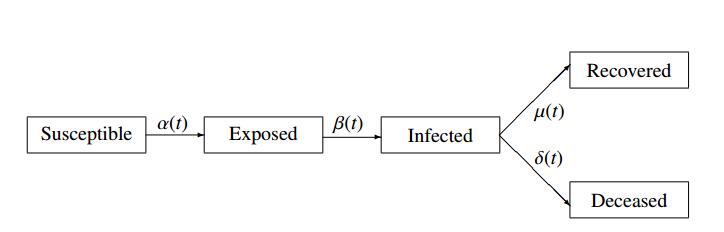
Citation: Baseem Khan, Hassan Haes Alhelou, Fsaha Mebrahtu. A holistic analysis of distribution system reliability assessment methods with conventional and renewable energy sources[J]. AIMS Energy, 2019, 7(4): 413-429. doi: 10.3934/energy.2019.4.413
[1] | Antonio N. Bojanic, Alejandro Jordán . Modeling the COVID-19 epidemic in Bolivia. Big Data and Information Analytics, 2020, 5(1): 47-57. doi: 10.3934/bdia.2020004 |
[2] | Ugo Avila-Ponce de León, Ángel G. C. Pérez, Eric Avila-Vales . A data driven analysis and forecast of an SEIARD epidemic model for COVID-19 in Mexico. Big Data and Information Analytics, 2020, 5(1): 14-28. doi: 10.3934/bdia.2020002 |
[3] | Nickson Golooba, Woldegebriel Assefa Woldegerima, Huaiping Zhu . Deep neural networks with application in predicting the spread of avian influenza through disease-informed neural networks. Big Data and Information Analytics, 2025, 9(0): 1-28. doi: 10.3934/bdia.2025001 |
[4] | Antonio N. Bojanic . Accounting for the Trump factor in modeling the COVID-19 epidemic: the case of Louisiana. Big Data and Information Analytics, 2021, 6(0): 74-85. doi: 10.3934/bdia.2021006 |
[5] | Jiaqi Ma, Hui Chang, Xiaoqing Zhong, Yueli Chen . Risk stratification of sepsis death based on machine learning algorithm. Big Data and Information Analytics, 2024, 8(0): 26-42. doi: 10.3934/bdia.2024002 |
[6] | Yiwen Tao, Zhenqiang Zhang, Bengbeng Wang, Jingli Ren . Motality prediction of ICU rheumatic heart disease with imbalanced data based on machine learning. Big Data and Information Analytics, 2024, 8(0): 43-64. doi: 10.3934/bdia.2024003 |
[7] | Bill Huajian Yang . Modeling path-dependent state transitions by a recurrent neural network. Big Data and Information Analytics, 2022, 7(0): 1-12. doi: 10.3934/bdia.2022001 |
[8] | Bill Huajian Yang . Resolutions to flip-over credit risk and beyond-least squares estimates and maximum likelihood estimates with monotonic constraints. Big Data and Information Analytics, 2018, 3(2): 54-67. doi: 10.3934/bdia.2018007 |
[9] | Anupama N, Sudarson Jena . A novel approach using incremental under sampling for data stream mining. Big Data and Information Analytics, 2018, 3(1): 1-13. doi: 10.3934/bdia.2017017 |
[10] | Amanda Working, Mohammed Alqawba, Norou Diawara, Ling Li . TIME DEPENDENT ATTRIBUTE-LEVEL BEST WORST DISCRETE CHOICE MODELLING. Big Data and Information Analytics, 2018, 3(1): 55-72. doi: 10.3934/bdia.2018010 |
An outbreak of pneumonia initially took place in Wuhan, China, which turned out to be coronavirus disease 2019 (COVID-19), caused by a novel coronavirus (SARS-CoV-2). The pandemic has brought about devastating public health, economic, and political crises. The outbreak has spread to 222 countries and territories, inflicted more than 116.17 million confirmed cases, and claimed more than 2.58 million lives, as reported on March 7th, 2021 [1]. People residing in the United States (US) have paid a high price; the pandemic has caused more than 29.05 million confirmed cases and claimed 527,726 lives, as reported on March 11th, 2021 [2,3]. The emergence of COVID-19 pandemic demonstrates the importance of research on accurately understanding and modeling the transmission and spread of the COVID-19 and SARS-CoV-2 [4,5,6]. It is essential to understand the mathematical mechanism and dynamics of COVID-19 to predict the pandemic better and evaluate the effects of intervention strategies and offer effective policies [7]. This paper is motivated by the need for in-depth investigations.
The COVID-19 is a communicable disease. An exposed person may interact with and transmit the virus to a susceptible individual. An interaction between the exposed person and the susceptible individual may result in the susceptible individual becoming exposed. An exposed individual may experience an incubation period before becoming ill, i.e., infected. Thus, an exposed person is infectious but not yet infected. An infected individual may be lucky enough to recover, at least to some extent, after a period of time. Alternatively, they will die. This process can be described by a dynamical system of susceptible-exposed-infected-recovered-deceased (SEIRD) differential equations, which can connect the numbers of susceptible, exposed, infected, recovered, and deceased individuals. Figure 1 shows the SEIRD model flow diagram of the transmission of COVID-19.
In the Figure 1, α(t) is the contact rate between susceptible and exposed people, β(t) is the incidence rate of the exposed people becoming infected, μ(t) is the recovery rate of the infected individuals, and δ(t) is the mortality rate of the infected individuals. In the literature, a system of susceptible-exposed-infected-recovered (SEIR) differential equations was developed to describe diseases like chickenpox and vector-borne diseases such as dengue hemorrhagic fever [8,9,10,11,12,13,14,15,16,17,18,19,20,21,22,23,24,25,26,27,28,29,30,31,32]. These diseases have a long incubation period, during which time the exposed individual cannot yet transmit the pathogen to others. The SEIR assumes that only an infected individual is infectious and can transmit the virus to a susceptible person and an exposed person is not infectious, as with susceptible-infected-recovered (SIR) models. For the very dangerous COVID-19, an exposed individual is infectious and can transmit the virus to susceptible people rapidly. An infected person is infectious but may be sent to hospitals quickly and separated from the susceptible people, making them unlikely to transmit the virus to susceptible people. Therefore, the existing SEIR and SIR systems cannot be used to analyze the COVID-19, and we need to build new models to fill the gap.
To get an intuitive idea of these rates for the COVID-19 transmission process, we obtain population data from US Census Bureau and COVID-19 daily reports from the Johns Hopkins University website through a GitHub repository [4,5,6]. The data are then combined for a unified analysis. We then calculate the empirical incidence, mortality, and recovered rates. Figure 2 provides trajectories of total numbers of people tested, confirmed cases, deaths, and recovered as well as related linear regression lines for COVID-19 in the US from April 16th to June 30th, 2020.
In Figures 3 and S1, we show trajectories of empirical testing rate, incidence rate, mortality rate, and recovery rate as well as related quadratic and linear regression lines for COVID-19 based on the results of Figure 2, respectively. It can be seen from the Figures 3 and S1 that both the testing rate and recovery rate increase, the incidence rate decreases, and the mortality rate increases at the beginning and then remains relatively stable before decreasing. The features of these rate may be due to people's being inexperienced at the beginning of the pandemic but gradually becoming more experienced at dealing with it and getting better prepared.
The empirical results shown in Figures 1, 3 and S1 provide us with a rationale for building the models. One noticeable feature is that these rates are not constants but time-dependent. Figures 1, 3 and S1 are based on COVID-19 data in the US from April 16th to June 30th, 2020, in which the sample size is large, and the deterministic SEIRD system is appropriate. The results of Figures 1, 3 and S1 provide a good estimate of the incidence, mortality, and recovery rates of COVID-19. Based on the empirical results, we can consider a few cases of contact, incidence, recovery, and mortality rates to model dynamics of COVID-19.
In this article, we build a system of SEIRD ordinary differential equations to describe relationship among the numbers of susceptible, exposed, infected, recovered, and deceased individuals in a town, state or country based on the empirical rates. The organization of the paper is as follows. In section 2, we build SEIRD models in details. We perform empirical analysis for the SEIRD models in section 3. In section 4, we provide some discussion about the property of the SEIRD models and some thoughts about future research direction.
Consider an independent time variable t which is measured in days. At time t, let s(t) be the number of susceptible individuals in a town, state or country; e(t), the number of exposed individuals that have transmitted the virus; i(t), the number of exposed individuals that are infected; r(t), the number of infected individuals that have recovered; and d(t), the number of infected individuals that have died. To describe the transmission process of COVID-19, consider a SEIRD system of ordinary differential equations
ds(t)dt=−α(t)s(t)e(t),de(t)dt=α(t)s(t)e(t)−β(t)e(t),di(t)dt=β(t)e(t)−μ(t)i(t)−δ(t)i(t),dr(t)dt=μ(t)i(t),dd(t)dt=δ(t)i(t), | (2.1) |
with initial conditions s(0)=S0,e(0)=E0,i(0)=I0,r(0)=0, and d(0)=0. In the system (2.1), α(t) is contact rate, β(t) is incidence rate, μ(t) is recovery rate, and δ(t) is mortality rate displayed in the Figure 1. Note all these rates are non-negative. The first equation in the system (2.1) is called susceptible equation, the second equation in the system (2.1) is called exposed equation, the third is called infected equation, the fourth is called recovery equation, and the fifth is called deceased equation, respectively. The system (2.1) is different from those in the literature of SEIR system as the susceptible and exposed equations are different. In the SEIR models, it is assumed that only an infected individual can transmit the virus to a susceptible person like SIR models and so the first two SEIR equations are
ds(t)dt=−α(t)s(t)i(t),de(t)dt=α(t)s(t)i(t)−β(t)e(t). |
For COVID-19, however, an exposed individual is infectious and may interact with a susceptible individual, thus transmitting the virus. This transmission is described by the susceptible equation in system (2.1). The exposed equation in system (2.1) implies that an exposed individual may contract coronavirus and experience an incubation period before becoming ill, i.e., infected. Once contracting the virus, an exposed person can transmit the virus to a susceptible individual. The infected can either recover with a recovery rate μ(t) or die with a mortality rate δ(t).
Let us denote N=S0+E0+I0. Note
ds(t)dt+de(t)dt+di(t)dt+dr(t)dt+dd(t)dt=0. |
It follows that s(t)+e(t)+i(t)+r(t)+d(t)=N is a constant. Since ds(t)dt=−α(t)s(t)e(t)≤0, the susceptible function s(t) is a decreasing function. Similarly, the recovery function r(t) and deceased function d(t) are increasing functions since dr(t)dt=μ(t)i(t)≥0 and dd(t)dt=δ(t)i(t)≥0, respectively. In the following, we impose conditions on incidence rate β(t), recovery rate μ(t), and mortality rate δ(t) based on the empirical results of the Figures 3 and S1 as follows
Quadratic decline of incidence rate: β(t)=β0−β1t+β2t2≥0,β0>0,β1>0,β2>0,Linear decline of incidence rate: β(t)=β0−β1t≥0,β0>0,β1>0,Linear increase of recovery rate: μ(t)=μ0+μ1t,μ0≥0,μ1≥0,Quadratic mortality rate: δ(t)=δ0+δ1t+δ2t2≥0,δ0≥0,δ1≥0,δ2<0. | (2.2) |
As shown in Figure 3, a declining incidence rate implies that the proportion of infected people is high at the beginning but decreases as time goes by. The empirical mortality rate is assumed to be a quadratic curve. It implies that the mortality rate increases initially, after which it remains stable and eventually decreases. One possible reason for this is that the virus is strongly virulent at the beginning but gradually weakens. Also, healthcare for infected people improves, and this contributes to an eventual decline in mortality. On the other hand, an increasing recovery rate is most likely due to improving healthcare and the fact that people are more experienced at handling the disease as time progresses. We assume that the recovery rate is linearly increasing from the empirical result of COVID-19 in the US, as shown in Figure 3.
For the contact rate α(t), we consider an exponential declining rate: α(t)=α0exp(−α1t),α0>0,α1>0. The rationale for a declining contact rate is that people may contact each other as normal at the beginning stage of the pandemic but reduce contact quickly as time moves on. The exponential decline means that the contact reduces to a minimum fast.
From the susceptible equation and the exposed equation in the system (2.1), we have
de(t)ds(t)=−1+β(t)α(t)s(t). |
Integrating the above equation gives
e(t)=E0+S0−s(t)+∫t0β(u)α(u)s(u)ds(u). |
If α(t)=α0 and β(t)=β0 are constants, the above equation provides an exact solution
e(t)=E0+S0−s(t)+1qln(s(t)/S0),q=α0β0. | (2.3) |
In addition, de(t)ds(t)=−1+β0α0s(t)=0 implies that e(t) reaches its maximum when s(t)=1/q. Hence, the maximal number of exposed individual is given by
emax=E0+S0−1q(1+ln(qS0)), if α(t)=α0 and β(t)=β0. | (2.4) |
Under an assumption of constant contacting rate α(t)=α0 and constant incidence rate β(t)=β0, we obtain the exposed function e(t) and the maximal number of exposed individual emax in relations (2.3) and (2.4). In general, the contact rates, incidence rates, recovery rates, and mortality rates are functions of time variable t. An exact solutions of SEIRD system (2.1) is not possible. Instead, a numerical approximation procedure can provide us a solution via Euler's method [33,34,35]. Taking a small time interval Δt and increment Δs(t)=s(t+Δt)−s(t), we may make an approximation ds(t)dt=Δs(t)Δt. Similarly, we may approximate di(t)dt=Δi(t)Δt, where Δi(t)=i(t+Δt)−i(t), etc. Then, we may obtain a system of recurrence equations as follows to approximate the numbers of susceptible, exposed, infected, recovered, and deceased
s(t+Δt)=s(t)−α(t)s(t)e(t)Δt,e(t+Δt)=e(t)+[α(t)s(t)e(t)−β(t)e(t)]Δt,i(t+Δt)=i(t)+[β(t)e(t)−μ(t)i(t)−δ(t)i(t)]Δt,r(t+Δt)=r(t)+μ(t)i(t)Δt,d(t+Δt)=d(t)+δ(t)i(t)Δt. | (3.1) |
Using the recurrence system (3.1) and initial conditions s(0)=S0,e(0)=E0,i(0)=I0,r(0)=0, and d(0)=0, we perform numerical approximations for a few cases of the rates defined in relations (2.2).
By using the rate functions provided in the Figure 3, we perform numerical calculation for approximate numbers of susceptible, exposed, infected, and deceased. The incidence, recovery, and mortality rates are from Figure 3: β(t)=0.01(21.0−0.30t+0.0018t2), μ(t)=0.01(14.65+0.188t), and δ(t)=0.01(5.25+0.045t−0.00067t2). The initial conditions are given by S0=106,E0=10, and I0=2. We consider four exponential decline contact functions α(t)=1.2×10−6exp(α1t): (a) α1=−0.01, (b) α1=−0.025, (c) α1=−0.05, and (d) α1=−0.065, respectively. Thus, the exponential declining rates of the four contact functions decrease from −0.01 to −0.065. The results are plotted in Figure 4.
It can be seen from Figure 4(a), (b) that the number of susceptible individuals rapidly decreases to zero when α1=−0.01 and α1=−0.025. If α1=−0.05, the number of susceptible individuals decreases and stabilizes around 100,000 when the time t is about 30 days (Figure 4 (c)); if α1=−0.065, the number of susceptible individuals decreases and stabilizes around 500,000 when the time t is about 30 days (Figure 4 (d)). For each plot, the numbers of exposed and infected start low and then increase. Once they reach their peak, they decrease to low numbers again. When α1=−0.01 and α1=−0.025, both the numbers of exposed and infected reach high levels when the time t is about 15 days, while fewer people are exposed and infected when α1=−0.05 and α1=−0.065, respectively. The numbers of recovered and deceased individuals increase. The numbers of recovered and deceased decrease as the exponential declining rate decreases exponentially from –0.01 to –0.065, e.g., the curve of recovered in Figure 4(a) is higher than that in Figure 4(d).
In the supplementary materials, we consider another set of incidence, recovery, and mortality rates from Figure S1: β(t)=0.01(19.25−0.17t),μ(t)=0.01(14.65+0.20t), and δ(t)=0.01(5.25+0.045t−0.001t2). In this set of rates, the incidence rate is fitted by linear regression, instead of a quadratic regression in Figure 3. Again, the initial conditions are given by S0=106,E0=10, and I0=2. Using the rates, approximate numbers of susceptible, exposed, infected, and deceased are given in Figure S2, which provides similar results as Figure 4.
In Figure 5, we use another set of rates to calculate approximate numbers of susceptible, exposed, infected, and deceased: β(t)=0.01(14.5−0.25t),μ(t)=0.01(8.5+0.30t), and δ(t)=0.01(4.5−0.05t−0.001t2). The features of the numbers of susceptible, recovered, and deceased are similar to that of Figure 4. However, the number of exposed is still high and does no reach a low level when time t is around 50 days, and the number of infected does not stabilize when time t is around 50 days. Hence, this set of rate functions leads to a good fit for the transmissions and progressions of US COVID-19 pandemic.
In this article, we build SEIRD differential equations to describe relationships among the numbers of susceptible, exposed, infected, recovered, and deceased individuals. Based on the empirical rates, empirical analyses for the SEIRD models are carried out to explore the applications and implications of the models. For COVID-19, a susceptible individual may interact with an exposed person and contract the virus. Hence, an exposed individual is infectious and can transmit the virus to susceptible people rapidly. The SEIRD system differs from SEIR and SIR differential equations in the literature. In these literature models, only an infected individual is infectious and can transmit the virus to a susceptible person. The proposed SEIRD system can directly describe the transmission of COVID-19 in a susceptible population.
Using the US empirical incidence, mortality, and recovery rate functions of COVID-19, we calculate approximate numbers of susceptible, exposed, infected, recovered, and deceased. The incidence, recovery, and mortality rate functions are functions of time in days and are not constant. We consider four exponential decline contact functions to investigate the impact of contact rate on the transmission of COVID-19. An exponential declining contact rate implies that people have contact with each other as usual at the beginning, with a reduction in contact quickly later on. Our empirical results show that a very rapid decline in contact significantly reduces the numbers of exposed, infected, and deceased. Therefore, it makes sense to wear masks and reduce social activities during the pandemic.
In the literature, the SEIR models were extended to be a susceptible-exposed-infected-recovered-susceptible (SEIRS) models, where recovered people may become susceptible again, i.e., recovery does not confer lifelong immunity [25]. For example, rotavirus and malaria are diseases with long incubation periods, and recovery only confers temporary immunity. In the SEIRD system (2.1), we assume immunity for COVID-19 and that recovery confers lifelong immunity. The models can be extended to remove this assumption. As time progresses, we may have empirical data supporting the fact that recovery does not confer lifelong immunity for COVID-19; SEIRD system (2.1) can then be extended to accommodate the finding. Another extension of SEIRD system (2.1) allows for immunity by vaccination. Because vaccine rollout began in December 2020, its effectiveness and influence can be evaluated and provide us with data to help us develop an extension of the SEIRD system that will accommodate immunity by vaccination.
In this article, we only consider a deterministic system of SEIRD differential equations which can not capture random fluctuations of the numbers of susceptible, exposed, infected, recovered, and deceased individuals. The deterministic models can be extended to include stochastic components to describe random fluctuations of the virus transmissions of COVID-19 and viral phylodynamics of SARS-CoV-2 [36,37,38,39,40,41,42,43,44,45]. More research is needed for further investigations.
This study was supported by U.S. National Science Foundation grant DMS-1915904 (Ruzong Fan). One anonymous reviewer and Dr. Jianhong Wu, provided very good and insightful comments for us to improve the manuscript. Mr. Marion L. Hartley helped us to edit the paper. Ruzong Fan conceives the project; Shuqi Wang, Wen Tang, Liyan Xiong, Menyu Fang, and Chi-Yang Chiu provide technical support for the project.
All authors declare no conflicts of interest in this paper.
In the supplementary materials, we consider another set of incidence, recovery, and mortality rates from Figure S1: β(t)=0.01(19.25−0.17t),μ(t)=0.01(14.65+0.20t), and δ(t)=0.01(5.25+0.045t−0.001t2). In this set of rates, the incidence rate is fitted by linear regression, instead of a quadratic regression in Figure 3. Again, the initial conditions are given by S0=106,E0=10, and I0=2. Using the rates, approximate numbers of susceptible, exposed, infected, and deceased are given in Figure S2, which provides similar results as Figure 4.
[1] |
Brown RE, Gupta S, Christie RD, et al. (1996) Distribution system reliability assessment using hierarchical Markov modeling. IEEE Trans Power Delivery 11: 1929–1934. doi: 10.1109/61.544278
![]() |
[2] |
López JC, Lavorato M, Rider MJ (2016) Optimal reconfiguration of electrical distribution systems considering reliability indices improvement. Electr Power Energy Syst 78: 837–845. doi: 10.1016/j.ijepes.2015.12.023
![]() |
[3] |
Hajian-Hoseinabadi H, Golshan MEH, Shayanfar HA (2014) Composite automated distribution system reliability model considering various automated substations. Electr Power Energy Syst 54: 211–220. doi: 10.1016/j.ijepes.2013.06.032
![]() |
[4] |
Hashemi Y, Valipour K (2014) FDM based multi-objective optimal sitting and design of TC-FLSFCL for study of distribution system reliability. Electr Power Energy Syst 61: 463–473. doi: 10.1016/j.ijepes.2014.04.002
![]() |
[5] |
Ray S, Bhattacharya A, Bhattacharjee S (2016) Optimal placement of switches in a radial distribution network for reliability improvement. Electr Power Energy Syst 76: 53–68. doi: 10.1016/j.ijepes.2015.09.022
![]() |
[6] |
Saboori H, Hemmati R, Jirdehi MA (2015) Reliability improvement in radial electrical distribution network by optimal planning of energy storage systems. Energy 93: 2299–2312. doi: 10.1016/j.energy.2015.10.125
![]() |
[7] |
Timashev SA, Bushinskaya AV (2015) Markov approach to early diagnostics, reliability assessment, residual life and optimal maintenance of pipeline systems. Struct Saf 56: 68–79. doi: 10.1016/j.strusafe.2015.05.006
![]() |
[8] |
Yssaad B, Abene A (2015) Rational reliability centered maintenance optimization for power distribution systems. Electr Power Energy Syst 73: 350–360. doi: 10.1016/j.ijepes.2015.05.015
![]() |
[9] | Ajenikoko GA, Olaomi AA, Aborisade DO (2014) A failure rate model for reliability assessment of Ibadan distribution systems. Comput Eng Intell Syst, 5: 21–33. |
[10] |
Bourezg A, Meglouli H (2015) Reliability assessment of power distribution systems using disjoint path-set algorithm. J Ind Eng Int 11: 45–57. doi: 10.1007/s40092-014-0083-5
![]() |
[11] | Bertling L, Allan R, Eriksson R (2005) A reliability-centered asset maintenance method for assessing the impact of maintenance in power distribution systems. IEEE Trans Power Syst 20: 75–82. |
[12] |
Wang P, Billinton R (2002) Reliability cost/worth assessment of distribution systems incorporating time-varying weather conditions and restoration resources. IEEE Trans Power Delivery 17: 260–265. doi: 10.1109/61.974216
![]() |
[13] | Brown RE (2001) Distribution reliability assessment and reconfiguration optimization. 2001 IEEE/PES Transmission and Distribution Conference and Exposition. Developing New Perspectives, Atlanta, GA 2: 994–999. |
[14] |
Wang Z, Shokooh F, Qiu J (2002) An efficient algorithm for assessing reliability indexes of general distribution systems. IEEE Trans Power Syst 17: 608–614. doi: 10.1109/TPWRS.2002.800904
![]() |
[15] | Lantharthong T, Phanthuna N (2012) Techniques for reliability evaluation in distribution system planning. WASET, Int J Electr, Comput, Energ, Electron Commun Eng 6. |
[16] |
Billinton R, Wang P (1998) Reliability-network-equivalent approach to distribution-system-reliability evaluation. IEE Proc-Gener, Transm Distrib 145: 149–153. doi: 10.1049/ip-gtd:19981828
![]() |
[17] | Anbalagan P, Ramachandran V (2011) An enhanced distributed model for reliability evaluation of power distribution systems. Int J Comput Electr Eng 3: 566–571. |
[18] |
Al-Muhaini M, Heydt GT (2013) A novel method for evaluating future power distribution system reliability. IEEE Trans Power Syst 28: 3018–3027. doi: 10.1109/TPWRS.2012.2230195
![]() |
[19] |
Li G, Bie Z, Xie H, et al. (2016) Customer satisfaction based reliability evaluation of active distribution networks. Appl Energy 162:1571–1578. doi: 10.1016/j.apenergy.2015.02.084
![]() |
[20] |
Milaca SA, Volpi NMP, Yuan J, et al. (2015) Expansion planning problem in distribution systems with reliability evaluation: An application in real network using geo referenced database. Electr Power Energy Syst 70: 9–16. doi: 10.1016/j.ijepes.2015.01.004
![]() |
[21] |
Salman AM, Li Y, Stewart MG (2015) Evaluating system reliability and targeted hardening strategies of power distribution systems subjected to hurricanes. Reliab Eng Syst Safety 144: 319–333. doi: 10.1016/j.ress.2015.07.028
![]() |
[22] | Tsao TF, Chang HC (2003) Composite reliability evaluation model for different types of distribution systems. IEEE Transactions on Power Systems 18: 924–930. |
[23] |
Ren H, Gao W (2010) A MILP model for integrated plan and evaluation of distributed energy systems. Appl Energy 87: 1001–1014. doi: 10.1016/j.apenergy.2009.09.023
![]() |
[24] | da Silva AML, Cassula AM, Nascimento LC, et al. (2006) Chronological Monte Carlo-Based assessment of distribution system reliability. International Conference on Probabilistic Methods Applied to Power Systems KTH, Stockholm, Sweden. |
[25] | Ge S, Xu L, Liu H, et al. (2014) Reliability assessment of active distribution system using Monte Carlo simulation method. J Appl Math 2014. |
[26] |
Conti S, Rizzo SA (2015) Monte Carlo Simulation by using a systematic approach to assess distribution system reliability considering intentional islanding. IEEE Trans Power Delivery 30: 64–73. doi: 10.1109/TPWRD.2014.2329535
![]() |
[27] | Billinton R, Wang P (1999) Teaching distribution system reliability evaluation using Monte Carlo simulation. IEEE Trans Power Syst 14: 397–403. |
[28] |
Martinez-Velasco JA, Guerra G (2014) Parallel Monte Carlo approach for distribution reliability assessment. IET Gener Transm Distrib 8: 1810–1819. doi: 10.1049/iet-gtd.2014.0075
![]() |
[29] | Zapata CJ, Gomez O (2006) Reliability Assessment of unbalanced distribution systems using sequential Monte Carlo simulation. 2006 IEEE/PES Transmission & Distribution Conference and Exposition: Latin America 1–6. |
[30] | Hussain B, Qadeer-Ul-Hassan (2016) Demand side management for smart homes in Pakistan. 2016 International Conference on Emerging Technologies (ICET), Islamabad 1–6. |
[31] | Meng FL, Zeng XJ (2014) An optimal Real-time pricing for demand side management: A stackelberg game and genetic algorithm approach. International Joint Conference on Neural Networks (IJCNN) Beijing, China. |
[32] | Talha M, Saeed MS, Mohiuddin G, et al. (2018) Energy optimization in home energy management system using artificial fish swarm algorithm and genetic algorithm. In: Barolli L, Woungang I, Hussain O, Advances in Intelligent Networking and Collaborative Systems. INCoS 2017. Lecture Notes on Data Engineering and Communications Technologies, Springer, Cham 8. |
[33] |
Logenthiran T, Srinivasan D, Shun TZ (2012) Demand side management in smart grid using heuristic optimization. IEEE Trans Smart Grid 3: 1244–1252. doi: 10.1109/TSG.2012.2195686
![]() |
[34] | Rahim S, Iqbal Z, Shaheen N, et al. (2016) Ant colony optimization based energy management controller for smart grid. 2016 IEEE 30th International Conference on Advanced Information Networking and Applications (AINA), Crans-Montana 1154–1159. |
[35] |
Costa PM, Matosb MA (2009) Assessing the contribution of micro-grids to the reliability of distribution networks. Electr Power Syst Res 79: 382–389. doi: 10.1016/j.epsr.2008.07.009
![]() |
[36] | Allahnoori M, Kazemi Sh, Abdi H, et al. (2014) Reliability assessment of distribution systems in presence of micro-grids considering uncertainty in generation and load demand. J of Oper Autom Power Eng 2: 113–120. |
[37] |
Conti S, Nicolosi R, Rizzo SA (2012) Generalized systematic approach to assess distribution system reliability with renewable distributed generators and Micro-grids. IEEE Trans Power Delivery 27: 261–270. doi: 10.1109/TPWRD.2011.2172641
![]() |
[38] |
Khodayar ME, Barati M, Shahidehpour M (2012) Integration of high reliability distribution system in microgrid operation. IEEE Trans Smart Grid 3: 1997–2006. doi: 10.1109/TSG.2012.2213348
![]() |
[39] |
Abul'Wafa AR, Taha ATM (2014) Reliability evaluation of distribution systems under μ Grid-Tied andislanded μ grid modes using Monte Carlo simulation. Smart Grid Renewable Energy 5: 52–62. doi: 10.4236/sgre.2014.53006
![]() |
[40] | Tuffaha T, AlMuhaini M (2015) Reliability assessment of a Micro-grid distribution system with PV and storage. 2015 International Symposium on Smart Electric Distribution Systems and Technologies (EDST), Vienna 195–199. |
[41] |
Wang S, Li Z, Wu L, et al. (2013) New metrics for assessing the reliability and economics of microgrids in distribution system. IEEE Trans Power Syst 28: 2852–2861. doi: 10.1109/TPWRS.2013.2249539
![]() |
[42] | Conti S, Rizzo SA, El-Saadany EF, et al. (2014) Reliability assessment of distribution systems considering telecontrolled switches and microgrids. IEEE Trans Power Syst 29: 598–607. |
[43] |
Pretea CL, Hobbsa BF, Normana CS, et al. (2012) Sustainability and reliability assessment of micro grids in a regional electricity market. Energy 41: 192–202. doi: 10.1016/j.energy.2011.08.028
![]() |
[44] | Hegazy YG, Salama MMA, Chickhani AY (2012) Distributed generation and distribution system reliability. Abbasia, Cairo, Egypt. |
[45] | Abdullah AM (2012) New method for assessment of distributed generation impact on distribution system reliability: Islanded operation. IEEE PES Innovative Smart Grid Technol 1–5. |
[46] |
Al-Muhaini M, Heydt GT (2013) Evaluating future power distribution system reliability including distributed generation. IEEE Trans Power Delivery 28: 2264–2272. doi: 10.1109/TPWRD.2013.2253808
![]() |
[47] |
Atwa YM, El-Saadany EF (2009) Reliability evaluation for distribution system with renewable distributed generation during islanded mode of operation. IEEE Trans Power Systems 24: 572–581. doi: 10.1109/TPWRS.2009.2016458
![]() |
[48] | Conti S, Rizzo SA (2015) An algorithm for reliability assessment of distribution systems in presence of distributed generators. Int J Electr Eng Inf 7: 502–516. |
[49] | Duttagupta SS, Singh C (2006) A reliability assessment methodology for distribution systems with distributed generation. 2006 IEEE Power Engineering Society General Meeting. |
[50] | Wang G, Liu Z, Liu N, et al. (2008) Reliability evaluation of distribution system with distributed generation based on Islanding algorithm. 2008 Third International Conference on Electric Utility Deregulation and Restructuring and Power Technologies, Nanjuing 2697–2701. |
[51] | Jahangiri P, Fotuhi-Firuzabad M (2008) Reliability assessment of distribution system with distributed generation. 2008 IEEE 2nd International Power and Energy Conference, Johor Bahru 1551–1556. |
[52] |
Li F (2005) Distributed processing of reliability index assessment and reliability-based network reconfiguration in power distribution systems. IEEE Trans Power Syst 20: 230–238. doi: 10.1109/TPWRS.2004.841231
![]() |
[53] |
Borges CLT (2012) An overview of reliability models and methods for distribution systems with renewable energy distributed generation. Renewable Sustainable Energy Rev 16: 4008–4015. doi: 10.1016/j.rser.2012.03.055
![]() |
[54] | Fotuhi-Firuzabad M, Rajabi-Ghahnavie A (2005) An analytical method to consider DG impacts on distribution system reliability. 2005 IEEE/PES Transmission & Distribution Conference & Exposition: Asia and Pacific, Dalian 1–6. |
[55] |
Chowdhury AA, Agarwal SK, Koval DO (2003) Reliability modeling of distributed generation in conventional distribution systems planning and analysis. IEEE Transactions on Industry Applications 39: 1493–1498. doi: 10.1109/TIA.2003.816554
![]() |
[56] |
Arya R, Choube SC, Arya LD (2012) Reliability evaluation and enhancement of distribution systems in the presence of distributed generation based on standby mode. Electr Power Energy Syst 43: 607–616. doi: 10.1016/j.ijepes.2012.05.045
![]() |
[57] |
Brown HE, Suryanarayanan S, Natarajan SA, et al. (2012) Improving reliability of islanded distribution systems with distributed renewable energy resources. IEEE Trans Smart Grid 3: 2028–2038. doi: 10.1109/TSG.2012.2200703
![]() |
[58] |
Li C, Liu X, Zhang W, et al. (2016) Assessment method and indexes of operating states classification for distribution system with distributed generations. IEEE Trans Smart Grid 7: 481–490. doi: 10.1109/TSG.2015.2402157
![]() |
[59] |
Issicaba D, Lopes JAP, da Rosa MA (2012) Adequacy and security evaluation of distribution systems with distributed generation. IEEE Trans Power Syst 27: 1681–1689. doi: 10.1109/TPWRS.2012.2184563
![]() |
[60] |
Yang H, Xie K, Wai R, et al. (2014) Reliability evaluation of electrical distribution network containing distributed generation using directed-relation-graph. J Electr Eng Technol 9: 1188–1195. doi: 10.5370/JEET.2014.9.4.1188
![]() |
[61] |
Arya R (2016) Ranking of feeder sections of distribution systems for maintenance prioritization accounting distributed generations and loads using diagnostic importance factor (DIF). Electr Power Energy Syst 74: 70–77. doi: 10.1016/j.ijepes.2015.07.011
![]() |
[62] |
Georgilakis PS, Hatziargyriou ND (2013) Optimal distributed generation placement in power distribution networks: Models, methods, and future research. IEEE Trans Power Syst 28: 3420–3428. doi: 10.1109/TPWRS.2012.2237043
![]() |
[63] |
Liu K, Sheng W, Hu L, et al. (2015) Simplified probabilistic voltage stability evaluation considering variable renewable distributed generation in distribution systems. IET Gener Transm Distrib 9: 1464–1473. doi: 10.1049/iet-gtd.2014.0840
![]() |
[64] | Paleti S, Potli M, Yadav MA (2015) Reliability evaluation of distribution system with Hybrid Wind/PV/Pico-Hydro Generation. 2015 International Conference on Advanced Computing and Communication Systems, Coimbatore 1–6. |
[65] |
Chaouachi A, Bompard E, Fulli G, et al. (2016) Assessment framework for EV and PV synergies in emerging distribution systems. Renewable Sustainable Energy Rev 55: 719–728. doi: 10.1016/j.rser.2015.09.093
![]() |
[66] |
Conti S, Raita S (2007) Probabilistic load flow using Monte Carlo techniques for distribution networks with photovoltaic generators. Solar Energy 81: 1473–1481. doi: 10.1016/j.solener.2007.02.007
![]() |
[67] |
DQ Hung, Mithulananthan N, Bansal RC (2014) Integration of PV and BES units in commercial distribution systems considering energy loss and voltage stability. Appl Energy 113: 1162–1170. doi: 10.1016/j.apenergy.2013.08.069
![]() |
[68] |
Shah R, Yan R, Saha TK (2015) Chronological risk assessment approach of distribution system with concentrated solar power plant. IET Renewable Power Gener 9: 629–637. doi: 10.1049/iet-rpg.2014.0262
![]() |
[69] |
Mostafa HA, El-Shatshat R, Salama MMA (2013) Multi-Objective optimization for the operation of an electric distribution system with a large number of single phase solar generators. IEEE Trans Smart Grid 4: 1038–1047. doi: 10.1109/TSG.2013.2239669
![]() |
[70] |
Alam MJE, Muttaqi KM, Sutanto D (2013) Mitigation of rooftop solar PV impacts and evening peak support by managing available capacity of distributed energy storage systems. IEEE Trans Power Syst 28: 3874–3884. doi: 10.1109/TPWRS.2013.2259269
![]() |
[71] |
Atwa YM, El-Saadany EF, Salama MMA, et al. (2011) Adequacy evaluation of distribution system including Wind/Solar DG during different modes of operation. IEEE Trans on Power Syst 26: 1945–1952. doi: 10.1109/TPWRS.2011.2112783
![]() |
[72] |
Yazdani A, Dash PP (2009) A control methodology and characterization of dynamics for a Photovoltaic (PV) system interfaced with a distribution network. IEEE Trans Power Delivery 24: 1538–1551. doi: 10.1109/TPWRD.2009.2016632
![]() |
[73] | Atwa YM, El-Saadany EF, Guise AC, et al. (2010) Supply adequacy assessment of distribution system including Wind-Based DG during different modes of operation. IEEE Trans Power Syst25: 78–86. |
[74] |
Abdelsamad SF, Morsi WG, Sidhu TS (2015) Impact of Wind-Based distributed generation on electric energy in distribution systems embedded with electric vehicles. IEEE Trans Sustainable Energy 6: 79–87. doi: 10.1109/TSTE.2014.2356551
![]() |
[75] | Vallee F, Lobry J, Deblecker O (2008) System reliability assessment method for wind power integration. IEEE Trans Power Syst 23: 1288–1297. |
[76] | Wu H, Guo J, Ding M (2016) Reliability evaluation of a distribution system with wind turbine generators based on the Switch-section partitioning method. J Electr Eng Technol 11: 709–718. |
[77] |
Aghaebrahimi MR, Mehdizadeh M (2011) A new procedure in reliability assessment of Wind–Diesel islanded grids. Electr Power Compon Syst 39: 1563–1576. doi: 10.1080/15325008.2011.596505
![]() |
1. | Marius‐F. Danca, Controlling the dynamics of a COVID‐19 mathematical model using a parameter switching algorithm, 2023, 0170-4214, 10.1002/mma.9014 | |
2. | Mohammad Masum, M.A. Masud, Muhaiminul Islam Adnan, Hossain Shahriar, Sangil Kim, Comparative study of a mathematical epidemic model, statistical modeling, and deep learning for COVID-19 forecasting and management, 2022, 80, 00380121, 101249, 10.1016/j.seps.2022.101249 | |
3. | Javaid Ali, Ali Raza, Nauman Ahmed, Ali Ahmadian, Muhammad Rafiq, Massimiliano Ferrara, Evolutionary optimized Padé approximation scheme for analysis of covid-19 model with crowding effect, 2021, 8, 22147160, 100207, 10.1016/j.orp.2021.100207 | |
4. | N. Raza, A. Bakar, A. Khan, C. Tunç, Numerical Simulations of the Fractional-Order SIQ Mathematical Model of Corona Virus Disease Using the Nonstandard Finite Difference Scheme, 2022, 16, 1823-8343, 391, 10.47836/mjms.16.3.01 | |
5. | Shimelis Bekele Zerefe, Tigabu Kasie Ayele, Surafel Luleseged Tilahun, Mehmet Ünver, Mathematical Modeling of COVID‐19 Disease Dynamics With Contact Tracing: A Case Study in Ethiopia, 2024, 2024, 1110-757X, 10.1155/2024/5556734 | |
6. | Anuradha Bhattacharjee, Kshama Jain, 2024, 3139, 0094-243X, 120002, 10.1063/5.0224325 |