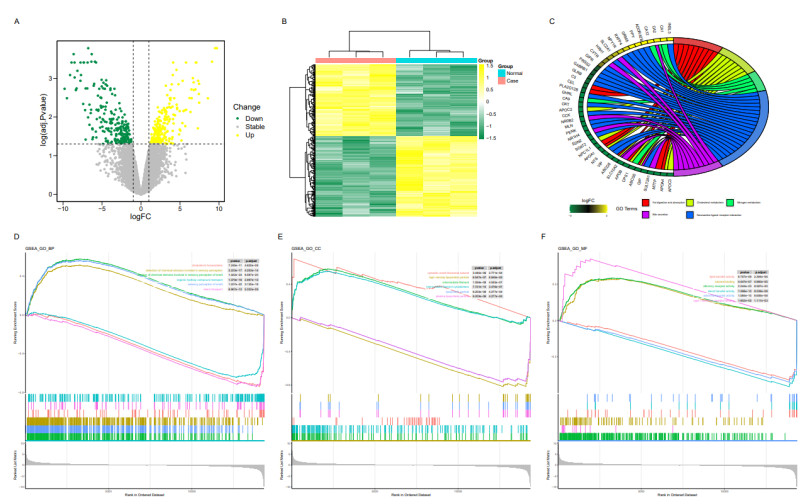
We considered the simulation of isentropic flow in pipelines and pipe networks. Standard operating conditions in pipe networks suggested an emphasis to simulate low Mach and high friction regimes—however, the system was stiff in these regimes and conventional explicit approximation techniques proved quite costly and often impractical. To combat these inefficiencies, we developed a novel asymptotic-preserving scheme that was uniformly consistent and stable for all Mach regimes. The proposed method for a single pipeline followed the flux splitting suggested in Haack et al., in which the flux was separated into stiff and non-stiff portions then discretized in time using an implicit-explicit approach. The non-stiff part was advanced in time by an explicit hyperbolic solver; we opted for the second-order central-upwind finite volume scheme. The stiff portion is advanced in time implicitly using an approach based on Rosenbrock-type Runge-Kutta methods, which ultimately reduced this implicit stage to a discretization of a linear elliptic equation. To extend to full pipe networks, the scheme on a single pipeline was paired with coupling conditions defined at pipe-to-pipe intersections to ensure a mathematically well-posed problem. We showed that the coupling conditions remained well-posed at the low Mach/high friction limit—which, when used to define the ghost cells of each pipeline, resulted in a method that was accurate across these intersections in all regimes. The proposed method was tested on several numerical examples and produced accurate, non-oscillatory results with run times independent of the Mach number.
Citation: Michael T. Redle, Michael Herty. An asymptotic-preserving scheme for isentropic flow in pipe networks[J]. Networks and Heterogeneous Media, 2025, 20(1): 254-285. doi: 10.3934/nhm.2025013
[1] | Yong-hong Hu, Xue-ying Wang, Xi-wen Zhang, Jian Chen, Fu Li . Investigation of the mechanisms and experimental verification of Shao yao gan cao decoction against Sphincter of Oddi Dysfunction via systems pharmacology. Mathematical Biosciences and Engineering, 2022, 19(12): 13374-13398. doi: 10.3934/mbe.2022626 |
[2] | Ze-Yu Zhang, Zhu-Jun Mao, Ye-ping Ruan, Xin Zhang . Computational identification of Shenshao Ningxin Yin as an effective treatment for novel coronavirus infection (COVID-19) with myocarditis. Mathematical Biosciences and Engineering, 2022, 19(6): 5772-5792. doi: 10.3934/mbe.2022270 |
[3] | Jingxi Xu, Jiangtao Li . Construction of a three commitment points for S phase entry cell cycle model and immune-related ceRNA network to explore novel therapeutic options for psoriasis. Mathematical Biosciences and Engineering, 2022, 19(12): 13483-13525. doi: 10.3934/mbe.2022630 |
[4] | Li Li, Xueqing Wang, Xiaoting Liu, Rui Guo, Ruidong Zhang . Integrative analysis reveals key mRNAs and lncRNAs in monocytes of osteoporotic patients. Mathematical Biosciences and Engineering, 2019, 16(5): 5947-5971. doi: 10.3934/mbe.2019298 |
[5] | Jun Hyung Bae, Sang-Mook Lee . Investigation of SIS epidemics on dynamic network models with temporary link deactivation control schemes. Mathematical Biosciences and Engineering, 2022, 19(6): 6317-6330. doi: 10.3934/mbe.2022295 |
[6] | Qianqian Zheng, Jianwei Shen, Lingli Zhou, Linan Guan . Turing pattern induced by the directed ER network and delay. Mathematical Biosciences and Engineering, 2022, 19(12): 11854-11867. doi: 10.3934/mbe.2022553 |
[7] | Rongxing Qin, Lijuan Huang, Wei Xu, Qingchun Qin, Xiaojun Liang, Xinyu Lai, Xiaoying Huang, Minshan Xie, Li Chen . Identification of disulfidptosis-related genes and analysis of immune infiltration characteristics in ischemic strokes. Mathematical Biosciences and Engineering, 2023, 20(10): 18939-18959. doi: 10.3934/mbe.2023838 |
[8] | Marios G. Krokidis, Themis P. Exarchos, Panagiotis Vlamos . Data-driven biomarker analysis using computational omics approaches to assess neurodegenerative disease progression. Mathematical Biosciences and Engineering, 2021, 18(2): 1813-1832. doi: 10.3934/mbe.2021094 |
[9] | Jiaxin Luo, Lin Wu, Dinghui Liu, Zhaojun Xiong, Linli Wang, Xiaoxian Qian, Xiaoqiang Sun . Gene regulatory network analysis identifies key genes and regulatory mechanisms involved in acute myocardial infarction using bulk and single cell RNA-seq data. Mathematical Biosciences and Engineering, 2021, 18(6): 7774-7789. doi: 10.3934/mbe.2021386 |
[10] | Ana Costa, Nuno Vale . Strategies for the treatment of breast cancer: from classical drugs to mathematical models. Mathematical Biosciences and Engineering, 2021, 18(5): 6328-6385. doi: 10.3934/mbe.2021316 |
We considered the simulation of isentropic flow in pipelines and pipe networks. Standard operating conditions in pipe networks suggested an emphasis to simulate low Mach and high friction regimes—however, the system was stiff in these regimes and conventional explicit approximation techniques proved quite costly and often impractical. To combat these inefficiencies, we developed a novel asymptotic-preserving scheme that was uniformly consistent and stable for all Mach regimes. The proposed method for a single pipeline followed the flux splitting suggested in Haack et al., in which the flux was separated into stiff and non-stiff portions then discretized in time using an implicit-explicit approach. The non-stiff part was advanced in time by an explicit hyperbolic solver; we opted for the second-order central-upwind finite volume scheme. The stiff portion is advanced in time implicitly using an approach based on Rosenbrock-type Runge-Kutta methods, which ultimately reduced this implicit stage to a discretization of a linear elliptic equation. To extend to full pipe networks, the scheme on a single pipeline was paired with coupling conditions defined at pipe-to-pipe intersections to ensure a mathematically well-posed problem. We showed that the coupling conditions remained well-posed at the low Mach/high friction limit—which, when used to define the ghost cells of each pipeline, resulted in a method that was accurate across these intersections in all regimes. The proposed method was tested on several numerical examples and produced accurate, non-oscillatory results with run times independent of the Mach number.
Hirschsprung disease (HSCR) is a congenital intestinal neuropathy that results in functional intestinal obstruction due to distal intestinal nerve derangement [1]. The disease occurs in approximately 1 in 5,000 live births [2]. HSCR has multifactorial causes, with RET [3] being the first gene identified to be involved in HSCR. The role of gene abnormalities, including EDNRB [4], EDN3 [5], ECE1 [6], SOX10 [7], PHOX2B [8] and other genes in the development of the disease, is gradually being explored. Clinical manifestations usually include delayed expulsion of meconium in newborns or severe constipation in older infants and children. The gold standard for the diagnosis of HSCR is the histological evaluation of tissue obtained from a deep rectal biopsy, showing a complete absence of submucosal (and intestinal muscle) ganglion cells [2]. Current treatment involves surgical removal of the intestine to eliminate the area where the enteric nervous system is missing [9]. Despite the removal of the diseased bowel segment, postoperative abnormalities in gastrointestinal function, such as constipation and bowel incontinence, are present [10]. Therefore, the search for treatment modalities to improve outcomes is of clinical importance.
Cistanche deserticola Y.C. Ma has long been used for medicinal purposes in China for a long time [11]. Ancient Chinese texts suggest that it can cure dryness and stagnation of the bowels in the elderly. One of these components, echinacoside (ECH), is gaining more and more attention as research progresses. Experimental studies strongly suggest that ECH exhibits a variety of beneficial pharmacological activities associated with anti-inflammatory, anti-endoplasmic reticulum stress, anti-apoptotic and neuroprotective effects [12]. ECH has been found to reverse myocardial remodeling and improve heart function [13]. In terms of nerve protection, ECH protects dopaminergic neurons by regulating the IL-6/JAK2/STAT3 pathway [14], and its metabolites may have neuroprotective activity and may directly complement neurotransmitter deficiencies [15]. Additionally, ECH attenuates lipopolysaccharide (LPS) induced inflammation and apoptosis in rat intestinal epithelial cells by inhibiting the mTOR/STAT3 pathway [16], and it can inhibit the NLRP3 inflammasome signaling pathway [17].
There are several challenges in managing HSCR, and identifying effective treatments is crucial for improving patient outcomes [18]. Stem cell therapy shows promise as a non-surgical alternative [19], but the limited proliferation and differentiation capacity of enteric neuronal stem cells presents a challenge [20]. Moreover, patients with HSCR often experience intestinal inflammation, which can lead to a poor long-term prognosis and the need for a reoperation [21,22]. What is more important to note is that children with HSCR are at risk of a potentially life-threatening inflammatory process [23]. Given its neuroprotective and anti-inflammatory properties, ECH may have potential as a treatment for HSCR. To explore this possibility, we conducted a network pharmacology analysis to assess the feasibility of using ECH in both clinical and experimental settings.
The HSCR-associated genes consist of two parts: the differential genes obtained through differential analysis of the normal and disease groups, respectively, and the other part collected through several databases. We downloaded microarray gene expression data from GSE96854, which included three normal and three disease samples from the Gene Expression Omnibus database (GEO, https://www.ncbi.nlm.nih.gov/geo/). We used the "limma" R package [24] (version 3.52.2) to identify differentially expressed genes (DEGs) between the disease and normal groups. Cut-off values for DEGs were set to |log2 (Fold Change) | > 1, with an adjusted P value < 0.05. The results are presented as volcano plots and heat maps. We collected the disease-associated genes from multiple databases. In the OMIM (https://omim.org/) database [25], we searched for "hirschsprung disease" and obtained 14 related genes. The disease code C0019569 was searched in the "DisGeNET" (https://www.disgenet.org/) database [26,27], and 55 related genes were obtained by using the screening criteria of Score_gda > 0.1. A search of the "Genecard" database [28] for HSCR yielded 983 results, which were further screened based on the criterion of score > 10, resulting in 385 genes. Finally, we searched the NCBI database (https://www.ncbi.nlm.nih.gov/) for "Hirschsprung's disease AND Homo sapiens" and obtained 96 genes.
The structural model of ECH was obtained via a download from the PubChem database (https://pubchem.ncbi.nlm.nih.gov/). We used three different databases, including PharmMapper [29] (http://lilab-ecust.cn/pharmmapper/), Drugbank [30] (https://go.drugbank.com/) and TargetNet [31] (http://targetnet.scbdd.com/), to predict the targets of drug action. And, all targets were screened according to " Homo sapiens ". After combining the results from the three databases, we obtained 93 ECH targets.
For the enrichment analysis of related genes, we used the "clusterProfiler" R package [32] (version 4.4.4) in the Gene Ontology (GO) [33] and Kyoto Encyclopedia of Genes and Genomes (KEGG) [34] databases. For the Gene Set Enrichment Analysis (GSEA) algorithm enrichment analysis, we selected results based on the following criteria: |NES| > 1, NOM P value < 0.05 and FDR (adjusted P value) < 0.25. Additionally, we applied a screening criterion of adjusted P value < 0.05 for the enrichment results. To visualize the results, we employed the enrichplot R package (version 1.16.2) and ggpubr R package (version 0.4.0).
The protein-protein interaction (PPI) networks were identified automatically by using Search Tool for the Retrieval of Interacting Genes/Proteins (version 11.5; https://string-db.org/). The setting parameters were as follows: "Homo sapiens", and the minimum required interaction score is "medium confidence (0.4)". The data for the construction of the ceRNA network map were obtained using the "multiMiR" R package [35] (version 1.18.0). And, we screened the validated miRNAs as the final results. Cytoscape software (version 3.9.1) was used for visualization.
By taking intersections of HSCR-associated genes and targets from ECH, we obtained eight potential binding locations. First, we downloaded the structures of the eight targets in the PDB database [36] (https://www.rcsb.org/). Then, we used PyMOL software [37] to perform the pre-processing steps, such as separating single chains and removing water. We downloaded the 2D structure of ECH in sdf format from the PubChem database (https://pubchem.ncbi.nlm.nih.gov/compound/5281771), and then converted it to pdb format for pre-processinig with PyMOL. The gridbox parameters were obtained in the following two ways before docking. (1) The ligand is present in the receptor file, and the gridbox parameters are obtained by ligand expansion using PyMOL's plugin getbox. (2) No ligand in the receptor file or no viable docking site can be obtained by ligand expansion: in this case, we chose to include all receptor molecules in the gridbox for docking. Using the pre-processed ligands and receptors, we performed molecular docking according to the official AutoDock tutorial in AutoDock-GPU [38]. Result analysis was performed by using mgltools [39]. The visualization of the molecular docking results was implemented in PyMOL.
Exploring and visualizing mutations in target genes from multiple types of cancer by the cBioPortal for Cancer Genomics [40] (http://www.cbioportal.org). Gene expression in tissues was analyzed by obtaining immunohistochemical results from the Human Protein Atlas [41] (https://www.proteinatlas.org/) database. The links to download the original images are as follows: CA1 (https://www.proteinatlas.org/ENSG00000133742-CA1/tissue/rectum#img), CA2 (https://www.proteinatlas.org/ENSG00000104267-CA2/tissue/colon#img), CA9 (https://www.proteinatlas.org/ENSG00000107159-CA9/tissue/small+intestine#img), CA12 (https://www.proteinatlas.org/ENSG00000074410-CA12/tissue/rectum#img), DNA methyltransferase 1 (DNMT1) (https://www.proteinatlas.org/ENSG00000130816-DNMT1/tissue/colon#img), regulating synaptic membrane exocytosis 2 (RIMS2) (https://www.proteinatlas.org/ENSG00000176406-RIMS2/tissue/colon#img), RPGRIP1L (https://www.proteinatlas.org/ENSG00000103494-RPGRIP1L/tissue/small+intestine#img) and zinc finger E-box-binding homeobox 2 (ZEB2) (https://www.proteinatlas.org/ENSG00000169554-ZEB2/tissue/cerebral+cortex#img).
All data processing and analysis were performed in R software (version 4.2.1) by RStudio. Statistical analysis was calculated using the built-in functions of the R package. The main statistical tests were P value and adjusted P value. All statistical P values were two-sided, and P < 0.05 was considered statistically significant.
The study was based on open-source data from multiple databases. Ethical approval has been provided for the patients involved in these databases. Therefore, there are no ethical issues with this article.
To investigate in depth the key genes causing HSCR, we obtained a total of 471 differential genes using the "limma" package in the expression matrix of GSE96854 data between healthy and diseased individuals. Of these, 223 were highly expressed genes in patients relative to the control group, and 248 were lowly expressed genes (Figure 1A). A heat map has been used to show the expression of differential genes in different groups, and the clustering results show that differential genes can distinguish well between diseased and normal individuals (Figure 1B). To better understand the mechanism of differential genes on disease development, we further explored the function of expressed genes by conducting enrichment analysis. The KEGG results showed that fat digestion and absorption, cholesterol metabolism, nitrogen metabolism, neuroactive ligand-receptor interaction and bile secretion were the significant pathways (Figure 1C). Through further analysis using the GSEA enrichment method, we could find that the pathways of Biological Process (BP) in highly expressed genes were associated with the detection of chemical stimuli involved in the sensory perception of smell, the sensory perception of smell and the detection of chemical stimuli involved in sensory perception. For the lowly expressed genes, the enrichment results showed that they were mainly involved in cholesterol homeostasis, sterol transport and organic hydroxy compound transport (Figure 1D). The top three terms of highly expressed genes of Cellular Component (CC) were intermediate filament, intermediate filament cytoskeleton and cytosolic small ribosomal subunit. Correspondingly, the lowly expressed genes were mainly concentrated in plasma lipoprotein particles, lipoprotein particles and high-density lipoprotein particles (Figure 1E). There were also significant differences in the Molecular Function (MF) pathways between the differentially expressed genes. Olfactory receptor activity, type I interferon receptor binding and odorant binding were mainly pathways concentrated in the diseased group. For the normal group, the top three enrichment results were lipid transfer activity, sterol transfer activity and sterol transporter activity (Figure 1F). The results of the enrichment analysis reveal possible mechanisms of intestinal dysfunction in disease states, which are closely related to clinical manifestations. We then constructed a PPI network using the STRING database to explore the interrelationships between proteins. The top 30 genes based on the degree algorithm were further demonstrated using the cytoHubba plug-in in Cytoscape (Supplemental Figure S1A). The complete protein network interaction map can be seen in the Supplemental Figure S1B.
In addition to the previous differential analysis to obtain possible potential pathogenic genes, we further obtained 461 genes through GeneCards, OMIM, DisGeNET and NCBI database collections (Figure 2A). Based on the enrichment results from the database genes, we further understood the mechanism of the disease. The top five terms in Biological Process were axon development, gland development, axonogenesis, embryonic organ development and gliogenesis (Figure 2B). The results of Cellular Component were mainly involved in the neuronal cell body, MKS complex, ciliary transition zone, membrane raft and membrane microdomain (Figure 2C). Signaling receptor activator activity, receptor ligand activity, G protein-coupled receptor binding, DNA-binding transcription factor binding and growth factor activity were the top five terms for Molecular Function (Figure 2D). The KEGG enrichment results, on the other hand, revealed that these genes play important roles in multiple pathways. The first five pathways were microRNAs in cancer, breast cancer, EGFR tyrosine kinase inhibitor resistance, PI3K-Akt signaling pathway and signaling pathways regulating pluripotency of stem cells (Figure 2E). We also mapped PPI networks for these 461 genes through the STRING database (Supplemental Figure S2). We combined the differential genes obtained from the differential analysis and the genes from the database collection as HSCR-associated genes for subsequent analysis. The list of genes is provided in Supplementary Materials.
TargetNet, Drugbank and PharmMapper databases were used to determine the targets of ECH, and we obtained a total of 93 targets (Figure 3A). The interaction of these targets with each other was shown by the PPI networks (Figure 3B). We further used GO enrichment analysis to predict the possible molecular functions of the targets (Figure 3C). Carbonate dehydratase activity, with its upstream signaling pathway, was the most prominent signaling pathway among these targets, and nuclear receptor activity also played an important role. The results of KEGG enrichment showed that the main pathways involved in the targets were nitrogen metabolism, prostate cancer, proximal tubule bicarbonate reclamation, antifolate resistance and the apelin signaling pathway (Figure 3D). Based on the enrichment results, we can infer the pharmacological mechanism of ECH.
By intersection analysis of ECH targets with HSCR-associated genes, we obtained eight potential action points (Supplemental Figure S3). They were RPGRIP1L, DNMT1, ZEB2, CA1, CA2, CA9, CA12 and RIMS2. The structural details of these targets for molecular docking are shown in the Table 1. Following the parameters in the methodology section, we performed molecular docking of the eight targets (Figure 4A–H). Information on the position and energy of molecular docking is shown in Table 2. These docking sites are the optimal results for multiple computational docking, predicting that, once the drug reaches the target site, it will bind to a specific target and perform a physiological function. The yellow dotted lines represent the hydrogen bonds in the drug receptor complex.
Target | Identifier | Method | Resolution | Chain | Positions |
CA1 | 7Q0D | X-ray | 1.24 Å | A/B | 1-261 |
CA2 | 1CIL | X-ray | 1.60 Å | A | 2-260 |
CA9 | 6RQQ | X-ray | 1.28 Å | A/C | 140-395 |
CA12 | 5MSA | X-ray | 1.20 Å | A/B/C/D | 30-291 |
DNMT1 | 6X9J | X-ray | 1.79 Å | A | 729-1600 |
RIMS2 | 1V27 | NMR | - | A | 807-934 |
RPGRIP1L | 2YRB | NMR | - | A | 595-737 |
ZEB2 | 2DA7 | NMR | - | A | 647-704 |
Target | Npts | Spacing | Affinity |
CA1 | 60, 60, 60 | 0.375 | -13.30 |
CA2 | 48, 48, 48 | 0.375 | -13.13 |
CA9 | 126,120,126 | 0.397 | -12.65 |
CA12 | 46, 51, 39 | 0.375 | -13.38 |
DNMT1 | 100, 80, 70 | 1.000 | -7.54 |
RIMS2 | 100,100,100 | 0.375 | -13.84 |
RPGRIP1L | 80,126,126 | 0.450 | -13.00 |
ZEB2 | 72,126,114 | 0.375 | -12.85 |
Through molecular docking, we further identified potential therapeutic targets. To investigate the expression of the targets in the gastrointestinal tract, we obtained the immunohistochemistry results for eight targets in the Human Protein Atlas project database. Among them, CA1, CA2, CA9 and CA12 have obvious gastrointestinal tissue specificity. The other three targets, including DNMT1, RIMS2 and RPGRIP1L, although less tissue-specific, were also positive in the gastrointestinal tract. For CA1, it exhibited selective cytoplasmic and nuclear expression in the large intestine. From the staining results, the staining of nuclei in endocrine cells was high and the intensity was strong (Figure 5A). Meanwhile, CA2 staining in the nuclei of endocrine cells, enterocytes and goblet cells showed their high incidence of occurrence, and it also showed strong positivity in enterocyte microvilli (Figure 5B). CA9 expression was concentrated in cytoplasmic and membranous glandular cells (Figure 5C). The staining of CA12 in endocrine cells, enterocytes, goblet cells and peripheral nerves/ganglia in rectum tissue was high and the intensity was strong (Figure 5D). For DNMT1, although its staining in the nuclei of glandular cells was medium, the intensity was strong (Figure 5E). RIMS2 showed only low staining results in glandular cells in colon tissue (Figure 5F). The cytoplasmic/membranous staining of RPGRIP1L in glandular cells was medium and the intensity was moderate (Figure 5G). In the HPA database, the tissue specificity of ZEB2 was mainly expressed in the brain (Figure 5H).
Based on the previous enrichment results showing close association with cancer pathways, we further explored the mutation of eight genes in a variety of mutations in multiple cancers. In terms of mutations, RIMS2 had the highest mutation rate of 9% and amplification was the predominant mutation type for RIMS2. CA1, CA2, CA9, CA12, RPGRIP1L DNMT1and ZEB2 were more conservative, with all seven genes having a mutation rate of less than 5% (Figure 6A). To gain insight into the regulatory mechanisms of target genes, we assembled validated target miRNAs to construct a ceRNA network map (Figure 6B). Green, yellow and red indicate one evidence, two evidences and greater than or equal to three evidences of association between the miRNA and the target gene, respectively. And, detailed miRNA information is provided in the Supplementary Material. Eight core genes were analyzed for enrichment, and the enrichment results were visualized using Cytoscape (Figure 6C). The green color represents the BP enrichment results. Yellow represents the CC enrichment results, and blue represents MF enrichment result. The pathways with the most significant enrichment results for BP and MF are one-carbon metabolic processes and carbonate dehydratase activity. For CC enrichment, the results mainly include cytoskeletons of the presynaptic active zone, axonemal microtubules and presynaptic cytoskeletons.
The absence of enteric ganglion cells is responsible for the presence of HSCR, which is characterized by persistent contraction of the affected bowel segment, leading to intestinal obstruction and distension of the proximal bowel segment [42]. As research progresses, more pathogenic mechanisms are being revealed, including epigenetic mechanisms such as DNA methylation, histone modifications and other epigenetic mechanisms [43]. However, there has been no significant breakthrough in treatment modalities, and postoperative constipation and its associated complications remain a long-term concern following surgery [10]. Therefore, the search for drugs to improve intestinal function is of great clinical importance, and the multiple properties of ECH have great potential for therapeutic use [12]. Molecular docking is a method for assessing the preferred orientation of one molecule to another as they bind to each other to form stable complexes, and it is one of the most commonly used methods in structure-based drug design [44]. The main purpose of this study is to explore the potential mechanism of ECH in the treatment of HSCR.
We obtained HSCR-associated genes through database collection and gene analysis. By enrichment analysis of HSCR-related genes, the results reflect the diversity of HSCR pathogenesis, with multiple pathways being involved in disease occurrence and progression. These included pathways associated with axon development (BP), the neuronal cell body (CC) and signaling receptor activator activity (MF). The targets of ECH were also collected from three databases. We also collected the targets of ECH from three databases and identified eight potential targets through molecular docking and immunohistochemistry analysis. These targets include CA1, CA2, CA9, CA12, DNMT1, RIMS2, RPGRIP1L and ZEB2.
CA1, CA2, CA9 and CA12 are all carbonic anhydrases (CAs), which constitute a large family of zinc metalloenzymes. These enzymes can catalyze the reversible hydration of carbon dioxide, a crucial process for maintaining the body's internal environment homeostasis. Specifically, CAs balance the CO2 and HCO3 pools to regulate the pH and homeostasis in the blood and various tissues. Additionally, CAs are involved in other processesm such as water formation, cerebrospinal fluid secretion, gastric fluid formation, gluconeogenesis, lipogenesis, urea production and more [45]. Due to their diverse functions, CAs have potential as therapeutic targets for drugs. CA inhibitors could serve as novel anti-obesity, anti-cancer and anti-infective agents [46]. In the present study, ECH demonstrated a strong affinity for CAs in molecular docking.
DNMT1 can transfer methyl groups to cytosine nucleotides of genomic DNA. Maintaining optimal levels of DNMT1 is essential for normal growth and health [47]. DNMT1 is being explored as a therapeutic target in pancreatic cancer, triple-negative breast cancer and oral squamous cell carcinoma [48,49,50]. Meanwhile, DNMT1 has an important role in maintaining genomic stability during intestinal development [51]. In animal studies, LINC00346 has been found to regulate cell migration and proliferation through competitive binding to DNMT1 in HSCR [52]. DNMT1 deletion in neurons leads to the apoptosis of differentiated cells [47]. As more research is conducted, DNA methylation is shown to play a key role in the development of enteric nervous system and HSCR pathogenesis [43]. Therefore, the combination of ECH with DNMT1 has great potential in the treatment of HSCR.
RIMS2 encodes a presynaptic protein. Research on it has gradually increased in recent years. With the help of whole-exome sequencing, it was found that RIMS2 plays a key role in tardive dyskinesia [53]. And, RIMS2 is an important synaptic locus in the cognitive progression of Parkinson's disease [54]. All of these findings suggest that RIMS2 is closely associated with neurological disorders.
RPGRIP1L can stabilize epidermal keratinocyte adhesion by regulating the endocytosis of desmoglein [55]. It has been shown that overexpression of RPGRIP1L increases the number of POMC cells, and that RPGRIP1L is required for the development of hypothalamic arcuate neurons [56]. The results of immunohistochemistry showed that the gene is highly expressed in glandular cells, which could be a potential therapeutic target for the relief of intestinal obstruction. It is worth noting that all seven genes mentioned above are expressed in the epithelial layer of the intestine. This is consistent with the study that showed that ECH attenuated apoptosis and inflammatory responses in rat intestinal epithelial cells [16]. Therefore, the location of ECH action in the intestine may be in the epithelial layer.
ZEB2 is a transcription factor famous for its role in the epithelial to mesenchymal transition (EMT) [57]. The EMT is crucial in the development of the embryo [58]. Heterozygous mutations or deletions in ZEB2 cause Mowat-Wilson syndrome, a multiple congenital anomaly syndrome that includes HSCR [59]. Given that the core genes have been shown to be associated with HSCR in numerous studies, we performed an additional in-depth analysis of them. Network analysis of ceRNA revealed the regulatory mechanisms of eight core genes. The enrichment pathways most significant for BP and MF were those associated with one-carbon metabolic processes and carbonate dehydratase activity. The results for CC enrichment mainly included cytoskeletons of the presynaptic active zone, axonemal microtubules and presynaptic cytoskeletons.
It has been shown that DNMT1 is involved in the regulation of the JAK2/STAT3 signaling pathway [60,61]. ZEB2 is induced to be produced in the presence of intestinal inflammation [62]. Activation of mTOR complex 1 was increased in RPGRIP1L-negative mice [63]. Meanwhile, CA9 was associated with the mTOR pathway [64]. Combined with the effects of ECH, including IL-6/JAK2/mTOR/STAT3 pathways [14,16], ECH may exert anti-inflammatory and intestinal lubrication effects by influencing core genes in the intestinal epithelium to treat HSCR.
In summary, this study identified eight genes that showed good docking results through molecular docking, and the immunohistochemistry results indicated their expression in the gastrointestinal tract and cerebral cortex. These findings suggest that ECH has great potential for the treatment of HSCR. However, this study has some limitations. The collection of HSCR-associated genes and ECH targets was limited to databases, which may have excluded relevant genes and targets. Additionally, the use of bioinformatic methods for exploring the mechanism of action of ECH targets through enrichment analysis may not fully represent the efficacy of ECH. Furthermore, the limited number of studies available for these eight genes requires further validation through subsequent pharmacodynamic and molecular experiments. Despite these limitations, the potential targets and mechanisms identified in this study have significant research implications and scope for development to improve the treatment of HSCR.
Based on bioinformatics and molecular docking techniques, this study investigated the mechanism of action of ECH targets and the signaling pathways involved in HSCR-related genes. The potential targets of ECH for the treatment of HSCR include CA1, CA2, CA9, CA12, DNMT1, RIMS2, RPGRIP1L and ZEB2. This study provides a reference for further research into the mechanisms of ECH and therapeutic approaches to HSCR.
The genetic data used in this study are available in the GEO database (https://www.ncbi.nlm.nih.gov/geo/). The following databases were used for target collection: the OMIM (https://omim.org/) database, the DisGeNET (https://www.disgenet.org/) database, Genecard (https://www.genecards.org/) database, NCBI (https://www.ncbi.nlm.nih.gov/) database, PharmMapper (http://lilab-ecust.cn/pharmmapper/) database, Drugbank (https://go.drugbank.com/) database and TargetNet (http://targetnet.scbdd.com/) database. Mutation information for the eight genes in multiple types of cancer were obtained from the cBioPortal for Cancer Genomics (http://www.cbioportal.org). We used the PubChem(https://pubchem.ncbi.nlm.nih.gov/)database to get the structure.
The authors declare they have not used Artificial Intelligence (AI) tools in the creation of this article.
We would like to acknowledge the public databases, including GEO, OMIM, DisGeNET, Genecard, NCBI, PharmMapper, Drugbank, TargetNet, PubChem, the cBioPortal and STRING, for their contributions to human medicine in which they share vast volumes of data. Thanks to the authors of the R package for their contribution to the advancement of bioinformatics. Thanks to all of the authors of the references for their hard work and contributions to the development of medicine. Finally, we would like to thank Professor BAO WG and his yeast model and molecular genetics laboratory for providing us with the computing platform. This research was funded by the Tianjin Health Science and technology project (ZC20014).
We declare that the research was conducted in the absence of any commercial or financial relationships that could be construed as a potential conflict of interest.
Supplementary Materials include HSCR-associated genes, miRNA information and Supplemental Figure S1-S3.
[1] | A. Osiadacz, Simulation and analysis of gas networks, 1987. Available from: https://www.osti.gov/biblio/5141539. |
[2] |
A. Bressan, S. Čanić, M. Garavello, M. Herty, B. Piccoli, Flows on networks: Recent results and perspectives, EMS Surv. Math. Sci., 1 (2014), 47–111. https://doi.org/10.4171/emss/2 doi: 10.4171/emss/2
![]() |
[3] |
J. Brouwer, I. Gasser, M. Herty, Gas pipeline models revisited: Model hierarchies, nonisothermal models, and simulations of networks, Multiscale Model. Simul., 9 (2011), 601–623. https://doi.org/10.1137/100813580 doi: 10.1137/100813580
![]() |
[4] |
H. Guillard, C. Viozat, On the behaviour of upwind schemes in the low Mach number limit, Comput. Fluids, 28 (1999), 63–86. https://doi.org/10.1016/S0045-7930(98)00017-6 doi: 10.1016/S0045-7930(98)00017-6
![]() |
[5] |
H. Guillard, A. Murrone, On the behavior of upwind schemes in the low Mach number limit: Ⅱ. Godunov type schemes, Comput. Fluids, 33 (2004), 655–675. https://doi.org/10.1016/j.compfluid.2003.07.001 doi: 10.1016/j.compfluid.2003.07.001
![]() |
[6] |
F. Rieper, On the dissipation mechanism of upwind-schemes in the low Mach number regime: A comparison between roe and hll, J. Comput. Phys., 229 (2010), 221–232. https://doi.org/10.1016/j.jcp.2009.09.043 doi: 10.1016/j.jcp.2009.09.043
![]() |
[7] |
S. Jin, Runge-Kutta methods for hyperbolic conservation laws with stiff relaxation terms, J. Comput. Phys., 122 (1995), 51–67. https://doi.org/10.1006/jcph.1995.1196 doi: 10.1006/jcph.1995.1196
![]() |
[8] |
S. Jin, Efficient asymptotic-preserving (AP) schemes for some multiscale kinetic equations, SIAM J. Sci. Comput., 21 (1999), 441–454. https://doi.org/10.1137/S1064827598334599 doi: 10.1137/S1064827598334599
![]() |
[9] | J. Hu, S. Jin, Q. Li, Asymptotic-preserving schemes for multiscale hyperbolic and kinetic equations, In: Handbook of Numerical Analysis, Elsevier, 18 (2007), 103–129. https://doi.org/10.1016/bs.hna.2016.09.001 |
[10] |
J. Hu, R. Shu, X. Zhang, Asymptotic-preserving and positivity-preserving implicit-explicit schemes for the stiff BGK equation, SIAM J. Numer. Anal., 56 (2018), 942–973. https://doi.org/10.1137/17M1144362 doi: 10.1137/17M1144362
![]() |
[11] |
S. Jin, L. Pareschi, G. Toscani, Uniformly accurate diffusive relaxation schemes for multiscale transport equations, SIAM J. Numer. Anal., 38 (2000), 913–936. https://doi.org/10.1137/S0036142998347978 doi: 10.1137/S0036142998347978
![]() |
[12] | S. Jin, Asymptotic preserving (AP) schemes for multiscale kinetic and hyperbolic equations: A review, Riv. Math. Univ. Parma (N.S.), 3 (2012), 177–216. |
[13] |
S. Jin, Asymptotic-preserving schemes for multiscale physical problems, Acta Numer., 31 (2022), 415–489. https://doi.org/10.1017/S0962492922000010 doi: 10.1017/S0962492922000010
![]() |
[14] |
W. Ren, H. Liu, S. Jin, An asymptotic-preserving Monte Carlo method for the Boltzmann equation, J. Comput. Phys., 276 (2014), 380–404. https://doi.org/10.1016/j.jcp.2014.07.029 doi: 10.1016/j.jcp.2014.07.029
![]() |
[15] |
B. Zhang, H. Liu, S. Jin, An asymptotic preserving Monte Carlo method for the multispecies Boltzmann equation, J. Comput. Phys., 305 (2016), 575–588. https://doi.org/10.1016/j.jcp.2015.11.006 doi: 10.1016/j.jcp.2015.11.006
![]() |
[16] |
F. Fei, A time-relaxed Monte Carlo method preserving the Navier-Stokes asymptotics, J. Comput. Phys., 486 (2023), 112128. https://doi.org/10.1016/j.jcp.2023.112128 doi: 10.1016/j.jcp.2023.112128
![]() |
[17] | F. Fei, A Navier-Stokes asymptotic preserving Direct Simulation Monte Carlo method for multi-species gas flows, arXiv preprint arXiv: 2410.20322, 2024. |
[18] |
S. Jin, Z. Ma, K. Wu, Asymptotic-preserving neural networks for multiscale time-dependent linear transport equations, J. Sci. Comput., 94 (2023), 57. https://doi.org/10.1007/s10915-023-02100-0 doi: 10.1007/s10915-023-02100-0
![]() |
[19] |
S. Jin, Z. Ma, K. Wu, Asymptotic-preserving neural networks for multiscale kinetic equations, Commun. Comput. Phys., 35 (2024), 693–723. https://doi.org/10.4208/cicp.oa-2023-0211 doi: 10.4208/cicp.oa-2023-0211
![]() |
[20] | K. R. Arun, S. Samantaray, An asymptotic preserving time integrator for low Mach number limits of the Euler equations with gravity, arXiv preprint arXiv: 1902.00221, 2019. |
[21] |
K. R. Arun, S. Samantaray, Asymptotic preserving low Mach number accurate IMEX finite volume schemes for the isentropic Euler equations, J. Sci. Comput., 82 (2020), 1–32. https://doi.org/10.1007/s10915-020-01138-8 doi: 10.1007/s10915-020-01138-8
![]() |
[22] |
S. Boscarino, J. M. Qiu, G. Russo, T. Xiong, A high order semi-implicit IMEX WENO scheme for the all-Mach isentropic Euler system, J. Comput. Phys., 392 (2019), 594–618. https://doi.org/10.1016/j.jcp.2019.04.057 doi: 10.1016/j.jcp.2019.04.057
![]() |
[23] |
P. Degond, M. Tang, All speed scheme for the low Mach number limit of the isentropic Euler equations, Commun. Comput. Phys., 10 (2011), 1–31. https://doi.org/10.4208/cicp.210709.210610a doi: 10.4208/cicp.210709.210610a
![]() |
[24] |
G. Dimarco, R. Loubère, M. H. Vignal, Study of a new asymptotic preserving scheme for the Euler system in the low Mach number limit, SIAM J. Sci. Comput., 39 (2017), A2099–A2128. https://doi.org/10.1137/16M1069274 doi: 10.1137/16M1069274
![]() |
[25] |
G. Dimarco, R. Loubère, V. Michel-Dansac, M. H. Vignal, Second-order implicit-explicit total variation diminishing schemes for the Euler system in the low Mach regime, J. Comput. Phys., 372 (2018), 178–201. https://doi.org/10.1016/j.jcp.2018.06.022 doi: 10.1016/j.jcp.2018.06.022
![]() |
[26] |
J. Haack, S. Jin, J. G. Liu, An all-speed asymptotic-preserving method for the isentropic Euler and Navier-Stokes equations, Commun. Comput. Phys., 12 (2012), 955–980. https://doi.org/10.4208/cicp.250910.131011a doi: 10.4208/cicp.250910.131011a
![]() |
[27] | S. Samantaray, Asymptotic preserving linearly implicit additive IMEX-RK finite volume schemes for low Mach number isentropic Euler equations, arXiv preprint arXiv: 2409.05854, 2024. |
[28] |
S. Avgerinos, F. Bernard, A. Iollo, G. Russo, Linearly implicit all Mach number shock capturing schemes for the Euler equations, J. Comput. Phys., 393 (2019), 278–312. https://doi.org/10.1016/j.jcp.2019.04.020 doi: 10.1016/j.jcp.2019.04.020
![]() |
[29] |
G. Bispen, M. Lukáčová-Medviová, L. Yelash, Asymptotic preserving IMEX finite volume schemes for low Mach number Euler equations with gravitation, J. Comput. Phys., 335 (2017), 222–248. https://doi.org/10.1016/j.jcp.2017.01.020 doi: 10.1016/j.jcp.2017.01.020
![]() |
[30] |
R. Klein, Semi-implicit extension of a Godunov-type scheme based on low Mach number asymptotics Ⅰ: One-dimensional flow, J. Comput. Phys., 121 (1995), 213–237. https://doi.org/10.1016/S0021-9991(95)90034-9 doi: 10.1016/S0021-9991(95)90034-9
![]() |
[31] |
V. Kučera, M. Lukáčová-Medviová, S. Noelle, J. Schütz, Asymptotic properties of a class of linearly implicit schemes for weakly compressible Euler equations, Numer. Math., 150 (2022), 79–103. https://doi.org/10.1007/s00211-021-01240-5 doi: 10.1007/s00211-021-01240-5
![]() |
[32] |
S. Noelle, G. Bispen, K. R. Arun, M. Lukáčová-Medviová, C. D. Munz, A weakly asymptotic preserving low Mach number scheme for the Euler equations of gas dynamics, SIAM J. Sci. Comput., 36 (2014), B989–B1024. https://doi.org/10.1137/120895627 doi: 10.1137/120895627
![]() |
[33] |
J. Zeifang, J. Schütz, K. Kaiser, A. Beck, M. Lukáčová-Medviová, S. Noelle, A novel full-Euler low Mach number IMEX splitting, Commun. Comput. Phys., 27 (2020), 292–320. https://doi.org/10.4208/cicp.oa-2018-0270 doi: 10.4208/cicp.oa-2018-0270
![]() |
[34] |
W. Boscheri, M. Dumbser, M. Ioriatti, I. Peshkov, E. Romenski, A structure-preserving staggered semi-implicit finite volume scheme for continuum mechanics, J. Comput. Phys., 424 (2021), 109866. https://doi.org/10.1016/j.jcp.2020.109866 doi: 10.1016/j.jcp.2020.109866
![]() |
[35] |
F. Cordier, P. Degond, A. Kumbaro, An asymptotic-preserving all-speed scheme for the Euler and Navier-Stokes equations, J. Comput. Phys., 231 (2012), 5685–5704. https://doi.org/10.1016/j.jcp.2012.04.025 doi: 10.1016/j.jcp.2012.04.025
![]() |
[36] |
M. Dumbser, V. Casulli, A conservative, weakly nonlinear semi-implicit finite volume scheme for the compressible Navier-Stokes equations with general equation of state, Appl. Math. Comput., 272 (2016), 479–497. https://doi.org/10.1016/j.amc.2015.08.042 doi: 10.1016/j.amc.2015.08.042
![]() |
[37] |
I. Peshkov, M. Dumbser, W. Boscheri, E. Romenski, S. Chiocchetti, M. Ioriatti, Simulation of non-Newtonian viscoplastic flows with a unified first order hyperbolic model and a structure-preserving semi-implicit scheme, Comput. Fluids, 224 (2021), 104963. https://doi.org/10.1016/j.compfluid.2021.104963 doi: 10.1016/j.compfluid.2021.104963
![]() |
[38] |
G. Bispen, K. R. Arun, M. Lukáčová-Medviová, S. Noelle, IMEX large time step finite volume methods for low Froude number shallow water flows, Commun. Comput. Phys., 16 (2014), 307–347. http://doi.org/10.4208/cicp.040413.160114a doi: 10.4208/cicp.040413.160114a
![]() |
[39] |
W. Boscheri, M. Tavelli, C. E. Castro, An all Froude high order IMEX scheme for the shallow water equations on unstructured Voronoi meshes, Appl. Numer. Math., 185 (2023), 311–335. https://doi.org/10.1016/j.apnum.2022.11.022 doi: 10.1016/j.apnum.2022.11.022
![]() |
[40] |
G. Huang, Y. Xing, T. Xiong, High order well-balanced asymptotic preserving finite difference WENO schemes for the shallow water equations in all Froude numbers, J. Comput. Phys., 463 (2022), 111255. https://doi.org/10.1016/j.jcp.2022.111255 doi: 10.1016/j.jcp.2022.111255
![]() |
[41] |
S. Vater, R. Klein, A semi-implicit multiscale scheme for shallow water flows at low Froude number, Commun. Appl. Math. Comput. Sci., 13 (2018), 303–336. https://doi.org/10.2140/camcos.2018.13.303 doi: 10.2140/camcos.2018.13.303
![]() |
[42] |
X. Xie, H. Dong, M. Li, High order well-balanced asymptotic preserving IMEX RKDG schemes for the two-dimensional nonlinear shallow water equations, J. Comput. Phys., 510 (2024), 113092. https://doi.org/10.1016/j.jcp.2024.113092 doi: 10.1016/j.jcp.2024.113092
![]() |
[43] | H. Zakerzadeh, The RS-IMEX scheme for the rotating shallow water equations with the Coriolis force, In: Finite volumes for complex applications Ⅷ—Hyperbolic, Elliptic and Parabolic Problems, Cham: Springer, 200 (2017), 199–207. https://doi.org/10.1007/978-3-319-57394-6-22 |
[44] |
S. Busto, M. Dumbser, A staggered semi-implicit hybrid finite volume / finite element scheme for the shallow water equations at all Froude numbers, Appl. Numer. Math., 175 (2022), 108–132. https://doi.org/10.1016/j.apnum.2022.02.005 doi: 10.1016/j.apnum.2022.02.005
![]() |
[45] |
A. Duran, F. Marche, R. Turpault, C. Berthon, Asymptotic preserving scheme for the shallow water equations with source terms on unstructured meshes, J. Comput. Phys., 287 (2015), 184–206. https://doi.org/10.1016/j.jcp.2015.02.007 doi: 10.1016/j.jcp.2015.02.007
![]() |
[46] |
A. Kurganov, Y. Liu, M. Lukáčová-Medviová, A well-balanced asymptotic preserving scheme for the two-dimensional rotating shallow water equations with nonflat bottom topography, SIAM J. Sci. Comput., 44 (2022), A1655–A1680. https://doi.org/10.1137/21M141573X doi: 10.1137/21M141573X
![]() |
[47] |
X. Liu, A. Chertock, A. Kurganov, An asymptotic preserving scheme for the two-dimensional shallow water equations with Coriolis forces, J. Comput. Phys., 391 (2019), 259–279. https://doi.org/10.1016/j.jcp.2019.04.035 doi: 10.1016/j.jcp.2019.04.035
![]() |
[48] |
H. Egger, J. Giesselmann, T. Kunkel, N. Philippi, An asymptotic-preserving discretization scheme for gas transport in pipe networks, IMA J. Numer. Anal., 43 (2023), 2137–2168. https://doi.org/10.1093/imanum/drac032 doi: 10.1093/imanum/drac032
![]() |
[49] | H. Egger, N. Philippi, An asymptotic preserving hybrid-dG method for convection-diffusion equations on pipe networks, arXiv preprint arXiv: 2209.04238, 2022. https://doi.org/10.48550/arXiv.2209.04238 |
[50] |
A. Kurganov, S. Noelle, G. Petrova, Semidiscrete central-upwind schemes for hyperbolic conservation laws and Hamilton-Jacobi equations, SIAM J. Sci. Comput., 23 (2001), 707–740. https://doi.org/10.1137/S1064827500373413 doi: 10.1137/S1064827500373413
![]() |
[51] |
A. Kurganov, E. Tadmor, Solution of two-dimensional Riemann problems for gas dynamics without Riemann problem solvers, Numer. Methods Partial Differ. Equations, 18 (2002), 584–608. https://doi.org/10.1002/num.10025 doi: 10.1002/num.10025
![]() |
[52] | G. Wanner, E. Hairer, Solving Ordinary Differential Equations II, New York: Springer Berlin Heidelberg, 1996. |
[53] |
X. Zhong, Additive semi-implicit Runge-Kutta methods for computing high-speed nonequilibrium reactive flows, J. Comput. Phys., 128 (1996), 19–31. https://doi.org/10.1006/jcph.1996.0193 doi: 10.1006/jcph.1996.0193
![]() |
[54] |
M. K. Banda, M. Herty, A. Klar, Gas flow in pipeline networks, Netw. Heterog. Media, 1 (2006), 41–56. https://doi.org/10.3934/nhm.2006.1.41 doi: 10.3934/nhm.2006.1.41
![]() |
[55] |
H. Egger, J. Giesselmann, Stability and asymptotic analysis for instationary gas transport via relative energy estimates, Numer. Math., 153 (2023), 701–728. https://doi.org/10.1007/s00211-023-01349-9 doi: 10.1007/s00211-023-01349-9
![]() |
[56] | K. Ehrhardt, M. C. Steinbach, Nonlinear optimization in gas networks, In: Modeling, Simulation and Optimization of Complex Processes, Berlin Heidelberg: Springer, 2005,139–148. https://doi.org/10.1007/3-540-27170-8-11 |
[57] |
M. Herty, N. Izem, M. Seaid, Fast and accurate simulations of shallow water equations in large networks, Comput. Math. Appl., 78 (2019), 2107–2126. https://doi.org/10.1016/j.camwa.2019.03.049 doi: 10.1016/j.camwa.2019.03.049
![]() |
[58] | J. Caputo, D. Dutykh, B. Gleyse, Coupling conditions for water waves at forks, Symmetry, 11 (2019), 434. https://www.mdpi.com/2073-8994/11/3/434 |
[59] |
R. M. Colombo, M. Garavello, A well posed Riemann problem for the -system at a junction, Netw. Heterog. Media, 1 (2006), 495–511. https://doi.org/10.3934/nhm.2006.1.495 doi: 10.3934/nhm.2006.1.495
![]() |
[60] | F. M. White, H. Xue, Fluid Mechanics, New York: McGraw-hill, 2003. |
[61] |
A. Ambroso, C. Chalons, F. Coquel, E. Godlewski, F. Lagoutière, P. A. Raviart, et al., Coupling of general Lagrangian systems, Math. Comp., 77 (2008), 909–941. https://doi.org/10.1090/S0025-5718-07-02064-9 doi: 10.1090/S0025-5718-07-02064-9
![]() |
[62] |
R. Borsche, Numerical schemes for networks of hyperbolic conservation laws, Appl. Numer. Math., 108 (2016), 157–170. https://doi.org/10.1016/j.apnum.2016.01.006 doi: 10.1016/j.apnum.2016.01.006
![]() |
[63] |
G. Bretti, R. Natalini, B. Piccoli, Fast algorithms for the approximation of a traffic flow model on networks, Discrete Contin. Dyn. Syst. Ser. B, 6 (2006), 427–448. https://doi.org/10.3934/dcdsb.2006.6.427 doi: 10.3934/dcdsb.2006.6.427
![]() |
[64] |
C. Chalons, P. A. Raviart, N. Seguin, The interface coupling of the gas dynamics equations, Quart. Appl. Math., 66 (2008), 659–705. https://doi.org/10.1090/S0033-569X-08-01087-X doi: 10.1090/S0033-569X-08-01087-X
![]() |
[65] |
F. Dubois, P. LeFloch, Boundary conditions for nonlinear hyperbolic systems of conservation laws, J. Differ. Equations, 71 (1988), 93–122. https://doi.org/10.1016/0022-0396(88)90040-X doi: 10.1016/0022-0396(88)90040-X
![]() |
[66] |
E. Godlewski, P. A. Raviart, The numerical interface coupling of nonlinear hyperbolic systems of conservation laws: Ⅰ. The scalar case, Numer. Math., 97 (2004), 81–130. http://link.springer.com/10.1007/s00211-002-0438-5 doi: 10.1007/s00211-002-0438-5
![]() |
[67] |
E. Godlewski, P. A. Raviart, A method of coupling non-linear hyperbolic systems: Examples in CFD and plasma physics, Int. J. Numer. Methods Fluids, 47 (2005), 1035–1041. https://doi.org/10.1002/fld.856 doi: 10.1002/fld.856
![]() |
[68] |
H. Holden, N. H. Risebro, A mathematical model of traffic flow on a network of unidirectional roads, SIAM J. Math. Anal., 26 (1995), 999–1017. https://doi.org/10.1137/S0036141093243289 doi: 10.1137/S0036141093243289
![]() |
[69] |
L. O. Müller, P. J. Blanco, A high order approximation of hyperbolic conservation laws in networks: Application to one-dimensional blood flow, J. Comput. Phys., 300 (2015), 423–437. https://doi.org/10.1016/j.jcp.2015.07.056 doi: 10.1016/j.jcp.2015.07.056
![]() |
[70] |
A. Harten, P. D. Lax, B. van Leer, On upstream differencing and Godunov-type schemes for hyperbolic conservation laws, SIAM Rev., 25 (1983), 35–61. https://doi.org/10.1137/1025002 doi: 10.1137/1025002
![]() |
[71] |
K. A. Lie, S. Noelle, On the artificial compression method for second-order nonoscillatory central difference schemes for systems of conservation laws, SIAM J. Sci. Comput., 24 (2003), 1157–1174. https://doi.org/10.1137/S1064827501392880 doi: 10.1137/S1064827501392880
![]() |
[72] |
H. Nessyahu, E. Tadmor, Nonoscillatory central differencing for hyperbolic conservation laws, J. Comput. Phys., 87 (1990), 408–463. https://doi.org/10.1016/0021-9991(90)90260-8 doi: 10.1016/0021-9991(90)90260-8
![]() |
[73] |
P. K. Sweby, High resolution schemes using flux limiters for hyperbolic conservation laws, SIAM J. Numer. Anal., 21 (1984), 995–1011. https://doi.org/10.1137/0721062 doi: 10.1137/0721062
![]() |
[74] | M. Garavello, K. Han, B. Piccoli, Models for Vehicular Traffic on Networks, American Institute of Mathematical Sciences, 2016. |
[75] |
O. Kolb, J. Lang, P. Bales, An implicit box scheme for subsonic compressible flow with dissipative source term, Numer. Algor., 53 (2010), 293–307. https://doi.org/10.1007/s11075-009-9287-y doi: 10.1007/s11075-009-9287-y
![]() |
[76] |
H. Egger, A robust conservative mixed finite element method for isentropic compressible flow on pipe networks, SIAM J. Sci. Comput., 40 (2018), A108–A129. https://doi.org/10.1137/16M1094373 doi: 10.1137/16M1094373
![]() |
[77] |
J. G. Caputo, D. Dutykh, Nonlinear waves in networks: Model reduction for the sine-Gordon equation, Phys. Rev. E, 90 (2014), 022912. https://link.aps.org/doi/10.1103/PhysRevE.90.022912 doi: 10.1103/PhysRevE.90.022912
![]() |
[78] |
D. Dutykh, J. G. Caputo, Wave dynamics on networks: Method and application to the sine-Gordon equation, Appl. Numer. Math., 131 (2018), 54–71. https://doi.org/10.1016/j.apnum.2018.03.010 doi: 10.1016/j.apnum.2018.03.010
![]() |
[79] |
R. Borsche, J. Kall, ADER schemes and high order coupling on networks of hyperbolic conservation laws, J. Comput. Phys., 273 (2014), 658–670. https://doi.org/10.1016/j.jcp.2014.05.042 doi: 10.1016/j.jcp.2014.05.042
![]() |
[80] |
M. Herty, N. Kolbe, S. Müller, Central schemes for networked scalar conservation laws, Netw. Heterog. Media, 18 (2023), 310–340. https://doi.org/10.3934/nhm.2023012 doi: 10.3934/nhm.2023012
![]() |
1. | Liguo Xu, Wenhao Huang, Yachao Lin, Zhendong Li, Interaction and binding mechanism of anticancer echinacoside phenylethanoid glycoside against hepatocellular carcinoma with subdomains of human serum albumin, 2024, 17, 18785352, 105869, 10.1016/j.arabjc.2024.105869 | |
2. | Enyang He, Bowen Shi, Miao Jia, Wenjing Sun, Kaili Chang, Hongyv Jiang, Wei Zhao, Hailan Zhao, Liang Dong, Xiaohong Die, Wei Feng, Hualei Cui, Hirschsprung’s disease may increase the incidence of inflammatory bowel disease through alterations in CA1, 2025, 0031-3998, 10.1038/s41390-025-03938-w |
Target | Identifier | Method | Resolution | Chain | Positions |
CA1 | 7Q0D | X-ray | 1.24 Å | A/B | 1-261 |
CA2 | 1CIL | X-ray | 1.60 Å | A | 2-260 |
CA9 | 6RQQ | X-ray | 1.28 Å | A/C | 140-395 |
CA12 | 5MSA | X-ray | 1.20 Å | A/B/C/D | 30-291 |
DNMT1 | 6X9J | X-ray | 1.79 Å | A | 729-1600 |
RIMS2 | 1V27 | NMR | - | A | 807-934 |
RPGRIP1L | 2YRB | NMR | - | A | 595-737 |
ZEB2 | 2DA7 | NMR | - | A | 647-704 |
Target | Npts | Spacing | Affinity |
CA1 | 60, 60, 60 | 0.375 | -13.30 |
CA2 | 48, 48, 48 | 0.375 | -13.13 |
CA9 | 126,120,126 | 0.397 | -12.65 |
CA12 | 46, 51, 39 | 0.375 | -13.38 |
DNMT1 | 100, 80, 70 | 1.000 | -7.54 |
RIMS2 | 100,100,100 | 0.375 | -13.84 |
RPGRIP1L | 80,126,126 | 0.450 | -13.00 |
ZEB2 | 72,126,114 | 0.375 | -12.85 |
Target | Identifier | Method | Resolution | Chain | Positions |
CA1 | 7Q0D | X-ray | 1.24 Å | A/B | 1-261 |
CA2 | 1CIL | X-ray | 1.60 Å | A | 2-260 |
CA9 | 6RQQ | X-ray | 1.28 Å | A/C | 140-395 |
CA12 | 5MSA | X-ray | 1.20 Å | A/B/C/D | 30-291 |
DNMT1 | 6X9J | X-ray | 1.79 Å | A | 729-1600 |
RIMS2 | 1V27 | NMR | - | A | 807-934 |
RPGRIP1L | 2YRB | NMR | - | A | 595-737 |
ZEB2 | 2DA7 | NMR | - | A | 647-704 |
Target | Npts | Spacing | Affinity |
CA1 | 60, 60, 60 | 0.375 | -13.30 |
CA2 | 48, 48, 48 | 0.375 | -13.13 |
CA9 | 126,120,126 | 0.397 | -12.65 |
CA12 | 46, 51, 39 | 0.375 | -13.38 |
DNMT1 | 100, 80, 70 | 1.000 | -7.54 |
RIMS2 | 100,100,100 | 0.375 | -13.84 |
RPGRIP1L | 80,126,126 | 0.450 | -13.00 |
ZEB2 | 72,126,114 | 0.375 | -12.85 |