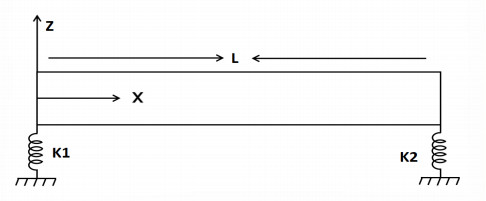
Citation: Timothy J. Ricker. The Role of Short-term Consolidation in Memory Persistence[J]. AIMS Neuroscience, 2015, 2(4): 259-279. doi: 10.3934/Neuroscience.2015.4.259
[1] | Muhammad Tariq, Hijaz Ahmad, Soubhagya Kumar Sahoo, Artion Kashuri, Taher A. Nofal, Ching-Hsien Hsu . Inequalities of Simpson-Mercer-type including Atangana-Baleanu fractional operators and their applications. AIMS Mathematics, 2022, 7(8): 15159-15181. doi: 10.3934/math.2022831 |
[2] | Saad Ihsan Butt, Artion Kashuri, Muhammad Umar, Adnan Aslam, Wei Gao . Hermite-Jensen-Mercer type inequalities via Ψ-Riemann-Liouville k-fractional integrals. AIMS Mathematics, 2020, 5(5): 5193-5220. doi: 10.3934/math.2020334 |
[3] | Saad Ihsan Butt, Erhan Set, Saba Yousaf, Thabet Abdeljawad, Wasfi Shatanawi . Generalized integral inequalities for ABK-fractional integral operators. AIMS Mathematics, 2021, 6(9): 10164-10191. doi: 10.3934/math.2021589 |
[4] | Jamshed Nasir, Saber Mansour, Shahid Qaisar, Hassen Aydi . Some variants on Mercer's Hermite-Hadamard like inclusions of interval-valued functions for strong Kernel. AIMS Mathematics, 2023, 8(5): 10001-10020. doi: 10.3934/math.2023506 |
[5] | Soubhagya Kumar Sahoo, Fahd Jarad, Bibhakar Kodamasingh, Artion Kashuri . Hermite-Hadamard type inclusions via generalized Atangana-Baleanu fractional operator with application. AIMS Mathematics, 2022, 7(7): 12303-12321. doi: 10.3934/math.2022683 |
[6] | Thabet Abdeljawad, Muhammad Aamir Ali, Pshtiwan Othman Mohammed, Artion Kashuri . On inequalities of Hermite-Hadamard-Mercer type involving Riemann-Liouville fractional integrals. AIMS Mathematics, 2021, 6(1): 712-725. doi: 10.3934/math.2021043 |
[7] | Yamin Sayyari, Mana Donganont, Mehdi Dehghanian, Morteza Afshar Jahanshahi . Strongly convex functions and extensions of related inequalities with applications to entropy. AIMS Mathematics, 2024, 9(5): 10997-11006. doi: 10.3934/math.2024538 |
[8] | Muhammad Zakria Javed, Muhammad Uzair Awan, Loredana Ciurdariu, Omar Mutab Alsalami . Pseudo-ordering and $ \delta^{1} $-level mappings: A study in fuzzy interval convex analysis. AIMS Mathematics, 2025, 10(3): 7154-7190. doi: 10.3934/math.2025327 |
[9] | Miguel Vivas-Cortez, Muhammad Aamir Ali, Artion Kashuri, Hüseyin Budak . Generalizations of fractional Hermite-Hadamard-Mercer like inequalities for convex functions. AIMS Mathematics, 2021, 6(9): 9397-9421. doi: 10.3934/math.2021546 |
[10] | Thongchai Botmart, Soubhagya Kumar Sahoo, Bibhakar Kodamasingh, Muhammad Amer Latif, Fahd Jarad, Artion Kashuri . Certain midpoint-type Fejér and Hermite-Hadamard inclusions involving fractional integrals with an exponential function in kernel. AIMS Mathematics, 2023, 8(3): 5616-5638. doi: 10.3934/math.2023283 |
L: Length; E: Young's modulus; I: a moment of inertia of cross section; A: cross section area; $ x $: longitudinal axis; $ \rho $: density; $ s $: Displacement; $ K_{1} $, $ K_{2} $: linear stiffness of the springs at right and left ends, respectively; $ S(x) $: normal function; $ \omega $: eigenfequencey; $ c $: wave speed; $ \eta $: eigenvalues; $ C_{i} (i = 1, 2, 3, 4) $: cnstant coefficient; $ Q(x) $: weighted functions; $ N_{i} (i = 1, 2, 3, 4) $: shape functions; $ {s_{j}} $: function of space and time component; $ \bar{s_{j}} $: amplitude of vibration varying with time; $ k^{\epsilon} $: stiffness matrix; $ m^{\epsilon} $: mass matrix; N: number of element
The beam, a fundamental structural element, is typically characterized by having one dimension significantly larger than its other dimensions. This elongated geometry allows beams to efficiently carry loads and distribute them along their length. Euler-Bernoulli beam (EBB) theory stands as one of the foundational frameworks used to describe the behavior of beams under certain conditions [1]. The development of beam equations has a rich historical context, with contributions from notable figures such as Vinci and Galilei. During the eighteenth century, significant advancements in beam theory were made by eminent mathematicians including Euler, Jacob, and Daniel, culminating in the establishment of comprehensive analytical tools for understanding beam behavior. The application of beam theory extends across a diverse range of engineering disciplines, reflecting its essential role in various practical applications. In transportation engineering, beams are integral components of bridges, roadways, and railway tracks, where they provide structural support and facilitate the safe passage of vehicles and pedestrians. Structural engineering heavily relies on beam theory to design and analyze buildings, bridges, and other infrastructure projects, ensuring their stability and resilience against external forces such as wind and seismic loads. In aerospace engineering, beams play a crucial role in the design and construction of aircraft, spacecraft, and aerospace structures, where they must withstand complex loading conditions and extreme environments. Scholarly research in the field of continuous structural beam systems has yielded valuable insights and methodologies that contribute to advancements in engineering practice. Works by [2,3] exemplify the breadth of studies focused on beam theory, covering topics ranging from structural analysis and design optimization to materials research and performance evaluation. These studies underscore the interdisciplinary nature of beam theory and its significance in addressing real-world engineering challenges across multiple sectors.
Numerous researchers have delved into the intricate dynamics of transverse vibrations exhibited by beam-spring systems, conducting both exact and approximate analyses to ascertain the natural frequencies governing their behavior. These investigations have explored the nuanced interplay between beam vibrations and various factors, including the characteristics of the elastic foundation supporting the beam. The effects of springs, rotary inertia, and mass distribution have been subjects of intensive scrutiny across a spectrum of studies, underscoring the multidimensional nature of beam-spring dynamics. The significance of springs in modulating beam vibrations has been a focal point of investigation, with studies such as [4,5] shedding light on their role in altering the structural response. Additionally, the influence of rotary inertia, mass distribution, and other dynamic parameters has been rigorously examined in works [6], among others. These investigations have contributed valuable insights into the intricate dynamics of beam-spring systems and their implications for diverse engineering applications. $ \ddot{O} $z [7] and $ \ddot{O} $zkaya [8] determined beam frequencies, incorporating mass and using both analytic and finite element methods. Grossi and Arenas [9] employed Rayleigh-Ritz and optimized Rayleigh-Schmidt methods to investigate frequency variations with changes in height and width. Smith et al. [10] introduced the fully Sinc-Galerkin method in space and time to solve beams with cantilever and fixed boundary conditions. Moaaz et al. [11] formulated mathematical expressions for transverse resonance in simply supported, axially compressed thermoelastic nanobeams, employing nonlocal elasticity theory and the dual-phase-lag heat transfer model to explore the impact of length scale and axial velocity on system responses. Baccouch [12] applied the Galerkin method to solve the Euler-Bernoulli beam equation, while Xie and Zhang [13] investigated difference methods for nonlinear equations with damping. Additionally, Shi et al. [14] explored the mixed finite element method for solving such equations.
The variational iteration technique was used by Liu and Gurran [15] to find the natural frequencies and mode shapes of the beam under different boundary conditions. Galerkin finite element method (FEM), Rayleigh-Ritz, and exact solutions were compared by Hamdan and Latif [16], and the exhibited FEM was preferable because of good accuracy. Jafari et al. [17] derived the equation of motion of beam by applying Hamilton's principle and obtained the eigenfrequencies and mode shapes for cantilever beam connected with linear spring at the tip by using the FEM.
Unlike boundary element method, finite difference method, and finite volume method, which are widely used in acoustic and fluid mechanics (see for example, [18,19]), the study of FEM is considered as the standard approach in presenting solutions to structural problems, see for instance, [20,21]. Many industries use FEM software such as ABAQUS (based on Abundant Beads Addition Calculation Utility System tool), NASA structure analysis, and analysis system for the commercial purpose. It has been widely discussed on static problems where numerous codes existed while having less validation and verification for standard problems with respect to dynamics of determining the eigenfrequencies. In the study of beam dynamics using FEM, consistent mass (CM) and lumped mass (LM) matrices are critical in accurately representing the system's behavior. The choice between these two matrices has an impact on the accuracy and computing efficiency of the analysis. The consistent mass matrix accurately preserves the system's mass distribution by taking into account the mass contributions of all finite constituents. This matrix is constructed directly from the discretized governing equations, yielding more accurate results, particularly for bigger models with variable element sizes. However, its bigger size necessitates more computing effort and storage. The lumped mass matrix, on the other hand, combines the mass contributions into nodal points, making the computing process easier. While this matrix decreases computational effort and storage needs, it may produce less accurate results, especially in systems with large mass variations or irregular geometries. Hence, the primary aim of this study is to present a comprehensive analysis of the vibration behavior of beam-spring systems using FEM with a focus on the comparative performance of CM and LM matrices. Our work bridges the gap between mathematical analysis and engineering applications by addressing two primary objectives:
(1) Determining the preferred matrix type (CM or LM) for such analyses from a mathematical perspective;
(2) Examining the deflection behavior while adjusting the stiffness of the supports at each end of the beam from an engineering perspective.
The novelty of our study lies in its detailed comparison of CM and LM matrices and providing insights into their respective accuracies in calculating eigenfrequencies and mode shapes, which has not been extensively covered in existing literature. By varying the stiffness of the beam supports, we analyze the dynamic behavior of the system, offering practical insights valuable for both theoretical and applied contexts. Additionally, we provide a numerical results section to include a deeper analysis of the outcomes prior to yielding a detailed introduction to the FEM procedure, including the assembly of global stiffness and mass matrices and the incorporation of boundary conditions. Therefore, the underlying study is crucial for both mathematical analysis and practical engineering applications, making our study a valuable contribution to the field of applied mathematics.
This article is categorized as follows: the governing problem is formulated in Section 2. The analytical and numerical results are presented in Section 3. Results and discussions are given in Section 4 and the validation has been performed in Section 5 while the study is summarized in Section 6.
Consider the beam configuration attached to linear springs at both ends having length, as can be viewed through Figure 1. The material of the beam is made of stainless steel. The Young's modulus is 210 GPa and material density is 7850 $ Kg/m^{3} $. The length, width, and thickness of the beam are taken as 1 m, 0.02 m, and 0.003 m, respectively.
The equation of motion for the transverse deflection function in case of free beam's vibration is given by [22]
$ EI∂4s(x,t)∂x4+ρA∂2s(x,t)∂t2=0. $
|
(2.1) |
Equation (2.1) together with the standard linear spring conditions of beam defined in [23] will be considered for the correct determination of eigenfrequencies and mode shapes. The objective is to provide a more optimal and accurate solution approach by considering the lumped and consistent mass matrices while providing the finite element solution in proceeding sections. The flexural boundary conditions at $ x = 0 $ and $ x = L $, representing the stiffness of the springs in the positive direction from left to right, are provided below:
$ EI∂2s(0,t)∂x2=0, $
|
(2.2a) |
$ EI∂3s(0,t)∂x3=−K1s(0,t), $
|
(2.2b) |
$ EI∂2s(L,t)∂x2=0, $
|
(2.2c) |
$ EI∂3s(L,t)∂x3=K2s(L,t). $
|
(2.2d) |
Equation (2.1) along with boundary conditions (2.2a–d) is solved by separation of variables to acquire the characteristics and mode shape equations where the roots of the characteristic equation are extracted through Mathematica-based code. These roots are termed as eigenfrequencies and will be useful in determining the eigenmodes in the subsequent section.
As we seek to determine the natural frequency and mode shape of the vibrating beam as given by previously defined boundary conditions, we use an analytical and numerical approach to obtain the desired results. It is appropriate to note that a numerical approach is used to consider CM and LM matrices, which provides a justification for solving more complex problems with a similar scheme.
We apply separation of variables to the governing problem given in Section 2 by letting
$ s(x,t)=S(x)T(t). $
|
(3.1) |
Therefore, Eq (2.1) can be written as [24]
$ c21S(x)∂4S(x)∂x4=−1T(t)∂2T(t)∂t2=ω2, $
|
(3.2) |
where
$ c = \sqrt{\frac{EI}{\rho A}}, $ |
and Eq (3.2) is further split as
$ d4S(x)dx4−η4S(x)=0 $
|
(3.3) |
and
$ d2T(t)dt2+ω2T(t)=0, $
|
(3.4) |
where $ \eta $ is given by
$ η=√ωc. $
|
(3.5) |
Equation (3.3) yields the following equation
$ S(x)=C1sin(ηx)+C2cos(ηx)+C3sinh(ηx)+C4cosh(ηx). $
|
(3.6) |
Using Eq (3.6) in Eq (2.2a–d), we obtain
$ −EIC2η2+EIC4η2=0, $
|
(3.7) |
$ −EIη3C1+C2K1+EIη3C3+C4K1=0, $
|
(3.8) |
$ −C1η2EIsin(ηL)−C2η2EIcos(ηL)+C3η2EIsinh(ηL)+C4η2EIcosh(ηL)=0 $
|
(3.9) |
and
$ C1[−EIη3cos(ηL)−K2sin(ηL)]+C2[EIη3sin(ηL)−K2cos(ηL)] +C3[EIcosh(ηL)−K2sinh(ηL)]+C4[EIη3sinh(ηL)−K2cosh(ηL)]=0. $
|
(3.10) |
By using Eq (3.7) in Eq (3.6), we obtain
$ S(x)=C1[C2C1(cosh(ηx)+cos(ηx))+sin(ηx)+C3C1sinh(ηx)]. $
|
(3.11) |
The results obtained by using boundary conditions (2.2a–d) are given by Eqs (3.7)–(3.10) representing a system of four equations with four unknowns $ C_{1} $–$ C_{4} $. In order to have a nontrivial solution, the determinant of the coefficient matrix must be zero, which leads to a frequency equation given as
$ −2η2EI[−η3EIcosh(η)(EIη3cos(ηL)+sin(ηL)(K1+K2)) +(EIη3(K1+K2)cos(ηL)+2sin(ηL)K1K2)sinh(ηL)+E2I2η6]=0. $
|
(3.12) |
After finding the values of $ \frac{C_{3}}{C_{1}} $ and $ \frac{C_{2}}{C_{1}} $ from Eqs (3.8) and (3.10), respectively, and putting into Eq (3.11), the $ n^{th} $ mode shape equation is determined. This equation is used to plot the mode shapes/eigenmodes subject to corresponding eigenfrequencies. The eigenfrequencies are computed by using Eq (3.5), after determining the eigenvalues ($ \eta $) from Eq (3.12).
The analytic solution for the beam attached to linear springs is achieved more conveniently. However, in case of more complex and challenging problems with added effects and flexural boundary conditions, and structural and material discontinuities [25], it becomes difficult to obtain analytical solutions. Therefore, we propose the finite element scheme with the consideration of LM and CM matrices to obtain numerical solutions for such problems in subsequent section. Albeit the scheme to be adopted herein will provide a reference model for the comparative analysis. In case of substantial problems related to beams, FEM provides a fast and easy method to address these problems.
This section aims to provide the workings of FEM by CM and LM matrices to calculate the eigenmodes and eigenfrequencies.
The first step in FEM is to discretize the beam into a finite number of elements. There are two end nodes, each with two degrees of freedom, for each beam element. As demonstrated in Figure 2, these degrees of freedom include both translational displacements $ (V_{i} $, where $ i $ = 1, 2) and rotational displacements $ (\theta_{j} $, where $ j $ = 1, 2).
The node's movement across the axis of the beam is indicated by the translational degrees of freedom, and its rotation about the corresponding axes is indicated by the rotational degrees of freedom. As the beam under examination is uniform, it is assumed that all elements, utilized to mesh the total beam, are indistinguishable. The subsequent step is to acquire the weak form of the differential equation. For this purpose, the weight functions are multiplied with the residual value of an approximate solution and are then integrated over the domain yielding zero value. While using the process of discretization and weak formation in Eq (2.1), it is found that
$ ∫L0Q(x)(EId4sdx4+ρAd2sdt2)dx=Q(x)EId3sdx3|L0−EId2sdx2dQdx|L0+∫L0EId2sdx2d2Qdx2dx+∫L0Q(x)(ρAd2sdt2)dx=0. $
|
(3.13) |
It is noted that the highest order of derivative in Eq (3.13) is three; therefore, an approximate function of thrice differentiable is selected. A cubic interpolation polynomial fulfills this requirement [26] normally. Applying the Galerkin FEM, a weight function is equated with the approximate function
$ Q_{i} = N_{i}, $ |
where these cubic interpolation functions are called cubic spline functions, given as
$ N1=1−3(xL)2+2(xL)3,N2=x(xL−1)2,N3=(xL)2(3−2xL),N4=x2L(xL−1). $
|
(3.14) |
While putting these shape functions, given by Figure 3, into Eq (3.13) and assuming
$ s = \sum\limits_{j = 1}^{4} s_{j} N{j}, $ |
we get
$ ∫L0Q(x)(EId4sdx4+ρAd2sdt2)dx=EIsjNiNj,xxx]L0−EIsjNj,xxNi,x]L0+∫L0EINi,xxNj,xxsjdx+ρA∫L0sj,ttNiNjdx=0. $
|
(3.15) |
Equation (3.15) can be written as
$ [kϵ]sj+[mϵ]sj,tt=0, $
|
(3.16) |
where $ {s_{j}} $ can be written as
$ sj={¯sj}eiωt. $
|
(3.17) |
Putting Eq (3.17) in Eq (3.16), we obtain
$ [kϵ]−ω2[mϵ]=0, $
|
(3.18) |
where $ [k^{\epsilon}] $ and $ [m^{\epsilon}] $ are given as
$ [kϵ]=EIL3[126L−126L6L4L2−6L2L2−12−6L12−6L6L2L2−6L4L2], $
|
(3.19) |
$ [mϵ]=ρAL420[15622L54−13L22L4L2L3L−3L25413L156−22L−13L−3L2−22L4L2], $
|
(3.20) |
and the LM matrix [21] would be
$ ρAL2[1000000000100000]. $
|
(3.21) |
Here, we clarify the computational procedures using MATLAB codes based on FEM with LM and CM matrices to calculate the eigenfrequencies and mode shapes of the beam subject to linear springs. Below is a detailed description of our approach:
(I) Development of global matrices
(1) The MATLAB code generates the global stiffness, and LM and CM matrices for the highest number of elements.
(2) Boundary conditions are incorporated after forming the global stiffness and mass matrices.
(II) Incorporating boundary conditions
(1) The stiffness of the linear spring attached to the left end is added to the first entry of the first row of the global stiffness matrix.
(2) Similarly, the stiffness of the spring at the right end is added to the second to last entry of the final row of the global stiffness matrix.
(III) Calculation of eigenvalues and eigenfrequencies
(1) Eigenvalues are calculated from the global stiffness and mass matrices according to Eq (3.18).
(2) Eigenfrequencies are then obtained by taking the square root of these eigenvalues.
This procedure follows the methods outlined in several studies, such as references [27,28,29,30,31].
This section aims to discuss the eigenmodes/mode shapes of the structural dynamics of the beam, which actually describes the deformation that the beam component would exhibit if it vibrates at the eigenfrequency. The deformation usually takes place through an excitation, which leads to the overall vibration of a component of the beam that includes the individual shapes of vibration. Therefore, eigenfrequencies and mode shapes indicate how the beam structure behaves under certain boundary conditions. It is noteworthy that the eigenmode characteristic is suitable for the qualitative evaluation of the dynamics of the beam component. The tabular and graphical analysis are presented to discuss beam dynamics at length.
Table 1 lists the comparison of eigenfrequencies determined by analytic method (AM), FEM by CM and LM matrices, receptively, with
$ K_{1}, K_{2} = 10000\ N/m. $ |
Modes | AM | FEM by CM | FEM by LM | Percentage error | |
For CM | For LM | ||||
$ N=5 $ | |||||
$ 1^{st} $ | $ 6.90724849 $ | 6.90796199 | 6.91434953 | 0.01032973 | 0.10280563 |
$ 2^{nd} $ | $ 26.09674381 $ | 26.13470717 | 26.42771700 | 0.14547164 | 1.26825473 |
$ 3^{rd} $ | $ 52.84510702 $ | 53.16067633 | 54.27922231 | 0.59715900 | 2.71380904 |
$ 4^{th} $ | $ 81.45836541 $ | 82.55087709 | 74.63946703 | 1.34119028 | 8.37102285 |
$ N=10 $ | |||||
$ 1^{st} $ | $ 6.90724849 $ | 6.90729342 | 6.90918512 | 0.00065048 | 0.02803765 |
$ 2^{nd} $ | $ 26.09674381 $ | 26.09915748 | 26.18963094 | 0.00924893 | 0.35593375 |
$ 3^{rd} $ | $ 52.84510702 $ | 52.86511702 | 53.29345172 | 0.03786538 | 0.84841289 |
$ 4^{th} $ | $ 81.45836541 $ | 81.53039633 | 80.56105229 | 0.08842667 | 1.10156043 |
$ N=50 $ | |||||
$ 1^{st} $ | $ 6.90724849 $ | 6.90724856 | 6.90732785 | 0.000000101 | 0.00114894 |
$ 2^{nd} $ | $ 26.09674382 $ | 26.09674771 | 26.10054215 | 0.00001487 | 0.01455480 |
$ 3^{rd} $ | $ 52.84510702 $ | 52.84513933 | 52.86350476 | 0.00006114 | 0.03481446 |
$ 4^{th} $ | $ 81.45836541 $ | 81.45848351 | 81.43321124 | 0.00014498 | 0.03087979 |
$ N=100 $ | |||||
$ 1^{st} $ | $ 6.90724849 $ | 6.90724851 | 6.90726831 | 0.00000029 | 0.00028694 |
$ 2^{nd} $ | $ 26.09674382 $ | 26.09674412 | 26.09769399 | 0.00000115 | 0.00028694 |
$ 3^{rd} $ | $ 52.84510702 $ | 52.84510912 | 52.84970909 | 0.00000397 | 0.00870860 |
$ 4^{th} $ | $ 81.45836541 $ | 81.45837285 | 81.45215478 | 0.00000913 | 0.00762930 |
The accuracy of eigenfrequencies are increased by increasing the number of elements of the beam. The percentage errors (with $ N $ = 5) for the CM matrix are 0.01032973, 0.14547164, 0.59715900, and 1.34119028, respectively.
While 0.10280563, 1.26825474, 2.71380904, and 8.37102285 are percentage errors for the LM matrix, this indicates that the CM matrix gives better accuracy for nonclassical boundary conditions as compared to the LM matrix even for the lower number of elements. Table 1 clearly demonstrates that CM gives excellent agreement at
$ N = 50, $ |
but does not get the same accuracy for LM even by using
$ N = 100. $ |
It is noteworthy that
$ N = 10 $ |
yields excellent accuracy; however,
$ N = 50 $ |
and
$ N = 100 $ |
are intended merely to demonstrate that increasing the number of elements improves accuracy.
Table 2 is a comparison of natural frequencies calculated by analytical and numerical methods, respectively, by decreasing the spring stiffness of the right end. The table shows that natural frequencies and stiffness of the right end spring are in a direct relationship, where natural frequencies decrease with decreasing spring stiffness. In addition, the numerical results are in close agreement with the analytical results when comparing the numerical solution for the CM matrices instead of the LM matrices. Rather, FEM by the consideration of CM matrices gives excellent accuracy not only for lower modes but also for higher modes.
Modes | AM | FEM by CM | FEM by LM | Percentage error | |
For CM | For LM | ||||
$ N=5 $ | |||||
$ 1^{st} $ | $ 6.35701924 $ | 6.35757819 | 6.38367463 | 0.00879264 | 0.41930642 |
$ 2^{nd} $ | $ 18.88435515 $ | 18.89870611 | 18.60125288 | 0.07599391 | 1.49913654 |
$ 3^{rd} $ | $ 37.23312923 $ | 37.33388399 | 34.14209661 | 0.27060513 | 8.30183410 |
$ 4^{th} $ | $ 66.98883703 $ | 67.54369195 | 61.04497484 | 0.82827967 | 8.87291440 |
$ N=10 $ | |||||
$ 1^{st} $ | $ 6.35701924 $ | 6.35705430 | 6.36418013 | 0.00055151 | 0.11264540 |
$ 2^{nd} $ | $ 18.88435515 $ | 18.88526930 | 18.82742317 | 0.00484077 | 0.30147696 |
$ 3^{rd} $ | $ 37.23312923 $ | 37.23999014 | 36.48814214 | 0.01842689 | 2.00087155 |
$ 4^{th} $ | $ 66.98883703 $ | 66.92997846 | 65.34527890 | 0.08786325 | 2.45348061 |
$ N=50 $ | |||||
$ 1^{st} $ | $ 6.35701924 $ | 6.35701929 | 6.35730648 | 0.00000078 | 0.00451846 |
$ 2^{nd} $ | $ 18.88435515 $ | 18.88435660 | 37.20428112 | 0.00000767 | 0.01115087 |
$ 3^{rd} $ | $ 37.23312923 $ | 37.23314046 | 37.20428112 | 0.00003016 | 0.07747968 |
$ 4^{th} $ | $ 66.98883703 $ | 66.98890259 | 66.92288123 | 0.00009786 | 0.09845789 |
$ N=100 $ | |||||
$ 1^{st} $ | $ 6.35701924 $ | 6.35701923 | 6.35709101 | 0.00000015 | 0.00112898 |
$ 2^{nd} $ | $ 18.88435515 $ | 18.88435528 | 18.88383000 | 0.00000068 | 0.00278087 |
$ 3^{rd} $ | $ 37.23312923 $ | 37.23312992 | 37.22592579 | 0.00000185 | 0.01934685 |
$ 4^{th} $ | $ 66.98883703 $ | 66.98884110 | 66.97235365 | 0.00000607 | 0.02460615 |
Table 3 indicates the comparison of eigenfrequencies by increasing the stiffness of the left end. From tables, it is observed easily by increasing the value of $ K_{1} $ that eigenfrequencies are also increased. A study has demonstrated that the CM matrices can give accurate eigenfrequencies for higher modes as well as lower ones, but LM cannot give the best accuracy for lower modes as well as for higher modes.
Modes | AM | FEM by CM | FEM by LM | Percentage error | |
For CM | For LM | ||||
$ N=5 $ | |||||
$ 1^{st} $ | $ 6.96985529 $ | 6.97058784 | 6.97318699 | 0.01051026 | 0.04780157 |
$ 2^{nd} $ | $ 27.03932973 $ | 27.08117845 | 27.20251475 | 0.15476981 | 0.60350986 |
$ 3^{rd} $ | $ 57.08313134 $ | 57.47001406 | 57.52811675 | 0.67775315 | 0.77953924 |
$ 4^{th} $ | $ 92.56612927 $ | 94.07004264 | 80.41180184 | 1.62469078 | 13.13042635 |
$ N=10 $ | |||||
$ 1^{st} $ | $ 6.96985529 $ | 6.96990142 | 6.97085023 | 0.00066185 | 0.01427757 |
$ 2^{nd} $ | $ 27.03932973 $ | 27.04200859 | 27.09268028 | 0.00990727 | 0.19730722 |
$ 3^{rd} $ | $ 57.08313134 $ | 57.10818680 | 57.34259598 | 0.04389293 | 0.45453827 |
$ 4^{th} $ | $ 92.56612927 $ | 92.67041635 | 90.82713601 | 0.11238909 | 1.87864964 |
$ N=50 $ | |||||
$ 1^{st} $ | $ 6.96985529 $ | 6.96985536 | 6.96989697 | 0.00000100 | 0.00059800 |
$ 2^{nd} $ | $ 27.03932973 $ | 27.03933403 | 27.04157469 | 0.00001590 | 0.00830257 |
$ 3^{rd} $ | $ 57.08313134 $ | 57.08317194 | 57.09446492 | 0.00007112 | 0.01985452 |
$ 4^{th} $ | $ 92.56612927 $ | 92.56630243 | 92.51496052 | 0.00018707 | 0.05527805 |
$ N=100 $ | |||||
$ 1^{st} $ | $ 6.96985529 $ | 6.96985538 | 6.96986568 | 0.00000129 | 0.00014907 |
$ 2^{nd} $ | $ 27.03932973 $ | 27.03932996 | 27.03989174 | 0.00000085 | 0.00207849 |
$ 3^{rd} $ | $ 57.08313134 $ | 57.08313385 | 57.08597067 | 0.00000440 | 0.00497403 |
$ 4^{th} $ | $ 92.56612927 $ | 92.56614015 | 92.55346771 | 0.00001175 | 0.01367839 |
Table 4 mentions the comparison of numerical and analytical results by increasing the spring stiffness of both ends and shows a similar behavior for natural frequencies and solution accuracy as in case of Tables 1–3.
Modes | AM | FEM by CM | FEM by LM | Percentage error | |
For CM | For LM | ||||
$ N=5 $ | |||||
$ 1^{st} $ | $ 7.00984547 $ | 7.01058818 | 7.01069925 | 0.01059524 | 0.01217972 |
$ 2^{nd} $ | $ 27.72533453 $ | 27.77009228 | 27.75837598 | 0.01614326 | 0.11917421 |
$ 3^{rd} $ | $ 61.16521834 $ | 61.63086692 | 61.09088070 | 0.76129635 | 0.12153580 |
$ 4^{th} $ | $ 105.4525306 $ | 107.82633768 | 101.05828707 | 2.25106688 | 4.16703475 |
$ N=10 $ | |||||
$ 1^{st} $ | $ 7.00984547 $ | 7.00989023 | 7.01218744 | 0.00063853 | 0.03340972 |
$ 2^{nd} $ | $ 27.72533453 $ | 27.72813738 | 27.74771968 | 0.01010934 | 0.08273897 |
$ 3^{rd} $ | $ 61.16521834 $ | 61.19584117 | 61.39079599 | 0.05006575 | 0.08073897 |
$ 4^{th} $ | $ 105.4525306 $ | 105.60832597 | 106.45143402 | 0.14773974 | 0.94725408 |
$ N=50 $ | |||||
$ 1^{st} $ | $ 7.00984547 $ | 7.00984337 | 7.00986020 | 0.00002995 | 0.00021013 |
$ 2^{nd} $ | $ 27.72533453 $ | 27.72529534 | 27.72631753 | 0.00014135 | 0.00354549 |
$ 3^{rd} $ | $ 61.16521834 $ | 61.16521665 | 61.17571474 | 0.00002763 | 0.01716073 |
$ 4^{th} $ | $ 105.4525306 $ | 105.45258028 | 105.49998929 | 0.00004703 | 0.04500470 |
$ N=100 $ | |||||
$ 1^{st} $ | $ 7.00984547 $ | 7.00984334 | 7.00984750 | 0.00003038 | 0.00002895 |
$ 2^{nd} $ | $ 27.72533453 $ | 27.72529099 | 27.72554833 | 0.00015704 | 0.00077113 |
$ 3^{rd} $ | $ 61.16521834 $ | 61.16516979 | 61.16781319 | 0.00007937 | 0.00424236 |
$ 4^{th} $ | $ 105.4525306 $ | 105.45234022 | 105.46427897 | 0.00018055 | 0.03111408 |
Figures 4–7 represent the first four natural modes for varying the spring stiffness of the underlying beam configuration. It is evident that the number of node points increases with each successive mode. The occurrence of node points indicates negligible deflection at certain locations of the beam. For higher natural modes, deflection becomes zero at multiple locations on the beam. Furthermore, the mode shapes clearly provide alternative symmetric and antisymmetric modes in case of even and odd modes, respectively. It is worth noting that the greatest amount of deflection occurs at the end of the beam because the spring beam system generally shows an initial deflection to obtain the equilibrium position. However, the spring no longer deflects after having static equilibrium.
The intent of this section is to demonstrate the validity of the results mentioned above. The underlying results for a few specific cases that have already been documented in the literature are rendered for this purpose. The simply supported EBB eigenfrequencies have been determined to be precisely comparable to those reported by Leissa [23] when
$ K_{1} = K_{2} = 10^{12} $ |
has been taken into account, as shown in Table 5. Furthermore, letting the stiffness parameters be
$ K_{1} = K_{2} = 0 $ |
Modes | [23] | FEM by CM | FEM by LM |
$ K_{1}=K_{2}=10^{12} $ | |||
$ 1^{st} $ | $ 9.8696 $ | 9.8696 | 9.8695 |
$ 2^{nd} $ | $ 39.478 $ | 39.4782 | 39.4737 |
$ 3^{rd} $ | $ 88.876 $ | 88.8769 | 88.7669 |
$ 4^{th} $ | $ 57.914 $ | 157.7529 | 157.5231 |
$ K_{1}=K_{2}=0 $ | |||
$ 1^{st} $ | $ 0.0000 $ | 0, 0000 | 0.0000 |
$ 2^{nd} $ | $ 0.000 $ | 0.0002 | 0.0041 |
$ 3^{rd} $ | $ 22.273 $ | 22.3740 | 21.7055 |
$ 4^{th} $ | $ 61.373 $ | 61.6881 | 58.6391 |
verifies the findings of the free free EBB [23].
In this article, the modal analysis of the Euler-Bernoulli beam subject to attached linear springs has been made. Eigenfrequencies and mode shapes were evaluated by comparing the analytic method and FEM using CM and LM matrices by varying the stiffness of the springs. It has been noted that more numbers of beam elements resulted in better accuracy of eigenfrequencies whereas consideration of the CM matrix showed excellent accuracy as compared to the LM matrix for $ N $ = 5 or $ N $ = 100. This has justified the preference of considering the CM matrix over the LM matrix even for a small number of beam elements for the greater advantage and better accuracy of the solution. Also, it has been observed that a large amount of deflection occurred at the end points of the beam which justified the dynamics of the spring beam system.
While dealing with more complex and practical problems of beam dynamics, obtaining analytical solutions often becomes challenging and sometimes impossible. It has been noticed that the use of FEM with the consideration of the LM matrix is rather convenient in addressing more challenging and practical problems related to beam dynamics. The underlying study concluded with the aim to provide an effective way to treat such problems numerically in a more efficient way. Furthermore, it is important to note that the suggested method can be easily extended to specific two-dimensional structures such as plates and shells with common boundary conditions, albeit with numerical concerns. However, it is critical to recognize that adding plates and shell-like structures may create complexities that are beyond the capability of typical numerical methods. In such circumstances, mode-matching algorithms have emerged as viable options for addressing complex structures; see, for reference, [32,33]. While mode-matching methods may neglect some effects, such as break-out, they provide useful insights into vibrating processes and serve as benchmark answers for fully numerical systems. Traditionally, numerical and analytical methods are used to describe finite-length plate or shell-like structures. Numerical methods, such as the finite element method or the boundary element method, allow for the analysis of structures of various shapes and sizes. However, as the excitation frequency and structure dimensions increase, so does the number of degrees of freedom, making these approaches unsuitable even for relatively small structures. As a result, in situations where typical numerical approaches are limited, mode-matching solutions provide a desirable and precisely computed option for handling the complexity inherent in modeling finite-length plate or shell-like structures.
M. Alkinidri: conceptualization, methodology, writing (review and editing), investigation, analysis, visualization, validation, resources; R. Nawaz: conceptualization, methodology, writing (original draft), investigation, analysis, visualization, validation, supervision; H. Alahmadi: methodology, writing (review and editing), investigation, analysis, visualization, validation, resources.
The authors declare they have not used Artificial Intelligence (AI) tools in the creation of this article.
The authors express their gratitude to Dr. Gulnaz Kanwal for her valuable suggestions, particularly regarding the computational aspects of the study, which significantly improved the quality of the article.
The authors declare no conflicts of interest.
[1] | Cowan N (1995) Attention and memory: An integrated framework. Oxford, England: Oxford University Press. |
[2] |
Jonides J, Lacey SC, Nee DE (2005) Processes of working memory in mind and brain. Curr Dir Psychol 14: 2-5. doi: 10.1111/j.0963-7214.2005.00323.x
![]() |
[3] |
Lewis-Peacock JA, Postle BR (2008) Temporary activation of long-term memory supports working memory. J Neurosci 28: 8765-8771. doi: 10.1523/JNEUROSCI.1953-08.2008
![]() |
[4] | Ruchkin DS, Grafman J, Cameron K, et al. (2003) Working memory retention systems: A state of activated long-term memory. Behav Brain Sci 26: 709-728. |
[5] |
Zimmer HD (2008) Visual and spatial working memory: from boxes to networks. Neurosci Biobehav Rev 32: 1373-1395. doi: 10.1016/j.neubiorev.2008.05.016
![]() |
[6] |
Cowan N (1984) On short and long auditory stores. Psychol Bull 96: 341-370. doi: 10.1037/0033-2909.96.2.341
![]() |
[7] |
Massaro DW (1975) Backward recognition masking. J Acoust Soc Am 58: 1059-1065. doi: 10.1121/1.380765
![]() |
[8] | Sperling G (1960) The information available in brief visual presentations. Psychol Monogr 74: 1-29. |
[9] |
Massaro DW (1972) Preperceptual images, processing time, and perceptual units in auditory perception. Psychol Rev 79: 124-145. doi: 10.1037/h0032264
![]() |
[10] |
Turvey MT (1973) On peripheral and central processes in vision: inferences from an information processing analysis of masking with patterned stimuli. Psychol Rev 80: 1-52. doi: 10.1037/h0033872
![]() |
[11] |
Vogel EK, Woodman GF, Luck SJ (2006) The time course of consolidation in visual working memory. J Exp Psychol-Hum Percept Perform 32: 1436-1451. doi: 10.1037/0096-1523.32.6.1436
![]() |
[12] |
Woodman GF, Vogel EK (2005) Fractionating working memory consolidation and maintenance are independent processes. Psychol Sci 16: 106-113. doi: 10.1111/j.0956-7976.2005.00790.x
![]() |
[13] |
Woodman GF, Vogel EK (2008) Selective storage and maintenance of an object's features in visual working memory. Psychon Bull Rev 15: 223-229. doi: 10.3758/PBR.15.1.223
![]() |
[14] |
Bradshaw GL, Anderson JR (1982) Elaborative encoding as an explanation of levels of processing. J Verb Learn Verb Beh 21: 165-174. 15. Craik FIM, Lockhart RS (1972) Levels of processing: A framework for memory research. J Verb Learn Verb Beh 11: 671-684. doi: 10.1016/S0022-5371(72)80001-X
![]() |
[15] | 16. Craik FI, Tulving E (1975) Depth of processing and the retention of words in episodic memory. J Exp Psychol Gen 104: 268-294. |
[16] | 17. Nieuwenstein M, Wyble B (2014) Beyond a mask and against the bottleneck: Retroactive dual-task interference during working memory consolidation of a masked visual target. J Exp Psychol Gen 143: 1409-1427. |
[17] | 18. Ricker TJ, Cowan N (2014) Differences in presentation methods in working memory procedures: A matter of working memory consolidation. J Exp Psychol Learn Mem Cogn 40: 4428. |
[18] |
19. Jolicoeur P, Dell'Acqua R (1998) The demonstration of short-term consolidation. Cogn Psychol 36: 138-202. doi: 10.1006/cogp.1998.0684
![]() |
[19] | 20. Baddeley AD (6) Working memory. New York, NY: Oxford University Press. |
[20] |
21. Barrouillet P, Camos V (2) As time goes by: Temporal constraints in working memory. Curr Dir Psychol 21: 413-419. doi: 10.1177/0963721412459513
![]() |
[21] |
22. Davelaar EJ, Goshen-Gottstein Y, Ashkenazi A, et al. (2005) The demise of short-term memory revisited: empirical and computational investigations of recency effects. Psychol Rev 112: 3-42. doi: 10.1037/0033-295X.112.1.3
![]() |
[22] |
23. Unsworth N, Engle RW (2007) The nature of individual differences in working memory capacity: Active maintenance in primary memory and controlled search from secondary memory. Psychol Rev 114: 104-132. doi: 10.1037/0033-295X.114.1.104
![]() |
[23] |
24. Brown GDA, Neath I, Chater N (2007) A temporal ratio model of memory. Psychol Rev 114: 539-576. doi: 10.1037/0033-295X.114.3.539
![]() |
[24] |
25. Farrell S (2012) Temporal clustering and sequencing in short-term memory and episodic memory. Psychol Rev 119: 223-271. doi: 10.1037/a0027371
![]() |
[25] |
26. Nairne JS (2002) Remembering over the short term: The case against the standard model. Annu Rev Psychol 53: 53-81. doi: 10.1146/annurev.psych.53.100901.135131
![]() |
[26] |
27. Tiitinen H, Reinikainen PMK, Näätänen R (1994) Attentive novelty detection in humans is governed by pre-attentive sensory memory. Nature 372: 90-92. doi: 10.1038/372090a0
![]() |
[27] | 28. Cowan N (1988) Evolving conceptions of memory storage, selective attention, and their mutual constraints within the human information processing system. Psychol Bull 104: 163-191. |
[28] |
29. Khader P, Burke M, Bien S, et al. (2005) Content-specific activation during associative long-term memory retrieval. Neuroimage 27: 805-816. doi: 10.1016/j.neuroimage.2005.05.006
![]() |
[29] |
30. Craik FI, Govoni R, Naveh-Benjamin M, et al. (1996) The effects of divided attention on encoding and retrieval processes in human memory. J Exp Psychol Gen 125: 159-180. doi: 10.1037/0096-3445.125.2.159
![]() |
[30] | 31. Naveh-Benjamin M, Craik FIM, Gavrilescu D, et al. (2000) Asymmetry between encoding and retrieval processes: Evidence from divided attention and a calibration analysis. Mem Cognit 28: 965-976. |
[31] | 32. Massaro DW (1975) Experimental psychology and information processing. Chicago, IL: Rand McNally. |
[32] |
33. Baddeley AD (2000) The episodic buffer: A new component of working memory? Trends Cogn Sci 4: 417-423. doi: 10.1016/S1364-6613(00)01538-2
![]() |
[33] | 34. Logie RH (2009) Working memory, In: Bayne T, Cleeremans T, Wilken P, The Oxford companion to consciousness, Oxford, UK: Oxford University Press, 667-670. |
[34] | 35. Massaro DW (1970) Perceptual processes and forgetting in memory tasks. Psychol Rev 77: 557-567. |
[35] | 36. Saults JS, Cowan N (2007) A central capacity limit to the simultaneous storage of visual and auditory arrays in working memory. J Exp Psychol Gen 136: 663-684. |
[36] |
37. Sligte IG, Scholte HS, Lamme VA (2008) Are there multiple visual short-term memory stores? PLoS One 3: e1699. doi: 10.1371/journal.pone.0001699
![]() |
[37] |
38. Pinto Y, Sligte IG, Shapiro KL, et al. (2013) Fragile visual short-term memory is an object-based and location-specific store. Psychon Bull Rev 20: 732-739. doi: 10.3758/s13423-013-0393-4
![]() |
[38] |
39. Cowan N, Ricker TJ, Clark KM, et al. (2015) Knowledge cannot explain the developmental growth of working memory capacity. Dev Sci 18: 132-145. doi: 10.1111/desc.12197
![]() |
[39] | 40. McKeown D, Mercer T (2012) Short-term forgetting without interference. J Exp Psychol Learn Mem Cogn 38: 1057-1068. |
[40] |
41. Morey CC, Bieler M (2013) Visual short-term memory always requires general attention. Psychon Bull Rev 20: 163-170. doi: 10.3758/s13423-012-0313-z
![]() |
[41] | 42. Ricker TJ, Cowan N (2010) Loss of visual working memory within seconds: The combined use of refreshable and non-refreshable features. J Exp Psychol Learn Mem Cogn 36: 1355-1368. |
[42] |
43. Ricker TJ, Spiegel LR, Cowan N (2014) Time-based loss in visual short-term memory is from trace decay, not temporal distinctiveness. J Exp Psychol Learn Mem Cogn 40: 1510-1523. doi: 10.1037/xlm0000018
![]() |
[43] |
44. Ricker TJ, Vergauwe E, Hinrichs GA, et al. (2015) No recovery of memory when cognitive load is decreased. J Exp Psychol Learn Mem Cogn 41: 872-880. doi: 10.1037/xlm0000084
![]() |
[44] |
45. Vergauwe E, Camos V, Barrouillet P (2014) The impact of storage on processing: How is information maintained in working memory? J Exp Psychol Learn Mem Cogn 40: 1072-1095. doi: 10.1037/a0035779
![]() |
[45] |
46. Woodman GF, Vogel EK, Luck SJ (2012) Flexibility in visual working memory: Accurate change detection in the face of irrelevant variations in position. Vis Cogn 20: 1-28. doi: 10.1080/13506285.2011.630694
![]() |
[46] |
47. Zhang W, Luck SJ (2009) Sudden death and gradual decay in visual working memory. Psychol Sci 20: 423-428. doi: 10.1111/j.1467-9280.2009.02322.x
![]() |
[47] |
48. Lewandowsky S, Geiger SM, Oberauer K (2008) Interference-based forgetting in verbal short-term memory. J Mem Lang 59: 200-222. doi: 10.1016/j.jml.2008.04.004
![]() |
[48] |
49. Oberauer K, Lewandowsky S, Farrell S, et al. (2012) Modeling working memory: An interference model of complex span. Psychon Bull Rev 19: 779-819. doi: 10.3758/s13423-012-0272-4
![]() |
[49] |
50. Barrouillet P, Bernardin S, Camos V (2004) Time constraints and resource sharing in adults' working memory spans. J Exp Psychol Gen 133: 83-100. doi: 10.1037/0096-3445.133.1.83
![]() |
[50] |
51. Vergauwe E, Barrouillet P, Camos V (2010) Do mental processes share a domain-general resource? Psychol Sci 21: 384-390. doi: 10.1177/0956797610361340
![]() |
[51] |
52. Oberauer K, Lewandowsky S (2011) Modeling working memory: a computational implementation of the Time-Based Resource-Sharing theory. Psychon Bull Rev 18: 10-45. doi: 10.3758/s13423-010-0020-6
![]() |
[52] |
53. Oberauer K, Lewandowsky S (2013) Evidence against decay in verbal working memory. J Exp Psychol Gen 142: 380-411. doi: 10.1037/a0029588
![]() |
[53] | 54. Crowder RG (1976) Principles of learning and memory. Hillsdale, NJ: Erlbaum. |
[54] | 55. Naveh-Benjamin M, Jonides J (1984) Maintenance rehearsal: A two component analysis. J Exp Psychol Learn Mem Cogn 10: 369-385. |
[55] |
56. Johnson MK, Reeder JA, Raye CL, et al. (2002) Second thoughts versus second looks: An age-related deficit in reflectively refreshing just-activated information. Psychol Sci 13: 64-67. doi: 10.1111/1467-9280.00411
![]() |
[56] |
57. Raye CL, Johnson MK, Mitchell KJ, Greene EJ, Johnson MR (2007) Refreshing: A minimal executive function. Cortex 43: 135-145. doi: 10.1016/S0010-9452(08)70451-9
![]() |
[57] | 58. Portrat S, Lemaire B (2015) Is attentional refreshing in working memory sequential? A computational modeling approach. Cognitive Computation 7: 333-345. |
[58] | 59. Vergauwe E, Cowan N (2014) A common short-term memory retrieval rate may describe many cognitive procedures. Front Hum Neurosci 8. |
[59] |
60. Bayliss DM, Bogdanovs J, Jarrold C (2015) Consolidating working memory: Distinguishing the effects of consolidation, rehearsal and attentional refreshing in a working memory span task. J Mem Lang 81: 34-50. doi: 10.1016/j.jml.2014.12.004
![]() |
[60] |
61. Stevanovski B, Jolicoeur P (2007) Visual short-term memory: Central capacity limitations in short-term consolidation. Vis Cogn 15: 532-563. doi: 10.1080/13506280600871917
![]() |
[61] |
62. Eichenbaum H (2000) A cortical–hippocampal system for declarative memory. Nat Rev Neurosci 1: 41-50. doi: 10.1038/35036213
![]() |
[62] |
63. Remondes M, Schuman EM (2004) Role for a cortical input to hippocampal area CA1 in the consolidation of a long-term memory. Nature 431: 699-703. doi: 10.1038/nature02965
![]() |
[63] | 64. Squire LR, Alvarez P (1995) Retrograde amnesia and memory consolidation: A neurobiological perspective. Curr Opin Neurobiol 5: 169-177. |
[64] |
65. Bliss TV, Collingridge GL (1993) A synaptic model of memory: Long-term potentiation in the hippocampus. Nature 361: 31-39. doi: 10.1038/361031a0
![]() |
[65] |
66. Teyler TJ, DiScenna P (1987) Long-term potentiation. Annu Rev Neurosci 10: 131-161. doi: 10.1146/annurev.ne.10.030187.001023
![]() |
[66] | 67. Hampson RE, Deadwyler SA (2000) Cannabinoids reveal the necessity of hippocampal neural encoding for short-term memory in rats. J Neurosci 20: 8932-8942. |
[67] |
68. Mitchell KJ, Johnson MK, Raye CL, et al. (2000) fMRI evidence of age-related hippocampal dysfunction in feature binding in working memory. Cogn Brain Res 10: 197-206. doi: 10.1016/S0926-6410(00)00029-X
![]() |
[68] |
69. Nichols EA, KaoYC, Verfaellie M, et al. (2006) Working memory and long‐term memory for faces: Evidence from fMRI and global amnesia for involvement of the medial temporal lobes. Hippocampus 16: 604-616. doi: 10.1002/hipo.20190
![]() |
[69] |
70. Vertes RP (2005) Hippocampal theta rhythm: A tag for short‐term memory. Hippocampus 15: 923-935. doi: 10.1002/hipo.20118
![]() |
[70] |
71. Cowan N, Li D, Moffitt A, Becker TM, et al. (2011) A neural region of abstract working memory. J Cogn Neurosci 23: 2852-2863. doi: 10.1162/jocn.2011.21625
![]() |
[71] |
72. Emrich SM, Riggall AC, LaRocque JJ, et al. (2013) Distributed patterns of activity in sensory cortex reflect the precision of multiple items maintained in visual short-term memory. J Neurosci 33: 6516-6523. doi: 10.1523/JNEUROSCI.5732-12.2013
![]() |
[72] | 73. Xu Y, Chun MM (2006) Dissociable neural mechanisms supporting visual short-term memory for objects. Nature 440: 91-95. |
[73] | 74. Todd JJ, Marois R (2004) Capacity limit of visual short-term memory in human posterior parietal cortex. Nature 428: 751-754. |
[74] | 75. Crick F, Koch C (1990) Toward a neurobiological theory of consciousness. Semin Neurosci 2: 263-275. |
[75] |
76. Engel AK, Singer W (2001) Temporal binding and the neural correlates of sensory awareness. Trends Cogn Sci 5: 16-25. doi: 10.1016/S1364-6613(00)01568-0
![]() |
[76] | 77. Lisman JE, Idiart MA (1995) Storage of 7+/-2 short-term memories in oscillatory subcycles. Science 267: 1512-1515. |
[77] |
78. Lisman JE, Jensen O (2013) The theta-gamma neural code. Neuron 1002-1016. doi: 10.1016/j.neuron.2013.03.007
![]() |
[78] |
79. Shipstead Z, Lindsey DR, Marshall RL, et al. (2014) The mechanisms of working memory capacity: Primary memory, secondary memory, and attention control. J Mem Lang 72: 116-141. doi: 10.1016/j.jml.2014.01.004
![]() |
1. | Maimoona Karim, Aliya Fahmi, Zafar Ullah, Muhammad Awais Tariq Bhatti, Ather Qayyum, On certain Ostrowski type integral inequalities for convex function via AB-fractional integral operator, 2023, 8, 2473-6988, 9166, 10.3934/math.2023459 | |
2. | Hasan Bayram, Sibel Yalçın, Jia-Bao Liu, Convolution and Coefficient Estimates for (p,q)-Convex Harmonic Functions Associated with Subordination, 2022, 2022, 2314-8888, 1, 10.1155/2022/5317797 | |
3. | Soubhagya Kumar Sahoo, Y.S. Hamed, Pshtiwan Othman Mohammed, Bibhakar Kodamasingh, Kamsing Nonlaopon, New midpoint type Hermite-Hadamard-Mercer inequalities pertaining to Caputo-Fabrizio fractional operators, 2023, 65, 11100168, 689, 10.1016/j.aej.2022.10.019 | |
4. | Soubhagya Kumar Sahoo, Ravi P. Agarwal, Pshtiwan Othman Mohammed, Bibhakar Kodamasingh, Kamsing Nonlaopon, Khadijah M. Abualnaja, Hadamard–Mercer, Dragomir–Agarwal–Mercer, and Pachpatte–Mercer Type Fractional Inclusions for Convex Functions with an Exponential Kernel and Their Applications, 2022, 14, 2073-8994, 836, 10.3390/sym14040836 | |
5. | Miguel Vivas-Cortez, Muhammad Uzair Awan, Sehrish Rafique, Muhammad Zakria Javed, Artion Kashuri, Some novel inequalities involving Atangana-Baleanu fractional integral operators and applications, 2022, 7, 2473-6988, 12203, 10.3934/math.2022678 | |
6. | Mirna Rodić, On the Converse Jensen-Type Inequality for Generalized f-Divergences and Zipf–Mandelbrot Law, 2022, 10, 2227-7390, 947, 10.3390/math10060947 | |
7. | Bandar Bin-Mohsin, Sehrish Rafique, Clemente Cesarano, Muhammad Zakria Javed, Muhammad Uzair Awan, Artion Kashuri, Muhammad Aslam Noor, Some General Fractional Integral Inequalities Involving LR–Bi-Convex Fuzzy Interval-Valued Functions, 2022, 6, 2504-3110, 565, 10.3390/fractalfract6100565 | |
8. | Saad Ihsan Butt, Iram Javed, Praveen Agarwal, Juan J. Nieto, Newton–Simpson-type inequalities via majorization, 2023, 2023, 1029-242X, 10.1186/s13660-023-02918-0 | |
9. | Fahd Jarad, Soubhagya Kumar Sahoo, Kottakkaran Sooppy Nisar, Savin Treanţă, Homan Emadifar, Thongchai Botmart, New stochastic fractional integral and related inequalities of Jensen–Mercer and Hermite–Hadamard–Mercer type for convex stochastic processes, 2023, 2023, 1029-242X, 10.1186/s13660-023-02944-y | |
10. | Jun Yang, Muhammad Kamran Siddiqui, Mazhar Hussain, Shazia Manzoor, Nazir Hussain, Zohaib Saddique, QSPR Analysis of Some Phenolic Compounds Present in Moringa Oleifera , 2024, 44, 1040-6638, 4197, 10.1080/10406638.2023.2247120 | |
11. | XIAOMAN YUAN, LEI XU, TINGSONG DU, SIMPSON-LIKE INEQUALITIES FOR TWICE DIFFERENTIABLE (s,P)-CONVEX MAPPINGS INVOLVING WITH AB-FRACTIONAL INTEGRALS AND THEIR APPLICATIONS, 2023, 31, 0218-348X, 10.1142/S0218348X2350024X | |
12. | Asfand Fahad, Saad Ihsaan Butt, Josip Pečarić, Marjan Praljak, Generalized Taylor’s Formula and Steffensen’s Inequality, 2023, 11, 2227-7390, 3570, 10.3390/math11163570 | |
13. | Mulugeta Dawud Ali, D. L. Suthar, On the fractional q-integral operators involving q-analogue of Mittag-Leffler function, 2024, 44, 0174-4747, 245, 10.1515/anly-2023-0107 | |
14. | Saad Ihsan Butt, Praveen Agarwal, Juan J. Nieto, New Hadamard–Mercer Inequalities Pertaining Atangana–Baleanu Operator in Katugampola Sense with Applications, 2024, 21, 1660-5446, 10.1007/s00009-023-02547-3 | |
15. | WENBING SUN, HAIYANG WAN, HERMITE–HADAMARD-TYPE INEQUALITIES INVOLVING SEVERAL KINDS OF FRACTIONAL CALCULUS FOR HARMONICALLY CONVEX FUNCTIONS, 2023, 31, 0218-348X, 10.1142/S0218348X23501098 | |
16. | Saad Ihsan Butt, Yamin Sayyari, Praveen Agarwal, Juan J. Nieto, Muhammad Umar, On some inequalities for uniformly convex mapping with estimations to normal distributions, 2023, 2023, 1029-242X, 10.1186/s13660-023-02997-z | |
17. | Muhammad Nadeem, Awais Yousaf, Seemab Sheik, A Novel Approach to Investigate Sadhana and PI Indices, 2024, 44, 1040-6638, 5060, 10.1080/10406638.2023.2259566 | |
18. | Muhammad Tariq, Sotiris K. Ntouyas, Bashir Ahmad, Ostrowski-Type Fractional Integral Inequalities: A Survey, 2023, 3, 2673-9321, 660, 10.3390/foundations3040040 | |
19. | Miguel Vivas–Cortez, Muhammad Zakria Javed, Muhammad Uzair Awan, Muhammad Aslam Noor, Silvestru Sever Dragomir, Bullen-Mercer type inequalities with applications in numerical analysis, 2024, 96, 11100168, 15, 10.1016/j.aej.2024.03.093 | |
20. | Ammara Nosheen, Sana Ijaz, Khuram Ali Khan, Khalid Mahmood Awan, Hüseyin Budak, Quantum symmetric integral inequalities for convex functions, 2024, 47, 0170-4214, 14878, 10.1002/mma.10310 | |
21. | Erhan Set, Ahmet Akdemir, Emin Özdemir, Ali Karaoğlan, Mustafa Dokuyucu, New integral inequalities for Atangana-Baleanu fractional integral operators and various comparisons via simulations, 2023, 37, 0354-5180, 2251, 10.2298/FIL2307251S | |
22. | Timur Gamilov, Ruslan Yanbarisov, Fractional-Order Windkessel Boundary Conditions in a One-Dimensional Blood Flow Model for Fractional Flow Reserve (FFR) Estimation, 2023, 7, 2504-3110, 373, 10.3390/fractalfract7050373 | |
23. | Muhammad Mudassar Hassan, Shamoona Jabeen, Haidar Ali, Parvez Ali, Connection-based modified Zagreb indices of Boron triangular sheet BTS(m,n), 2023, 0026-8976, 10.1080/00268976.2023.2226242 | |
24. | Asfand Fahad, Saad Ihsaan Butt, Bahtiyar Bayraktar, Mehran Anwar, Yuanheng Wang, Some New Bullen-Type Inequalities Obtained via Fractional Integral Operators, 2023, 12, 2075-1680, 691, 10.3390/axioms12070691 | |
25. | Tahir Rasheed, Saad Ihsan Butt, Ðilda Pečarić, Josip Pečarić, New bounds of Popoviciu's difference via weighted Hadamard type inequalities with applications in information theory, 2024, 47, 0170-4214, 5750, 10.1002/mma.9889 | |
26. | Shanhe Wu, Muhammad Adil Khan, Shah Faisal, Tareq Saeed, Eze R. Nwaeze, Derivation of Hermite-Hadamard-Jensen-Mercer conticrete inequalities for Atangana-Baleanu fractional integrals by means of majorization, 2024, 57, 2391-4661, 10.1515/dema-2024-0024 | |
27. | Zeynep Çiftci, Merve Coşkun, Çetin Yildiz, Luminiţa-Ioana Cotîrlă, Daniel Breaz, On New Generalized Hermite–Hadamard–Mercer-Type Inequalities for Raina Functions, 2024, 8, 2504-3110, 472, 10.3390/fractalfract8080472 | |
28. | Muhammad Amer Latif, New extensions related to Fejér-type inequalities for GA-convex functions, 2024, 57, 2391-4661, 10.1515/dema-2024-0006 | |
29. | PANUMART SAWANGTONG, K. LOGESWARI, C. RAVICHANDRAN, KOTTAKKARAN SOOPPY NISAR, V. VIJAYARAJ, FRACTIONAL ORDER GEMINIVIRUS IMPRESSION IN CAPSICUM ANNUUM MODEL WITH MITTAG-LEFFLER KERNAL, 2023, 31, 0218-348X, 10.1142/S0218348X23400492 | |
30. | Barış Çelik, Hüseyin Budak, Erhan Set, On generalized Milne type inequalities for new conformable fractional integrals, 2024, 38, 0354-5180, 1807, 10.2298/FIL2405807C | |
31. | Çetin Yıldız, Samet Erden, Seth Kermausuor, Daniel Breaz, Luminiţa-Ioana Cotîrlă, New estimates on generalized Hermite–Hadamard–Mercer-type inequalities, 2025, 2025, 1687-2770, 10.1186/s13661-025-02012-y |
Modes | AM | FEM by CM | FEM by LM | Percentage error | |
For CM | For LM | ||||
$ N=5 $ | |||||
$ 1^{st} $ | $ 6.90724849 $ | 6.90796199 | 6.91434953 | 0.01032973 | 0.10280563 |
$ 2^{nd} $ | $ 26.09674381 $ | 26.13470717 | 26.42771700 | 0.14547164 | 1.26825473 |
$ 3^{rd} $ | $ 52.84510702 $ | 53.16067633 | 54.27922231 | 0.59715900 | 2.71380904 |
$ 4^{th} $ | $ 81.45836541 $ | 82.55087709 | 74.63946703 | 1.34119028 | 8.37102285 |
$ N=10 $ | |||||
$ 1^{st} $ | $ 6.90724849 $ | 6.90729342 | 6.90918512 | 0.00065048 | 0.02803765 |
$ 2^{nd} $ | $ 26.09674381 $ | 26.09915748 | 26.18963094 | 0.00924893 | 0.35593375 |
$ 3^{rd} $ | $ 52.84510702 $ | 52.86511702 | 53.29345172 | 0.03786538 | 0.84841289 |
$ 4^{th} $ | $ 81.45836541 $ | 81.53039633 | 80.56105229 | 0.08842667 | 1.10156043 |
$ N=50 $ | |||||
$ 1^{st} $ | $ 6.90724849 $ | 6.90724856 | 6.90732785 | 0.000000101 | 0.00114894 |
$ 2^{nd} $ | $ 26.09674382 $ | 26.09674771 | 26.10054215 | 0.00001487 | 0.01455480 |
$ 3^{rd} $ | $ 52.84510702 $ | 52.84513933 | 52.86350476 | 0.00006114 | 0.03481446 |
$ 4^{th} $ | $ 81.45836541 $ | 81.45848351 | 81.43321124 | 0.00014498 | 0.03087979 |
$ N=100 $ | |||||
$ 1^{st} $ | $ 6.90724849 $ | 6.90724851 | 6.90726831 | 0.00000029 | 0.00028694 |
$ 2^{nd} $ | $ 26.09674382 $ | 26.09674412 | 26.09769399 | 0.00000115 | 0.00028694 |
$ 3^{rd} $ | $ 52.84510702 $ | 52.84510912 | 52.84970909 | 0.00000397 | 0.00870860 |
$ 4^{th} $ | $ 81.45836541 $ | 81.45837285 | 81.45215478 | 0.00000913 | 0.00762930 |
Modes | AM | FEM by CM | FEM by LM | Percentage error | |
For CM | For LM | ||||
$ N=5 $ | |||||
$ 1^{st} $ | $ 6.35701924 $ | 6.35757819 | 6.38367463 | 0.00879264 | 0.41930642 |
$ 2^{nd} $ | $ 18.88435515 $ | 18.89870611 | 18.60125288 | 0.07599391 | 1.49913654 |
$ 3^{rd} $ | $ 37.23312923 $ | 37.33388399 | 34.14209661 | 0.27060513 | 8.30183410 |
$ 4^{th} $ | $ 66.98883703 $ | 67.54369195 | 61.04497484 | 0.82827967 | 8.87291440 |
$ N=10 $ | |||||
$ 1^{st} $ | $ 6.35701924 $ | 6.35705430 | 6.36418013 | 0.00055151 | 0.11264540 |
$ 2^{nd} $ | $ 18.88435515 $ | 18.88526930 | 18.82742317 | 0.00484077 | 0.30147696 |
$ 3^{rd} $ | $ 37.23312923 $ | 37.23999014 | 36.48814214 | 0.01842689 | 2.00087155 |
$ 4^{th} $ | $ 66.98883703 $ | 66.92997846 | 65.34527890 | 0.08786325 | 2.45348061 |
$ N=50 $ | |||||
$ 1^{st} $ | $ 6.35701924 $ | 6.35701929 | 6.35730648 | 0.00000078 | 0.00451846 |
$ 2^{nd} $ | $ 18.88435515 $ | 18.88435660 | 37.20428112 | 0.00000767 | 0.01115087 |
$ 3^{rd} $ | $ 37.23312923 $ | 37.23314046 | 37.20428112 | 0.00003016 | 0.07747968 |
$ 4^{th} $ | $ 66.98883703 $ | 66.98890259 | 66.92288123 | 0.00009786 | 0.09845789 |
$ N=100 $ | |||||
$ 1^{st} $ | $ 6.35701924 $ | 6.35701923 | 6.35709101 | 0.00000015 | 0.00112898 |
$ 2^{nd} $ | $ 18.88435515 $ | 18.88435528 | 18.88383000 | 0.00000068 | 0.00278087 |
$ 3^{rd} $ | $ 37.23312923 $ | 37.23312992 | 37.22592579 | 0.00000185 | 0.01934685 |
$ 4^{th} $ | $ 66.98883703 $ | 66.98884110 | 66.97235365 | 0.00000607 | 0.02460615 |
Modes | AM | FEM by CM | FEM by LM | Percentage error | |
For CM | For LM | ||||
$ N=5 $ | |||||
$ 1^{st} $ | $ 6.96985529 $ | 6.97058784 | 6.97318699 | 0.01051026 | 0.04780157 |
$ 2^{nd} $ | $ 27.03932973 $ | 27.08117845 | 27.20251475 | 0.15476981 | 0.60350986 |
$ 3^{rd} $ | $ 57.08313134 $ | 57.47001406 | 57.52811675 | 0.67775315 | 0.77953924 |
$ 4^{th} $ | $ 92.56612927 $ | 94.07004264 | 80.41180184 | 1.62469078 | 13.13042635 |
$ N=10 $ | |||||
$ 1^{st} $ | $ 6.96985529 $ | 6.96990142 | 6.97085023 | 0.00066185 | 0.01427757 |
$ 2^{nd} $ | $ 27.03932973 $ | 27.04200859 | 27.09268028 | 0.00990727 | 0.19730722 |
$ 3^{rd} $ | $ 57.08313134 $ | 57.10818680 | 57.34259598 | 0.04389293 | 0.45453827 |
$ 4^{th} $ | $ 92.56612927 $ | 92.67041635 | 90.82713601 | 0.11238909 | 1.87864964 |
$ N=50 $ | |||||
$ 1^{st} $ | $ 6.96985529 $ | 6.96985536 | 6.96989697 | 0.00000100 | 0.00059800 |
$ 2^{nd} $ | $ 27.03932973 $ | 27.03933403 | 27.04157469 | 0.00001590 | 0.00830257 |
$ 3^{rd} $ | $ 57.08313134 $ | 57.08317194 | 57.09446492 | 0.00007112 | 0.01985452 |
$ 4^{th} $ | $ 92.56612927 $ | 92.56630243 | 92.51496052 | 0.00018707 | 0.05527805 |
$ N=100 $ | |||||
$ 1^{st} $ | $ 6.96985529 $ | 6.96985538 | 6.96986568 | 0.00000129 | 0.00014907 |
$ 2^{nd} $ | $ 27.03932973 $ | 27.03932996 | 27.03989174 | 0.00000085 | 0.00207849 |
$ 3^{rd} $ | $ 57.08313134 $ | 57.08313385 | 57.08597067 | 0.00000440 | 0.00497403 |
$ 4^{th} $ | $ 92.56612927 $ | 92.56614015 | 92.55346771 | 0.00001175 | 0.01367839 |
Modes | AM | FEM by CM | FEM by LM | Percentage error | |
For CM | For LM | ||||
$ N=5 $ | |||||
$ 1^{st} $ | $ 7.00984547 $ | 7.01058818 | 7.01069925 | 0.01059524 | 0.01217972 |
$ 2^{nd} $ | $ 27.72533453 $ | 27.77009228 | 27.75837598 | 0.01614326 | 0.11917421 |
$ 3^{rd} $ | $ 61.16521834 $ | 61.63086692 | 61.09088070 | 0.76129635 | 0.12153580 |
$ 4^{th} $ | $ 105.4525306 $ | 107.82633768 | 101.05828707 | 2.25106688 | 4.16703475 |
$ N=10 $ | |||||
$ 1^{st} $ | $ 7.00984547 $ | 7.00989023 | 7.01218744 | 0.00063853 | 0.03340972 |
$ 2^{nd} $ | $ 27.72533453 $ | 27.72813738 | 27.74771968 | 0.01010934 | 0.08273897 |
$ 3^{rd} $ | $ 61.16521834 $ | 61.19584117 | 61.39079599 | 0.05006575 | 0.08073897 |
$ 4^{th} $ | $ 105.4525306 $ | 105.60832597 | 106.45143402 | 0.14773974 | 0.94725408 |
$ N=50 $ | |||||
$ 1^{st} $ | $ 7.00984547 $ | 7.00984337 | 7.00986020 | 0.00002995 | 0.00021013 |
$ 2^{nd} $ | $ 27.72533453 $ | 27.72529534 | 27.72631753 | 0.00014135 | 0.00354549 |
$ 3^{rd} $ | $ 61.16521834 $ | 61.16521665 | 61.17571474 | 0.00002763 | 0.01716073 |
$ 4^{th} $ | $ 105.4525306 $ | 105.45258028 | 105.49998929 | 0.00004703 | 0.04500470 |
$ N=100 $ | |||||
$ 1^{st} $ | $ 7.00984547 $ | 7.00984334 | 7.00984750 | 0.00003038 | 0.00002895 |
$ 2^{nd} $ | $ 27.72533453 $ | 27.72529099 | 27.72554833 | 0.00015704 | 0.00077113 |
$ 3^{rd} $ | $ 61.16521834 $ | 61.16516979 | 61.16781319 | 0.00007937 | 0.00424236 |
$ 4^{th} $ | $ 105.4525306 $ | 105.45234022 | 105.46427897 | 0.00018055 | 0.03111408 |
Modes | [23] | FEM by CM | FEM by LM |
$ K_{1}=K_{2}=10^{12} $ | |||
$ 1^{st} $ | $ 9.8696 $ | 9.8696 | 9.8695 |
$ 2^{nd} $ | $ 39.478 $ | 39.4782 | 39.4737 |
$ 3^{rd} $ | $ 88.876 $ | 88.8769 | 88.7669 |
$ 4^{th} $ | $ 57.914 $ | 157.7529 | 157.5231 |
$ K_{1}=K_{2}=0 $ | |||
$ 1^{st} $ | $ 0.0000 $ | 0, 0000 | 0.0000 |
$ 2^{nd} $ | $ 0.000 $ | 0.0002 | 0.0041 |
$ 3^{rd} $ | $ 22.273 $ | 22.3740 | 21.7055 |
$ 4^{th} $ | $ 61.373 $ | 61.6881 | 58.6391 |
Modes | AM | FEM by CM | FEM by LM | Percentage error | |
For CM | For LM | ||||
$ N=5 $ | |||||
$ 1^{st} $ | $ 6.90724849 $ | 6.90796199 | 6.91434953 | 0.01032973 | 0.10280563 |
$ 2^{nd} $ | $ 26.09674381 $ | 26.13470717 | 26.42771700 | 0.14547164 | 1.26825473 |
$ 3^{rd} $ | $ 52.84510702 $ | 53.16067633 | 54.27922231 | 0.59715900 | 2.71380904 |
$ 4^{th} $ | $ 81.45836541 $ | 82.55087709 | 74.63946703 | 1.34119028 | 8.37102285 |
$ N=10 $ | |||||
$ 1^{st} $ | $ 6.90724849 $ | 6.90729342 | 6.90918512 | 0.00065048 | 0.02803765 |
$ 2^{nd} $ | $ 26.09674381 $ | 26.09915748 | 26.18963094 | 0.00924893 | 0.35593375 |
$ 3^{rd} $ | $ 52.84510702 $ | 52.86511702 | 53.29345172 | 0.03786538 | 0.84841289 |
$ 4^{th} $ | $ 81.45836541 $ | 81.53039633 | 80.56105229 | 0.08842667 | 1.10156043 |
$ N=50 $ | |||||
$ 1^{st} $ | $ 6.90724849 $ | 6.90724856 | 6.90732785 | 0.000000101 | 0.00114894 |
$ 2^{nd} $ | $ 26.09674382 $ | 26.09674771 | 26.10054215 | 0.00001487 | 0.01455480 |
$ 3^{rd} $ | $ 52.84510702 $ | 52.84513933 | 52.86350476 | 0.00006114 | 0.03481446 |
$ 4^{th} $ | $ 81.45836541 $ | 81.45848351 | 81.43321124 | 0.00014498 | 0.03087979 |
$ N=100 $ | |||||
$ 1^{st} $ | $ 6.90724849 $ | 6.90724851 | 6.90726831 | 0.00000029 | 0.00028694 |
$ 2^{nd} $ | $ 26.09674382 $ | 26.09674412 | 26.09769399 | 0.00000115 | 0.00028694 |
$ 3^{rd} $ | $ 52.84510702 $ | 52.84510912 | 52.84970909 | 0.00000397 | 0.00870860 |
$ 4^{th} $ | $ 81.45836541 $ | 81.45837285 | 81.45215478 | 0.00000913 | 0.00762930 |
Modes | AM | FEM by CM | FEM by LM | Percentage error | |
For CM | For LM | ||||
$ N=5 $ | |||||
$ 1^{st} $ | $ 6.35701924 $ | 6.35757819 | 6.38367463 | 0.00879264 | 0.41930642 |
$ 2^{nd} $ | $ 18.88435515 $ | 18.89870611 | 18.60125288 | 0.07599391 | 1.49913654 |
$ 3^{rd} $ | $ 37.23312923 $ | 37.33388399 | 34.14209661 | 0.27060513 | 8.30183410 |
$ 4^{th} $ | $ 66.98883703 $ | 67.54369195 | 61.04497484 | 0.82827967 | 8.87291440 |
$ N=10 $ | |||||
$ 1^{st} $ | $ 6.35701924 $ | 6.35705430 | 6.36418013 | 0.00055151 | 0.11264540 |
$ 2^{nd} $ | $ 18.88435515 $ | 18.88526930 | 18.82742317 | 0.00484077 | 0.30147696 |
$ 3^{rd} $ | $ 37.23312923 $ | 37.23999014 | 36.48814214 | 0.01842689 | 2.00087155 |
$ 4^{th} $ | $ 66.98883703 $ | 66.92997846 | 65.34527890 | 0.08786325 | 2.45348061 |
$ N=50 $ | |||||
$ 1^{st} $ | $ 6.35701924 $ | 6.35701929 | 6.35730648 | 0.00000078 | 0.00451846 |
$ 2^{nd} $ | $ 18.88435515 $ | 18.88435660 | 37.20428112 | 0.00000767 | 0.01115087 |
$ 3^{rd} $ | $ 37.23312923 $ | 37.23314046 | 37.20428112 | 0.00003016 | 0.07747968 |
$ 4^{th} $ | $ 66.98883703 $ | 66.98890259 | 66.92288123 | 0.00009786 | 0.09845789 |
$ N=100 $ | |||||
$ 1^{st} $ | $ 6.35701924 $ | 6.35701923 | 6.35709101 | 0.00000015 | 0.00112898 |
$ 2^{nd} $ | $ 18.88435515 $ | 18.88435528 | 18.88383000 | 0.00000068 | 0.00278087 |
$ 3^{rd} $ | $ 37.23312923 $ | 37.23312992 | 37.22592579 | 0.00000185 | 0.01934685 |
$ 4^{th} $ | $ 66.98883703 $ | 66.98884110 | 66.97235365 | 0.00000607 | 0.02460615 |
Modes | AM | FEM by CM | FEM by LM | Percentage error | |
For CM | For LM | ||||
$ N=5 $ | |||||
$ 1^{st} $ | $ 6.96985529 $ | 6.97058784 | 6.97318699 | 0.01051026 | 0.04780157 |
$ 2^{nd} $ | $ 27.03932973 $ | 27.08117845 | 27.20251475 | 0.15476981 | 0.60350986 |
$ 3^{rd} $ | $ 57.08313134 $ | 57.47001406 | 57.52811675 | 0.67775315 | 0.77953924 |
$ 4^{th} $ | $ 92.56612927 $ | 94.07004264 | 80.41180184 | 1.62469078 | 13.13042635 |
$ N=10 $ | |||||
$ 1^{st} $ | $ 6.96985529 $ | 6.96990142 | 6.97085023 | 0.00066185 | 0.01427757 |
$ 2^{nd} $ | $ 27.03932973 $ | 27.04200859 | 27.09268028 | 0.00990727 | 0.19730722 |
$ 3^{rd} $ | $ 57.08313134 $ | 57.10818680 | 57.34259598 | 0.04389293 | 0.45453827 |
$ 4^{th} $ | $ 92.56612927 $ | 92.67041635 | 90.82713601 | 0.11238909 | 1.87864964 |
$ N=50 $ | |||||
$ 1^{st} $ | $ 6.96985529 $ | 6.96985536 | 6.96989697 | 0.00000100 | 0.00059800 |
$ 2^{nd} $ | $ 27.03932973 $ | 27.03933403 | 27.04157469 | 0.00001590 | 0.00830257 |
$ 3^{rd} $ | $ 57.08313134 $ | 57.08317194 | 57.09446492 | 0.00007112 | 0.01985452 |
$ 4^{th} $ | $ 92.56612927 $ | 92.56630243 | 92.51496052 | 0.00018707 | 0.05527805 |
$ N=100 $ | |||||
$ 1^{st} $ | $ 6.96985529 $ | 6.96985538 | 6.96986568 | 0.00000129 | 0.00014907 |
$ 2^{nd} $ | $ 27.03932973 $ | 27.03932996 | 27.03989174 | 0.00000085 | 0.00207849 |
$ 3^{rd} $ | $ 57.08313134 $ | 57.08313385 | 57.08597067 | 0.00000440 | 0.00497403 |
$ 4^{th} $ | $ 92.56612927 $ | 92.56614015 | 92.55346771 | 0.00001175 | 0.01367839 |
Modes | AM | FEM by CM | FEM by LM | Percentage error | |
For CM | For LM | ||||
$ N=5 $ | |||||
$ 1^{st} $ | $ 7.00984547 $ | 7.01058818 | 7.01069925 | 0.01059524 | 0.01217972 |
$ 2^{nd} $ | $ 27.72533453 $ | 27.77009228 | 27.75837598 | 0.01614326 | 0.11917421 |
$ 3^{rd} $ | $ 61.16521834 $ | 61.63086692 | 61.09088070 | 0.76129635 | 0.12153580 |
$ 4^{th} $ | $ 105.4525306 $ | 107.82633768 | 101.05828707 | 2.25106688 | 4.16703475 |
$ N=10 $ | |||||
$ 1^{st} $ | $ 7.00984547 $ | 7.00989023 | 7.01218744 | 0.00063853 | 0.03340972 |
$ 2^{nd} $ | $ 27.72533453 $ | 27.72813738 | 27.74771968 | 0.01010934 | 0.08273897 |
$ 3^{rd} $ | $ 61.16521834 $ | 61.19584117 | 61.39079599 | 0.05006575 | 0.08073897 |
$ 4^{th} $ | $ 105.4525306 $ | 105.60832597 | 106.45143402 | 0.14773974 | 0.94725408 |
$ N=50 $ | |||||
$ 1^{st} $ | $ 7.00984547 $ | 7.00984337 | 7.00986020 | 0.00002995 | 0.00021013 |
$ 2^{nd} $ | $ 27.72533453 $ | 27.72529534 | 27.72631753 | 0.00014135 | 0.00354549 |
$ 3^{rd} $ | $ 61.16521834 $ | 61.16521665 | 61.17571474 | 0.00002763 | 0.01716073 |
$ 4^{th} $ | $ 105.4525306 $ | 105.45258028 | 105.49998929 | 0.00004703 | 0.04500470 |
$ N=100 $ | |||||
$ 1^{st} $ | $ 7.00984547 $ | 7.00984334 | 7.00984750 | 0.00003038 | 0.00002895 |
$ 2^{nd} $ | $ 27.72533453 $ | 27.72529099 | 27.72554833 | 0.00015704 | 0.00077113 |
$ 3^{rd} $ | $ 61.16521834 $ | 61.16516979 | 61.16781319 | 0.00007937 | 0.00424236 |
$ 4^{th} $ | $ 105.4525306 $ | 105.45234022 | 105.46427897 | 0.00018055 | 0.03111408 |
Modes | [23] | FEM by CM | FEM by LM |
$ K_{1}=K_{2}=10^{12} $ | |||
$ 1^{st} $ | $ 9.8696 $ | 9.8696 | 9.8695 |
$ 2^{nd} $ | $ 39.478 $ | 39.4782 | 39.4737 |
$ 3^{rd} $ | $ 88.876 $ | 88.8769 | 88.7669 |
$ 4^{th} $ | $ 57.914 $ | 157.7529 | 157.5231 |
$ K_{1}=K_{2}=0 $ | |||
$ 1^{st} $ | $ 0.0000 $ | 0, 0000 | 0.0000 |
$ 2^{nd} $ | $ 0.000 $ | 0.0002 | 0.0041 |
$ 3^{rd} $ | $ 22.273 $ | 22.3740 | 21.7055 |
$ 4^{th} $ | $ 61.373 $ | 61.6881 | 58.6391 |