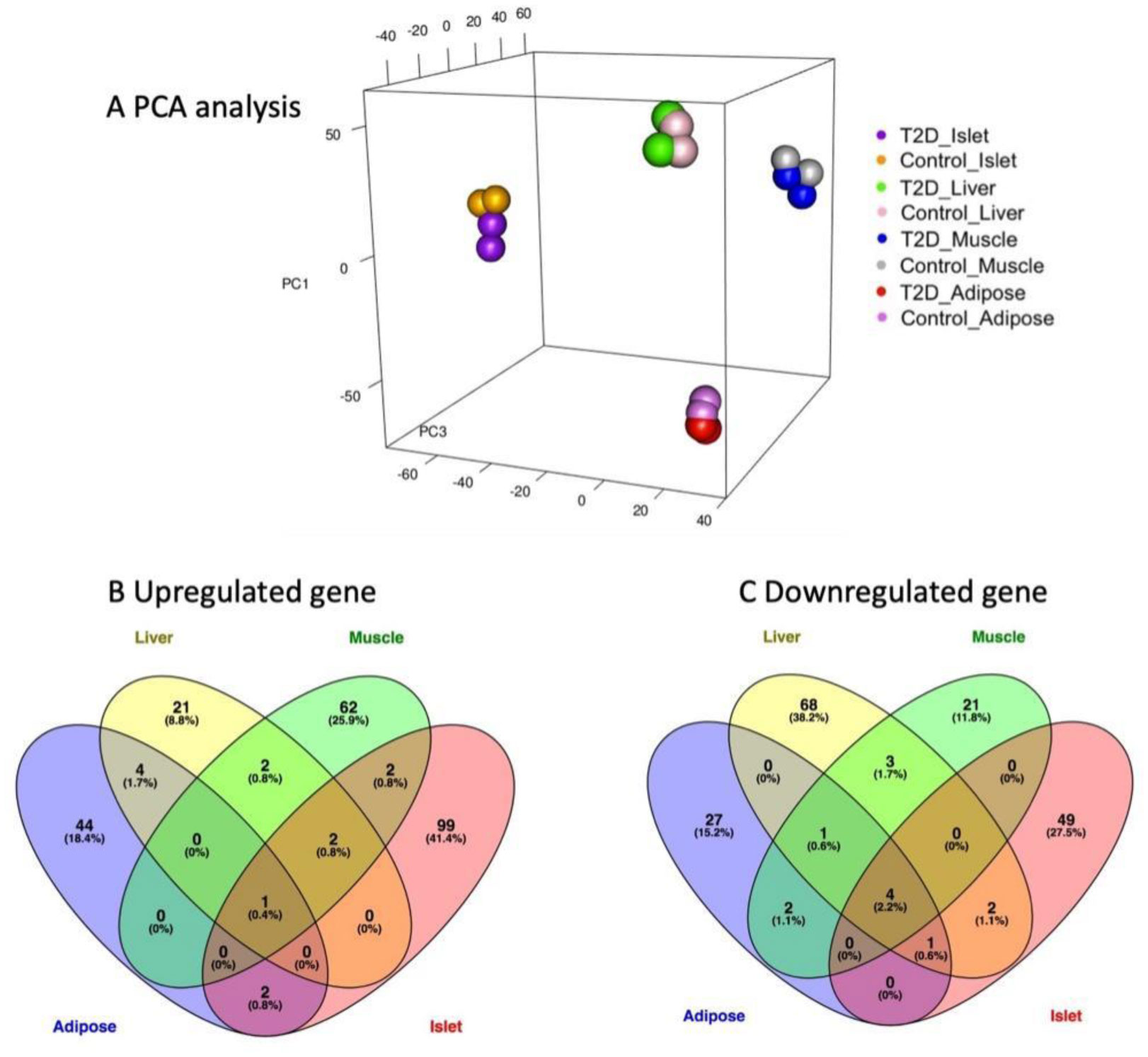
Type 2 diabetes (T2D) is a major global health problem often caused by the inability of pancreatic islets to compensate for the high insulin demand due to apoptosis. However, the complex mechanisms underlying the activation of apoptosis and its counter process, anti-apoptosis, during T2D remain unclear. In this study, we employed bioinformatics and systems biology approaches to understand the anti-apoptosis-associated gene expression and the biological network in the pancreatic islets of T2D mice. First, gene expression data from four peripheral tissues (islets, liver, muscle and adipose) were used to identify differentially expressed genes (DEGs) in T2D compared to non-T2D mouse strains. Our comparative analysis revealed that Gm2036 is upregulated across all four tissues in T2D and is functionally associated with increased cytosolic Ca2+ levels, which may alter the signal transduction pathways controlling metabolic processes. Next, our study focused on islets and performed functional enrichment analysis, which revealed that upregulated genes are significantly associated with sucrose and fructose metabolic processes, as well as negative regulation of neuron apoptosis. Using the Ingenuity Pathway Analysis (IPA) tool of QIAGEN, gene regulatory networks and their biological effects were analyzed, which revealed that glucose is associated with the underlying change in gene expression in the islets of T2D; and an activated gene regulatory network—containing upregulated CCK, ATF3, JUNB, NR4A1, GAST and downregulated DPP4—is possibly inhibiting apoptosis of islets and β-cells in T2D. Our computational-based study has identified a putative regulatory network that may facilitate the survival of pancreatic islets in T2D; however, further validation in a larger sample size is needed. Our results provide valuable insights into the underlying mechanisms of T2D and may offer potential targets for developing more efficacious treatments.
Citation: Firoz Ahmed. Deciphering the gene regulatory network associated with anti-apoptosis in the pancreatic islets of type 2 diabetes mice using computational approaches[J]. AIMS Bioengineering, 2023, 10(2): 111-140. doi: 10.3934/bioeng.2023009
[1] | Xuemei XIA, Xuemei HU . Identification of diagnostic biomarkers of gestational diabetes mellitus based on transcriptome gene expression and alternations of microRNAs. AIMS Bioengineering, 2023, 10(3): 202-217. doi: 10.3934/bioeng.2023014 |
[2] | Islam Uddin, Salman A. AlQahtani, Sumaiya Noor, Salman Khan . Deep-m6Am: a deep learning model for identifying N6, 2′-O-Dimethyladenosine (m6Am) sites using hybrid features. AIMS Bioengineering, 2025, 12(1): 145-161. doi: 10.3934/bioeng.2025006 |
[3] | Xiao-Li Gu, Li Yu, Yu Du, Xiu-Peng Yang, Yong-Gang Xu . Identification and validation of aging-related genes and their classification models based on myelodysplastic syndromes. AIMS Bioengineering, 2023, 10(4): 440-465. doi: 10.3934/bioeng.2023026 |
[4] | Vered Tzin, Ilana Rogachev, Sagit Meir, Michal Moyal Ben Zvi, Tania Masci, Alexander Vainstein, Asaph Aharoni, Gad Galili . Altered Levels of Aroma and Volatiles by Metabolic Engineering of Shikimate Pathway Genes in Tomato Fruits. AIMS Bioengineering, 2015, 2(2): 75-92. doi: 10.3934/bioeng.2015.2.75 |
[5] | Marianthi Logotheti, Eleftherios Pilalis, Nikolaos Venizelos, Fragiskos Kolisis, Aristotelis Chatziioannou . Development and validation of a skin fibroblast biomarker profile for schizophrenic patients. AIMS Bioengineering, 2016, 3(4): 552-565. doi: 10.3934/bioeng.2016.4.552 |
[6] | Ozgun Firat Duzenli, Sezer Okay . Promoter engineering for the recombinant protein production in prokaryotic systems. AIMS Bioengineering, 2020, 7(2): 62-81. doi: 10.3934/bioeng.2020007 |
[7] | Rabia Aziz, C.K. Verma, Namita Srivastava . Dimension reduction methods for microarray data: a review. AIMS Bioengineering, 2017, 4(1): 179-197. doi: 10.3934/bioeng.2017.1.179 |
[8] | Pawel Jajesniak, Tuck Seng Wong . From genetic circuits to industrial-scale biomanufacturing: bacterial promoters as a cornerstone of biotechnology. AIMS Bioengineering, 2015, 2(3): 277-296. doi: 10.3934/bioeng.2015.3.277 |
[9] | Khalil Essani, Anton Glieder, Martina Geier . Combinatorial pathway assembly in yeast. AIMS Bioengineering, 2015, 2(4): 423-436. doi: 10.3934/bioeng.2015.4.423 |
[10] | Nguyen Hoai Nguyen . Histone variant H2A.Z and transcriptional activators may antagonistically regulate flavonoid biosynthesis. AIMS Bioengineering, 2020, 7(1): 55-59. doi: 10.3934/bioeng.2020005 |
Type 2 diabetes (T2D) is a major global health problem often caused by the inability of pancreatic islets to compensate for the high insulin demand due to apoptosis. However, the complex mechanisms underlying the activation of apoptosis and its counter process, anti-apoptosis, during T2D remain unclear. In this study, we employed bioinformatics and systems biology approaches to understand the anti-apoptosis-associated gene expression and the biological network in the pancreatic islets of T2D mice. First, gene expression data from four peripheral tissues (islets, liver, muscle and adipose) were used to identify differentially expressed genes (DEGs) in T2D compared to non-T2D mouse strains. Our comparative analysis revealed that Gm2036 is upregulated across all four tissues in T2D and is functionally associated with increased cytosolic Ca2+ levels, which may alter the signal transduction pathways controlling metabolic processes. Next, our study focused on islets and performed functional enrichment analysis, which revealed that upregulated genes are significantly associated with sucrose and fructose metabolic processes, as well as negative regulation of neuron apoptosis. Using the Ingenuity Pathway Analysis (IPA) tool of QIAGEN, gene regulatory networks and their biological effects were analyzed, which revealed that glucose is associated with the underlying change in gene expression in the islets of T2D; and an activated gene regulatory network—containing upregulated CCK, ATF3, JUNB, NR4A1, GAST and downregulated DPP4—is possibly inhibiting apoptosis of islets and β-cells in T2D. Our computational-based study has identified a putative regulatory network that may facilitate the survival of pancreatic islets in T2D; however, further validation in a larger sample size is needed. Our results provide valuable insights into the underlying mechanisms of T2D and may offer potential targets for developing more efficacious treatments.
Type 2 diabetes (T2D) is a complex metabolic disorder characterized by a continuously high glucose level in the serum which can lead to long-term complications, including cardiovascular disease and damage to renal, ocular and nerve cells. The global prevalence of diabetes is predicted to rise from 425 million in 2017 to 629 million in 2045 (https://www.idf.org). According to the World Health Organization (WHO), diabetes is among the top ten causes of death worldwide (https://www.who.int/). T2D is the most prevalent type of diabetes, constituting about 90% of total diabetic populations [1]. It starts with insulin resistance (IR), in which cells do not respond to the normal level of plasma insulin, leading to a continuously high blood glucose level (hyperglycemia). Obesity is considered one of the significant contributors to the initiation and progression of IR and the development of T2D [2],[3]. Furthermore, the etiology of T2D involves a combination of several factors, including genetic, epigenetic and environmental factors. The biological pathways and gene regulatory networks involved in glucose and lipid metabolism are prone to impairment in individuals with a T2D-susceptible genetic background. Exposure to environmental factors, such as the toxic effects of excess glucose and free fatty acids with less physical activity, triggers IR and the onset of the T2D [4]–[7]. Previous studies have revealed several critical genetic variants and pathways predisposing to T2D, such as SLC30A8 [8], KCNJ11 and TCF7L2 [9]. The use of genome-wide association studies (GWAS) identified more than 120 genetic loci [10] and 143 variants [11] associated with T2D risk.
The regulation of glucose metabolism is primarily achieved through the hormones and enzymes produced by pancreatic islets [12]. The major component of pancreatic islets is the β-cells (60–80% in mice, 50–70% in humans) that produce and secret the hormone insulin to facilitate glucose transport inside the cells [13]. In addition, islets contain α-cells (alpha), δ-cells (delta), γ-cells (gamma) and ϵ-cells (epsilon), which produce the hormones glucagon, somatostatin, pancreatic polypeptide and ghrelin, respectively [14]. Additionally, proper glucose metabolism involves intricate regulations by insulin-responsive tissues, liver, adipose and skeletal muscle, which showed aberrant gene expression during T2D [15],[16]. During T2D progression, the requirement for insulin increases to compensate for the IR. Consequently, a high glucose level induces the proliferation of the islet β-cells for increased production and secretion of insulin [17]. However, prolonged exposure to hyperglycemia causes long-term unsustainable demand for insulin, resulting in exhaustion and loss of β-cells mass, eventually leading to a steady decline in the amount of insulin in circulation [18]–[24]. Under normal physiological conditions, the proliferation and apoptosis of β-cells are tightly regulated to maintain the appropriate mass of β-cells needed to produce the proper amount of insulin for glucose metabolism [25]. The biological process of apoptosis is induced by activating caspases-3 protein either by intrinsic pathway (cell stress) or by extrinsic pathway (signals from other cells) [26],[27]. However, multiple mechanisms have been suggested to activate programmed cell death in islets and β-cells during T2D [25]. Previous studies showed that a high glucose concentration induces apoptosis in the human endothelial cells [28] and β-cells [29] via activating pro-apoptotic genes: Bik, Bid and Bad. The high demand for insulin also creates excessive stress in the endoplasmic reticulum (ER), triggering the β-cells' apoptosis [30]. A study suggested an alternative mechanism of insulin loss in T2D through the dedifferentiation of β-cells into “naïve” cells due to the loss of transcription factor FoxO1 [31] and dysregulation of other genes [32].
In vitro studies on the islets of obese (ob/ob) mice strain have shown that increasing the glucose concentration from 0 to 11 mmol/l decreases β-cells' apoptosis, while increasing the glucose concentration above the 11 mmol/l enhances the β-cells' apoptosis [33]. The study concluded that an optimum high glucose concentration (up to 11 mmol/l) could prevent the β-cells' apoptosis and increase insulin production [33]. Thus, accumulating evidence suggests the presence of complex mechanisms of prevention and activation of apoptosis in islets/β-cells during T2D progression; however, the complete molecular mechanisms have not been fully known. Understanding the complex mechanisms of anti-apoptosis and apoptosis regulation in islets/β-cells during T2D progression is crucial for the development of effective therapeutic strategies.
The present study utilized bioinformatics and systems biology approaches to decipher the molecular mechanisms underlying peripheral tissues in T2D and to elucidate the gene regulatory networks associated with anti-apoptosis in pancreatic islet tissue in T2D. This study utilized a publicly available gene expression profile of 14-week-old obese male mice with severe T2D (BTBR-ob/ob) compared to T2D-resistant (B6-ob/ob) strains. These two groups of mice exhibit high differences in T2D-associated properties, such as elevated fasting glucose, low fasting insulin and high fasting triglyceride in T2D mice strains [15]. First, differentially expressed genes (DEGs) were identified in four different tissues (islets, liver, muscle and adipose) in T2D mice compared to the control. Then, the DEGs of each tissue were analyzed for functional enrichment and comparative study. Finally, the DEGs of islet tissue were analyzed and interpreted with the QIAGEN Ingenuity Pathway Analysis (IPA) tool, which identified activated regulatory networks in T2D associated with preventing apoptosis of pancreatic islets and β-cells. This work provides insight into a plausible mechanism that might be activated in T2D to induce the survival and proliferation of islet cells. Therefore, the findings of our computational-based study are important for further investigations to comprehend the pathways that might be linked to counter the apoptosis process in diabetes islets and possibly identify new therapeutic targets for improved management and treatment of diabetic patients [34]–[36].
To investigate the molecular cross-talk between peripheral tissues and altered gene regulatory networks in islets of T2D, we searched the Gene Expression Omnibus (GEO: www.ncbi.nlm.nih.gov/geo/) for gene expression datasets using the search term “islets, liver, muscle, adipose, type 2 diabetes”. We obtained only one microarray gene expression dataset, with the accession number GSE2899, that examined gene expression alteration in four tissues (islets, liver, muscle, adipose) in T2D [15]. In this microarray study, eight male mice aged 14 weeks were used, including four severe T2D obese mice strains [BTBR-ob/ob] and four T2D-resistant obese mice strains [C57BL/6J (B6-ob/ob)]. For each tissue type, RNA samples from two mice were pooled together and applied to an Affymetrix Murine Genome U74A Version 2 Array (MG_U74Av2) to measure gene expression. Due to the inadequate number of islets in the T2D strains [BTBR-ob/ob], an additional four mice were pooled to obtain RNA from islets. Thus, for each tissue type, two replicates from each mouse strain were used to monitor gene expression levels.
Preprocessing of raw microarray data, including background correction, normalization with GeneChip Robust Multi-array Average (GCRMA) and log2 transformation, was performed using the simpleaffy package version 2.50.0 of Bioconductor/R release 3.3.3. The differential gene expression analysis was performed to compare each tissue between T2D and T2D-resistant obese mice using the RankProducts method with the RankProdIt tool (http://strep-microarray.sbs.surrey.ac.uk/RankProducts/) [37]. RankProducts is a powerful technique that is more suitable for detecting differentially expressed genes in microarray with fewer replicates [38]. Affymetrix probe set IDs were mapped to gene symbols using DAVID 6.8 (https://david.ncifcrf.gov/). A gene was considered differentially expressed (DEGs) if the absolute value of the log2 Fold Change (|log2FC|) was ≥ 2 and the p-value was < 0.01. To avoid missing potential false-negative DEGs, a lenient p-value < 0.01 was used instead of the adjusted p-value < 0.05, which excluded most genes from the list of DEGs. If multiple probe IDs were mapped to the same gene, the probe ID with the highest |log2FC| was selected. Venn diagrams were used to illustrate the overlapping DEGs across the four tissues and were constructed using Venny version 2.1 (http://bioinfogp.cnb.csic.es/tools/venny/).
To investigate the biological processes associated with T2D, we performed the Gene Ontology (GO) enrichment analysis for Biological Process (BP), Molecular Function (MF) and Cellular Component (CC) using the list of DEGs of all four tissues. These enrichment analyses were conducted using the GENEONTOLOGY tool (http://geneontology.org/) with Reference List: Mus musculus; Test Type: Fisher's Exact; and Correction: Calculate False Discovery Rate. The significance level for the enrichment was set at p-value < 0.05.
In order to understand the Gene-Disease Associations, the DEGs of islets were mapped to diseases using the DisGeNet program [39] of the Enrichr tool [40]. The disease enrichment with a p-value < 0.05 was selected, and a bar plot was created for the top 10 diseases using Appyters [41].
IPA (QIAGEN Inc. Redwood City, CA, USA, https://digitalinsights.qiagen.com/IPA) is a powerful commercial software package for comprehensive downstream analysis of gene expression data to deduce the altered biological pathways, regulatory network and possible molecular mechanism in a disease condition using established biological relationships [42]. The IPA uses a core Ingenuity Pathways Knowledge Base (IPKB) for data analysis and interpretation. The IPKB holds the most extensive manually curated biological relationship data, including causal and directional, identified through extracting and integrating millions of publications and third-party databases. Therefore, we used QIAGEN IPA software (build version: 478438M, content version: 44691306, release date: 2018-06-14) to decipher the gene regulatory networks and functional consequences significantly associated with DEGs of the islet tissue. We uploaded a list of upregulated and downregulated genes and their expression levels (log2FC and p-value) into IPA. Then, the “Core Analysis” module was used to identify significantly associated “Canonical Pathways”, “Upstream Regulators”, “Diseases and Bio Functions”, and “Regulator Effects”. We then used the “Causal Network Analysis (CNA)” algorithm to identify novel upstream master regulators associated with the causal network of “Type 2 Diabetic Obesity”. The upstream master regulators are connected with DEGs with direct or indirect relationships whose activation or inhibition results in the observed gene expression changes. Therefore, CNA could provide possible mechanisms and root causes of observed gene expression in T2D. These upstream master regulators could be potential therapeutic targets for treating T2D. Finally, we used IPA's Downstream Effects Analysis (DEA) algorithm to predict functional and phenotypic consequences of altered gene expression. The IPA used two statistical metrics: (i) p-value: The right-tailed Fisher's exact test was used to calculate the p-value, which indicates whether an association between a set of genes and a specific biological process is significant or non-significant. (ii) Z-score: It determines the direction of activation for a biological process. A positive Z-score indicates an upregulated biological process, while a negative Z-score indicates a downregulated biological process. This work considered a biological function or canonical pathway as significant if it shows a p-value < 0.05. Furthermore, a biological function or canonical pathway is considered significantly activated if the Z-score is ≥ 2 and significantly inhibited if the Z-score is ≤ –2.
To identify the genes associated with T2D, publicly available gene expression profiles from four different tissue types were used [15]. Principal component analysis (PCA) of the expression data revealed clear differences between the four tissue types (Figure 1A), with each tissue showing a distinct subpopulation of data points between T2D and its control sample (Figure SIA, B, C, D). Using cutoffs of a |log2FC| > 2 and a p-value < 0.01, we identified the distribution of differentially expressed genes in each tissue type of T2D compared to its control.
In islets, 162 genes were identified as DEGs, comprising 106 upregulated genes and 56 downregulated genes. The top 10 genes with increased expression were APOF, CCK, EEF1A2, ALDOB, REG3A, FBP1, DCN, WWC1, Gm2663 and CEBPB (Table 1), while the top 10 genes with decreased expression were FAM151A, ANKRD33B, Defb1, FGF1, APLP2, Snhg6, Uox, PIP4K2C, RBCK1 and PRDX2 (Table 1).
In addition to islets, 109 genes were identified as DEGs in the liver, comprising 30 upregulated genes and 79 downregulated genes. The top 10 genes with increased expression were CTSE, SERPINA3, HOMER2, MYL1, PEG3, TK1, UBA5, Gm2036, GFRA1 and USP48 (Table 1), while the top 10 genes with decreased expression were C5, CIDEA, CIDEC, AQP4, SPRR1A, CADM1, RGS16, PLIN4, COL1A2 and MAPKAPK2 (Table 1).
In muscle, 100 genes were identified as DEGs, comprising 69 upregulated genes and 31 downregulated genes. The top 10 genes with increased expression were Arxes1/Arxes2, Gm2036, SERPINA3, Orm1, PSAT1, LEP, Acot1, RRAD, CIDEC and NPNT (Table 2), while the top 10 genes with decreased expression were PVALB, Mup1, ANKRD33B, RGS5, Snhg6, PRDX2, HIST1H2BI, NEDD1, CTSH and Actn3 (Table 2).
Islets | Liver | |||||
Upregulated | Gene | Log2FC | p-value | Gene | Log2FC | p-value |
APOF | 7.36 | 0.00E+00 | CTSE | 7.21 | 0.00E+00 | |
CCK | 8.00 | 0.00E+00 | SERPINA3 | 5.73 | 0.00E+00 | |
EEF1A2 | 7.29 | 0.00E+00 | HOMER2 | 4.46 | 8.05E-06 | |
ALDOB | 7.15 | 1.61E-06 | MYL1 | 4.28 | 1.29E-05 | |
REG3A | 6.27 | 1.61E-06 | PEG3 | 3.95 | 2.25E-05 | |
FBP1 | 5.89 | 3.22E-06 | TK1 | 3.42 | 6.28E-05 | |
DCN | 5.36 | 1.29E-05 | UBA5 | 3.42 | 7.41E-05 | |
WWC1 | 4.69 | 2.58E-05 | Gm2036 | 2.97 | 1.59E-04 | |
Gm2663 | 4.35 | 3.86E-05 | GFRA1 | 3.11 | 2.14E-04 | |
CEBPB | 4.28 | 4.19E-05 | USP48 | 2.80 | 2.43E-04 | |
Downregulated | FAM151A | −5.42 | 0.00E+00 | C5 | −8.12 | 0.00E+00 |
ANKRD33B | −5.36 | 0.00E+00 | CIDEA | −7.85 | 0.00E+00 | |
Defb1 | −4.36 | 3.22E-06 | CIDEC | −6.09 | 1.61E-06 | |
FGF1 | −4.05 | 6.44E-06 | AQP4 | −5.57 | 4.83E-06 | |
APLP2 | −4.08 | 6.44E-06 | SPRR1A | −5.06 | 1.05E-05 | |
Snhg6 | −3.87 | 1.29E-05 | CADM1 | −4.57 | 2.25E-05 | |
Uox | −3.88 | 1.45E-05 | RGS16 | −4.82 | 2.25E-05 | |
PIP4K2C | −3.57 | 2.90E-05 | PLIN4 | −4.25 | 5.47E-05 | |
RBCK1 | −3.61 | 3.86E-05 | COL1A2 | −4.07 | 5.80E-05 | |
PRDX2 | −3.62 | 4.35E-05 | MAPKAPK2 | −4.17 | 5.96E-05 |
Muscle | Adipose | |||||
Upregulated | Gene | Log2FC | p-value | Gene | Log2FC | p-value |
Arxes1/Arxes2 | 6.43 | 0.00E+00 | SERPINA1 | 7.98 | 8.05E-07 | |
Gm2036 | 5.61 | 0.00E+00 | HAL | 6.56 | 3.22E-06 | |
SERPINA3 | 4.60 | 1.77E-05 | PTGIS | 4.64 | 1.29E-05 | |
Orm1 | 4.37 | 2.25E-05 | NCF4 | 4.49 | 2.90E-05 | |
PSAT1 | 4.51 | 2.25E-05 | Gm2036 | 3.92 | 4.35E-05 | |
LEP | 4.06 | 5.96E-05 | ADRB3 | 4.41 | 4.35E-05 | |
Acot1 | 3.59 | 1.05E-04 | GABRR2 | 3.83 | 4.35E-05 | |
RRAD | 3.56 | 1.11E-04 | Ces1g | 3.39 | 1.16E-04 | |
CIDEC | 3.58 | 1.45E-04 | CAP1 | 3.07 | 1.96E-04 | |
NPNT | 3.43 | 1.55E-04 | IKBIP | 2.84 | 2.70E-04 | |
Downregulated | PVALB | −6.14 | 0.00E+00 | Ear2 | −5.07 | 0.00E+00 |
Mup1 | −5.08 | 0.00E+00 | Snhg6 | −4.63 | 0.00E+00 | |
ANKRD33B | −4.45 | 6.44E-06 | Mup1 | −4.98 | 0.00E+00 | |
RGS5 | −4.42 | 6.44E-06 | CCK | −4.89 | 0.00E+00 | |
Snhg6 | −4.02 | 1.29E-05 | FCGR2B | −4.79 | 0.00E+00 | |
PRDX2 | −3.65 | 3.38E-05 | PRDX2 | −4.21 | 1.61E-06 | |
HIST1H2BI | −3.46 | 3.86E-05 | SLC1A5 | −4.08 | 4.83E-06 | |
NEDD1 | −3.38 | 4.83E-05 | Ear3 | −3.85 | 1.13E-05 | |
CTSH | −3.40 | 5.47E-05 | GRK5 | −3.52 | 2.25E-05 | |
Actn3 | −3.91 | 6.92E-05 | HIST1H2BI | −3.28 | 3.06E-05 |
In adipose, 86 genes were identified as DEGs, comprising 51 upregulated genes and 35 downregulated genes. The top 10 genes with increased expression were SERPINA1, HAL, PTGIS, NCF4, Gm2036, ADRB3, GABRR2, Ces1g, CAP1 and IKBIP (Table 2); while the top 10 genes with decreased expression were Ear2, Snhg6, Mup1, CCK, FCGR2B, PRDX2, SLC1A5, Ear3, GRK5 and HIST1H2BI (Table 2). Detailed information on the DEGs for each tissue is provided in supplementary Table S1 (islets), Table S2 (liver), Table S3 (muscle) and Table S4 (adipose).
Each tissue has a unique gene expression profile, indicating that T2D may affect distinct patterns of upregulated and downregulated genes. However, the disease can also affect a common set of genes and pathways across different tissues. Therefore, we compared the expression patterns across different tissues to understand the unique and common behavior of gene expression in T2D relative to the control.
A comparative study of upregulated genes across different tissues of T2D compared to the control revealed that (a) Chil3, S100A9, Tgtp1 and Ifi47 were upregulated in adipose and liver; (b) PTGER3 and C7orf43 were upregulated in adipose and islets; (c) SERPINA3 and UBA5 were upregulated in liver, muscle and islets; (d) PSAT1 and ATF3 were upregulated in muscle and islets; (e) SSR1 and S100A8 were upregulated in liver and muscle; and (f) Gm2036 was upregulated across all four tissues. The numbers of tissue-specific genes upregulated in islets, liver, muscle and adipose were 99, 21, 62 and 44, respectively (Figure 1B).
A similar comparative analysis of downregulated genes across different tissues of T2D compared to the control showed that (a) RGS5 and FAM213A were downregulated in adipose and muscle; (b) SPG21, Gsta4 and C1QB were downregulated in liver and muscle; (c) ANXA1 and ACTA2 were downregulated in liver and islets; (d) Mup1 was downregulated across adipose, liver and muscle; (e) C5orf34 was downregulated across adipose, liver and islets; (f) Snhg6, PRDX2, HIST1H2BI and ANKRD33B were downregulated across all four tissues. The numbers of tissue-specific genes downregulated in islets, liver, muscle and adipose were 49, 68, 21 and 27, respectively (Figure 1C).
To gain insight into the biological functions and key pathways altered in each tissue of T2D, we performed functional annotation and enrichment analysis for upregulated and downregulated genes using the GENEONTOLOGY webserver (http://geneontology.org/).
In islets, the BP and MF categories of Gene Ontology analysis revealed that the upregulated genes were enriched in several metabolic processes, including sucrose metabolic process, fructose metabolic process, positive regulation of mitochondrial depolarization, fructose 1,6-bisphosphate metabolic process, negative regulation of neuron apoptotic process, regulation of programmed cell death, regulation of cell death and protein binding (Table S5A and S5B). In addition, the CC category of Gene Ontology analysis indicated that the upregulated genes were significantly associated with perikaryon and neuronal cell body (Table S5C). On the other hand, the MF category of Gene Ontology analysis revealed that the downregulated genes were enriched in 1-phosphatidylinositol-4-phosphate 5-kinase activity and phosphatidylinositol phosphate kinase activity (Table S5D). However, downregulated genes did not show significant enrichment for BP and CC categories of Gene Ontology.
In liver, the BP and MF categories of Gene Ontology analysis revealed that the downregulated genes were significantly associated with several processes, including positive regulation of TRAIL-activated apoptotic signaling pathway, response to stilbenoid, fat-soluble vitamin metabolic process, collagen fibril organization, positive regulation of cell death, lipid metabolic process and platelet-derived growth factor binding (Table S6A and S6B). Additionally, the CC category of Gene Ontology analysis revealed that the downregulated genes were significantly associated with collagen type I trimer and plasma lipoprotein particle (Table S6C). However, the upregulated genes did not show significant enrichment for BP, MF and CC categories of Gene Ontology.
In muscle, the BP category of Gene Ontology analysis revealed that the upregulated genes were enriched with steroid metabolic process and lipid metabolic process (Table S7A), while the downregulated genes were enriched in collagen-containing extracellular matrix of CC category of Gene Ontology (Table S7B). However, the upregulated genes did not show significant enrichment for MF and CC categories of Gene Ontology, while the downregulated genes did not show significant enrichment for BP and MF categories of Gene Ontology.
In adipose, the BP category of Gene Ontology analysis revealed that the upregulated genes were enriched with cellular response to interferon-beta and negative regulation of developmental process (Table S8A), while the downregulated genes were enriched in muscle tendon junction and extracellular space of CC category of GO (Table S8B). However, the upregulated genes did not show significant enrichment for MF and CC categories, and the downregulated genes did not show significant enrichment for BP and MF categories of Gene Ontology.
In order to explore the relationship between dysregulated gene expression in T2D islets and diseases, we utilized the DisGeNet tool to map the DEGs to their associated diseases. Our analysis revealed that the upregulated genes were significantly linked to diabetes mellitus, non-insulin-dependent, while the downregulated genes were significantly linked to Barrett's esophagus (Figure 2). For a complete list of the Gene-Disease Associations of both upregulated and downregulated genes, please refer to supplementary Table S9 and Table S10, respectively.
The BP annotation of Gene Ontology revealed that the upregulated genes in islets were significantly associated with negative regulation of neuron apoptotic process, regulation of apoptotic process, regulation of programmed cell death and regulation of cell death (as shown in Table 3 and Table S5A). To interpret the gene-expression data and to decipher the molecular mechanisms and causal networks underpinning the anti-apoptosis of islets during T2D, we analyzed the DEGs of islets using the IPA “Core Analysis” module. The canonical pathway analysis showed the top 15 enriched pathways of DEGs of islets of T2D compared with normal (Figure 2C and Table S11A). The upregulated genes of T2D islets showed enrichment with and activation of Gluconeogenesis I (p-value: 3.15E-04; Z-score: 2), Glycolysis I (p-value: 5.69E-04; Z-score: 2), Glutamine Biosynthesis (p-value: 1.80E-02) and FXR/RXR Activation (p-value: 2.81E-02) pathways (Figure 2C). Meanwhile, downregulated genes of T2D islets showed enrichment of D-myo-inositol (1,4,5)-trisphosphate biosynthesis (p-value: 2.88E-02), pyrimidine deoxyribonucleotides de novo biosynthesis I (p-value: 3.23E-02) and alpha-tocopherol Degradation (p-value: 3.54E-02) pathways (Figure 2C).
We then analyzed the upstream regulators responsible for altered gene expression in T2D islets. The results showed that PDGF BB (p-value: 6.65E-06; Z-score: 3.782), STAT3 (p-value: 1.63E-03; Z-score: 3.417), CREB1 (p-value: 2.40E-02; Z-score: 3.227), IL6 (p-value: 1.17E-06; Z-score: 3.03), IGF1 (p-value: 2.19E-03; Z-score: 2.776) and Ca2+ (p-value: 1.04E-02; Z-score: 2.742) were the top six potential upstream regulators activated in the islets of T2D, which may be the reason for changes in gene expression in islets of T2D (Table S11B). Based on the enrichment status of downstream molecules, D-glucose was also predicted as a significant upstream regulator (p-value:2.83E-07), though its activation Z-score is less than 2 (Z-score: 1.227).
Furthermore, IPA was used to predict the causal networks and their upstream master regulators that control the gene expression in T2D islets. Causal networks having target genes directly controlled by upstream master regulators include the activation of PDGF BB (p-value: 1.49E-05; Z-score: 3.873) and activation of IL6 (p-value: 1.48E-07; Z-score: 3). Additionally, the results also indicated the activation of D-glucose (p-value: 1.19E-08; Z-score: 1.177) and the inhibition of GW3965 (p-value: 5.90E-08; Z-score: −2.746) and PPARa-RXRa (p-value: 6.51E-08; Z-score: −2.611) as master regulators, as shown in Figure 3 and Table S11C. Therefore, these findings suggest that PDGF BB, IL6 and Glucose could be potential inducers of the altered gene expression observed in the islets of T2D.
GO biological process | M. musculus REFLIST (21988) | Upregulated (104) | Upregulated gene | Upregulated (expected) | Upregulated (fold Enrichment) | Upregulated (raw P-value) | Upregulated (FDR) |
Negative regulation of neuron apoptotic process (GO:0043524) | 183 | 7 | Reg3b, CEBPB, BDNF, SLC1A1, LGMN, fam134b, KDR | 0.87 | 8.09 | 3.28E-05 | 2.15E-02 |
Regulation of apoptotic process (GO:0042981) | 1468 | 19 | CLDN7, PHLDA3, OLFM1, EIF2AK3, ZFP36L1, GADD45A, Reg3b, CEBPB, BDNF, SLC1A1, ZBTB16, EEF1A2, LGMN, fam134b, ATF3, CCK, KDR, BCL6, NR4A1 | 6.94 | 2.74 | 5.91E-05 | 2.59E-02 |
Regulation of programmed cell death (GO:0043067) | 1504 | 19 | CLDN7, PHLDA3, OLFM1, EIF2AK3, ZFP36L1, GADD45A, Reg3b, CEBPB, BDNF, SLC1A1, ZBTB16, EEF1A2, LGMN, fam134b, ATF3, CCK, KDR, BCL6, NR4A1 | 7.11 | 2.67 | 8.14E-05 | 3.13E-02 |
Regulation of cell death (GO:0010941) | 1667 | 20 | CLDN7, PHLDA3, CTGF, OLFM1, EIF2AK3, ZFP36L1, GADD45A, Reg3b, CEBPB, BDNF, SLC1A1, ZBTB16, EEF1A2, LGMN, fam134b, ATF3, CCK, KDR, BCL6, NR4A1 | 7.88 | 2.54 | 1.04E-04 | 3.88E-02 |
Using the IPA tool, our analysis of altered gene expression in the islets of T2D-susceptible mice revealed downstream effects on various diseases and functions. These effects included an increase in the release of metal (p-value: 7.18E-04, Z-score: 2.115), an increase in the permeability of the vascular system (p-value: 2.51E-03, Z-score: 2.103) and an increase in the quantity of metal (p-value: 3.25E-03, Z-score: 2.012). Our analysis also showed an increase in the quantity of insulin in the blood (p-value: 2.62E-03; Z-score: 1.188) and a decrease in insulin resistance (p-value: 5.60E-03; Z-score: −1.00). However, the Z-scores for these effects were not statistically significant (Figure 4A, Table S11D).
Interestingly, our network analysis suggests that altered gene expression in islets of T2D mice strains may contribute to the reduction in cell death of islets of Langerhans (p-value: 6.44E-03, Z-score: −2.412), reduction in cell death of beta islets' cells (p-value: 1.10E-02, Z-score: −2.219) and reduction in apoptosis of islets of Langerhans (p-value: 1.66E-02, Z-score: −2.2) (Figure 4B, Table S11D). The biological network predicted to decrease the apoptosis of islets of Langerhans in T2D islet cells includes upregulated ATF3, CCK, GAST, JUNB and NR4A1, as well as downregulated DPP4. However, the upstream regulators and molecular mechanisms responsible for preventing apoptosis in islets are still largely unknown. To address this gap, we used DEGs from T2D islet cells to construct “regulator effect networks” using IPA, which led to the identification of two networks with their upstream regulators that may be involved in preventing apoptosis of islets of Langerhans in T2D. One network contains the activation of five upstream regulators (CREB1, EGR1, FGF2, P38 MAPK and Pka), which drives expression change in four genes (ATF3, CCK, JUNB and NR4A1) to cause decreased cell death of beta islets' cells and apoptosis of islets of Langerhans (Figure 5A). This network has the highest consistency score of 11.5, which we used to identify as the most prioritized network for the actionable hypothesis. Another network contains activation of upstream regulator tumor necrosis factor (TNF), which drives expression change in five genes (ATF3, DPP4, GAST, JUNB and NR4A1) to cause decreased apoptosis of islets of Langerhans (Figure 5B). Details of the networks, their regulators and associated diseases and functions are given in (Table 4 and Table S11E). Thus, our study has provided insights into potential gene regulatory networks and their upstream regulators that may prevent the apoptosis of islets of Langerhans in T2D and can guide future research in this area. However, further experimental validation is required to confirm these hypotheses.
In order to validate the presence of anti-apoptosis pathways in islets at moderately high glucose concentrations, we analyzed the gene expression data from young mice islets cultured at 3 and 11 mM glucose concentrations (GEO: GSE42591) [43]. Using GEO2R with a p-value < 0.05, the differentially expressed genes (DEGs2) of islet tissue in high glucose concentrations (11 mM) compared to low glucose concentrations (3 mM) were identified (Table S12). The BP category of Gene Ontology enrichment analysis with the GENEONTOLOGY (http://geneontology.org/) showed that the upregulated genes in response to high glucose concentration were significantly associated with negative regulation of apoptotic process (Table S13 and Table S14). However, we observed that the genes associated with the anti-apoptosis process are not common between the two studied gene expression datasets (GSE2899 and GSE42591). Therefore, further research is needed to understand the gene specificity and mechanisms involved in the anti-apoptosis pathway in islets.
Our computational analysis identified a gene regulatory network associated with preventing apoptosis of islets of Langerhans containing upregulated genes CCK, ATF3, JUNB, NR4A1, GAST and downregulated gene DPP4. To further support our finding, we investigated the role of the identified genes in T2D using the Open Targets Platform (https://platform.opentargets.org).
The Open Targets Platform provides a framework to investigate the target and its relevant relationships with phenotypes, diseases and drugs based on integrated data and annotation information. We found that DPP4, which was downregulated in our predicted gene regulatory network, has the highest overall association score (0.64) with T2D (Figure 6, Table S15). In addition, DPP4 is targeted by 21 clinical candidates or approved drugs, including saxagliptin and sitagliptin, commonly used to treat T2D (Table S16). The MGI database (http://www.informatics.jax.org/) also showed that homozygous knockout of DPP4 in mouse strain C57BL/6-Dpp4tm1Nwa leads to increased insulin secretion and improved glucose tolerance, suggesting that DPP4 is a potential therapeutic target of T2D.
Regulators | Target molecules in dataset | Diseases & Functions | Consistency score |
CREB1,EGR1,FGF2,P38 MAPK,Pka | ATF3,CCK,JUNB,NR4A1 | Apoptosis of islets of Langerhans, Cell death of beta islets cells | 11.5 |
ERK1/2,NFkB (complex) | BDNF,C3,CCK,EIF2AK3,FGF1,GAST,KDR,LCN2,RGS16 | Quantity of metal, Release of metal | 5 |
EGF,P38 MAPK | ATF3,CTGF,IGFBP5,KLF10 | Increased Levels of Alkaline Phosphatase | 3 |
TNF | ATF3,DPP4,GAST,JUNB,NR4A1 | Apoptosis of islets of Langerhans | −4.919 |
CTNNB1 | ACTA2,ANXA1,AQP4,GNAO1,KDR,NR4A1 | Permeability of vascular system | −5.307 |
Type 2 diabetes (T2D) is a global epidemic associated with both acute and long-term complications. To better understand the molecular mechanisms underlying T2D and the regulatory network associated with anti-apoptosis of pancreatic islet tissue in T2D, we conducted a study using gene expression data (GEO: GSE2899) obtained from islets, liver, muscle and adipose tissues of T2D [BTBR-ob/ob] and T2D-resistant [C57BL/6J] mice. The gene expression data was generated by a previous study [15], which showed that T2D mice with severe symptoms exhibited upregulated inflammatory and immune response genes in adipose tissue and liver, respectively. Additionally, the study suggested that the upregulation of hepatic lipogenic genes played a role in protecting T2D-resistant mice against diabetes. However, the study did not report on the activation of anti-apoptosis-associated genes and regulatory networks in T2D islets [15]. Knowledge of anti-apoptosis-associated genes activation is highly required to develop strategies to protect the islets from damage in diabetes. Our comprehensive analysis of gene expression data from these four tissues revealed several important genes and pathways associated with T2D.
In the analysis of differentially expressed genes (DEGs) in the tissues of T2D mice, we found that 162, 109, 100 and 86 genes were differentially expressed in islets, liver, muscle, and adipose tissues, respectively. Furthermore, a comparative analysis of DEGs was performed to understand common genes and biological pathways affected in all tissues during T2D (Figure 1B,C). One common upregulated gene across all four tissues in T2D compared to controls was Gm2036 [ENTREZ_GENE_ID: 67704; RefSeq Transcript ID: NM_024461], which encodes a 65 amino acid long uncharacterized protein, C4orf3 [another-regulin (ALN)] homolog. The ALN is a transmembrane micro peptide and is known to inhibit the activity of SERCA2b (sarco/endoplasmic reticulum Ca2+ ATPase) pump [44]. SERCA pump promotes muscle relaxation by taking up Ca2+ into the sarcoplasmic endoplasmic reticulum [45]. We speculate that the upregulation of Gm2036 may result in a decrease in the rate of Ca2+ uptake into the sarcoplasmic endoplasmic reticulum, which leads to an influx of cytosolic Ca2+. This may result in the dysregulation of Ca2+ signaling in all four tissues of T2D, as the IPA tool also identified Ca2+ as a potential upstream regulator responsible for altered gene expression in T2D islets (Table S11B). Under normal physiology, glucose uptake by β-cells raises the cytosolic Ca2+ that induces exocytosis of insulin vesicles and increases cell survival. However, the exact role of SERCA2b/ALN on cytosolic Ca2+ needs further investigation in T2D pathophysiological conditions, as persistent hyperglycemia and stimulation of Ca2+ signaling pathways are detrimental to β-cells [46]–[48].
In addition, our analysis found that T2D leads to the downregulation of Snhg6, PRDX2, HIST1H2BI and ANKRD33B in all four tissues. Snhg6 is a long noncoding RNA (lncRNA), which regulates gene expression by sponging miRNAs [49]. Prior studies have shown decreased expression of Snhg6 in the placenta of patients with gestational diabetes mellitus [50]. Furthermore, in vitro experiments demonstrated that cells treated with high glucose resulting reduced expression of Snhg6 and increased expression of miRNA-26a-5p, which targets EZH2 [50]. The PRDX2 encodes antioxidant enzymes, and its downregulation could reduce a cell's protection against oxidative stress and inflammatory injury. HIST1H2BI encodes a core component of the nucleosome that helps organize chromatin structure, while the function of ANKRD33B encoding protein is still unclear. Our analysis also found downregulation of the Regulator-of-G-protein-signaling-5 (RGS5) encoding gene in adipose and muscle tissues of T2D obese mice. RGS5 is a member of GTPase activating proteins and plays a role in suppressing G protein signaling. Overexpression of RGS5 has been linked to inducing apoptosis in different cells, including the lung cancer cell line [51]. Deng et al. showed RGS5 knockout (KO) mice have exhibited obesity, severe hepatic steatosis, inflammation and increased insulin resistance [52]. Furthermore, our GO enrichment analysis showed the activation of various processes in all four tissues of T2D, including carbohydrate metabolic processes in islets, which is the hallmark of the T2D [53].
The gene expression profiles have been extensively used to understand the molecular mechanisms of diseases and to find biomarkers and novel targets [54]–[57]. Therefore, the present work analyzed DEGs to understand the biological processes affected in the islets of T2D mice. In this study, Gene-Disease associations analysis with the DisGeNet tool identified that the upregulated genes were significantly associated with diabetes mellitus non-insulin-dependent (Figure 2A). Further evidence of T2D's effect on islets came from the Gene Ontology enrichment analysis, which showed that the upregulated genes are involved in the sucrose and fructose metabolic process, confirming the association of altered gene expression pattern with T2D (Table S5A). Furthermore, canonical pathway analysis with the IPA showed the upregulation of ALDOB, FBP1, FBP2 and GPI genes resulting in the activation of both glycolysis (Z-score: 2) and gluconeogenesis (Z-score: 2) pathways in islets of T2D (Figure 2C). Under normal conditions, gluconeogenesis is inhibited when glycolysis is highly active, and vice versa. The simultaneous activation of both glycolysis and gluconeogenesis results in the metabolism of glucose to pyruvate by glycolysis and subsequently back to glucose by gluconeogenesis. This reaction maintains the glucose level and only consumes ATP, which is referred to as a glucose futile cycle. Earlier works showed an increase in glucose futile cycle in the islets of neonatally streptozocin-induced diabetic (STZ-D) rats model of T2D compared to the control, thus supporting our hypotheses [58],[59].
The major pathogenesis of severe T2D includes the reduction of pancreatic islets and β-cells mass, resulting in insufficient insulin release and, consequently, impaired glucose metabolism [15]. Previous studies identified different pathways responsible for inducing apoptosis in β-cells in severe T2D [60]–[62]. In contrast, other studies found that the culture of mouse islet β-cells and MIN6 insulinoma cells reduced the rate of apoptosis at high glucose concentration compared to low-glucose concentration [33],[47]. Furthermore, a study presents evidence that glucose induces the β-cells survival through activating the PI3-kinase/Akt-signaling pathway [47]. Thus, accumulating evidence suggests that activation of anti-apoptosis and apoptosis pathways during the progression of IR to severe T2D. However, the molecular mechanism of an anti-apoptosis process in the islets of T2D is not fully known.
Interestingly, the BP of Gene Ontology indicates that the upregulated genes in T2D islets were significantly associated with the negative regulation of the neuron apoptotic process (Table 3). The islet β-cells have many common molecular and biochemical mechanisms with neurons [47], thus indicating that anti-apoptotic pathways might be activated to prevent programmed cell death to increase the cells in islets during T2D; however, the causal relationship is not yet fully understood. Another study showed that high glucose concentration induces the proliferation of islet β-cells isolated from ob/ob mice while reducing their apoptosis process to maximize the production and secretion of insulin [33]. Still, prolonged hyperglycemia activates apoptosis of islet β-cells, leading to decreases in the islet β-cells mass and insulin secretion [17]. Therefore, to understand the molecular mechanism of survival of islet β-cells during T2D progression, further analysis was carried out on pancreatic islets using IPA.
Our analysis identified several possible upstream regulators, including high glucose and the activation of PDGF-BB, STAT3 and IL6, that were responsible for the dysregulated genes in T2D islets (Figure 3 and Table S11C). Platelet-derived growth factors (PDGFs) are released by platelets into the serum and act as potent activators of their target cells for growth, division and cell survival [63]. The PDGF-B isoform becomes active by forming a homodimer (PDGF-BB), which induces cellular effects through binding to tyrosine kinase transmembrane receptors (PDGFRs) by several signaling pathways, including (i) activating the PI3K/Akt/mTOR pathway, which results in enhanced proliferation and inhibits apoptosis; (ii) activating the JAK/STAT pathway, which enhances proliferation and angiogenesis [63]. A previous study reported an increased level of PDGF-BB in gestational diabetes mellitus patients [64]. Based on in vitro experiments, it was suggested that PDGF-BB promotes cell proliferation but downregulates the β-cell-specific genes, which may be responsible for β-cells dedifferentiation and dysfunction [64]. STAT3 is a transcription factor whose activity is tightly regulated through phosphorylation and dephosphorylation to maintain the cell's physiological condition. Overexpression of STAT3 has been reported to enhance cell proliferation and survival and prevent apoptosis in several cancers [65],[66]. According to research, active STAT3 plays a crucial role in insulin secretion, maintaining the β-cells' function and their mass maintenance [67]. Weng et al. discovered that knocking out STAT3 induces β-cells apoptosis in mice by accumulating PTEN, which represses the AKT phosphorylation [68]. Several ligands, including IL6, PDGF and IGF1, can activate STAT3 [69],[70]. In vitro and in vivo studies showed that IL-6 prevents pancreatic islets or β-cells from cytokine-induced cell death [71]. CREB is another TF required to maintain insulin secretion and β-cells survival [72]. Several ligands, including IGF1, have been reported to phosphorylate and activate the CREB, allowing it to express its target genes in β-cells [72].
Our analysis found that DEGs in islets affect several crucial biological processes related to T2D, including reducing insulin resistance, increasing insulin levels in the blood and inhibiting apoptosis of islet cells (Figure 4). These processes are the hallmarks of IR and T2D progression, supporting previous findings on the disease [33],[47]. Previous investigations have reported on the effects of various genes on insulin resistance in mice. For example, depletion of BDNF protein [73], inactivation of mouse VEGFR-2 (Kdr) [74] and homozygous knockout of Lcn2 [75] have all been shown to increase insulin resistance. Interestingly, our study found that the expression of BDNF, KDR and LCN2 is upregulated in islets, suggesting that they may play a role in reducing insulin resistance in T2D. Another gene, Rbpj, has been shown to decrease insulin resistance with homozygous knockout [76]. In our dataset, we observed that the expression of RBPJ is downregulated in T2D, further supporting its role in decreasing insulin resistance (Figure 4A).
Additionally, our analysis identified genes whose knockout or depletion has been associated with impaired glucose metabolism in mice. For example, previous studies have shown that homozygous knockout of the Cebpb gene in mice increases hypoglycemia and impaired glucose metabolism [77]–[79]. In our analysis, we observed upregulation of CEBPB, indicating its potential role in reducing hypoglycemia in T2D. Similarly, a homozygous knockout of the Cck gene in mice has been found to increase hyperglycemia in fasting ob/ob mice [80]. However, in our dataset, Cck is upregulated, suggesting that it may reduce hyperglycemia in T2D. BDNF protein has been reported to decrease hyperglycemia in many studies [81]–[83]. In our dataset, we observed upregulation of BDNF, indicating its potential role in reducing hyperglycemia in T2D. Furthermore, a mouse mutant with the Eif2ak3 (Perk) gene or deficiency of EIF2AK3 has been found to increase hyperglycemia [84]–[86]. In humans, mutations in the EIF2AK3 gene [c.440T > G (rs1057524886); c.1751G > A (rs150314450)] are associated with monogenic diabetes. In our dataset, Eif2ak3 is upregulated, indicating its potential role in reducing hyperglycemia in T2D. Thus, altering gene expression may potentially lead to the simultaneous reduction of hypoglycemia and hyperglycemia, trying to maintain blood sugar levels within a healthy range. However, further experimental validation is necessary to confirm the role of these genes in T2D.
In our study, we observed that the expressions of ATF3, CCK, GAST, JUNB and NR4A1 genes were upregulated, while the expression of DPP4 was downregulated in the islets of T2D. The diseases and biofunctions analysis indicated that the dysregulated expression of these genes may contribute to decreased cell death of islets of Langerhans (Figure 4B, Table S11D). However, a previous study reported the upregulation of CCK and ATF3 in islets of severe T2D mouse strains [15], which is consistent with our findings. Furthermore, our analysis revealed the gene regulatory networks with potential upregulators using the IPA tool (Figure 5). Previous studies have found that ATF3 [87], JUNB [87], CCK [80], GAST [88] and NR4A1 [89] proteins inhibit apoptosis and improve the β-cells regeneration and survival. Furthermore, EEF1A2 is upregulated in islets of T2D, although its role in apoptosis of islet cells is not yet known. However, studies have indicated its role in apoptosis inhibition through activating PI3K/Akt/NF-κB signaling pathway in non-pancreatic cells [90]–[92]. A prior study has identified the role of glucose in promoting the survival of mouse islet β-cells via the PI3K/Akt-signaling pathway [47], indicating the potential importance of this pathway in islet cell apoptosis.
In our analysis, GAST (GASTRIN) is upregulated in islets of T2D, which may help alleviate the glucose metabolism disorder. Gastrin is a hormone normally secreted by the stomach, and its expression is activated in the islets of the developing pancreas but typically disappears in the postnatal period [93]. A study showed that gastrin administration stimulates regeneration of β-cells and improves glucose tolerance in pancreatectomized rats [88]. Basically, the CCK-A receptor (cckar gene) is expressed in the islet cells and is activated by binding with either Gastrin or cholecystokinin. Though the binding affinity of CCK was hundreds of times greater than gastrin, the study suggested that both gastrin and CCK reduce apoptosis and enhance the cell mass of islet β-cells through binding with CCK-A receptor [88],[94].
In vitro studies have shown that the cytokines tumor necrosis factor (TNF)-α and interferon (IFN)-γ induce JunB expression in both human and rodent β-cells [87]. Consequently, the JunB transcriptionally binds and induces the expression of ATF3. ATF3 plays a crucial role in protecting against apoptosis and promoting β-cells' survival under inflammatory stress [87]. Thus, accumulating evidence supports the role of our proposed biological network in anti-apoptosis (Figure 5B). Moreover, the CHIP assay has demonstrated that high expression of NR4A1 in mouse islets or MIN6 cells binds to the promoter ( from −1872 to −1866 bp) of survivin and increases its expression [89]. This, in turn, inhibits Caspase 3 activation and ultimately prevents ER stress-induced apoptosis [89].
We found that DPP4 is downregulated in islets of T2D (Figure 4B, Figure 5). Dipeptidyl-peptidase (DPP) 4 is a type II transmembrane glycoprotein with a molecular weight of 110 kDa which functions through both enzymatic and non-enzymatic activity. It circulates in the bloodstream in soluble and membrane-bound forms. DPP4 is a serine protease that cleaves and inactivates a wide range of substrates, including incretins (GLP-1 and GIP), and participates in a number of biological and signaling activities [95]. Incretins are gut hormones that are released into the bloodstream after a meal and control glucose metabolism by stimulating the release of insulin, decreasing the release of glucagon, suppressing β-cells' apoptosis and promoting β-cells' proliferation [96]. However, within a few minutes, incretins are inactivated by DPP4. Accumulating evidence shows that DPP4 plays a role in inducing apoptosis in β-cells, while its inhibitors stimulate insulin secretion and promote β-cell proliferation through various pathways [97]–[100]. One study demonstrated that subfractions of Abelmoschus esculentus (AE), commonly called okra, can prevent palmitate-induced apoptosis of β cells by inhibiting the expression of DPP-4 and increasing the expression of GLP-1 receptor (GLP-1R) [101]. Further study showed that the prevention of β cell apoptosis appears to be mediated through the regulation of AMPK/mTOR, PI3K and caspase 3 signaling pathways [101]. Additionally, other studies have demonstrated that the proliferative and antiapoptotic effects of GIP in the β-cells are mediated through the activation of signaling transduction pathways such as cAMP/PKA, PKA/CREB, MAPK and PI3K-dependent activation of PKB [102]–[104]. Furthermore, the role of DPP4 in T2D was supported with the highest association score (0.64) using the Open Targets Platform (Figure 6, Table S15). Interestingly, several drugs, including sitagliptin and vildagliptin, inhibitors of DPP4, are under clinical trials for the improvement of β-cells' function and better treatment of T2D [105]–[109] (https://clinicaltrials.gov/ct2/show/study/NCT02312063). Thus, growing evidence suggests that DPP4 could be a promising target for the treatment of T2D. It is noteworthy that the current gene expression-based analysis was conducted using T2D mice that showed a low number of islets, indicating its loss due to apoptosis [15]. Furthermore, our analysis suggests the activation of the anti-apoptosis regulatory networks in islets. The mechanisms of anti-apoptosis and pro-apoptosis are complex and mediated through various pathways; therefore, further investigation is warranted to determine how both pathways are activated in the islets during the progression of T2D.
Our study was based on publicly available gene expression data from a small number of mice samples. Therefore, further study is required with a large sample size to validate our findings. Furthermore, islets contain different types of cells; therefore, the results did not reflect the specific type of islet cell. Finally, the findings of this study are based on computational analysis and were not experimentally validated.
It is known that the progression of T2D involves the initial activation of the anti-apoptotic process in islet cells, followed by the activation of the pro-apoptotic process. However, the molecular mechanism underlying the anti-apoptosis process and its role in islet cell proliferation during T2D remains incompletely understood. Our study used the IPA tool on gene expression data from mice islets and revealed that the anti-apoptosis regulatory networks are activated during T2D, contributing to islet cell survival and proliferation. Specifically, we identified five upstream regulators (CREB1, EGR1, FGF2, P38 MAPK, Pka and TNF) that drive the expression change of six downstream genes (ATF3, CCK, JUNB, NR4A1, GAST and DPP4), inhibiting apoptosis of islets and β-cells and promoting β-cells' regeneration to improve glucose metabolism. (Figure 5). These findings suggest a potential mechanism for the survival of pancreatic islets cell in T2D, which requires experimental validation. In addition, our study has identified DPP4 as a potential therapeutic target for preventing damage to islet cells in T2D. These findings could aid in the identification of potential genetic markers and new therapeutic target molecules, ultimately leading to the development of drugs to prevent β-cells failure in T2D and improve diabetes management and treatment.
[1] |
Zheng Y, Ley SH, Hu FB (2018) Global aetiology and epidemiology of type 2 diabetes mellitus and its complications. Nat Rev Endocrinol 14: 88-98. https://doi.org/10.1038/nrendo.2017.151 ![]() |
[2] |
van Dam RM (2003) The epidemiology of lifestyle and risk for type 2 diabetes. Eur J Epidemiol 18: 1115-1125. https://doi.org/10.1023/b:ejep.0000006612.70245.24 ![]() |
[3] |
Shaw JE, Chisholm DJ (2003) 1: Epidemiology and prevention of type 2 diabetes and the metabolic syndrome. Med J Aust 179: 379-383. https://doi.org/10.5694/j.1326-5377.2003.tb05677.x ![]() |
[4] |
Dendup T, Feng X, Clingan S, et al. (2018) Environmental risk factors for developing type 2 diabetes mellitus: a systematic review. Int J Environ Res Public Health 15: 78. https://doi.org/10.3390/ijerph15010078 ![]() |
[5] | Joseph JS, Anand K, Malindisa ST, et al. (2021) Exercise, CaMKII, and type 2 diabetes. EXCLI J 20: 386-399. https://doi.org/10.17179/excli2020-3317 |
[6] |
Tanabe K, Liu Y, Hasan SD, et al. (2011) Glucose and fatty acids synergize to promote B-cell apoptosis through activation of glycogen synthase kinase 3β independent of JNK activation. PLoS One 6: e18146. https://doi.org/10.1371/journal.pone.0018146 ![]() |
[7] |
Poitout V, Robertson RP (2008) Glucolipotoxicity: fuel excess and beta-cell dysfunction. Endocr Rev 29: 351-366. https://doi.org/10.1210/er.2007-0023 ![]() |
[8] |
Rutter GA, Chimienti F (2015) SLC30A8 mutations in type 2 diabetes. Diabetologia 58: 31-36. https://doi.org/10.1007/s00125-014-3405-7 ![]() |
[9] |
Al-Sinani S, Woodhouse N, Al-Mamari A, et al. (2015) Association of gene variants with susceptibility to type 2 diabetes among Omanis. World J Diabetes 6: 358-366. https://doi.org/10.4239/wjd.v6.i2.358 ![]() |
[10] |
Prasad RB, Groop L (2015) Genetics of type 2 diabetes-pitfalls and possibilities. Genes 6: 87-123. https://doi.org/10.3390/genes6010087 ![]() |
[11] |
Xue A, Wu Y, Zhu Z, et al. (2018) Genome-wide association analyses identify 143 risk variants and putative regulatory mechanisms for type 2 diabetes. Nat Commun 9: 2941. https://doi.org/10.1038/s41467-018-04951-w ![]() |
[12] |
Röder PV, Wu B, Liu Y, et al. (2016) Pancreatic regulation of glucose homeostasis. Exp Mol Med 48: e219. https://doi.org/10.1038/emm.2016.6 ![]() |
[13] |
Dolenšek J, Rupnik MS, Stožer A (2015) Structural similarities and differences between the human and the mouse pancreas. Islets 7: e1024405. https://doi.org/10.1080/19382014.2015.1024405 ![]() |
[14] |
Campbell JE, Newgard CB (2021) Mechanisms controlling pancreatic islet cell function in insulin secretion. Nat Rev Mol Cell Biol 22: 142-158. https://doi.org/10.1038/s41580-020-00317-7 ![]() |
[15] |
Lan H, Rabaglia ME, Stoehr JP, et al. (2003) Gene expression profiles of nondiabetic and diabetic obese mice suggest a role of hepatic lipogenic capacity in diabetes susceptibility. Diabetes 52: 688-700. https://doi.org/10.2337/diabetes.52.3.688 ![]() |
[16] | da Silva Rosa SC, Nayak N, Caymo AM, et al. (2020) Mechanisms of muscle insulin resistance and the cross-talk with liver and adipose tissue. Physiol Rep 8: e14607. https://doi.org/10.14814/phy2.14607 |
[17] |
Donath MY, Ehses JA, Maedler K, et al. (2005) Mechanisms of beta-cell death in type 2 diabetes. Diabetes 54: S108-S113. https://doi.org/10.2337/diabetes.54.suppl_2.s108 ![]() |
[18] |
Chang-Chen KJ, Mullur R, Bernal-Mizrachi E (2008) Beta-cell failure as a complication of diabetes. Rev Endocr Metab Disord 9: 329-343. https://doi.org/10.1007/s11154-008-9101-5 ![]() |
[19] |
Rahier J, Guiot Y, Goebbels RM, et al. (2008) Pancreatic beta-cell mass in European subjects with type 2 diabetes. Diabetes Obes Metab 10: 32-42. https://doi.org/10.1111/j.1463-1326.2008.00969.x ![]() |
[20] |
Butler AE, Janson J, Bonner-Weir S, et al. (2003) Beta-cell deficit and increased beta-cell apoptosis in humans with type 2 diabetes. Diabetes 52: 102-110. https://doi.org/10.2337/diabetes.52.1.102 ![]() |
[21] |
Butler AE, Janson J, Soeller WC, et al. (2003) Increased beta-cell apoptosis prevents adaptive increase in beta-cell mass in mouse model of type 2 diabetes: evidence for role of islet amyloid formation rather than direct action of amyloid. Diabetes 52: 2304-2314. https://doi.org/10.2337/diabetes.52.9.2304 ![]() |
[22] |
Rhodes CJ (2005) Type 2 diabetes-a matter of beta-cell life and death?. Science 307: 380-384. https://doi.org/10.1126/science.1104345 ![]() |
[23] |
Araujo TG, Oliveira AG, Saad MJ (2013) Insulin-resistance-associated compensatory mechanisms of pancreatic Beta cells: a current opinion. Front Endocrinol (Lausanne) 4: 146. https://doi.org/10.3389/fendo.2013.00146 ![]() |
[24] |
Migliorini A, Bader E, Lickert H (2014) Islet cell plasticity and regeneration. Mol Metab 3: 268-274. https://doi.org/10.1016/j.molmet.2014.01.010 ![]() |
[25] |
Johnson JD, Yang YHC, Luciani DS (2015) Mechanisms of pancreatic β-cell apoptosis in diabetes and its therapies. Islets of Langerhans. Netherlands: Springer 873-894. ![]() |
[26] |
Tomita T (2010) Immunocytochemical localisation of caspase-3 in pancreatic islets from type 2 diabetic subjects. Pathology 42: 432-437. https://doi.org/10.3109/00313025.2010.493863 ![]() |
[27] |
Tomita T (2016) Apoptosis in pancreatic beta-islet cells in Type 2 diabetes. Bosn J Basic Med Sci 16: 162-179. https://doi.org/10.17305/bjbms.2016.919 ![]() |
[28] |
Ho FM, Liu SH, Liau CS, et al. (2000) High glucose-induced apoptosis in human endothelial cells is mediated by sequential activations of c-Jun NH(2)-terminal kinase and caspase-3. Circulation 101: 2618-2624. https://doi.org/10.1161/01.CIR.101.22.2618 ![]() |
[29] |
Federici M, Hribal M, Perego L, et al. (2001) High glucose causes apoptosis in cultured human pancreatic islets of Langerhans: a potential role for regulation of specific Bcl family genes toward an apoptotic cell death program. Diabetes 50: 1290-1301. https://doi.org/10.2337/diabetes.50.6.1290 ![]() |
[30] |
Johnson JD, Luciani DS (2010) Mechanisms of pancreatic beta-cell apoptosis in diabetes and its therapies. Adv Exp Med Biol 654: 447-462. https://doi.org/10.1007/978-90-481-3271-3_19 ![]() |
[31] |
Talchai C, Xuan S, Lin HV, et al. (2012) Pancreatic beta cell dedifferentiation as a mechanism of diabetic beta cell failure. Cell 150: 1223-1234. https://doi.org/10.1016/j.cell.2012.07.029 ![]() |
[32] |
Avrahami D, Wang YJ, Schug J, et al. (2020) Single-cell transcriptomics of human islet ontogeny defines the molecular basis of β-cell dedifferentiation in T2D. Mol Metab 42: 101057. https://doi.org/10.1016/j.molmet.2020.101057 ![]() |
[33] |
Efanova IB, Zaitsev SV, Zhivotovsky B, et al. (1998) Glucose and tolbutamide induce apoptosis in pancreatic beta-cells. A process dependent on intracellular Ca2+ concentration. J Biol Chem 273: 33501-33507. https://doi.org/10.1074/jbc.273.50.33501 ![]() |
[34] |
Lickert H (2013) Betatrophin fuels beta cell proliferation: first step toward regenerative therapy?. Cell Metab 18: 5-6. https://doi.org/10.1016/j.cmet.2013.06.006 ![]() |
[35] |
Crunkhorn S (2013) Metabolic disorders: betatrophin boosts beta-cells. Nat Rev Drug Discov 12: 504. https://doi.org/10.1038/nrd4058 ![]() |
[36] |
Kugelberg E (2013) Diabetes: Betatrophin--inducing beta-cell expansion to treat diabetes mellitus?. Nat Rev Endocrinol 9: 379. https://doi.org/10.1038/nrendo.2013.98 ![]() |
[37] |
Laing E, Smith CP (2010) RankProdIt: A web-interactive rank products analysis tool. BMC Res Notes 3: 221. https://doi.org/10.1186/1756-0500-3-221 ![]() |
[38] |
Breitling R, Armengaud P, Amtmann A, et al. (2004) Rank products: a simple, yet powerful, new method to detect differentially regulated genes in replicated microarray experiments. FEBS Lett 573: 83-92. https://doi.org/10.1016/j.febslet.2004.07.055 ![]() |
[39] |
Piñero J, Queralt-Rosinach N, Bravo À, et al. (2015) DisGeNET: a discovery platform for the dynamical exploration of human diseases and their genes. Database 2015: bav028. https://doi.org/10.1093/database/bav028 ![]() |
[40] | Xie Z, Bailey A, Kuleshov MV, et al. (2021) Gene set knowledge discovery with enrichr. Curr Protoc 1: e90. https://doi.org/10.1002/cpz1.90 |
[41] |
Clarke DJB, Jeon M, Stein DJ, et al. (2021) Appyters: Turning jupyter notebooks into data-driven web apps. Patterns 2: 100213. https://doi.org/10.1016/j.patter.2021.100213 ![]() |
[42] |
Krämer A, Green J, Pollard J, et al. (2014) Causal analysis approaches in Ingenuity Pathway Analysis. Bioinformatics 30: 523-530. https://doi.org/10.1093/bioinformatics/btt703 ![]() |
[43] |
Moreno-Asso A, Castaño C, Grilli A, et al. (2013) Glucose regulation of a cell cycle gene module is selectively lost in mouse pancreatic islets during ageing. Diabetologia 56: 1761-1772. https://doi.org/10.1007/s00125-013-2930-0 ![]() |
[44] |
Anderson DM, Makarewich CA, Anderson KM, et al. (2016) Widespread control of calcium signaling by a family of SERCA-inhibiting micropeptides. Sci Signal 9: ra119. https://doi.org/10.1126/scisignal.aaj1460 ![]() |
[45] |
Rossi AE, Dirksen RT (2006) Sarcoplasmic reticulum: the dynamic calcium governor of muscle. Muscle Nerve 33: 715-731. https://doi.org/10.1002/mus.20512 ![]() |
[46] |
Sabatini PV, Speckmann T, Lynn FC (2019) Friend and foe: β-cell Ca. Mol Metab 21: 1-12. https://doi.org/10.1016/j.molmet.2018.12.007 ![]() |
[47] |
Srinivasan S, Bernal-Mizrachi E, Ohsugi M, et al. (2002) Glucose promotes pancreatic islet beta-cell survival through a PI 3-kinase/Akt-signaling pathway. Am J Physiol Endocrinol Metab 283: E784-E793. https://doi.org/10.1152/ajpendo.00177.2002 ![]() |
[48] |
Klec C, Ziomek G, Pichler M, et al. (2019) Calcium signaling in ß-cell physiology and pathology: a revisit. Int J Mol Sci 20: 6110. https://doi.org/10.3390/ijms20246110 ![]() |
[49] |
Li K, Jiang Y, Xiang X, et al. (2020) Long non-coding RNA SNHG6 promotes the growth and invasion of non-small cell lung cancer by downregulating miR-101-3p. Thorac Cancer 11: 1180-1190. https://doi.org/10.1111/1759-7714.13371 ![]() |
[50] | Meng Q, Zhang F, Chen H, et al. lncRNA SNHG6 improves placental villous cell function in an in vitro model of gestational diabetes mellitus (2020). https://doi.org/10.5114/aoms.2020.100643 |
[51] |
Xu Z, Zuo Y, Wang J, et al. (2015) Overexpression of the regulator of G-protein signaling 5 reduces the survival rate and enhances the radiation response of human lung cancer cells. Oncol Rep 33: 2899-2907. https://doi.org/10.3892/or.2015.3917 ![]() |
[52] |
Deng W, Wang X, Xiao J, et al. (2012) Loss of regulator of G protein signaling 5 exacerbates obesity, hepatic steatosis, inflammation and insulin resistance. PLoS One 7: e30256. https://doi.org/10.1371/journal.pone.0030256 ![]() |
[53] |
Sun Y, Gao HY, Fan ZY, et al. (2020) Metabolomics signatures in type 2 diabetes: A systematic review and integrative analysis. J Clin Endocrinol Metab 105: 1000-1008. https://doi.org/10.1210/clinem/dgz240 ![]() |
[54] |
Ahmed F, Khan AA, Ansari HR, et al. (2022) A systems biology and LASSO-based approach to decipher the transcriptome-interactome signature for predicting non-small cell lung cancer. Biology 11: 1752. https://doi.org/10.3390/biology11121752 ![]() |
[55] |
Ahmed F (2020) A network-based analysis reveals the mechanism underlying vitamin D in suppressing cytokine storm and virus in SARS-CoV-2 infection. Front Immunol 11: 590459. https://doi.org/10.3389/fimmu.2020.590459 ![]() |
[56] |
Ahmed F (2019) Integrated network analysis reveals FOXM1 and MYBL2 as key regulators of cell proliferation in non-small cell lung cancer. Front Oncol 9: 1011. https://doi.org/10.3389/fonc.2019.01011 ![]() |
[57] |
Marselli L, Thorne J, Dahiya S, et al. (2010) Gene expression profiles of Beta-cell enriched tissue obtained by laser capture microdissection from subjects with type 2 diabetes. PLoS One 5: e11499. https://doi.org/10.1371/journal.pone.0011499 ![]() |
[58] |
Ostenson CG, Khan A, Abdel-Halim SM, et al. (1993) Abnormal insulin secretion and glucose metabolism in pancreatic islets from the spontaneously diabetic GK rat. Diabetologia 36: 3-8. https://doi.org/10.1007/BF00399086 ![]() |
[59] |
Khan A, Chandramouli V, Ostenson CG, et al. (1990) Glucose cycling in islets from healthy and diabetic rats. Diabetes 39: 456-459. https://doi.org/10.2337/diab.39.4.456 ![]() |
[60] |
Thomas HE, McKenzie MD, Angstetra E, et al. (2009) Beta cell apoptosis in diabetes. Apoptosis 14: 1389-1404. https://doi.org/10.1007/s10495-009-0339-5 ![]() |
[61] |
Costes S, Bertrand G, Ravier MA (2021) Mechanisms of beta-cell apoptosis in type 2 diabetes-prone situations and potential protection by GLP-1-based therapies. Int J Mol Sci 22: 5303. https://doi.org/10.3390/ijms22105303 ![]() |
[62] |
Šrámek J, Němcová-Fürstová V, Kovář J (2021) Molecular mechanisms of apoptosis induction and its regulation by fatty acids in pancreatic β-cells. Int J Mol Sci 22: 4285. https://doi.org/10.3390/ijms22084285 ![]() |
[63] |
Shen S, Wang F, Fernandez A, et al. (2020) Role of platelet-derived growth factor in type II diabetes mellitus and its complications. Diab Vasc Dis Res 17: 1479164120942119. https://doi.org/10.1177/1479164120942119 ![]() |
[64] |
Shan Z, Xu C, Wang W, et al. (2019) Enhanced PDGF signaling in gestational diabetes mellitus is involved in pancreatic β-cell dysfunction. Biochem Biophys Res Commun 516: 402-407. https://doi.org/10.1016/j.bbrc.2019.06.048 ![]() |
[65] |
Qin JJ, Yan L, Zhang J, et al. (2019) STAT3 as a potential therapeutic target in triple negative breast cancer: a systematic review. J Exp Clin Cancer Res 38: 195. https://doi.org/10.1186/s13046-019-1206-z ![]() |
[66] |
Jiang M, Li B (2022) STAT3 and its targeting inhibitors in oral squamous cell carcinoma. Cells 11: 3131. https://doi.org/10.3390/cells11193131 ![]() |
[67] |
Gorogawa S, Fujitani Y, Kaneto H, et al. (2004) Insulin secretory defects and impaired islet architecture in pancreatic beta-cell-specific STAT3 knockout mice. Biochem Biophys Res Commun 319: 1159-1170. https://doi.org/10.1016/j.bbrc.2004.05.095 ![]() |
[68] |
Weng Q, Zhao M, Zheng J, et al. (2020) STAT3 dictates β-cell apoptosis by modulating PTEN in streptozocin-induced hyperglycemia. Cell Death Differ 27: 130-145. https://doi.org/10.1038/s41418-019-0344-3 ![]() |
[69] |
Kisseleva T, Bhattacharya S, Braunstein J, et al. (2002) Signaling through the JAK/STAT pathway, recent advances and future challenges. Gene 285: 1-24. https://doi.org/10.1016/s0378-1119(02)00398-0 ![]() |
[70] |
Zong CS, Chan J, Levy DE, et al. (2000) Mechanism of STAT3 activation by insulin-like growth factor I receptor. J Biol Chem 275: 15099-15105. https://doi.org/10.1074/jbc.M000089200 ![]() |
[71] |
De Groef S, Renmans D, Cai Y, et al. (2016) STAT3 modulates β-cell cycling in injured mouse pancreas and protects against DNA damage. Cell Death Dis 7: e2272. https://doi.org/10.1038/cddis.2016.171 ![]() |
[72] |
Dalle S, Quoyer J, Varin E, et al. (2011) Roles and regulation of the transcription factor CREB in pancreatic β-cells. Curr Mol Pharmacol 4: 187-195. https://doi.org/10.2174/1874467211104030187 ![]() |
[73] |
Krabbe KS, Nielsen AR, Krogh-Madsen R, et al. (2007) Brain-derived neurotrophic factor (BDNF) and type 2 diabetes. Diabetologia 50: 431-438. https://doi.org/10.1007/s00125-006-0537-4 ![]() |
[74] |
Sun K, Wernstedt Asterholm I, Kusminski CM, et al. (2012) Dichotomous effects of VEGF-A on adipose tissue dysfunction. Proc Natl Acad Sci USA 109: 5874-5879. https://doi.org/10.1073/pnas.1200447109 ![]() |
[75] |
Guo H, Jin D, Zhang Y, et al. (2010) Lipocalin-2 deficiency impairs thermogenesis and potentiates diet-induced insulin resistance in mice. Diabetes 59: 1376-1385. https://doi.org/10.2337/db09-1735 ![]() |
[76] |
Pajvani UB, Shawber CJ, Samuel VT, et al. (2011) Inhibition of notch signaling ameliorates insulin resistance in a FoxO1-dependent manner. Nat Med 17: 961-967. https://doi.org/10.1038/nm.2378 ![]() |
[77] |
Liu S, Croniger C, Arizmendi C, et al. (1999) Hypoglycemia and impaired hepatic glucose production in mice with a deletion of the C/EBPbeta gene. J Clin Invest 103: 207-213. https://doi.org/10.1172/JCI4243 ![]() |
[78] |
Greenbaum LE, Li W, Cressman DE, et al. (1998) CCAAT enhancer-binding protein beta is required for normal hepatocyte proliferation in mice after partial hepatectomy. J Clin Invest 102: 996-1007. https://doi.org/10.1172/JCI3135 ![]() |
[79] |
Wang L, Shao J, Muhlenkamp P, et al. (2000) Increased insulin receptor substrate-1 and enhanced skeletal muscle insulin sensitivity in mice lacking CCAAT/enhancer-binding protein beta. J Biol Chem 275: 14173-14181. https://doi.org/10.1074/jbc.m000764200 ![]() |
[80] |
Lavine JA, Raess PW, Stapleton DS, et al. (2010) Cholecystokinin is up-regulated in obese mouse islets and expands beta-cell mass by increasing beta-cell survival. Endocrinology 151: 3577-3588. https://doi.org/10.1210/en.2010-0233 ![]() |
[81] |
Meek TH, Wisse BE, Thaler JP, et al. (2013) BDNF action in the brain attenuates diabetic hyperglycemia via insulin-independent inhibition of hepatic glucose production. Diabetes 62: 1512-1518. https://doi.org/10.2337/db12-0837 ![]() |
[82] |
Mattson MP, Maudsley S, Martin B (2004) BDNF and 5-HT: a dynamic duo in age-related neuronal plasticity and neurodegenerative disorders. Trends Neurosci 27: 589-594. https://doi.org/10.1016/j.tins.2004.08.001 ![]() |
[83] |
Mattson MP (2007) Calcium and neurodegeneration. Aging Cell 6: 337-350. https://doi.org/10.1111/j.1474-9726.2007.00275.x ![]() |
[84] |
Harding HP, Zeng H, Zhang Y, et al. (2001) Diabetes mellitus and exocrine pancreatic dysfunction in perk-/- mice reveals a role for translational control in secretory cell survival. Mol Cell 7: 1153-1163. https://doi.org/10.1016/s1097-2765(01)00264-7 ![]() |
[85] |
Zhang P, McGrath B, Li S, et al. (2002) The PERK eukaryotic initiation factor 2 alpha kinase is required for the development of the skeletal system, postnatal growth, and the function and viability of the pancreas. Mol Cell Biol 22: 3864-3874. https://doi.org/10.1128/mcb.22.11.3864-3874.2002 ![]() |
[86] |
Cavener DR, Gupta S, McGrath BC (2010) PERK in beta cell biology and insulin biogenesis. Trends Endocrinol Metab 21: 714-721. https://doi.org/10.1016/j.tem.2010.08.005 ![]() |
[87] |
Gurzov EN, Barthson J, Marhfour I, et al. (2012) Pancreatic beta-cells activate a JunB/ATF3-dependent survival pathway during inflammation. Oncogene 31: 1723-1732. https://doi.org/10.1038/onc.2011.353 ![]() |
[88] |
Tellez N, Joanny G, Escoriza J, et al. (2011) Gastrin treatment stimulates beta-cell regeneration and improves glucose tolerance in 95% pancreatectomized rats. Endocrinology 152: 2580-2588. https://doi.org/10.1210/en.2011-0066 ![]() |
[89] |
Yu C, Cui S, Zong C, et al. (2015) The orphan nuclear receptor NR4A1 protects pancreatic beta-cells from endoplasmic reticulum (ER) stress-mediated apoptosis. J Biol Chem 290: 20687-20699. https://doi.org/10.1074/jbc.M115.654863 ![]() |
[90] | Ogura A, Nishida T, Hayashi Y, et al. (1991) The development of the uteroplacental vascular system in the golden hamster mesocricetus auratus. J Anat 175: 65-77. |
[91] |
Ruest LB, Marcotte R, Wang E (2002) Peptide elongation factor eEF1A-2/S1 expression in cultured differentiated myotubes and its protective effect against caspase-3-mediated apoptosis. J Biol Chem 277: 5418-5425. https://doi.org/10.1074/jbc.M110685200 ![]() |
[92] |
Sun Y, Du C, Wang B, et al. (2014) Up-regulation of eEF1A2 promotes proliferation and inhibits apoptosis in prostate cancer. Biochem Biophys Res Commun 450: 1-6. https://doi.org/10.1016/j.bbrc.2014.05.045 ![]() |
[93] |
Larsson LI, Rehfeld JF, Sundler F, et al. (1976) Pancreatic gastrin in foetal and neonatal rats. Nature 262: 609-610. https://doi.org/10.1038/262609a0 ![]() |
[94] |
Povoski SP, Zhou W, Longnecker DS, et al. (1994) Stimulation of in vivo pancreatic growth in the rat is mediated specifically by way of cholecystokinin-A receptors. Gastroenterology 107: 1135-1146. https://doi.org/10.1016/0016-5085(94)90239-9 ![]() |
[95] |
Röhrborn D, Wronkowitz N, Eckel J (2015) DPP4 in diabetes. Front Immunol 6: 386. https://doi.org/10.3389/fimmu.2015.00386 ![]() |
[96] |
Boer GA, Holst JJ (2020) Incretin Hormones and Type 2 Diabetes-Mechanistic Insights and Therapeutic Approaches. Biology 9: 473. https://doi.org/10.3390/biology9120473 ![]() |
[97] |
Nagamine A, Hasegawa H, Hashimoto N, et al. (2017) The effects of DPP-4 inhibitor on hypoxia-induced apoptosis in human umbilical vein endothelial cells. J Pharmacol Sci 133: 42-48. https://doi.org/10.1016/j.jphs.2016.12.003 ![]() |
[98] |
Sato K, Aytac U, Yamochi T, et al. (2003) CD26/dipeptidyl peptidase IV enhances expression of topoisomerase II alpha and sensitivity to apoptosis induced by topoisomerase II inhibitors. Br J Cancer 89: 1366-1374. https://doi.org/10.1038/sj.bjc.6601253 ![]() |
[99] | Pro B, Dang NH (2004) CD26/dipeptidyl peptidase IV and its role in cancer. Histol Histopathol 19: 1345-1351. https://doi.org/10.14670/HH-19.1345 |
[100] |
Gaetaniello L, Fiore M, de Filippo S, et al. (1998) Occupancy of dipeptidyl peptidase IV activates an associated tyrosine kinase and triggers an apoptotic signal in human hepatocarcinoma cells. Hepatology 27: 934-942. https://doi.org/10.1002/hep.510270407 ![]() |
[101] |
Huang CN, Wang CJ, Lee YJ, et al. (2017) Active subfractions of abelmoschus esculentus substantially prevent free fatty acid-induced β cell apoptosis via inhibiting dipeptidyl peptidase-4. PLoS One 12: e0180285. https://doi.org/10.1371/journal.pone.0180285 ![]() |
[102] |
Kim W, Egan JM (2008) The role of incretins in glucose homeostasis and diabetes treatment. Pharmacol Rev 60: 470-512. https://doi.org/10.1124/pr.108.000604 ![]() |
[103] |
Kim SJ, Winter K, Nian C, et al. (2005) Glucose-dependent insulinotropic polypeptide (GIP) stimulation of pancreatic beta-cell survival is dependent upon phosphatidylinositol 3-kinase (PI3K)/protein kinase B (PKB) signaling, inactivation of the forkhead transcription factor Foxo1, and down-regulation of bax expression. J Biol Chem 280: 22297-22307. https://doi.org/10.1074/jbc.M500540200 ![]() |
[104] |
Ehses JA, Casilla VR, Doty T, et al. (2003) Glucose-dependent insulinotropic polypeptide promotes beta-(INS-1) cell survival via cyclic adenosine monophosphate-mediated caspase-3 inhibition and regulation of p38 mitogen-activated protein kinase. Endocrinology 144: 4433-4445. https://doi.org/10.1210/en.2002-0068 ![]() |
[105] |
Farngren J, Persson M, Ahren B (2018) Effects on the glucagon response to hypoglycaemia during DPP-4 inhibition in elderly subjects with type 2 diabetes: A randomized, placebo-controlled study. Diabetes Obes Metab 20: 1911-1920. https://doi.org/10.1111/dom.13316 ![]() |
[106] |
Senmaru T, Fukui M, Kobayashi K, et al. (2012) Dipeptidyl-peptidase IV inhibitor is effective in patients with type 2 diabetes with high serum eicosapentaenoic acid concentrations. J Diabetes Investig 3: 498-502. https://doi.org/10.1111/j.2040-1124.2012.00220.x ![]() |
[107] |
Kim YG, Hahn S, Oh TJ, et al. (2013) Differences in the glucose-lowering efficacy of dipeptidyl peptidase-4 inhibitors between Asians and non-Asians: a systematic review and meta-analysis. Diabetologia 56: 696-708. https://doi.org/10.1007/s00125-012-2827-3 ![]() |
[108] |
Park H, Park C, Kim Y, et al. (2012) Efficacy and safety of dipeptidyl peptidase-4 inhibitors in type 2 diabetes: meta-analysis. Ann Pharmacother 46: 1453-1469. https://doi.org/10.1345/aph.1R041 ![]() |
[109] |
Panina G (2007) The DPP-4 inhibitor vildagliptin: robust glycaemic control in type 2 diabetes and beyond. Diabetes Obes Metab 9: 32-39. https://doi.org/10.1111/j.1463-1326.2007.00763.x ![]() |
![]() |
![]() |
1. | Firoz Ahmed, Nitish Kumar Mishra, Othman A Alghamdi, Mohammad Imran Khan, Aamir Ahmad, Nargis Khan, Mohammad Rehan, Deciphering KDM8 dysregulation and CpG methylation in hepatocellular carcinoma using multi-omics and machine learning , 2024, 16, 1750-1911, 961, 10.1080/17501911.2024.2374702 |
Islets | Liver | |||||
Upregulated | Gene | Log2FC | p-value | Gene | Log2FC | p-value |
APOF | 7.36 | 0.00E+00 | CTSE | 7.21 | 0.00E+00 | |
CCK | 8.00 | 0.00E+00 | SERPINA3 | 5.73 | 0.00E+00 | |
EEF1A2 | 7.29 | 0.00E+00 | HOMER2 | 4.46 | 8.05E-06 | |
ALDOB | 7.15 | 1.61E-06 | MYL1 | 4.28 | 1.29E-05 | |
REG3A | 6.27 | 1.61E-06 | PEG3 | 3.95 | 2.25E-05 | |
FBP1 | 5.89 | 3.22E-06 | TK1 | 3.42 | 6.28E-05 | |
DCN | 5.36 | 1.29E-05 | UBA5 | 3.42 | 7.41E-05 | |
WWC1 | 4.69 | 2.58E-05 | Gm2036 | 2.97 | 1.59E-04 | |
Gm2663 | 4.35 | 3.86E-05 | GFRA1 | 3.11 | 2.14E-04 | |
CEBPB | 4.28 | 4.19E-05 | USP48 | 2.80 | 2.43E-04 | |
Downregulated | FAM151A | −5.42 | 0.00E+00 | C5 | −8.12 | 0.00E+00 |
ANKRD33B | −5.36 | 0.00E+00 | CIDEA | −7.85 | 0.00E+00 | |
Defb1 | −4.36 | 3.22E-06 | CIDEC | −6.09 | 1.61E-06 | |
FGF1 | −4.05 | 6.44E-06 | AQP4 | −5.57 | 4.83E-06 | |
APLP2 | −4.08 | 6.44E-06 | SPRR1A | −5.06 | 1.05E-05 | |
Snhg6 | −3.87 | 1.29E-05 | CADM1 | −4.57 | 2.25E-05 | |
Uox | −3.88 | 1.45E-05 | RGS16 | −4.82 | 2.25E-05 | |
PIP4K2C | −3.57 | 2.90E-05 | PLIN4 | −4.25 | 5.47E-05 | |
RBCK1 | −3.61 | 3.86E-05 | COL1A2 | −4.07 | 5.80E-05 | |
PRDX2 | −3.62 | 4.35E-05 | MAPKAPK2 | −4.17 | 5.96E-05 |
Muscle | Adipose | |||||
Upregulated | Gene | Log2FC | p-value | Gene | Log2FC | p-value |
Arxes1/Arxes2 | 6.43 | 0.00E+00 | SERPINA1 | 7.98 | 8.05E-07 | |
Gm2036 | 5.61 | 0.00E+00 | HAL | 6.56 | 3.22E-06 | |
SERPINA3 | 4.60 | 1.77E-05 | PTGIS | 4.64 | 1.29E-05 | |
Orm1 | 4.37 | 2.25E-05 | NCF4 | 4.49 | 2.90E-05 | |
PSAT1 | 4.51 | 2.25E-05 | Gm2036 | 3.92 | 4.35E-05 | |
LEP | 4.06 | 5.96E-05 | ADRB3 | 4.41 | 4.35E-05 | |
Acot1 | 3.59 | 1.05E-04 | GABRR2 | 3.83 | 4.35E-05 | |
RRAD | 3.56 | 1.11E-04 | Ces1g | 3.39 | 1.16E-04 | |
CIDEC | 3.58 | 1.45E-04 | CAP1 | 3.07 | 1.96E-04 | |
NPNT | 3.43 | 1.55E-04 | IKBIP | 2.84 | 2.70E-04 | |
Downregulated | PVALB | −6.14 | 0.00E+00 | Ear2 | −5.07 | 0.00E+00 |
Mup1 | −5.08 | 0.00E+00 | Snhg6 | −4.63 | 0.00E+00 | |
ANKRD33B | −4.45 | 6.44E-06 | Mup1 | −4.98 | 0.00E+00 | |
RGS5 | −4.42 | 6.44E-06 | CCK | −4.89 | 0.00E+00 | |
Snhg6 | −4.02 | 1.29E-05 | FCGR2B | −4.79 | 0.00E+00 | |
PRDX2 | −3.65 | 3.38E-05 | PRDX2 | −4.21 | 1.61E-06 | |
HIST1H2BI | −3.46 | 3.86E-05 | SLC1A5 | −4.08 | 4.83E-06 | |
NEDD1 | −3.38 | 4.83E-05 | Ear3 | −3.85 | 1.13E-05 | |
CTSH | −3.40 | 5.47E-05 | GRK5 | −3.52 | 2.25E-05 | |
Actn3 | −3.91 | 6.92E-05 | HIST1H2BI | −3.28 | 3.06E-05 |
GO biological process | M. musculus REFLIST (21988) | Upregulated (104) | Upregulated gene | Upregulated (expected) | Upregulated (fold Enrichment) | Upregulated (raw P-value) | Upregulated (FDR) |
Negative regulation of neuron apoptotic process (GO:0043524) | 183 | 7 | Reg3b, CEBPB, BDNF, SLC1A1, LGMN, fam134b, KDR | 0.87 | 8.09 | 3.28E-05 | 2.15E-02 |
Regulation of apoptotic process (GO:0042981) | 1468 | 19 | CLDN7, PHLDA3, OLFM1, EIF2AK3, ZFP36L1, GADD45A, Reg3b, CEBPB, BDNF, SLC1A1, ZBTB16, EEF1A2, LGMN, fam134b, ATF3, CCK, KDR, BCL6, NR4A1 | 6.94 | 2.74 | 5.91E-05 | 2.59E-02 |
Regulation of programmed cell death (GO:0043067) | 1504 | 19 | CLDN7, PHLDA3, OLFM1, EIF2AK3, ZFP36L1, GADD45A, Reg3b, CEBPB, BDNF, SLC1A1, ZBTB16, EEF1A2, LGMN, fam134b, ATF3, CCK, KDR, BCL6, NR4A1 | 7.11 | 2.67 | 8.14E-05 | 3.13E-02 |
Regulation of cell death (GO:0010941) | 1667 | 20 | CLDN7, PHLDA3, CTGF, OLFM1, EIF2AK3, ZFP36L1, GADD45A, Reg3b, CEBPB, BDNF, SLC1A1, ZBTB16, EEF1A2, LGMN, fam134b, ATF3, CCK, KDR, BCL6, NR4A1 | 7.88 | 2.54 | 1.04E-04 | 3.88E-02 |
Regulators | Target molecules in dataset | Diseases & Functions | Consistency score |
CREB1,EGR1,FGF2,P38 MAPK,Pka | ATF3,CCK,JUNB,NR4A1 | Apoptosis of islets of Langerhans, Cell death of beta islets cells | 11.5 |
ERK1/2,NFkB (complex) | BDNF,C3,CCK,EIF2AK3,FGF1,GAST,KDR,LCN2,RGS16 | Quantity of metal, Release of metal | 5 |
EGF,P38 MAPK | ATF3,CTGF,IGFBP5,KLF10 | Increased Levels of Alkaline Phosphatase | 3 |
TNF | ATF3,DPP4,GAST,JUNB,NR4A1 | Apoptosis of islets of Langerhans | −4.919 |
CTNNB1 | ACTA2,ANXA1,AQP4,GNAO1,KDR,NR4A1 | Permeability of vascular system | −5.307 |
Islets | Liver | |||||
Upregulated | Gene | Log2FC | p-value | Gene | Log2FC | p-value |
APOF | 7.36 | 0.00E+00 | CTSE | 7.21 | 0.00E+00 | |
CCK | 8.00 | 0.00E+00 | SERPINA3 | 5.73 | 0.00E+00 | |
EEF1A2 | 7.29 | 0.00E+00 | HOMER2 | 4.46 | 8.05E-06 | |
ALDOB | 7.15 | 1.61E-06 | MYL1 | 4.28 | 1.29E-05 | |
REG3A | 6.27 | 1.61E-06 | PEG3 | 3.95 | 2.25E-05 | |
FBP1 | 5.89 | 3.22E-06 | TK1 | 3.42 | 6.28E-05 | |
DCN | 5.36 | 1.29E-05 | UBA5 | 3.42 | 7.41E-05 | |
WWC1 | 4.69 | 2.58E-05 | Gm2036 | 2.97 | 1.59E-04 | |
Gm2663 | 4.35 | 3.86E-05 | GFRA1 | 3.11 | 2.14E-04 | |
CEBPB | 4.28 | 4.19E-05 | USP48 | 2.80 | 2.43E-04 | |
Downregulated | FAM151A | −5.42 | 0.00E+00 | C5 | −8.12 | 0.00E+00 |
ANKRD33B | −5.36 | 0.00E+00 | CIDEA | −7.85 | 0.00E+00 | |
Defb1 | −4.36 | 3.22E-06 | CIDEC | −6.09 | 1.61E-06 | |
FGF1 | −4.05 | 6.44E-06 | AQP4 | −5.57 | 4.83E-06 | |
APLP2 | −4.08 | 6.44E-06 | SPRR1A | −5.06 | 1.05E-05 | |
Snhg6 | −3.87 | 1.29E-05 | CADM1 | −4.57 | 2.25E-05 | |
Uox | −3.88 | 1.45E-05 | RGS16 | −4.82 | 2.25E-05 | |
PIP4K2C | −3.57 | 2.90E-05 | PLIN4 | −4.25 | 5.47E-05 | |
RBCK1 | −3.61 | 3.86E-05 | COL1A2 | −4.07 | 5.80E-05 | |
PRDX2 | −3.62 | 4.35E-05 | MAPKAPK2 | −4.17 | 5.96E-05 |
Muscle | Adipose | |||||
Upregulated | Gene | Log2FC | p-value | Gene | Log2FC | p-value |
Arxes1/Arxes2 | 6.43 | 0.00E+00 | SERPINA1 | 7.98 | 8.05E-07 | |
Gm2036 | 5.61 | 0.00E+00 | HAL | 6.56 | 3.22E-06 | |
SERPINA3 | 4.60 | 1.77E-05 | PTGIS | 4.64 | 1.29E-05 | |
Orm1 | 4.37 | 2.25E-05 | NCF4 | 4.49 | 2.90E-05 | |
PSAT1 | 4.51 | 2.25E-05 | Gm2036 | 3.92 | 4.35E-05 | |
LEP | 4.06 | 5.96E-05 | ADRB3 | 4.41 | 4.35E-05 | |
Acot1 | 3.59 | 1.05E-04 | GABRR2 | 3.83 | 4.35E-05 | |
RRAD | 3.56 | 1.11E-04 | Ces1g | 3.39 | 1.16E-04 | |
CIDEC | 3.58 | 1.45E-04 | CAP1 | 3.07 | 1.96E-04 | |
NPNT | 3.43 | 1.55E-04 | IKBIP | 2.84 | 2.70E-04 | |
Downregulated | PVALB | −6.14 | 0.00E+00 | Ear2 | −5.07 | 0.00E+00 |
Mup1 | −5.08 | 0.00E+00 | Snhg6 | −4.63 | 0.00E+00 | |
ANKRD33B | −4.45 | 6.44E-06 | Mup1 | −4.98 | 0.00E+00 | |
RGS5 | −4.42 | 6.44E-06 | CCK | −4.89 | 0.00E+00 | |
Snhg6 | −4.02 | 1.29E-05 | FCGR2B | −4.79 | 0.00E+00 | |
PRDX2 | −3.65 | 3.38E-05 | PRDX2 | −4.21 | 1.61E-06 | |
HIST1H2BI | −3.46 | 3.86E-05 | SLC1A5 | −4.08 | 4.83E-06 | |
NEDD1 | −3.38 | 4.83E-05 | Ear3 | −3.85 | 1.13E-05 | |
CTSH | −3.40 | 5.47E-05 | GRK5 | −3.52 | 2.25E-05 | |
Actn3 | −3.91 | 6.92E-05 | HIST1H2BI | −3.28 | 3.06E-05 |
GO biological process | M. musculus REFLIST (21988) | Upregulated (104) | Upregulated gene | Upregulated (expected) | Upregulated (fold Enrichment) | Upregulated (raw P-value) | Upregulated (FDR) |
Negative regulation of neuron apoptotic process (GO:0043524) | 183 | 7 | Reg3b, CEBPB, BDNF, SLC1A1, LGMN, fam134b, KDR | 0.87 | 8.09 | 3.28E-05 | 2.15E-02 |
Regulation of apoptotic process (GO:0042981) | 1468 | 19 | CLDN7, PHLDA3, OLFM1, EIF2AK3, ZFP36L1, GADD45A, Reg3b, CEBPB, BDNF, SLC1A1, ZBTB16, EEF1A2, LGMN, fam134b, ATF3, CCK, KDR, BCL6, NR4A1 | 6.94 | 2.74 | 5.91E-05 | 2.59E-02 |
Regulation of programmed cell death (GO:0043067) | 1504 | 19 | CLDN7, PHLDA3, OLFM1, EIF2AK3, ZFP36L1, GADD45A, Reg3b, CEBPB, BDNF, SLC1A1, ZBTB16, EEF1A2, LGMN, fam134b, ATF3, CCK, KDR, BCL6, NR4A1 | 7.11 | 2.67 | 8.14E-05 | 3.13E-02 |
Regulation of cell death (GO:0010941) | 1667 | 20 | CLDN7, PHLDA3, CTGF, OLFM1, EIF2AK3, ZFP36L1, GADD45A, Reg3b, CEBPB, BDNF, SLC1A1, ZBTB16, EEF1A2, LGMN, fam134b, ATF3, CCK, KDR, BCL6, NR4A1 | 7.88 | 2.54 | 1.04E-04 | 3.88E-02 |
Regulators | Target molecules in dataset | Diseases & Functions | Consistency score |
CREB1,EGR1,FGF2,P38 MAPK,Pka | ATF3,CCK,JUNB,NR4A1 | Apoptosis of islets of Langerhans, Cell death of beta islets cells | 11.5 |
ERK1/2,NFkB (complex) | BDNF,C3,CCK,EIF2AK3,FGF1,GAST,KDR,LCN2,RGS16 | Quantity of metal, Release of metal | 5 |
EGF,P38 MAPK | ATF3,CTGF,IGFBP5,KLF10 | Increased Levels of Alkaline Phosphatase | 3 |
TNF | ATF3,DPP4,GAST,JUNB,NR4A1 | Apoptosis of islets of Langerhans | −4.919 |
CTNNB1 | ACTA2,ANXA1,AQP4,GNAO1,KDR,NR4A1 | Permeability of vascular system | −5.307 |