allocation: Portfolio Choice for Long-Term Investors. Oxford University Press, 2002. https://doi.org/10.1093/0198296940.001.0001 |
[32] | Faulwasser T, Kellett C M, Weller S R (2018) MPC-DICE: An open-source Matlab implementation of receding horizon solutions to DICE. In Proc. 1st IFAC Workshop on Integrated Assessment Modeling for Environmental Systems, Brescia, Italy, May 2018. |
[33] | Fiacco AV (1983) Introduction to Sensitivity and Stability Analysis in Nonlinear Programming. Academic Press, 1983. |
1. | Sergey Orlov, Elena Rovenskaya, Julia Puaschunder, Willi Semmler, Green bonds, transition to a low-carbon economy, and intertemporal welfare allocation: Evidence from an extended DICE model, 2024, 11, 2372-0352, 628, 10.3934/environsci.2024031 |
Parameter | Value | Unit |
Δ | 5 | years |
t0 | 2015 | year |
N | 100 | time steps |
μ0 | 0.03 | |
Climate diffusion parameters | ||
ϕ11 | 0.8718 | |
ϕ12 | 0.0088 | |
ϕ21 | 0.025 | |
ϕ22 | 0.975 | |
Carbon cycle diffusion parameters | ||
ζ11 | 0.88 | |
ζ12 | 0.196 | |
ζ21 | 0.12 | |
ζ22 | 0.797 | |
ζ23 | 0.001465 | |
ζ32 | 0.007 | |
ζ33 | 0.99853488 | |
Other geophysical parameters | ||
η | 3.6813 | W/m2 |
ξ1 | 0.1005 | |
ξ2 | 12/44 | GtC/GtCO2 |
MAT,1750 | 588 | GtC |
f0 | 0.5 | W/m2 |
f1 | 1.0 | W/m2 |
tf | 17 | time steps |
Parameter | Value | Unit |
EL0 | 2.6 | GtCO2/yr |
δEL | 0.115 | |
Socioeconomic parameters | ||
γ | 0.3 | |
θ2 | 2.6 | n/a |
a2 | 0.00236 | |
a3 | 2 | n/a |
δK | 0.1 | |
α | 1.45 | |
ρ | 0.015 | |
L0 | 7403 | millions people |
La | 11500 | millions people |
ℓg | 0.134 | |
A0 | 5.115 | |
ga | 0.076 | |
δA | 0.005 | |
σ0 | 0.3503 | GtC / trillions 2010USD |
gσ | 0.0152 | |
δσ | 0.001 | |
pb | 550 | 2010USD/tCO2 |
δpb | 0.025 | |
scale1 | 0.030245527 | n/a |
scale2 | 10993.704 | 2010USD |
Parameter | Value | Unit |
Δ | 5 | years |
t0 | 2015 | year |
N | 100 | time steps |
μ0 | 0.03 | |
Climate diffusion parameters | ||
ϕ11 | 0.8718 | |
ϕ12 | 0.0088 | |
ϕ21 | 0.025 | |
ϕ22 | 0.975 | |
Carbon cycle diffusion parameters | ||
ζ11 | 0.88 | |
ζ12 | 0.196 | |
ζ21 | 0.12 | |
ζ22 | 0.797 | |
ζ23 | 0.001465 | |
ζ32 | 0.007 | |
ζ33 | 0.99853488 | |
Other geophysical parameters | ||
η | 3.6813 | W/m2 |
ξ1 | 0.1005 | |
ξ2 | 12/44 | GtC/GtCO2 |
MAT,1750 | 588 | GtC |
f0 | 0.5 | W/m2 |
f1 | 1.0 | W/m2 |
tf | 17 | time steps |
Parameter | Value | Unit |
EL0 | 2.6 | GtCO2/yr |
δEL | 0.115 | |
Socioeconomic parameters | ||
γ | 0.3 | |
θ2 | 2.6 | n/a |
a2 | 0.00236 | |
a3 | 2 | n/a |
δK | 0.1 | |
α | 1.45 | |
ρ | 0.015 | |
L0 | 7403 | millions people |
La | 11500 | millions people |
ℓg | 0.134 | |
A0 | 5.115 | |
ga | 0.076 | |
δA | 0.005 | |
σ0 | 0.3503 | GtC / trillions 2010USD |
gσ | 0.0152 | |
δσ | 0.001 | |
pb | 550 | 2010USD/tCO2 |
δpb | 0.025 | |
scale1 | 0.030245527 | n/a |
scale2 | 10993.704 | 2010USD |