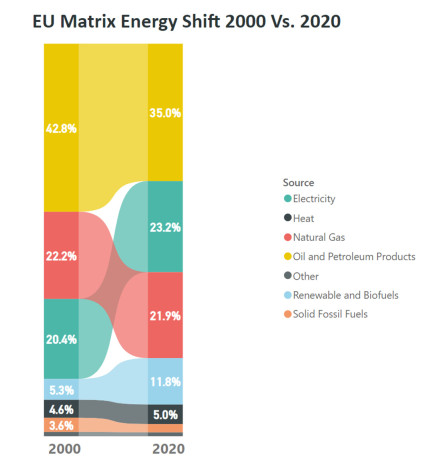
Nowadays, the increased demand for energy and electrification associated with higher production costs from renewable and cleaner sources has driven up prices, impacting the industrial, commercial, and residential sectors. With a direct influence on the development of these economic sectors, its direct and indirect impacts to products and services have become important to find more efficient ways and best practices on energy use to support sustainable development. Aiming to shed light on this topic, and how individuals and society behave in this energy market transformation, this article explores opportunities for reducing electricity consumption through the use of modern technologies, such as of monitoring, optimization, automation, and adjustment of routines. At the same time, it is also our intention to bring to the surface a discussion around the rational use of everyday resources and raising the awareness of its impact to individuals and institutions. At its core, this work consists of continuous data collection of single devices and equipment in regard to status, energy consumption, and other relevant data of a typical household. Through behavioral changes and introduction of smart home automation techniques, it was possible to trace a parallel comparison between different scenarios and their influence on the energy consumption without negative impact to the comfort of individuals. Seeking a continuous improvement approach, extensive iterations were conducted, and it was possible to notice not only an energy efficiency improvement, but at the same time gains in living standards and safety. The significant results observed over subsequent months and years highlight not only practical and financial benefits, but also increased awareness and behavioral changes toward the rational use of electricity in households.
Citation: Gunnar Lima, Andreas Nascimento, Marcelo P. Oliveira, Fagner L. G. Dias. Energy efficiency analysis: A household digital transformation[J]. AIMS Energy, 2024, 12(4): 774-808. doi: 10.3934/energy.2024037
[1] | Aurora Martínez-Corral, Javier Cárcel-Carrasco, Jangveer Kaur, Fabiola Colmenero Fonseca . Analysis of thermal insulation in social housing in Spain (1939–1989) and its possible adaptation to the Sustainable Development Goals (SDGs). AIMS Energy, 2022, 10(6): 1190-1215. doi: 10.3934/energy.2022056 |
[2] | HVV Priyadarshana, MA Kalhan Sandaru, KTMU Hemapala, WDAS Wijayapala . A review on Multi-Agent system based energy management systems for micro grids. AIMS Energy, 2019, 7(6): 924-943. doi: 10.3934/energy.2019.6.924 |
[3] | Chiara D’Alpaos, Michele Moretto . Do smart grid innovations affect real estate market values?. AIMS Energy, 2019, 7(2): 141-150. doi: 10.3934/energy.2019.2.141 |
[4] | Hamza El Hafdaoui, Ahmed Khallaayoun, Kamar Ouazzani . Activity and efficiency of the building sector in Morocco: A review of status and measures in Ifrane. AIMS Energy, 2023, 11(3): 454-485. doi: 10.3934/energy.2023024 |
[5] | Dalong Guo, Chi Zhou . Potential performance analysis and future trend prediction of electric vehicle with V2G/V2H/V2B capability. AIMS Energy, 2016, 4(2): 331-346. doi: 10.3934/energy.2016.2.331 |
[6] | Bei Li, Siddharth Gangadhar, Pramode Verma, Samuel Cheng . Maximize Producer Rewards in Distributed Windmill Environments: A Q-Learning Approach. AIMS Energy, 2015, 3(1): 162-172. doi: 10.3934/energy.2015.1.162 |
[7] | Kangli Xiang, Keren Chen, Simin Chen, Wanqing Chen, Jinyu Chen . Model for sustainable carbon emission reduction energy development and smart grid technology strategy. AIMS Energy, 2024, 12(6): 1206-1224. doi: 10.3934/energy.2024055 |
[8] | Syed Sabir Hussain Rizvi, Krishna Teerth Chaturvedi, Mohan Lal Kolhe . A review on peak shaving techniques for smart grids. AIMS Energy, 2023, 11(4): 723-752. doi: 10.3934/energy.2023036 |
[9] | Lilias Makashini, Austine Ng'ombe, Henry Abanda, Albert Malama, Priscilla Mudenda . Household lifestyle, energy related practices and perceptions of energy efficiency: Evidence from Kitwe, Zambia. AIMS Energy, 2014, 2(3): 276-294. doi: 10.3934/energy.2014.3.276 |
[10] | Amine Ben Alaya, Charfeddine Mrad, Férid Kourda . Piezoelectric energy harvesting under free and forced vibrations for different operating conditions. AIMS Energy, 2024, 12(6): 1334-1365. doi: 10.3934/energy.2024060 |
Nowadays, the increased demand for energy and electrification associated with higher production costs from renewable and cleaner sources has driven up prices, impacting the industrial, commercial, and residential sectors. With a direct influence on the development of these economic sectors, its direct and indirect impacts to products and services have become important to find more efficient ways and best practices on energy use to support sustainable development. Aiming to shed light on this topic, and how individuals and society behave in this energy market transformation, this article explores opportunities for reducing electricity consumption through the use of modern technologies, such as of monitoring, optimization, automation, and adjustment of routines. At the same time, it is also our intention to bring to the surface a discussion around the rational use of everyday resources and raising the awareness of its impact to individuals and institutions. At its core, this work consists of continuous data collection of single devices and equipment in regard to status, energy consumption, and other relevant data of a typical household. Through behavioral changes and introduction of smart home automation techniques, it was possible to trace a parallel comparison between different scenarios and their influence on the energy consumption without negative impact to the comfort of individuals. Seeking a continuous improvement approach, extensive iterations were conducted, and it was possible to notice not only an energy efficiency improvement, but at the same time gains in living standards and safety. The significant results observed over subsequent months and years highlight not only practical and financial benefits, but also increased awareness and behavioral changes toward the rational use of electricity in households.
The world has been witnessing continuous growth in electrical consumption, which presents increasing challenges as the use of fossil fuels is progressively reduced and restricted. Growing concerns about gas emission rates and their effects on the climate in recent decades have prompted a transformation in energy sources globally.
According to a report concerning energy consumption and efficiency in Europe published by JRC [1], there has been a considerable reduction in fossil fuel shares in the world's energy matrix. Oil and petroleum product use dropped nearly 8% over 20 years from 2000 to 2020. Conversely, natural gas exhibited a less significant reduction, as shown in Figure 1.
Simultaneously, Figure 1 also shows an almost 3% increase in the share of electricity, promoting it to the second largest source of energy in Europe. This places electricity as the main source in energy decarbonization transformation efforts. That trend is also confirmed by the projections in IRENA's report [2], which forecasts a share of 29% by 2030 and up to 50% by 2050, driven largely by the strong electrification of the transportation market, which is also expected to grow 36 times by 2030, according to IRENA.
Another positive trend seen in Figure 1, is the doubling of renewable and biofuel usage over 20 years. Although falling short of European environmental agencies' expectations, these values show a clear trend towards to cleaner energy sources. According to IRENA, around 28% of global electricity production in recent years has come from renewable source, with projections estimating this share to reach 68% by 2030 and 91% by 2050.
In a more recent context, the geopolitical situation following the start of the Ukraine war has forced governments, especially in Europe, to review their high dependency on resources such as oil and gas, which have historically provided a great portion of Europe's energy. The restrictions and economic sanctions imposed on Russia, along with the terrorist attack on the Nord Stream pipeline in September of 2022 [3], which supplies a significant portion of Germany's gas, has forced significant changes in this landscape. Precise numbers and the overall impact of these market changes are yet to be published, although it is expected that such events will have long-term effects on the energy market, both in Europe and globally, as indicated by BP in its annual report in July of 2023 [4]. Despite the negative financial implications worldwide, this opens an opportunity to accelerate the transformation as new suppliers and solutions are being considered to address the energy crises seen in recent months.
During the years between 2020 and 2022, there was a slight decrease in the energy demands for a short period, primarily due to COVID19 and its impacts on the transportation sector. Despite this and other factors previously mentioned, there is a clear consensus on the increasing demand for electrical energy in the coming years and decades. These trends have been intensified by the transportation sector, with the increasing popularity of electric vehicles (EVs), which today represents 9% of the world's total car sales according to [5], around 10.5 million cars on the street according to IRENA [2], and with ambitious targets for the future as mentioned previously. Another important contributing factor to this demand increase is the rapid digital transformation of other sectors of the industry and economy, such as datacenters, cryptocurrencies, e-commerce, etc. Taking cryptocurrencies as an example, Bitcoin mining alone today consumes 127 terawatts, more than entire small countries such as Norway or Sweden [6].
In Figure 2, is possible to verify the world electricity generation projection for the following decades. An impressive estimation of a 100% increase in 30 years with an estimate of 14,700 gigawatts per year by 2050 [7].
The constant search for renewable energy sources is a response to the continuously growing demand for additional energy sources with low environmental impact. With an increase in demand linked to a higher production cost than traditional sources, the world has seen a constant rise in energy prices, which affects all sectors of the economy [8,9].
Another pivotal factor contributing to this scenario is the transformation catalyzed by the energy market within the global economy, particularly within the European Union. Despite the undeniable increase in the adoption of renewable sources over the years, which has notably reduced implementation costs, short-term fluctuations in electricity prices underscore the need for intelligent consumption management strategies. According to the International Renewable Energy Agency report [10], the levelized cost of electricity (LCOE) for utility-scale photovoltaic (PV) solar power plants witnessed a remarkable 85% decrease between 2010 and 2020, dropping from $0.381/kilowatt-hour (kWh) to $0.057/kWh in 2020. During the same period, the LCOE reductions were 9% for offshore wind, 13% for onshore wind, and an impressive 16% for concentrated solar power (CSP) [10].
This article focusses primarily on the usage and cost dynamics within the European electricity market, which has been at the forefront of the global transformation and expansion towards renewable energy sources. Furthermore, Europe has been experiencing a significant surge in energy prices due to low availability resulting from the conflict in Ukraine, further highlighting the importance of studies like the one presented here.
The impact of energy prices extends across various sectors of the economy. A study published in Eurostat [11] examined the profound changes brought about by the energy transition, highlighting the need for broad social and political support. The report assessed the importance and impact of energy costs on the economy, businesses, and households.
Energy prices play a crucial role in the industrial sector as it is energy-intensive and heavily relies on electricity and fuels for its operations. For medium-sized companies in Europe, energy costs represent a small percentage of production costs (0–3%). However, they are more significant for industrial sectors with higher energy intensity, ranging between 3% and 20%, and can reach elevated levels in certain industrial subsectors (e.g., 40% in primary aluminum, 31% in zinc, 28% in primary aluminum, 25% in flat glass, 71% in fertilizers, and 20% in steel in electric furnaces).
The services sector is an essential part of the European economy, encompassing a wide range of activities from financial and commercial services to tourism and hospitality. Although direct energy consumption in services may be low compared to other sectors, energy prices still have a significant impact due to heating, cooling, lighting, and IT operation's needs. Additionally, higher energy prices can affect the competitiveness of businesses in the services sector, leading to a reduction in demand for services and potentially resulting in job cuts and investment reductions.
In Table 1, extracted from the Eurostat report [11] is possible to see the energy share of the production by sector.
Examples of sectors | Energy share of production costs (indicative range) |
Average European business | 0–3% |
Trade, computers, motor vehicles, electrical equipment, pharmaceutics, construction | 0.4–1% |
Waste management, plastics, textiles, grain | 2–4% |
Accommodation and restaurants | 3–5% |
Energy intensive sectors in manufacturing: Cement, clay building materials, pulp and paper, glass, iron and steel, basic chemicals, non-ferrous metals, refineries | 3–20% |
Air transport, land transport | 20–30% |
Data centers | 10–15% |
Mining of metals and others, electricity, and gas | 10–20% |
In addition to the manufacturing industry, energy procurement can also be a significant source of costs in certain sectors that provide transportation services, data processing, hospitality, and restaurants. According to an article published by Milewska and Milewski [12], energy consumption and its associated costs represent 4.1% of the total revenue of the manufacturing industry. However, there are five industries (chemical, metallurgy, petroleum, paper, and construction auxiliaries) that are more energy-dependent, ranging from 7.2% (chemical) to 13.6% (construction auxiliary). The authors concluded that these are the most vulnerable sectors and have been under greater pressure to increase the selling prices of their products. Additionally, energy consumption accounts for 15% of the costs for producers of food and beverages.
Figure 3 illustrates the increase in the average price of kWh in Europe in recent years, with a remarkable 85% rise between 2008 and 2022.
Similar to the industrial sector, residential use has been heavily impacted by this price increase. This has triggered constant discussions, both commercial and political, on ways to mitigate its impacts on societies across Europe and beyond. This impact has even a greater impact on the livelihood of low-income families already living on tight budgets, as discussed by Scheier [13] in his recent publication on strategies for lifting energy access disparities among families.
It is worth noting that, while some European countries have agencies regulating energy contracts and prices, electricity is essentially treated as a commodity, having its price determined by the stock market. Thus, the price per kWh can have changed in accordance with the supply and demand balancing, varying throughout the years and months. In some cases, like in the Nordic countries [14], these fluctuations can occur on the span of days or even hours. In Figure 4, it is possible to see how such fluctuation can impact end consumers and the prices they pay depending on the time of day.
Consequently, consumers who chose to charge their car or operate high-power demand equipment during peak periods may face unpleasant surprises when receiving expensive bills for such activities. It is also curious to notice that, in some short moments, the kWh can reach negative values, becoming excellent opportunities for these activities. However, the challenge is to identify this precise moment to be able to take advantage of it.
There is a tendency in Europe to migrate to markets like these, especially with the decentralization and increased private electricity production seen in recent years. Governments have been investing heavily through tax incentives and loans for companies and householders to pursue renewable and clean electricity production, such as solar panels, windmills, geothermic, etc. As consequence, it requires from the government the ability to also create the means to absorb the excess production and bring it back into the grid, both technically and commercially. All of this contributes to creating a more dynamic electrical market, where decisions about when to consume electricity can be crucial on the span of hours.
With all these perspectives, the need of having the right information to support consumers to make the right decisions and engage in best practices on the use of electricity becomes clear.
Just in the residential sector, electricity consumption can make up 30% of the overall European consumption, as displayed in Figure 5.
When it comes to the cost of electricity in a household, it is directly proportional to its consumption. It is worth noting that electricity prices can vary significantly from region to region, especially in certain European countries [9]. Nevertheless, one possible solution to help families and companies reduce their electricity costs is by decreasing overall consumption. The answer does not necessarily mean cutting or eliminating items or services, but rather focusing on enhancing their efficiency in an attempt to avoid waste through rational and mindful use.
An average household's electricity consumption is divided among fundamental categories, such as water heaters and/or showers, air conditioning and/or heating, refrigerators, lights, TVs, radio sets, electric ovens, microwaves, washing machines, irons, computers, and electronics in general, among others. Figure 6 provides an overview of the distribution of the total electricity consumption in a typical European household [15].
With numerous areas and electrical appliances within a home, there are ample opportunities for optimization, ranging from simple habits and configuration changes, to more complex investments in new and more energy-efficient equipment that can reduce consumption even further.
There are numerous strategies to seek better sustainable solutions, such as the use of low-carbon technologies. In the work by Shang and Lv [16], three main aspects of Carbon Neutrality through investigation were presented in a comprehensive discussion: The new energy-supported Low-Carbon Technologies (LCTs), building-oriented Energy Conservation and Emission Reduction (ECER), and Carbon Capture, Storage, and Utilization (CCSU) technology. According to the authors, energy consumption in buildings is especially a major carbon emitter and poses a challenge for Sustainable Urban Development (SUD), which reinforces initiatives proposed in this work.
According to the United States Energy Information Administration [17], the projection for residential electricity consumption is expected to increase by 14% to 22% by 2050. On the other hand, the largest electricity consumer in Europe has much more ambitious plans. According to Walter Kahlenborn [18], the German government has plans to reduce consumption by 20% by 2020, eventually reaching 50% by 2050. As described in his guide, Walter Kahlenborn emphasizes the inevitable need to achieve high levels of electrical efficiency, which is also reinforced and highlighted by Francesco La Camera, director of IRENA [2]. In their annual report, IRENA also outlines the targets and financial investments required to achieve these goals worldwide. These initiatives not only address regulatory and financial incentives to companies and individuals, but also includes extensive information and training campaigns around the topic. It is estimated that by 2030, the market will achieve yearly improvements of 3.3% in electricity intensity factors, along with $1780 Billion increase in investments per year [2].
As estimated by Walter Kahlenborn in his work for the German government [18], these reductions could amount to a 25% decrease in energy consumption, as originally estimated by Lackner [19] and shown in Figure 7. Most of them (15%) are considered simple activities with no need for additional investments in products and/or services.
One way to demonstrate the price decomposition and its proportionality to consumption can be described by the simplified Eq 1:
TotalCost=(Consumption×PriceperkWh)+Fees+Taxes | (1) |
Fees and taxes are variables dependent on government policies and are beyond consumers' influence, who usually have none or little to say about it. In some countries, the kWh price is a combination between energy and infrastructure associated with the distribution, transformation, and transmission of electricity to the end customer.
Regarding energy management, we can consider the use of a well-known methodology called PDCA (Plan, Do, Check, Act), where Walter Kahlenborn recommends employing a cycle of continuous improvement for companies and organizations. This work has simplified the model of energy management to apply it to a household and achieve meaningful results, as suggested by Figure 7.
From the technology enablement perspective, in their research [20], Lv advocates for the broader adoption and expansion of Digital Twins (DTs) in Smart Cities (SCs). They conducted a comprehensive review of the impact of DTs and digitization on urban areas, aiming to assess city progression and standardize management practices. A DT-enabled city represents an innovative integration of various technologies, including perception, transmission, computing, modeling, and simulation.
Aligned with these goals, leveraging digital representation technologies holds promise for enhancing efficiency in managing electricity consumption in residences, thereby providing homeowners with a potent decision-making tool. Furthermore, the incorporation of Artificial Intelligence (AI) offers opportunities for further expansion, thereby magnifying the effects and positive impacts of these technologies, as also highlighted by Rojek in his recent article [21].
The overall objective of this project is to establish the average energy distribution in an ordinary house and to demonstrate methods and improvements to be made in order to guide householders to optimize its electricity use.
Additionally, this project aims to:
● Measure the overall consumption of a household.
● Measure individual electrical/electronic devices' energy consumption.
● Evaluate the energy efficiency of different electrical appliances.
● Develop routines, automations, and methods for energy optimization.
● Validate costs associated with consumption.
● Compare energy usage before and after improvements.
As for future goals, there is a potential to explore a business plan for selling a product and/or service for continuous monitoring of energy consumption in households and/or commercial establishments.
To conduct the experiments, as well as to achieve the objectives outlined in this project, a series of intelligent devices were used along with a list of common appliances and equipment found in most households.
For the energy analysis and optimization of a house, cutting edge devices with IoT functions were used. They enabled measuring and controlling the different equipment remotely and efficiently, being used in routines that aim to improve comfort and the rational use of energy.
The case study involved a typical 60 m2 apartment comprised of a living room, kitchen, two bedrooms, one bathroom, and hallway as shown in the blueprint in Figure 8. Various electrical appliances and devices, such as refrigerators, freezers, water heaters, televisions, lamps, computers, electric ovens, routers, radios, fans, and others, were included.
The Shelly Energy Monitoring PM and EM series, shown in Figure 9 [22], were utilized as a smart meter and relay with many functions crucial to this work:
● Remote control via Wi-Fi
● On/Off Actuators
● Continuous consumption meters; instantaneous and per period
● Cloud Support through standard APIs
● MQTT Support
● Routines and Scheduling tasks via software
● Advanced configurations
Philips Hue Lights, shown in Figure 10 [23], were installed as a set of smart bulbs with several key functions:
● LED lights with low consumption (compared to traditional bulbs)
● Remote control via Wi-Fi
● On/Off Actuators and Dimming
● Support to routines and scheduling of tasks via software
Both the Philips Hue sensor shown in
Figure 11 [23] and the Aqara Sensor shown in Figure 12 [24] were utilized as smart motion and presence sensor, offering functions such as:
● Remote control via Wi-Fi
● Motion Detection
● Presence and location detection (Aqara only)
● Sensitivity control
● Light intensity detection (LUX)
● Measurement of room temperature
● Routines and Scheduling of tasks via software
With the adoption of the Shelly ecosystem, it was possible to also include the additional sensors shown in Figure 13 [22] to monitor multiple temperature locations. The MQTT support and facilitate real-time comparisons between power consumption and temperature variations for boilers and refrigerators.
For establishing an MQTT broker, an SBC (single board computer) from the company Raspberry Pi [25] was utilized. The model Raspberry Pi 3B (Figure 14) was selected as it offers high flexibility, low energy consumption, low investment cost, and a large IoT community. It was used for installing both the MQTT Broker Mosquitto and Node-Red automation flows described in Section 2.7.
● Shelly App: application developed and maintained by Shelly [26]. Allows for configuration, remote control, and access of Shelly's meters and sensors.
● Hue App: Application developed and maintained by Philips [23]. Allows for configuration, remote control, and access of Philips Hue lights and sensors.
● Aqara App: Application developed and maintained by Aqara [24]. Allows for configuration, remote control, and access of Aqara presence sensors.
● Microsoft Power BI: Application developed and maintained by Microsoft [27]. Allows for creation/visualization/optimization and remote access of reports.
● Microsoft Azure: Cloud Platform provided by Microsoft [27]. Allows for collection, transformation, and storage of data and information from the energy meters in the cloud.
● Node-Red: Application developed and maintained by OpenJS [28]. Allows for advanced automation flows. Supports several types of protocols such as: MQTT, Https, JSON, APIS, etc.
● Eclipse Mosquitto: Application developed and maintained by Eclipse foundation [29] Used as an MQTT Broker for managing network device messages.
During the planning phase, activities for software development, installation of devices, sketching routines, and automation flows followed the 6Ws methodology: Who, What, When, Where, Why, and How [30], a problem-solving methodology widely used by the industry and companies worldwide that adopt Lean Six Sigma principles [31].
The main driving factors for selecting such methodology during the planning phase were due to contain the following advantages:
● Fast, simple, and comprehensive
● Flexible
● Structured thinking
● Problem definition and breakdown
The energy management development phase, which involved monitoring and enhancing energy efficiency, was supported by the well-known PDCA methodology (Plan, Do, Check, Act), also suggested by Walter Kahlenborn in his guide [18]. Combined with an Agile approach, it made an excellent blend of classic and leading-edge methodologies to support the work. As a result, an information model was created and represented by the list below, and illustrated in Figures 15 and 16:
● Plan-Data collection: In this phase, the emphasis is on gathering relevant data related to energy consumption, production, and usage patterns. This includes collecting data from various sources such as energy meters, sensors, and historical records. The goal is to establish a comprehensive understanding of the current energy landscape within the house. Data collection is streamlined through two distinct protocols. First, the Cloud API facilitates remote access and control via the internet (off-site access), avoiding the necessity for physical or direct access to the devices. Second, MQTT enables local real-time telemetry management, adeptly handling thousands of messages per minute and generating a substantial dataset for comprehensive analysis.
● Do-Data transformation, information: Once the data is collected, it undergoes transformation processes to convert raw data into actionable information. This involves tasks such as data cleaning, normalization, and integration to ensure accuracy and consistency. The process focuses on extracting relevant information from the extensive dataset, emphasizing value changes to reduce model size and storage while enhancing application performance. The transformed data is then utilized to derive valuable insights into energy usage trends, inefficiencies, and potential areas for improvement.
● Check-Information Analysis: In this phase, the focus shifts to analyzing the transformed data to identify patterns, anomalies, and opportunities for optimization. Various analytical techniques such as statistical analysis and predictive modeling may be employed to uncover insights and correlations within the data. The goal is to gain a deeper understanding of energy consumption patterns and to pinpoint areas where energy efficiency can be enhanced.
● Act-Optimization: Drawing from insights gleaned through data analysis, actionable steps are taken to optimize energy usage and improve efficiency. This may encompass implementing energy-saving measures, upgrading equipment, adjusting operational processes, or integrating renewable energy sources. Additionally, consideration can be given to incorporating alarms and flaw detection points, enhancing convenience and security for homeowners. The optimization process is iterative, with ongoing monitoring and evaluation to ensure that implemented changes are effective and sustainable.
As an essential part of the development of this work, an IT solution outline was required to be created. To achieve this, a set of architectural principles was defined to guide rules to steer the solution towards the objective. These principles were:
● Minimize human interventions as a manual action.
● Remote and continuous access 24/7.
● "Cloud First" approach.
o Enable easy scaling.
o Reduce upfront investments.
o Reliable cybersecurity
o Access and Identity Management (AIM)
o Trustworthy Backup and RTOs (Return to Operations)
● Easy to integrate: Standard APIs and protocols.
● Scalable for other customers/users.
● Support for DevOps and documentation.
Achieving any good development always starts by having a good plan. As a fundamental part of the continuous improvement cycle, the planning phase has been constantly enhanced through multiple interactions. Initially, a brainstorming session following the 6Ws methodology described in Section 2.8 was conducted.
As proposed by Simon Sinek in his book "Start with the why" [32], the "why" is the core of the problem we are trying to resolve. The "why" gives the purpose and justification for this work and provides direction to the project and all subsequent activities. The outcome of that exercise is shown in Figure 17 and is listed in Table 2.
W's | Findings |
Why? | ● Help Consumers reduce electrical consumption and costs. ● Reduce Environment impact. ● Reinforce good habits and behaviors. ● Grow electrical use awareness. Improve living quality and safety. |
How? | ● By creating an efficient Energy management system o Measuring continuously consumption per equipment o Measuring continuously total consumption ● By deploying smart devices: Plugs, lights, and sensors o Dimmable LED lights o Plugs and switches with remote on/off. o Wireless sensors: flexible positioning ● By setting a fit-to-purpose IT architecture o Solid and reliable Databases. o Comprehensive and easy to use data reports. o IoT infrastructure o Realtime monitoring system. ● Process oriented approach o Powerful workflows o Well-designed Routines o Customizable Automations |
What? | ● Energy management system ● Energy optimization strategies ● Alarms and notification |
Who? | ● Householders ● Energy companies ● Governments and institutions |
Where? | ● Houses ● Possible commercial applications |
When? | ● Short and long terms ● Minimum 1 year of data collection ● Continuous services ● Started in 2019 |
The energy meters were electrically installed in power sockets and power strips for each individual measuring device, and one at the central electrical board for the total consumption. All devices were wired following the supplier schematics, as represented in Figure 18.
Once properly wired, installed, and configured via the Shelly App, the plugs (represented in Red in Figure 19) were located throughout the residence, ready to monitor and control the equipment consumption. Each Shelly device was installed interfacing the wall socket and the equipment.
The smart Philips Hue lights (represented in Yellow in Figure 19) were also distributed throughout the rooms and classified into Direct and Indirect lights. This configuration allowed the creation of scenarios and more suitable setups to enhance comfort and user experience.
The Philips and Aqara sensors (represented in Green in Figure 19) were strategically placed to better cover the presence and movements in rooms. This was only possible due to the wireless capability, opening new possibilities for behavioral optimization, as will be discussed in Section 3.5.
In Figure 19, all devices and their precise locations in the apartment are shown.
Considering the architectural principles described in the previous section 2.9-IT Architectural Principles, as well as the project requirements, the cloud platform selected was Microsoft Azure [27]. Inside the Azure platform, the elements and resources were selected, and the solution outline was sketched and represented in Figure 20.
At this point, it is worth mentioning the agile and continuous improvement characteristic of the project and how the design has been enhanced and improved over the years. Figure 20 represents the latest version of the IT solution.
With the solution outline, information flow, and integrations defined, the project moved to the execution phase. Cloud resources were configured as per the requirements and solution definition.
Starting in early 2020, the acquisition of the meters and actuators were completed. Two applications were used for collecting data:
● EVE Measurements App (Figure 21):
Application developed and provided by the manufacturer of the device to collect and export the data for all devices.
● Power App (Figure 21):
Our developed application aiming for digital and efficient data collection from analog clocks (common residential clocks) into "soft" data.
As the data started to be collected, data transformation methods began using ETL (Extract, Transform, Load) methods [33], defined and shown in Figure 15. This reinteraction allowed for simultaneous analysis of the information and continuous improvements. Initially, due to the low volume of data, Excel spreadsheets and/or CSV files were used as the database. This brought agility and low complexity to the first stage of the project. In the following months, the use of Azure SQL databases brought stability and scalability to the model as described in the IT architecture in Section 3.2.3.
In 2022, a new set of hardware and software was introduced to improve data collection and real-time analysis. Introducing Shelly Professional Energy Monitoring devices enabled cloud collection of data, ensuring continuous data availability 24/7, eliminating manual processes and loss of data as shown previously in the solution outline, Figure 20.
A relational database was built containing multiple tables, such as: Customers, Clocks, Devices. This configuration offers what is known as "dimensions" to the "fact table". The fact table, allreadings, is the main energy consumption reading table where multiple entries are stored. It is a time series database containing multiple periodic measurements. Each entry contains a set of relevant information gathered from multiple Shelly devices. Consequently, the allreadings table is the largest table and is constantly written to by the integration. The Customer table contains the household customer details, and it is important to segregate device clocks and readings from one household to another. The clock table contains different physical or virtual electrical clocks installed in a household infrastructure. It is also the table that connects the different related costs to the devices, readings, and the information used by the Eq 1 presented previously. The device table contains the individual list of all installed Shelly devices. It is also an important dimension table as it relates all the readings to the device and its assigned household and clocks. It is also important to support the integration as it provides the list of devices to be queried by the integration.
As a crucial part of this work, the integration enables data to flow from Shelly devices to the database. This automation is an important piece of the solution as it eliminates manual export/import actions from the meters, saving effort and increasing reliability and consistency. It uses the Shelly's standard API to query each individual meter for its data. Complementarily, it transforms the data, selecting, filtering, and formatting before loading it into the database. Figure 22 below illustrates this integration.
The information was collected over a 12-month period (extended to 36 months) to capture seasonal effects, such as winter and summer, which could be observed. This seasonality demonstrated representative variations in the consumption pattern. For example, fridges and freezers during warmer summer days, or water heating during cold winter days having to work more to keep the set temperatures.
The process followed the schema previously shown in Figure 15. Starting with the consumption measurements being collected remotely via two applications at a frequency of 10 minutes, the data were incrementally loaded into a database created for this purpose. As part of the data processing, the Microsoft Power BI application was used for this purpose. In the optimization part, DOE models were applied, and algorithms in R were used to determine trends and optimization settings. Once the information was analyzed, they were interactively compared with optimized scenarios.
With all the resources described before, it was possible to create intuitive dashboards with yearly projections and average consumption KPIs to assist in continuous monitoring, as well as setting targets as shown on the Figure 23.
Another capability that allowed further analysis of energy consumption, among other features, was real-time monitoring, also known as telemetry. This functionality allows for the monitoring of events at the precise time they occur. Using the message protocol MQTT, the advanced features found on Shelly devices combined with the Mosquitto message broker installed on a Raspberry Pi, it was possible to manage messages from several devices. With the "event hub" correctly installed and configured, it was possible to set NodeRed to receive relevant messages by subscribing for specific topics enabling information flows, automations, and plot real-time graphs. Figure 24 shows one of these graphs used to monitor in real-time the temperature variation over the last 24 hours.
At the same time, it is also possible to create all sorts of information flows for home automations. This is particularly crucial for the optimization scenarios to expand the enhancements to less intuitive scenarios. These opportunities are described in Section 3.5. In Figure 25, it is possible to see some of the workflows to plot the graphs, create automations, and even set alarms integrated with the WhatsApp messaging system.
The outcome of the monitoring and analysis allowed the evaluation of different scenarios and their opportunities for energy savings. The potential gains were divided into short-term and long-term opportunities, as shown in Table 3 below.
Short-term gains (1–2 years) | Long-term gains (3–5 years) |
Replace traditional bulbs with LEDs (smart) | Exchange high consumption equipment for lower consumption devices |
Appropriate use of lighting for the occasion | Relocation of appliances for optimize thermal efficiencies and energy savings optimization |
Motion sensors to detect presence and drive automations to avoid waste | Temperature/Thermostat optimizations for better fit the needs and find advanced savings |
Identify and switch off equipment with high stand-by consumption | Behavioral and cultural change |
Calculation for minimum time to when to turn off appliances | |
Design routines for clever use of devices |
As mentioned, these opportunities for savings lead to a design of routines and automations for the effective use of the devices and appliances. See some other examples in Figure 26 below.
Several improvements were designed around the energy savings opportunities found. Some of them are summarized in the following:
● Bulb replacement
Starting with the most intuitive enhancement, replacing all traditional low efficiency light bulbs by Smart Philips HUE LED lights. It enables not only the direct savings of a more efficient light technology, but also the added ability to use dimerization, remote access, and include them in well-designed routines.
● Turning off high "stand-by" consumption devices
During the late evenings and early mornings, devices with high "stand-by" consumptions such as TVs, TV receivers, radios, computers, Bluetooth speakers, etc. were tuned off by the smart plugs, saving considerable amounts of energy during idle portions of the day. Presence sensors were used to determine the correct moments those devices needed to be on "stand-by" or completely off (individual controlled power strips were also used to ensure individual control of single devices)
● Turning off appliances during long trips (such as boilers and fridges)
Following a similar methodology as above, for longer trips, a change in behavior and planning allowed for taking further saving actions by switching off more complex logistic equipment. This prevented unnecessary use of energy to keep an empty cool fridge or hot water for nobody. All these associated to the convenience to turn them on prior to arrival, manually via geolocation, ensuring convenience and maintaining the right level of comfort.
● Adaptive lighting
It is designed to offer the right level of lighting needed for the occasion. As traditional switches only provide "on/off" capabilities, with smart bulbs it was possible to set dimmer lights for evenings. This does not only reduce electricity consumption, but also enhances comfort. Via motion and presence sensors, it was possible to take the automation a step further and only trigger the lights where needed and in unconventional places. E.g., LED strips under the bed to light up the way to the toilet in the middle of the night, avoiding disturbing a partner or walking in the dark.
● Use of geolocation
Use of mobile geolocation of the residents to only turn on security cameras when the occupants are away. Turn on water heaters only when the occupants are in a 100 m radius of the residence. Reducing energy waste due to thermal loss.
● Reducing relapses
Common relapses include leaving the lights on when leaving a room, leaving doors and windows open by mistake, not properly closing the door of the fridge, etc. Presence sensors to switch off lights and devices when the presence is not detected in the room for some time. Door and window sensors to notify when doors or windows were left open reducing heating demands. Also applicable to fridges and freezers to notify if the doors were not fully sealed.
● Repositioning of equipment.
Tracking the energy consumption data was possible to experiment and validate different configurations for certain equipment to reduce energy consumption. E.g., moving the freezer to a more ventilated area for better heat transfer and avoiding extra work from the compressor. Additional 5–10% savings on freezer consumption.
● Overwrite factory thermostats
Using MQTT, it was possible to combine temperature and energy consumption information and run distinct sets of temperature ranges, overwrite default factory setups, and adapt to a more optimized temperature operational range. In Figure 27, the original thermostat range is shown, set to operate between 50 and 65 degrees Celsius, representing a total of 228 Wh per day, and the final optimized range between 50 and 60 degrees Celsius, representing a total of 181 Wh per day. A total savings of 20% for the kitchen boiler alone was found, and the same methodology was used for all boilers.
● Other facilities
Additionally, making use of the "smart" functions of the device, it was also possible to include voice assistance to help with convenience and better control of the device on a specific occasion (e.g.: "goodnight" command to turn off all the unnecessary devices during the night).
Through the Power BI reports, it is possible to compare consumption and projections over the years. In the first years, where many of the short-term activities took place, it was possible to measure a considerable reduction in energy consumption and costs. Figure 28 shows a reduction in annual consumption from 2805 (2311 + 494) kWh per year in 2020 to 2262 (1745 + 517) kWh per year in 2022, a reduction of 19%. These initial findings align with the expectations proposed by Lackner [19], demonstrating and confirming the saving potential.
It is also possible to see the reflection on the financials between those two years, with a savings of 11 EUR on monthly costs, totaling 132 EUR per year. Therefore, this represents a very motivational incentive for further developments and investments. As stated previously, the year of 2022 saw a significant price increase for the electricity in Europe. This price increase emphasizes even further the importance of such work and its impact on household finances. As shown in Figure 29, despite the additional savings achieved in 2023, another 26% from 2022, and an overall 40% if compared to 2020 figures, the total cost has increased to 67 EUR per month. If calculated based on the current prices of kWh, and the consumption of 2020, the total costs would represent 112 EUR a month. Therefore, it is fair to assume that the current study has saved around 45 EUR a month and a total of 546 EUR per year.
In the following picture, Figure 30, it is possible to compare the breakdown of the consumption by segment and understand where big savings occurred and when short- and long-term actions started to have a significant impact.
Despite the impressive energy savings achieved throughout this extensive experiment, it is pertinent to highlight several significant factors that could potentially hinder implementation and affect results:
● Initial investment: The deployment of a continuous monitoring system featuring cutting-edge IoT devices can often entail a considerable upfront cost. Depending on the size of the residence, this initial investment may be prohibitive for households with tight budgets. Consequently, some homeowners may be discouraged from embarking on this journey or may limit the number of devices installed throughout their homes. This limitation can result in a restricted amount of data collected, thereby diminishing the opportunities for exploration and the implementation of potential optimizations. While prices have gradually decreased and technology has advanced in recent years, the perceived high cost of smart devices compared to non-smart alternatives remains a concern.
● High current limitations for Low-Voltage systems: The majority of electrical IoT devices are engineered for low-current applications, typically rated for up to 16 A. When integrated into 220−240 V systems, these devices can handle a maximum nominal power of 3600 Watts, suitable for a wide range of applications. However, when utilized in 110−120 V systems, the total power output is halved, significantly limiting the number of devices that can be connected to smart plugs. Additionally, this reduction in power availability may impede the ability to combine multiple devices under the same meter, further constraining operational flexibility.
● Lack of basic electrical knowledge: Moreover, linked to the preceding point, the absence of fundamental electrical knowledge can present a hurdle for homeowners delving into this area. It is common to encounter expressions like: "This seems complicated, " "What if I cause a fire, " or "Could I get shocked?" These statements reflect concerns stemming from a lack of comprehension or the necessary skill sets to handle and maintain electrical infrastructure.
● Limited logical thinking: Many homeowners find logical thinking to be a daunting task. The ability to conceptualize "if this, then that" scenarios and devise logical frameworks tailored to individual needs is crucial for achieving optimal results. How to create a logic that better represents individual needs is at the same time crucial to a good result. In the end, understanding and describing routines, multiple variables, boundary conditions, and desired outcomes can be overwhelming even for the most enthusiastic minds.
● Behavioral Psychology: First and foremost, this project underscores the importance of playing the "long game". Most of the limitations mentioned above are closely intertwined with behavioral science. Understanding what influences individuals to embrace or resist change, and focusing on long-term benefits over immediate gratification, lies at the heart of this exploration. Motivations for change can be broadly categorized as intrinsic or extrinsic.
o Extrinsic Motivation: External factors such as energy-saving laws, financial incentives like tax breaks or bonuses, mandated targets, societal norms, competitive environments, and tangible rewards.
o Intrinsic Motivation: Internally driven factors such as curiosity, ecological and environmental beliefs, a cooperative and pro-social mindset aimed at both personal well-being and community welfare. It involves a desire to participate actively and align with community norms by following positive examples.
It is known that in most cases it requires not one or another, but rather a combination of extrinsic and intrinsic motivations. That aims not only at short-term incentives and high adoption of behavioral change, but also draws a strategy to ensure long-term gains to the individuals and a collective win for society.
According to the management thinker Peter Drucker, "If you don't measure, you don't improve". For a good and rational measurement and more efficient use of electricity in a residence, it is crucial to constantly monitor its use. The digitalization of a home opens several opportunities for energy savings, reducing not only costs but also helping to reduce the carbon footprint and government investments.
An analysis of the energy efficiency of IoT devices was carried out by Vasilieva [34], including comparing the energy consumption before and after installation of the automation devices. The analysis revealed the substantial potential for conserving energy through the use of Internet of Things devices. Particularly noteworthy is the 34% reduction in energy consumption by smart thermostats, underscoring their role in optimizing indoor temperature control. Additionally, a 23% decline in energy usage was observed in smart security systems, showcasing their contribution to energy-efficient surveillance. Smart HVAC systems made a significant impact on energy conservation with an impressive 27% reduction in energy consumption. Even though smart lighting showed a more modest 12% decrease, it still played a part in enhancing energy efficiency. Furthermore, smart appliances achieved a 15% reduction in energy consumption, aligning with the broader goal of sustainability.
Smart thermostats, for example, can learn the household's patterns and adjust heating and cooling systems accordingly, leading to significant energy savings without sacrificing comfort as shown in Figure 27. This not only reduces energy bills, but also contributes to a more comfortable living environment. In the study of Abuhussain [35], a novel fuzzy controller with additional input parameters to keep indoor thermal comfort consistent with the corresponding number of occupants was developed. The proposed method was able to save up to 50% energy if occupants do not worry about comfort.
Smart lighting systems can be programmed to turn off lights in unoccupied rooms or adjust brightness based on natural light levels, thus reducing energy waste. Se-Hyun [36] proposed a lighting control method based on RILL (Ratio of Illuminance Influence of individual Lighting) that can realize the reduction of lighting energy within buildings.
Additionally, smart appliances such as energy-efficient washers, dryers, and refrigerators can be remotely controlled and optimized for energy usage, contributing to overall energy efficiency.
Moreover, smart home automation can enhance security and safety through features like motion-activated cameras, smart locks, and smoke/CO detectors linked to mobile devices. This not only provides peace of mind, but also contributes to a higher living standard.
In summary, smart home automation positively affects energy efficiency and living standards by optimizing energy usage, increasing convenience, enhancing security, and providing more control over various aspects of home management.
This study allowed us to confirm that by conscientious behavior, a thoughtful mindset, and combined with constant digital monitoring of information, it is possible to create wisdom and awareness about efficient energy use, as suggested in the DIKW Pyramid in Figure 16.
The IoT improvements and rational use of energy allowed for significant reductions in daily average consumption as shown in Figure 30, achieving approximately 20% on overall electricity consumption with small and short-term interventions up to 40%, including more long-term investments. Consequently, the costs were reduced by close to 500 Euros a year on current European prices, representing a return of investment of 1–2 years.
The author declares that he has not used artificial intelligence (AI) tools in the creation of this article.
The author declares no conflicts of interest.
Conceptualization, Gunnar Lima; methodology, Gunnar Lima and Andreas Nascimento; validation, Gunnar Lima, Andreas Nascimento, Marcelo P. Oliveira and Fagner L. G. Dias; formal analysis, Gunnar Lima, Andreas Nascimento, Marcelo P. Oliveira and Fagner L. G. Dias; writing—original draft preparation, Gunnar Lima; writing—review and editing, Gunnar and Lima Fagner L. G. Dias; supervision, Andreas Nascimento. All authors have read and agreed to the published version of the manuscript.
This study was supported by National Council for Scientific and Technological Development (CNPq), Funding Authority for Studies and Projects (FINEP), and Ministry of Science, Technology and Innovation (MCTI), through the PRH 34.1 FEG/UNESP program, process number 0444/1.
[1] | Tzeiranaki ST, Bertold P, Castellazz L, et al. (2022) Energy consumption and energy efficiency trends in the EU, 2000–2020. European Commission, Joint Research Centre, Publications Office of the European Union, 2022. https://dx.doi.org/10.2760/727548 |
[2] | IRENA (2023) World energy transitions outlook 2023: 1.5 ℃ pathway. Abu Dhabi: Int Renewable Energy Agency. Available from: https://www.irena.org/Publications/2023/Jun/World-Energy-Transitions-Outlook-2023. |
[3] | BBC (2022) Nord Stream blast 'blew away 50 meters of pipe'. BBC News. Available from: https://www.bbc.com/news/world-europe-63297085. |
[4] | BP p.l.c. (2023) Energy outlook 2023 edition. BP p.l.c. Available from: https://www.bp.com/content/dam/bp/business-sites/en/global/corporate/pdfs/energy-economics/energy-outlook/bp-energy-outlook-2023.pdf. |
[5] | Edelstein S (2022) Global EV sales more than doubled in 2021 vs. 2020, tripled vs. 2019. Green Car Rep. Available from: https://www.greencarreports.com/news/1134999_global-ev-sales-more-than-doubled-in-2021-vs-2020-tripled-vs-2019. |
[6] | RMI (2023) Cryptocurrency's energy consumption problem. RMI. Available from: https://rmi.org/cryptocurrencys-energy-consumption-problem/#: ~: text = Bitcoin%20alone%20is%20estimated%20to, fuel%20used%20by%20US%20railroads. |
[7] | Statista Inc (2021) Projected installed electricity generation capacity from battery storage worldwide from 2020 to 2050. Statista. Available from: https://www.statista.com/statistics/1307203/world-battery-storage-electricity-generation-capacity/. |
[8] |
Roth L, Lowitzsch J, Yildiz Ö (2021) An empirical study of how household energy consumption is affected by co-owning different technological means to produce renewable energy and the production purpose. Energies 14: 3996. https://doi.org/10.3390/en14133996 doi: 10.3390/en14133996
![]() |
[9] |
Matuszewska-Janica A, Żebrowska-Suchodolska D, Zalewska ME (2023) Differences in the structure of household electricity prices in EU countries. Energies 16: 6636. https://doi.org/10.3390/en16186636 doi: 10.3390/en16186636
![]() |
[10] | IRENA (2022) Renewable power generation costs in 2021. Int Renewable Energy Agency, Abu Dhabi. Available from: https://www.irena.org/publications/2022/Jul/Renewable-Power-Generation-Costs-in-2021. |
[11] | Eurostat (2020) Energy prices and costs in Europe. European Commission Brussels, Belgium. Available from: https://energy.ec.europa.eu/data-and-analysis/energy-prices-and-costs-europe_en. |
[12] |
Milewska B, Milewski D (2023) The Impact of energy consumption costs on the profitability of production companies in Poland in the context of the energy crisis. Energies 16: 6519. https://doi.org/10.3390/en16186519 doi: 10.3390/en16186519
![]() |
[13] |
Scheier E (2022) A measurement strategy to address disparities across household energy burdens. Nat Commun 13: 288. https://doi.org/10.1038/s41467-021-27673-y doi: 10.1038/s41467-021-27673-y
![]() |
[14] | Nord Pool AS (2023) System price curve data. Nord Pool. Available from: https://www.nordpoolgroup.com/en/elspot-price-curves/. |
[15] | Eurostat (2023) Electricity prices for household consumers—bi-annual data (from 2007 onwards). Eurostat. Available from: https://ec.europa.eu/eurostat/databrowser/view/NRG_PC_204__custom_7314052/default/table?lang = en. |
[16] |
Shang WL, Lv Z (2023) Low carbon technology for carbon neutrality in sustainable cities: A survey. Sustainable Cities Soc 92: 104489. https://doi.org/10.1016/j.scs.2023.104489 doi: 10.1016/j.scs.2023.104489
![]() |
[17] | EIA (2023) Annual energy outlook 2023. U.S. Energy Inf Adm. Available from: https://www.eia.gov/todayinenergy/detail.php?id = 56040#: ~: text = Our%20projections%20of%20residential%20consumption, 5.9%20quads%20and%206.3%20quads. |
[18] | Kahlenborn W, Kabisch S, Klein J (2012) Energy management systems in practice-ISO 50001: A guide for companies and organizations. Federal Ministry for the Environment, Nature Conservation and Nuclear Safety (BMU), Berlin, Germany. |
[19] | Lackner P, Holanek N (2007) Step by step guide for implementing energy management. Vienna: Austrian Energy Agency. |
[20] |
Lv Z, Shang WL, Guiza M (2022) Impact of digital twins and metaverse on cities: history, current situation, and application perspectives. Appl Sci 12: 12820. https://doi.org/10.3390/app122412820 doi: 10.3390/app122412820
![]() |
[21] |
Rojek I, Mikołajewski D, Mroziński A (2023) Machine learning- and Artificial intelligence-derived prediction for home smart energy systems with PV installation and battery energy storage. Energies 13: 6613. https://doi.org/10.3390/en16186613 doi: 10.3390/en16186613
![]() |
[22] | Shelly Cloud (2023) Devices. Shelly Knowledge Base. Available from: https://kb.shelly.cloud/knowledge-base/shelly-plus-devices. |
[23] | Signify Netherlands B.V. (2023) Hue white starter kit. Philips-Hue. Available from: https://www.philips-hue.com/en-us/p/hue-white-starter-kit-e26/046677563080. |
[24] | Lumi United Technology Co. Ltd. (2023) Presence sensor FP2. Aqara. Available from: https://www.aqara.com/us/product/presence-sensor-fp2/. |
[25] | Raspberry Pi Ltd. (2023) Raspberry Pi for home. Raspberry Pi. Available from: https://www.raspberrypi.com/. |
[26] | Shelly Cloud (2023) Energy metering (energy efficiency). Shelly Cloud. Available from: https://www.shelly.com/. |
[27] | Microsoft Corporate (2023) Power BI. Microsoft. Available from: https://powerbi.microsoft.com/en-us/. |
[28] | OpenJS Foundation (2023) Node-RED Low-code programming for event-driven applications. Node-RED. Available from: https://nodered.org/. |
[29] | Eclipse Foundation Inc. (2023) Eclipse Mosquitto: An open source MQTT broker. Eclipse Mosquitto. Available from: https://mosquitto.org/. |
[30] | Wikimedia Foundation Inc. (2023) Five Ws. Wikipedia. Available from: https://en.wikipedia.org/wiki/Five_Ws. |
[31] | Lean Six Sigma Institute (2023) Lean six sigma certification program. Available from: https://leansixsigmainstitute.org/. |
[32] | Sinek S (2009) Start with why: how great leaders inspire everyone to take action. Portfolio. |
[33] | IBM (2020) What is ETL extract transform load? IBM Corporation. Available from: https://www.ibm.com/topics/etl. |
[34] |
Vasilieva E, Deepa B, Soosan C (2024) IoT in home automation: A data-driven user behavior analysis and user adoption test. BIO Web Conf. 86: 1–9. https://doi.org/10.1051/bioconf/20248601085 doi: 10.1051/bioconf/20248601085
![]() |
[35] |
Abuhussain MA, Alotaibi BS, Aliero MS (2023) Adaptive HVAC system based on fuzzy controller approach. Appl Sci 13: 11354. https://doi.org/10.3390/app132011354 doi: 10.3390/app132011354
![]() |
[36] |
Lee SH, Oh ST, Lim JH (2024) Lighting control method based on RIIL to reduce building energy consumption. Energy Rep 11: 2090–2098. https://doi.org/10.1016/j.egyr.2024.01.075 doi: 10.1016/j.egyr.2024.01.075
![]() |
1. | Gustavo Henrique Romeu da Silva, Andreas Nascimento, Diego Nascimento, Julian David Hunt, Mauro Hugo Mathias, Analysis of the Sugarcane Biomass Use to Produce Green Hydrogen: Brazilian Case Study, 2025, 15, 2076-3417, 1675, 10.3390/app15031675 |
Examples of sectors | Energy share of production costs (indicative range) |
Average European business | 0–3% |
Trade, computers, motor vehicles, electrical equipment, pharmaceutics, construction | 0.4–1% |
Waste management, plastics, textiles, grain | 2–4% |
Accommodation and restaurants | 3–5% |
Energy intensive sectors in manufacturing: Cement, clay building materials, pulp and paper, glass, iron and steel, basic chemicals, non-ferrous metals, refineries | 3–20% |
Air transport, land transport | 20–30% |
Data centers | 10–15% |
Mining of metals and others, electricity, and gas | 10–20% |
W's | Findings |
Why? | ● Help Consumers reduce electrical consumption and costs. ● Reduce Environment impact. ● Reinforce good habits and behaviors. ● Grow electrical use awareness. Improve living quality and safety. |
How? | ● By creating an efficient Energy management system o Measuring continuously consumption per equipment o Measuring continuously total consumption ● By deploying smart devices: Plugs, lights, and sensors o Dimmable LED lights o Plugs and switches with remote on/off. o Wireless sensors: flexible positioning ● By setting a fit-to-purpose IT architecture o Solid and reliable Databases. o Comprehensive and easy to use data reports. o IoT infrastructure o Realtime monitoring system. ● Process oriented approach o Powerful workflows o Well-designed Routines o Customizable Automations |
What? | ● Energy management system ● Energy optimization strategies ● Alarms and notification |
Who? | ● Householders ● Energy companies ● Governments and institutions |
Where? | ● Houses ● Possible commercial applications |
When? | ● Short and long terms ● Minimum 1 year of data collection ● Continuous services ● Started in 2019 |
Short-term gains (1–2 years) | Long-term gains (3–5 years) |
Replace traditional bulbs with LEDs (smart) | Exchange high consumption equipment for lower consumption devices |
Appropriate use of lighting for the occasion | Relocation of appliances for optimize thermal efficiencies and energy savings optimization |
Motion sensors to detect presence and drive automations to avoid waste | Temperature/Thermostat optimizations for better fit the needs and find advanced savings |
Identify and switch off equipment with high stand-by consumption | Behavioral and cultural change |
Calculation for minimum time to when to turn off appliances | |
Design routines for clever use of devices |
Examples of sectors | Energy share of production costs (indicative range) |
Average European business | 0–3% |
Trade, computers, motor vehicles, electrical equipment, pharmaceutics, construction | 0.4–1% |
Waste management, plastics, textiles, grain | 2–4% |
Accommodation and restaurants | 3–5% |
Energy intensive sectors in manufacturing: Cement, clay building materials, pulp and paper, glass, iron and steel, basic chemicals, non-ferrous metals, refineries | 3–20% |
Air transport, land transport | 20–30% |
Data centers | 10–15% |
Mining of metals and others, electricity, and gas | 10–20% |
W's | Findings |
Why? | ● Help Consumers reduce electrical consumption and costs. ● Reduce Environment impact. ● Reinforce good habits and behaviors. ● Grow electrical use awareness. Improve living quality and safety. |
How? | ● By creating an efficient Energy management system o Measuring continuously consumption per equipment o Measuring continuously total consumption ● By deploying smart devices: Plugs, lights, and sensors o Dimmable LED lights o Plugs and switches with remote on/off. o Wireless sensors: flexible positioning ● By setting a fit-to-purpose IT architecture o Solid and reliable Databases. o Comprehensive and easy to use data reports. o IoT infrastructure o Realtime monitoring system. ● Process oriented approach o Powerful workflows o Well-designed Routines o Customizable Automations |
What? | ● Energy management system ● Energy optimization strategies ● Alarms and notification |
Who? | ● Householders ● Energy companies ● Governments and institutions |
Where? | ● Houses ● Possible commercial applications |
When? | ● Short and long terms ● Minimum 1 year of data collection ● Continuous services ● Started in 2019 |
Short-term gains (1–2 years) | Long-term gains (3–5 years) |
Replace traditional bulbs with LEDs (smart) | Exchange high consumption equipment for lower consumption devices |
Appropriate use of lighting for the occasion | Relocation of appliances for optimize thermal efficiencies and energy savings optimization |
Motion sensors to detect presence and drive automations to avoid waste | Temperature/Thermostat optimizations for better fit the needs and find advanced savings |
Identify and switch off equipment with high stand-by consumption | Behavioral and cultural change |
Calculation for minimum time to when to turn off appliances | |
Design routines for clever use of devices |