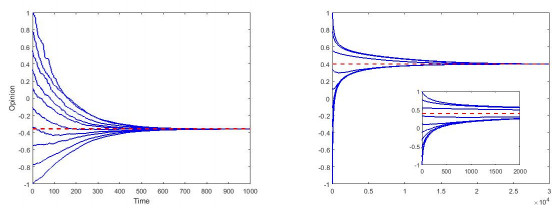
In this work we study the formation of consensus in homogeneous and heterogeneous populations, and the effect of attractiveness or fitness of the opinions. We derive the corresponding kinetic equations, analyze the long time behavior of their solutions, and characterize the consensus opinion.
Citation: Mayte Pérez-Llanos, Juan Pablo Pinasco, Nicolas Saintier. Opinion fitness and convergence to consensus in homogeneous and heterogeneous populations[J]. Networks and Heterogeneous Media, 2021, 16(2): 257-281. doi: 10.3934/nhm.2021006
[1] | Mayte Pérez-Llanos, Juan Pablo Pinasco, Nicolas Saintier . Opinion fitness and convergence to consensus in homogeneous and heterogeneous populations. Networks and Heterogeneous Media, 2021, 16(2): 257-281. doi: 10.3934/nhm.2021006 |
[2] | Sabrina Bonandin, Mattia Zanella . Effects of heterogeneous opinion interactions in many-agent systems for epidemic dynamics. Networks and Heterogeneous Media, 2024, 19(1): 235-261. doi: 10.3934/nhm.2024011 |
[3] | Sharayu Moharir, Ananya S. Omanwar, Neeraja Sahasrabudhe . Diffusion of binary opinions in a growing population with heterogeneous behaviour and external influence. Networks and Heterogeneous Media, 2023, 18(3): 1288-1312. doi: 10.3934/nhm.2023056 |
[4] | Clinton Innes, Razvan C. Fetecau, Ralf W. Wittenberg . Modelling heterogeneity and an open-mindedness social norm in opinion dynamics. Networks and Heterogeneous Media, 2017, 12(1): 59-92. doi: 10.3934/nhm.2017003 |
[5] | Yuntian Zhang, Xiaoliang Chen, Zexia Huang, Xianyong Li, Yajun Du . Managing consensus based on community classification in opinion dynamics. Networks and Heterogeneous Media, 2023, 18(2): 813-841. doi: 10.3934/nhm.2023035 |
[6] | Nastassia Pouradier Duteil . Mean-field limit of collective dynamics with time-varying weights. Networks and Heterogeneous Media, 2022, 17(2): 129-161. doi: 10.3934/nhm.2022001 |
[7] | Sergei Yu. Pilyugin, Maria S. Tarasova, Aleksandr S. Tarasov, Grigorii V. Monakov . A model of voting dynamics under bounded confidence with nonstandard norming. Networks and Heterogeneous Media, 2022, 17(6): 917-931. doi: 10.3934/nhm.2022032 |
[8] | Marina Dolfin, Mirosław Lachowicz . Modeling opinion dynamics: How the network enhances consensus. Networks and Heterogeneous Media, 2015, 10(4): 877-896. doi: 10.3934/nhm.2015.10.877 |
[9] | Sergei Yu. Pilyugin, M. C. Campi . Opinion formation in voting processes under bounded confidence. Networks and Heterogeneous Media, 2019, 14(3): 617-632. doi: 10.3934/nhm.2019024 |
[10] | Catherine Choquet, Ali Sili . Homogenization of a model of displacement with unbounded viscosity. Networks and Heterogeneous Media, 2009, 4(4): 649-666. doi: 10.3934/nhm.2009.4.649 |
In this work we study the formation of consensus in homogeneous and heterogeneous populations, and the effect of attractiveness or fitness of the opinions. We derive the corresponding kinetic equations, analyze the long time behavior of their solutions, and characterize the consensus opinion.
In the last few years, several physicists and mathematicians devoted their attention to opinion dynamics. Different techniques were used, depending on the specific problem. For instance, computer simulations are preferred when agents contact through networks, although they appear also in mean field problems [5,15,32,33,37], Markov processes and other probabilistic tools for finitely many opinions [17,18,21], large systems of ordinary differential equations for active particles, and the associated Boltzmann type equations for the evolution of several observables [12,20,27,29,31,35,38], together with their Fokker-Planck limits. We can cite the books [8,9,19,28], and the surveys [1,24] for details.
Despite these different tools, the microscopic interactions among agents are based on sociological theories like social impact and social pressure theory [3,13,23], where agents modify their opinions trying to fit in some social group, and the persuasive argument theory [11,25], where the new opinion appears after an interchange of arguments among agents.
However, the space of opinions is typically assumed as homogeneous, without considering the advantages of holding some specific opinions. Recently, in [30] we have proposed a model where the opinions are weighted by a function
w′=w+γλ(w∗)(w∗−w)w′∗=w∗+γλ(w)(w−w∗). | (1) |
where
Let us mention briefly that in [6,7,22] there are two competing opinions, say
w′=w+γP(w)(w∗−w)w′∗=w∗+γP(w∗)(w−w∗), | (2) |
where
In this work we study the long time behavior of a population interacting through rule (1), and we add more heterogeneity on the agents by introducing two parameters
In Section §2 we introduce the model and the mean field first order equation, satisfied by the density of agents in the space of opinions. In Section §3 we focus on the effect of the fitness of opinions in an homogeneous population, and we derive the precise value of the consensus opinion
m∞=Λ−1(∫Λ(w)df0(w)) |
where
Let us introduce our model of opinion formation. We consider a population composed of infinitely many agents. The opinion of an agent with respect to some statement is represented by a real number
In addition, we take into account the ability (or difficulty) of an individual to persuade another agent, denoted by
Each agent is thus characterized by the three parameters
Our model also takes into account the possibility that the fitness of an individual opinion may affect other agents. We introduce an influence function
● Quadratic :
● Linear :
● Uniform :
● Co-Linear :
● Co-Quadratic :
Individuals which held extreme opinions will have more influence under the linear and quadratic functions (extremist influence functions), while the co-linear and co-quadratic functions endow more influence to agents with moderate opinions, (centrist influence functions).
We now describe the up-dating rules of the opinions. Consider two interacting agents with parameters
w′=w+γqp∗λ(w∗)(w∗−w),w′∗=w∗+γq∗pλ(w)(w−w∗). | (3) |
Observe that the term
First of all we establish some notations. Let
W1(μ,ν)=supϕ∫ϕd(μ−ν), | (4) |
where the supremum is taken among the 1-Lipschitz functions
Denote as
In the case of binary interactions and assuming, as usually done, that the joint distibution
ddt∫Kϕ(ϖ)dfγt(ϖ)=∫K(ϕ(ϖ′)−ϕ(ϖ))dfγt(ϖ∗)dfγt(ϖ). |
A fixed point argument yields existence and uniqueness for this equation.
Our next purpose is to describe the time evolution of this density for a given initial condition
ϕ(ϖ′)−ϕ(ϖ)≈(w′−w)∂wϕ(ϖ)+12(w′−w)2∂wwϕ(ϖ)=γqp∗λ(w∗)(w∗−w)∂wϕ(ϖ)+12(γqp∗λ(w∗))2(w∗−w)2∂wwϕ(ϖ) |
to obtain, after simplification,
1γddt∫Kϕ(ϖ)dfγt(ϖ)≈∫K(mγ(t)−w)⟨pλ⟩γq∂wϕ(ϖ)dfγt(ϖ)+γ2∫K∂wwϕ(ϖ)Dγt(ϖ)dfγt(ϖ) |
with
⟨pλ⟩γ=∫Kpλ(w)dfγt(ϖ),mγ(t)=∫Kpλ(w)⟨pλ⟩γwdfγt(ϖ),Dγt(ϖ)=q2(⟨p2w2λ2⟩−2w⟨p2wλ2⟩+w2⟨p2λ2⟩). |
Notice that
Rescaling time considering
ddτ∫Kϕ(ϖ)dfγτ(ϖ)≈∫K(mγ(τ)−w)⟨pλ⟩γq∂wϕ(ϖ)dfγτ(ϖ)+γ2∫K∂wwϕ(ϖ)Dγτ(ϖ)dfγτ(ϖ). | (5) |
This procedure can be justified showing that
ddτ∫Kϕ(ϖ)dfτ(ϖ)=∫K(m(τ)−w)⟨pλ⟩q∂wϕ(ϖ)dfτ(ϖ). |
This is the core of the following Theorem, whose proof (except for minor changes) can be found in [31].
Theorem 2.1. There exists
∫Kϕdfτ=∫Kϕdf0+∫τ0∫K(m(s)−w)⟨pλ⟩q∂wϕ(ϖ)dfs(ϖ)ds, | (6) |
for any
⟨pλ⟩=∫Kpλ(w)dfτ(ϖ) | (7) |
is the mean value at time
m(τ)=∫Kpλ(w)⟨pλ⟩wdfτ(ϖ). | (8) |
is the mean opinion weighted by the normalized actions of both the power of persuasion and the influence exerted by the agents.
Remark 1. Notice that (6) is the weak formulation of the transport equation
∂τf+∂w((m(τ)−w)⟨pλ⟩qf)=0. |
The rest of the paper is devoted to the study of the long-time behaviour of
In both cases we will prove that
This indeed happens as shown by the numerical experiments at the end of the paper. We also refer to [28] for more details on this issue.
It is worth to emphasize here an important difference with respect to the model studied in [31], which corresponds to taking
From now on we denote
Before going further we recall a useful trick concerning transport equations.
If
F−1(ρ)=inf{x∈[−1,1] such that F(x)≥ρ}. | (9) |
Observe that
[F−1(0+),F−1(1)]⊃suppf. | (10) |
In addition, for any
If F(x)>0 then F−1(F(x))≤x while F(F−1(ρ))≥ρ. | (11) |
See the note of Embrechts and Hofert [16] for the above (and further) properties of
∫10ϕ(F−1(r))dr=∫1−1ϕ(w)df(w), | (12) |
for any
This change of variables will be a key point in the subsequent arguments. More precisely, consider
∂tft+∂x(V(t,x)ft)=0. | (13) |
Denote as
Proposition 1. Let
∫1−1ϕ(x)dft(x)=∫1−1ϕ(x)df0(x)+∫t0∫1−1ϕ′(x)v(s,x)dfs(x)ds, | (14) |
if and only if for any
∂tXt(r)=v(t,Xt(r)). | (15) |
Here,
The proof can be found essentially in Theorem 3.1 in [2]. See also [31], where it is rewritten under the point of view of the ordinary differential equation for the flux (15).
In this section we evaluate the impact of the attractiveness of the opinion independently of any other consideration. Thus, an agent is now completely characterized by its opinion
w′=w+λ(w∗)(w∗−w). | (16) |
This synergy after encounters was indeed introduced in [30]. A simpler form that takes the model with homogeneity, allowed us to find the kinetic equations with the use of the empirical distribution for
The resultant continuous distribution
∫1−1ϕdft=∫1−1ϕdf0+∫t0∫1−1(mt−w)⟨λ⟩ϕ′(w)dfs(w)ds. | (17) |
where
mt=∫1−1λ(w)⟨λ⟩wdft(w),⟨λ⟩=∫1−1λ(w)dft(w), |
which is consistent with (6) taking
Moreover, in [30] we identified a conserved quantity for the evolution equation (17), whenever
ddt∫1−1Λ(w)dft=∫1−1(mt−w)⟨λ⟩Λ′(w)dft(w)=∫1−1(mt−w)⟨λ⟩λ(w)dft(w). |
Recall that
∫1−1Λdft=∫1−1Λdf0for any t≥0. |
Sending
Λ(m∞)=∫Λdf0. | (18) |
Hence, the candidate to be the value of consensus is
m∞=Λ−1(∫1−1Λ(w)df0(w)). |
In the next result we perform a rigorous proof of the dynamics contraction towards this value.
Theorem 3.1. Assume that
λ(w)≥λ_for any w∈conv(supp(f0)), | (19) |
being
Then, there exists
W1(ft,δm∞)≤|conv(supp(f0))|e−λ_t. | (20) |
Moreover, the limit opinion
m∞=Λ−1(∫Λ(w)df0(w)), | (21) |
where
Before the proof, some remarks are in order:
Remark 2. Notice that we just require
Remark 3. In the case
The proof of Theorem 3.1 goes as follows:
Proof of Theorem 3.1. Let
∂tXt(r)=(mt−Xt(r))⟨λ⟩. |
Moreover,
mt=∫1−1λ(w)⟨λ⟩wdft(w)=∫10Xt(r)λ(Xt(r))⟨λ⟩dr. |
Since
mt≤Xt(1)∫10λ(Xt(r))⟨λ⟩dr=Xt(1)∫1−1λ(w)⟨λ⟩dft(w)=Xt(1), |
and similarly
Xt(0+)≤mt≤Xt(1). |
Note that
conv(supp(ft))⊂conv(supp(f0))t≥0. |
Indeed,
∂t[(Xt(1)−Xt(r))2]=−2(Xt(1)−Xt(r))2⟨λ⟩≤−2λ_(Xt(1)−Xt(r))2, |
and Gronwall's Lemma gives, for any
|Xt(1)−Xt(r)|≤|X0(1)−X0(r)|e−λ_t. |
In particular,
|Xt(1)−Xt(0+)|≤|X0(1)−X0(0+)|e−λ_t, |
which reveals that the length of
Recalling that
|Xt(r)−m∞|≤|Xt(1)−Xt(0+)|≤|X0(1)−X0(0+)|e−λ_t. |
Inequality (20) follows now by observing that
W1(ft,δm∞)≤∫1−1|w−m∞|dft(w)=∫10|Xt(r)−m∞|dr≤|X0(1)−X0(0+)|e−λ_t. |
It remains to show (21). Notice that
A:=Λ(conv(supp(f0)))=[Λ(X0(0+)),Λ(X0(1))]. |
Furthermore,
Λ(X0(0+))≤∫1−1Λdf0(w)=∫10Λ(X0(r))dr≤Λ(X0(1)), |
and consequently
We would like to close this section by emphasizing some relevant considerations of the result above.
First of all, the hypothesis of strict positivity of the influence function on the convex hull of the initial density is absolutely necessary to obtain Theorem 3.1.
Indeed in [30] we already noticed singular behaviour at the roots of
This fact becomes more evident the larger the order of the zero is. If
z=∫1−1Λ(w)df0(w), |
two phenomena occur. One is the slow formation of consensus due to a frozen dynamics due to the small values that
Let us note that the first order equation is interpreted in a weak sense, so the differentiability of
It is worth to mention a related dynamics appearing in [2,38], where agents are influenced by their own opinion when interact,
{w′=w+γP(w)(w∗−w)w′∗=w∗+γP(w∗)(w−w∗). | (22) |
Typical examples of function
∫1−1ϕdft=∫1−1ϕdf0+∫t0∫1−1P(w)(⟨w⟩−w)ϕ′(w)dfs(w)ds, | (23) |
where
ddt∫Π(w)dft(w)=∫1−1P(w)(⟨w⟩−w)1P(w)dfs(w)=∫1−1(⟨w⟩−w)dfs(w) |
which is equal to
Π(˜m∞)=∫Πdf0 |
so that
This section is devoted to study the long-time behaviour of the unique solution to the transport equation (6), arising from an initial distribution
ft=0=α0f00+(1−α0)f10, | (24) |
being
ft=α0f00+(1−α0)f1t,t≥0. |
Before stating our main result, we give an informal deduction of the possible value to be the limiting opinion, presuming that the non-stubborn agents reach a consensus at
In view of the transport term, whenever consensus is reached among the non-stubborn agents, it should take place at
f1t→f10(p,q)dpdq⊗δm∞t→+∞, |
where
⟨pλ⟩mt=∫Kpλ(w)wdft(ϖ). | (25) |
On the one hand,
∫Kpλ(w)wdft(ϖ)=α0∫1−1∫10pλ(w)wdf00(w,p)+(1−α0)∫Kpλ(w)wdf1t(ϖ)→α0∫1−1∫10pλ(w)wdf00(w,p)+(1−α0)λ(m∞)m∞∫Kpdf10(ϖ). |
While on the other hand,
⟨pλ⟩=α0∫1−1∫10pλ(w)df00(w,p)+(1−α0)∫Kpλ(w)df1t(ϖ)→α0∫1−1∫10pλ(w)df00(w,p)+(1−α0)λ(m∞)∫Kpdf10(ϖ). |
Taking now limits in (25) we get
m∞(α0∫1−1∫10pλ(w)df00(w,p)+(1−α0)λ(m∞)∫Kpdf10(ϖ))=α0∫1−1∫10pλ(w)wdf00(w,p)+(1−α0)λ(m∞)m∞∫Kpdf10(ϖ). |
Since
m∞∫1−1∫10pλ(w)df00(w,p)=∫1−1∫10pλ(w)wdf00(w,p). |
Conclusion: If the non-stubborn population reaches consensus, then the consensus opinion
m∞:=∫1−1∫10pλ(w)w⟨pλ⟩0df00(w,p), | (26) |
whenever the term
⟨pλ⟩0:=∫1−1∫10pλ(w)df00(w,p), | (27) |
that stands for the mean value of
This shows that, admitting long-time consensus among the non-stubborn population, its shared opinion
At this stage, it is crucial to underline a relevant fact about the candidate for consensus found above. If the influence function is constant, this model lays on our previous work studied in [31], and hence the value for
m∞:=∫1−1∫10pw⟨p⟩0df00(w,p). |
Our main result shows that non-stubborn agents indeed reach consensus asymptotically at the value
Before stating it recall that
We are now ready to state our main result of this section
Theorem 4.1. Assume
(p,q)∈[0,1]×[ε0,1]→f10|(p,q)∈P([−1,1]), |
is globally Lipschitz for the
W1(f10|(p,q),f10|(p′,q′))≤L(|q−q′|+|p−p′|). | (28) |
In addition, assume that
Then, for any
W1(f1t,f10(p,q)dpdq⊗δm∞)≤(4+κt)e−ε0α0⟨pλ⟩0t | (29) |
where
κ=8‖λ‖∞λ_(‖λ′‖∞+‖λ″‖∞)+4‖λ′‖∞. |
The global idea of the proof of Theorem 4.1 follows the lines of the proof in [31], where the case
In the first step we consider
Step 4.1. For any
{∂tf1t|(p,q)+∂w((mt−w)q⟨pλ⟩f1t|(p,q))=0,f1t=0|(p,q)=f0|(p,q), | (30) |
in
Moreover, the function
W1(f1t|(p,q),f1t|(p′,q′))≤Ct(|q−q′|+|p−p′|). |
Furthermore, it fulfils
∫Kϕdf1t=∫10∫10(∫1−1ϕdf1t|(p,q)(w))df10(p,q),∀ϕ∈C(K). | (31) |
In the next item, we take advantage of the tendency to compromise modeled by the interaction rules (3) to prove that non-stubborn agents with given
⟨λ⟩(p,q)=∫1−1λ(w)df1t|(p,q)(w), | (32) |
and
m(t,p,q)=∫1−1λ(w)⟨λ⟩(p,q)wdf1t|(p,q)(w), | (33) |
the mean value of
Step 4.2. For any
W1(f1t|(p,q),δm(t,p,q))≤2e−ε0α0⟨pλ⟩0tt≥0, | (34) |
being
Proof. Let
∂tXt(r)=(mt−Xt(r))q⟨pλ⟩. |
In particular
∂t[(Xt(1)−Xt(r))2]=−2(Xt(1)−Xt(r))2q⟨pλ⟩≤−2(Xt(1)−Xt(r))2ε0⟨pλ⟩. |
The nonnegativity of
⟨pλ⟩=∫pλ(w)dft=α0⟨pλ⟩0+(1−α0)∫pλ(w)df1t≥α0⟨pλ⟩0, |
hence
∂t[(Xt(1)−Xt(r))2]≤−2α0ε0(Xt(1)−Xt(r))2⟨pλ⟩0. |
Gronwall's Lemma implies now that
|Xt(1)−Xt(r)|≤|X0(1)−X0(r)|e−α0ε0⟨pλ⟩0t, | (35) |
which sending
|Xit(1)−Xit(0+)|≤|Xi0(1)−Xi0(0+)|e−α0ε0⟨pλ⟩0t. | (36) |
This shows that the length of the support of
Invoking (12), we express
m(t,p,q)=∫1−1λ(w)⟨λ⟩(p,q)wdft|(p,q)(w)=∫10Xt(r)λ(Xt(r))⟨λ⟩(p,q)dr. |
Since
m(t,p,q)≤Xt(1)∫10λ(Xt(r))⟨λ⟩(p,q)dr=Xt(1)∫1−1λ(w)⟨λ⟩(p,q)dft|(p,q)(w)=Xt(1), |
and similarly
Xt(0+)≤mit≤Xit(1). | (37) |
Therefore, for any
|Xt(r)−m(t,p,q)|≤|Xt(1)−Xt(0+)|≤|X0(1)−X0(0+)|e−α0ε0⟨pλ⟩0t. |
The proof now concludes by noticing that
W1(ft|(p,q),δm(t,p,q))≤∫1−1|w−m(t,p,q)|dft|(p,q)(w)=∫10|Xt(r)−m(t,p,q)|dr≤|X0(1)−X0(0+)|e−α0ε0⟨pλ⟩0t. |
As a result of the previous step, it is desirable to study the asymptotic behavior of the function
Step 4.3. For any
∂tm(t,p,q)=α0q⟨pλ⟩0[m∞−m(t,p,q)]+(1−α0)q∫1−1p′λ(m(t,p′,q′))[m(t,p′,q′)−m(t,p,q)]df10(p′,q′)+R(t,p,q) | (38) |
where
|R(t,p,q)|≤{8‖λ‖∞λ_(‖λ′‖∞+‖λ″‖∞)+4‖λ′‖∞}e−ε0α0⟨pλ⟩0t. | (39) |
Proof. Using equation (30), the evolution in time of the conditioned mean
ddt⟨λ⟩(p,q)=ddt∫1−1λ(w)df1t|(p,q)(w)=q⟨pλ⟩∫1−1(mt−w)λ′(w)df1t|(p,q)(w) |
and
ddt∫1−1wλ(w)df1t|(p,q)=q⟨pλ⟩∫1−1(mt−w)(wλ′(w)+λ(w))df1t|(p,q)(w). |
Thus,
∂∂tm(t,p,q)=∂∂t{1⟨λ⟩(p,q)∫1−1wλ(w)df1t|(p,q)(w)}=q⟨pλ⟩⟨λ⟩(p,q){∫1−1(mt−w)λ′(w)(w−m(t,p,q))df1t|(p,q)(w)+∫1−1(mt−w)λ(w)df1t|(p,q)(w)}. |
Summing up,
∂∂tm(t,p,q)=q⟨pλ⟩⟨λ⟩(p,q)∫1−1(mt−w)λ′(w)(w−m(t,p,q))df1t|(p,q)(w)+q⟨pλ⟩(mt−m(t,p,q)). | (40) |
Estimate (34) allows to bound the intergral in the r.h.s. as follows. Let
∫1−1(mt−w)λ′(w)(w−m(t,p,q))df1t|(p,q)(w)=∫1−1ϕ(w)−ϕ(m(t,p,q))df1t|(p,q)(w)=∫1−1ϕ(w)(df1t|(p,q)(w)−δm(t,p,q)). |
According to the definition of the
∫1−1(mt−w)λ′(w)(w−m(t,p,q))df1t|(p,q)(w)≤Lip(ϕ)W1(f1t|(p,q),δm(t,p,q)). |
It is easily seen that
∫1−1(mt−w)λ′(w)(w−m(t,p,q))df1t|(p,q)(w)≤8(‖λ′‖∞+‖λ″‖∞)e−ε0α0⟨pλ⟩0t. |
Thus,
∂∂tm(t,p,q)=q⟨pλ⟩(mt−m(t,p,q))+˜R(t,p,q) |
with
|˜R(t,p,q)|≤q⟨pλ⟩⟨λ⟩(p,q)8(‖λ′‖∞+‖λ″‖∞)e−ε0α0⟨pλ⟩0t≤8‖λ‖∞λ_(‖λ′‖∞+‖λ″‖∞)e−ε0α0⟨pλ⟩0t, |
given that the assumption
We now focus on the second term in the right hand side of (40). First,
⟨pλ⟩mt=⟨pwλ⟩=α0⟨pwλ⟩0+(1−α0)∫Kp′w′λ(w′)df1t(ϖ′), |
where the integral can be written using (31) as
∫10∫10p′(∫1−1w′λ(w′)df1t|(p′,q′)(w))df10(p′,q′)=∫10∫10p′⟨λ⟩(p′,q′)m(t,p′,q′)df10(p′,q′). |
Recalling in addition that
⟨pλ⟩mt=α0⟨pλ⟩0m∞+(1−α0)∫10∫10p′⟨λ⟩(p′,q′)m(t,p′,q′)df10(p′,q′). |
On the other hand,
⟨pλ⟩=α0⟨pλ⟩0+(1−α0)∫Kp′λ(w′)df1t(ϖ′)=α0⟨pλ⟩0+(1−α0)∫10∫10p′(∫1−1λ(w′)df1t|(p′,q′)(w))df10(p′,q′)=α0⟨pλ⟩0+(1−α0)∫10∫10p′⟨λ⟩(p′,q′)df10(p′,q′). |
Merging the former identities together,
∂∂tm(t,p,q)=α0q⟨pλ⟩0[m∞−m(t,p,q)]+(1−α0)q∫10∫10p′⟨λ⟩(p′,q′)[m(t,p′,q′)−m(t,p,q)]df10(p′,q′)+˜R(t,p,q). |
According to (34) we have for any
|⟨λ⟩(p,q)−λ(m(t,p,q))|=|(f1t|(p,q)−δm(t,p,q),λ)|≤2e−ε0α0⟨pλ⟩0tLip(λ)≤2e−ε0α0⟨pλ⟩0t‖λ′‖∞. |
As a result,
∂∂tm(t,p,q)=α0q⟨pλ⟩0[m∞−m(t,p,q)]+(1−α0)q∫p′λ(m(t,p′,q′))[m(t,p′,q′)−m(t,p,q)]df10(p′,q′)+˜R(t,p,q)+ˆR(t,p,q) |
with
ˆR(t,p,q)≤(1−α0)q2e−ε0α0⟨pλ⟩0t‖λ′‖∞×∫10∫10p′|m(t,p′,q′)−m(t,p,q)|df10(p′,q′)≤4e−ε0α0⟨pλ⟩0t‖λ′‖∞ |
where we used that
To understand intuitively the infinite system of equations (38) it is useful to consider the elementary situation where only a finite number of values for
f1t=N∑i=1αigit(w)dw⊗δp=pi,q=qi, |
where
ddtmit=A−Bmit+N∑j=1cjλ(mjt)(mjt−mit)+Ri(t) | (41) |
where
The regularity of
Step 4.4. For any
Proof. According to Step 4.1,
W1(f1t|(p,q),f1t|(p′,q′))≤Ct(|q−q′|+|p−p′|). |
The functions
|⟨λ⟩(p,q)−⟨λ⟩(p′,q′)|=|(f1t|(p,q)−f1t|(p′,q′),λ)|≤Lip(λ)W1(f1t|(p,q),f1t|(p′,q′))≤Ct‖λ‖∞(|p−p′|+|q−q′|) |
and in the same way
|⟨wλ⟩(p,q)−⟨wλ⟩(p′,q′)|≤CtLip(wλ(w))(|p−p′|+|q−q′|). |
Consequently, for a given
Step 4.5. For any
|m(t,p,q)−m∞|≤e−ε0α0⟨pλ⟩0t(|m(0,p,q)−m∞|+κt) |
where
κ=8‖λ‖∞λ_(‖λ′‖∞+‖λ″‖∞)+4‖λ′‖∞. |
Proof. Relation (38) implies that for any
12∂∂t|m(t,p,q)−m∞|2=∂tm(t,p,q)[m(t,p,q)−m∞]=−qα0⟨pλ⟩0[m∞−m(t,p,q)]2+q(1−α0)[m(t,p,q)−m∞]×∫10∫10p′λ(m(t,p′,q′))[m(t,p′,q′)−m(t,p,q)]df10(p′,q′)+R(t,p,q)[m(t,p,q)−m∞]. | (42) |
Recall that
maxsupp(f10(p,q)dpdq)|m(t,.)−m∞|=|m(t,p∗,q∗)−m∞|. |
In particular, we can write (42) at
12∂∂t|m(t,.)−m∞|2|(p∗,q∗)=−q∗α0⟨pλ⟩0[m∞−m(t,p∗,q∗)]2+q∗(1−α0)[m(t,p∗,q∗)−m∞]×∫10∫10p′λ(m(t,p′,q′))[m(t,p′,q′)−m∞]df10(p′,q′)−q∗(1−α0)[m(t,p∗,q∗)−m∞]2∫10∫10p′λ(m(t,p′,q′))df10(p′,q′)+R(t,p∗,q∗)[m(t,p∗,q∗)−m∞]=:I+II+III+IV. |
The choice of
II≤q∗(1−α0)|m(t,p∗,q∗)−m∞|2∫10∫10p′λ(m(t,p′,q′))df10(p′,q′)=−III. |
The cancelation of these two terms and
∂∂t|m(t,.)−m∞|2|(p∗,q∗)≤−2ε0α0⟨pλ⟩0|m∞−m(t,p∗,q∗)|2+2R(t,p∗,q∗)|m(t,p∗,q∗)−m∞|. | (43) |
Let
The Envelope Theorem (see Theorem 4.2 below) ensures that the function
V(t):=max(p,q)∈supp(f10)h(t;(p,q)) |
is absolutely continuous with derivative
V′(t)=∂t(|m(t,p∗,q∗)−m∞|2)a.e. |
Furthermore, in view of (43) and (39)
V′(t)≤−2ε0α0⟨pλ⟩0V(t)+2κ√V(t)e−ε0α0⟨pλ⟩0t, |
where
κ=8‖λ‖∞λ_(‖λ′‖∞+‖λ″‖∞)+4‖λ′‖∞. |
Dividing by
(√V)′(t)≤−ε0α0⟨pλ⟩0√V(t)+κe−ε0α0⟨pλ⟩0t |
which integrated gives
√V(t)≤e−ε0α0⟨pλ⟩0t√V(0)+∫t0e−ε0α0⟨pλ⟩0(t−s)κe−ε0α0⟨pλ⟩0sds=e−ε0α0⟨pλ⟩0t(√V(0)+κt). |
This shows that
|m(t,p,q)−m∞|≤e−ε0α0⟨pλ⟩0t(|m(0,p,q)−m∞|+κt), |
for any
In the course of the proof of the previous step we used the following envelope Theorem, due to Milgrom and Segal in [26]:
Theorem 4.2. Consider the function
Assuming further that
V(t)=V(0)+∫t0∂th(x∗(s),s)ds. |
We are now in position to finish the proof of Theorem 4.1.
Step 4.6. There holds
W1(f1t,f10(p,q)dpdq⊗δm∞)≤(4+κt)e−ε0α0⟨pλ⟩0t, |
for any
Proof. Step 4.5 ensures that for any
W1(δm(t,p,q),δm∞)=|m(t,p,q)−m∞|≤(2+κt)e−ε0α0⟨pλ⟩0t, |
while according to (34), we have that
W1(f1t|(p,q),δm∞)≤(4+κt)e−ε0α0⟨pλ⟩0t. |
In fact, we claim that
W1(f1t,δm∞⊗f10(p,q)dpdq)≤(4+κt)e−ε0α0⟨pλ⟩0t. |
Let
∫Kψ(df1t−δm∞⊗f10(p,q)dpdq)=∫10∫10(∫1−1ψ(w,p,q)(dft|(p,q)−δm∞))df0(p,q). |
The inner integral is bounded above by
∫Kψ(df1t−δm∞⊗f10(p,q)dpdq)≤(4+κt)e−ε0α0⟨pλ⟩0t∫10∫10df0(p,q)=(4+κt)e−ε0α0⟨pλ⟩0t. |
The claim follows by taking supremum among the functions
In this section we perform some agent based simulations of the results studied along this work. We assess qualitatively the effect of the influence function
λ(w)=(w−0.5)2+ε,ε=0.01, |
on the dynamics. We first consider a homogeneous population and ratify that, indeed, the conclusions of Theorem 3.1 are true. Then, to illustrate the conclusion of Theorem 4.1, we add to this scenario several stubborn agents positioned at two specific values for
In all of the simulations presented here, we consider a population of
Since opinions are initially distributed uniformly in
Λ(m∞)=12∫1−1Λ(w)dw, |
where
m∞≈−0.35. |
In Figure 1 (left) we show the time evolution of the opinions of 10 agents of the population (blue curves). Consensus clearly occurs at the value
This is completely in contrast to consider that the influence is exerted, instead, by one's own opinion agent, see [2,38]. Indeed, under the interaction rules (22), we prove in subsection §3.3 that if consensus is reached then, the consensus opinion
Π(˜m∞)=∫1−1Π(w)df0(w), |
where
˜m∞≈0.41. |
The result of the agent-based simulation shown in the right figure of Figure 1 confirms that consensus takes place at
We can also observe from the simulation that the consensus is attained much faster for the interaction rule (16) than for (22). Recall that the interaction rule (22) takes into account the attractiveness of the opinion of the agent one is interacting with. Intuitively, this is due to the fact that agents with opinion
ln(maxsuppftw−minsuppftw). |
In the rest of this section we examine the impact of stubborn agents combined with the influence function.
We consider a population of
f00=12δp=1⊗δw=14+12δp=1⊗δw=34. |
Notice that, in particular
⟨pλ⟩0=∫pλ(w)df00(w,p)=∫1−1λ(w)df00(w)=12(λ(1/4)+λ(3/4))=116+ε. |
and
⟨pλ(w)w⟩0=∫1−1λ(w)wdf0(w)=12(λ(1/4)14+λ(3/4)34)=12(116+ε). |
The theoretical limit opinion is then
m∞=⟨pλ(w)w⟩0⟨pλ⟩0=12. |
To evaluate the impact driven just by the proportion
We observe a perfect compliance between the agent-based simulations and the theoretical prediction. Furthermore, in the simulations the consensus is clearly achieved in two steps. First non-stubborn agents quickly reach a consensus, and then all together move slowly towards the final limit opinion
This fact is specially well observed on the left figure, where a smaller proportion of stubborn population is considered (
Finally, we wish to appreciate the qualitative impact of the parameter
The authors appreciated the fine comments of both referees which helped to enhance the previous version of the manuscript.
[1] |
G. Aletti, A. K. Naimzada and G. Naldi., Mathematics and physics applications in sociodynamics simulation: The case of opinion formation and diffusion, Mathematical Modeling of Collective Behavior in Socio-Economic and Life Sciences, Birkhäuser Boston, (2010), 203–221. doi: 10.1007/978-0-8176-4946-3_8
![]() |
[2] |
First-order continuous models of opinion formation. SIAM J. Appl. Math. (2007) 67: 837-853. ![]() |
[3] |
Opinions and social pressure. Scientific American (1955) 193: 31-35. ![]() |
[4] | R. B. Ash, Real Analysis and Probability, Probability and Mathematical Statistics, Academic Press, New York-London, 1972. |
[5] | P. Balenzuela, J. P. Pinasco and V. Semeshenko, The undecided have the key: Interaction driven opinion dynamics in a three state model, PLoS ONE, 10 (2016), e0139572, 1–21. |
[6] |
Opinion strength influences the spatial dynamics of opinion formation. The Journal of Mathematical Sociology (2016) 40: 207-218. ![]() |
[7] | B. O. Baumgaertner, P. A. Fetros, R. C. Tyson and S. M. Krone, Spatial Opinion Dynamics and the Efects of Two Types of Mixing, Phys Rev E., 98 (2018), 022310. |
[8] | N. Bellomo, Modeling Complex Living Systems A Kinetic Theory and Stochastic Game Approach, Birkhauser, 2008. |
[9] |
N. Bellomo, G. Ajmone Marsan and A. Tosin, Complex Systems and Society. Modeling and Simulation, SpringerBriefs in Mathematics, 2013. doi: 10.1007/978-1-4614-7242-1
![]() |
[10] | K. C. Border and C. D. Aliprantis, Infinite Dimensional Analysis - A Hitchhiker's Guide, 3rd Edition, Springer, 2006. |
[11] |
What a person thinks upon learning he has chosen differently from others: Nice evidence for the persuasive-arguments explanation of choice shifts. Journal of Experimental Social Psychology (1975) 11: 412-426. ![]() |
[12] |
A well-posedness theory in measures for some kinetic models of collective motion. Mathematical Models and Methods in Applied Sciences (2011) 21: 515-539. ![]() |
[13] | R. B. Cialdini and M. R. Trost, Social influence: Social norms, conformity and compliance, The Handbook of Social Psychology, McGraw-Hill, (1998), 151–192. |
[14] |
Emergent behavior in flocks. IEEE Transactions on Automatic Control (2007) 52: 852-862. ![]() |
[15] |
Mixing beliefs among interacting agents. Advances in Complex Systems (2000) 3: 87-98. ![]() |
[16] |
A note on generalized inverse. Mathematical Methods of Operations Research (2013) 77: 423-432. ![]() |
[17] |
Social influence and opinions. Journal of Mathematical Sociology (1990) 15: 193-206. ![]() |
[18] | A simple model of consensus formation. Okayama Economic Review (1999) 31: 95-100. |
[19] |
S. Galam, Sociophysics: A Physicist's Modeling of Psycho-Political Phenomena, , Springer Science & Business Media, 2012. doi: 10.1007/978-1-4614-2032-3
![]() |
[20] | R. Hegselmann and U. Krause, Opinion dynamics and bounded confidence: Models, analysis and simulation, Journal of Artificial Societies and Social Simulation, 5 (2002). |
[21] |
Ergodic theorems for weakly interacting infinite systems and the voter model. The Annals of Probability (1975) 3: 643-663. ![]() |
[22] |
C. La Rocca, L. A. Braunstein and F. Vázquez, The influence of persuasion in opinion formation and polarization, Europhys. Letters, 106 (2014), 40004. doi: 10.1209/0295-5075/106/40004
![]() |
[23] | The psychology of social impact. American Psychologist (1981) 36: 343-356. |
[24] |
Continuous opinion dynamics under bounded confidence: A survey. International Journal of Modern Physics C (2007) 18: 1819-1838. ![]() |
[25] | M. Mäs and A. Flache, Differentiation without distancing. Explaining bi-polarization of opinions without negative influence, PloS one, 8 (2013), e74516. |
[26] |
Envelope theorems for arbitrary choice sets. Econometrica (2002) 70: 583-601. ![]() |
[27] |
Simulation of Sznajd sociophysics model with convincing single opinions. International Journal of Modern Physics C (2001) 12: 1091-1092. ![]() |
[28] | (2014) Interacting Multiagent Systems: Kinetic Equations and Monte Carlo Methods. Oxford: Oxford University Press. |
[29] |
Measure-valued opinion dynamics. M3AS: Mathematical Models and Methods in Applied Sciences (2020) 30: 225-260. ![]() |
[30] |
M. Pérez-Llanos, J. P. Pinasco and N. Saintier, Opinion attractiveness and its effect in opinion formation models, Phys. A, 559 (2020), 125017, 9 pp. doi: 10.1016/j.physa.2020.125017
![]() |
[31] |
Opinion formation models with heterogeneous persuasion and zealotry. SIAM Journal on Mathematical Analysis (2018) 50: 4812-4837. ![]() |
[32] | F. Vazquez, N. Saintier and J. P. Pinasco, The role of voting intention in public opinion polarization, Phys. Rev. E, 101 (2020), 012101, 13pp. |
[33] |
N. Saintier, J. P. Pinasco and F. Vazquez, A model for a phase transition between political mono-polarization and bi-polarization, Chaos: An Interdisciplinary Journal of Nonlinear Science, 30 (2020), 063146, 17 pp. doi: 10.1063/5.0004996
![]() |
[34] |
Modeling opinion dynamics: Theoretical analysis and continuous approximation. Chaos, Solitons & Fractals (2017) 98: 210-215. ![]() |
[35] |
F. Slanina and H. Lavicka, Analytical results for the Sznajd model of opinion formation, The European Physical Journal B, 35 (2003) 279–288. doi: 10.1140/epjb/e2003-00278-0
![]() |
[36] | (1993) Probability Theory, An Analytic View.Cambridge University Press. |
[37] |
Opinion evolution in closed community. International Journal of Modern Physics - C (2000) 11: 1157-1165. ![]() |
[38] |
Kinetic models of opinion formation. Communications in Mathematical Sciences (2006) 4: 481-496. ![]() |
[39] |
C. Villani, Topics in optimal transportation, Grad.Studies in Math., American Mathematical Soc., (2003). doi: 10.1090/gsm/058
![]() |
1. | Natalia Lucía Kontorovsky, Carlo Giambiagi Ferrari, Juan Pablo Pinasco, Nicolas Saintier, Kinetic modeling of coupled epidemic and behavior dynamics: The social impact of public policies, 2022, 32, 0218-2025, 2037, 10.1142/S0218202522500488 | |
2. | Juan Pablo Pinasco, Mauro Rodriguez Cartabia, Nicolas Saintier, Interacting particles systems with delay and random delay differential equations, 2022, 214, 0362546X, 112524, 10.1016/j.na.2021.112524 | |
3. | Juan Pablo Pinasco, Mauro Rodriguez Cartabia, Nicolas Saintier, Evolutionary game theory in mixed strategies: From microscopic interactions to kinetic equations, 2021, 14, 1937-5077, 115, 10.3934/krm.2020051 | |
4. | Lucía Pedraza, Juan Pablo Pinasco, Nicolas Saintier, Pablo Balenzuela, An analytical formulation for multidimensional continuous opinion models, 2021, 152, 09600779, 111368, 10.1016/j.chaos.2021.111368 | |
5. | Azmy S Ackleh, Rainey Lyons, Nicolas Saintier, Finite difference schemes for a size structured coagulation-fragmentation model in the space of Radon measures, 2022, 0272-4979, 10.1093/imanum/drac071 |