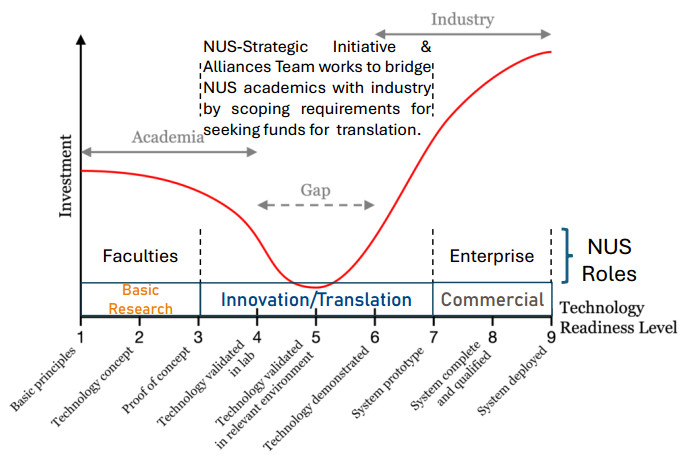
Campus as a Living Lab at the National University of Singapore is an initiative to bridge technologies from academia to industry across the valley of death [
Citation: Thomas Chee Tat Ho, George Chee Ping Loh. Translating research through the National University of Singapore campus as a living laboratory[J]. Applied Computing and Intelligence, 2025, 5(1): 82-93. doi: 10.3934/aci.2025006
[1] | Noah Gardner, Hafiz Khan, Chih-Cheng Hung . Definition modeling: literature review and dataset analysis. Applied Computing and Intelligence, 2022, 2(1): 83-98. doi: 10.3934/aci.2022005 |
[2] | Mohamed Wiem Mkaouer, Tarek Gaber, and Zaineb Chelly Dagdia . Effects of COVID-19 pandemic on computational intelligence and cybersecurity: Survey. Applied Computing and Intelligence, 2022, 2(2): 173-194. doi: 10.3934/aci.2022010 |
[3] | Xu Ji, Fang Dong, Zhaowu Huang, Xiaolin Guo, Haopeng Zhu, Baijun Chen, Jun Shen . Edge-assisted multi-user millimeter-wave radar for non-contact blood pressure monitoring. Applied Computing and Intelligence, 2025, 5(1): 57-76. doi: 10.3934/aci.2025004 |
[4] | Nima Khodadadi, El-Sayed M. El-Kenawy, Francisco De Caso, Amal H. Alharbi, Doaa Sami Khafaga, Antonio Nanni . The Mountain Gazelle Optimizer for truss structures optimization. Applied Computing and Intelligence, 2023, 3(2): 116-144. doi: 10.3934/aci.2023007 |
[5] | Yongcan Huang, Jidong J. Yang . Semi-supervised multiscale dual-encoding method for faulty traffic data detection. Applied Computing and Intelligence, 2022, 2(2): 99-114. doi: 10.3934/aci.2022006 |
[6] | Elvis Twumasi, Ebenezer Archer, Emmanuel O. Addo, Emmanuel A. Frimpong . Modification of coot optimization algorithm (COA) with adaptive sigmoid increasing inertia weight for global optimization. Applied Computing and Intelligence, 2024, 4(1): 93-106. doi: 10.3934/aci.2024006 |
[7] | Abigail Wiafe, Pasi Fränti . Affective algorithmic composition of music: A systematic review. Applied Computing and Intelligence, 2023, 3(1): 27-43. doi: 10.3934/aci.2023003 |
[8] | Alessio Bechini, Alessandro Bondielli, Pietro Dell'Oglio, Francesco Marcelloni . From basic approaches to novel challenges and applications in Sequential Pattern Mining. Applied Computing and Intelligence, 2023, 3(1): 44-78. doi: 10.3934/aci.2023004 |
[9] | Abrhalei Tela, Abraham Woubie, Ville Hautamäki . Transferring monolingual model to low-resource language: the case of Tigrinya. Applied Computing and Intelligence, 2024, 4(2): 184-194. doi: 10.3934/aci.2024011 |
[10] | Xian Wen Sim, Sie Long Kek, Sy Yi Sim . State estimation and optimal control of an inverted pendulum on a cart system with stochastic approximation approach. Applied Computing and Intelligence, 2023, 3(1): 79-92. doi: 10.3934/aci.2023005 |
Campus as a Living Lab at the National University of Singapore is an initiative to bridge technologies from academia to industry across the valley of death [
The Singapore government increased its research and development (R & D) spending to retain its economic competitiveness on a worldwide scale[2,3,4]. Having an economy that can continuously attract foreign investors to Singapore is crucial[5]. R & D is seen as a key driving force for creating new industries and generating new avenues of growth. Through R & D investments and spearheaded by innovation, Singapore aims to become a knowledge-based economic identity. This allows Singapore to produce high-value products and services to attract foreign investments for its benefit and growth[6].
This was challenging for Singapore due to COVID-19 severely affecting the global economy. It shrank Singapore's economy by 4.1% in 2020, making it one of the worst full-year recessions since its independence[7]. Local e-commerce platforms such as Shopee, Lazada, and Grab, recognized innovative digital technologies as the key to their survival during the Singapore lockdown years of COVID-19. However, due to the difficulties of working with reduced finance and resources, such companies became prudent in their R & D investments. This meant that they sought new technologies that were nearer to the market for rapid deployment.
According to the technology readiness level (TRL) chart in Figure 1, fundamental research is at TRL 1–4. Companies, on the other TRL spectrum, are only interested in investing in technologies nearer to the market at TRL 6–9. Hence, innovations in the translation or applied research stage of TRL 4–6 were neglected. This meant that there were no resources to support TRL 4–6. This is coined as the valley of death for innovative technologies as shown in Figure 1.
The National University of Singapore (NUS) was keen to help its academics and local companies already having research agreements to bridge this valley of death. Currently, TRL 1–4 are extensively supported by Singapore national R & D investments[8] through universities, ensuring continuous research activities and supply of innovations.TRL 6–9, rarely 6 in the Singapore context, are funded by companies. They must possess the financial means and expertise to translate academic technologies.
It can be seen that TRL 4–6 mostly entails technology translation. This meant demonstrating that the translated technologies can scale and sustain when released into the real-world environment. The university with its population and campus can be regarded as a microcosm of Singapore[9]. For example, the university has its own micro-grid and water distribution system. A company wanting to test its solar panels and their impacts on an actual live electrical grid can be easily deployed in NUS without affecting the nation's grid. Likewise, companies testing the impacts of different coolants in a centralized district cooling system on the thermal comfort of NUS residents is also possible. During testing, our researchers can be invited by the companies to further improve their products. The university would also get an insight into the technologies' suitability for campus deployment. The university can assist in reducing the costs of technology translation in TRL 4–6. This can be achieved by helping to find resources and also using NUS grounds as part of the Campus as a living lab initiative.
The Campus as a living lab at NUS (CALL@NUS) initiative was officially announced by NUS' president, Professor Tan Eng Chye. It is part of NUS' research capabilities that provide R & D and translation opportunities while fostering the environment for academics to learn and industry collaborators to innovate cutting-edge technologies and up-skill their workforce. In recent years, there have been developments to build digital twins of the world[10,11,12]. They are simulated representations of any real-world physical models and systems. They are used for modeling, simulating, and testing real-world actions and processes instead of their physical counterparts. This is because the actual real-world actions are dangerous, expensive, or both.
Living labs, emergent from the interactions between user experiences and an environment's background intelligence, are eco-systems where new technologies are tested and feedback is collected iteratively until they reach a steady state where they make a difference to the real world. The main cyclical processes of living labs are innovation, prototype development, and testing for proof-of-concept followed by proof-of-value, feedback, and tuning. The living lab is often the final step before products, frameworks, and processes are released into the real world. The NUS campus grounds are living lab materials for products and processes[13,14]. By integrating NUS' physical systems and infrastructure with NUS' digital twin (DT) platform, physical and virtual platforms can be leveraged to serve as a testbed for innovations beyond traditional boundaries.
This initiative was developed to bridge the valley of death. However, not all technologies are feasible. According to Figure 2, the entry into CALL@NUS can begin either from the industry, NUS, or both. The university must have the physical systems and infrastructures for testbedding. It is then followed by feasibility studies on DT. It is a digitized manifestation of NUS' physical systems, infrastructures, and environment with precise real-world geo-spatial coordinates. To complete the initiative, NUS' strategic initiative & alliances' (NUS-SIA) team develops realistic business use cases for NUS and its industry partners. A spill-over effect of this was that it enabled NUS to become an augmented learning environment where students can practice their acquired skills in real-world applications.
DT is a manifestation of its physical infrastructure, environment, and domain in digital space. Its creation is driven by three motivational concepts (i.e., virtualize, visualize and venturize [15]) shown in Figure 3.
The first concept, virtualize, is converting the real-world physical NUS into its digital form. This was achieved through a professional point-cloud scan using light detection and ranging (Lidar). This step is not trivial and the process of turning point-clouds into polygon meshes is explained below.
The second concept, visualize, is the rendition of DT in three-dimensional digital space. The point-clouds are actual points in space where the light is reflected into the Lidar scanner. Hence, the points are not annotated with the reflected surface. Currently, there are software platforms[16] that can extract certain features with artificial intelligence. To allow for better extraction, these platforms allow human annotation to augment the extraction process as shown in Figure 4.
Once the point-clouds are grouped into their respective sets, such as infrastructure, vegetation, and ground, they are converted into polygon meshes by joining the cloud points and extracting the bounding shape of the polygon meshes. These shapes are representative of NUS and its surroundings. The shapes are placed on a digital terrain model with a reference coordinate anchor set to the southwest corner of the campus. The purpose is to reduce digital spatial floating point x, y, and z axis coordinates for faster visualization. Anomalies and point-of-interests are distinguished for easier visualization. Objects of interest can also be selected to reduce compute overheads for DT visualization.
The third concept, venturize is to make a feasible business case through DT simulation before real-world implementation. This concept is to implement the business case in the digital twin and simulates its profitability for analysis. These simulations can be on traffic[17], balancing campus electricity demand[18], and many others. This concept covers optimizing variables under constraints. The end results of interest are discovered through the iterative feedback between the variables, constraints, and environment. Using the three concepts shown in Figure 3, we can simulate various conceptualized business cases virtually in the DT before their real-world deployments. The DT can simulate their respective economic aspects providing insights to make better-informed decisions. All these eventually lead to a more sustainable real-world deployment through using the DT.
Good provable business cases invite companies to test their products using the campus. The NUS-SIA team considered each living lab proposal based on the capital expenditures (CAPEX), operational expenditures (OPEX), and likely generalized return of investments (ROI)[19,20,21]. The CAPEX refers to expenses that were required to set up the infrastructure in both physical NUS and DT. The OPEX was the daily cost required to keep the system running. The ROI is either profits from revenue generated or savings in costs vis-a-vis without the technology. In addition, the university must have the space to house the project and equipment. Their relationships can be modeled as Eq (1).
R=E−n∑i=1(Ci+Oi),whereR=ReturnofInvestments,E=Earnings,C=CAPEX,O=OPEX,i=ithCAPEXanditsassociatedOPEX. | (1) |
The valley of death is bridged when ROI is positive, meaning that there is a viable business case. As shown in Eq (2), the university assists in making the business case viable by contributing in-kind resources. If existing NUS infrastructures, systems, and equipment are usable, it contributes to lowering CAPEX.
R=E−min‖n∑i=1(Ci+Oi)‖wherei=ithCAPEXanditsassociatedOPEX. | (2) |
Shown in Figure 5 is a generalized process of how the university decides on the feasibility of a business case for NUS. There is a main process flow depicted by the orange boxes, which are gates to the next stage. The NUS-SIA team examines peripheral questions in the blue boxes to ensure that the main gates can be successfully opened.
In the next section, two case studies with different outcomes will explain how Eq (2) was used to determine the feasibility and decision to advance the living lab proposals.
In this section, we describe two of NUS' initial use cases below. Using Eq (2) in Section 2, we demonstrate how the first use case was not able to proceed beyond simulation in the digital twin while the second use case was able to progress into the real-world environment.
One of the CALL@NUS projects is to provide sustainable and competitive solutions across the energy chain. This was validated using a smart AC/DC micro-grid in the NUS campus, shown in Figure 6.
If the solution is developed and validated, it would be a profitable model for energy retailing model. To achieve this, an innovative grid that can easily integrate renewable and distributed energy resources with greater flexibility and efficiency is needed. However, the trials were at risk of disrupting the national electric grid if tested in a live environment. The NUS-SIA team suggested conducting the trials within NUS' controlled campus grid.
As it was surmised that this would be a heavy investment into equipment, outfitting works, and other required services, there is a need to know if there would be viable ROI if the project goes into operation. We used Eq (2) to determine if the project would be feasible for real-world implementation. The CAPEX costs include items, such as new solar panels; their installation; and a 0.5 megawatt-hour (MWh) battery. Even though NUS does have solar panels, they are old and operating at sub-optimal efficiency. Their solar cells can be retrograded to improve their irradiance absorption. However, it will not be good compared to brand-new solar panels. Moreover, retrograding costs are not cheaper. Hence, a new solar panel installation was selected. A 0.5 MWh was included for more flexibility in energy acquisition like buying from the grid at low prices or storing surplus energy when solar generation is strong. To find the most effective placement of solar panels for maximum energy production, we had to virtualize the solar panels and their probable roof placements.
As shown in Figure 7, the electrical distribution grid is connected to the solar panels and various energy loads such as lights and air-conditioning systems. The solar irradiance can be simulated over the placed solar panels, taking into account building shadows and obstructions, to estimate the generated energy. These are visualized on the DT user interface.
Once the optimal solar panel placement is found, test loads, OPEX costs like maintenance, operations and CAPEX are estimated, the third concept of venturise can be applied. Using Singapore irradiance data and energy market data, simulations were conducted by NUS Professor Sanjib k. Panda and his team on DT to estimate the daily earnings, E, in Eq (2) for the smart hybrid AC/DC grid. When the collaborators were presented with the simulation results, they commented that the ROI was longer than expected.
The project did not proceed under the evaluation criteria because retrofitting the current grid to support DC requires high CAPEX. In addition, as innovation cycles shorten, better and cheaper technologies may become available, so the long wait for ROI based on the current innovations might not be a worthy investment.
A multi-national information technology company in Singapore was developing innovative physical and digital security with NUS' School of Computing professors. They have a joint laboratory on NUS. The NUS-SIA team helped to pitch a series of security technologies to the NUS Information Technology (NUSIT) department. Out of the series, the NUSIT department was excited to try this anti-phishing software. The developed security program identifies emerging phishing websites and pages without the assistance of iterating through a list of compromised uniform resource locators. Using meta-information and image recognition techniques as inputs combined with artificial intelligence on the landing page of the suspected phishing website address, the lab was able to determine its authenticity. If not authentic, the email will be filtered before it reaches anyone.
This is a pure venturize project. There isn't a need to virtualize or visualize the email system as we are not tracking the processes. Equation (2) was used to determine its feasibility for deployment on the NUS email system. The variables are substituted as shown in Eq (3). However, the Eq (3) for this case, the ROI is only applicable when the email system is compromised.
R=E−n∑i=1(Ci+Oi),whereR=ReturnofInvestments,E=Costofavoidingsystemcompromised,C=Costofprogrammingintoexistingphishingdetection,O=Costofrepairingacompromisedsystem,i=ithCanditsassociatedO. | (3) |
Given that currently, cyber-physical of critical systems are a major concern worldwide, a breach will cost an organization, the university included, very significantly[22,23]. The business case for NUSIT is very straightforward as the cost of avoiding a system compromised due to a cyber-physical breach far surpassed the cost of implementing the additional anti-phishing feature. The last step is to try it on the campus as a living lab initiative for its efficacy. The improvement was found to be 0.5%. As mentioned previously about the cost of a compromised system[22,23], there is no improvement too small. After the initial trial on the living lab, the NUSIT department has adopted the solution as part of the suite of email cybersecurity solutions. The award agreement between NUSIT and the company will commence in January 2025.
From both case studies, the same framework was applied to evaluate both proposals. To better understand the framework application, we have shown a case that was rejected and a case that was approved for implementation. The first case study was a partial of the living lab initiative with the simulations running in DT. For the second case study, DT was absent as the plug-in was used directly on the current system. Nonetheless, the chance to advance the processes according to Figure 2 was very encouraging. This has proved that the framework was applicable.
Singapore is a land-scare country. The university is not likely to expand larger horizontally beyond its current boundaries. Hence, the use of space for test-bedding technologies comes under heavy scrutiny in different NUS offices and departments. In this paper, we have examined how to use available campus real estate to conduct living-lab trials. We have devised a stochastic framework to analyze its cost and benefits feasibility before its real-world implementation. We have demonstrated success in deciding the correct living laboratory project for implementation. Moving forward, we would like to refine this framework to deploy beyond NUS campus grounds. We would also like to test a case where we can complete the cycle as shown in Figure 2.
The case studies mentioned here are the first two cases from the NUS Campus as a Living Lab initiative. Currently, DT is not able to support all probable business cases. The NUS-SIA team is working to make DT that can better support NUS research and its translation. One of the immediately forthcoming works that DT supports is to plant the appropriate campus greenery for sustainability. We would also like to explore moving the initiative involving DT to the hospital services and hospitality industry. We are currently in discussions and proposal preparation on this. Hopefully, with more adoption of projects this initiative, we will accumulate sufficient quantitative data that allows us to do a good comparison between traditional methods and using digital twin simulations to conduct a cost and benefit analysis.
The authors declare they have not used Artificial Intelligence (AI) tools in the creation of this article.
We would like to thank the CoolNUS project for its support in contributing to our digital twin. We would also like to thank various offices and faculties for their knowledge that enriches the twin. We would also like to thank NUS Professor Sanjib K. Panda and his team for their valuable contributions to evaluating the feasibility of the smart AC/DC hybrid grid.
The authors declare no conflict of interest.
[1] | S. Nwaka, Technology readiness levels, the valley of death and scaling up innovations, In: Social and technological innovation in Africa, Singapore: Palgrave Macmillan, 2021, 99–107. https://doi.org/10.1007/978-981-16-0155-2_7 |
[2] | The Business Times, Singapore spending Sfanxiexian_myfh25b in next five-year R and D plan, SPH Media, 2020. Available from: https://www.businesstimes.com.sg/singapore/economy-policy/singapore-spending-s25b-next-five-year-rd-plan. |
[3] | National Research Foundation Singapore, Technology and Research, National Survey of Research, Innovation and Enterprise in Singapore 2021, Agency for Science, Technology and Research (A*STAR), 2024. Available from: https://www.a-star.edu.sg/docs/librariesprovider1/default-document-library/rie/2021-rie-survey-publication_finalforpublication_amended.pdf. |
[4] | M. Teo, A. Loo, K. Leong, Economic Survey of Singapore: Third Quarter 2019, Ministry of Trade and Industry Republic of Singapore, 2019. Available from: https://www.mti.gov.sg/-/media/MTI/Resources/Economic-Survey-of-Singapore/2019/Economic-Survey-of-Singapore-Third-Quarter-2019/FullReport_3Q19.pdf. |
[5] | P. Wong, Y. Ho, A. Singh, The impact of R & D on the Singaporean economy over 1978–2019, Singap. Econ. Rev., in press. https://doi.org/10.1142/S0217590823500480 |
[6] | P. Wong, The development of Singapore's innovation and entrepreneurship ecosystem, In: Clusters of innovation in the age of disruption, Cheltenham: Edward Elgar Publishing, 2022,206–244. https://doi.org/10.4337/9781800885165.00019 |
[7] | Ministry of Finance, Economy and Labour Market: Sustaining Singapore's global relevance and competitive edge, Government of Singapore, 2024. Available from: https://spor.performancereports.gov.sg/businesses/strong-and-resilient-economy/economy-and-labour-market. |
[8] |
T. Tat, G. Ping, Innovating services and digital economy in Singapore, Commun. ACM, 63 (2020), 58–59. https://doi.org/10.1145/3378554 doi: 10.1145/3378554
![]() |
[9] | The University Campus Infrastructure (UCI) cluster of offices, Smart Safe Sustainable, National University of Singapore, 2019. Available from: https://uci.nus.edu.sg/wp-content/uploads/2024/03/Annual-report-2019-FINAL-BLUE.pdf. |
[10] | M. Batty, Digital twins, Environ. Plan. B-Urban, 45 (2018), 817–820. https://doi.org/10.1177/2399808318796416 |
[11] |
Y. Jiang, S. Yin, K. Li, H. Luo, O. Kaynak, Industrial applications of digital twins, Phil. Trans. R. Soc. A, 379 (2021), 20200360. https://doi.org/10.1098/rsta.2020.0360 doi: 10.1098/rsta.2020.0360
![]() |
[12] |
M. Juarez, V. Botti, A. Giret, Digital twins: review and challenges, J. Comput. Inf. Sci. Eng., 21 (2021), 030802. https://doi.org/10.1115/1.4050244 doi: 10.1115/1.4050244
![]() |
[13] | A Følstad, Living labs for innovation and development of information and communication technology: a literature review, Electronic Journal of Organizational Virtualness, 10 (2008), 99–131. |
[14] |
M. Hossain, S. Leminen, M. Westerlund, A systematic review of living lab literature, J. Clean. Prod., 213 (2019), 976–988, https://doi.org/10.1016/j.jclepro.2018.12.257 doi: 10.1016/j.jclepro.2018.12.257
![]() |
[15] | P. Tay, N. Wang, TCI 2015 Innovation Clusters–-a National Strategy to Build Technology Capabilities in Singapore, SlideShare from Scribd, 2015. Available from: https://www.slideshare.net/slideshow/tci-2015-innovation-clusters-a-national-strategy-to-build-technology-capabilities-in-singapore/54947121. |
[16] | R. Takken, One generic viewer for all current data types, GeoInformatics, 17 (2014), 44. |
[17] | T. Ho, R. Yu, J. Lim, P. Fränti, Modelling implications & impacts of going green with EV in Singapore with multi-agent systems, Proceedings of Signal and Information Processing Association Annual Summit and Conference (APSIPA), 2014 Asia-Pacific, 2014, 1–6. https://doi.org/10.1109/APSIPA.2014.7041769 |
[18] | W. Yang, T. Ho, L. Xiang, C. Chai, R. Yu, An overview and evaluation on demand response program in Singapore electricity market, Proceedings of IEEE Conference on Energy Conversion (CENCON), 2014, 61–66. https://doi.org/10.1109/CENCON.2014.6967477 |
[19] |
H. Altalib, ROI calculations for electronic performance support systems, Perform. Improv., 41 (2002), 12–22. https://doi.org/10.1002/pfi.4140411005 doi: 10.1002/pfi.4140411005
![]() |
[20] |
B. Boehm, R. Valerdi, E. Honour, The ROI of systems engineering: some quantitative results for software‐intensive systems, Systems Eng., 11 (2008), 221–234. https://doi.org/10.1002/sys.20096 doi: 10.1002/sys.20096
![]() |
[21] |
G. Bockle, P. Clements, J. McGregor, D. Muthig, K. Schmid, Calculating ROI for software product lines, IEEE Software, 21 (2004), 23–31. https://doi.org/10.1109/MS.2004.1293069 doi: 10.1109/MS.2004.1293069
![]() |
[22] |
M. Tahmasebi, Cyberattack ramifications, the hidden cost of a security breach, Journal of Information Security, 15 (2024), 87–105. https://doi.org/10.4236/jis.2024.152007 doi: 10.4236/jis.2024.152007
![]() |
[23] | IBM and Ponemon Institute, Cost of a Data Breach: Report 2024, IBM, 2024. Available from: https://www.ibm.com/reports/data-breach. |