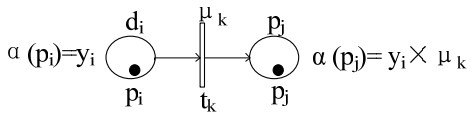
Citation: R. S. Solaiman, T. G. Kherbek, A. S. Ahmad. Defining a new method to set certainty factors to improve power systems prognosis with fuzzy petri nets[J]. AIMS Energy, 2020, 8(4): 686-700. doi: 10.3934/energy.2020.4.686
[1] | Amer Braik, Asaad Makhalfih, Ag Sufiyan Abd Hamid, Kamaruzzaman Sopian, Adnan Ibrahim . Impact of photovoltaic grid-tied systems on national grid power factor in Palestine. AIMS Energy, 2022, 10(2): 236-253. doi: 10.3934/energy.2022013 |
[2] | Akash Talwariya, Pushpendra Singh, Mohan Lal Kolhe, Jalpa H. Jobanputra . Fuzzy logic controller and game theory based distributed energy resources allocation. AIMS Energy, 2020, 8(3): 474-492. doi: 10.3934/energy.2020.3.474 |
[3] | Chrish Kavuma, Diego Sandoval, Hakizimana Khan Jean de Dieu . Analysis of power generating plants and substations for increased Uganda's electricity grid access. AIMS Energy, 2021, 9(1): 178-192. doi: 10.3934/energy.2021010 |
[4] | Minh Y Nguyen . Optimal voltage controls of distribution systems with OLTC and shunt capacitors by modified particle swarm optimization: A case study. AIMS Energy, 2019, 7(6): 883-900. doi: 10.3934/energy.2019.6.883 |
[5] | Abdollah Kavousi-Fard, Amin Khodaei . Multi-objective optimal operation of smart reconfigurable distribution grids. AIMS Energy, 2016, 4(2): 206-221. doi: 10.3934/energy.2016.2.206 |
[6] | Mowffaq M. Oreijah, Mohammed Yunus . A parametric analysis to evaluate the performance metrics of power generation system involving Trilateral Flash Cycle using three different working fluids for low grade waste heat. AIMS Energy, 2019, 7(4): 483-492. doi: 10.3934/energy.2019.4.483 |
[7] | Ryuto Shigenobu, Oludamilare Bode Adewuyi, Atsushi Yona, Tomonobu Senjyu . Demand response strategy management with active and reactive power incentive in the smart grid: a two-level optimization approach. AIMS Energy, 2017, 5(3): 482-505. doi: 10.3934/energy.2017.3.482 |
[8] | Foday Conteh, Shota Tobaru, Mohamed E. Lotfy, Atsushi Yona, Tomonobu Senjyu . An effective Load shedding technique for micro-grids using artificial neural network and adaptive neuro-fuzzy inference system. AIMS Energy, 2017, 5(5): 814-837. doi: 10.3934/energy.2017.5.814 |
[9] | Ahmed Neffati, Amira Marzouki . Local energy management in hybrid electrical vehicle via Fuzzy rules system. AIMS Energy, 2020, 8(3): 421-437. doi: 10.3934/energy.2020.3.421 |
[10] | Ahsan Iqbal, Ayesha Ayoub, Asad Waqar, Azhar Ul-Haq, Muhammad Zahid, Syed Haider . Voltage stability enhancement in grid-connected microgrid using enhanced dynamic voltage restorer (EDVR). AIMS Energy, 2021, 9(1): 150-177. doi: 10.3934/energy.2021009 |
Power systems performance assessment is receiving increasing attention from researchers because of its importance to keep working requirements acceptable; its main idea is to determine performance indicators for the system and its elements that define weak elements, possible faulted sections and reliable working. Fault diagnosis and prognosis are two important forms of performance assessment that help in decreasing faults bad effects on the electrical system, fault diagnosis deals with possible faults in the current situation of the system, while prognosis issue deals with the future system status which means predicting how would system behave in expected conditions to prevent malfunctions before its occurrence [1]. Electrical networks are wide spread systems which consist of large number of elements which exposes it to many disturbances affect its services, we can divide them into two categories internal and external affected factors. The former includes influences caused by changes in the basic electrical parameters (voltage, current, frequency), which results in problems in providing consumers demands and internal faults in some elements. The latter includes influences come from outside resources like the surrounding environment, temperature, lightning and human errors [2]. These effects and others may reduce system's efficiency so it's important to take them in consideration when evaluating system performance; they are changing in turn so there is always a need to adapt the changes, which makes this process more complex.
Many methods have been used to achieve fault diagnosis for electrical networks such as neural networks [3] which have effective representation to determine faulted sections when historical data are available but it has a long training time with every connect/disconnect case, genetic algorithm [4] used to define the most reasonable fault hypotheses but it may fail to complete the process in case of insufficient background information, fuzzy logic [5] is suitable for diagnosis process because of its ability to cover wide range of possibilities even in case of lack data.
Fuzzy petri nets are very important tools used in this field, because of their abilities to represent the networks graphically and mathematically, their extensions adapt with a lot of applications and constantly evolving to increase works accuracy. Most of diagnosis methods use FPN depends on separate representation of elements [6], in [7] the analysis of incidence matrix is used to make large networks formulation easier, another developments included with FPNs to fit topological changes [8], take relays time features in consideration [9]. Fault prognosis is a recent term in power systems operations aims to prevent hazard situations and keep operation under reliable recommendations by rising correct operation time and reducing interruption time, intelligent methods such as neural networks (NN) [10,11] are powerful tools can represent the behavior of dynamic complex systems, a recurrent NN (RNN) shown in [12] have suggested clustering technique results in a smaller error compared with conventional (NNs) shown in [13], in [14] power flow had been calculated then neural network should trained to predict the hazards meet corresponding loads, probability theory can provide a perfect avenue for diagnosis and prognosis processes. Combination of intelligent method and Bayesian algorithm is used to develop a prognosis method for hydro control valve system [15]. Probability prediction is used to determine voltage problems in transmission networks [16], knowledge based methods like fuzzy logic method apply experts knowledge in a form of if-then rules to predict the dynamics of failure in various operating conditions [17,18]. An important application of fuzzy logic is shown in [19] where fuzzy logic and adaptive-neuro fuzzy systems are used to evaluate levels of road traffic congestion. A new hybrid fault prognosis methodology, which was a combination of the distributed neural networks and the recursive Bayesian method, was proposed for the application of the aircraft MFS System in [20]. Petri nets also have been used to prognosis faults depending on calculating probabilities of transitions firing [21], included fuzzy logic to PN allowed dealing with uncertainty and incomplete information to performance analysis [22]. A full description of the most important methods of forecasting faults is available in [23].
Reasoning in traditional FPN use the information of relays and circuit breakers as inputs and the relations between them determines the faulted element or section, transitions describe system transfer from one state to another and the confidence degree of the rule related to a transition is set as constant certainty factor [24], initial truth degrees is set as the statistical average correct operation rates of elements. The actual reliability operation rates cannot be accurately detected so to get a representation closer to the real operation in [25] they used Weibull distribution to estimate parameters of reliability then used them to measure the initial information certainty, in addition the relative values of reliabilities are used to determine the weights of places during reasoning.
The basic contribution of this research is to adapt with changing operating conditions when FPN diagnosis and prognosis tasks are carried out, research related to this field focus on a specific state or element in computations because of the difficulty to deal with many states and conditions at the same time. In this paper we proposed a different view of prognosis electrical systems performance using FPN, it depends on the relationships between the elements to build the model and use fuzzy rules to determine certainty factors instead of set them as constant values. After that we take different operation conditions (load cases, environmental factors) to analysis their effects on system performance and take appropriate procedures to avoid danger cases. The main contribution in this paper is:
1. Submitting a new way to set CF values so they can adapt with changes in working circumstances, this makes the representation closer to system real status.
2. Building the FPN based on the relationships between elements allows to take the interact effect in reasoning so many hazards may be taken in consideration.
3. In view of 1 and 2 dealing with system transformation will be more flexible since the consequent FPN covers many disturbances may affect the system.
This paper is organized as follows: section 2 shows a review of fuzzy Petri net theory and the process of traditional diagnosis with FPN, section 3 explains the proposed prognosis method, in section 4 three cases at different conditions have been calculated and finally section 5 shows the conclusions.
FPN is one of Petri nets extension which combines the graphical representation of petri nets with the reasoning logic of fuzzy logic that makes them effective tools in dealing with uncertainty and lack of data [26]:
A FPN can be defined as the following:
FPN=(P,T,D,I,O,f,α,β) | (1) |
where:
P = (p1, p2... pi, pn) is a finite nonempty set of places, n number of places;
T = (t1, t2... ti, tm) is a finite nonempty set of transitions, m is number of transitions;
D = (d1, d2………dn) is a finite nonempty set of propositions;
I: P→T is an m × n matrix, it represents input relationships which described by directed arcs from places to transitions;
O: T→P is an m × n matrix, it represents output relationships which described by directed arcs from transitions to places;
f: T→[0, 1] is a function attaches transitions to real values between zero and one;
α: P→[0, 1] is a function attaches places to real values between zero and one;
β: P→D is a function attaches places to propositions.
There are two different kinds of directed arcs that connect the nodes of the net; the arc from place node to transition node is called input arc and the arc from transition to the place is the output one. When the input subset of a place x has no element it is called source place and is called sink if its output subset has no element. The dynamic characteristic of a net is described by markings and the enabling and firing rule of the transitions. Each transition ti ∈ T is associated with a real value μi ∈ [0, 1] which is called certainty factor (CF) of ti. The place pi ∈ P is marked with a token associated with a value α (pi) = yi, yi ∈ [0, 1]. If β (pi) = di, di ∈ D then the place pi is associated with the proposition di with the truth degree equals as yi. The token in a place pi in a marked FPN is drawn as (•) inside the place pi. A transition ti in marked FPN is fired if for all pj ∈ I (ti), α (pi) ≥ λ, where λ ∈ [0, 1] is a predefined threshold value. Besides, the firing fuzzy rules can be summarized as follows:
1) If pi is a place and β (pi) = di, α (pi) = yi then yi ∈ [0, 1] is a truth degree of the proposition di.
2) If pi is an antecedent place, and the pj is a consequence place of the transition tk, where pi ∈ I (tk), pj ∈ O (tk), then the truth degree of the pj is defined based on the fuzzy production rule as following:
α(pj)=yi×μk | (2) |
The simple rule of FPN is shown in Figure 1.
3) For a transition tk, if pj ∈ O (tk) is the output place and the number of input places pi ∈ I (tk) is more than one, then the truth degree of the pj is defined based on the fuzzy conjunctive rule as following:
α(pj)=min(yi1,yi2....yin)×μk | (3) |
The conjunctive rule of FPN is depicted in Figure 2.
4) If pj is a terminal place where tkj ∈ I (pj), pj ∈ O (tkj), k = 1, …, n, then the truth degree of the pj is defined as following:
α(pj)=max(yi1×μ1,yi2×μ2....yin×μn) | (4) |
This is the disjunctive rule of FPN and it is shown in Figure 3.
In traditional FPN diagnosis alarm messages and protection devices information are used to define the diagnosis results. This way doesn't take in consideration the relationships between elements and doesn't allow representing internal and external factors affect the system.
The basic purpose of our method is preventing dangerous situation from damaging system services, to achieve this task we analyze systems performance at different predicted conditions to know how can they affect the system and take preventive procedures when there is a possibility of danger. For these goals we offer some suggestions to make the FPN model closer to the real state of the system, we can summarize the differences between the original fuzzy petri nets and the proposed Fuzzy Petri nets by:
1- Building the FPN based on the relationships between the elements, that means the FPN will reflect the mutual interaction between elements each other while the original FPNs depends on the protection devices to determine the probable faulted section.
2- Determine the initial places as fuzzy numbers so it shows the real initial state of the element instead of considering them as average values as original FPNs.
3- Attaching operation factors to the CF of the transitions to adapt any change in operating conditions instead of giving them a constant value.
4- For reasoning process there isn't any difference between the proposed FPNs and the original ones.
Since FPN have the same reasoning principle as fuzzy logic then we head for using it to replace the constant CF with changing value CF which reflects more accurate representation of the network real state. We represent the conditions as simple fuzzy framework where the membership functions represent the strength of the conditions and there ranges, and the fuzzy rules represent the relationships between parameters and the consequently CF denotes how the condition affect the system.
Here we recognize three kinds of CF in the supposed FPN:
1- Permanent CF: this kind is attached to transitions that describe operational parameters which any change of them affects the system, they always enter the assessment.
2- Constraint CF: this kind is attached to transitions that describe parameters affect the system in special cases or specific range, they enter the reasoning just when they exceed the limited values; that requires to put high threshold λ that prevent the transition from firing, when there is danger changes in condition λ will be low and t is enable.
3- Normal CF: this kind is attached to transitions that work for getting reasoning results.
In addition, two kinds of initial places are defined:
1- Places which represent the steady state elements situation their truth degrees are set as a fuzzy rule between the reliable rate operation and the basic parameters related to the element.
2- Places which represent measured parameters their truth degrees are set as their fuzzy equivalent numbers.
After building the FPN model and determining initial truth degrees and convenient CF values we get the prognosis results by achieving a normal reasoning process.
We take the IEEE 9 bus system from [27] to clarify the proposed method. First we choose two important parameters affecting power systems operation to include them in the computations; they are: load changes and environmental factors. The former is a vital parameter in electrical systems performance assessment, it affects voltage stability, lines status and system frequency, the latter is a fundamental parameter in evaluating many elements working especially outside sections as lines. These parameters are important to investigate two serious risk states may cause a huge damage to the whole system, they are voltage collapse and line interruption, both of them start with limited elements then accelerate to include wide areas which we want to prevent from happening, the Table 1 shows summary information about these hazards.
Risk state | Causes | Solutions |
Voltage collapse | A significant increase in load active power. Insufficient reactive power resources. | Install special protection systems for the weakest contract. Reactive Power Compensation. |
Line interruption | Over voltage, overload, and heating. | Maintain operating parameters within appropriate limits. |
Here we carried out computations at high load demands to prognosis there effects on the system and suggest convenient solutions. In addition we insert environmental factors in the calculations as danger factor because of its effects on the elements operation especially when high currents flow. Figure 4 shows the IEEE9 bus system which consists of 3 controlled voltage sources, 3 loads, 3 transformers, 9 bars; basic voltage levels are 13.8 kV, 16.5 kV, and 18 kV.
The proposed FPN model for the network is illustrated in Figure 5. We divided the network into three local areas based on the distances between buses (local1 B1, B4, and B5), (local2 B2, B7 and B8), (local3 B3, B6 and B9). Every area has been evaluated on basis of its buses assessment, which have been estimated by fuzzy relationship between the lines connected to it and its node voltage, all this information gives finally the prognosis result.
Initial places (L1 to L9) give the initial representation of lines performance according to their reliable operation, nominal power flow and line's long. Next layer of places (L11 to L99) represents the current status of the lines after taking in consideration the change in load value by means of including fuzzy factor with the transitions.
Initial places (v1 to v9) represent the node voltage of the bus bars and their truth degrees are carried out by take the equivalent fuzzy number of the real values of voltages.
To represent the environmental factors we use transitions with constraint CF
We designed four fuzzy controllers with fuzzy inference system (FIS) in mat lab [28] to get permanent and constrained CF values.
● The first one allows inserting the effect of load changes with time on lines state.
● The second one aims to insert environmental changes' effect on the system.
● The third one describes the reliability relationships between bars to determine the effect of their work on section estimation.
● The fourth one describes the reliability relationship between sections to determine the effect of their work on network estimation.
We illustrate our additional suggestions by taking three cases to discuss:
1. At total load 8 p.u lasted for 1 hour.
2. At total load 8 p.u lasted for 2 hours.
3. When the environmental conditions exceeds the limited value.
We used Psat tool in Mat lab toolbox [29] to curry out load flow computations, and FIS tool to design fuzzy controllers to determine permanent and constraint CF values.
FIS1: describes the effect of load changes with time on line state
(Inputs: line load, its period; output CF).
FIS2: describes the effect of environmental conditions on the line state
(Inputs: temperature, wind speed; output CF).
FIS3: bus bars estimation
(Inputs: bus I, bus II, bus III; output CF).
FIS4: sections estimation
(Inputs: local I, local II, local III; output CF).
Here we insert a controlled threshold λ, that means in the normal state λ = 1 and the transition is blocked, when temp exceeds 38℃ and wind speed exceeds 70 km.h-1, λ becomes 0.5 and t becomes enabled.
Lines initial truth degrees are shown in Table 2.
Line number | Reliable operation | Normal flow pu | Line long km | Truth degree |
1 | 0.95 | 0.24 | 106 | 0.93 |
2 | 0.95 | 0.76 | 76 | 0.96 |
3 | 0.95 | 0.6 | 179 | 0.92 |
4 | 0.95 | 0.86 | 170 | 0.92 |
5 | 0.95 | 0.4 | 89 | 0.92 |
6 | 0.95 | 0.3 | 97 | 0.94 |
7 | 0.99 | - | - | 0.99 |
8 | 0.99 | - | - | 0.99 |
9 | 0.99 | - | - | 0.99 |
CF values for transitions figured out from FIS1 and they are shown in Table 3.
Transition | CF |
t 1 | 0.87 |
t 2 | 0.85 |
t 3 | 0.9 |
t 4 | 0.86 |
t 5 | 0.72 |
t 6 | 0.72 |
t 7 | 0.95 |
t 8 | 0.95 |
t 9 | 0.95 |
Table 4 shows voltage and load flow values from the simulation of Psat tool and results of truth degrees for each bus from FIS3 and for the local sections from FIS4.
B6 | B9 | B3 | |||||||||||||||
V | L3 | L6 | T.D | V | L1 | L3 | L8 | T.D | V | L8 | T.D | ||||||
8 pu/1h | 1.04 | 0.1 | 2.9 | 0.6 | 1.025 | 0.1 | 1.63 | 0.72 | 1.025 | 0.85 | 0.84 | ||||||
Local 3 = min (B3, B6, B9) * CF = 0.6 * 0.93 = 0.55 | |||||||||||||||||
B4 | B5 | B1 | |||||||||||||||
V | L5 | L6 | L9 | T.D | V | L4 | L5 | T.D | V | L9 | T.D | ||||||
8 pu/1h | 0.86 | 2.9 | 2.9 | 6.3 | 0.59 | 0.81 | 0.16 | 2.9 | 0.59 | 0.81 | 6.3 | 0.84 | |||||
Local 1 = min(B4, B5, B1) * CF = 0.59 *0.93 = 0.55 | |||||||||||||||||
B7 | B8 | B2 | |||||||||||||||
V | L2 | L4 | L7 | T.D | V | L1 | L2 | T.D | V | L7 | T.D | ||||||
8 pu/1h | 0.95 | 1.7 | 0.16 | 1.63 | 0.71 | 0.92 | 0.95 | 1.79 | 0.72 | 0.96 | 1.63 | 0.84 | |||||
Local 2 = min (B7, B8, B2) * CF = 0.71*0.95 = 0.66 |
NETWORK1 = min (local1, local2, local3) * CF = 0.54*0.95 = 0.51
We find in this experiment that the overloaded lines appears as less truth degree which helps in determine the weak element and then the weak section; notice that in original FPNs the constant values of initial places and CF will give confusion results because all lines truth degrees will be the same and we couldn’t find out the weak element.
The CF values will be changed because the period of time is changed; Table 5 illustrates the new CF.
Transition | CF |
t 1 | 0.84 |
t 2 | 0.8 |
t 3 | 0.87 |
t 4 | 0.8 |
t 5 | 0.64 |
t 6 | 0.64 |
t 7 | 0.9 |
t 8 | 0.9 |
t 9 | 0.9 |
We get results as the same in the first case; Table 6 illustrates them:
B6 | B9 | B3 | |||||||||||||||
8 pu/2h | 1.04 | 0.1 | 2.9 | 0.54 | 1.025 | 0.1 | 1.475 | 0.7 | 1.025 | 0.85 | 0.8 | ||||||
Local 3 = min (B3.B6, B9) * CF = 0.54*0.92 = 0.49 | |||||||||||||||||
B4 | B5 | B1 | |||||||||||||||
V | L5 | L6 | L9 | T.D | V | L4 | L5 | T.D | V | L9 | T.D | ||||||
8 pu/2h | 0.86 | 2.9 | 2.9 | 6.3 | 0.52 | 0.81 | 0.16 | 2.9 | 0.54 | 0.81 | 6.3 | 0.8 | |||||
Local 1 = min(B4.B5, B1) * CF = 0.52*0.92 = 0.47 | |||||||||||||||||
B7 | B8 | B2 | |||||||||||||||
V | L2 | L4 | L7 | T.D | V | L1 | L2 | T.D | V | L7 | T.D | ||||||
8 pu/2h | 0.95 | 9.8 | 0.16 | 1.63 | 0.64 | 0.92 | 13.5 | 1.79 | 0.7 | 0.96 | 1.63 | 0.8 | |||||
Local 2 = min (B7.B8, B2) * CF = 0.64*0.93 = 0.59 |
NETWORK2 = min (local1, local2, local3) * CF = 0.47*0.93 = 0.44
When comparing this experiment with the previous one, we see that the increase in overloading time appears as a decrease in the final truth degree which shows a greater danger than first case, notice that original FPNs don't allow to control cf then they can't deal with this changing parameter unless a new net had been designed with every change.
The difference here is entering the constraint transitions which change the initial values of the lines; Table 7 shows CF values for constraint transitions and Table 8 shows the results.
Constraint transitions | CF |
t 1' | 0.88 |
t 2 ' | 0.88 |
t 3' | 0.88 |
t 4' | 0.9 |
t 5' | 0.87 |
t 6' | 0.88 |
t 7' | 0.9 |
t 8' | 0.9 |
t 9' | 0.9 |
B6 | B9 | |||||||||||||
L3 | L6 | T.D | L1 | L3 | L8 | T.D | L8 | T.D | ||||||
8 | 0.92*0.88 | 0.94*0.88 | 0.63 | 0.93*0.88 | 0.92*0.88 | 0.95*0.9 | 0.72 | 0.95*0.9 | 0.75 | |||||
Local 3 = min (B3, B6, B9) * CF = 0.63*0.92 = 0.58 | ||||||||||||||
B4 | B5 | B1 | ||||||||||||
L5 | L6 | L9 | T.D | L4 | L5 | T.D | L9 | T.D | ||||||
8 | 0.92*0.88 | 0.94*0.88 | 0.95*0.9 | 0.51 | 0.92*0.9 | 0.92*0.88 | 0.51 | 0.95*0.9 | 0.75 | |||||
Local 1 = min (B4, B5, B1) * CF = 0.51*0.9 = 0.45 | ||||||||||||||
B7 | B8 | B2 | ||||||||||||
L2 | L4 | L7 | T.D | L1 | L2 | T.D | L7 | T.D | ||||||
8 | 0.92*0.88 | 0.92*0.9 | 0.95*0.9 | 0.64 | 0.93*0.88 | 0.92*0.88 | 0.64 | 0.95*0.9 | 0.75 | |||||
Local 2 = min (B7, B8, B2) * CF = 0.58 |
NETWORK3 = min (local1, local2, local3) * CF = 0.50.45*0.93 = 0.41
In this case it's obviously clear that the network is in bad operating status because all conditions exceed the safe limits, the network here is still working but it's close to a danger state so it's necessary to take convenient procedures to avoid any serious dangers. Notice that in original FPNs it's not possible to find out these effects because of the constant transitions from one state to another, in addition, network will be more complex when many factors enter the representation.
After checking the results shown above we notice from the first two cases that long period time of high loads appears in the truth degrees of the buses which are useful when we need to know bus state at every change in loads. Also inserting constraint transitions helps in detecting their effects when it's necessary. As we notice the flexibility of the net because there is no need to rebuild the model with every change in factors since the new CFs will deal with any new condition and the constraint ones will enter just in specific cases without making the reasoning complex.
After the analytical study we use prognosis results in determining the weak element to take appropriate procedures, here installing redundant power compensation devices will be useful to keep the system safe, as the real load approaches the expected loads, it can start with establishing substations to relieve pressure on the lines. Another useful of this method is the ability to use it in network design and expansion, by entering the expected loads or expected future conditions to prognosis network response and determine the appropriate procedures (size of generators, number of lines.) It's also possible to use prognosis results to avoid voltage problems on the bars and support system stability related to voltage and power flow problems.
In order to clarify the characteristics of the proposed method we review in the Table 9 a comparison between these networks and the most important intelligence methods used in the field of prognosis.
Intelligent methods | Dealing with several risk states | Graphical representation | Complexity | Insert changing conditions | Process time |
Neural networks | hard | Not possible | complex for large networks | hard | long since the need of training the NN with any changes |
Fuzzy logic | hard | Not possible | complex for large networks | hard | long with every change a new computations figured out |
Fuzzy petri nets | Possible | powerful | complex for large networks | Hard with constant CF | Long when changes happened we need to rebuild the FPN |
Proposed FPN | Possible | Powerful | Less since combining more than one state and condition | possible by changing CF | less since making the computation for several conditions |
Our research focuses on making the performance prognosis of power systems closer to reality in order to take appropriate measures to increase operation uptime relative to downtime. Two basic developments are introduced here to adapt to various operating conditions: defining new kinds of certainty factors and representing initial places with fuzzy relations.
We have applied our proposed method on the IEEE 9 bus test system under different load conditions and environmental changes. The results show the advantages of the proposed method. Two of which are to improve the representation of FPN, so there is no need to rebuild it at every change in operation, and to enter the conditions at specific limits to avoid complexity. The researchers were able to apply this method on any electrical network and define any important factors as permanent or constraint certainty factors according to the purpose of the study.
The authors declare no conflict of interests.
The authors declare that contents included in this article are purely scholarly ideas of the authors. These are not related to any organizations. However, the data used in the article have been properly referred.
[1] |
Chang L, Wu Z (2011) Performance and reliability of electrical power grids under cascading failures. Int J Electr Power Energy Syst 33: 1410-1419. doi: 10.1016/j.ijepes.2011.06.021
![]() |
[2] | Innal F (2008) Contribution to modelling safety instrumented systems and to assessing their performance-critical analysis of IEC 61508 standard. PhD thesis, University of Bordeaux. |
[3] |
Cardoso G, Rolim JG, Zurn HH (2004) Application of neural network modules to electric power system fault section estimation. IEEE Trans Power Del 19: 1034-41. doi: 10.1109/TPWRD.2004.829911
![]() |
[4] |
Leao FB, Pereira RAF, Mantovani JRS (2014) Fast fault section estimation in distribution control centers using adaptive genetic algorithm. Int J Electr Power Energy Syst 63: 787-805. doi: 10.1016/j.ijepes.2014.06.052
![]() |
[5] |
Sang-Won M, Jin-Man S, Jong-Keun P, et al. (2004) Adaptive fault section estimation using matrix representation with fuzzy relations. IEEE Trans Power Syst 19: 842-848. doi: 10.1109/TPWRS.2003.821036
![]() |
[6] |
Liu HC, Lin QL, Ren ML (2013) Fault diagnosis and cause analysis using fuzzy evidential reasoning approach and dynamic adaptive fuzzy Petri nets. Comput Ind Eng 66: 899-908. doi: 10.1016/j.cie.2013.09.004
![]() |
[7] | Yang JW, HeZheng Y, Tan XJ, et al. (2010) A distributed fault diagnosis approach in power system based on fuzzy reasoning Petri net. IEEE Fund project: Project Supported by National Science Foundation of China. |
[8] |
Yan Z, Yong Z, Fushuan W, et al. (2016) A fuzzy Petri net based approach for fault diagnosis in power systems considering temporal constraints. Electr Power Energy Syst J 78: 215-224. doi: 10.1016/j.ijepes.2015.11.095
![]() |
[9] | Yang J, He Z (2011) Power system fault diagnosis approach based on time sequence fuzzy Petri net. Autom Electr Power Syst 35: 46-51. |
[10] | Jagabondhu H, Sinha AK (2006) Prognosis of catastrophic failures in electric power systems. IEEE international conference on industrial technology ICIT. |
[11] |
David L, Zhaoyin C, Oliver S, et al. (2019) A neural network evolutionary computational framework for remaining useful life estimation of mechanical systems. Neural Netw 116: 178-187. doi: 10.1016/j.neunet.2019.04.016
![]() |
[12] |
Malhi A, Yan R, Gao RX (2011) Prognosis of defect propagation based on recurrent neural networks. IEEE Trans Instrum Meas 60: 703-711. doi: 10.1109/TIM.2010.2078296
![]() |
[13] |
Jun Z, Nan C, Weiwen P (2019) Estimation of bearing remaining useful life based on multiscale convolutional neural network. IEEE Trans Ind Electr 66: 3208-3216. doi: 10.1109/TIE.2018.2844856
![]() |
[14] |
Yun SL, Goran S (2002) Analytical approach to probabilistic prediction of voltage sags on transmission networks. IEE Proc-gener Transm Distrib 149: 7-14. doi: 10.1049/ip-gtd:20020008
![]() |
[15] | Mojtaba K, Amir Z, Marcos O, et al. (2019) A modular fault diagnosis and prognosis method for hydro-control valve system based on redundancy in multisensor data information. IEEE Trans 68: 330-341. |
[16] | Ammour R, Leclercq E, Sanlaville E, et al. (2016) Faults prognosis using partially observed stochastic Petri nets. Proceedings of the 13th International Workshop on Discrete Event Systems, Xi'an, China. |
[17] |
Balaje T, Miles F, Jagannathan S (2014) A model-based fault detection and prognostics scheme for Takagi-Sugeno fuzzy systems. IEEE Trans Fuzzy Syst 22: 736-748. doi: 10.1109/TFUZZ.2013.2272584
![]() |
[18] | Wang Z, Hu C, Wang W, et al. (2012) An off-online fuzzy modelling method for fault prognosis with an application. Proceedings of the IEEE 2012 Prognostics and System Health Management Conference (PHM-2012 Beijing), 1-7. |
[19] | Panita P, Poj T, Kanokchai N (2007) Evaluation of road traffic congestion using fuzzy techniques. TENCON, IEEE Region 10 International Conference. |
[20] | Mojtaba K, Foad S, Mehrdad S (2020) A new hybrid fault prognosis method for MFS systems based on distributed neural networks and recursive bayesian algorithm. IEEE Syst J. |
[21] | Mariana D (2013) Combined Fuzzy-Petri nets hybrid model for power system performability analysis. Computing Science and Automatic Control (CCE) Mexico City, Mexico. |
[22] |
Chen SM (2000) Fuzzy backward reasoning using fuzzy Petri nets. IEEE Trans Syst Ma Cybern Part B: Cybern 30: 846-856. doi: 10.1109/3477.891146
![]() |
[23] | Mojtaba K, Mehrdad S, Marcos O, et al. (2019) Failure prognosis and applications-A survey of recent literature. IEEE Trans Reliab. |
[24] | Zhao XL, Zhou JZ, Liu H (2008) Research of fault diagnostic model based on probability Petri nets. Comput Eng 24: 7-224. |
[25] |
Hongbo C, Zhengyou H, Qi W, et al. (2015) Fault diagnosis method based on Petri nets considering service feature of information source devices. Comput Electr Eng 46:1-13. doi: 10.1016/j.compeleceng.2015.06.016
![]() |
[26] | Iman K, Saeed L (2020) Fault section identification in smart distribution systems using Multi-Source data based on fuzzy Petri nets. IEEE Trans Smart Grid. |
[27] | Dhananjay K, Shivam P, Akash T, et al. (2018) Identification of weak bus using load variation. Int Res J Eng Technol 5: 841-847. |
[28] | Vdokuments khnowledge project. Available from: https://vdocuments.mx/fuzzy-logic-toolbox-users-guide.html. Accessed on 13-Jan-2017. |
[29] | Vdokuments khnowledge project. Available from: https://vdocuments.mx/psat-eecswsuedu-ee521material20120927psat-psat-power-system-analysis.html. Accessed on 19-Jul-2018. |
1. | Zhongwei Li, Wenqi Jiang, Xiaosheng Liu, Kai Tan, Xianji Jin, Ming Yang, GAN model using field fuzz mutation for in-vehicle CAN bus intrusion detection, 2022, 19, 1551-0018, 6996, 10.3934/mbe.2022330 | |
2. | Hamed Alqahtani, Gulshan Kumar, Deep learning-based intrusion detection system for in-vehicle networks with knowledge graph and statistical methods, 2024, 1868-8071, 10.1007/s13042-024-02465-0 |
Risk state | Causes | Solutions |
Voltage collapse | A significant increase in load active power. Insufficient reactive power resources. | Install special protection systems for the weakest contract. Reactive Power Compensation. |
Line interruption | Over voltage, overload, and heating. | Maintain operating parameters within appropriate limits. |
Line number | Reliable operation | Normal flow pu | Line long km | Truth degree |
1 | 0.95 | 0.24 | 106 | 0.93 |
2 | 0.95 | 0.76 | 76 | 0.96 |
3 | 0.95 | 0.6 | 179 | 0.92 |
4 | 0.95 | 0.86 | 170 | 0.92 |
5 | 0.95 | 0.4 | 89 | 0.92 |
6 | 0.95 | 0.3 | 97 | 0.94 |
7 | 0.99 | - | - | 0.99 |
8 | 0.99 | - | - | 0.99 |
9 | 0.99 | - | - | 0.99 |
Transition | CF |
t 1 | 0.87 |
t 2 | 0.85 |
t 3 | 0.9 |
t 4 | 0.86 |
t 5 | 0.72 |
t 6 | 0.72 |
t 7 | 0.95 |
t 8 | 0.95 |
t 9 | 0.95 |
B6 | B9 | B3 | |||||||||||||||
V | L3 | L6 | T.D | V | L1 | L3 | L8 | T.D | V | L8 | T.D | ||||||
8 pu/1h | 1.04 | 0.1 | 2.9 | 0.6 | 1.025 | 0.1 | 1.63 | 0.72 | 1.025 | 0.85 | 0.84 | ||||||
Local 3 = min (B3, B6, B9) * CF = 0.6 * 0.93 = 0.55 | |||||||||||||||||
B4 | B5 | B1 | |||||||||||||||
V | L5 | L6 | L9 | T.D | V | L4 | L5 | T.D | V | L9 | T.D | ||||||
8 pu/1h | 0.86 | 2.9 | 2.9 | 6.3 | 0.59 | 0.81 | 0.16 | 2.9 | 0.59 | 0.81 | 6.3 | 0.84 | |||||
Local 1 = min(B4, B5, B1) * CF = 0.59 *0.93 = 0.55 | |||||||||||||||||
B7 | B8 | B2 | |||||||||||||||
V | L2 | L4 | L7 | T.D | V | L1 | L2 | T.D | V | L7 | T.D | ||||||
8 pu/1h | 0.95 | 1.7 | 0.16 | 1.63 | 0.71 | 0.92 | 0.95 | 1.79 | 0.72 | 0.96 | 1.63 | 0.84 | |||||
Local 2 = min (B7, B8, B2) * CF = 0.71*0.95 = 0.66 |
Transition | CF |
t 1 | 0.84 |
t 2 | 0.8 |
t 3 | 0.87 |
t 4 | 0.8 |
t 5 | 0.64 |
t 6 | 0.64 |
t 7 | 0.9 |
t 8 | 0.9 |
t 9 | 0.9 |
B6 | B9 | B3 | |||||||||||||||
8 pu/2h | 1.04 | 0.1 | 2.9 | 0.54 | 1.025 | 0.1 | 1.475 | 0.7 | 1.025 | 0.85 | 0.8 | ||||||
Local 3 = min (B3.B6, B9) * CF = 0.54*0.92 = 0.49 | |||||||||||||||||
B4 | B5 | B1 | |||||||||||||||
V | L5 | L6 | L9 | T.D | V | L4 | L5 | T.D | V | L9 | T.D | ||||||
8 pu/2h | 0.86 | 2.9 | 2.9 | 6.3 | 0.52 | 0.81 | 0.16 | 2.9 | 0.54 | 0.81 | 6.3 | 0.8 | |||||
Local 1 = min(B4.B5, B1) * CF = 0.52*0.92 = 0.47 | |||||||||||||||||
B7 | B8 | B2 | |||||||||||||||
V | L2 | L4 | L7 | T.D | V | L1 | L2 | T.D | V | L7 | T.D | ||||||
8 pu/2h | 0.95 | 9.8 | 0.16 | 1.63 | 0.64 | 0.92 | 13.5 | 1.79 | 0.7 | 0.96 | 1.63 | 0.8 | |||||
Local 2 = min (B7.B8, B2) * CF = 0.64*0.93 = 0.59 |
Constraint transitions | CF |
t 1' | 0.88 |
t 2 ' | 0.88 |
t 3' | 0.88 |
t 4' | 0.9 |
t 5' | 0.87 |
t 6' | 0.88 |
t 7' | 0.9 |
t 8' | 0.9 |
t 9' | 0.9 |
B6 | B9 | |||||||||||||
L3 | L6 | T.D | L1 | L3 | L8 | T.D | L8 | T.D | ||||||
8 | 0.92*0.88 | 0.94*0.88 | 0.63 | 0.93*0.88 | 0.92*0.88 | 0.95*0.9 | 0.72 | 0.95*0.9 | 0.75 | |||||
Local 3 = min (B3, B6, B9) * CF = 0.63*0.92 = 0.58 | ||||||||||||||
B4 | B5 | B1 | ||||||||||||
L5 | L6 | L9 | T.D | L4 | L5 | T.D | L9 | T.D | ||||||
8 | 0.92*0.88 | 0.94*0.88 | 0.95*0.9 | 0.51 | 0.92*0.9 | 0.92*0.88 | 0.51 | 0.95*0.9 | 0.75 | |||||
Local 1 = min (B4, B5, B1) * CF = 0.51*0.9 = 0.45 | ||||||||||||||
B7 | B8 | B2 | ||||||||||||
L2 | L4 | L7 | T.D | L1 | L2 | T.D | L7 | T.D | ||||||
8 | 0.92*0.88 | 0.92*0.9 | 0.95*0.9 | 0.64 | 0.93*0.88 | 0.92*0.88 | 0.64 | 0.95*0.9 | 0.75 | |||||
Local 2 = min (B7, B8, B2) * CF = 0.58 |
Intelligent methods | Dealing with several risk states | Graphical representation | Complexity | Insert changing conditions | Process time |
Neural networks | hard | Not possible | complex for large networks | hard | long since the need of training the NN with any changes |
Fuzzy logic | hard | Not possible | complex for large networks | hard | long with every change a new computations figured out |
Fuzzy petri nets | Possible | powerful | complex for large networks | Hard with constant CF | Long when changes happened we need to rebuild the FPN |
Proposed FPN | Possible | Powerful | Less since combining more than one state and condition | possible by changing CF | less since making the computation for several conditions |
Risk state | Causes | Solutions |
Voltage collapse | A significant increase in load active power. Insufficient reactive power resources. | Install special protection systems for the weakest contract. Reactive Power Compensation. |
Line interruption | Over voltage, overload, and heating. | Maintain operating parameters within appropriate limits. |
Line number | Reliable operation | Normal flow pu | Line long km | Truth degree |
1 | 0.95 | 0.24 | 106 | 0.93 |
2 | 0.95 | 0.76 | 76 | 0.96 |
3 | 0.95 | 0.6 | 179 | 0.92 |
4 | 0.95 | 0.86 | 170 | 0.92 |
5 | 0.95 | 0.4 | 89 | 0.92 |
6 | 0.95 | 0.3 | 97 | 0.94 |
7 | 0.99 | - | - | 0.99 |
8 | 0.99 | - | - | 0.99 |
9 | 0.99 | - | - | 0.99 |
Transition | CF |
t 1 | 0.87 |
t 2 | 0.85 |
t 3 | 0.9 |
t 4 | 0.86 |
t 5 | 0.72 |
t 6 | 0.72 |
t 7 | 0.95 |
t 8 | 0.95 |
t 9 | 0.95 |
B6 | B9 | B3 | |||||||||||||||
V | L3 | L6 | T.D | V | L1 | L3 | L8 | T.D | V | L8 | T.D | ||||||
8 pu/1h | 1.04 | 0.1 | 2.9 | 0.6 | 1.025 | 0.1 | 1.63 | 0.72 | 1.025 | 0.85 | 0.84 | ||||||
Local 3 = min (B3, B6, B9) * CF = 0.6 * 0.93 = 0.55 | |||||||||||||||||
B4 | B5 | B1 | |||||||||||||||
V | L5 | L6 | L9 | T.D | V | L4 | L5 | T.D | V | L9 | T.D | ||||||
8 pu/1h | 0.86 | 2.9 | 2.9 | 6.3 | 0.59 | 0.81 | 0.16 | 2.9 | 0.59 | 0.81 | 6.3 | 0.84 | |||||
Local 1 = min(B4, B5, B1) * CF = 0.59 *0.93 = 0.55 | |||||||||||||||||
B7 | B8 | B2 | |||||||||||||||
V | L2 | L4 | L7 | T.D | V | L1 | L2 | T.D | V | L7 | T.D | ||||||
8 pu/1h | 0.95 | 1.7 | 0.16 | 1.63 | 0.71 | 0.92 | 0.95 | 1.79 | 0.72 | 0.96 | 1.63 | 0.84 | |||||
Local 2 = min (B7, B8, B2) * CF = 0.71*0.95 = 0.66 |
Transition | CF |
t 1 | 0.84 |
t 2 | 0.8 |
t 3 | 0.87 |
t 4 | 0.8 |
t 5 | 0.64 |
t 6 | 0.64 |
t 7 | 0.9 |
t 8 | 0.9 |
t 9 | 0.9 |
B6 | B9 | B3 | |||||||||||||||
8 pu/2h | 1.04 | 0.1 | 2.9 | 0.54 | 1.025 | 0.1 | 1.475 | 0.7 | 1.025 | 0.85 | 0.8 | ||||||
Local 3 = min (B3.B6, B9) * CF = 0.54*0.92 = 0.49 | |||||||||||||||||
B4 | B5 | B1 | |||||||||||||||
V | L5 | L6 | L9 | T.D | V | L4 | L5 | T.D | V | L9 | T.D | ||||||
8 pu/2h | 0.86 | 2.9 | 2.9 | 6.3 | 0.52 | 0.81 | 0.16 | 2.9 | 0.54 | 0.81 | 6.3 | 0.8 | |||||
Local 1 = min(B4.B5, B1) * CF = 0.52*0.92 = 0.47 | |||||||||||||||||
B7 | B8 | B2 | |||||||||||||||
V | L2 | L4 | L7 | T.D | V | L1 | L2 | T.D | V | L7 | T.D | ||||||
8 pu/2h | 0.95 | 9.8 | 0.16 | 1.63 | 0.64 | 0.92 | 13.5 | 1.79 | 0.7 | 0.96 | 1.63 | 0.8 | |||||
Local 2 = min (B7.B8, B2) * CF = 0.64*0.93 = 0.59 |
Constraint transitions | CF |
t 1' | 0.88 |
t 2 ' | 0.88 |
t 3' | 0.88 |
t 4' | 0.9 |
t 5' | 0.87 |
t 6' | 0.88 |
t 7' | 0.9 |
t 8' | 0.9 |
t 9' | 0.9 |
B6 | B9 | |||||||||||||
L3 | L6 | T.D | L1 | L3 | L8 | T.D | L8 | T.D | ||||||
8 | 0.92*0.88 | 0.94*0.88 | 0.63 | 0.93*0.88 | 0.92*0.88 | 0.95*0.9 | 0.72 | 0.95*0.9 | 0.75 | |||||
Local 3 = min (B3, B6, B9) * CF = 0.63*0.92 = 0.58 | ||||||||||||||
B4 | B5 | B1 | ||||||||||||
L5 | L6 | L9 | T.D | L4 | L5 | T.D | L9 | T.D | ||||||
8 | 0.92*0.88 | 0.94*0.88 | 0.95*0.9 | 0.51 | 0.92*0.9 | 0.92*0.88 | 0.51 | 0.95*0.9 | 0.75 | |||||
Local 1 = min (B4, B5, B1) * CF = 0.51*0.9 = 0.45 | ||||||||||||||
B7 | B8 | B2 | ||||||||||||
L2 | L4 | L7 | T.D | L1 | L2 | T.D | L7 | T.D | ||||||
8 | 0.92*0.88 | 0.92*0.9 | 0.95*0.9 | 0.64 | 0.93*0.88 | 0.92*0.88 | 0.64 | 0.95*0.9 | 0.75 | |||||
Local 2 = min (B7, B8, B2) * CF = 0.58 |
Intelligent methods | Dealing with several risk states | Graphical representation | Complexity | Insert changing conditions | Process time |
Neural networks | hard | Not possible | complex for large networks | hard | long since the need of training the NN with any changes |
Fuzzy logic | hard | Not possible | complex for large networks | hard | long with every change a new computations figured out |
Fuzzy petri nets | Possible | powerful | complex for large networks | Hard with constant CF | Long when changes happened we need to rebuild the FPN |
Proposed FPN | Possible | Powerful | Less since combining more than one state and condition | possible by changing CF | less since making the computation for several conditions |