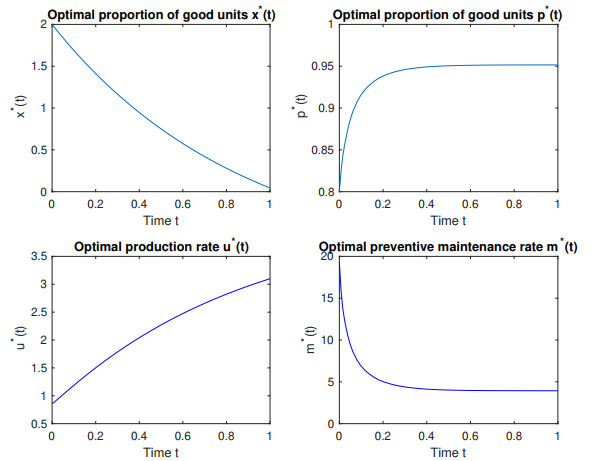
Citation: Katharina Meixner, Mona Kubiczek, Ines Fritz. Microplastic in soil–current status in Europe with special focus on method tests with Austrian samples[J]. AIMS Environmental Science, 2020, 7(2): 174-191. doi: 10.3934/environsci.2020011
[1] | Mahidin, Asri Gani, Saiful, Muhammad Irham, Wulan Windari, Erdiwansyah . An overview of the potential risks, sources, and analytical methods for microplastics in soil. AIMS Environmental Science, 2022, 9(2): 185-216. doi: 10.3934/environsci.2022013 |
[2] | Judith S. Weis . Improving microplastic research. AIMS Environmental Science, 2019, 6(5): 326-340. doi: 10.3934/environsci.2019.5.326 |
[3] | Fabiana Carriera, Cristina Di Fiore, Pasquale Avino . Trojan horse effects of microplastics: A mini-review about their role as a vector of organic and inorganic compounds in several matrices. AIMS Environmental Science, 2023, 10(5): 732-742. doi: 10.3934/environsci.2023040 |
[4] | Abigail W. Porter, Sarah J. Wolfson, Lily. Young . Pharmaceutical transforming microbes from wastewater and natural environments can colonize microplastics. AIMS Environmental Science, 2020, 7(1): 99-116. doi: 10.3934/environsci.2020006 |
[5] | Gina M. Moreno, Keith R. Cooper . Morphometric effects of various weathered and virgin/pure microplastics on sac fry zebrafish (Danio rerio). AIMS Environmental Science, 2021, 8(3): 204-220. doi: 10.3934/environsci.2021014 |
[6] | B. Ravit, K. Cooper, G. Moreno, B. Buckley, I. Yang, A. Deshpande, S. Meola, D. Jones, A. Hsieh . Microplastics in urban New Jersey freshwaters: distribution, chemical identification, and biological affects. AIMS Environmental Science, 2017, 4(6): 809-826. doi: 10.3934/environsci.2017.6.809 |
[7] | Andrew Wirnkor Verla, Christian Ebere Enyoh, Isiuku Beniah Obinna, Evelyn Ngozi Verla, Wang Qingyue, Md. Akhter Hossain Chowdhury, Emmanuel Chinedu Enyoh, Tanzin Chowdhury . Effect of macro-and micro-plastics in soil on growth of Juvenile Lime Tree (Citrus aurantium). AIMS Environmental Science, 2020, 7(6): 526-541. doi: 10.3934/environsci.2020033 |
[8] | B. Ravit, K. Cooper, B. Buckley, I. Yang, A. Deshpande . Organic compounds associated with microplastic pollutants in New Jersey, U.S.A. surface waters. AIMS Environmental Science, 2019, 6(6): 445-459. doi: 10.3934/environsci.2019.6.445 |
[9] | Akekawat Vitheepradit, Taeng-On Prommi . Microplastics in surface water and tissue of white leg shrimp, Litopenaeus vannamei, in a cultured pond in Nakhon Pathom Province, Central Thailand. AIMS Environmental Science, 2023, 10(4): 478-503. doi: 10.3934/environsci.2023027 |
[10] | Nasrul Arahman, Cut Meurah Rosnelly, Sri Mulyati, Wafiq Alni Dzulhijjah, Nur Halimah, Rinal Dia'ul Haikal, Syahril Siddiq, Sharfina Maulidayanti, Muhammad Aziz, Mathias Ulbricht . Investigation of microplastics in community well water in Banda Aceh, Indonesia: a separation technique using polyethersulfone-poloxamer membrane. AIMS Environmental Science, 2025, 12(1): 53-71. doi: 10.3934/environsci.2025003 |
The last couple of decades have seen a large number of researchers emphasizing the integration of two or more models into a single one to coordinate the efforts of two or more functions in a firm. Indeed, as shown in many studies, decision making in an integrated model can lead to significant savings over decision making in the separate models. The integrated models we have in mind are the production planning model and the maintenance model. This integrated model has been largely studied, see for example the overviews of Ben-Daya and Rahim [1] and Van Horenbeek et al. [2]. The model of interest to us was introduced by Cho et al. [3]. The performance of the production process they considered worsens over time in the absence of maintenance. They measured the performance of the process in terms of the proportion of 'good' (non-defective) units produced.
Using the maximum principle, the authors obtained the necessary conditions for the controls (production rate and preventive maintenance rate) to be optimal in the case of a quadratic production cost function and linear maintenance cost function. They used a two-stage algorithm, tailored to this problem, to solve for the optimal controls.
This model has been further investigated by other researchers. Maurer et al. [4] studied the same model under different cost structures. They used a second order sufficiency test to prove optimality of the computed controls. The dynamics of the model were also studied by Bukhari [5] who used an adaptive control approach. Assuming a system of the tracking type, Bukhari and El-Gohary [6] substituted a tracking error objective function for the profit objective function of Cho et al. [3]. They also used the maximum principle to obtain the optimal production and maintenance rates. Besides production and maintenance, Li [7] incorporated emission tax and pollution R&D investment. Again, the maximum principle was used to derive the optimal production rate, the optimal maintenance rate, and the optimal pollution R&D investment rate.
Our intention in this paper is to further investigate the model of Cho et al. [3] in the following two ways: 1) By generalizing the model; and 2) by applying a different solution technique.
Concerning the model, first we introduce the deterioration of items while in stock. This is a very important topic in inventory theory as shown by the numerous survey papers that have been written on the subject. The field of deteriorating and perishable inventory models has undergone substantial evolution, as detailed in several comprehensive reviews. Bakker et al. [8] and Janssen et al. [9] analyze developments in inventory systems since the early 2000s, focusing on deterioration rates, demand variability, and supply chain coordination. These reviews highlight the importance of incorporating realistic assumptions and the potential for empirical studies to enhance model applicability. Goyal and Giri [10] and Raafat [11] provide foundational insights, mapping the trajectory of inventory models that address perishability and the economic implications of inventory deterioration.
In more recent analyses, Chaudhary et al. [12] and Kaushik [13] examine inventory models tailored for perishable products, emphasizing the role of time-dependent demand, shelf life, and pricing strategies. These reviews underscore the necessity of sophisticated models to reduce waste and optimize inventory levels, suggesting that integrating stochastic elements and customer behavior can further enhance model robustness. Li et al. [14] also contribute by reviewing the various replenishment policies and demand types, offering a comprehensive overview of the deteriorating inventory models and their applications.
Additionally, Karaesmen et al. [15] and Pahl and Voß [16] delve into the management of perishable and aging inventories, discussing the integration of deterioration and lifetime constraints in production and supply chain planning. Their work emphasizes dynamic models that adapt to changing conditions and the potential of big data and machine learning to refine inventory management. Nahmias [17] provides a classic review of perishable inventory theory, setting a foundation for understanding early inventory policies. Sharan and Sayyed [18] conclude with a focus on mathematical approaches to deteriorating inventory models, advocating for optimization algorithms and simulation techniques to advance the field further.
Thus, not only does the process deteriorate over time, but the end product also deteriorates while it is on the shelves. Second, we use a more general cost function of which the objective function of Cho et al. [3] is a special case. Third, we assume that all the exogenous parameters involved (items deterioration rate, obsolescence rate of process performance in the absence of maintenance, and demand rate) are general functions of time.
Concerning the solution method, Cho et al. [3] used an algorithm that was adapted to their control problem. In contrast, we employ the nonlinear model predictive control (NMPC), which seems particularly well-suited to control a dynamic model with nonlinear constraints and an objective function.
We notice that our work treats the model introduced and studied in Cho et al. [3]. We tried to refer to all the relevant works studying the same model such as the ones cited in the introduction. Unfortunately, we found in the literature a small number of works on this subject and we are trying to expand the utility of their model in the hope that it will attract other researchers to work on the same topic.
We will be following the notation of Cho et al. [3] as much as possible. Let θ(t), α(t), and s(t) denote the items deterioration rate at time t, the obsolescence rate of process performance in the absence of maintenance (non-decreasing function of time), and demand rate at time t, respectively. The state of the system is described by the state variables x(t) and p(t) (0≤p(t)≤1), which denote the inventory level at time t and the proportion of good units of end items produced at time t, respectively. Control over the system is achieved through the control variables u(t) and m(t), representing the scheduled production rate at time t and maintenance rate applied at time t, respectively.
Next, we derive the equations that describe the dynamics of the inventory level and the proportion of good units produced over time in the production system in response to production rates, maintenance activities, demand, and deterioration. For the inventory dynamics, we note that the inventory increases at a rate proportional to the product of the proportion of good units produced, p(t), and the scheduled production rate, u(t); and the inventory decreases due to demand, s(t), and the deterioration of items in stock at a rate of θ(t)x(t). Therefore, the change in the inventory level, ˙x(t) is such that:
˙x(t)=p(t)u(t)−s(t)−θ(t)x(t),x(0)=x0. | (2.1) |
For the production quality dynamics, we note that the proportion of good units decreases at a rate dependent on the obsolescence of the production process, α(t), and the maintenance rate, m(t), both of which reduce p(t); and the maintenance efforts, m(t), also contribute positively to the proportion of good units. Therefore, the change in the proportion of good units produced, ˙p(t), is such that:
˙p(t)=−[α(t)+m(t)]p(t)+m(t),p(0)=p0. | (2.2) |
Here, the initial inventory level at time t=0 is given by x0 and the initial proportion of good units at time t=0 is given by p0.
To determine the optimal production and maintenance cost, we follow the same idea as in Cho et al. [3] and minimize the prediction horizon a cost function that is a combination of various cost elements, namely production, inventory, and maintenance. The forms of the cost elements are taken from Cho et al. [3] in nonlinear forms.
The first cost element penalizes the production of defective items, encouraging the system to maintain a high p(t) (i.e., a high proportion of good units). Since p(t) is the proportion of good units produced, 1−p(t) is the proportion of defective units. Multiplying the production rate u(t) by 1−p(t) gives the rate at which defective items are produced. Letting w represent a cost factor that quantifies the cost associated with producing defective items, the cost associated with the production of defective or substandard items during the time interval [t0,t0+T] is given by
∫t0+Tt0[w(1−p(t))u(t)]dt. |
The second cost element penalizes high inventory levels, encouraging the system to avoid excess inventory. Using a quadratic function to account for increasing costs due to higher levels of inventory (e.g., storage, spoilage, or insurance costs), the inventory holding cost during the time interval [t0,t0+T] is given by
∫t0+Tt0[h1x(t)+h2x2(t)]dt, |
where h1,h2>0.
The third cost element balances the cost of production, discouraging both excessive production (due to the quadratic term) and underproduction (which could lead to unmet demand). Using again a quadratic cost to reflect possible increasing marginal costs at higher production rates (e.g., overtime pay, wear and tear on machinery), the production cost during the time interval [t0,t0+T] is given by
∫t0+Tt0[r1u(t)+r2u2(t)]dt, |
where r1,r2>0.
The fourth cost element controls the maintenance activity, balancing the cost of maintenance against the need to maintain a high p(t) by preventing obsolescence (managed by m(t)). Using again a quadratic cost to account for the fact that aggressive maintenance might incur higher costs (e.g., more frequent servicing, downtime), the production cost during the time interval [t0,t0+T] is given by
∫t0+Tt0[c1m(t)+c2m2(t)]dt, |
where c1,c2>0.
Combining these cost elements, we write our objective function as
J:=∫t0+Tt0F(t)dt, | (2.3) |
where
F(t)=w(1−p(t))u(t)+[h1x(t)+h2x2(t)]+[r1u(t)+r2u2(t)]+[c1m(t)+c2m2(t)]. | (2.4) |
By taking into account production quality, inventory costs, production costs, and maintenance costs, our objective is to find the optimal balance between production, inventory, and maintenance activities that minimizes the total cost over the time period of interest.
We are faced with the problem of minimizing the cost function (2.3) subject to the state equations (2.1) and (2.2). We proceed using the trapezoid formula to calculate the integral. For simplicity, we will be writing t instead of t0. Let n be a positive integer and divide the interval [t,t+T] into n sub-intervals of equal length h=Tn. Then, by the trapezoid formula for n intervals, the objective function (2.3) can be approximated as follows:
J≃h2[F(t)+2n−1∑i=1F(t+ih)+F(t+nh)]. | (3.1) |
To calculate the summation in expression (3.1) above, we apply the first-order Taylor approximation to x(t+ih) and p(t+ih) to get:
x(t+ih)≃A1(t,i)+A2(t,i)u(t),p(t+ih)≃C1(t,i)+C2(t,i)m(t), |
where
A1(t,i)=x(t)−ih[s(t)+θ(t)x(t)],A2(t,i)=ihp(t),C1(t,i)=[1−ihα(t)]p(t),C2(t,i)=ih[1−p(t)]. |
Note that taking the square of x(t+ih) yields:
x2(t+ih)≃B1(t,i)+B2(t,i)u(t)+B3(t,i)u2(t), |
where
B1(t,i)=A21(t,i),B2(t,i)=2A1(t,i)A2(t,i),B3(t,i)=A22(t,i). |
Substituting in (2.4), we get:
F(t+ih)=h1A1(t,i)+h2B1(t,i)+[h1A2(t,i)+h2B2(t,i)]u(t)+h2B3(t,i)u2(t)+{w[1−C1(t,i)]+r1}u(t+ih)+r2u2(t+ih)−wC2(t,i)m(t)u(t+ih)+c1m(t+ih)+c2m2(t+ih). |
Let
A1(t):=n−1∑i=1A1(t,i);A2(t):=n−1∑i=1A2(t,i);B1(t):=n−1∑i=1B1(t,i);B2(t):=n−1∑i=1B2(t,i);B3(t):=n−1∑i=1B3(t,i). |
Then,
2n−1∑i=1F(t+ih)≃2h1A1(t)+2h2B1(t)+[2h1A2(t)+2h2B2(t)]u(t)+2h2B3(t)u2(t)+2n−1∑i=1{w[1−C1(t,i)]+r1}u(t+ih)+2r2n−1∑i=1u2(t+ih)−2wm(t)n−1∑i=1C2(t,i)u(t+ih)+2c1n−1∑i=1m(t+ih)+2c2n−1∑i=1m2(t+ih). |
Hence, substituting this expression in (3.1) yields
J≃h2[h1x(t)+h2x2(t)]+h2[h1A1(t,n)+h2B1(t,n)]+hh1A1(t)+hh2B1(t)+h2{w[1−p(t)]+r1+2[h1A2(t)+h2B2(t)]+h1A2(t,n)+h2B2(t,n)}u(t)+hn−1∑i=1{w[1−C1(t,i)]+r1}u(t+ih)+h2c1m(t)+hc1n−1∑i=1m(t+ih)−hwm(t)n−1∑i=1C2(t,i)u(t+ih)+h2[r2+2h2B3(t)+h2B3(t,n)]u2(t)+hr2n−1∑i=1u2(t+ih)+h2c2m2(t)+hc2n−1∑i=1m2(t+ih). |
Therefore, using a matrix notation, we can write:
J≃C(t)+G⊤1(t)U(t)+G⊤2(t)M(t)−M⊤(t)Q12(t)U(t)+U⊤(t)Q1(t)U(t)+M⊤(t)Q2(t)M(t), |
where
C(t):=h2[h1x(t)+h2x2(t)]+hh1A1(t)+hh2B1(t)+h2[h1A1(t,n)+h2A21(t,n)];U(t):=[u(t),u(t+h),u(t+2h),⋯,u(t+(n−1)h)]⊤n×1;M(t):=[m(t),m(t+h),m(t+2h),⋯,m(t+(n−1)h)]⊤n×1;G1(t):=(g(1)i(t))i=0,1,⋯,n−1;G2(t):=(g(2)i(t))i=0,1,⋯,n−1;Q1(t):=diag(q(1)i(t))i=0,1,⋯,n−1;Q2(t):=diag(q(2)i(t))i=0,1,⋯,n−1, |
with
g(1)i(t)={h2{w[1−p(t)]+r1+2[h1A2(t)+h2B2(t)]+h1A2(t,n)+h2B2(t,n)},i=0,h{w[1−C1(t,i)]+r1},1≤i≤n−1; |
g(2)i(t)={h2c1,i=0,hc1,1≤i≤n−1; |
q(1)i(t)={h2{r2+2h2B3(t)+h2B3(t,n)},i=0,hr2,1≤i≤n−1; |
q(2)i(t)={h2c2,i=0,hc2,1≤i≤n−1; |
Q12(t):=[0hwC2(t,1)…hwC2(t,n−1)000⋯00000⋯00]n×n. |
Simple computations permit us to write
A1(t)=[(n−1)−hγ1θ(t)]x(t)−hγ1s(t) with γ1:=n−1∑i=1i=n(n−1)2;B2(t)=2h(γ1−hθ(t)γ2)p(t)x(t)−2γ2h2p(t)s(t) with γ2:=n−1∑i=1i2=n(n−1)(2n−1)6;B3(t)=γ2h2p2(t). |
The unique global maximum of the objective function J is reached at the couple (U∗(t),M∗(t)), which is the solution of the vectorial system of two equations:
G2(t)−Q12(t)U(t)+2Q2(t)M(t)=0, | (3.2) |
G1(t)−Q⊤12(t)M(t)+2Q1(t)U(t)=0. | (3.3) |
From (3.2) we have
U∗(t)=12Q−11(t)Q⊤12(t)M∗(t)−12Q−11(t)G1(t). | (3.4) |
Inserting (3.4) into (3.3), we obtain
M∗(t)=[2Q2(t)−12Q12(t)Q−11(t)Q⊤12(t)]−1[−12Q12(t)Q−11(t)G1(t)−G2(t)]. |
Substituting the value of M∗(t) in (3.4), we obtain the value of U∗(t) as follows
U∗(t)=12Q−11(t)Q⊤12(t)M∗(t)−12Q−11(t)G1(t). |
Based on the principal of Model Predictive Control (MPC), only the first components m∗(t), u∗(t) of M∗(t), U∗(t) are applied in the state equations (2.1) and (2.2), respectively. Then, by solving (2.1) and (2.2) we get the optimal state variables x∗(t) and p∗(t). Therefore, we obtain
m∗(t)=c1r2+whγ1(w+r1)(1−p(t))+w2hp(t)(1−p(t))[hγ2α(t)−γ1]w2γ2h2(1−p(t))2−2c2r2 | (3.5) |
and
u∗(t)=D1+D2p(t)+D3p(t)x(t)+D4p(t)s(t)2r2+D5p2(t), | (3.6) |
with
D1:=−w−r1,D2:=w−hh1(2γ1+n),D3(t):=2n2h2θ(t)−2nh−4hh2(γ1−hγ2θ(t)),D4:=4h2h2γ2+2n2h2,D5:=2h2h2(n2+2γ2). |
Inserting the previous explicit forms of m∗(t) and u∗(t) in the system of differential equations (2.1) and (2.2) yields a system of nonlinear differential equations that can be solved numerically. We summarize, in the following steps of the solution procedure:
Algorithm 1 Solution procedure |
Input: System parameters: x0, p0, T, c1, c2, w, h1, h2, r1, r2, n. |
Input: Auxiliary functions: s(t), α(t), θ(t). |
Step 1: Select the system parameters x0, p0, T, c1, c2, w, h1, h2, r1, r2, n. |
Step 2: Choose the auxiliary functions s(t), α(t), θ(t). |
Step 3: Insert the optimal controls m∗ and u∗ from (3.5) and (3.6) into the differential system (2.1) and (2.2). |
Step 4: Solve the differential system obtained in Step 3 for the state variables. |
Step 5: Substitute the state solutions obtained in Step 4 into (3.5) and (3.6) to compute the optimal control variables. |
Step 6: Calculate the optimal objective function value J∗ by substituting the optimal state and control variables into the objective function (3.1). |
Output: Optimal state and control variables (x∗,p∗,u∗,m∗) and the optimal objective function value J∗. |
Let us take the same data as in reference [3]. Assume that x0=2, p0=0.8, T=1, c1=0.025, c2=0.1, w=1, h1=0.01, h2=1, r1=0.01, r2=4, and n=20. In a first example, we assume that the three known functions s, α, and θ are constant, that is, s(t)=4, α(t)=0.02, and θ(t)=0.01. In this case, the optimal solutions x∗ and p∗ of the system of state differential equations are shown in Figure 1. Also shown in Figure 1 are the optimal control variables u∗ and p∗.
The optimal inventory level x∗(t) consistently diminishes as time progresses. Starting from an initial value of approximately 2, it gradually declines to 0 by the end of the prediction horizon. This indicates that the system exhausts its inventory during production, depending on the produced units to restock. The optimal proportion of good units p∗(t) commences below 0.9 and swiftly rises, nearing a plateau around 0.95. This implies an enhancement in production quality over time, stabilizing close to an upper threshold. The optimal production rate u∗(t) initiates slightly above 0.5 and steadily escalates throughout the prediction period, reaching about 3.5. This mirrors a gradual increase in production activity as the system advances. Finally, the optimal rate of preventive maintenance m∗(t) commences at a notably high level (approximately 20) and swiftly diminishes over time, eventually stabilizing at a low level near 5. This indicates that intensive preventive maintenance is crucial at the beginning of the process to ensure production quality, but the necessity for maintenance decreases as the system stabilizes. The optimal objective function value in this example is J∗=58.1242.
Assume now that the known functions s, α, and θ are not constant and are such that s(t)=0.4t+2, α(t)=0.110+t, and θ(t)=0.01+0.04sin(t). We take the rest of the parameters as follows: x0=1.2, p0=0.8, T=1, c1=0.025, c2=0.1, w=1, h1=0.01, h2=1, r1=0.01, r2=4, and n=20. In this non constant case, the optimal inventory level x∗, the optimal proportion of good units p∗, the optimal production rate u∗, and the optimal preventive maintenance rate m∗ are shown in Figure 2.
The optimal level of inventory x∗(t) shows a consistent decline over time, starting at approximately 1.2 and reaching 0 by the end of the prediction horizon. This implies that as production progresses, the inventory is being used up, and the demand is met with the units produced. The optimal proportion of good units p∗(t) initiates at around 0.8 and experiences a rapid increase, converging towards a value close to 1 by the end of the forecast horizon. This suggests a swift enhancement in production quality, stabilizing at almost perfect quality (nearly 100% good units). The optimal rate of production u∗(t) commences at a relatively low level of about 0.5 and steadily rises over time, reaching approximately 2. This indicates a gradual escalation in production activity as the system progresses towards the optimum output. Finally, the optimal rate of preventive maintenance m∗(t) starts at a high point (around 25) and diminishes rapidly, eventually stabilizing at a lower level close to 5. This signifies that initially, a substantial amount of preventive maintenance is necessary to enhance the production process's quality. However, the requirement for maintenance declines as the system stabilizes over time. The optimal objective function value is J∗=25.4288.
As is well known, conducting sensitivity analysis on system parameters provides valuable insights and aids in informed managerial decision-making. We choose some key parameters and assess their impact on the optimal solution and the optimal objective functional value. The sensitivity analysis is performed in the general case where the known functions s, α, and θ are not constant.
Sensitivity to w. This sensitivity analysis reflects the weight of production quality control (i.e., penalizing the proportion of defective units) on the total cost.
First, we note that the change in the values of w does not affect the optimal inventory level and the optimal production rate. Looking at Figure 3 (top), we observe that the optimal proportion of good units p∗(t) increases continuously as w increases. This suggests that the system seeks to retain a higher proportion of good units to reduce the penalty associated with faulty goods when the cost of quality (expressed by w) is more significant. Also, Figure 3 (bottom) shows that for all values of w, the first preventive maintenance rate m∗(t) spikes dramatically at the beginning, indicating an immediate attempt to increase or maintain the proportion of good units. On the other hand, m∗(t) rapidly decays with time, suggesting that following an initial burst of maintenance actions, the system stabilizes and requires less work.
Sensitivity analysis in Table 1 shows that the optimal objective function value J∗ rises dramatically with an increase in w. For example, with w=0.8, the total cost is relatively low (J∗=18.25), while it increases exponentially to 332.30 when w=1.6. This rapid growth shows the substantial impact that increased focus on product quality has on the overall cost. The cost of maintaining higher-quality products becomes more expensive. This makes intuitive sense because ensuring a higher proportion of good units (through increased maintenance or production adjustments) requires more resources.
w | 0.8 | 0.9 | 1 | 1.1 | 1.2 | 1.3 | 1.4 | 1.5 | 1.6 |
J∗ | 18.25 | 18.87 | 22.90 | 25.92 | 37.20 | 58.60 | 100.98 | 182.15 | 332.30 |
Some important managerial insights can be gained from these experiments. First, in order to effectively manage the production process, quality and cost must be balanced. In proportion to the system's increased emphasis on quality (seen by rising w), overall expenses climb dramatically. Businesses that prioritize excellent quality will see significant cost increases; those that can tolerate a little lower proportion of good units can retain manageable expenses. Beyond a certain point, expenses start to grow sharply, indicating that more quality improvements might not always be worth the money. To prevent unjustified cost rises, managers must carefully consider how much to invest in reaching greater quality levels. Second, initial preventative maintenance is critical in every case, irrespective of the quality level selected. Early maintenance investments contribute to the establishment of a reliable and effective manufacturing process. As continuous maintenance rates level out over time, long-term cost benefits are realized. Thus, even if the initial expenses could be substantial, businesses gain from a proactive approach centered on preventive maintenance since it eventually lowers operating costs and effectively preserves output quality over time.
Sensitivity to p0. This analysis reflects the impact of the initial proportion of favorable items on the optimal policy.
We also note that the change in the values of p0 does not affect the optimal inventory level and the optimal production rate. Figure 4 (top) shows that the system attains a greater initial fraction of good units as p0 rises. It follows that the higher the starting quality level p0, the less work is required to maintain or increase this proportion during the production process. Also, Figure 4 (bottom) shows that the initial preventive maintenance rate m∗(t) is larger as p0 drops. This suggests that greater effort (in the form of maintenance) is needed early on to improve the manufacturing quality if the initial proportion of good units is low. Similarly, the initial maintenance rate is comparatively low for higher p0 values (e.g., 0.95 or 0.9), indicating that fewer corrective actions are required when the system starts at a higher quality level.
Sensitivity analysis in Table 2 demonstrates that when p0 falls, the overall cost J∗ increases. For instance, the cost is J∗=17.07 with p0=0.95, but J∗=104.67 with p0=0.55. This is a notable rise that shows that, because of the greater requirement for corrective and preventive maintenance, starting with a lower initial proportion of good units leads to a significantly higher ultimate cost. A lower initial quality level results in higher maintenance costs as well as higher expenses over time due to manufacturing inefficiencies and errors, as shown by the increase in J∗ with decreasing p0.
p0 | 0.95 | 0.9 | 0.85 | 0.8 | 0.75 | 0.7 | 0.65 | 0.6 | 0.55 |
J∗ | 17.07 | 18.10 | 19.12 | 22.89 | 24.16 | 30.27 | 39.71 | 54.23 | 104, 67 |
Important managerial insights can be gained from these analyses. First, setting a high priority for early quality p0 turns out to be essential for lowering production and maintenance expenses over the long run. Higher p0 systems from the outset require less intervention and continuous maintenance, which reduces overall costs and maximizes resource efficiency. Managers should invest in quality control processes early in the production cycle to avoid costly corrective steps later, as indicated by the significant increase in costs when p0 is low. Because of this, establishing a high-quality baseline before beginning manufacturing is a crucial tactic for cost effectiveness. Second, stabilizing production quality is mostly dependent on preventive maintenance, especially in cases where initial quality is poor. Early maintenance efforts are essential for maintaining stable, long-term operations, regardless of where you start. It is evident from the decrease of the preventive maintenance rate m∗(t) with time that fewer resources are needed to maintain quality once it has stabilized. As a result, early maintenance investments not only raise quality levels right away but also lower the need for maintenance down the road, leading to long-term sustainable production methods. Managers can effectively manage the trade-off between quality and cost by striking a balance between long-term maintenance methods and initial investments in quality.
Sensitivity to x0. This analysis shows how the initial inventory level affects the best strategy.
This time, we note that the change in the values of x0 does not affect the optimal proportion of good units and the optimal maintenance rate. From Figure 5 (top), it can be seen that as production increases, inventory gradually depletes, as shown by the decreasing optimal inventory level x∗(t) over time. Starting with a higher x0, however, enables the system to rely on the initial stock instead of rapidly increasing production, which results in more cost-effective operations. Also, Figure 5 (bottom) shows that the optimal pace of production over time, u∗(t) exhibits an increasing trend, indicating that preventative maintenance efforts are high at first but become less so as the system stabilizes. The stability across several x0 values suggests that the proportion of good units rather than the initial inventory has a greater influence on the production rate.
Sensitivity analysis in Table 3 illustrates that the optimal objective function value J∗ experiences a decline as the initial inventory level x0 rises. For instance, when x0 is set at 0.05, the total cost is J∗=45.27, which decreases to 19.80 when x0 reaches 1.25. This trend indicates that higher initial inventory levels are associated with reduced total costs. The reduction in J∗ implies that commencing with a greater inventory level can lead to lower overall production and maintenance expenses. This phenomenon likely arises from the fact that a higher initial inventory minimizes the need for frequent adjustments in production and maintenance efforts. It is important to note that the decrease in J∗ is not linear in relation to variations in x0. As x0 increases, the incremental reductions in J∗ become progressively smaller. Although enhancing the initial inventory proves advantageous, the benefits tend to diminish as inventory levels rise. This observation suggests the existence of a threshold beyond which additional increases in initial inventory may not yield significant cost reductions, highlighting the importance of balancing inventory investments.
x0 | 0.05 | 0.2 | 0.35 | 0.5 | 0.65 | 0.8 | 0.95 | 1.1 | 1.25 |
J∗ | 45.27 | 36.95 | 33.71 | 30.91 | 28.26 | 25.81 | 23.59 | 21.59 | 19.80 |
This analysis yields several conclusions and insights for managers. To begin, it underscores the crucial significance of maintaining a high initial inventory level x0. Managers should give priority to accumulating a sufficient stock of high-quality units before production commences, as this leads to substantial cost savings over time. The inverse correlation between x0 and total cost J∗ illustrates that early investments in quality inventory can lead to significant savings in both production and maintenance. By starting with a higher proportion of good units, the system requires fewer aggressive interventions, making the process more cost-effective and efficient. The second insight from this analysis is that as production advances, a gradual increase in maintenance efforts is crucial, especially for lower initial inventory levels. However, with a higher starting inventory, the system stabilizes more effectively, enabling managers to implement preventive maintenance more gradually, leading to improved long-term cost optimization. In terms of resource management, the key insight is that early investment in inventory quality, combined with a strategic, dynamically adjusted maintenance plan, enables better control over costs while ensuring production efficiency.
We emphasize the crucial importance of optimal control in optimizing production and maintenance functions in manufacturing systems. We considered a very important model introduced in the work by Cho et al. [3] in which the authors used the maximum principal to solve the system, and we considered a completely different method called (NMPC) to solve a more general model as explained in Section 3. By conducting sensitivity analysis and providing numerical examples, we have illustrated that a larger initial inventory of good units substantially decreases total costs, underscoring the significance of early investments in quality management. The results indicated that preventive maintenance not only stabilizes production processes but also leads to cost efficiency over time, especially when combined with a robust inventory strategy. Furthermore, dynamically adjusting maintenance efforts based on initial inventory levels offers managers valuable insights into resource allocation and cost management. As systems stabilize, a gradual reduction in maintenance efforts can be strategically implemented, ensuring long-term operational efficiency.
Researchers can build upon this work by exploring several avenues. For example, extending the model to include stochastic elements could provide a more realistic representation of production and maintenance processes, enabling a deeper understanding of how these factors interact. Additionally, investigating the impact of external variables, such as technological advancements, could enhance the model's applicability and relevance in dynamic environments. Researchers could also examine the effects of different quality control methodologies, comparing traditional approaches with emerging techniques like machine learning and predictive analytics.
The authors declare they have not used Artificial Intelligence (AI) tools in the creation of this article.
The first author extends his appreciation to Researchers Supporting Project number (RSPD2025R1001), King Saud University, Riyadh, Saudi Arabia.
The authors declare there is no conflicts of interest.
[1] | PlasticsEurope (2015) Plastic-the facts 2015, 1160 Brussels-Belgium, PlasticsEurope. |
[2] | PlasticsEurope (2018) Plastic-the facts 2018, 1040 Brussels-Belgium, PlasticsEurope. |
[3] | Secretariat-General (2018) Communication from the commission of the European parliament, the council, the European economic and social committee and the committee of the regions-A European Strategy for Plastics in a Circular Economy, European Commission. |
[4] | Sexlinger K (2019) Kunststoffe im Boden, Montfortstraße 4, 6900 Bregenz, Institut für Umwelt und Lebensmittelsicherheit (Umweltinstitut). |
[5] |
Lechner A, Keckeis H, Lumesberger-Loisl F, et al. (2014) The Danube so colourful: A potpourri of plastic litter outnumbers fish larvae in Europe"s second largest river. Environ Pollut 188: 177-181. doi: 10.1016/j.envpol.2014.02.006
![]() |
[6] |
Derraik JGB (2002) The pollution of the marine environment by plastic debris: a review. Mar Pollut Bull 44: 842-852. doi: 10.1016/S0025-326X(02)00220-5
![]() |
[7] | A European Strategy for Plastics in a Circular Economy (2018) Brussels, Belgium, European Commission. |
[8] | Bertling J, Hamann L, Bertling R (2018) Kunststoffe in der Umwelt. Fraunhofer UMSICHT. |
[9] | ISO (2019) Plastics-Environmental aspects-Analysis of relevant terms used in the sector and need for standardization, ISO. |
[10] | Liebmann B, Brielmann H, Heinfellner H, et al. (2015) Mikroplastik in der Umwelt Vorkommen, Nachweis und Handlungsbedarf, Wien, Umweltbundesamt. |
[11] | Braun U, Jekel M, Gerdts G, et al. (2018) Microplastics Analytics-Sampling, Preparation and Detection Methods, Bundesministerium für Bildung und Forschung Unterabteilung Nachhaltigkeit; Zukunftsvorsorge. |
[12] | Ibeh CC (2011) Thermoplastic Materials: Properties, Manufacturing Methods, and Applications, CRC Press. |
[13] |
de Souza Machado AA, Kloas W, Zarfl C, et al. (2018) Microplastics as an emerging threat to terrestrial ecosystems. Glob Change Biol 24: 1405-1416. doi: 10.1111/gcb.14020
![]() |
[14] | Circular Economy Package Report: Questions & Answers (2019) Brussels, European Commission. |
[15] | Bundesministerium für Nachhaltigkeit und Tourismus (2020) Fremdstoffe, Mikroplastik und deren Inhaltsstoffe im Boden, Wien, Arbeitsgruppe des Fachbeirates für Bodenfruchtbarkeit und Bodenschutz. |
[16] | Weithmann N, Möller JN, Löder MGJ, et al. (2018) Organic fertilizer as a vehicle for the entry of microplastic into the environment. Sci Adv 4. |
[17] |
Mahon AM, O'Connell B, Healy MG, et al. (2017) Microplastics in Sewage Sludge: Effects of Treatment. Environ Sci Technol 51: 810-818. doi: 10.1021/acs.est.6b04048
![]() |
[18] |
He D, Luo Y, Lu S, et al. (2018) Microplastics in soils: Analytical methods, pollution characteristics and ecological risks. TrAC Trends Anal Chem 109: 163-172. doi: 10.1016/j.trac.2018.10.006
![]() |
[19] |
Bläsing M, Amelung W (2018) Plastics in soil: Analytical methods and possible sources. Sci Total Environ 612: 422-435. doi: 10.1016/j.scitotenv.2017.08.086
![]() |
[20] | Nadherny-Borutin S (2019) Kunststoffrecycling-eine potentielle Mikrokunststoffquelle?, In: University of Natural Sources and Life Sciences, Vienna, Institute of Waste Management (ABF-BOKU) (Ed.), Vom Makro-zum Mikro-Kunststoff-Wo ist die Abfallwirtschaft gefordert?, Muthgasse 18, 1190 Vienna. |
[21] | Eurostat-Population (2019) 2019. Available from: https://ec.europa.eu/eurostat/tgm/table.do?tab=table&init=1&plugin=1&language=de&pcode=tps00001. |
[22] | Biologisch abbaubare Mulchfolien aus nachwachsenden Rohstoffen-Informationen und Verwendungshinweise-(2009) Hannover, Freising. |
[23] | European Commission (2019) European Commission-European Commission, Closing the loop: Commission delivers on Circular Economy Action Plan, 2019. Available from: https://ec.europa.eu/commission/presscorner/detail/en/IP_19_1480. |
[24] | Council Directive 86/278/EEC of 12 June 1986 on the protection of the environment, and in particular of the soil, when sewage sludge is used in agriculture (1986). |
[25] | 40 CFR 503-STANDARDS FOR THE USE OR DISPOSAL OF SEWAGE SLUDGE (2003). |
[26] | Verordnung des Bundesministers für Land-und Forstwirtschaft, Umwelt und Wasserwirtschaft, mit der Bestimmungen zur Durchführung des Düngemittelgesetzes 1994 erlassen werden (2004). |
[27] | Verordnung des Bundesministers für Land-und Forstwirtschaft, Umwelt und Wasserwirtschaft über Qualitätsanforderungen an Komposte aus Abfällen (2001). |
[28] | Verordnung der Landesregierung zur Durchführung des Gesetzes zum Schutz der Bodenqualität (2018). |
[29] | Verordnung des Bundesministers für Land-und Forstwirtschaft über die allgemeine Begrenzung von Abwasseremissionen in Fließgewässer und öffentliche Kanalisationen (1996). |
[30] | Verordnung über das Inverkehrbringen von Düngemitteln, Bodenhilfsstoffen, Kultursubstraten und Pflanzenhilfsmitteln (2012). |
[31] | Verordnung über die Verwertung von Bioabfällen auf landwirtschaftlich, forstwirtschaftlich und gärtnerisch genutzten Böden (2017). |
[32] | Directive 94/62/EC of 20 December 1994 on packaging and packaging waste (1994). |
[33] | EU DIRECTIVE 2019/904 OF THE EUROPEAN PARLIAMENT AND OF THE COUNCIL of 5 June 2019 on the reduction of the impact of certain plastic products on the environment (2019). |
[34] | Nachhaltigkeitsagenda für Getränkeverpackungen 2018-2030-Fortschreibung bis zum Jahr 2030 unterzeichnet (2019) WKO, 2019. Available from: https://www.wko.at/service/netzwerke/Grundsaetze-Nachhaltigkeitsagenda_fuer_Getraenkeverpackunge.html. |
[35] | Madigan MT, Martinko JM, Bender KS, et al. (2014) Brock Biology of Microorganisms, Boston, Pearson. |
[36] |
de Souza Machado AA, Lau CW, Till J, et al. (2018) Impacts of Microplastics on the Soil Biophysical Environment. Environ Sci Technol 52: 9656-9665. doi: 10.1021/acs.est.8b02212
![]() |
[37] | Amlinger F, Peyr S, Geszti J, et al. (2006) Evaluierung der nachhaltig positiven Wirkung von Kompost auf die Fruchtbarkeit und Produktivität von Böden, Lebensministerium. |
[38] |
de Souza Machado AA, Lau CW, Kloas W, et al. (2019) Microplastics Can Change Soil Properties and Affect Plant Performance. Environ Sci Technol 53: 6044-6052. doi: 10.1021/acs.est.9b01339
![]() |
[39] |
Van A, Rochman CM, Flores EM, et al. (2012) Persistent organic pollutants in plastic marine debris found on beaches in San Diego, California. Chemosphere 86: 258-263. doi: 10.1016/j.chemosphere.2011.09.039
![]() |
[40] |
Dierkes G, Lauschke T, Becher S, et al. (2019) Quantification of microplastics in environmental samples via pressurized liquid extraction and pyrolysis-gas chromatography. Anal Bioanal Chem 411: 6959-6968. doi: 10.1007/s00216-019-02066-9
![]() |
[41] |
Liu M, Lu S, Song Y, et al. (2018) Microplastic and mesoplastic pollution in farmland soils in suburbs of Shanghai, China. Environ Pollut 242: 855-862. doi: 10.1016/j.envpol.2018.07.051
![]() |
[42] |
Paul A, Wander L, Becker R, et al. (2019) High-throughput NIR spectroscopic (NIRS) detection of microplastics in soil. Environ Sci Pollut Res 26: 7364-7374. doi: 10.1007/s11356-018-2180-2
![]() |
[43] |
Scheurer M, Bigalke M (2018) Microplastics in Swiss Floodplain Soils. Environ Sci Technol 52: 3591-3598. doi: 10.1021/acs.est.7b06003
![]() |
[44] |
Dümichen E, Eisentraut P, Bannick CG, et al. (2017) Fast identification of microplastics in complex environmental samples by a thermal degradation method. Chemosphere 174: 572-584. doi: 10.1016/j.chemosphere.2017.02.010
![]() |
[45] |
Smidt E, Meissl K, Tintner J (2008) The influence of waste sample preparation on reproducibility of thermal data. Thermochim Acta 468: 55-60. doi: 10.1016/j.tca.2007.11.021
![]() |
[46] | Eckert S (2019) Analyse von Mikroplastikpartikel. |
[47] | Watteau F, Dignac M-F, Bouchard A, et al. (2018) Microplastic Detection in Soil Amended With Municipal Solid Waste Composts as Revealed by Transmission Electronic Microscopy and Pyrolysis/GC/MS. Front Sustain Food Syst 2. |
[48] |
Zhang S, Yang X, Gertsen H, et al. (2018) A simple method for the extraction and identification of light density microplastics from soil. Sci Total Environ 616-617: 1056-1065. doi: 10.1016/j.scitotenv.2017.10.213
![]() |
[49] | Löder MGJ, Gerdts G (2015) Methodology Used for the Detection and Identification of Microplastics-A Critical Appraisal, In: Bergmann M, Gutow L, Klages M (Eds.), Marine Anthropogenic Litter, Cham, Springer International Publishing, 201-227. |
[50] |
Claessens M, Van Cauwenberghe L, Vandegehuchte MB, et al. (2013) New techniques for the detection of microplastics in sediments and field collected organisms. Mar Pollut Bull 70: 227-233. doi: 10.1016/j.marpolbul.2013.03.009
![]() |
[51] |
Hurley RR, Lusher AL, Olsen M, et al. (2018) Validation of a Method for Extracting Microplastics from Complex, Organic-Rich, Environmental Matrices. Environ Sci Technol 52: 7409-7417. doi: 10.1021/acs.est.8b01517
![]() |
1. | Frederick Büks, Martin Kaupenjohann, Global concentrations of microplastics in soils – a review, 2020, 6, 2199-398X, 649, 10.5194/soil-6-649-2020 | |
2. | Anastasiia Sholokhova, Gintaras Denafas, Valeriy Mykhaylenko, The organic output from mechanical–biological treatment plants as a source of microplastics: Mini-review on current knowledge, research methodology and future study perspectives, 2021, 0734-242X, 0734242X2098391, 10.1177/0734242X20983914 | |
3. | Daniela Thomas, Berit Schütze, Wiebke Mareile Heinze, Zacharias Steinmetz, Sample Preparation Techniques for the Analysis of Microplastics in Soil—A Review, 2020, 12, 2071-1050, 9074, 10.3390/su12219074 | |
4. | Valter Castelvetro, Andrea Corti, Alessio Ceccarini, Antonella Petri, Virginia Vinciguerra, Nylon 6 and nylon 6,6 micro- and nanoplastics: A first example of their accurate quantification, along with polyester (PET), in wastewater treatment plant sludges, 2021, 407, 03043894, 124364, 10.1016/j.jhazmat.2020.124364 | |
5. | Qinglan Li, Anrong Zeng, Xin Jiang, Xueyuan Gu, Are microplastics correlated to phthalates in facility agriculture soil?, 2021, 412, 03043894, 125164, 10.1016/j.jhazmat.2021.125164 | |
6. | Yuxin Huo, Feike A. Dijkstra, Malcolm Possell, Balwant Singh, 2022, 175, 9780323989596, 1, 10.1016/bs.agron.2022.05.002 | |
7. | Reza Bakhshoodeh, Rafael M. Santos, Comparative bibliometric trends of microplastics and perfluoroalkyl and polyfluoroalkyl substances: how these hot environmental remediation research topics developed over time , 2022, 12, 2046-2069, 4973, 10.1039/D1RA09344D | |
8. | Angela Barreto, Joana Santos, Lara Almeida, Vítor Tavares, Edgar Pinto, Maria Celeiro, Carmen Garcia-Jares, Vera L. Maria, First approach to assess the effects of nanoplastics on the soil species Folsomia candida: A mixture design with bisphenol A and diphenhydramine, 2023, 29, 24520748, 100450, 10.1016/j.impact.2023.100450 | |
9. | Mehmet Murat Monkul, Hakkı O. Özhan, Microplastic Contamination in Soils: A Review from Geotechnical Engineering View, 2021, 13, 2073-4360, 4129, 10.3390/polym13234129 | |
10. | Zhu Ya-di, Shao Tian-jie, Wang Yan-hua, Wang Rui-yuan, Review and future trends of soil microplastics research: visual analysis based on Citespace, 2022, 34, 2190-4707, 10.1186/s12302-022-00703-2 | |
11. | Tomasz E. Burghardt, Anton Pashkevich, Darko Babić, Harald Mosböck, Dario Babić, Lidia Żakowska, Microplastics and road markings: the role of glass beads and loss estimation, 2022, 102, 13619209, 103123, 10.1016/j.trd.2021.103123 | |
12. | L. Joos, C. De Tender, Soil under stress: The importance of soil life and how it is influenced by (micro)plastic pollution, 2022, 20, 20010370, 1554, 10.1016/j.csbj.2022.03.041 | |
13. | Shuling Zhao, Zhiqin Zhang, Li Chen, Qingliang Cui, Yongxing Cui, Danxia Song, Linchuan Fang, Review on migration, transformation and ecological impacts of microplastics in soil, 2022, 176, 09291393, 104486, 10.1016/j.apsoil.2022.104486 | |
14. | T. I. Kukharchyk, V. D. Chernyk, Soil Pollution with Microplastic in the Impact Area of a Plant Producing Expanded Polystyrene, 2022, 55, 1064-2293, 377, 10.1134/S1064229322030085 | |
15. | Katherine K. Porterfield, Sarah A. Hobson, Deborah A. Neher, Meredith T. Niles, Eric D. Roy, Microplastics in composts, digestates, and food wastes: A review, 2023, 0047-2425, 10.1002/jeq2.20450 | |
16. | Farhad Jazaei, Tareq Jamal Chy, Maryam Salehi, Can Microplastic Pollution Change Soil-Water Dynamics? Results from Controlled Laboratory Experiments, 2022, 14, 2073-4441, 3430, 10.3390/w14213430 | |
17. | Lingzi Mo, Augusto Zanella, Cristian Bolzonella, Andrea Squartini, Guo-Liang Xu, Damien Banas, Mauro Rosatti, Enrico Longo, Massimo Pindo, Giuseppe Concheri, Ines Fritz, Giulia Ranzani, Marco Bellonzi, Marco Campagnolo, Daniele Casarotto, Michele Longo, Vitalyi Linnyk, Lucas Ihlein, Allan James Yeomans, Land Use, Microorganisms, and Soil Organic Carbon: Putting the Pieces Together, 2022, 14, 1424-2818, 638, 10.3390/d14080638 | |
18. | Anastasiia Sholokhova, Justinas Ceponkus, Valdas Sablinskas, Gintaras Denafas, Abundance and characteristics of microplastics in treated organic wastes of Kaunas and Alytus regional waste management centres, Lithuania, 2022, 29, 0944-1344, 20665, 10.1007/s11356-021-17378-6 | |
19. | Anastasiia Sholokhova, Gintaras Denafas, Valeriy Mykhaylenko, Microplastics generation and concentration during mechanical-biological treatment of mixed municipal solid waste, 2022, 214, 00139351, 113815, 10.1016/j.envres.2022.113815 | |
20. | Kellie Boyle, Nimitha Choran, Banu Örmeci, 2023, 9780323999083, 405, 10.1016/B978-0-323-99908-3.00006-3 | |
21. | Minoo Giyahchi, Hamid Moghimi, 2023, 1459, 9780841297012, 33, 10.1021/bk-2023-1459.ch003 | |
22. | Mónica Calero, Mario J. Muñoz, Rafael R. Solís, Emilio J. Lozano, Verónica Godoy, Mª. Ángeles Martín-Lara, 2023, Chapter 3, 978-981-99-0694-9, 37, 10.1007/978-981-99-0695-6_3 | |
23. | D.C.S. Vieira, F. Yunta, D. Baragaño, O. Evrard, T. Reiff, V. Silva, A. de la Torre, C. Zhang, P. Panagos, A. Jones, P. Wojda, Soil pollution in the European Union – An outlook, 2024, 161, 14629011, 103876, 10.1016/j.envsci.2024.103876 | |
24. | Fanny C. D. Berset, Serge Stoll, Microplastic Contamination in Field-Side Composting in Geneva, Switzerland (CH), 2024, 3, 2673-8929, 477, 10.3390/microplastics3030030 | |
25. | Tomasz E. Burghardt, Anton Pashkevich, Road markings and microplastics – A critical literature review, 2023, 119, 13619209, 103740, 10.1016/j.trd.2023.103740 | |
26. | Archana Prajapati, Pradip Sahebrao Jadhao, Ankur Khare, Atul Narayan Vaidya, Asirvatham Ramesh Kumar, Sera Das, Characterization of Microplastics and Associated Heavy Metals in Urban Soils Affected by Anthropogenic Littering: Distribution, Spatial Variation, and Influence of Soil Properties, 2024, 33, 1532-0383, 1018, 10.1080/15320383.2023.2286524 | |
27. | Mohammed matouq, Microplastics in Wastewater and Environmental Aspects, 2024, 6, 2617-720X, 11, 10.48103/jjeci732024 | |
28. | Farmaan Mushtak, Jai Prakash, Surjit Singh Katoch, Microplastics in complex soil matrix: Recovery, identification and removal using micro nano techniques, 2024, 22, 25900072, 100237, 10.1016/j.mne.2023.100237 | |
29. | Abhishek Kumar, Kirpa Ram, Microplastics in Different Environmental Matrices: Co-Contaminants and its Monitoring Techniques, 2024, 235, 0049-6979, 10.1007/s11270-024-07469-8 | |
30. | Kanika Dogra, Manish Kumar, Kanchan Deoli Bahukhandi, Jian Zang, Traversing the prevalence of microplastics in soil-agro ecosystems: Origin, occurrence, and pollutants synergies, 2024, 266, 01697722, 104398, 10.1016/j.jconhyd.2024.104398 | |
31. | Piyush Pandey, Avinash Pratap Gupta, 2024, Chapter 15, 978-3-031-64252-4, 293, 10.1007/978-3-031-64253-1_15 | |
32. | Agnieszka Medyńska-Juraszek, Anna Szczepańska, Microplastic Pollution in EU Farmland Soils: Preliminary Findings from Agricultural Soils (Southwestern Poland), 2023, 13, 2077-0472, 1733, 10.3390/agriculture13091733 | |
33. | Wenshan Shi, Nan Wu, Zengli Zhang, Yuting Liu, Jingsi Chen, Jiafu Li, A global review on the abundance and threats of microplastics in soils to terrestrial ecosystem and human health, 2024, 912, 00489697, 169469, 10.1016/j.scitotenv.2023.169469 | |
34. | Nicolas Estoppey, Gabriela Castro, Gøril Aasen Slinde, Caroline Berge Hansen, Mari Engvig Løseth, Katinka Muri Krahn, Viona Demmer, Jørgen Svenni, Teresa-Van-Anh Thi Tran, Alexandros G. Asimakopoulos, Hans Peter H. Arp, Gerard Cornelissen, Exposure assessment of plastics, phthalate plasticizers and their transformation products in diverse bio-based fertilizers, 2024, 918, 00489697, 170501, 10.1016/j.scitotenv.2024.170501 | |
35. | Ursula Nopp-Mayr, Sarah Layendecker, Marcia Sittenthaler, Matthias Philipp, Ralf Kägi, Irene Weinberger, Microplastic loads in Eurasian otter (Lutra lutra) feces—targeting a standardized protocol and first results from an alpine stream, the River Inn, 2024, 196, 0167-6369, 10.1007/s10661-024-12791-z | |
36. | Arunagiri Ragu Prasath, Chinnappan Sudhakar, Kandasamy Selvam, Microplastics in the environment: Types, sources, and impact on human and aquatic systems, 2025, 2589014X, 102055, 10.1016/j.biteb.2025.102055 | |
37. | Alireza Bakhshaee, Peyman Babakhani, Muhammad Masood Ashiq, Kati Bell, Maryam Salehi, Farhad Jazaei, Potential impacts of microplastic pollution on soil–water–plant dynamics, 2025, 15, 2045-2322, 10.1038/s41598-025-93668-0 | |
38. | Claudia Augusta Aguiar Machado Gemba, Eloana Janice Bonfleur, Deise Paludo, Vander Freitas Melo, Microplastics in Soils with Contrasting Organic Carbon Content and Clay Fraction Mineralogy: Effects on Copper and Phosphorus Adsorption, 2025, 1532-0383, 1, 10.1080/15320383.2025.2484590 |
w | 0.8 | 0.9 | 1 | 1.1 | 1.2 | 1.3 | 1.4 | 1.5 | 1.6 |
J∗ | 18.25 | 18.87 | 22.90 | 25.92 | 37.20 | 58.60 | 100.98 | 182.15 | 332.30 |
p0 | 0.95 | 0.9 | 0.85 | 0.8 | 0.75 | 0.7 | 0.65 | 0.6 | 0.55 |
J∗ | 17.07 | 18.10 | 19.12 | 22.89 | 24.16 | 30.27 | 39.71 | 54.23 | 104, 67 |
x0 | 0.05 | 0.2 | 0.35 | 0.5 | 0.65 | 0.8 | 0.95 | 1.1 | 1.25 |
J∗ | 45.27 | 36.95 | 33.71 | 30.91 | 28.26 | 25.81 | 23.59 | 21.59 | 19.80 |