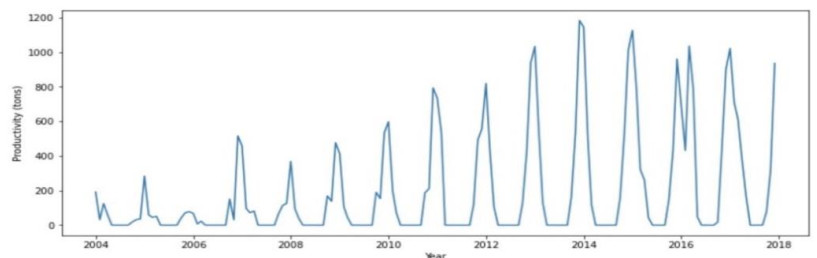
Citation: Katharina Biely, Erik Mathijs, Steven Van Passel. Causal loop diagrams to systematically analyze market power in the Belgian sugar value chain[J]. AIMS Agriculture and Food, 2019, 4(3): 711-730. doi: 10.3934/agrfood.2019.3.711
[1] | Jahanara Akter, Sadia Islam Nilima, Rakibul Hasan, Anamika Tiwari, Md Wali Ullah, Md Kamruzzaman . Artificial intelligence on the agro-industry in the United States of America. AIMS Agriculture and Food, 2024, 9(4): 959-979. doi: 10.3934/agrfood.2024052 |
[2] | Ayomikun D. Ajayi, Boris Boiarskii, Kouya Aoyagi, Hideo Hasegawa . Utilizing MapBox API, Java, and ICT in the creation of agricultural interactive maps for improved farm management and decision-making. AIMS Agriculture and Food, 2024, 9(2): 393-410. doi: 10.3934/agrfood.2024023 |
[3] | Mohammad M. Islam, Majed Alharthi, Rotana S. Alkadi, Rafiqul Islam, Abdul Kadar Muhammad Masum . Crop yield prediction through machine learning: A path towards sustainable agriculture and climate resilience in Saudi Arabia. AIMS Agriculture and Food, 2024, 9(4): 980-1003. doi: 10.3934/agrfood.2024053 |
[4] | Radhwane Derraz, Farrah Melissa Muharam, Noraini Ahmad Jaafar . Uncertainty sources affecting operational efficiency of ML algorithms in UAV-based precision agriculture: A 2013–2020 systematic review. AIMS Agriculture and Food, 2023, 8(2): 687-719. doi: 10.3934/agrfood.2023038 |
[5] | Nicholas Ngepah, Regret Sunge . Agricultural expenditure and agricultural total factor productivity growth in South Africa. AIMS Agriculture and Food, 2023, 8(2): 637-661. doi: 10.3934/agrfood.2023035 |
[6] | Jan Willem Erisman, Nick van Eekeren, Jan de Wit, Chris Koopmans, Willemijn Cuijpers, Natasja Oerlemans, Ben J. Koks . Agriculture and biodiversity: a better balance benefits both. AIMS Agriculture and Food, 2016, 1(2): 157-174. doi: 10.3934/agrfood.2016.2.157 |
[7] | Emilio J. González-Sánchez, Amir Kassam, Gottlieb Basch, Bernhard Streit, Antonio Holgado-Cabrera, Paula Triviño-Tarradas . Conservation Agriculture and its contribution to the achievement of agri-environmental and economic challenges in Europe. AIMS Agriculture and Food, 2016, 1(4): 387-408. doi: 10.3934/agrfood.2016.4.387 |
[8] | Cíntia Sorane Good Kitzberger, Maria Brígida dos Santos Scholz, João Batista Gonçalves Dias da Silva, Marta de Toledo Benassi, Luiz Filipe Protasio Pereira . Free choice profiling sensory analysis to discriminate coffees. AIMS Agriculture and Food, 2016, 1(4): 455-469. doi: 10.3934/agrfood.2016.4.455 |
[9] | Tineka R. Burkhead, Vincent P. Klink . American agricultural commodities in a changing climate. AIMS Agriculture and Food, 2018, 3(4): 406-425. doi: 10.3934/agrfood.2018.4.406 |
[10] | Edward L. Kick, Kelly Zering, John Classen . Approaches to agricultural innovation and their effectiveness. AIMS Agriculture and Food, 2017, 2(4): 370-373. doi: 10.3934/agrfood.2017.4.370 |
In the agricultural sector, there remain challenges in agricultural management in response to customer needs. The cause of this problem is from lack of know-how and knowledge management [1,2,3]. These issues indicate that entrepreneurs need effective tools for developing and increasing productivity in the production process for long-term stability. Additionally, inaccurate yield prediction can have far-reaching effects on food production, supply systems, economies and global food security. Uncertainty in predicting crop yields and quality can lead to lower crop yields, reduced income, financial instability for agricultural producers, increased production costs, shortages and price fluctuations [4]. In marketing, it affects price volatility, suboptimal policy choices and resource misallocation and disrupts international trade agreements and negotiations, affecting trade balances and economic stability [5]. Accurate forecasting is more critical for farmers who need to adapt their practices to changing climate conditions [6].
We focused on arabica coffee (Coffea arabica L.) grown in northern Thailand. It is among the most popular species of coffee due to its features and flavor that offer superior quality than other types [7]. Currently, the coffee business is becoming more competitive. Its production forecast is of great interest to stakeholders involved. A lack of certainty in forecasting coffee production is especially vulnerable, affecting the whole supply chain from coffee farmers to exporters, importers, roasters and retailers, leading to supply gaps, disappointing customers and potentially damaging brand reputation [8]. Furthermore, price volatility will affect their profitability and financial planning [9]. Uncertain forecasts can lead to overstocking or understocking [10]. Coffee cultivation is usually long-term. If the farmer or entrepreneurs lack knowledge of the processing management, it will result in the uncertainty in production forecasts [11].
To address these challenges in coffee businesses, artificial intelligence (AI) is considered essential for modern manufacturing processes in agriculture and industry. This technology is likely to generate increased efficacy and effectiveness in the production process to enhance companies' potential according to international standards. Over the past few years, the agriculture and industry sectors have introduced various technologies to increasingly modernize their manufacturing and agricultural operations. AI is also being used to analyze the data and accelerate the operating system with flexibility that leads to more effectiveness in producing products or services in accordance with customer needs [12,13,14].
The artificial neural network (ANN), an algorithm of machine learning (ML) model, is viewed as a vital data-modeling tool [15]. It can calculate the data through the functional structures of neural networks. Input and output data processes are run through a neuron network, including single-layer perceptions, multilayer perceptions, recurrent ANN and self-organization mapping [16]. Recently, many agriculture sectors applied the ANN to predict the productivity of products [17,18]. Kittichotsatsawat et al. [19] used basic ANN models to predict the productivity of coffee in northern Thailand. Bhojani et al. [20] applied ANN to wheat yield prediction. Palanivel et al. [21] also utilized ANN to predict crop yield. Important factors considered include the area, productivity zone, rainfall, relative humidity, temperature, etc. [22,23,24,25,26]. It is noted here that, apart from applying ML models, other methods can help to improve the productivity of cherry coffee. Examples are the analytical hierarchy process (AHP) and frequency ratio (FR), attention mechanism (AM), convolutional neural network (CNN), hyperspectral image (HSI), spectral–spatial features from principal component analysis (PCA), weighted linear combination (WLC), attitude determination and control subsystem (ADCS) models [27,28,29,30].
Autoregressive integrated moving average (ARIMA) model is one of the statistical tools that has been used to predict various agricultural product output. It can detect the data through Box-Jenkins in order to create the ARIMA model, including (i) stationary, (ii) co–integration and (iii) error correction mechanism [31]. Padhan [32] employed ARIMA to forecast agricultural productivity in India. ARIMA was used to predict the productivity of goods in terms of area, zone, relative humidity, rainfall, temperature, etc. [33,34,35,36].
Techniques such as ANN and ARIMA may be used to determine the productivity of coffee to meet customer requirements. From the literature review, there have been several works applying the ANN and ARIMA models to predict agricultural productivity, such as commodities, agricultural products, crop price, etc. [37,38,39]. However, it was noticed that ARIMA and ANN are not yet employed in coffee production forecast. Therefore, we aim to predict the cherry coffee yield and compare the performance between the ARIMA and ANN models. This finding will benefit and help in analyzing the trends of Thai coffee effectively and sustainably.
In this study, datasets were collected for 15 years from 2004 to 2018 (180 months). The input predictor data considered were from the Thai Agricultural Economics Office and the Meteorological Department. They included the cultivated area, productivity zone, monthly rainfall, monthly RH and monthly temperatures. Furthermore, the output data was from the productivity yield each year. It was noted that the coffee was yielded only six months in a year with increasing trend for the past several years, shown in Figure 1.
Based on the literature, it is necessary to normalize and standardize the values of input features and output targets before developing ML models [40,41]. In this work, the input and output variables are normalized in the range 0‒1, using:
N=(X−Xmin)(Xmax−Xmin) | (1) |
where N is the normalized data; X is the measured value: Xmin and Xmax are the minimum and maximum values.
The ARIMA and ANN performances had to be measured according to a validation of variables dataset. The ANN selected was tested. Then, the coefficient of determination (R2), the root means square error (RMSE) [42,43] as well as the mean squared error (MSE), were compared.
SST=∑ni=1(y−¯y)2=(y−¯y)′(y−1¯y) | (2) |
SSR=∑ni=1(ˆyi−¯y)2=(ˆy−¯y)′(ˆy−1¯y) | (3) |
R2=1−SSregressSStotal | (4) |
where yi and ŷ are the square of the sample correlation, SSregression is the sum of squares due to regression (explained sum of squares) and SStotal is the total sum of squares.
RMSE=√1n∑ni=1[E(xi)−M(xi)]2 | (5) |
MSE=(RMSE)2 | (6) |
where n is the sample size of the testing dataset, while E(xi) and M(xi) are interpolated/predicted and observed values, respectively.
A prediction is built on the foundation of some scientific calculation based on historical data. The variable datasets were analyzed through the ARIMA model with Python programming and ANN model using MATLAB programming.
ARIMA model is a technique of statistics and econometrics that evaluates the events that will happen over each period of time.
yt=θ0+∅1yt−1+∅2yt−2+⋯+∅pyt−p+εt−θ1εt−1−θ2εt−2−⋯−θqεt−q, | (7) |
where yt and ɛt are the actual value and random error at time period t, respectively; ɸi (I = 1, 2…., p) and ɵj (j = 0, 1, 2…, q) are model parameter. p and q are integers and often referred to as order of the model. Random errors, ɛt, are assumed to be independently and identically distributed with a mean of zero and a constant variance of σ2.
Yt=f(t)+εt | (8) |
where, Yt signifies production for the time t in year, f (t) denotes a function of time t and ɛt denotes production error (i.e., the difference between observed and forecasted production for time t year). Once a functional link between production and time (in other words, a time series model) has been built, production for year t + 1 can be forecasted. The first stage in creating this model is determining whether the time series under consideration is stationary or non-stationary.
φp(B)Δdht=c+θq(B)gt | (9) |
where, ht is variable under forecasting at time t, B is lag operator, g is error term (Y-Ŷ in which Ŷ is the estimated value of Y), 𝜑𝑝(𝐵) is non-seasonal AR i.e., the autoregressive operator, represented as a polynomial in the back shift operator, (1 − B) d is non-seasonal difference, θq(B) is non-seasonal moving average i.e., the moving average operator, represented as a polynomial in the backshift operator, φ′ s and θ′ s are the parameters to be estimated.
The variable datasets were prepared to consider the time series component, including trend, season, cycle and irregularity. ARIMA model was split into two parts, with 156 data for training and 24 for testing. The historical observations and random mistakes (errors) were used to estimate the future variables dataset. It was shown on Box-Jenkins to predict the future value through ARIMA modeling, including a three-step iterative technique (i) model identification, (ii) parameter estimation and (iii) residual diagnostics testing [44].
The unit root test series graphs revealed the autocorrelation function (ACF) and partial ACF (PACF) [45]. The zig-zag trend will show the increase to meet the stationary series graphs. After the stationary time series process was identified, the ARIMA model was defined by the autoregressive integrated moving average model (p, d, q). Python programming was used to detect a suitable residual of the ACF graph with a 95% confidence band [46]. Next, a suitable ARIMA model was used in prediction (156 data for training and 24 data for testing).
ANN is a complex multivariate model to approximate the unknown expectation function of a random variable. Weights will be used to estimate the parameters in the ANN model.
ti=∑nj−1WijXj | (10) |
where n is number of inputs, w is weight of the connection between ith and jth node and x is input from node j. Calculation of output will be analyzed through a transfer function of Oi;
Oi=f(ti) | (11) |
The variable datasets are randomly separated into three groups to prevent overfitting. The variable data were divided randomly whose 70% used for training, 15% for validating and 15% for testing [47]. During ANN training, the algorithm was furnished with the performance of minimum or maximum through the shortest path in order to gain the network's yield size. The neural network performance was accomplished by backpropagation via the training set in order to update the minimum MSE during the training set [48].
The neural networks were trained by means of a training set, and the output datasets were compared with the fixed weight. Then, feed-forward backpropagation was utilized to compare output datasets with the fixed weight. The MSE was utilized to test and calculate epochs to validate the variable dataset in the part of the neural network running. Neurons of the neural network will utilize a definite function in the hidden layer and gather the combination and bias. Lastly, the output of variable data will give the predicted model [49]. The crop yield index was determined via the input and output variables set. During the neural network process, each independent variable set was assessed and revealed by a partial dependence plot (PDP) [50,51]. The highest importance value showed the relative importance of that parameter from each index.
The crop yield of cherry coffee was validated through the input variables dataset based on the difference between observed and predicted coffee crops. The leave-one-out cross-validation technique was used to be randomly evaluated with the ANN model, while the ARIMA model used time-based cross-validation. The 156 months were evenly partitioned in order to evaluate the cross-validation. Three rounds of ANN and ARIMA were randomly evaluated throughout variable datasets. The variable datasets were trained and treated individually in each dataset. Finally, the performance model was evaluated and showed the RMSE and MSE. The best model was determined through the largest R2 and the smallest RMSE.
Time series analysis of ARIMA was completed based on the 180 monthly input variables dataset. Variable datasets were detected through the unit root with stationary test in order to examine the stationary or non-stationary data. A statistical test was used to consider these data, specifically the Augmented Dickey-Fuller (ADF) test, and based on the p-value of the ADF-test, if the result shows a value less than 0.05, it will be identified as stationary [52].
After the stationary test, the p-value of 0.9210 was obtained and variable datasets were non-stationary. However, when examining the data with ACF and PACF as in Figure 2, it was shown that the time series was revealed as seasonal and stationary. Nonetheless, the data was adjusted and rearranged to investigate the difference of the first number (d = 1). This time, the p-value was 1.426 x 10−13, thus, the variable data was identified as stationary. However, the ACF and PACF showed the space of seasonal components (12, 24, 36 units) in Figure 3.
The data was divided into two parts, including 156 for training data and 24 for testing data. Then, tuning the model by training data through ARIMA model fitting was carried out in order to define the parameters through 64 conditions based on the autoregressive (AR(p)), integrated (I(d)) and moving average (MA(q)) [53]. The Akaike information criteria (AIC) was used to return the conditions of each value [54], as shown in Table 1.
No. | Parameter | AIC |
1 | (2, 1, 2) | −168.0802 |
2 | (2, 1, 3) | −166.9035 |
3 | (2, 0, 3) | −166.7370 |
4 | (3, 1, 2) | −166.4853 |
5 | (3, 0, 2) | −165.7990 |
Table 1 shows the five most minor (p, d, q) condition parameters; the AIC of (p, d, q) is 168.0802 at (2, 1, 2). However, when (p, d, q) parameters were identified, it led to cross validation based on time-based cross-validation. The data was changed from random sampling to one by one through a training model or forward chaining by identifying the data ratio between training data and data of testing amounted 12 rounds.
In Table 2, the ARIMA model shows R2 of 0.0741, RMSE of 0.1348, and MSE of 0.0181. However, the relation of target and output of variable datasets, which is the trends of the data association, was unidirectional, as shown in Figure 4. While the results of cross-validation showed an average R2 to be higher, while the average of MSE of test data was smaller. So, this model configuration is suitable, and it can be used in prediction.
Training and Testing | Time-based cross-validation | ||||||||
MSE Train |
MSE Test |
RMSE Train |
RMSE Test |
R2 Train |
R2 Test |
R2 Train | R2 Test |
MSE Train |
MSE Test |
0.0181 | 0.0469 | 0.1348 | 0.2167 | 0.7041 | 0.3521 | 0.7383 | 0.3931 | 0.0046 | 0.0451 |
The ANN analysis was achieved based on the 180 monthly input data. The variable datasets included the cultivated area (X1) for the coffee. The productivity zone (X2) is the factor that implies the quantity of cherry coffee in each crop. The rainfall data (X3) is a crucial factor for the output of coffee in each year. The proper amount of RH (X4) will enable the high quantity of coffee yield. The maximum and minimum ambient temperatures (X5 and X6) are essential to the coffee productivity.
For ANN hyperparameter setting, the number of hidden layers and the number of neurons (i.e., processing elements (PEs)) for each hidden layer were optimized by trial and error in predicting the coffee yields. The MSE, RMSE and R2 values were used to evaluate the optimal parameters. From Table 3, the network properties were arranged with (a) network type of feed-forward backpropagation, (b) training and adaptation learning functions through Levenberg-Marquardt algorithm with TRAINLM and LEARNGDM, (c) MSE of performance function, (d) varying hidden layers and six neurons and (e) TANSIG transfer function [55]. After that, the performances of the ANN models to predict cherry coffee productivity yield were evaluated for various ANN configurations. The best training results of the ANN model were two hidden layers and one PE for each hidden layer, which provided the R values of the training, testing, validating data phases to be 0.9921, 0.9384 and 0.8723, respectively, and the MSE of the validating data to be 19576, as also shown in Figures 5 and 6.
Number of hidden layers | PEs | MSE | R Training |
R Testing |
R Validation |
R Overall |
R2 |
1 | 1 | 20791 | 0.9014 | 0.5100 | 0.9196 | 0.8491 | 0.7210 |
1 | 2 | 27138 | 0.7977 | 0.7603 | 0.5115 | 0.7764 | 0.6028 |
1 | 3 | 40226 | 0.8079 | 0.8405 | 0.7176 | 0.7965 | 0.6344 |
1 | 4 | 42773 | 0.7506 | 0.3192 | 0.7525 | 0.7096 | 0.5035 |
1 | 5 | 50880 | 0.8474 | 0.7186 | 0.4740 | 0.7795 | 0.6076 |
1 | 6 | 39929 | 0.8580 | 0.6132 | 0.7423 | 0.7794 | 0.6075 |
1 | 7 | 72995 | 0.9761 | 0.6014 | 0.4396 | 0.8464 | 0.7164 |
1 | 8 | 20092 | 0.7720 | 0.6825 | 0.6804 | 0.7431 | 0.5522 |
1 | 9 | 20419 | 0.9798 | 0.8299 | 0.8797 | 0.9291 | 0.8632 |
1 | 10 | 15591 | 0.9810 | 0.5857 | 0.9452 | 0.9000 | 0.8100 |
2 | 1 | 19576 | 0.9921 | 0.9384 | 0.8723 | 0.9643 | 0.9299 |
2 | 2 | 20384 | 0.7900 | 0.7736 | 0.6675 | 0.7807 | 0.6095 |
2 | 3 | 14133 | 0.7776 | 0.8219 | 0.7922 | 0.7747 | 0.6002 |
2 | 4 | 27636 | 0.9143 | 0.5913 | 0.8700 | 0.8816 | 0.7772 |
2 | 5 | 28923 | 0.9297 | 0.7595 | 0.9057 | 0.9085 | 0.8254 |
2 | 6 | 16209 | 0.9131 | 0.7751 | 0.7987 | 0.8690 | 0.7552 |
2 | 7 | 37072 | 0.7236 | 0.6636 | 0.6070 | 0.7042 | 0.4959 |
2 | 8 | 16657 | 0.9825 | 0.9049 | 0.8930 | 0.9629 | 0.9272 |
2 | 9 | 7218 | 0.8151 | 0.6677 | 0.8071 | 0.8039 | 0.6463 |
2 | 10 | 16288 | 0.9761 | 0.6510 | 0.8018 | 0.9164 | 0.8398 |
Figure 5 shows the validation performance with the MSE value of the validating data for the best training results of the ANN model. The learning rate was set to be 0.02, while the learning cycles of the model was done to be 1000 epochs. The optimal validation performance of the ANN model with two hidden layers and one PE each was found at 13 epochs, which was based on the lowest MSE value of the validating data for this ANN configuration.
Figure 6 shows the predicted values versus measured values for training, testing, validating data and whole data. The perfect prediction established the accuracy of the neural network in predicting the cherry coffee productivity yield based on the calculation of the index of the variable.
Figure 7 shows one-way partial dependence plots (PDPs) for each variable's relative importance. The monthly temperature (X5) (Figure 7e) showed a higher effect on a variable dataset in this model, with the PDP value varied from 0.0501 to 0.5211. The second most important predictor was the productivity zone (X2) (Figure 7b), with the PDP value from 0.0896 to 0.2929. Similarly, the third crucial variable was revealed to be monthly rainfall (X3) (Figure 7c), showing the PDP value from 0.0899 to 0.2760. Moreover, the cultivated area (X1) (Figure 7a) and minimum monthly temperature (X6) (Figure 7f) showed the PDP value of small difference, which were 0.1373 to 0.2010 and 0.1883 to 0.1275, respectively. Lastly, the relative humidity (X4) showed marginal effects on the model PDP with values from 0.1295 to 0.1670.
The maximum temperature (e) affected the amount of productivity significantly. If the temperature was higher than or equal to 29 ℃, the productivity was decreased. If the minimum temperature (f) was less than or equal to 15–20 ℃, the productivity was improved. The rainfall (c) was one of the essential factors because the coffee productivity depended on the amount of rainfall each year. A suitable rainfall should be less than 100 mm, leading to good coffee plantation condition. The productivity zone (b) and cultivated area (a) were directly affected the quantity of coffee production If the farmers have more productivity zone and area, they will have higher production. Finally, relative humidity (d) should be high because it is preferable for coffee cultivation.
Yield of arabica coffee is relatively unstable due to many factors, for example, changing weather conditions, different soil pH, fluctuation of ambient temperature, alteration of moisture in air, etc. Therefore, it is essential to forecast the coffee productivity to go along with customer' expectations.
In this study, ARIMA and ANN were deployed to analyze and predict the crop yield of arabica coffee using data from 2004 to 2018. Both models have been demonstrated to be efficient in forecasting coffee production. The prediction performances of these models were evaluated using R2 and RMSE. The ARIMA model was optimized for (p, d, q) at (2, 1, 2). Its R2 and RMSE were 0.7041 and 0.1348, respectively. The ANN model employed the Levenberg-Marquardt algorithm with TrainLM and LearnGDM training functions, two hidden layers and one PEs for each hidden layer. Its performance regarding R2 and RMSE values of 0.9299 and 0.0642 was highly acceptable. Apparently, with respect to the R2 and RMSE, the ANN model was better than the ARIMA model.
Table 4 shows comparison between other works concerning different agricultural products. When comparing the R2 and RMSE between ANN and ARIMA, the ANN showed a better R2 than the ARIMA, and the RMSE of the ARIMA was higher than that for the ANN, like those in the forecasting of rainfall, predicting pod damage from pigeons [35,56,57,58,59,60,61]. While some of the agriculture predictions are favorable, the R2 of ARIMA is better than the ANN model, such as predicting soil salt and water content in crop rootzones and prediction for sugarcane production in Bihar, etc. [62].
Reference | Output | Period (yrs) | Predictors | R2 | RMSE | ||
ANN | ARIMA | ANN | ARIMA | ||||
[56] | Chickpea production | 5 | rainfall, minimum and maximum temperatures | 0.960 | 0.591 | 66.72 | 159.63 |
[59] | Wheat production | 58 | total annual precipitation, applied fertilizer, population and cultivated area | 0.930 | - | 0.39 | 1.46 |
[62] | Soil salt and water content | 5 | crop rootzone | 0.886 | 0.898 | - | - |
[57] | Behavioral pattern of rainfall | 93 | rainfall | 0.984 | 0.953 | 5.518 | 35.88 |
[61] | Sugarcane production | 81 | area, production, yield | - | - | 12.99 | 13.82 |
[58] | Crop planning | 32 | rainfall | 0.790 | 0.750 | 93.97 | 97.12 |
[35] | Pod damage of pigeon pea | 27 | relative humidity | 0.770 | 0.650 | 1.97 | 2.16 |
[60] | Agricultural and water resources | 100 | rainfall, temperature | - | - | 59.03 | 76.78 |
We aim to forecast the cherry coffee production of arabica coffee cultivated in northern Thailand. Two models in forecasting arabica coffee yields through ARIMA and ANN models were compared. The ARIMA model yielded a correlation coefficient (R2) of 0.704 and an RMSE of 0.1348. The ANN model produced a higher R2 of 0.9299 and a lower RMSE of 0.0642. In estimating yearly arabica coffee production, both models were determined to be adequate, but the ANN model appeared to perform better. However, when comparing the R2 and RMSE with others in literature, shown in Table 4, it was found that the ANN and ARIMA models gave the reasonable R2 and RMSE. They were suitable for coffee prediction.
With respect to the shortcomings of this work, they include missing data and the quality and quantity of data for coffee yield prediction. We considered merely six variable datasets; the area and productivity zone, rainfall, RH and temperature. The available amount of data remained low for these factors. Other factors that affect the coffee productivity, such as the amount of fertilizer, climate uncertainty each year, soil moisture, wind speed and amount of sunlight should also be considered, as they will help capture the full complexity of coffee yield. Moreover, flexible models that can capture the dynamic relationships between various factors affecting coffee yield may also be considered.
For future works, application of other ML algorithms such as decision tree, random forest, support vector machine, K-nearest neighbors, K-mean clustering, principal component analysis, naive Bayes etc. may be considered. Other techniques such as data augmentation from multiple sources, sensitivity analysis and sustainability analysis may be incorporated. Moreover, the coffee prediction model may be combined with assessing the feasibility of using remote sensing data, such as satellite imagery, to supplement the existing predictor variables and improve the forecasting models. Factors affected by climate change may also be considered.
The productivity of arabica coffee varies depending on the cultivated area, total rainfall, ambient temperature and RH, among other factors. They affect the yield of cherry coffee in each month. Accurate forecast oof the crop yield is crucial in response to customer needs. We used ANN and ARIMA models to predict the yield of arabica coffee using time-series data from 2004 to 2018. It was shown that both models could forecast coffee production satisfactorily. Within the dataset considered, the ANN (R2 and RMSE of 0.9299 and 0.0642) appeared to perform better than the ARIMA (R2 and RMSE of 0.7041 and 0.1348) model.
The authors declare that they have not used Artificial Intelligence (AI) tools in the creation of this article.
This work was partially supported by Chiang Mai University. One of the authors (Y.K.) wishes to acknowledge the CMU Graduate School for Research Assistant grant. We also wish to thank the Supply Chain and Engineering Management Research Unit (SCEM), Chiang Mai University for providing research facilities. This research is part of the project "A Strategic Roadmap Toward the Next Level of Intelligent, Sustainable and Human-Centered SME: SME 5.0" from the European Union's Horizon 2021 research and innovation program under the Marie Skłodowska-Curie Grant agreement No. 101086487.
Conceptualization, K.Y.T. and N.T.; Methodology, Y.K. and N.T.; Data curation, Y.K.; Formal analysis, Y.K., A.B. and E.R.; Investigation, Y.K. and A.B.; Writing—original draft preparation, Y.K.; Writing—review and editing, N.T. and E. R.; Supervision, K.Y.T.; Funding acquisition, K.Y.T.
The data from the Climate Department included rainfall, RH and minimum and maximum temperature. The Agricultural Economics Office and Meteorological Department provide the area and productivity zone.
All authors declare no conflicts of interest.
[1] | McMichael P (2019) A food regime genealogy. J Peasant Stud 36: 139–169. |
[2] | European Commission (2018) Proposal for a Directive of the European Parliament and of the Council on unfair trading practices in business-to-business relationships in the food supply chain. |
[3] |
Swinnen JFM, Vandeplas A (2010) Market power and rents in global supply chains. Agric Econ 41: 109–120. doi: 10.1111/j.1574-0862.2010.00493.x
![]() |
[4] |
Podszun R (2016) The arbitrariness of market definition and an evolutionary concept of markets. The Antitrust Bull 61: 121–132. doi: 10.1177/0003603X15625109
![]() |
[5] | Cabral LMB (2000) Introduction to Industrial Organization. Cambridge, Massachusetts, London: The MIT Press. |
[6] |
Gereffi G, Humphrey J, Sturgeon T (2005) The governance of global value chains. Rev Int Political Econ 12: 78–104. doi: 10.1080/09692290500049805
![]() |
[7] | Cox A, Sanderson J, Watson G (2001) Supply chains and power regimes: Toward an analytic framework for managing extended networks of buyer and supplier relationships. J Supply Chain Manage 37: 28–35. |
[8] |
Slade ME (2004) Competing models of firm profitability. Int J Ind Organ 22: 289–308. doi: 10.1016/j.ijindorg.2003.12.001
![]() |
[9] | Ferguson PR, Ferguson GJ (1994) Industrial Economics: Issues and Perspectives. 2 Eds., London: The Macmillan Press lTD, 309. |
[10] |
Perloff JM, Shen EZ (2012) Collinearity in linear structural models of market power. Rev Ind Organ 40: 131–138. doi: 10.1007/s11151-012-9336-x
![]() |
[11] |
Martin S (2012) Market structure and market performance. Rev Ind Organ 40: 87–108. doi: 10.1007/s11151-012-9338-8
![]() |
[12] | Sterman J (2000) Business Dynamics: Systems Thinking and Modeling for a Complex World. USA: McGraw-Hill Education. |
[13] | Grether ET (1970) Industrial organization: Past history and future problems. Am Econ Rev 60: 83–89. |
[14] | FAOSTAT (2017) Crop statistics. |
[15] | Overheidsdiensten BF (2017) Tab A landbouwcijfers 2016-Resultaten volgens uitgebreide lijst van variabelen: voor België, de Gewesten, de Provincies, de Landbouwstreken, FOD Economie. |
[16] | CBB (2017) Activiteitenrapport 20-16-2017. CBB-Confederatie van de Belgische Bietenplanters. |
[17] | VILT (2018) Verkoopprijs van suiker bereikt nieuw dieptepunt in EU, VILT. |
[18] | Mason ES (1939) Price and production policies of large-scale enterprise. Am Econ Rev 29: 61. |
[19] | Fałkowski J, Menard C, Sexton RJ, et al. (2017) Unfair trading practices in the food supply chain: A literature review on methodologies, impacts and regulatory aspects. In: Marcantonio FD, Ciaian P, JRC Technical Report. |
[20] |
Carter N, Bryant-Lukosius D, Dicenso A, et al. (2014) The use of triangulation in qualitative research. Oncol Nurs Forum 41: 545–547. doi: 10.1188/14.ONF.545-547
![]() |
[21] | Strauss A, Corbin J (1998) Basics of Qualitative Reserach: Techniques and Procedures for Developing Frounded Theory. 2Eds., Thousand Oaks, London, New Delhi: Sage Publications. |
[22] |
Spicer DP (1998) Linking mental models and cognitive maps as an aid to organisational learning. Career Dev Int 3: 125–132. doi: 10.1108/13620439810211126
![]() |
[23] |
Clemens RA, Jones JM, Kern M, et al. (2016) Functionality of sugars in foods and health. Compr Rev Food Sci Food Saf 15: 433–470. doi: 10.1111/1541-4337.12194
![]() |
[24] |
Pouch T, Trouvé A (2018) Deregulation and the crisis of dairy markets in Europe: facts for economic interpretation. Stud Political Econ 99: 194–212. doi: 10.1080/07078552.2018.1492216
![]() |
[25] | Sexton RJ, Zhang M, Chalfant JA (2010) Grocery Retailer Behavior in Perishable Fresh Produce Procurement. J Agric Food Ind Organ 3. |
[26] | Solomon S (2000) Post-Harvest cane deterioration and its milling consequences. Sugar Tech 2: 1. |
[27] |
Higgins A, Thorburn P, Archer A, et al. (2007) Opportunities for value chain research in sugar industries. Agric Syst 94: 611–621. doi: 10.1016/j.agsy.2007.02.011
![]() |
[28] |
Kahneman D (2003) Maps of bounded rationality: Psychology for behavioral economics. Am Econ Rev 93: 1449–1475. doi: 10.1257/000282803322655392
![]() |
[29] |
Malawska A, Topping CJ (2016) Evaluating the role of behavioral factors and practical constraints in the performance of an agent-based model of farmer decision making. Agric Syst 143: 136–146. doi: 10.1016/j.agsy.2015.12.014
![]() |
[30] | ABW (2018) Sucrerie de Seneffe: La coopérative des planteurs. Available from: http://abwserres.be. |
[31] | Lee C (2007) SCP, NEIO and Beyond, in Working Paper Series, 2007-05, U.o. Nottingham. |
No. | Parameter | AIC |
1 | (2, 1, 2) | −168.0802 |
2 | (2, 1, 3) | −166.9035 |
3 | (2, 0, 3) | −166.7370 |
4 | (3, 1, 2) | −166.4853 |
5 | (3, 0, 2) | −165.7990 |
Training and Testing | Time-based cross-validation | ||||||||
MSE Train |
MSE Test |
RMSE Train |
RMSE Test |
R2 Train |
R2 Test |
R2 Train | R2 Test |
MSE Train |
MSE Test |
0.0181 | 0.0469 | 0.1348 | 0.2167 | 0.7041 | 0.3521 | 0.7383 | 0.3931 | 0.0046 | 0.0451 |
Number of hidden layers | PEs | MSE | R Training |
R Testing |
R Validation |
R Overall |
R2 |
1 | 1 | 20791 | 0.9014 | 0.5100 | 0.9196 | 0.8491 | 0.7210 |
1 | 2 | 27138 | 0.7977 | 0.7603 | 0.5115 | 0.7764 | 0.6028 |
1 | 3 | 40226 | 0.8079 | 0.8405 | 0.7176 | 0.7965 | 0.6344 |
1 | 4 | 42773 | 0.7506 | 0.3192 | 0.7525 | 0.7096 | 0.5035 |
1 | 5 | 50880 | 0.8474 | 0.7186 | 0.4740 | 0.7795 | 0.6076 |
1 | 6 | 39929 | 0.8580 | 0.6132 | 0.7423 | 0.7794 | 0.6075 |
1 | 7 | 72995 | 0.9761 | 0.6014 | 0.4396 | 0.8464 | 0.7164 |
1 | 8 | 20092 | 0.7720 | 0.6825 | 0.6804 | 0.7431 | 0.5522 |
1 | 9 | 20419 | 0.9798 | 0.8299 | 0.8797 | 0.9291 | 0.8632 |
1 | 10 | 15591 | 0.9810 | 0.5857 | 0.9452 | 0.9000 | 0.8100 |
2 | 1 | 19576 | 0.9921 | 0.9384 | 0.8723 | 0.9643 | 0.9299 |
2 | 2 | 20384 | 0.7900 | 0.7736 | 0.6675 | 0.7807 | 0.6095 |
2 | 3 | 14133 | 0.7776 | 0.8219 | 0.7922 | 0.7747 | 0.6002 |
2 | 4 | 27636 | 0.9143 | 0.5913 | 0.8700 | 0.8816 | 0.7772 |
2 | 5 | 28923 | 0.9297 | 0.7595 | 0.9057 | 0.9085 | 0.8254 |
2 | 6 | 16209 | 0.9131 | 0.7751 | 0.7987 | 0.8690 | 0.7552 |
2 | 7 | 37072 | 0.7236 | 0.6636 | 0.6070 | 0.7042 | 0.4959 |
2 | 8 | 16657 | 0.9825 | 0.9049 | 0.8930 | 0.9629 | 0.9272 |
2 | 9 | 7218 | 0.8151 | 0.6677 | 0.8071 | 0.8039 | 0.6463 |
2 | 10 | 16288 | 0.9761 | 0.6510 | 0.8018 | 0.9164 | 0.8398 |
Reference | Output | Period (yrs) | Predictors | R2 | RMSE | ||
ANN | ARIMA | ANN | ARIMA | ||||
[56] | Chickpea production | 5 | rainfall, minimum and maximum temperatures | 0.960 | 0.591 | 66.72 | 159.63 |
[59] | Wheat production | 58 | total annual precipitation, applied fertilizer, population and cultivated area | 0.930 | - | 0.39 | 1.46 |
[62] | Soil salt and water content | 5 | crop rootzone | 0.886 | 0.898 | - | - |
[57] | Behavioral pattern of rainfall | 93 | rainfall | 0.984 | 0.953 | 5.518 | 35.88 |
[61] | Sugarcane production | 81 | area, production, yield | - | - | 12.99 | 13.82 |
[58] | Crop planning | 32 | rainfall | 0.790 | 0.750 | 93.97 | 97.12 |
[35] | Pod damage of pigeon pea | 27 | relative humidity | 0.770 | 0.650 | 1.97 | 2.16 |
[60] | Agricultural and water resources | 100 | rainfall, temperature | - | - | 59.03 | 76.78 |
No. | Parameter | AIC |
1 | (2, 1, 2) | −168.0802 |
2 | (2, 1, 3) | −166.9035 |
3 | (2, 0, 3) | −166.7370 |
4 | (3, 1, 2) | −166.4853 |
5 | (3, 0, 2) | −165.7990 |
Training and Testing | Time-based cross-validation | ||||||||
MSE Train |
MSE Test |
RMSE Train |
RMSE Test |
R2 Train |
R2 Test |
R2 Train | R2 Test |
MSE Train |
MSE Test |
0.0181 | 0.0469 | 0.1348 | 0.2167 | 0.7041 | 0.3521 | 0.7383 | 0.3931 | 0.0046 | 0.0451 |
Number of hidden layers | PEs | MSE | R Training |
R Testing |
R Validation |
R Overall |
R2 |
1 | 1 | 20791 | 0.9014 | 0.5100 | 0.9196 | 0.8491 | 0.7210 |
1 | 2 | 27138 | 0.7977 | 0.7603 | 0.5115 | 0.7764 | 0.6028 |
1 | 3 | 40226 | 0.8079 | 0.8405 | 0.7176 | 0.7965 | 0.6344 |
1 | 4 | 42773 | 0.7506 | 0.3192 | 0.7525 | 0.7096 | 0.5035 |
1 | 5 | 50880 | 0.8474 | 0.7186 | 0.4740 | 0.7795 | 0.6076 |
1 | 6 | 39929 | 0.8580 | 0.6132 | 0.7423 | 0.7794 | 0.6075 |
1 | 7 | 72995 | 0.9761 | 0.6014 | 0.4396 | 0.8464 | 0.7164 |
1 | 8 | 20092 | 0.7720 | 0.6825 | 0.6804 | 0.7431 | 0.5522 |
1 | 9 | 20419 | 0.9798 | 0.8299 | 0.8797 | 0.9291 | 0.8632 |
1 | 10 | 15591 | 0.9810 | 0.5857 | 0.9452 | 0.9000 | 0.8100 |
2 | 1 | 19576 | 0.9921 | 0.9384 | 0.8723 | 0.9643 | 0.9299 |
2 | 2 | 20384 | 0.7900 | 0.7736 | 0.6675 | 0.7807 | 0.6095 |
2 | 3 | 14133 | 0.7776 | 0.8219 | 0.7922 | 0.7747 | 0.6002 |
2 | 4 | 27636 | 0.9143 | 0.5913 | 0.8700 | 0.8816 | 0.7772 |
2 | 5 | 28923 | 0.9297 | 0.7595 | 0.9057 | 0.9085 | 0.8254 |
2 | 6 | 16209 | 0.9131 | 0.7751 | 0.7987 | 0.8690 | 0.7552 |
2 | 7 | 37072 | 0.7236 | 0.6636 | 0.6070 | 0.7042 | 0.4959 |
2 | 8 | 16657 | 0.9825 | 0.9049 | 0.8930 | 0.9629 | 0.9272 |
2 | 9 | 7218 | 0.8151 | 0.6677 | 0.8071 | 0.8039 | 0.6463 |
2 | 10 | 16288 | 0.9761 | 0.6510 | 0.8018 | 0.9164 | 0.8398 |
Reference | Output | Period (yrs) | Predictors | R2 | RMSE | ||
ANN | ARIMA | ANN | ARIMA | ||||
[56] | Chickpea production | 5 | rainfall, minimum and maximum temperatures | 0.960 | 0.591 | 66.72 | 159.63 |
[59] | Wheat production | 58 | total annual precipitation, applied fertilizer, population and cultivated area | 0.930 | - | 0.39 | 1.46 |
[62] | Soil salt and water content | 5 | crop rootzone | 0.886 | 0.898 | - | - |
[57] | Behavioral pattern of rainfall | 93 | rainfall | 0.984 | 0.953 | 5.518 | 35.88 |
[61] | Sugarcane production | 81 | area, production, yield | - | - | 12.99 | 13.82 |
[58] | Crop planning | 32 | rainfall | 0.790 | 0.750 | 93.97 | 97.12 |
[35] | Pod damage of pigeon pea | 27 | relative humidity | 0.770 | 0.650 | 1.97 | 2.16 |
[60] | Agricultural and water resources | 100 | rainfall, temperature | - | - | 59.03 | 76.78 |