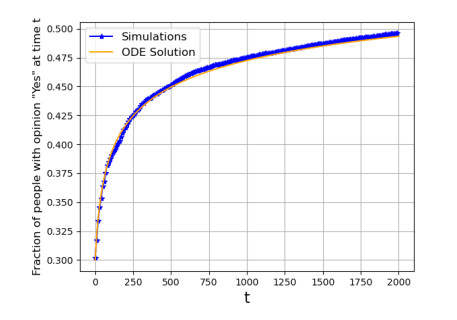
We consider a growing population of individuals with binary opinions, namely, 0 or 1, that evolve in discrete time. The underlying interaction network is complete. At every time step, a fixed number of individuals are added to the population. The opinion of the new individuals may or may not depend on the current configuration of opinions in the population. Further, in each time step, a fixed number of individuals are chosen and they update their opinion in three possible ways: they organically switch their opinion with some probability and with some probability they adopt the majority or the minority opinion. We study the asymptotic behaviour of the fraction of individuals with either opinion and characterize conditions under which it converges to a deterministic limit. We analyze the behaviour of the limiting fraction as a function of the probability of new individuals having opinion 1 as well as with respect to the ratio of the number of people being added to the population and the number of people being chosen to update opinions. We also discuss the nature of fluctuations around the limiting fraction and study the transitions in scaling depending on the system parameters. Further, for this opinion dynamics model on a finite time horizon, we obtain optimal external influencing strategies in terms of when to influence to get the maximum expected fraction of individuals with opinion 1 at the end of the finite time horizon.
Citation: Sharayu Moharir, Ananya S. Omanwar, Neeraja Sahasrabudhe. Diffusion of binary opinions in a growing population with heterogeneous behaviour and external influence[J]. Networks and Heterogeneous Media, 2023, 18(3): 1288-1312. doi: 10.3934/nhm.2023056
[1] | Sergei Yu. Pilyugin, Maria S. Tarasova, Aleksandr S. Tarasov, Grigorii V. Monakov . A model of voting dynamics under bounded confidence with nonstandard norming. Networks and Heterogeneous Media, 2022, 17(6): 917-931. doi: 10.3934/nhm.2022032 |
[2] | Sergei Yu. Pilyugin, M. C. Campi . Opinion formation in voting processes under bounded confidence. Networks and Heterogeneous Media, 2019, 14(3): 617-632. doi: 10.3934/nhm.2019024 |
[3] | Sabrina Bonandin, Mattia Zanella . Effects of heterogeneous opinion interactions in many-agent systems for epidemic dynamics. Networks and Heterogeneous Media, 2024, 19(1): 235-261. doi: 10.3934/nhm.2024011 |
[4] | Yuntian Zhang, Xiaoliang Chen, Zexia Huang, Xianyong Li, Yajun Du . Managing consensus based on community classification in opinion dynamics. Networks and Heterogeneous Media, 2023, 18(2): 813-841. doi: 10.3934/nhm.2023035 |
[5] | Clinton Innes, Razvan C. Fetecau, Ralf W. Wittenberg . Modelling heterogeneity and an open-mindedness social norm in opinion dynamics. Networks and Heterogeneous Media, 2017, 12(1): 59-92. doi: 10.3934/nhm.2017003 |
[6] | Mayte Pérez-Llanos, Juan Pablo Pinasco, Nicolas Saintier . Opinion fitness and convergence to consensus in homogeneous and heterogeneous populations. Networks and Heterogeneous Media, 2021, 16(2): 257-281. doi: 10.3934/nhm.2021006 |
[7] | Rainer Hegselmann, Ulrich Krause . Opinion dynamics under the influence of radical groups, charismatic leaders, and other constant signals: A simple unifying model. Networks and Heterogeneous Media, 2015, 10(3): 477-509. doi: 10.3934/nhm.2015.10.477 |
[8] | Aylin Aydoğdu, Sean T. McQuade, Nastassia Pouradier Duteil . Opinion Dynamics on a General Compact Riemannian Manifold. Networks and Heterogeneous Media, 2017, 12(3): 489-523. doi: 10.3934/nhm.2017021 |
[9] | Marina Dolfin, Mirosław Lachowicz . Modeling opinion dynamics: How the network enhances consensus. Networks and Heterogeneous Media, 2015, 10(4): 877-896. doi: 10.3934/nhm.2015.10.877 |
[10] | Michael Herty, Lorenzo Pareschi, Giuseppe Visconti . Mean field models for large data–clustering problems. Networks and Heterogeneous Media, 2020, 15(3): 463-487. doi: 10.3934/nhm.2020027 |
We consider a growing population of individuals with binary opinions, namely, 0 or 1, that evolve in discrete time. The underlying interaction network is complete. At every time step, a fixed number of individuals are added to the population. The opinion of the new individuals may or may not depend on the current configuration of opinions in the population. Further, in each time step, a fixed number of individuals are chosen and they update their opinion in three possible ways: they organically switch their opinion with some probability and with some probability they adopt the majority or the minority opinion. We study the asymptotic behaviour of the fraction of individuals with either opinion and characterize conditions under which it converges to a deterministic limit. We analyze the behaviour of the limiting fraction as a function of the probability of new individuals having opinion 1 as well as with respect to the ratio of the number of people being added to the population and the number of people being chosen to update opinions. We also discuss the nature of fluctuations around the limiting fraction and study the transitions in scaling depending on the system parameters. Further, for this opinion dynamics model on a finite time horizon, we obtain optimal external influencing strategies in terms of when to influence to get the maximum expected fraction of individuals with opinion 1 at the end of the finite time horizon.
Opinion dynamics in social networks is an area that has received significant attention in recent years [28,33]. This body of work has applications in the areas of advertising, election campaigns, opinion evolution in online social networks, and public policy.
One widely used approach to study opinion dynamics in networks is to model it as a stochastic process on a network of individuals. The voter model [19] is the most popular model used for such studies. Under the voter model, the network is modeled using a bidirectional graph with the set of individuals represented by nodes. There exists an edge between two individuals if they interact with each other. Opinions of individuals are binary. In each time-slot, a node and one of its neighbours are chosen uniformly at random. The node then adopts the opinion of the chosen neighbour. It is well-known that the voter model dynamics on a d-dimensional lattice converges almost surely to a state where all individuals have the same opinion [7]. The consensus opinion depends on the initial state of the system. This behaviour is similar to that of a Pólya urn process, where a ball is drawn uniformly at random from the urn at every time-step and it is replaced in the urn along with another ball of same colour. Such phenomenon of reinforcement is found is several real-life processes like opinion evolution, disease transmission, modeling ant walks etc. Pólya process has been used to model opinion evolution in [20,32]. Numerous other way of modeling evolution of binary opinions and variants of the voter model have been proposed and studied (See recent surveys [1,29]). In the majority-rule version of the voter model, instead of sampling a single neighbour, the updating node/individual adopts the majority opinion in its neighbourhood [5,8,11]. Voter model on various kinds of fixed and evolving network topology have been studied [4,10,12,27,34]. The voter model has also been extended to incorporate biased or stubborn behaviour of individuals [25,35]. In [26] authors combine some of these extensions to study voter model with majority rule in presence of biased and stubborn individuals. The central question is to understand the conditions for asymptotic consensus and the rate of convergence to the consensus. For instance, in [26] authors show that the expected time to reach consensus is \mathcal O(logN), where N is the size of the population. Most of these models assume Markovian evolution and ideas from Markov Processes, Mean Field Theory and Branching Processes have been used to address these questions.
In this work, we study a generalization of the voter model and analyze its finite time as well as asymptotic behaviour. Our generalization has two key aspects. Firstly, we model three types of behaviours, namely, strong-willed, conformist, and rebel. We say that an individual is strong-willed when their opinion is not affected by the opinion of their neighbours. Further, we say that an individual is conformist/rebellious when their opinion is positively/negatively affected by the opinion of their neighbours. These three types of behaviours have been studied in [31] and [14]. In the model proposed in this paper, new individuals are added to the population over time and we allow their initial opinions to depend on the state of the system at the time of joining. We study the asymptotic behaviour of the opinion dynamics of this growing population on a complete network as a function of various system parameters.
Although studying asymptotic behaviour has been the main focus of opinion modeling for many years, in various real-life situations like rumour spread in small communities or online opinion polls, it is important to understand how close does the opinion profile get to the limiting behaviour in a given time-period and what happens in presence of an external influencing agent. Opinion evolution over finite horizon has been studied in [3,31]. The information about the finite time behaviour is particularly important for opinion shaping by advertising and/or influencing agencies. In this paper, in addition to the asymptotic behaviour, we also study the effect of external influence over finite time horizons. Opinion manipulation or opinion shaping has been studied before (See [9,18,30]) but most of the research is focused on determining which nodes of the network should be influenced to have a favourable cascading effect. In this paper, we are interested in understanding whether it is more advantageous to influence closer to the voting deadline or at the beginning, and how does this depend on the three types of behaviour described above. Characterizing optimal influence strategies over finite time horizons in fixed networks is the focus of [16,23,31]. In [23], the authors show that if individuals only exhibit strong-willed behaviour, the optimal strategy is to influence towards the end of the finite time horizon, while if individuals are conformists, it may be optimal to influence towards the beginning of the time horizon. In [31], the authors show that if individuals are predominantly strong-willed/rebel, the optimal strategy is to influence towards the end of the finite time horizon. While [23,31] consider a complete graph between individuals in the network, in [16], the authors study the effect of the nature of the graph (random/fixed) on the nature of optimal influencing strategies. Unlike [16,23,31], where the network of individuals is fixed, in this work we model a system with new individuals entering the network over time. However, we still restrict our analysis to complete graphs, thus when the chosen individuals behave conformist or rebellious they take into account the complete opinion profile of the population at that time.
The main contributions of this work are as follows:
Asymptotic Behaviour: As mentioned above, while the three types of behaviour studied in this paper are similar to that of [14], this paper advances the investigation of asymptotic properties of heterogenous populations by incorporating the feature of growing population with arbitrary but fixed number individuals, extending beyond the single-individual addition model. This gives us an opportunity to explore the dependence of the opinion profile on the ratio of number of people added versus the number of people chosen for opinion update at each step. Our analysis of asymptotic behaviour is divided into three parts:
(i) when the probability of an incoming individuals holding opinion 1 or 0 is independent of the current state of the system.
(ii) when the probability of an incoming individuals holding opinion 1 is proportional to the fraction of individuals with opinion 1 in the system at that time.
(iii) when the probability of an incoming individuals holding opinion 1 is proportional to the fraction of individuals with opinion 0 in the system at that time.
Since the individuals can behave strong-willed with positive probability, in all the three cases the limiting fraction of people with either opinion converges almost surely to a deterministic limit, independent of the initial configuration of opinion. The main result on the limiting opinion profile allows us to study the dependence of the limiting fraction of individuals with either opinion on the ratio of number of individuals being chosen for opinion update at every time-step and the number of individuals being added to the population. We also compare the limiting fraction of individuals with opinion 1 for the three cases and obtain conditions that determine what kind of behaviour of the incoming individuals leads to a higher fraction of people with opinion 1 asymptotically. Further, we observe that in the Central Limit Theorem (CLT) type results for cases (i) and (iii), the critical and superdiffusive regimes do not exist, while case (ii) exhibits all the three regimes. Explicit conditions on system parameters for transitions from subdiffusive, critical and superdiffusive regimes are obtained.
Finite Time Behaviour: We study the system over a time-horizon of T consecutive time-slots out of which external influence is exerted in bT time-slots for some b∈(0,1). The opinion dynamics of the network under external influence tends to move towards a specific direction preferred by the influencer as compared to its organic evolution in the absence of any external influence. An influence strategy is defined by the time-slots in which external influence is exerted. We show that the optimal influence strategy, i.e., the strategy that maximizes the number of nodes with the opinion supported by the influencer at the end of the time-horizon is a function of the number of new nodes joining the network in each time-slot, and the mechanisms (i)–(iii) via which new nodes form their initial opinion.
The paper is organized as follows. In Section 2 we describe the opinion evolution model. In Section 3 we state the results concerning the asymptotic properties of the system. More precisely, we obtain the limiting fraction of people with opinion 1 for various cases and state the fluctuation limit theorems for each case. In Section 4, we use Martingale concentration to show that the random process governing the evolution of fraction of people with either opinion can be approximated by trajectories of an ODE. Using this approximation we analyse the finite-time behaviour of the fraction of people with opinion 1 and its dependence on certain parameters of the system. Further, in Section 4.3, we introduce external influence and obtain optimal influencing strategies to maximize the expected fraction of people with opinion 1 at time T. Finally, Section 5 contains the proofs of the theorems from Sections 3 and 4. We conclude with discussion on the results obtained in this paper and possible future directions in Section 6.
We consider a growing population with binary opinions: 1 or 0 (denoted by 1 and 0 respectively). We start with M0>0 individuals at time t=0. Let Xt denote the fraction of people with opinion 1 at time t. For t≥1, at each discrete time step, the system evolves in two steps:
1. A fixed number of individuals, denoted by Rc(≤M0), are chosen uniformly at random and they update their opinions.
2. A fixed number of individuals, denoted by Ra having opinion 1 with probability αt and 0 with probability 1−αt, are added to the population. We study three cases.
(i) αt=α ∀t≥1 and some fixed α∈[0,1]. That is, probability of the new individuals having opinion 1 remains constant throughout the fixed time interval [0,T].
(ii) αt=αCXt ∀t≥1 and some fixed αC∈[0,1], that is the probability of the new individuals having opinion 1 is proportional to the fraction of individuals of opinion 1 in the population at time t.
(iii) αt=αR(1−Xt) ∀t≥1 and some fixed αR∈[0,1], that is the probability of the new individuals having opinion 1 is proportional to the fraction of individuals of opinion 0 in the population at time t.
We now describe how the opinions of the chosen individual are updated. Define random variables {Ii(t)}1≤i≤Mt, t≥0 taking values in {0,1}, where Ii(t) denotes the opinion of the ith individual at time t. Note that the total number of individuals at time t+1 is given by Mt+1=Mt+Ra. Thus, the population increases linearly and deterministically in t. Define random variables: Yt=Mt∑i=1Ii(t) and Nt=Mt−Yt as total number of people with opinion 1 and the total number of people with opinion 0 at time t respectively. Then, Xt=YtMt. The opinion of a chosen individual j evolves in time-slot according the following transition probabilities.
P(Ij(t+1)=0|Ij(t)=1)=pt,P(Ij(t+1)=1|Ij(t)=1)=1−pt,P(Ij(t+1)=1|Ij(t)=0)=qt,P(Ij(t+1)=0|Ij(t)=0)=1−qt. | (2.1) |
We model three types of behaviour in the population.
– Strong-willed: the chosen individuals are not influenced by peers and change their opinions independent of anyone else in the population. In this case, pt=p and qt=q.
– Conformist: the chosen individuals change their opinion based on the majority opinion at that given time and tend to adopt the "popular" opinion at that time. In this case, pt=p(1−Xt) and qt=qXt.
– Rebel: the chosen individuals change their opinion based on the majority opinion at that given time and tend to adopt the "unpopular" opinion at that time. In this case, pt=pXt and qt=q(1−Xt).
Let λ,μ∈[0,1]. At each time-step t, with probability λ the chosen individuals behave as strong-willed, with probability μ they behave as conformist and with probability 1−λ−μ they behave rebellious. Let Ot+1 be the change in the number of people of opinion 1 from time t to t+1. That is, Yt+1=Yt+Ot+1. Then, we have
Xt+1=Yt+1Mt+1=MtMt+1Xt+Ot+1Mt+1. | (2.2) |
The random variable Ot+1 depends on the two independent processes that we can write as
Ot+1=ORct+1+ORat+1, |
where ORct+1 is the change due to opinion evolution of the chosen individuals and ORat+1 is the change due to the newly added individuals. We have
E[Ot+1|Ft]=E[ORct+1+ORat+1|Ft]=Rc[(1−Xt)qt−Xtpt]+αtRa=−Rc(1−λ−2μ)(p−q)X2t+(Rc[(3μ+λ−2)q−(λ+μ)p])Xt+αtRa+q(1−μ)Rc=Rar(1−λ−2μ)(q−p)X2t+Ra[r[(3μ+λ−2)q−(λ+μ)p])Xt+αt+q(1−μ)r], | (2.3) |
where r=Rc/Ra is the ratio of people chosen and people added at each time step. Note that E[Ot+1|Ft] is a linear function of Xt provided (1) λ+2μ=1 or (2) p=q. The first case is that of a mixed population where probability of a chosen individual being conformist is same as that of her being a rebel, and the second case is inspired from the conventional voter model transition rule. Throughout this paper, we assume the following.
Assumption 1. We assume that the probability of a chosen individual behaving as conformist is same as that of her behaving as a rebel. That is, λ+2μ=1. Further, we assume that Ra>0.
As we shall see, the results for the case p=q can be obtained as a special case under Assumption 1. The linearity of E[Ot+1|Ft] as a function of Xt implies that E[Xt+1|Ft] is linear in Xt. This allows us to give an ODE approximation for the recursion with explicit error bounds. In the next section, we investigate the asymptotic properties of the fraction of people with opinion 1.
We use the stochastic approximation theory to analyse the behaviour of fraction of individuals of opinion 1. Note that the recursion in Eq (5.2) is of the form
xt+1=xt+at(h(xt)+St+1), |
where 1/at is a linear function of t, h is a linear function of Xt (whenever λ+2μ=1), St is a square integrable zero mean martingale and xt is bounded. Therefore the limiting point of the recursion is same as that of the stable limit point of the ODE ˙xt=h(xt) (See Chapter 1 in [2]). Thus, the following is immediate.
Theorem 3.1. Under Assumption 1, Xt→X∗ almost surely as t→∞, where
X∗={α+q(1−μ)r1+(p+q)(1−μ)r for αt=α,q(1−μ)r1−αC+(p+q)(1−μ)r for αt=αCXt,αR+q(1−μ)r1+αR+(p+q)(1−μ)r for αt=αR(1−Xt). | (3.1) |
Note that for large r, that is when the number of individuals chosen at every step for opinion update is much larger than the number of people being added to the population at every step, X∗ is approximately qp+q in all cases. That is, when Rc>>Ra, the asymptotic composition of the opinion in the population does not depend on the initial inclination of people getting added to the population or on the behaviour of people chosen at each step. For small r, in cases αt=α and αR(1−Xt), the limiting fraction of of people with opinion 1 is close to α and αR1+αR respectively, whereas for α=αCXt, it is close to zero. A similar trend is observed when everyone in the population is conformist with probability 1.
Remark 1. For the case p=q, we get
X∗={α+q(1−μ)r1+2q(1−μ)r for p=q and αt=αq(1−μ)r1−αC+2q(1−μ)r for p=q and αt=αCXtαR+q(1−μ)r1+αR+2q(1−μ)r for p=q and αt=αR(1−Xt), |
which turns out to be a special case of Theorem 3.1. This is because the ODE under assumption p=q is same as that obtained under Assumption 1 along with p=q. However, as we shall see the fluctuations of Xt around the limit X∗ have a different behaviour under λ+2μ=1 and p=q and latter cannot be obtained as special case of the former.
In the corollary below we compare the limiting fraction of people with opinion 1 in the three cases and obtain conditions that lead to a larger fraction of people with opinion 1 asymptotically.
Corollary 3.2. Let X∗S,X∗C and X∗R denote the limiting fraction of individuals with opinion 1 asymptotically for cases αt=α,αt=αCXt and αt=αR(1−Xt) respectively. Under Assumption 1,
(i) X∗S≥X∗R if if α≥αR or α≥1−X∗S. In particular, X∗S≥X∗R when αR=α.
(ii) X∗S≥X∗C if α≥αC or α≥X∗S. In particular, X∗S≥X∗C when αC=α.
(iii) X∗C≥X∗R if (1−μ)r(qαC−pαR)−αR+αRαC≥0. In particular, X∗C≥X∗R if αC=1 and q≥p or αC=αR=α≥1+(1−μ)r(p−q).
Remark 2. Note that if αR=0, in case (iii), new people have opinion 0 with probability 1 and therefore X∗C≥X∗R. This is straightforward from Eq (5.5). Similarly, when αC=0, X∗C≤X∗R. By the argument in the proof above, we also get that X∗C≤X∗R whenever αR=1 and p≥q.
Finally, we show that a phase transition in the fluctuation around the limit exists only for the case when αt=αCXt, that is when the probability of new individuals having opinion 1 is directly proportional to the fraction of individuals with opinion 1 at that time. The classification of diffusive, critical and superdiffusive regime is based on the values of parameters r,p,q,μ and αC. In the other two cases viz. αt=α and αt=αR(1−Xt), we only have the diffusive case with √t scaling.
Theorem 3.3. Let X∗S,X∗C and X∗R be as in Corollary 3.2 and suppose Assumption 1 holds.
1. For αt=α, as t→∞
√t(Xt−X∗S)d→N(0,σ), |
where σ=Ra2Ra[r(1−μ)(p+q)+1]−1[r(1−μ)(p−q)(r(1−μ)q+αr(1−μ)(p+q)+1)+r(1−μ)q+α(1−α)].
2. For αt=αR(1−Xt), as t→∞
√t(Xt−X∗R)d→N(0,σR), |
where
σR=Ra[r(1−μ)(p−q)+2α2−αR]2Ra[r(1−μ)(p+q)+1+αR]−1(r(1−μ)q+αRr(1−μ)(p+q)+1+αR)+Ra[r(1−μ)q+αR(1−αR)]2Ra[r(1−μ)(p+q)+1+αR]−1. |
3. For αt=αCXt, as t→∞
(a) if αC<r(1−μ)(p+q)+1−12Ra then
√t(Xt−X∗C)d→N(0,σC), |
where
σC=Ra[r(1−μ)(p−q)+αC]2Ra[r(1−μ)(p+q)+1−αC]−1(r(1−μ)qr(1−μ)(p+q)+1−αC)+Rar(1−μ)q2Ra[r(1−μ)(p+q)+1−αC]−1. |
(b) if αC=r(1−μ)(p+q)+1−12Ra then
√tlogt(Xt−X∗C)d→N(0,σC), |
where σC=[(r(1−μ)(p−q)+αC)(r(1−μ)qr(1−μ)(p+q)+1−αC)+r(1−μ)q].
(c) if
αC>r(1−μ)(p+q)+1−12Ra |
then as as
t→∞,t−Dh(X∗)(Xt−X∗C) |
almost surely converges to a finite random variable, where
−Dh(X∗)=Ra(r(1−μ)(p+q)+1−αC). |
A similar result can be obtained for the case p=q. The proofs of the above theorems are in Section 5.
The scaling limits of this nature can also be obtained for general reinforced stochastic processes, including urn models and reinforced random walk. While we have used stochastic approximation theory, such limiting behaviour can also be obtained by exploiting the martingale structure as done in [15,24] for urn models or using results from [17]. As mentioned before, the random process governing the reinforcement of opinions is similar to the behaviour of a generalized two-colour Pólya urn. The new individuals being added to the population corresponds to adding new balls to the urn independently or based on the composition of the urn at that time. The chosen individuals correspond to multiple drawings of balls from the urn that are then replaced after some re-colouring. In this context, the behaviour of Xt observed here is similar in spirit to that observed in [15,21,24] for the fraction of balls of a given colour in generalized two-colour Pólya urns.
In the next section, we study the same model and obtain optimal influencing strategies over a finite time horizon.
We now analyse the evolution of opinions over a finite time interval [0,T]. We are interested in understanding how the parameters of the system affect the final opinion profile at time T and what kind of influencing strategies result in a larger fraction of people with opinion 1 at time T. The key mathematical idea is to approximate the random process of the fraction of people with a given opinion with an ODE.
It can be shown that under Assumption 1, the iterates of the recursion for Xt remain close to the trajectories of the ODEs given by
dxtdt={−[r(1−μ)(p+q)+1]xt+q(1−μ)r+αM0/Ra+t when αt=α−[r(1−μ)(p+q)+1−αC]xt+q(1−μ)rM0/Ra+t when αt=αCXt−[r(1−μ)(p+q)+1+αR]xt+q(1−μ)r+αRM0/Ra+t when αt=αR(1−Xt). |
Thus it is enough to analyse ODE of the form
dxtdt=−[r(1−μ)(p+q)+1+A]xt+q(1−μ)r+BM0/Ra+t with x0=X0, | (4.1) |
where
A={0 for αt=α−αC for αt=αCXtαR for αt=αR(1−Xt) and B={α for αt=α0 for αt=αCXtαR for αt=αR(1−Xt). |
The solution for ODE in Eq (4.1) is given by:
xt=rq(1−μ)+Br(1−μ)(p+q)+1+A+(x0−rq(1−μ)+Br(1−μ)(p+q)+1+A)(t+1+M0/Ra1+M0/Ra)−(r(1−μ)(p+q)+1+A). | (4.2) |
The following theorem asserts that the recursion Xt remains close to the trajectories of the ODE in Eq (4.1).
Theorem 4.1 (Martingale concentration). Suppose Assumption 1 holds. Let xT denote the solution at time T of the ODE in Eq (4.1). Then for DM0=O(1M0),
P(|XT−xT|≥ϵ+DM0)≤2e−ϵ2CT, |
for some constant C>0.
The constant C depends on various parameters of the system, including the initial population. Figures 1 and 2 illustrate how well the ODE solution tracks the simulated trajectories of the recursion for Xt. In the rest of the paper, we use this approximation to analyse the recursion by studying the ODE solution. We refer to the random process by Xt and the ODE solution by xt.
We first put the above ODE approximation to use for analysing the dependence of the final xT (which approximates the fraction of individuals with opinion 1 at the time of voting) on r, that is the ratio of number of people who may change their opinion at time t and the number of people added to the population at each time-step t. Clearly, the behaviour of xT depends of p,q,x0 and αt. Differentiating the solution of Eq (4.1) at T with respect to r we get
x′T=((A+1)q(1−μ)−B(p+q)(1−μ)(r(1−μ)(p+q)+1+A)2)[1−(τ1τT+1)r(1−μ)(p+q)+1+A]+(x0−rq(1−μ)+Br(1−μ)(p+q)+1+A)[(p+q)(1−μ)(τ1τT+1)r(1−μ)(p+q)+1+Alog(τ1τT+1)], | (4.3) |
where τk=k+M0/Ra. We consider the αt=α case first. In this case,
x′T=(1−μ)(q−α(p+q)(r(1−μ)(p+q)+1)2)[1−(τ1τT+1)r(1−μ)(p+q)+1]+(x0−rq(1−μ)+αr(1−μ)(p+q)+1)[(p+q)(1−μ)(τ1τT+1)r(1−μ)(p+q)+1log(τ1τT+1)]. | (4.4) |
Clearly, for α=qq+p, we get that x′T is positive for x0>qp+q, negative for x0<qp+q and zero for x0=qp+q. For α>qq+p, it is immediate that x′T<0 for
x0<rq(1−μ)+αr(p+q)(1−μ)+1. |
For,
x0>rq(1−μ)+αr(p+q)(1−μ)+1. |
Observe that for T not very small,
|qq+p−α|τurT+1>τur1[(r(p+q)(1−μ)+1)2(rq(1−μ)+αr(p+q)(1−μ)+1−x0)log(τ1τT+1)+|qq+p−α|], |
where
ur=r(1−μ)(p+q)+1. |
Therefore, x′T<0 for all r provided α>qq+p. A similar argument for α<qq+p shows that xT is a non-decreasing function of r. For α=qq+p, we get
x′T=(x0−qp+q)[(p+q)(1−μ)(τ1τT+1)r(1−μ)(p+q)+1log(τ1τT+1)]. |
Therefore,
● for x0>qp+q, xT is a non-increasing function of r.
● for x0=qp+q, xT is a constant function of r.
● for x0<qp+q, xT is a non-decreasing function of r.
Using similar arguments we characterize the behaviour of xT as a function of r for the rest of the cases as well. Table 1 details the behaviour of the final fraction of individuals with opinion 1 as a function of r.
Behaviour of xT as a function of r. | (i) αt=α | (ii) αt=αCXt | (iii) αt=αR(1−Xt) |
xT is a non-increasing function of r. | α>qq+p or α=qq+p<x0 | αC=1 and x0>qp+q | αR>qp or αR=qp,x0>qp+q |
xT is a non-decreasing function of r. | α<qq+p or x0<α=qq+p | αC∈[0,1) or αC=1,x0<qp+q | αR<qp or αR=qp,x0<qp+q |
xT is a constant function of r. | α=qq+p=x0 | αC=1 and x0=qp+q | αR=qp |
In the next section, we state and discuss the main result for the finite time opinion evolution under external influence and optimal strategies for influencing the dynamics to obtain higher number of individuals with opinion 1 at the end of the finite time horizon.
In this section, we study the opinion evolution over a finite time interval [0,T]. We assume that there is an external influencing agency with a limited budget that tries to skew the opinion of the population in their favour at the end of time T. Due to budgetary constraints, the advertising agency can influence the opinion in exactly bT of the T time-slots, where b∈[0,1] is such that bT∈N. The influence is exerted by manipulating the transition probabilities of the Markov process defined in Eq (2.1). That is, if the chosen individuals are being externally influenced in time-slot t, pt=˜p and qt=˜q, else, their opinion evolves as described before. Without loss of generality, we assume that the aim of the advertising agency is to maximise the number of individuals with the opinion 1 at the end of the T time-slots. Similar model of opinion evolution in presence of external influence has been studied for fixed population in [31]. Our aim is to obtain optimal influencing strategies in different regimes depending on the model parameters. In particular, we want to study the dependence on Rc and Ra. We begin by defining influencing strategy and what we mean by optimality here.
Definition 4.2 (Influencing strategy). An influencing strategy S∈[0,1]T is defined as binary string of length T that has exactly bT number of 1s. For all i∈{0,…,T−1} such that Si=1, the transition parameters are pi=˜p and qi=˜q. The strategies to influence in the first bT and the last bT time-slots are denoted by SF and SL respectively.
For two strategies S1 and S2, we write S1≫S2 if influence according to S1 leads to a higher expected number of 1 at the end of time T than the expected number of 1 at the end of time T under S2.
Definition 4.3 (Optimal strategy). A strategy is called optimal if the influence according to that strategy results in a higher expected number of 1 at the end of time T than the expected number of 1 at the end of time T using any other influence strategy.
Thus, an optimal strategy S∗ is such that S∗≫S, where S is any other collection of bT time-slots to be influenced. As we shall see, due to monotonicity, in most cases, influencing the first or the last bT slots is optimal. We assume that the influencing strategy is rational. That is, during influence, the probability of switching from 0 to 1 increases from what it is when the individuals behave strong-willed. Similarly, under rational influence the probability of switching from 1 to 0 decreases. More, precisely, we have the following assumption.
Assumption 2 (Rational influence). We assume that the external influence is such that ˜p<˜q, ˜p<p and q<˜q.
We now state our results for optimal strategies for the cases αt=α, αt=αCXt and αt=αR(1−Xt). Again, a transition in optimality of influencing strategy is observed in the case αt=αCXt at the critical value αC=r(˜p+˜q).
Figures 3 and 4 compare the strategies SL,SF and a strategy S where the slots in the interval [0.4T,0.7T] are influenced. In Figure 5, we compare SL,SF and a split strategy S where the influence is over time-slots in the intervals [0.3T,0.5T] and [0.8T,T]. The figures illustrate that SL is optimal for the cases αt=α and αR(1−Xt) whereas there is a transition in optimality of the strategies for a certain threshold value of αC in the case when α=αCXt.
Theorem 4.4. Suppose δ=r[(˜p+˜q)−(1−μ)(p+q)]=0. Then, under Assumptions 1 and 2,
1. For αt=α, it is optimal to influence in the last bT slots.
2. For αt=αCXt,
(a) if r(˜p+˜q)>αC, it is optimal to influence in the last bT slots.
(b) If r(˜p+˜q)<αC, it is optimal to influence in the first bT slots.
(c) If r(˜p+˜q)=αC, all strategies perform equally well.
3. For αt=αR(1−Xt), it is optimal to influence in the last bT slots.
The main idea is to compare the strategies SL and SF and then get optimality using monotonicity of the ODE solution with respect to the initial conditions. Suppose XLT and XFT denote the corresponding ODE solutions for the influencing strategies SL and SF, respectively. Then, we have
XFT=r(1−μ)q+Br(1−μ)(p+q)+1+A+(x0−r˜q+Br(˜p+˜q)+1+A)[bT+1+M0/Ra1+M0/Ra]−r(˜p+˜q)−1−A×[T+1+M0/RabT+1+M0/Ra]−r(1−μ)(p+q)−1−A+Δ[T+1+M0/RabT+1+M0/Ra]−r(1−μ)(p+q)−1−A, | (4.5) |
where Δ=r˜q+Br(˜p+˜q)+1+A−r(1−μ)q+Br(1−μ)(p+q)+1+A. Similarly,
XLT=r˜q+Br(˜p+˜q)+1+A+(x0−r(1−μ)q+Br(1−μ)(p+q)+1+A)[T(1−b)+1+M0/Ra1+M0/Ra]−r(p+q)−1−A×[T+1+M0/Ra(1−b)T+1+M0/Ra]−r(˜p+˜q)−1−A−Δ[T+1+M0/Ra(1−b)T+1+M0/Ra]−r(˜p+˜q)−1−A. | (4.6) |
Define ˜T=T1+M0Ra and DT=XLT−XFT to be the difference between fraction of people with opinion 1 at time T when under influencing strategies SL and SF. We get
DT=XLT−XFT=Δ[1−(˜T+1(1−b)˜T+1)−r(˜p+˜q)−1−A−(˜T+1b˜T+1)−r(p+q)−1−A]+(x0−r(1−μ)q+Br(1−μ)(p+q)+1+A)((1−b)˜T+1)δ(˜T+1)−r(˜p+˜q)−1−A−(x0−r˜q+Br(˜p+˜q)+1+A)(b˜T+1)−δ(˜T+1)−r(p+q)−1−A. | (4.7) |
To compare SL and SF, it is enough to analyse whether DT is positive or negative. The detailed proof is in Section 5.
We need the assumption δ=0 to ensure the mathematical tractability of the expression for DT. In general, when b is bounded away from 0 and 1 (which is reasonable since we would like to study scenarios where influence is over a non-trivial subset of [0,T]) and T is large, we have
(1−b)˜T+1˜T+1=1−b˜T˜T+1≈1−b and b˜T+1˜T+1≈b. |
Using these approximations, we get
DT≈Δ[1−(1−b)r(˜p+˜q)+1+A−br(p+q)+1+A]+(x0−r(1−μ)q+Br(1−μ)(p+q)+1+A)(1−b)δ(˜T+1)δ(˜T+1)−r(˜p+˜q)−1−A−(x0−r˜q+Br(˜p+˜q)+1+A)b−δ(˜T+1)−δ(˜T+1)−r(p+q)−1−A. |
For large ˜T, the second and the third term are very small. Since for αt=α or αR, r(p+q)+1+A>1, we have
1=(1−b)+b≥(1−b)r(˜p+˜q)−1−A+br(p+q)−1−A, |
which along with Δ>0, implies DT≥0. Combining this with the optimality argument, we get that if the voting happens after a large time T, in case αt=α or αR, it is better to influence towards the end. Also, if r<<1 and αt=α, DT≈0, making all strategies more or less comparable in terms of effectiveness for getting more 1's at the end of time T.
In this section, we prove the results from previous sections. The main tool to prove the results from Section 3 is the stochastic approximation theory. For the results in Section 4, we first prove Theorem 4.1 to show that the discrete dynamics can be approximated well by an O.D.E. and then use the corresponding O.D.E. to show the transition in optimal influence strategy as stated in Theorem 4.4.
We first prove the results establishing asymptotic behaviour of Xt. From Eq (2.2) we have
Xt+1=Mt+1−RaMt+1Xt+E[Ot+1|Ft]Mt+1+Ot+1−E[Ot+1|Ft]Mt+1=Xt+1Mt+1[E[Ot+1|Ft]−RaXt]+St+1Mt+1, | (5.1) |
where St+1=Ot+1−E[Ot+1|Ft] is a zero-mean martingale with respect to {\mathcal Ft=σ(Os;0≤s≤t)}t≥1. We have,
E[Ot+1|Ft]=E[ORct+1+ORat+1|Ft]=Rc[(1−Xt)qt−Xtpt]+αtRa. |
Using this in Eq (5.1) and substituting pt=λp+μp(1−Xt)+(1−λ−μ)pXt and qt=λq+μqXt+(1−λ−μ)q(1−Xt), we get the following recursion.
Xt+1=Xt+1Mt+1h(Xt)+St+1Mt+1, | (5.2) |
where from Eq (2.3) we get that
h(Xt)=E[Ot+1|Ft]−RaXt=−Rc(1−λ−2μ)(p−q)X2t+(Rc[(3μ+λ−2)q−(λ+μ)p]−Ra)Xt+αtRa+q(1−μ)Rc=Rar(1−λ−2μ)(q−p)X2t+Ra[(r[(3μ+λ−2)q−(λ+μ)p]−1)Xt+αt+q(1−μ)r]. |
The recursion for Xt can thus be written as a stochastic approximation scheme (See [2]) and the corresponding ODE is given by
dxtdt=Ra[r(1−λ−2μ)(q−p)x2t+(r[(3μ+λ−2)q−(λ+μ)p]−1)xt+αt+q(1−μ)r]=Ra[r(1−λ−2μ)(q−p)x2t+(r[(3μ+λ−2)q−(λ+μ)p]−1−A)xt+q(1−μ)r+B], | (5.3) |
where A and B are as in Eq (4.1). It is easy to verify that Eq (5.2) satisfies the conditions of a stochastic approximation scheme since the martingale difference is bounded, Xt≤1∀t≥0, h(⋅) is Lipschitz in Xt and the step-size 1/Mt is inverse of a linear function of t. From the stochastic approximation theory, we know that the recursion for Xt converges almost surely to the stable limit points of the ODE, which are given by h(xt)=0. Define
D(x):=∂h∂x=Ra[2r(1−λ−2μ)(q−p)x+r{(3μ+λ−2)q−(λ+μ)p}−1−A]. |
Proof of Theorem 3.1. Under Assumption 1, the corresponding ODE is given by
dxtdt=Ra[−[r(1−μ)(p+q)+1+A]xt+q(1−μ)r+B], |
where r=RcRa. Clearly, xt=rq(1−μ)+Br(1−μ)(p+q)+1+A is a limit point. Further, it is easy to verify that for λ+2μ=1 or p=q, D(x)<0 for all x. Thus, rq(1−μ)+Br(1−μ)(p+q)+1+A is a stable fixed point. Thus, as t→∞, Xt→X∗ almost surely where
X∗={α+q(1−μ)r1+(p+q)(1−μ)r for λ=1−2μ and αt=αq(1−μ)r1−αC+(p+q)(1−μ)r for λ=1−2μ and αt=αCXtαR+q(1−μ)r1+αR+(p+q)(1−μ)r for λ=1−2μ and αt=αR(1−Xt) | (5.4) |
The p=q case mentioned in the remark 1 is obtained in the same way or by simply putting p=q in Eq (5.4) above since the ODE for p=q is a special case of the ODE under Assumption 1. In general, h(xt) is a polynomial of degree 2 in xt. Let ρ1 and ρ2 be the roots h(xt)=0 with ρ1>ρ2. Note that
ρ1ρ2=q(1−μ)r+B(1−λ−2μ)(q−p) |
and
ρ1+ρ2=−(r[(3μ+λ−2)q−(λ+μ)p]−1−A)(1−λ−2μ)(q−p). |
Thus, D(x):=Ra(1−λ−2μ)(q−p)[2x−(ρ1+ρ2)] and we get the following.
1. for the case λ+2μ>1 and q>p or λ+2μ<1 and q<p, ρ1>0 and ρ2<0 as ρ1ρ2<0. Also, D(ρ1)<0 and D(ρ2)>0. So, in these cases, ρ1 is a stable limit point.
2. For the case λ+2μ>1 and q<p or λ+2μ<1 and q>p, ρ1>0 and ρ2>0 as ρ1ρ2>0 and ρ1+ρ2>0. Also, D(ρ1)>0 and D(ρ2)<0. Hence, in these cases, ρ2 is a stable limit point.
While the asymptotic analysis is possible, a martingale argument for ODE approximation as done in Section 4 is not possible when h is non-linear. Further, the ODE ˙x(t)=h(xt) yields fairly complicated solutions and obtaining optimal strategies is non-tractable.
Proof of Corollary 3.2. We first compare X∗S,X∗R.
X∗S−X∗R=α+q(1−μ)r1+(p+q)(1−μ)r−αR+q(1−μ)r1+αR+(p+q)(1−μ)r=α[1+αR+(p+q)(1−μ)r]−αR(1+p(1−μ)r)(1+(p+q)(1−μ)r)(1+αR+(p+q)(1−μ)r)=(α−αR)[1+(p+q)(1−μ)r]+αR[α+q(1−μ)r](1+(p+q)(1−μ)r)(1+αR+(p+q)(1−μ)r)=(α−αR)+αRX∗S(1+αR+(p+q)(1−μ)r) |
Thus, X∗S−X∗R≥0 iff α−αR(1−X∗S)≥0. Therefore, α≥αR or α≥1−X∗S implies X∗S≥X∗R. Next we compare X∗S,X∗C.
X∗S−X∗C=α+q(1−μ)r1+(p+q)(1−μ)r−q(1−μ)r1−αC+(p+q)(1−μ)r=α[1−αC+(p+q)(1−μ)r]−αCq(1−μ)r(1+(p+q)(1−μ)r)(1−αC+(p+q)(1−μ)r)=α[1+(p+q)(1−μ)r]−αC[α+q(1−μ)r](1+(p+q)(1−μ)r)(1−αC+(p+q)(1−μ)r) |
Clearly, X∗S−X∗C≥0 iff α≥αCX∗S. Thus, α≥αC or α≥X∗S implies X∗S−X∗C≥0. Further, when αC=αR=α, X∗S≥X∗C for all α∈[0,1].
For the case αt=αR(1−Xt) and αt=αCXt we get that
X∗C≥X∗R⟺rq(1−μ)r(p+q)(1−μ)+1−αC≥rq(1−μ)+αRr(p+q)(1−μ)+1+αR, |
which holds if and only if
(1−μ)r(qαC−pαR)−αR+αRαC≥0. | (5.5) |
Clearly, this holds for αC=1 and q≥p since p≥pαR. Further, for αC=αR=α, we have X∗C≥X∗R iff α≥1+(1−μ)r(p−q).
We now prove the fluctuation limit theorem.
Proof of Theorem 3.3. We use results from [36]. We first compute Γ=limt→∞E[S2t+1|Ft]. Note that E[S2t+1|Ft]=E[(Ot+1−E[Ot+1|Ft])2|Ft]. We have
E[(Ot+1−E[Ot+1|Ft])2|Ft]=Var[Ot+1|Ft]=Var[ORct+1+ORat+1|Ft]=Var[ORct+1|Ft]+Var[ORat+1|Ft]=Var[Mt∑i=1Oi(t+1)|Ft]+Var[ORat+1|Ft]=αt(1−αt)Ra+Mt∑i=1RcMt((1−Xt)qt+Xtpt)−R2cM2t((1−Xt)qt−Xtpt)2=αt(1−αt)Ra+Rc((1−Xt)qt+Xtpt)−R2cMt((1−Xt)qt−Xtpt)2. |
The term R2cMt((1−Xt)qt−Xtpt)2 goes to zero as t→∞. For pt=λp+μp(1−Xt)+(1−λ−μ)pXt and qt=λq+μqXt+(1−λ−μ)q(1−Xt) we get that limt→∞E[S2t+1|Ft] is same as
limt→∞Ra[(r(1−λ−2μ)(p+q)+A1)X2t+(r((λ+3μ−2)q+(λ+μ)p)+A2)Xt+r(1−μ)q+A3], | (5.6) |
where A1={0 for αt=α−α2C for αt=αCXt−α2R for αt=αR(1−Xt),A2={0 for αt=ααC for αt=αCXt2αR2−αR for αt=αR(1−Xt)
and, A3={α(1−α) for αt=α0 for αt=αCXtαR(1−αR) for αt=αR(1−Xt).
Under Assumption 1, we get
Γ=Ra[{r(1−μ)(p−q)+A2}(r(1−μ)q+Br(1−μ)(p+q)+1+A)+r(1−μ)q+A3], |
We now compute the limiting variance σ. Thus using Theorems 2.1–2.3 from [36] we have:
● For −Dh(X∗)>12, σ=∫∞0e−(−Dh(X∗)−12)uΓe−(−Dh(X∗)−12)udu.
● For −Dh(X∗)=12, σ=limt→∞1logt∫logt0e−(−Dh(X∗)−12)uΓe−(−Dh(X∗)−12)udu.
Therefore, whenever −Dh(X∗)=Ra(r(1−μ)(p+q)+1+A)>12 we have,
σ=∫∞0e−(−Dh(X∗)−12)uΓe−(−Dh(X∗)−12)udu=∫∞0e−2(−Dh(X∗)−12)uΓdu=Γ∫∞0e−2(Ra(r(1−μ)(p+q)+1+A)−12)udu=Γ2Ra(r(1−μ)(p+q)+1+A)−1. |
Thus, with Assumption 1, we get the following.
(a) For αt=α and X∗=r(1−μ)q+αr(1−μ)(p+q)+1, we get Dh(X∗)=−Ra(r(1−μ)(p+q)+1). Therefore,
√t(Xt−X∗)d→t→∞N(0,σ), | (5.7) |
where σ=Ra2Ra[r(1−μ)(p+q)+1]−1[r(1−μ)(p−q)(r(1−μ)q+αr(1−μ)(p+q)+1)+r(1−μ)q+α(1−α)].
(b) For αt=αR(1−Xt) and X∗=r(1−μ)q+αRr(1−μ)(p+q)+1+αR, we get Dh(X∗)=−Ra[r(1−μ)(p+q)+1+αR]. Therefore,
√t(Xt−X∗)d→t→∞N(0,σR), | (5.8) |
where
σR=Ra[r(1−μ)(p−q)+2αR2−αR]2Ra[r(1−μ)(p+q)+1+αR]−1(r(1−μ)q+αRr(1−μ)(p+q)+1+αR)+Rar(1−μ)q+αR(1−αR)2Ra[r(1−μ)(p+q)+1+αR]−1. |
(c) For αt=αCXt and X∗=r(1−μ)qr(1−μ)(p+q)+1−αC
(i) if −Dh(X∗)=Ra[r(1−μ)(p+q)+1−αC]>12 that is αC<r(1−μ)(p+q)+1−12Ra then
√t(Xt−X∗)d→t→∞N(0,σC), | (5.9) |
with σC=Ra2Ra[r(1−μ)(p+q)+1−αC]−1[(r(1−μ)(p−q)+αC)(r(1−μ)qr(1−μ)(p+q)+1−αC)+r(1−μ)q].
(ii) if −Dh(X∗)=Ra[r(1−μ)(p+q)+1−αC]=12 that is αC=r(1−μ)(p+q)+1−12Ra then
√tlogt(Xt−X∗)L→t→∞N(0,σC), | (5.10) |
with σC=[(r(1−μ)(p−q)+αC)(r(1−μ)qr(1−μ)(p+q)+1−αC)+r(1−μ)q].
(iii) if −Dh(X∗)=Ra(r(1−μ)(p+q)+1−αC)<12 that is αC>r(1−μ)(p+q)+1−12Ra then as t→∞, t−Dh(X∗))(Xt−X∗) almost surely converges to a finite random variable.
This completes the proof.
A similar argument can be used to obtain scaling limits for the case p=q as well. We get
limt→∞E[μ2t+1|Ft]=Ra[{2r(1−λ−2μ)q+A1}(r(1−μ)q+B2r(1−μ)q+1+A)2+{2r(λ+2μ−1)q+A2}(r(1−μ)q+B2r(1−μ)q+1+A)+r(1−μ)q+A3]. |
Following the same argument as above yields the following.
(a) For αt=α and X∗=r(1−μ)q+α2r(1−μ)q+1 we get Dh(X∗)=−Ra[2r(1−μ)q+1] and Eq (5.7) holds with
σ=Ra2Ra[2r(1−μ)q+1]−1[2rq(1−λ−2μ)(r(1−μ)q+α2r(1−μ)q+1)2+2rq(λ+2μ−1)(r(1−μ)q+α2r(1−μ)q+1)+r(1−μ)q+α(1−α)]. |
(b) For αt=αR(1−Xt) and X∗=r(1−μ)q+αR2r(1−μ)q+1+αR, we get Dh(X∗)=−Ra[2r(1−μ)q+1+αR] and Eq (5.8) holds with
σR=Ra2Ra[2r(1−μ)q+1+αR]−1[(2r(1−λ−2μ)q−α2R)(r(1−μ)q+αR2r(1−μ)q+1+αR)2+(2r(λ+2μ−1)q+2αR2−αR)(r(1−μ)q+αR2r(1−μ)q+1+αR)+r(1−μ)q+αR(1−αR)]. |
(c) For αt=αCXt and X∗=r(1−μ)q2r(1−μ)q+1−αC. If −Dh(X∗)=Ra(r(1−μ)(p+q)+1−αC)=12 then Eq (5.9) holds with
σC=[(2r(1−λ−2μ)q−α2C)(r(1−μ)q2r(1−μ)q+1−αC)2+(2r(λ+2μ−1)q+αC)(r(1−μ)q2r(1−μ)q+1−αC)+r(1−μ)q]. |
Thus, unlike for the limiting fraction X∗, the limiting second moment or the variance for the case p=q cannot be obtained as a special case of λ+2μ=1.
In this section, we prove results from Section 4. We use a Martingale Concentration Inequality to prove the ODE approximation (from Section 4.1) of the recursion of Xt and then use the approximation to prove Theorem 4.4 for optimal influencing strategy.
Proof of Theorem 4.1. Under Assumption 1, the recurrence is given by
Xt+1=Xt+RaMt+1[−(r(1−μ)(p+q)+1+A)Xt+q(1−μ)r+B]+StMt+1. |
This gives,
E[Xt+1|\mathcal Ft]=Xt+1M′t+1[−(r(1−μ)(p+q)+1+A)Xt+q(1−μ)r+B], |
where M′t+1=Mt+1Ra. Thus,
E[Xt+1|Ft]=Xt(1−r(p+q)(1−μ)+1+AM′t+1)+r(1−μ)q+BM′t+1. |
Define αt=(1−r(p+q)(1−μ)+1+AM′t+1),βt=r(1−μ)q+BM′t+1 and
Zt=Xtt−1∏i=0α−1k−t−1∑i=0βii∏j=0α−1j. |
Note that Zt is an {Ft}t≥0-martingale by definition. For a fixed T, define Yt=(∏T−1k=0αk)Zt is also an {Ft}t≥0-martingale. Using Azuma-Hoeffding inequality, we get
P(|YT−Y0|≥ϵ)≤2exp(−ϵ22T∑i=1c2i), |
where |Yt−Yt−1|≤ct for all 1≤t≤T. We first obtain a reasonable bound on |Yt−Yt−1|. We have
Yt−Yt−1=T−1∏k=0αk(Xtt−1∏k=0α−1k−Xt−1t−2∏k=0α−1k−βt−1t−1∏j=0α−1j)=T−1∏k=tαk(Xt−Xt−1αt−1−βt−1) |
From Lemma 2 in [6], we get |∏T−1k=tαk|≤K(Tt)−C′, where C′=r(p+q)(1−μ)+1+A and K=K(C′,M0/Ra) is a positive constant. Further, |Xt−Xt−1αt−1−βt−1|≤Bt for some constant B>0. Then, T∑t=0c2t=T−2C′T∑t=1K2B2t2C′−2≤K2B2(2C′−1)T. This implies,
P(|YT−Y0|≥ϵ)≤2exp(−ϵ2(2 C^\prime −1)TK2B2). |
Note 2C′−1>0. Equivalently, we have
P(|XT−t−1∑i=0βiT−1∏j=i+1αj−T−1∏k=0αkX0|≥ϵ)≤2exp(−ϵ2(2 C^\prime −1)TK2B2). |
Next, we show that t−1∑i=0βi∏T−1j=i+1αj−∏T−1k=0αkX0 is close to the ODE solution. We have,
T−1∏i=0(1−r(p+q)(1−μ)+1+AM′i+1)∼(T+1+M0/Ra1+M0/Ra)−(r(p+q)(1−μ)+1+A) | (5.11) |
and,
T−1∑i=01M′i+1T−1∏j=i+1(1−r(p+q)(1−μ)+1+AM′j+1)∼(T+1+M0/Ra)−(r(p+q)(1−μ)+1+A)r(p+q)(1−μ)+1+A(T+1+M0/Ra)r(p+q)(1−μ)+1+A−(T+1+M0/Ra)−(r(p+q)(1−μ)+1+A)r(p+q)(1−μ)+1+A(1+M0/Ra)r(p+q)(1−μ)+1+A | (5.12) |
To be precise, the approximations in Eqs (5.11) and (5.12) give
|t−1∑i=0βiT−1∏j=i+1αj−T−1∏k=0αkX0−xT|≤DM0, |
where DM0=O(1M0). We have
P(|XT−t−1∑i=0βiT−1∏j=i+1αj−T−1∏k=0αkX0|≥ϵ+DM0)≤2exp(−ϵ2(2 C^\prime −1)T4K2B2). |
We are now ready to prove Theorem 4.4. In addition to the ODE approximation, we need the following Lemma.
Lemma 5.1 (xt as a function of x0). The ODE solution obtained by solving Eq (4.1) is an increasing function of the initial configuration x0.
Proof. Note that the solution (4.2) of the ODE (4.1) is of the form
f(x)=a1+(x−a1)(b1)−c1, |
where a1,b1>0 and c1≥0 ∀ A and B. Let x1<x2 then
x1<x2⟺x1−a1<x2−a1⟺(x1−a1)(b1)−c1<(x2−a1)(b1)−c1. |
Thus, the ODE solution is an increasing function of the initial configuration x0.
Before we prove Theorem 4.4, note that restriction of a strategy to any subset of [0,T] defines an influencing strategy on that subset. For any strategy S, we denote the strategy on [T1,T2]⊂[0,T] given by the substring of S on [T1,T2] by S|[T1,T2].
Proof of Theorem 4.4. We first compare SF and SL. For ˜p+˜q=(1−μ)(p+q) we get
DT=Δ[1−(˜T+1(1−b)˜T+1)−r(˜p+˜q)−1−A−(˜T+1b˜T+1)−r(˜p+˜q)−1−A+(˜T+1)−r(˜p+˜q)−1−A] | (5.13) |
and
Δ=r˜q+Br(˜p+˜q)+1+A−r(1−μ)q+Br(1−μ)(p+q)+1+A=r(˜q−(1−μ)q)r(˜p+˜q)+1+A. |
Due to rational influence, Δ>0. Define F:[0,1]→[0,1] such that
F(b)=1−((1−b)˜T+1˜T+1)r(˜p+˜q)+1+A−(b˜T+1˜T+1)r(p+q)+1+A+(1˜T+1)r(˜p+˜q)+1+A. |
Differentiating with respect to b once and twice we get
F′(b)=(r(˜p+˜q)+1+A)˜T(˜T+1)r(˜p+˜q)+1+A[((1−b)˜T+1)r(˜p+˜q)+A−(b˜T+1)r(˜p+˜q)+A] | (5.14) |
F″(b)=(r(˜p+˜q)+1+A)(r(˜p+˜q)+A)˜T2(˜T+1)r(˜p+˜q)+1+A[−((1−b)˜T+1)r(˜p+˜q)+A−1−(b˜T+1)r(˜p+˜q)+A−1] | (5.15) |
Clearly, F′(b)=0 for b=1/2. For the case αt=α or αR(1−Xt), A≥0. Thus, F is increasing in b∈[0,1/2) as F′(b)>0 and F is decreasing in b∈(1/2,1]. Since, A≥0 we also have for any b∈[0,1], F″(1/2)<0. Thus, b=12 is a point of maxima for F(b). Since, F(0)=F(1)=0, we get that F(b)≥0 for b∈[0,1]. This implies, DT≥0. Hence, SL≫SF for the case αt=α or αR(1−Xt).
We now address the case αt=αCXt, A=−αC. If r(˜p+˜q)>αC the same argument as above works and we get SL>>SF. If r(˜p+˜q)<αC, we get that F is decreasing in b∈[0,1/2) and F is increasing in b∈(1/2,1]. It is also easy to check that F″(1/2)>0. Thus, b=1/2 is a point of minima for F(⋅). Again, using F(0)=F(1)=0, we conclude that F(b)≤0 for b∈[0,1] and therefore DT≤0. Hence, SL<<SF for the case r(˜p+˜q)<αC. Finally, for the case r(˜p+˜q)=αC, it can be easily verified that DT=0 and therefore SL=SF.
We now prove the optimality using Lemma 5.1. We give the argument for optimality of SL when SL≫SF. A similar argument works for the rest of the cases. Let S be an influencing strategy. Scanning from the left (from the first coordinate), let t1,t1+1 be the first time we encounter a '10' subsequence in S. Since SL≫SF, S|[0,t1+1]<<S′, where S′ is a strategy on [0,t1+1] with S′i=Si for all i≤t1−1 and S′t1=0,S′t1+1=1. In other words, a local swap of 10 to 01 improves the strategy. This combined with the Lemma 5.1 shows that SL is optimal.
We consider a population of M0 individuals on a complete graph, each holding an opinion 1 or 0 at time t=0. At every time-step a fixed number of individuals are added to the population and a fixed number of uniformly chosen individuals update their opinion. New individuals can have opinion 1 with probability that may or may not depend on the current state of the system. Similarly, chosen individuals may update their opinion independently of the state of the system or depending on the fraction of individuals of opinion 1 or 0 at that time. We observed that the limiting fraction of individuals with opinion 1 depends crucially on various parameters that can be adjusted in order to obtain a higher fraction of individuals with opinion 1 in the long run. Further, we demonstrate that the case when the incoming individuals have opinion 1 with probability proportional to the number of individuals with opinion 1 in the population, the fluctuations exhibit all three regimes (diffusive, critical and superdiffusive) of scaling, which is not the case otherwise. On the finite horizon version of the problem, we study optimal influencing strategies to obtain maximum expected fraction of people with opinion 1 at the end of the finite time T. Again, a transition in the type of the influencing strategy is observed only in the case when the incoming individuals have opinion 1 with probability proportional to the number of individuals with opinion 1 in the population. We also remark that we consider a particular method of influencing the population that works by tweaking the transition probabilities of the underlying Markov chain. Another possible way to influence such a system is to add a certain number of bots or stubborn individuals to the system. Further, while modeling evolution of binary opinion for a growing population is an important direction of extension of the existing body of work, the same methods could be employed to study a similar multi-opinion model. One of the important future directions to explore would be to study the transitions in scaling of fluctuations around the limit as well as the transitions in optimality of the influencing strategies of such growing population models on a fixed or random graphs with nearest-neighbour interaction. It would also be interesting to study the same model without the restrictions of Assumption 1. It is clear from Eq (2.3), that this leads to a non-linear structure in the expression for E[Xt+1|Ft], thereby making the problem more challenging. Finally, we remark that similar phase transition for asymptotic behaviour has been observed in reinforced random walks with non-trivial memory effects (for instance, see [13,22]). This would be a very interesting aspect to incorporate in this opinion dynamics model as dropping the Markovian update and introducing some memory would bring these models closer to reality.
The work of Neeraja Sahasrabudhe was supported by the DST-INSPIRE fellowship and SERB-MATRICS MTR/2019/001072 grant.
The authors declare that there is no conflict of interest.
[1] |
L. Becchetti, A. Clementi, E. Natale, Consensus dynamics: An overview, SIGACT News, 51 (2020), 58–104. https://doi.org/10.1145/3388392.3388403 doi: 10.1145/3388392.3388403
![]() |
[2] | V. S. Borkar, Stochastic Approximation: A Dynamical Systems Viewpoint, Gurgaon: Hindustan Book Agency, 2008. https://doi.org/10.1007/978-93-86279-38-5 |
[3] |
F. Bullo, F. Fagnani, B. Franci, Finite-time influence systems and the wisdom of crowd effect, SIAM J. Control Optim., 58 (2020), 636–659. https://doi.org/10.1137/18M1232267 doi: 10.1137/18M1232267
![]() |
[4] |
A. Carro, R. Toral, M. San Miguel, The noisy voter model on complex networks, Sci. Rep., 6 (2016), 24775. https://doi.org/10.1038/srep24775 doi: 10.1038/srep24775
![]() |
[5] | P. Chen, S. Redner, Majority rule dynamics in finite dimensions, Phys. Rev. E, 71 (2005), 036101. https://link.aps.org/doi/10.1103/PhysRevE.71.036101 |
[6] |
K. P. Choi, G. Kaur, T. Wu, On asymptotic joint distributions of cherries and pitchforks for random phylogenetic trees, J. Math. Biol., 83 (2021), 40. https://doi.org/10.1007/s00285-021-01667-2 doi: 10.1007/s00285-021-01667-2
![]() |
[7] |
J. T. Cox, Coalescing random walks and voter model consensus times on the torus in Zd, Ann. Probab., 17 (1989), 1333–1366. https://doi.org/10.1214/aop/1176991158 doi: 10.1214/aop/1176991158
![]() |
[8] |
J. Cruise, A. Ganesh, Probabilistic consensus via polling and majority rules, Queueing Syst., 78 (2014), 99–120. https://doi.org/10.1007/s11134-014-9397-7 doi: 10.1007/s11134-014-9397-7
![]() |
[9] |
F. Dietrich, S. Martin, M. Jungers, Control via leadership of opinion dynamics with state and time-dependent interactions, IEEE Trans. Automat. Contr., 63 (2018), 1200–1207. https://doi.org/10.1109/TAC.2017.2742139 doi: 10.1109/TAC.2017.2742139
![]() |
[10] | R. Durrett, J. P. Gleeson, A. L. Lloyd, P. J. Mucha, F. Shi, D. Sivakoff, et al., Graph fission in an evolving voter model, Proc. Natl. Acad. Sci., 109 (2012), 3682–3687. https://www.pnas.org/doi/abs/10.1073/pnas.1200709109 |
[11] |
S. Galam, Minority opinion spreading in random geometry, Eur. Phys. J. B, 25 (2002), 403–406. https://doi.org/10.1140/epjb/e20020045 doi: 10.1140/epjb/e20020045
![]() |
[12] |
M. T. Gastner, K. Ishida, Voter model on networks partitioned into two cliques of arbitrary sizes, Journal of Physics A: Mathematical and Theoretical, 52 (2019), 505701. https://dx.doi.org/10.1088/1751-8121/ab542f doi: 10.1088/1751-8121/ab542f
![]() |
[13] |
M. González-Navarrete, R. Hernández, Reinforced random walks under memory lapses, J. Stat. Phys., 185 (2021), 3. https://doi.org/10.1007/s10955-021-02826-x doi: 10.1007/s10955-021-02826-x
![]() |
[14] |
M. González-Navarrete, R. Lambert, The diffusion of opposite opinions in a randomly biased environment, J. Math. Phys., 60 (2019), 113301. https://doi.org/10.1063/1.5095762 doi: 10.1063/1.5095762
![]() |
[15] | R. Gouet, Martingale functional central limit theorems for a generalized pólya urn, Ann. Probab., 21 (1993), 1624–1639. http://www.jstor.org/stable/2244591 |
[16] |
A. Gupta, S. Moharir, N. Sahasrabudhe, Influencing opinion dynamics in networks with limited interaction, IFAC-PapersOnLine, 54 (2021), 684–689. https://doi.org/10.1016/j.ifacol.2021.06.130 doi: 10.1016/j.ifacol.2021.06.130
![]() |
[17] | P. Hall, C. C. Heyde, Martingale Limit Theory and its Applications, New York: Academic Press, 1980. https://doi.org/10.1016/C2013-0-10818-5 |
[18] |
Q. He, X. Wang, B. Yi, F. Mao, Y. Cai, M. Huang, Opinion maximization through unknown influence power in social networks under weighted voter model, IEEE Syst. J., 14 (2020), 1874–1885. https://doi.org/10.1109/JSYST.2019.2922373 doi: 10.1109/JSYST.2019.2922373
![]() |
[19] |
R. A. Holley, T. M. Liggett, Ergodic theorems for Weakly Interacting infinite systems and the voter model, Ann. Probab., 3 (1975), 643–663. https://doi.org/10.1214/aop/1176996306 doi: 10.1214/aop/1176996306
![]() |
[20] | A. Jadbabaie, A. Makur, E. Mossel, R. Salhab, Inference in opinion dynamics under social pressure, IEEE Trans. Automat. Contr., (2022), 1–15. https://doi.org/10.1109/TAC.2022.3191791 |
[21] |
S. Janson, Functional limit theorems for multitype branching processes and generalized pólya urns, Stoch. Process. Appl., 110 (2004), 177–245. https://doi.org/10.1016/j.spa.2003.12.002 doi: 10.1016/j.spa.2003.12.002
![]() |
[22] |
N. Kubota, M. Takei, Gaussian fluctuation for superdiffusive elephant random walks, J. Stat. Phys, 177 (2019), 1157–1171. https://doi.org/10.1007/s10955-019-02414-0 doi: 10.1007/s10955-019-02414-0
![]() |
[23] | B. Kumar, N. Sahasrabudhe, S. Moharir, On influencing opinion dynamics over finite time horizons, in The 23rd International Symposium on Mathematical Theory of Networks and Systems, Hong kong, 2018. |
[24] |
L. Laulin, A martingale approach for pólya urn processes, Electron. Commun. Probab., 25 (2020), 1–13. https://doi.org/10.1214/20-ecp321 doi: 10.1214/20-ecp321
![]() |
[25] |
M. Mobilia, A. Petersen, S. Redner, On the role of zealotry in the voter model, J. Stat. Mech. Theory Exp., 2007 (2007), P08029. https://dx.doi.org/10.1088/1742-5468/2007/08/P08029 doi: 10.1088/1742-5468/2007/08/P08029
![]() |
[26] |
A. Mukhopadhyay, R. R. Mazumdar, R. Roy, Voter and majority dynamics with biased and stubborn agents, J. Stat. Phys., 181 (2020), 1239–1265. https://doi.org/10.1007/s10955-020-02625-w doi: 10.1007/s10955-020-02625-w
![]() |
[27] | T. Nakata, H. Imahayashi, M. Yamashita, Probabilistic local majority voting for the agreement problem on finite graphs, in Computing and Combinatorics, Berlin, Heidelberg: Springer, 1627 (1999), 330–338. https://doi.org/10.1007/3-540-48686-0-33 |
[28] |
H. Noorazar, Recent advances in opinion propagation dynamics: a 2020 survey, Eur. Phys. J. Plus, 135 (2020), 521. https://doi.org/10.1140/epjp/s13360-020-00541-2 doi: 10.1140/epjp/s13360-020-00541-2
![]() |
[29] | S. Redner, Reality-inspired voter models: A mini-review, C. R. Phys., 20 (2019), 275–292. https://www.sciencedirect.com/science/article/pii/S1631070519300325 |
[30] | G. Romero Moreno, E. Manino, L. Tran-Thanh, M. Brede, Zealotry and influence maximization in the voter model: When to target partial zealots?, in Complex Networks XI, Cham: Springer, (2020), 107–118. https://doi.org/10.1007/978-3-030-40943-2-10 |
[31] | A. Saxena, B. Kumar, A. Gupta, N. Sahasrabudhe, S. Moharir, Influencing opinions of heterogeneous populations over finite time horizons, in 2021 International Conference on COMmunication Systems and NETworkS, Bangalore, (2021), 474–482. https://doi.org/10.1109/COMSNETS51098.2021.9352905 |
[32] |
S. Singh, F. Alajaji, B. Gharesifard, Consensus using a network of finite memory p{ó}lya urns, IEEE Syst. Control. Lett., 6 (2022), 2780–2785. https://doi.org/10.1109/LCSYS.2022.3177428 doi: 10.1109/LCSYS.2022.3177428
![]() |
[33] | A. Sîrbu, V. Loreto, V. D. P. Servedio, F. Tria, Opinion Dynamics: Models, Extensions and External Effects, Cham, Springer, (2017), 363–401. https://doi.org/10.1007/978-3-319-25658-0-17 |
[34] | V. Sood, S. Redner, Voter model on heterogeneous graphs, Phys. Rev. Lett., 94 (2005), 178701. https://link.aps.org/doi/10.1103/PhysRevLett.94.178701 |
[35] |
E. Yildiz, A. Ozdaglar, D. Acemoglu, A. Saberi, A. Scaglione, Binary opinion dynamics with stubborn agents, ACM Trans. Econ. Comput., 1 (2013), 1–30. https://doi.org/10.1145/2538508 doi: 10.1145/2538508
![]() |
[36] |
L. X. Zhang, Central limit theorems of a recursive stochastic algorithm with applications to adaptive designs, Ann. Appl. Probab., 26 (2016), 3630–3658. https://doi.org/10.1214/16-AAP1187 doi: 10.1214/16-AAP1187
![]() |
Behaviour of xT as a function of r. | (i) αt=α | (ii) αt=αCXt | (iii) αt=αR(1−Xt) |
xT is a non-increasing function of r. | α>qq+p or α=qq+p<x0 | αC=1 and x0>qp+q | αR>qp or αR=qp,x0>qp+q |
xT is a non-decreasing function of r. | α<qq+p or x0<α=qq+p | αC∈[0,1) or αC=1,x0<qp+q | αR<qp or αR=qp,x0<qp+q |
xT is a constant function of r. | α=qq+p=x0 | αC=1 and x0=qp+q | αR=qp |
Behaviour of xT as a function of r. | (i) αt=α | (ii) αt=αCXt | (iii) αt=αR(1−Xt) |
xT is a non-increasing function of r. | α>qq+p or α=qq+p<x0 | αC=1 and x0>qp+q | αR>qp or αR=qp,x0>qp+q |
xT is a non-decreasing function of r. | α<qq+p or x0<α=qq+p | αC∈[0,1) or αC=1,x0<qp+q | αR<qp or αR=qp,x0<qp+q |
xT is a constant function of r. | α=qq+p=x0 | αC=1 and x0=qp+q | αR=qp |