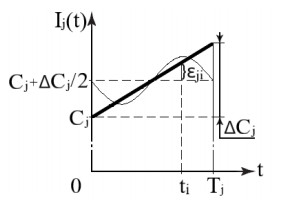
In this paper, I demonstrate the adequacy of economic systems to the basic provisions of synergetics, which makes the latter eligible for macroeconomic analysis. In justifying this statement, a synergetic approach to the development of a model of economic cycles was considered. The novelty of this model was related to the probabilistic description of the investment function and the perception of the economic system as a material object with certain properties. According to the model, the income oscillations are induced by both exogenous (investment fluctuations) and endogenous (system elasticity) causes. The cycle's amplitudes correlate with the intensity of investment fluctuations as well as the efficiency of the economic system. The duration of the economic cycle is determined by the inclusive wealth of the system and its dynamic factor, which characterizes the ability of the system to withstand investment fluctuations and eliminates their consequences. Thus, the economic cycle is interpreted as the "natural noise" accompanying the functioning of market economics.
The proposed model creates a mathematical basis for the numerical analysis of empirical economic data. An example of possible econometric applications of the model was considered using the current cyclic contraction in US incomes.
Citation: Karmalita Viacheslav. Stochastic model of economic cycles and its econometric application[J]. Data Science in Finance and Economics, 2024, 4(4): 615-628. doi: 10.3934/DSFE.2024026
[1] | Anatoly A. Kilyachkov, Larisa A. Chaldaeva, Nikolai A. Kilyachkov . Dynamics of stability of the world economic system. Data Science in Finance and Economics, 2023, 3(1): 101-111. doi: 10.3934/DSFE.2023006 |
[2] | Farooq Ahmad Bajwa, Jingtao Fu, Ishtiaq Ahmad Bajwa, Manzar Rehman, Karim Abbas . Digital financial inclusion and its dual impact on economic and environmental outcomes in ASEAN countries. Data Science in Finance and Economics, 2025, 5(1): 53-75. doi: 10.3934/DSFE.2025004 |
[3] | John Leventides, Evangelos Melas, Costas Poulios . Extended dynamic mode decomposition for cyclic macroeconomic data. Data Science in Finance and Economics, 2022, 2(2): 117-146. doi: 10.3934/DSFE.2022006 |
[4] | Georgios Alkis Tsiatsios, John Leventides, Evangelos Melas, Costas Poulios . A bounded rational agent-based model of consumer choice. Data Science in Finance and Economics, 2023, 3(3): 305-323. doi: 10.3934/DSFE.2023018 |
[5] | Fangzhou Huang, Jiao Song, Nick J. Taylor . The impact of time-varying risk on stock returns: an experiment of cubic piecewise polynomial function model and the Fourier Flexible Form model. Data Science in Finance and Economics, 2021, 1(2): 141-164. doi: 10.3934/DSFE.2021008 |
[6] | Kexian Zhang, Min Hong . Forecasting crude oil price using LSTM neural networks. Data Science in Finance and Economics, 2022, 2(3): 163-180. doi: 10.3934/DSFE.2022008 |
[7] | Roylan Martinez . Optimization proposals to the payment clearing. Data Science in Finance and Economics, 2023, 3(1): 76-100. doi: 10.3934/DSFE.2023005 |
[8] | Matthew Ki, Junfeng Shang . Prediction of minimum wages for countries with random forests and neural networks. Data Science in Finance and Economics, 2024, 4(2): 309-332. doi: 10.3934/DSFE.2024013 |
[9] | Nurun Najwa Bahari, Hafizah Bahaludin, Munira Ismail, Fatimah Abdul Razak . Network, correlation, and community structure of the financial sector of Bursa Malaysia before, during, and after COVID-19. Data Science in Finance and Economics, 2024, 4(3): 362-387. doi: 10.3934/DSFE.2024016 |
[10] | Jawad Saleemi . Political-obsessed environment and investor sentiments: pricing liquidity through the microblogging behavioral perspective. Data Science in Finance and Economics, 2023, 3(2): 196-207. doi: 10.3934/DSFE.2023012 |
In this paper, I demonstrate the adequacy of economic systems to the basic provisions of synergetics, which makes the latter eligible for macroeconomic analysis. In justifying this statement, a synergetic approach to the development of a model of economic cycles was considered. The novelty of this model was related to the probabilistic description of the investment function and the perception of the economic system as a material object with certain properties. According to the model, the income oscillations are induced by both exogenous (investment fluctuations) and endogenous (system elasticity) causes. The cycle's amplitudes correlate with the intensity of investment fluctuations as well as the efficiency of the economic system. The duration of the economic cycle is determined by the inclusive wealth of the system and its dynamic factor, which characterizes the ability of the system to withstand investment fluctuations and eliminates their consequences. Thus, the economic cycle is interpreted as the "natural noise" accompanying the functioning of market economics.
The proposed model creates a mathematical basis for the numerical analysis of empirical economic data. An example of possible econometric applications of the model was considered using the current cyclic contraction in US incomes.
As is known, in accordance with the objects of research, sciences are divided into natural, engineering, and social. Natural sciences focus on objects of the material world and the phenomena associated with them. In engineering sciences, the results of human activity in the forms of artificially created objects and processes occurring in them are investigated. The objects of social sciences include human society and relations both between people and their groups. Economics belongs to the social sciences (Samuelson, Nordhaus, 2004), although it includes objects of all sciences:
● Natural in the form of human population, flora and fauna of the earth, mineral resources, and natural phenomena;
● engineering – artificial objects that produce goods and provide services;
● social – connections/relationships of people during the production of goods/services and their distribution/exchange.
From the point of view of system analysis, the presence of subjects of various sciences in the object of economic research enables us to present it as a set of different subsystems. This approach is typical for the post-nonclassical stage (second half of the 20th century - beginning of the 21st century) of the development of science, which is characterized by the synergetics paradigm (Haken, 2004). The term "synergetics" denotes the doctrine of general laws operating in a system consisting of subsystems, the interaction of which can lead to self-ordering of its behavior. The possible diversity of the nature of subsystems determines the search for general patterns of the emergence of the macrostructure of the system, i.e., the search for isomorphic models. The property of isomorphism enables us to transfer the results obtained in the study of phenomena of one physical nature to phenomena of a different nature. According to (Haken, 2004), the general principles discovered in this way are applicable, among other things, to the social sciences. His statement is confirmed by many years of practice in applying synergetic approaches in economic science. Moreover, the term "Synergetic Economics" (Zang, 1999) is commonly used.
It should be noted that, as a paradigm of cognitive activity, synergetics are present in economics, as a rule, mainly at the methodological level (Essays on economic synergetics, 2017). This prompted me to justify the use of the synergetic approach in modeling economic cycles.
Hermann Haken singed out the major provisions of synergetics as follows:
● The interaction between subsystems leads to the emergence of space-time structures;
● the systems under consideration are subject to external and internal oscillations;
● such systems are open and non-linear;
● in many cases, they have a mathematical description;
● the presence of a self-organization mechanism for the system.
Let us turn to the macroeconomic problem of developing models of various (regional, national, and global) economic systems. As a material object, they represent a geographically distributed (in two coordinates) multidimensional (multiple inputs/outputs) system, that is, its input I(t) and output X(t) are vectors.
Consider one of the system inputs in the form of an investment function, which we denote as I(t). Its value is the result of actions of N(t) agents, each of which generates an investment Ij(t), characterized by the initial capital Cj, duration Tj, and return ΔCj. We will assume that the change in return over time occurs in a linear manner (Figure 1).
The presented description of the investment function with a linear change in time does not contradict its real behavior. Indeed, the current value of investment returns is usually an order of magnitude less than their values. In addition, possible fluctuations in actual yield values relative to the accepted linear trend can be interpreted as small deviations. Thus, they represent values that are two orders of magnitude smaller than the values of the investment function. This justifies the use of the made assumption of a linear change in investment returns.
The variable values of the investment cycle correspond to the period of the so-called sawtooth function Fj(t) with amplitude Aj. This periodic function can be represented by the infinite Fourier series (Pavleino, Romadanov, 2007):
Fj(t)=2Ajπ∑∞l=11lsin(2πlt/Tj)=ΔCjπ∑∞l=11lsin(2πlt/Tj). | (1) |
In particular, the first (l = 1) harmonic sin(2πft) with frequency f = 1/Tj is shown in Figure 1. This implies the validity of the statement about the susceptibility of economic systems to external oscillations.
Consider one of the outputs of the economic system the income function denoted as X(t), which is the monetary value of the manufactured products and provided services. Usually, it is represented by a gross domestic product hereinafter G(t), which is the total value of X(t) over a given interval of time. As an example, Figure 2 illustrates the rate of change in time of the world's annual gross product (Korotaev, Tsirel, 2010).
It is clear from the figure that the rate of the world gross product has fluctuations in the form of irregular oscillations. The above examples confirm the presence of both external (input) and generated (output) oscillations in economic systems.
Let us recall that synergetics deals only with nonlinear systems. Spectral analysis of fluctuations in Figure 2 established that the Kuznetz swing is the third harmonic of the Kondratyev wave (Korotaev, Tsirel, 2010). This fact and the well-known trigonometric formula
sin32πft=0.75sin2πft−0.25sin2π(3f)t | (2) |
enable us to assume the cubic nonlinearity of the considered economic system.
As an economic system has interactions with the external operating environment (market) in the form of matter and information, the system can be classified as open. Moreover, it is dissipative (Willems, 1971) because it supplies (loses) a product (matter) to the market. As is known (Ashby, 1962), in distributed dissipative systems, due to their inherent self-organization mechanisms, localized and interconnected objects can appear (disappear). In the case of economic systems, such objects are business entities, and the elements of the self-organization mechanism are their establishment, merger, acquisition, restructuring, bankruptcy, and so on.
Summarizing the above, we can say that economic systems are non-linear space-time structures, which, since Isaak Newton's time, have been mathematically described in the form of partial differential equations (PDE):
ΛX(t)=I(t). | (3) |
The mathematical operator Λ (a system of differential equations) relates the input I(t) to a system's state X(t) under boundary (interactions with a market) conditions.
The demonstrated conformity of the essence of economic systems with the basic provisions of synergetics justifies its use in macroeconomic studies. This statement is illustrated in the next section using the example of a one-dimensional model of economic cycles.
It should be noted that both in the seminal work of modern business cycle theory (Kydland, Prescott, 1982), as well as in subsequent ones, for example, (Kehoe et al., 2018) there is no clear explanation of its "driving force". Usually, some external shocks of unformalized nature and cause are mentioned as sources of cycles (Golosov, Menzio, 2020). This means that the mechanism of economic cycles, as well as its formal (mathematical) model within the framework of the modern theory of economic cycles, are not clearly defined. This fact leads to the development of mathematical models that only describe (approximate) the results of observing cycles.
However, the mention of external shocks as the "driving force" of fluctuations in economic systems enables us to classify the latter as elastic. Such systems, after an external shock, generate free oscillations relative to the equilibrium state with the natural frequency of the system and exponentially decreasing amplitude (Bolotin, 1984). The property of elasticity of economic systems, which enables them to withstand market fluctuations, is due, as noted earlier, to the self-organization mechanism inherent in economic entities.
In the case when the economic system has only one input and one output, its model will be one-dimensional and mathematically written as the following nonlinear differential equation (Karmalita, 2020):
F(t,I,X,˙X,¨X)=0. | (4) |
The input of this model is the investment function I(t), and its output X(t) is the total income.
Formally, the jth investment (Figure 1) can be written as follows:
Ij(t)=Cj+ΔCj/2+Ej(t), | (5) |
where Ej(t) represents the fluctuations of the investments with respect to the value (Cj+ΔCj/2). Since investments in a market economy are the result of the independent activity of N(t) agents, they can have a random beginning. Accordingly, the deviation εji = Ej(ti) in Figure 1 is stochastic due to the random (equiprobable) position of the moment ti within the range 0…Tj. Representing the system input I(t) as the sum of existing investments made it possible to write the investment function in terms of the deterministic M(t) and stochastic E(t) components:
I(t)=∑N(t)j=1Ij(t)=∑N(t)j=1(Cj+ΔCj2)+∑N(t)j=1ϵj(t)=M(t)+E(t). | (6) |
The component M(t) determines the long-term trend on investments, and the process E(t) – their fluctuations around the trend. The summation of independent equiprobable values ϵj(t) leads to a Gaussian distribution of the process E(t) with zero mathematical expectation and variance:
Dε=σ2ε=∑N(t)j=1ΔC2j12. | (7) |
The value of Dε is always finite due to the boundedness of ΔCj and N(t). In fact, the investment cycles differ in their durations: for some, it is days, for others, years or even decades. This means that fluctuations E(t) are a broadband random process with independent and finite values. A mathematical model of such a process can be "white" noise.
It should be noted that the expression (6) confirms the legitimacy of the postulate (Cooley, Prescott, 1995) on the need to consider economic growth and fluctuations together. Therefore, the income function can also be represented by two components:
X(t)=L(t)+δX(t), | (7) |
where L(t) is the long-term trend in income due to the investment trend M(t), and the deviation δX(t) is caused by investment fluctuations E(t). δX(t) includes all known business cycles (Kondratiev, Kuznets, Juglar, and Kitchin) as well as other possible income oscillations, that is:
δX(t)=ΞKon(t)+ΞKuz(t)+ΞJug(t)+ΞKit(t)+… | (8) |
From Figure 2, it follows that amplitudes of fluctuations are an order of magnitude smaller than the values of X(t), that is, the cycles are small deviations of the income function. Therefore, to describe the cycle Ξ(t) with duration T0, equation (4) can be linearized and reduced to an ordinary differential equation of the second order:
¨Ξ(t)+2h˙Ξ(t)+(2πf0)2Ξ(t)=E(t). | (9) |
The description of small deviations by linear equation corresponds to the practice of modeling nonlinear dynamic systems.
Equation (9) describes the dynamics of an elastic system with the natural frequency f0 = 1/T0 and damping factor h under the influence of white noise. In this case, the system's output consists of random oscillations, whose frequencies are concentrated mainly in the vicinity of f0. Therefore, there is another name for random oscillations - a narrow-band random process.
To determine quantitative characteristics of random oscillations, a notion of a "dynamic shaping system" can be implemented. This approach enables us to consider the observing process as the output of a hypothetical system, with the input being the white noise. Accordingly, parameters of this system are used to characterize (describe) the observing process. This means that the random oscillations may be exhaustively described both by the parameters of a linear elastic system (f0, h) and by their intensity (σξ=√Dξ).
Model (9) has been successfully used to describe oscillatory processes in objects of natural (vibrational combustion) and engineering (structural vibrations) sciences (Karmalita, 2019). Its isomorphism enables us to use this model to study cyclical processes in economic systems. The only thing is that its parameters must have an interpretation corresponding to the conceptual basis of economic theory.
We start by looking at the damping factor h, which characterizes the loss of energy in a mechanical system. In other words, it can characterize the efficiency of the elastic system. With regards to the economic systems, h can be interpreted as an indicator of their expenses. However, such an interpretation of the damping factor is not supported by any quantitative correlation of its value with expenses of economic systems. Therefore, in (Karmalita, 2025) the root-mean-square (rms) gain is proposed as an indicator of the economic system's efficiency in the form of the following ratio:
Kσ=σξσϵ. | (10) |
Here, σξ and σε are rms values of the income oscillations Ξ(t) and investment fluctuations E(t), respectively. Kσ characterizes the increase of income generated by the system; therefore, the greater the gain, the higher the efficiency of the system.
Since both Kσ and h are related to the efficiency of the elastic system, there must be a quantitative relationship between them. In the case of a discrete representation of Ξ(t) with a dimensionless time interval Δt = 1, this relationship is shown in Figure 3.
As for the natural frequency f0 of the system, its value within the framework of the theory (Karmalita, 2025) under consideration correlates with system-inclusive wealth WI :
f0=1T0=12π⋅√wsWI. | (11) |
Here, WI is the monetary value of all system assets (production, labour, inventory, and finance), and ws is the dynamic factor that characterizes the system's ability to withstand investment fluctuations and eliminate their consequences.
The probabilistic description of investments gave meaning to their representation in the frequency domain, identifying the harmonic period with the duration of investments. So, the properties of a linear-elastic system can be analyzed in the frequency domain, where they are described by the system's amplitude A(f) and phase Φ(f) frequency characteristics of the system (Figure 4).
A(f) determines the ratio of the amplitudes of the input (investment) and output (income) harmonics. The values of random oscillations Ξ(t) are determined mainly by harmonics in the vicinity of f0 (f1 = 0.7f0 ≤ f ≤ f2 = 1.4 f0).
Φ(f) is the difference between phases of the investment and income harmonics, which is equivalent to the time delay of the output with respect to the input. From Figure 4 it follows that investment manipulations lead to a tangible change in the intensity of the cycle with a time delay T0/4, and a complete change after half the cycle period.
Model (9) not only explains the phenomenon of the cycle but also provides its quantitative description. The parameter f0 determines the the position of the AF characteristic peak on the frequency axis, and h characterizes its bandwidth Δf0.707 at points where A(f) = 0.707A(f0) as shown in Figure 4 The relationship between Δf0.707 and the damping factor h is as follows (Pain, 2005):
h=π⋅Δf0.707. | (12) |
Since the amplitude spectrum of the output of a linear dynamic system is equal to the product of its amplitude frequency characteristic and the amplitude spectrum of the input, then Aξ(f) = A(f)·Aε(f). Note that white noise has a uniform spectrum (see Figure 5).
The value σε = √Dϵ in above figure is the root-mean square value of white noise E(t), and θ is the relative (dimensionless) frequency (Δt = 1). Therefore, Aξ(f) is directly proportional to the amplitude frequency characteristic of the system due to the uniformity of Aε(f). This fact means that estimates of the parameters of model (9) can be determined from the amplitude spectrum of deviations δX(t). In other words, this model provides a theoretical basis for the mathematical analysis of the behavior of economic cycles, as shown in the next section.
In econometric studies, to quantify the income function X(t) the gross domestic product (GDP) is usually used. First, we need to assess the suitability of G(t) for estimating the parameters f0 and h of model (4). Recall that the value of GDP is a monetary estimate of manufactured goods and provisioned services for a certain period ΔT, that is, G(t) is mathematically described in the following form:
G(t)=∫tt−ΔTX(t)dt=∫t0hG(τ)X(t−τ)dτ=∫t0hG(τ)L(t−τ)dτ+∫t0hG(τ)δX(t−τ)dτ=GL(t)+g(t)=GL(t)+…+gΞj(t)+⋯. | (12) |
Therefore, GDP function can be interpreted as the result of measuring the income function by the estimator described by the impulse response hG(τ):
hG(τ)={1,0≤τ≤ΔT;0,τ<0;τ>ΔT. | (13) |
Applying the linear estimator operator to a homogeneous version of equation (4) leads it to the following form:
¨gΞ(t)+2h˙gΞ(t)+(2πf0)2gΞ(t)=0. | (14) |
Hence, it follows that the parameters f0 and h also characterize the properties of the GDP.
Let us turn to the procedure for estimating GDP, conditionally presented in Figure 6.
The diagram includes the measurement noise Z(t) describing the actual properties of the estimation procedure. It arises, for instance, due to unreliable statistical data, their incompleteness, as well as the skill of the performers. Thus, the available data related to the values of GDP are the estimates ˜G(t) determined as:
˜G(t)=G(t)+Z(t)=˜GL(t)+˜g(t). | (15) |
The Z(t) is usually a broadband process and is modeled as "white" noise. Here, and further in the text, the sign "~" denotes a quantitative estimate of the noted variable.
Consider quarterly (Δt = ΔT = 0.25 year) GDP estimates of US income for the period 1995–2019 are shown in Figure 7.
To increase the representativeness of the empirical data (number of samples), additional terms were calculated via linear interpolation of quarterly GDP estimates. Thus, the sampling interval was reduced to Δt = ΔT/2 = 0.125 years, and the number of samples increased to n = 199. The GDP deviation ˜gi=˜g(ti)=˜g(Δt⋅i) (Figure 8) were calculated relative to the regression ˆGLi = 0.054·i2 + 57.289·i + 7522 obtained by the least squares method (Brandt, 2014).
If we proceed to the dimensionless interval of sampling Δt = 1, then the results will be presented in terms of relativenatural frequency 0 ≤ θ ≤ 0.5.The amplitude frequency spectrum (θ) shown in Figure 9 can be determined through the Fourier transform (Cho, 2018) of GDP deviations i.
It should be noted that the mode (peak) of each cycle in Figure 9 corresponds to θh=√θ20−(h⋅Δt/2π)2, which is its natural frequency corrected for damping. From the above spectrogram, the estimates of these frequencies are ˜θKuz ≈ 0.0093 (˜TKuz = Δt/˜θKuz=0.1250.0093≈ 13.5 years), ˜θJug ≈ 0.0198 (˜TJug ≈ 6.3 years) and ˜θKit ≈ 0.0344 (˜TKit ≈ 3.6 years). The frequency values were determined with a resolution Δθ = 0.5/2048 ≈ 0.000244.
From the Wiener-Khinchin theorem, it follows that the cycle intensity is correlated with the area under its amplitude spectrum:
Dξ=∫0.50A2ξ(θ)dθ. | (16) |
Figure 9 clearly demonstrates that the Kuznets swing is dominant. Therefore, knowing the moment of reaching the last trough of the Kuznets swing and its duration, it is possible to determine the moments of the subsequent peak (tp) and trough (tt). Its previous trajectory (Figure 10) formed from ˜gi using a low-pass FIR filter ˜gKuz,(i−127)=∑128l=1cl˜gi+l−128(i=128,…,199),as suggested in (Karmalita, 2025).
The cross-correlation coefficient (Brandt, 2014) of this trajectory with deviations ˜gKuz,i at the contraction stage is ˜r˜g˜ΞKuz≈0.94. It means that the Kuznets swing was a determining factor of the 2008 recession. The above allows us to forecast the next cyclic contraction in GDP based on a knowledge of the last trough of the Kuznets swing (symbol in Figure 10) and its period.
However, due to technological and managerial progress in the economy, the properties (characteristics) of economic systems are changing. As a result, the period TKuz in the predicting interval (say, 2020–2025) may be different. In (Karmalita VA, 2025) the trend of estimates ˜θKuz for the period 1960-2020 was analyzed. It was demonstrated that estimates of relative frequency (durations in years) of the Kuznets swing increased (decreased) from 0.018 to 0.02 (13.7 → 12.3). On average, each subsequent period of the cycle saw a continuous decrease of ≈2.2%. Consequently, the change in the value of TKuz over the forecast interval (2020–2025) will be ≈1.1%. The relative (to ˜θKuz) frequency resolution of the spectrum (≈1.2%) is of the same order. This fact allows us to use the value ˜TKuz = 12.3 years to assess the behavior of the cycle during its next cyclical contraction. Note that the last trough of the Kuznets swing occurred in the 3rd quarter of 2011, so the next time point tt will be at the beginning of 2024.
Recall that the actual behavior of the cycle is determined by both endogenous (parameters of the elastic system) and exogenous causes. The latter include fluctuations in investment E(t), the manipulation of which leads to a change in the intensity (amplitude) of the cycle. The intensity, as it follows from expression (3), depends on the number N(t) of investments and returns ΔCj. The return of the jth investment can be represented as a function of its interest and duration:
ΔCj(t)=Cj⋅R(t)⋅αj⋅Tj, | (17) |
where R(t) is the bank's prime loan rate (hereinafter the prime rate), and αj is a constant. The recognized existence of an inverse relationship between investment activity and the prime rate (Blanchard, 2017) allows us to present it as:
N(t)=k/R(t), | (18) |
where k is a constant. Now the sum in expression (3) can be written in the following form:
∑N(t)j=1ΔC2j=R2(t)∑N(t)j=1C2j⋅α2j⋅T2j=R2(t)⋅N(t)⋅β(t)=k⋅β(t)⋅R(t), | (19) |
where β(t) = ∑N(t)j=1C2j⋅α2j⋅T2j/N(t).
Previously, the relationship between the intensities σξ and σε is determined by the root-mean-square gain Кσ of the economic system:
σξ=Kσ⋅σε. | (20) |
Therefore, the above expression transforms into:
σξ=Kσ⋅σϵ=Kσ√k⋅β(t)⋅R(t)/12. | (21) |
It follows from expression (21) that a change in R(t) leads to a change in the intensity of the cycle. If at the stage of cyclic contraction, the prime rate rises, then the trough of the cycle will fall lower. Moreover, the delay between investments and income (see Figure 4) will postpone the achievement of a new trough for the future. Such a cycle metamorphosis can be illustrated by the example of the Kuznets harmonica SKuz(t) = АR(t)·cos 2πfKuz(t - tp) during the current cyclic contraction. Recall that its time delay is a quarter of the period, that is, ˜τKuz = ˜TKuz /4 ≈ 3.0 years.
As you know, the prime rate was reduced to 3.25% on March 16, 2020, and did not change until March 17, 2022 (Figure 11).
Considering the value of τKuz, the amplitude A3.25 of the Kuznets harmonic stabilizes in the first quarter of 2023, remaining unchanged at the through moment (Figure 12).
The first increase in the primary rate in 2022 to 3.5% (see Figure 11) will lead to a change in the amplitude AR(t) in the first quarter of 2025 (symbol in Figure 12). This and subsequent changes in the harmonic trajectory are estimated as SKuz(t) = А3.25·√R(t)/3.25⋅ cos 2πfKuz(t - tp).
From the presented trajectory of the symbol it follows that the change in the cycle values has stopped with the growth of the base rate. Moreover, an increase in the R(t) value, for example, in the first half of 2021, could lead to both an increase in the cycle contraction and a shift of the trough to a later time.
The synergetic approach led to the creation of a stochastic model of economic cycles, which explains their phenomenon and provides a quantitative (parametric) description of cycles. According to this model, economic cycles in the form of random oscillations of income are induced by both exogenous (investment fluctuations) and endogenous (system elasticity) causes. Cycle's amplitudes relate to the intensity of investment fluctuation as well as the rms gain (efficiency) of the corresponding economic system. In turn, the cycle duration depends on the inclusive wealth of the system and its dynamic factor, which characterizes the system's ability to withstand investment fluctuations and eliminate their consequences. The above enables us to interpret cycles as the „natural noise "accompanying the functioning of economic systems in market conditions. The economic crisis is caused by a slump in the volume of investments due to the state of the market, that is, in the values of the deterministic component of the investment function.
The practical application of the proposed model for econometric purposes is demonstrated by analyzing the current cyclic contraction in US income.
The author declares they have not used Artificial Intelligence (AI) tools in the creation of this article.
The author declares no conflicts of interest in this paper.
[1] | Ashby WR (1962) Principles of the Self-Organizing System, In: Principles of Self Organization: Transactions of the University of Illinois Symposium, Eds: H. von Foerster and G. Zopf, Pergamon Press, 255–278. |
[2] | Blanchard OJ (2017) Macroeconomics, 7th ed., Boston: Pearson. |
[3] | Bolotin VV (1984) Random vibrations of elastic systems, Heidelberg: Springer. |
[4] | Brandt S (2014) Data Analysis: Statistical and Computational Methods for Scientists and Engineers, 4th ed., Cham, Switzerland: Springer. |
[5] | Cho S (2018) Fourier Transform and Its Applications Using Microsoft EXCEL®, San Rafael, CA: Morgan & Claypool. |
[6] | Cooley TF, Prescott EC (1995) Economic growth and business cycles, In: Frontiers of business cycle research, ed: Cooley TF, Princeton: Princeton University Press, 1–38. |
[7] |
Golosov M, Menzio G (2020) Agency business cycles. Theor Econ 15: 123–158. https://doi.org/10.3982/TE3379 doi: 10.3982/TE3379
![]() |
[8] | Haken H (2004) Synergetics: Introduction and Advanced Topics, Berlin, Heidelberg: Springer-Verlag. |
[9] | Essays on economic synergetics (2017) Eds: Mayevsky V I, Kirdina-Chandler S G, Deryabina M A, Moscow: Institute of Economics, Russian Academy of Sciences (in Russian). |
[10] | Karmalita V (2019) Digital processing of random oscillations, Berlin: De Gruyter. |
[11] |
Karmalita VA (2023) Recovering the actual trajectory of economic cycles. J Econ Math Method 59: 19–25. https://doi.org/10.31857/S042473880024867-2 doi: 10.31857/S042473880024867-2
![]() |
[12] | Karmalita VA (2025) Stochastic dynamics of economic cycles: macroeconomic theory and econometric application, Moscow: Prometheus (in Russian). |
[13] |
Kehoe PJ, Midrigan V, Pastorino E (2018) Evolution of modern business cycle models: accounting or the great recession. J Econ Perspect 32: 141–166. https://doi.org/10.1257/jep.32.3.141 doi: 10.1257/jep.32.3.141
![]() |
[14] |
Korotaev AV, Tsirel SV (2010) Spectral analysis of World GDP Dynamics: Kondratieff Waves, Kuznets Swings, Juglar and Kitchin Cycles in Global Economic Development, and the 2008–2009 Economic Crisis. J Biomol Struct Dyn 4: 3–57. https://doi.org/10.5070/SD941003306 doi: 10.5070/SD941003306
![]() |
[15] | Kydland F, Prescott E (1982) Time to build and aggregate fluctuations. J Econometrica 50: 1345–1370. |
[16] | Pain HJ (2005) The physics of vibrations and waves, 6th ed., Chichester, England: Wiley. |
[17] | Pavleino MA, Romadanov VM (2007) Spectral transforms in MATLAB®, St.-Petersburg: SPbSU (in Russian). |
[18] | Samuelson P, Nordhaus W (2004) Economics, 18th ed., Irwin: McGraw-Hill. |
[19] |
Willems JC (1971) Dissipative Dynamic Systems, Part I: General Theory. Arch Rational Mech Anal 45: 321–352. https://doi.org/10.1007/BF00276493 doi: 10.1007/BF00276493
![]() |
[20] | Zhang WB (1999) Synergetic Economics: Time and Change in Nonlinear Economics, Berlin, Heidelberg: Springer Verlag. |