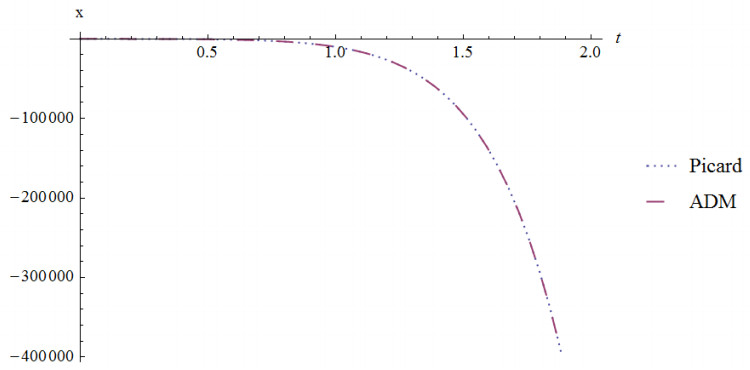
Citation: Wenyuan Xie, Jason Wei Jun Low, Arunmozhiarasi Armugam, Kandiah Jeyaseelan, Yen Wah Tong. Regulation of Aquaporin Z osmotic permeability in ABA tri-block copolymer[J]. AIMS Biophysics, 2015, 2(3): 381-397. doi: 10.3934/biophy.2015.3.381
[1] | Debao Yan . Existence results of fractional differential equations with nonlocal double-integral boundary conditions. Mathematical Biosciences and Engineering, 2023, 20(3): 4437-4454. doi: 10.3934/mbe.2023206 |
[2] | Abdon Atangana, Jyoti Mishra . Analysis of nonlinear ordinary differential equations with the generalized Mittag-Leffler kernel. Mathematical Biosciences and Engineering, 2023, 20(11): 19763-19780. doi: 10.3934/mbe.2023875 |
[3] | Allaberen Ashyralyev, Evren Hincal, Bilgen Kaymakamzade . Crank-Nicholson difference scheme for the system of nonlinear parabolic equations observing epidemic models with general nonlinear incidence rate. Mathematical Biosciences and Engineering, 2021, 18(6): 8883-8904. doi: 10.3934/mbe.2021438 |
[4] | Sebastian Builes, Jhoana P. Romero-Leiton, Leon A. Valencia . Deterministic, stochastic and fractional mathematical approaches applied to AMR. Mathematical Biosciences and Engineering, 2025, 22(2): 389-414. doi: 10.3934/mbe.2025015 |
[5] | Hardik Joshi, Brajesh Kumar Jha, Mehmet Yavuz . Modelling and analysis of fractional-order vaccination model for control of COVID-19 outbreak using real data. Mathematical Biosciences and Engineering, 2023, 20(1): 213-240. doi: 10.3934/mbe.2023010 |
[6] | Barbara Łupińska, Ewa Schmeidel . Analysis of some Katugampola fractional differential equations with fractional boundary conditions. Mathematical Biosciences and Engineering, 2021, 18(6): 7269-7279. doi: 10.3934/mbe.2021359 |
[7] | Jian Huang, Zhongdi Cen, Aimin Xu . An efficient numerical method for a time-fractional telegraph equation. Mathematical Biosciences and Engineering, 2022, 19(5): 4672-4689. doi: 10.3934/mbe.2022217 |
[8] | Yingying Xu, Chunhe Song, Chu Wang . Few-shot bearing fault detection based on multi-dimensional convolution and attention mechanism. Mathematical Biosciences and Engineering, 2024, 21(4): 4886-4907. doi: 10.3934/mbe.2024216 |
[9] | H. M. Srivastava, Khaled M. Saad, J. F. Gómez-Aguilar, Abdulrhman A. Almadiy . Some new mathematical models of the fractional-order system of human immune against IAV infection. Mathematical Biosciences and Engineering, 2020, 17(5): 4942-4969. doi: 10.3934/mbe.2020268 |
[10] | Guodong Li, Ying Zhang, Yajuan Guan, Wenjie Li . Stability analysis of multi-point boundary conditions for fractional differential equation with non-instantaneous integral impulse. Mathematical Biosciences and Engineering, 2023, 20(4): 7020-7041. doi: 10.3934/mbe.2023303 |
Fractional calculus is a main branch of mathematics that can be considered as the generalisation of integration and differentiation to arbitrary orders. This hypothesis begins with the assumptions of L. Euler (1730) and G. W. Leibniz (1695). Fractional differential equations (FDEs) have lately gained attention and publicity due to their realistic and accurate computations [1,2,3,4,5,6,7]. There are various types of fractional derivatives, including Riemann–Liouville, Caputo, Grü nwald–Letnikov, Weyl, Marchaud, and Atangana. This topic's history can be found in [8,9,10,11]. Undoubtedly, fractional calculus applies to mathematical models of different phenomena, sometimes more effectively than ordinary calculus [12,13]. As a result, it can illustrate a wide range of dynamical and engineering models with greater precision. Applications have been developed and investigated in a variety of scientific and engineering fields over the last few decades, including bioengineering [14], mechanics [15], optics [16], physics [17], mathematical biology, electrical power systems [18,19,20] and signal processing [21,22,23].
One of the definitions of fractional derivatives is Caputo-Fabrizo, which adds a new dimension in the study of FDEs. The new derivative's feature is that it has a nonsingular kernel, which is made from a combination of an ordinary derivative with an exponential function, but it has the same supplementary motivating properties with various scales as in the Riemann-Liouville fractional derivatives and Caputo. The Caputo-Fabrizio fractional derivative has been used to solve real-world problems in numerous areas of mathematical modelling for example, numerical solutions for groundwater pollution, the movement of waves on the surface of shallow water modelling [24], RLC circuit modelling [25], and heat transfer modelling [26,27] were discussed.
Rach (1987), Bellomo and Sarafyan (1987) first compared the Adomian Decomposition method (ADM) [28,29,30,31,32] to the Picard method on a variety of examples. These methods have many benefits: they effectively work with various types of linear and nonlinear equations and also provide an analytic solution for all of these equations with no linearization or discretization. These methods are more realistic compared with other numerical methods as each technique is used to solve a specific type of equations, on the other hand ADM and Picard are useful for many types of equations. In the numerical examples provided, we compare ADM and Picard solutions of multidimentional fractional order equations with Caputo-Fabrizio.
The fractional derivative of Caputo-Fabrizio for the function $ x\left(t\right) $ is defined as [33]
$ CFDα0x(t)=B(α)1−α∫t0dds(x(s)) e−α1−α(t−s)ds, $
|
(1.1) |
and its corresponding fractional integral is
$ CFIαx(t)=1−αB(α)x(t)+αB(α)∫t0x (s)ds, 0<α<1, $
|
(1.2) |
where $ x\left(t\right) $ be continuous and differentiable on [0, T]. Also, in the above definition, the function $ B\left(\alpha \right) > 0 $ is a normalized function which satisfy the condition $ B\left(0\right) = B\left(1\right) = 0. $ The relation between the Caputo–Fabrizio fractional derivate and its corresponding integral is given by
$ (CFIα0)(CFDα0f(t))=f(t)−f(a). $
|
(1.3) |
In this section, we will introduce a multidimentional FDE subject to the initial condition. Let $ \alpha \in \; (0, 1] $, $ 0 < \alpha _{1} < \alpha _{2} < ..., \alpha _{m} < 1, $ and $ m $ is integer real number,
$ CFDx=f(t,x,CFDα1x,CFDα2x,...,CFDαmx,) ,x(0)=c0, $
|
(2.1) |
where $ x = x\left(t\right), t\in J = \left[ 0, T\right], T\in R^{+}, x\in C\left(J\right) $.
To facilitate the equation and make it easy for the calculation, we let $ x\left(t\right) = c_{0}+X\left(t\right) $ so Eq (2.1) can be witten as
$ CFDαX=f(t,c0+X,CFDα1X,CFDα2X,...,CFDαmX), X(0)=0. $
|
(2.2) |
the algorithm depends on converting the initial condition from a constant $ c_{0} $ to 0.
Let $ ^{CF}D^{\alpha }X = y\left(t\right) $ then $ X = \; ^{CF}I^{\alpha }y, $ so we have
$ CFDαiX= CFIα−αi CFDαX= CFIα−αiy, i=1,2,...,m. $
|
(2.3) |
Substituting in Eq (2.2) we obtain
$ y=f(t,c0+ CFIαy, CFIα−α1y,..., CFIα−αmy). $
|
(2.4) |
Assume $ f $ satisfies Lipschtiz condition with Lipschtiz constant$ \ L $ given by,
$ |f(t,y0,y1,...,ym)|−|f(t,z0,z1,...,zm)|≤Lm∑i=0|yi−zi|, $
|
(2.5) |
which implies
$ |f(t,c0+CFIαy,CFIα−α1y,..,CFIα−αmy)−f(t,c0+CFIαz,CFIα−α1z,..,CFIα−αmz)|≤Lm∑i=0| CFIα−αiy− CFIα−αiz|. $
|
(2.6) |
The solution algorithm of Eq (2.4) using ADM is,
$ y0(t)=a(t)yn+1(t)=An(t), j⩾0. $
|
(2.7) |
where $ a\left(t\right) $ pocesses all free terms in Eq (2.4) and $ A_{n} $ are the Adomian polynomials of the nonlinear term which takes the form [34]
$ An=f(Sn)−n−1∑i=0Ai, $
|
(2.8) |
where $ f\left(S_{n}\right) = \sum_{i = 0}^{n}A_{i} $. Later, this accelerated formula of Adomian polynomial will be used in convergence analysis and error estimation. The solution of Eq (2.4) can be written in the form,
$ y(t)=∞∑i=0yi(t). $
|
(2.9) |
lastly, the solution of the Eq (2.4) takes the form
$ x(t)=c0+X(t)=c0+ CFIαy(t). $
|
(2.10) |
At which we convert the parameter to the initial form $ y $ to $ x $ in Eq (2.10), so we have the solution of the original Eq (2.1).
Define a mapping $ F:E\rightarrow E $ where $ E = \left(C\left[ J\right], \left\Vert \cdot \right\Vert \right) $ is a Banach space of all continuous functions on $ J $ with the norm $ \left\Vert x\right\Vert = \underset{t\epsilon J}{\text{ }\max\limits } \; x\left(t\right) $.
Theorem 3.1. Equation (2.4) has a unique solution whenever $ 0 < \phi < 1 $ where $ \phi = L\left(\sum_{i = 0}^{m}\frac{\left[ \left(\alpha-\alpha _{i}\right) \left(T-1\right) \right] +1}{B\left(\alpha -\alpha_{i}\right) }\right) $.
Proof. First, we define the mapping $ F:E\rightarrow E $ as
$ Fy=f(t,c0+ CFIαy, CFIα−α1y,..., CFIα−αmy). $
|
Let $ y $ and $ z\in E $ are two different solutions of Eq (2.4). Then
$ Fy−Fz=f(t,c0+CFIαy,CFIα−α1y,..,CFIα−αmy)−f(t,c0+CFIαz,CFIα−α1z,...,CFIα−αmz) $
|
which implies that
$ |Fy−Fz|=|f(t,c0+ CFIαy, CFIα−α1y,..., CFIα−αmy)−f(t,c0+ CFIαz, CFIα−α1z,..., CFIα−αmz)|≤Lm∑i=0| CFIα−αiy− CFIα−αiz|≤Lm∑i=0|1−(α−αi)B(α−αi)(y−z)+α−αiB(α−αi)∫t0(y−z)ds|‖Fy−Fz‖≤Lm∑i=01−(α−αi)B(α−αi)maxtϵJ|y−z|+α−αiB(α−αi)maxtϵJ|y−z|∫t0ds≤Lm∑i=01−(α−αi)B(α−αi)‖y−z‖+α−αiB(α−αi)‖y−z‖T≤L‖y−z‖(m∑i=01−(α−αi)B(α−αi)+α−αiB(α−αi)T)≤L‖y−z‖(m∑i=0[(α−αi)(T−1)]+1B(α−αi))≤ϕ‖y−z‖. $
|
under the condition $ 0 < \phi < 1, $ the mapping $ F $ is contraction and hence there exists a unique solution $ y\in C\left[ J\right] $ for the problem Eq (2.4) and this completes the proof.
Theorem 3.2. The series solution of the problem Eq (2.4)converges if $ \left\vert y_{1}\left(t\right) \right\vert < c $ and $ c $ isfinite.
Proof. Define a sequence $ \left\{ S_{p}\right\} $ such that $ S_{p} = \sum_{i = 0}^{p}y_{i}\left(t\right) $ is the sequence of partial sums from the series solution $ \sum_{i = 0}^{\infty }y_{i}\left(t\right), $ we have
$ f(t,c0+ CFIαy, CFIα−α1y,..., CFIα−αmy)=∞∑i=0Ai, $
|
So
$ f(t,c0+ CFIαSp, CFIα−α1Sp,..., CFIα−αmSp)=p∑i=0Ai, $
|
From Eq (2.7) we have
$ ∞∑i=0yi(t)=a(t)+∞∑i=0Ai−1 $
|
let $ S_{p}, S_{q} $ be two arbitrary sums with $ p\geqslant q $. Now, we are going to prove that $ \left\{ S_{p}\right\} $ is a Caushy sequence in this Banach space. We have
$ Sp=p∑i=0yi(t)=a(t)+p∑i=0Ai−1,Sq=q∑i=0yi(t)=a(t)+q∑i=0Ai−1. $
|
$ Sp−Sq=p∑i=0Ai−1−q∑i=0Ai−1=p∑i=q+1Ai−1=p−1∑i=qAi−1=f(t,c0+ CFIαSp−1, CFIα−α1Sp−1,..., CFIα−αmSp−1)−f(t,c0+ CFIαSq−1, CFIα−α1Sq−1,..., CFIα−αmSq−1) $
|
$ |Sp−Sq|=|f(t,c0+ CFIαSp−1, CFIα−α1Sp−1,..., CFIα−αmSp−1)−f(t,c0+ CFIαSq−1, CFIα−α1Sq−1,..., CFIα−αmSq−1)|≤Lm∑i=0| CFIα−αiSp−1− CFIα−αiSq−1|≤Lm∑i=0|1−(α−αi)B(α−αi)(Sp−1−Sq−1)+α−αiB(α−αi)∫t0(Sp−1−Sq−1)ds|≤Lm∑i=01−(α−αi)B(α−αi)|Sp−1−Sq−1|+α−αiB(α−αi)∫t0|Sp−1−Sq−1|ds $
|
$ ‖Sp−Sq‖≤Lm∑i=01−(α−αi)B(α−αi)maxtϵJ|Sp−1−Sq−1|+α−αiB(α−αi)maxtϵJ|Sp−1−Sq−1|∫t0ds≤L‖Sp−Sq‖m∑i=0(1−(α−αi)B(α−αi)+α−αiB(α−αi)T)≤L‖Sp−Sq‖(m∑i=0[(α−αi)(T−1)]+1B(α−αi))≤ϕ‖Sp−Sq‖ $
|
let $ p = q+1 $ then,
$ ‖Sq+1−Sq‖≤ϕ‖Sq−Sq−1‖≤ϕ2‖Sq−1−Sq−2‖≤...≤ϕq‖S1−S0‖ $
|
From the triangle inequality we have
$ ‖Sp−Sq‖≤‖Sq+1−Sq‖+‖Sq+2−Sq+1‖+...‖Sp−Sp−1‖≤[ϕq+ϕq+1+...+ϕp−1]‖S1−S0‖≤ϕq[1+ϕ+...+ϕp−q+1]‖S1−S0‖≤ϕq[1−ϕp−q1−ϕ]‖y1(t)‖ $
|
Since $ 0 < \phi < 1, p\geqslant q $ then $ \left(1-\phi ^{p-q}\right) \leq 1 $. Consequently
$ ‖Sp−Sq‖≤ϕq1−ϕ‖y1(t)‖≤ϕq1−ϕmax∀tϵJ|y1(t)| $
|
(3.1) |
but $ \left\vert y_{1}\left(t\right) \right\vert < \infty $ and as $ q\rightarrow \infty $ then, $ \left\Vert S_{p}-S_{q}\right\Vert \rightarrow 0 $ and hence, $ \left\{ S_{p}\right\} $ is a Caushy sequence in this Banach space then the proof is complete.
Theorem 3.3. The maximum absolute truncated error Eq (2.4)is estimated to be $ \underset{t\epsilon J}{\max }\left\vert y\left(t\right)-\sum_{i = 0}^{q}y_{i}\left(t\right) \right\vert \leq \frac{\phi ^{q}}{1-\phi }\underset{t\epsilon J}{\max }\left\vert y_{1}\left(t\right) \right\vert $
Proof. From the convergence theorm inequality (Eq 3.1) we have
$ ‖Sp−Sq‖≤ϕq1−ϕmaxtϵJ|y1(t)| $
|
but, $ S_{p} = \sum_{i = 0}^{p}y_{i}\left(t\right) $ as $ p\rightarrow \infty $ then, $ S_{p}\rightarrow y\left(t\right) $ so,
$ ‖y(t)−Sq‖≤ϕq1−ϕmaxtϵJ|y1(t)| $
|
so, the maximum absolute truncated error in the interval $ J $ is,
$ maxtϵJ|y(t)−q∑i=0yi(t)|≤ϕq1−ϕmaxtϵJ|y1(t)| $
|
(3.2) |
and this completes the proof.
In this part, we introduce several numerical examples with unkown exact solution and we will use inequality (Eq 3.2) to estimate the maximum absolute truncated error.
Example 4.1. Application of linear FDE
$ CFDx(t)+2aCFD1/2x(t)+bx(t)=0, x(0)=1. $
|
(4.1) |
A Basset problem in fluid dynamics is a classical problem which is used to study the unsteady movement of an accelerating particle in a viscous fluid under the action of the gravity [36]
Set
$ X(t)=x(t)−1 $
|
Equation (4.1) will be
$ CFDX(t)+2aCFD1/2X(t)+bX(t)=0, X(0)=0. $
|
(4.2) |
Appling Eq (2.3) to Eq (4.2), and using initial condition, also we take a = 1, b = 1/2,
$ y=−12−2I1/2y−12I y $
|
(4.3) |
Appling ADM to Eq (4.3), we find the solution algorithm become
$ y0(t)=−12,yi(t)=−2 CFI1/2yi−1−12 CFI yi−1, i≥1. $
|
(4.4) |
Appling Picard solution to Eq (4.2), we find the solution algorithm become
$ y0(t)=−12,yi(t)=−12−2 CFI1/2yi−1−12 CFI yi−1, i≥1. $
|
(4.5) |
From Eq (4.4), the solution using ADM is given by $ y\left(t\right) = \underset{q\rightarrow \infty }{Lim}{_{i = 0}^{q} y_{i}} \left(t\right) $ while from Eq (4.5), the solution using Picard technique is given by $ y\left(t\right) = \; \underset{i\rightarrow \infty }{ Lim} \; y_{i}\left(t\right) $. Lately, the solution of the original problem Eq (4.2), is
$ x(t)=1+ CFI y(t). $
|
One the same processor (q = 20), the time consumed using ADM is 0.037 seconds, while the time consumed using Picard is 7.955 seconds.
Figure 1 gives a comparison between ADM and Picard solution of Ex. 4.1.
Example 4.2. Consider the following nonlinear FDE [35]
$ CFD1/2x=8t3/23√π−t7/44Γ(114)−t44+18 CFD1/4x+14x2, x(0)=0. $
|
(4.6) |
Appling Eq (2.3) to Eq (4.6), and using initial condition,
$ y=8t3/23√π−t7/44Γ(114)−t44+18 CFI1/4y+14(CFI1/2y)2. $
|
(4.7) |
Appling ADM to Eq (4.7), we find the solution algorithm will be become
$ y0(t)=8t3/23√π−t7/44Γ(114)−t44,yi(t)=18 CFI1/4yi−1+14(Ai−1), i≥1. $
|
(4.8) |
at which A$ _{\text{i}} $ are Adomian polynomial of the nonliner term $ \left(^{CF}I^{1/2}y\right) ^{2}. $
Appling Picard solution to Eq (4.7), we find the the solution algorithm become
$ y0(t)=8t3/23√π−t7/44Γ(114)−t44,yi(t)=y0(t)+18 CFI1/4yi−1+14(CFI1/2yi−1)2, i≥1. $
|
(4.9) |
From Eq (4.8), the solution using ADM is given by $ y\left(t\right) = \underset{q\rightarrow \infty }{Lim}{_{i = 0}^{q}y_{i}} \left(t\right) $ while from Eq (4.9), the solution using Picard technique is given by $ y\left(t\right) = \underset{i\rightarrow \infty }{Lim} y_{i}\left(t\right) $. Finally, the solution of the original problem Eq (4.7), is.
$ x(t)= CFI1/2y. $
|
One the same processor (q = 2), the time consumed using ADM is 65.13 seconds, while the time consumed using Picard is 544.787 seconds.
Table 1 showed the maximum absolute truncated error of of ADM solution (using Theorem 3.3) at different values of m (when t = 0:5; N = 2):
q | max. absolute error |
2 | 0.114548 |
5 | 0.099186 |
10 | 0.004363 |
Figure 2 gives a comparison between ADM and Picard solution of Ex. 4.2.
Example 4.3. Consider the following nonlinear FDE [35]
$ CFDαx=3t2−128125πt5+110(CFD1/2x)2,x(0)=0. $
|
(4.10) |
Appling Eq (2.3) to Eq (4.10), and using initial condition,
$ y=3t2−128125πt5+110(CFI1/2y)2 $
|
(4.11) |
Appling ADM to Eq (4.11), we find the solution algorithm become
$ y0(t)=3t2−128125πt5,yi(t)=110(Ai−1), i≥1 $
|
(4.12) |
at which A$ _{\text{i}} $ are Adomian polynomial of the nonliner term $ \left(^{CF}I^{1/2}y\right) ^{2}. $
Then appling Picard solution to Eq (4.11), we find the solution algorithm become
$ y0(t)=3t2−128125πt5,yi(t)=y0(t)+110(CFI1/2yi−1)2, i≥1. $
|
(4.13) |
From Eq (4.12), the solution using ADM is given by $ y\left(t\right) = \underset{q\rightarrow \infty }{Lim}{_{i = 0}^{q}y_{i}} \left(t\right) $ while from Eq (4.13), the solution is $ y\left(t\right) = \underset{i\rightarrow \infty }{Lim}y_{i}\left(t\right) $. Finally, the solution of the original problem Eq (4.11), is
$ x(t)=CFIy(t). $
|
One the same processor (q = 4), the time consumed using ADM is 2.09 seconds, while the time consumed using Picard is 44.725 seconds.
Table 2 showed the maximum absolute truncated error of of ADM solution (using Theorem 3.3) at different values of m (when t = 0:5; N = 4):
q | max. absolute error |
2 | 0.00222433 |
5 | 0.0000326908 |
10 | 2.88273*10$ ^{-8} $ |
Figure 3 gives a comparison between ADM and Picard solution of Ex. 4.3 with $ \alpha = 1 $.
Example 4.4. Consider the following nonlinear FDE [35]
$ CFDαx=t2+12 CFDα1x+14 CFDα2x+16 CFDα3x+18x4,x(0)=0. $
|
(4.14) |
Appling Eq (2.3) to Eq (4.10), and using initial condition,
$ y=t2+12(CFIα−α1y)+14(CFIα−α2y)+16(CFIα−α3y)+18(CFIαy)4, $
|
(4.15) |
Appling ADM to Eq (4.15), we find the solution algorithm become
$ y0(t)=t2,yi(t)=12(CFIα−α1y)+14(CFIα−α2y)+16(CFIα−α3y)+18Ai−1, i≥1 $
|
(4.16) |
where A$ _{\text{i}} $ are Adomian polynomial of the nonliner term $ \left(^{CF}I^{\alpha }y\right) ^{4}. $
Then appling Picard solution to Eq (4.15), we find the solution algorithm become
$ y_{0}\left( t\right) = t^{2}, \\ y_{i}\left( t\right) = t^{2}+\frac{1}{2}\left( ^{CF}I^{\alpha -\alpha _{1}}y_{i-1}\right) +\frac{1}{4}\left( ^{CF}I^{\alpha -\alpha _{2}}y_{i-1}\right) \\+\frac{1}{6}\left( ^{CF}I^{\alpha -\alpha 3}y_{i-1}\right) +\frac{1}{8}\left( ^{CF}I^{\alpha }y_{i-1}\right) ^{4}\ \ \ \ \ i\geq 1. $ | (4.17) |
From Eq (4.16), the solution using ADM is given by $ y\left(t\right) = \underset{q\rightarrow \infty }{Lim}{_{i = 0}^{q}y_{i}} \left(t\right) $ while from Eq (4.17), the solution using Picard technique is $ y\left(t\right) = \underset{i\rightarrow \infty }{Lim} y_{i}\left(t\right) $. Finally, the solution of the original problem Eq (4.14), is
$ x(t)=CFIαy(t). $
|
One the same processor (q = 3), the time consumed using ADM is 0.437 seconds, while the time consumed using Picard is (16.816) seconds. Figure 4 shows a comparison between ADM and Picard solution of Ex. 4.4 $ at \; \alpha = 0.7, \; \alpha _{1} = 0.1, \alpha _{2} = 0.3, \alpha _{3} = 0.5. $
The Caputo-Fabrizo fractional deivative has a nonsingular kernel, and consequently, this definition is appropriate in solving nonlinear multidimensional FDE [37,38]. Since the selected numerical problems have an unkown exact solution, the formula (3.2) can be used to estimate the maximum absolute truncated error. By comparing the time taken on the same processor (i7-2670QM), it was found that the time consumed by ADM is much smaller compared with the Picard technique. Furthermore Picard gives a more accurate solution than ADM at the same interval with the same number of terms.
The authors declare there is no conflict of interest.
[1] |
Kumar M, Grzelakowski M, Zilles J, et al. (2007) Highly permeable polymeri membranes based on the incorporation of the functional water channel protein Aquaporin Z. PNAS 104: 20719-20724. doi: 10.1073/pnas.0708762104
![]() |
[2] | Service RF (2006) Desalination freshens up. Science 313:1088-1090. |
[3] | Tal A (2006) Seeking sustainability: Israel's evolving water management strategy. Science 313: 1081-1084. |
[4] |
Discher BM, Won YY, Ege DS, et al. (1999) Polymersomes: tough vesicles made from diblock copolymers. Science 284:1143-1146. doi: 10.1126/science.284.5417.1143
![]() |
[5] |
Calamita G, Kempf B, Rudd KE, et al. (1997) The aquaporin-Z water channel gene of Escherichia coli: Structure, organization and phylogeny. Biology of the Cell 89: 321-329. doi: 10.1111/j.1768-322X.1997.tb01029.x
![]() |
[6] |
Calamita G, Bishai WR, Preston GM, et al. (1995) Molecular cloning and characterization of AqpZ, a water channel from Escherichia coli. J Biol Chem 270: 29063-29066. doi: 10.1074/jbc.270.49.29063
![]() |
[7] | Scheuring S, Ringler P, Borgnia M, et al. (1999) High resultion AFM topographs of the Escherichia coli water channel aquaporin Z. EMBO J 18: 4981-4987. |
[8] | Gorin MB, Yancey SB, Cline J, et al. (1984) The major intrinsic protein (MIP) of the bovine lens fiber membrane: Characterization and structure based on cDNA cloning. Cell 39: 49-59. |
[9] |
Ishibashi K, Kuwahara M, Gu Y, et al. (1997) Cloning and functional expression of a new water channel abundantly expressed in the testis permeable to water, glycerol and urea. J Biol Chem 272: 20782-20786. doi: 10.1074/jbc.272.33.20782
![]() |
[10] | Soupene E, King N, Lee H, et al. (2001) Aquaporin Z of Escherichia coli: Reassessment of Its Regulation and Physiological Role. J Bacter 184: 4304-4307. |
[11] |
Calamita G, Kempf B, Bonhivers B, et al. (1998) Regulation of the Escherichia coli water channel gene AqpZ. Proc Natl Acad Sci U S A 95: 3627-3631. doi: 10.1073/pnas.95.7.3627
![]() |
[12] | Borgnia MJ, Kozono D, Calamita G, et al. (1999) Funcation Reconstitution and Characterization of AqpZ, the E. coli Water Channel Protein. J Mol Biol 291: 1169-1179. |
[13] |
Kozono D, Yasui M, King LS, et al. (2002). Aquaporin water channels: atomic structure molecular dynamics meet clinical medicine. J Clin Inves 109: 1395-1399. doi: 10.1172/JCI0215851
![]() |
[14] |
Nemeth-Cahalan KL, Hall JE (2000) pH and Calcium Regulate the Water Permeability of Aquaporin 0. J Biol Chem 275: 6777-6782. doi: 10.1074/jbc.275.10.6777
![]() |
[15] | Cahalan K, Kalman K, Hall JE (2004) Molecular Basis of pH and Ca2+ Regulation of Aquaporin Water Permeability. J Gen Physiol 123: 573-580 |
[16] | Zhou W, Jones SW (1996) The effects of external pH on calcium channel currents in bullfrog sympathetic neurons. Biophys J 70: 1326-1334 |
[17] | Gonen T, Walz T (2006) The structure of aquaporins. Q Rev Biophys 39: 361-396. |
[18] |
Chaumont F, Moshelion F, Daniels MJ (2005) Regulation of plant aquaporin activity. Biol Cell 97: 749-764. doi: 10.1042/BC20040133
![]() |
[19] |
Tong J, Canty JT, Briggs MM, et al. (2013) The water permeability of lens aquaporin-0 depends on its lipid bilayer environment. Exp Eye Res 113: 32-40. doi: 10.1016/j.exer.2013.04.022
![]() |
[20] |
Andersen OS, Bruno MJ, Sun H, et al. (2007) Single-molecule methods for monitoring changes in bilayer elastic properties. Meth Mol Biol 400: 543-570 doi: 10.1007/978-1-59745-519-0_37
![]() |
[21] |
Hong H, Tamm LK (2004) Elastic coupling of integral membrane protein stability to lipid bilayer forces. Proc Natl Acad Sci U S A 101: 4065-4070. doi: 10.1073/pnas.0400358101
![]() |
[22] |
Nyholm TK, Ozdirekcan S, Killian JA (2007) How protein transmembrane segments sense the lipid environment. Biochemistry 46: 1457-1465. doi: 10.1021/bi061941c
![]() |
[23] |
Phillips R, Ursell T, Wiggins P, et al. (2009) Emerging roles for lipids in shaping membrane-protein function. Nature 459: 379-385. doi: 10.1038/nature08147
![]() |
[24] | Yuan C, O'Connell RJ, Jacob RF, et al. (2007) Regulation of the gating of BKCa channel by lipid bilayer thickness. J Biol Chem 282: 7276-7286. |
[25] |
Dumas F, Tocanne JF, Leblanc G, et al. (2000) Consequences of hydrophobic mismatch between lipids and melibiose permease on melibiose transport. Biochem 39: 4846-4854. doi: 10.1021/bi992634s
![]() |
[26] |
Perozo E, Kloda A, Cortes DM, et al. (2002) Physical principles underlying the transduction of bilayer deformation forces during mechano senditive channel gating. Nat Struct Biol 9: 696-703. doi: 10.1038/nsb827
![]() |
[27] |
Xie W, He F, Wang B, et al. (2013) An aquaporin-based vesicle-embedded polymeric membrane for low energy water filtration. J Mater Chem A 1: 7592-7600. doi: 10.1039/c3ta10731k
![]() |
[28] | Wang H, Chung TS, Tong YW, et al. (2011) Preparation and characterization of pore-suspending biomimetic membranes embedded with Aquaporin Z on carboxylated polyethylene glycol polymer cushion. Soft Matter 7: 7274-7280. |
[29] | Wang H, Chung TS, Tong YW, et al. (2012) Highly permeable and selective pore-spanning biomimetic membrane embedded with aquaporin Z. Small 8: 1185-1190, 1125. |
[30] | Duong PHH, Chung TS, Jeyaseelan K, et al. (2012) Planar biomimetic aquaporin-incorporated triblock copolymer membranes on porous alumina supports for nanofiltration. J Membr Sci 409: 34-43. |
[31] | Zhong PS, Chung TS, Jeyaseelan K, et al. (2012) Aquaporin-embedded biomimetic membranes for nanofiltration. J Membr Sci 407: 27-33. |
[32] | Savage DF, Egea PF, Colmenares YR, et al. (2013) Architecture and selectivity in aquaporins: 2.5A X-Ray Structure of Aquaporin Z. PLoS Biol 1: 334-340. |
[33] |
Nielsen CH (2009) Biomimetic membranes for sensor and separation applications. Bioanal Chem 395: 697-718. doi: 10.1007/s00216-009-2960-0
![]() |
[34] | Discher DE, Eisenberg A(2002) Polymer vesicles. Science 297: 967-973. |
[35] |
Ahmed F, Photos PJ, Discher DE (2006) Polymersomes as viral capsid mimics. Drug Develop Res 67: 4-14. doi: 10.1002/ddr.20062
![]() |
[36] |
Lewis BA, Engelman DM (1983) Lipid Bilayer Thickness Varies Linearly with Acyl Chain Length in Fluid Phosphatidylcholine Vesicles. J Mol Biol 166: 211-217. doi: 10.1016/S0022-2836(83)80007-2
![]() |
[37] |
Dave PC, Tiburu EK, Damodaran K, et al. (2004) Investigating Structural Changes in the Lipid Bilayer upon Insertion of the Transmembrane Domain of the Membrane-Bound Protein Phospholamban Utilizing 31P and 2H Solid-State NMR Spectroscopy. Biophys J 86: 1564-1573. doi: 10.1016/S0006-3495(04)74224-1
![]() |
[38] | Marsh D (2008) Energetics of Hydrophobic Matching in Lipid-Protein Interactions. Biophys J 94: 3996-4013. |
[39] |
Xu Q, Kim M, David Ho KW, et al. (2008) Membrane Hydrocarbon Thickness Modulates the Dynamics of a Membrane Transport Protein. Biophys J 95: 2849-2858. doi: 10.1529/biophysj.108.133629
![]() |
[40] |
He F, Tong YW (2014) A mechanistic study on amphiphilic block co-polymer poly(butadiene-b-(ethylene oxide)) vesicles reveals the water permeation mechanism through a polymeric bilayer. RSC Adv 4: 15304-15313. doi: 10.1039/c3ra48063a
![]() |
[41] |
Yang B, Verkman AS (1997) Water and Glycerol Permeabilities of Aquaporins 1-5 and MIP Determined Quantitatively by Expression of Epitope-tagged Constructs in Xenopus Oocytes. J Biol Chem 272: 16140-16146. doi: 10.1074/jbc.272.26.16140
![]() |
[42] |
Mehdizadeh H, Dickson JM, Eriksson PK (1989) Temperature effects on the performance of thin-film composite, aromatic polyamide membranes. Ind Eng Chem Res 28: 814-824. doi: 10.1021/ie00090a025
![]() |
1. | Eman A. A. Ziada, Salwa El-Morsy, Osama Moaaz, Sameh S. Askar, Ahmad M. Alshamrani, Monica Botros, Solution of the SIR epidemic model of arbitrary orders containing Caputo-Fabrizio, Atangana-Baleanu and Caputo derivatives, 2024, 9, 2473-6988, 18324, 10.3934/math.2024894 | |
2. | H. Salah, M. Anis, C. Cesarano, S. S. Askar, A. M. Alshamrani, E. M. Elabbasy, Fourth-order differential equations with neutral delay: Investigation of monotonic and oscillatory features, 2024, 9, 2473-6988, 34224, 10.3934/math.20241630 | |
3. | Said R. Grace, Gokula N. Chhatria, S. Kaleeswari, Yousef Alnafisah, Osama Moaaz, Forced-Perturbed Fractional Differential Equations of Higher Order: Asymptotic Properties of Non-Oscillatory Solutions, 2024, 9, 2504-3110, 6, 10.3390/fractalfract9010006 | |
4. | A.E. Matouk, Monica Botros, Hidden chaotic attractors and self-excited chaotic attractors in a novel circuit system via Grünwald–Letnikov, Caputo-Fabrizio and Atangana-Baleanu fractional operators, 2025, 116, 11100168, 525, 10.1016/j.aej.2024.12.064 | |
5. | Zahra Barati, Maryam Keshavarzi, Samaneh Mosaferi, Anatomical and micromorphological study of Phalaris (Poaceae) species in Iran, 2025, 68, 1588-4082, 9, 10.14232/abs.2024.1.9-15 |
q | max. absolute error |
2 | 0.114548 |
5 | 0.099186 |
10 | 0.004363 |
q | max. absolute error |
2 | 0.00222433 |
5 | 0.0000326908 |
10 | 2.88273*10$ ^{-8} $ |