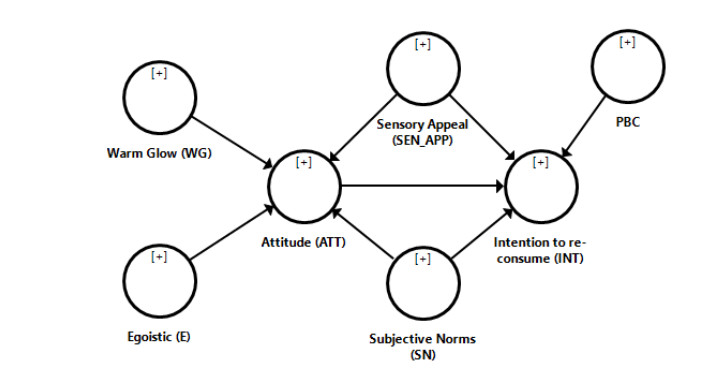
The integration of artificial intelligence (AI) into healthcare has ushered in transformative advancements. In diagnostics, AI has revolutionized precision by excelling in radiology, pathology, and novel approaches like liquid biopsy analysis. Personalized treatment plans benefit from AI's ability to tailor interventions, minimizing risks and maximizing therapeutic efficacy. Administrative efficiency sees notable improvements as AI automates tasks, optimizes resource allocation, and addresses scalability challenges. Ethical considerations, encompassing data privacy, algorithmic bias, and transparency, are crucial for responsible AI adoption. Guided by the fair principles, stakeholders can promote fairness, accountability, and transparency. Findings in this paper reveal AI's rapid advancements in healthcare enhance diagnostics, surgery, and patient care, yet reliance on its accuracy requires caution. The future of AI in healthcare relies on ongoing collaboration, research, and adherence to ethical frameworks, promising a patient-centered and equitable healthcare landscape.
Citation: Segun Akinola. Advancing healthcare with AI: designing frameworks for diagnostics, personalized treatment, and enhanced efficiency[J]. AIMS Medical Science, 2024, 11(3): 248-264. doi: 10.3934/medsci.2024019
[1] | Daniele Asioli, Rungsaran Wongprawmas, Erika Pignatti, Maurizio Canavari . Can information affect sensory perceptions? Evidence from a survey on Italian organic food consumers. AIMS Agriculture and Food, 2018, 3(3): 327-344. doi: 10.3934/agrfood.2018.3.327 |
[2] | Austin Rong-Da Liang, Wai Mun Lim, Wei Tung, Shu-Ying Lin . Understanding different types of consumers: A multi-group analysis based on convenience food-related lifestyle. AIMS Agriculture and Food, 2023, 8(2): 374-390. doi: 10.3934/agrfood.2023021 |
[3] | Bentivoglio Deborah, Margherita Rotordam, Staffolani Giacomo, Chiaraluce Giulia, Finco Adele . Understanding consumption choices of innovative products: an outlook on the Italian functional food market. AIMS Agriculture and Food, 2021, 6(3): 818-837. doi: 10.3934/agrfood.2021050 |
[4] | Masoud Ghanbari, Jahan B Ghasemi, Amir M Mortazavian . Comparison of three sensory characterization methods based on consumer perception for the development of a novel functional cereal-based dessert. AIMS Agriculture and Food, 2017, 2(3): 258-278. doi: 10.3934/agrfood.2017.3.258 |
[5] | Luca Altamore, Marzia Ingrassia, Stefania Chironi, Pietro Columba, Giuseppe Sortino, Ana Vukadin, Simona Bacarella . Pasta experience: Eating with the five senses—a pilot study. AIMS Agriculture and Food, 2018, 3(4): 493-520. doi: 10.3934/agrfood.2018.4.493 |
[6] | Alessandro Scuderi, Claudio Bellia, Vera Teresa Foti, Luisa Sturiale, Giuseppe Timpanaro . Evaluation of consumers’ purchasing process for organic food products. AIMS Agriculture and Food, 2019, 4(2): 251-265. doi: 10.3934/agrfood.2019.2.251 |
[7] | Wei Yang, Bryan Anh, Phuc Le . Do consumers care about environmentally sustainable attributes along the food supply chain? —A systematic literature review. AIMS Agriculture and Food, 2023, 8(2): 513-533. doi: 10.3934/agrfood.2023027 |
[8] | Marcelo Augusto de Carvalho, Cíntia Sorane Good Kitzberger, Altamara Viviane de Souza Sartori, Marta de Toledo Benassi, Maria Brígida dos Santos Scholz, Clandio Medeiros da Silva . Free choice profiling sensory analysis and principal component analysis as tools to support an apple breeding program. AIMS Agriculture and Food, 2020, 5(4): 769-784. doi: 10.3934/agrfood.2020.4.769 |
[9] | Giorgia Liguori, Giuseppe Sortino, Giuseppe Gianguzzi, Paolo Inglese, Vittorio Farina . Evaluation of quality attributes and consumer preference of fresh or imported mangoes in Italy. AIMS Agriculture and Food, 2018, 3(4): 426-440. doi: 10.3934/agrfood.2018.4.426 |
[10] | Maurizio Canavari, Federico Gori, Selene Righi, Elena Viganò . Factors fostering and hindering farmers' intention to adopt organic agriculture in the Pesaro-Urbino province (Italy). AIMS Agriculture and Food, 2022, 7(1): 108-129. doi: 10.3934/agrfood.2022008 |
The integration of artificial intelligence (AI) into healthcare has ushered in transformative advancements. In diagnostics, AI has revolutionized precision by excelling in radiology, pathology, and novel approaches like liquid biopsy analysis. Personalized treatment plans benefit from AI's ability to tailor interventions, minimizing risks and maximizing therapeutic efficacy. Administrative efficiency sees notable improvements as AI automates tasks, optimizes resource allocation, and addresses scalability challenges. Ethical considerations, encompassing data privacy, algorithmic bias, and transparency, are crucial for responsible AI adoption. Guided by the fair principles, stakeholders can promote fairness, accountability, and transparency. Findings in this paper reveal AI's rapid advancements in healthcare enhance diagnostics, surgery, and patient care, yet reliance on its accuracy requires caution. The future of AI in healthcare relies on ongoing collaboration, research, and adherence to ethical frameworks, promising a patient-centered and equitable healthcare landscape.
The consumption of organic food has developed into a new trend as a way to a more sustainable paradigm. Accordingly, the purchase of organic food has increased worldwide. Although the sale of organic food is still centered in developed countries such as the United States, Canada, and several major European countries (Germany, France, United Kingdom, and Italy), the concept of organic farming is accepted in developing countries because one-third of the world's land is managed organically be there [1]. This becomes an opportunity in the development of organic consumerism in developing countries.
In Indonesia, which is a developing country, organic food consumers are still concentrated in big cities, with middle-class households with higher education [2]. Although the government has been supporting the development of organic food in Indonesia [3], official organic food consumption data are not yet available on a national scale. According to the data released by the Indonesian Organic Alliance in 2017, 94% of the respondents consumed organic food for health reasons, while the rest did so to protect the environment and keep up with trends. The most frequently consumed organic foods are vegetables, followed by rice and fruits [4]. Furthermore, the data in 2019 reported that only 7.92% of 274 respondents were regular consumers of organic food.
To identify and target the consumers of organic food in Indonesia, it is important to understand the factors behind consumers' motivations to purchase organic food [5]. Few publications are focusing on the discussion on organic agriculture in Indonesia, particularly on the consumer aspect [2]. The results of research on consumer attitudes and behavior on the purchase and consumption of organic food from the perspective of developed countries may differ from those of developing countries [6]. Based on the understanding of these factors, marketers and policy makers can develop private and public strategies aimed at increasing the consumption of organic food in Indonesia.
The theory of planned behavior (TPB) has been widely used as a framework for understanding the buying and consumption of organic food [1,6,7,8,9,10]. TPB said that intention to perform a given behavior is the best predictor of actual behavioral performance, wherein intention is determined by three sociopsychological constructs: attitudes, subjective norms, and perceived behavioral control [11,12]. However, other variables like background factors that influence the dimensions in the basic theory of TPB can be considered in line with TPB which is open to develop [13].
Literature has focused on the study of consumer behavior by attempting to investigate the consumer perceptions of organic food and the factors that influence the behavior to choose to consume organic food [10]. Consumers are judged to place a high value on the benefits of sensory characteristics and seek out the specific traits that engage all the senses and stimulate deeper associations with the products they consume [14,15]. Nonetheless, researchers [16] note a lack of effort to investigate the role of sensory attractiveness (appearance, taste, aroma, and texture) in investigating organic food consumption.
Previous research has also shown that the decision to buy organic food is influenced by the perceptions of good taste, health, environmental benefits, and animal welfare [17]. Consumer attention to nutrition and healthy eating patterns has revealed that the attributes associated with healthy food become as important as the attributes related to the flavor or sensory appeal. Consumer's increasing demand for organic food may also be attributed to their increased social awareness, triggering their willingness to pay more for the products that are safer for the environment or more ethical than conventional products [18].
Furthermore, studies on evolving consumer emotions have depicted that consumers can help predict better [19]. When consumers engage in pro-social and pro-environmental behavior, like purchasing organic products, they are identified to gain psychological benefits in the form of emotional experiences [20]. This situation demonstrates another benefit that consumers receive despite having to pay higher prices than conventional products for altruistic reasons. In the long run, this motivation leads to increased consumption of organic food [21]. However, the studies linking the psychological benefits obtained in the context of organic food consumerism with an individual's attitude or intention to re-consume organic food are still rare.
Research that explains the role of perceived health benefits (egoistic motives) and perceived psychological benefits on pro-environmental and pro-environmental behavior (warm glow) as well as the influence of perceptions of sensory appeal using the complete TPB model is still lacking [22,23,24,25]. To fill the gap in the literature, this study proposes a full TPB model by adding egoistic motives, warm glow, and sensory appeal perception that might influence attitudes and intentions. Furthermore, this study pioneered how the warm glow motif affects the buying behavior of consumers of organic food in Indonesia.
The conceptual model of this study is based on the theory of planned behavior [11], as it has been widely used as a framework for understanding the purchase and consumption of organic food [1,6,7,8,9,10,23,24,25]. TPB shows that human behavior is the result of careful planning [11]. In this study, consumer's intention to re-consume organic food is used to describe future organic food consumption behavior as actual behavior. This is because the TPB theorizes that the intention to perform a particular behavior is the best direct predictor of actual behavioral performance [11]. Stronger intentions to engage in certain behaviors usually result in that behavior [11,26]. Although, there are a gap between intention and behavior which is empirical studies in the field of pro-environmental and ethical buying behavior have found that strong intentions may not result in purchasing behavior [7,12,27]. The gap has drawn criticism against TPB and a strategy is needed to strengthen the intention to manifest it in real behavior [28,29]. Such gaps may occur when consumers may intend to buy a product but then face barriers related to psychological (e.g. trust, satisfaction, learning and motivation), economic (e.g. affordability) and physical (e.g. distance and availability) factors in making purchasing decisions [7].
Intention reflects motivation and cognitive planning to engage in behavior, which is determined by the three tractors in the basic concept of TPB, including attitude, subjective norms, and perceived behavioral control (PBC) [9]. Attitude towards behavior refers to the extent to which a person has a positive or negative evaluation or assessment of the consequences of behavior [11]. Because the relationship between attitudes and behavioral intentions in the TPB framework is considered the strongest among others, attitude becomes an important factor in prediction [7]. This is supported by the previous research, which has reported that attitudes significantly affect the intentions of organic consumers, both in developed countries [9,17,30,31] and in developing countries [1,12,20,23,32]. In addition, attitudes reflect individual preferences to perform or not to perform a behavior [6].
Subjective norms describe the views of other people (in the consumer environment) on consumer choices to consume organic food. Furthermore, subjective norms shape the perceived social pressure to engage in behavior [9]. In general, subjective norms form consumer behavior based on agreed-upon elements in community customs or traditional views [7]. Ajzen [11] states that the global measure of subjective norms is the extent to which other people important to an individual approve or disapprove of his or her behavior. Subjective norms reflect the level of an individual's desire to adjust to his social group, including teachers, colleagues, parents, admired people, and other social groups [6], which then encourages individuals to do or not do certain behaviors.
Several studies on organic food consumption have found a strong and significant relationship between subjective norms and organic food consumption intentions [7,9,33]. In addition, Ajzen [34] has acknowledged that attitudes and subjective norms may be interdependent. Researchers [30] have proposed a TPB model that shows the influence of subjective norms on the attitudes of organic food consumers in Finland.
PBC, according to researchers [11], is based on individual beliefs about its influence on situations as well as internal factors that facilitate behavior. PBC is related to the individual's belief in the self ability to carry out the behavior. TPB regards behavior as a concept that must include both voluntary and involuntary components. Furthermore, based on TPB, individuals who have a positive attitude towards organic food, believe that there is normative support for its consumption and find it easy for consumers to consume [35]. Thus, the following hypothesis is advanced:
H1: Attitude, subjective norms, and PBC has a positive and significant effect on the intention to re-consume organic food in the future.
Although TPB has been reported successful in predicting behavior, the theory keeps evolving, and various researchers have expressed confidence that for certain behaviors and contexts, the addition of other variables can improve the model's predictive ability [35]. Scholars [13] have suggested that the TPB model is inherent to modification and the addition of additional predictors. Therefore, there is a growing body of evidence in the recent literature supporting the inclusion of predictor variables in TPB. Researchers [36] added that individual characteristics such as values can increase the predictive power of TPB. In the context of developing countries, health, beliefs about organic food, and quality were discovered to be the most influential factors on the intention to consume organic food [37]. This study also attempts to incorporate new constructs related to food values/attributes, namely sensory attractiveness, warm glow, and egoistic motivation in TPB, by taking the support from the existing literature.
The sensory aspect of food has been reported to be the most important factor in food selection [18]. Sensory attributes relate to the appearance, smell, and taste of food. Other studies have also found that sensory characteristics are significant determinants of purchasing organic food [38,39]. The sensory appeal is identified as one of the categories of product quality that add value to organic food production besides its ethical values [40,41]. According to researchers [14], consumers place a high value on the hedonistic benefits of food as well as sensory characteristics and seek out particular traits that involve all the senses to stimulate deeper associations with the product. Sensory attributes of organic food may stimulate emotional influence/consumer experience, resulting in organic food evaluations that are primarily based on these values [18]. Therefore, the following hypothesis is proposed:
H2: Consumer perceptions of the sensory appeal attribute of organic food have a positive and significant effect on consumer attitudes an consumers' intention to re-consume organic food in the future.
The literature related to consumption shows that selfish (pro-self) values are an important driving factor in behavioral decision-making. This is consistent with the general belief that individuals are selfish or prioritize their interests, which are then reflected in individual consumption behavior [21]. Concern for one's health demonstrates the concept of pro-self, which can be interpreted as selfish [42]. Consumers want what they eat to be at least harmless (safe for consumption), especially when it comes to food [43]. Consumers gradually became more concerned about the health, quality, and nutritional value of food [44] and slowly put aside the issue of price. It further motivates people to change dietary habits [1].
Organic food is considered healthier because it is produced with fewer harmful chemical fertilizers and synthetic pesticides [45]. Increased awareness of health aspects directs consumers to engage in organic consumption behavior that is considered to improve or maintain their health. Individuals with a high level of health awareness are more likely to adhere to dietary recommendations and are more concerned with the desired nutrition. Furthermore, food safety concerns are a factor to consider when purchasing organic food. Individuals expect organic food to be consumed as a non-harmful product to the body [46,47].
A growing body of literature on organic food consumption indicates that health and food safety concerns are among the important drivers of positive attitudes. Researchers mentioned that consumers who are more concerned with their health problems have better attitudes towards purchasing organic products [5]. Several previous studies have also supported health care as a major factor motivating consumer attitudes [48,49,50]. In this study, the egoistic value is described by consumers' concern for personal health and food safety because they best represent personal interests in consumption motives.
Organic food consumption is concerned not only with personal aspects such as health but also with the environment, where organic food is considered to have a less negative impact than conventionally grown food, as well as supports local economic welfare [51]. The action is pro-environmental and pro-social, with an altruistic value. Altruistic values have been conceptualized as part of a personal value structure or overall guiding principle that motivates individuals to contribute to the well-being of others or society as a whole [52,53]. Altruism involves actions to improve the welfare of others which then incur personal costs but tend to ignore personal gains [21,54].
The consumer's desire to consume products that indirectly contribute to environmental sustainability also supports the welfare of farmers by selecting organic food ingredients, requiring consumers to incur additional costs. Recent studies suggest that seeking pleasure and pursuing happiness may be important motives for pro-social and pro-environmental behavior [21]. According to a study of organic food, consumers experience psychological benefits when engaging in altruistic behavior [20]. Even though the price of organic food is high, the psychological benefit is considered as an additional reward [55].
Findings related to psychological benefits that become motives for pro-social/pro-environmental behavior support the emerging debate regarding whether there are truly altruistic individuals without considering their interests. Further, researchers [56] refer to the rewards obtained in the form of emotional experiences for pro-social/pro-environment actions as a warm glow of giving [57], in which individuals may be motivated by both egoistic and altruistic motives, impurely altruist. Consumers with socially and environmentally responsible behavior experience an intrinsic warm feeling. It can lead to positive attitudes toward behavior as a result of contributing to the common good [17]. Therefore, the following hypothesis is proposed:
H3: Warm glow and egoistic motive from pro-environmental and pro-social behavior has a positive and significant effect on consumer attitudes toward organic food.
Based on the discussion, a research theoretical framework has been developed, as presented in Figure 1.
An online survey was conducted on Indonesian consumers from September 2020 to October 2020. The research questionnaires were administered and distributed through an online survey platform. An online survey collects information from people who respond to a form or instrument that is distributed through internet channels. Online surveys have several advantages and disadvantages. They are easy to conduct using free platforms, e.g Google forms. They can be quickly created and distributed with global reach. Furthermore, online surveys are completed only by persons who are literate and who have access to the internet, and by those who are sufficiently biased to be interested in the subject [58]. Although online surveys may exclude a target population that does not have internet access, the benefits of Indonesia's wide geographical reach and the usual reduction in social desire are the reasons for adopting the online approach [59]. With the limitation, the small reach of researchers doing snowball techniques made the number of samples may not be able to generalize the population of organic consumers in Indonesia.
This online survey was conducted using Google forms platforms which were distributed in a snowball technique through Individual emails, Mailing Lists, and Social media platforms with both individually and in groups (WhatsApp, Facebook, Instagram, and Telegram). Pretest was carried out on 50 online consumers to ensure the fluency and understanding of the questionnaire. Through pretesting, ambiguous and imprecise questions were modified. To avoid filling out surveys repeatedly, each IP address was limited to one survey participation. To meet the survey requirements, respondents had to be at least 17 years old and had purchased organic food, including rice, vegetables, fruit, and animal protein, such as chicken eggs or meat [7]. Responses from the respondents were obtained and then screened, which yielded 337 respondents who met the criteria for further analysis.
The survey measurements were compiled from the previous studies and then modified for this study. The questionnaires in this study were written in Indonesian to facilitate the equating of meaning for each measurement item. The questionnaire was divided into two parts. The first section covers demographic characteristics, including age, gender, education level, marital status, and occupation. The second part of the questionnaire includes a series of questions used to measure the intention to re-consume (INT), attitude (ATT), subjective norms (SN), perceived behavior control (PBC), perceived sensory appeal (SEN_APP), warm glow (WG), and egoistic motivation (E).
Based on Ajzen's recommendation [35], a scale containing multiple items was developed to measure each measurement item. Respondents in this study were asked to provide a scale responding agree or disagree with the statements presented to measure each variable using a five-point Likert scale (from very low = 1 to very high = 5). Questionnaire items and sources of literature adoption are presented in Table 1.
Partial Least Squares-Structural Equation Modeling (PLS-SEM) was used to test the direct effect of the proposed model. PLS is an SEM technique based on an iterative approach that maximizes the described variance of endogenous constructs. In contrast to CB-SEM, which seeks to validate theory by determining how well a model can estimate the covariance matrix for sample data, PLS-SEM functions similarly to multiple regression analysis. PLS is frequently used to address several issues that SEM encounters, including, namely (1) abnormal data; (2) small sample size; and (3) formally measured constructs [60].
PLS-SEM is able to modeling a latent constructions under abnormal conditions with small to medium sample sizes. Furthermore, PLS-SEM can work with reflective and/or formative indicators [7]. PLS-SEM does not strictly require study data to follow a normal distribution. According to researcher [61], PLS is an appropriate model for compiling research that focuses on prediction and theory development. In this research, TPB was developed by introducing several new variables into the model. Finally, PLS-SEM was considered to be able to identify the most effective variables in understanding the consumption behavior of organic food. Therefore, PLS-SEM was adopted in this study.
The steps that must be taken in the SEM-PLS analysis include designing model specifications, evaluating the outer model, and evaluating the inner model. The inner and outer models are defined during the model specification stage. The inner model, also known as the structural model, depicts the relationships between the latent constructs under consideration. The items presented in Table 1 serve as reflective indicators of their respective constructs. Furthermore, the outer model, also known as the measurement model, was used to assess the relationship between the manifest indicator variable and the corresponding latent construct. Therefore, the PLS model was interpreted in two stages. First, validity and reliability in the measurement model were assessed. Second, the structural model was measured by evaluating the explanatory power and the significance level of the path coefficients.
Variable | Item | Measurement Item | Source |
Intention to re-consume (INT) | INT1 | I intend to repurchase organic foods. | [17,35] |
INT2 | I intend to eat more organically processed foods in the future. | ||
INT3 | I would like to repurchase organic food because it is the best option. | ||
INT4 | I would like to reintroduce organic food, particularly environmentally friendly products. | ||
INT5 | I would like to re-consume organic food, preferably healthy and nutritious food. | ||
INT6 | For the welfare of farmers, I would like to re-consume organic food. | ||
Attitude (ATT) | ATT1 | Buying organic food is a good idea. | [17,35,62] |
ATT2 | Buying organic food is a wise decision. | ||
ATT3 | Buying organic food is important. | ||
ATT4 | Consuming organic food is advantageous. | ||
ATT5 | Consuming organic food is valuable. | ||
ATT6 | Consuming organic food is interesting. | ||
ATT7 | I like organic food because it is processed without the use of chemicals. | ||
ATT8 | I like organic food because it is more nutritious. | ||
ATT9 | I like organic food because it is healthier to consume. | ||
ATT10 | I like organic food because it is environmentally friendly. | ||
ATT11 | I like organic food because it allows me to contribute to the well-being of farmers. | ||
Subjective norms (SN) | SN1 | Most of the people important to me think that I should eat organic food. | [62,63,64] |
SN2 | My family believes that I should consume organic food. | ||
SN3 | People whose opinions I value believe that I should consume organic food. | ||
SN4 | My friend's positive feedback encourages me to consume organic food. | ||
SN5 | My loved ones expect me to consume organic food. | ||
SN6 | Because of external pressure (social, environmental, network, etc.), I choose organic food. | ||
Perceived Behavior Control (PBC) | PBC1 | It is entirely up to me whether or not I buy organic food. | [17,62,63,64] |
PBC2 | Consumers believe they can buy organic food. | ||
PBC3 | Consumers believe they have the resources, time, and willingness to buy organic food. | ||
PBC4 | Customers believe that they have control over whether or not they consume organic food. | ||
PBC5 | Consumers believe that there will be numerous opportunities for them to consume organic food. | ||
PBC6 | Consumers find it simple to consume organic food. | ||
PBC7 | Consumers believe they can consume organic food if they desire to do so. | ||
Sensory Appeal Perceived (SEN_APP) | SEN_APP1 | In my opinion, organic food is more attractive. | [18,40,62,65] |
SEN_APP2 | In my opinion, organic food appears to be tasty. | ||
SEN_APP3 | In my opinion, organic food looks fresher. (especial for rice, in question is natural / no over-processing after harvest) | ||
SEN_APP4 | In my opinion, organic food has a pleasant texture. | ||
Warm Glow (WG) | WG1 | Buying organic food gives me a pleasant feeling. | [17,21,51,66,67] |
WG2 | Consuming organic food makes me happy because it makes me feel that I have done something good. | ||
WG3 | I am happy when I can make choices to consume organic food, for myself and my family. | ||
WG4 | Every time I consume organic food, I feel good about myself because I have protected the environment. | ||
WG5 | I am satisfied because I have contributed to the efficiency of natural resources by consuming organic food. | ||
WG6 | Consuming organic food makes me feel good because it contributes to the preservation of the quality of the Earth (soil, water, etc.). | ||
WG7 | Purchasing organic food makes me happy because I am contributing to the well-being of farmers. | ||
WG8 | I am pleased that I have contributed to the growth of the local economy by purchasing organic food. | ||
Egoistic motive (E) | E1 | I eat organic food to better maintain my health. | [1,23,45,68] |
E2 | Organic food is the food that my family consumes for the sake of our family's health. | ||
E3 | I eat organic food to provide my body with more nutrition. | ||
E4 | I choose organic foods to obtain products that are safer to consume. |
The demographic characteristics of the 337 respondents are reported in Table 2. According to the age classification by the Ministry of Health of the Republic of Indonesia, the majority of the participants are under the age of 35, implying that the respondents in this study are young organic food consumers. The samples are not gendered balanced, i.e. the majority of consumers are women. Consumers with a bachelor's degree dominate the samples. Organic food consumers in this study are working people, with more than 60% of the total respondents are single.
Demographic Variable | N | % | |
Age (years old) | 17–25 | 179 | 53.12 |
26–35 | 97 | 28.78 | |
36–45 | 25 | 7.42 | |
56–55 | 31 | 9.20 | |
> 56 | 5 | 1.48 | |
Gender | Male | 95 | 28.19 |
Female | 242 | 71.81 | |
Education qualification | Higher secondary | 52 | 15.43 |
Graduate | 212 | 62.91 | |
Postgraduate | 71 | 21.07 | |
Any other | 2 | 0.59 | |
Marital status | Single | 210 | 62.31 |
Married | 127 | 37.69 | |
Employment status | Employed | 171 | 50.74 |
Unemployed | 12 | 3.56 | |
Retired | 2 | 0.59 | |
Homemaker | 37 | 10.98 | |
Student | 115 | 34.12 |
The measurement model used in this study is a reflective model, where the existence of indicators reflects the latent variables. Evaluation of the measurement model, also known as the outer model, is carried out by checking the value of indicator reliability, internal consistency reliability, convergent validity, and discriminant validity [60]. The reliability of the indicator is determined based on the value of the outer loading. The value of the outer loading or factor loading of an indicator must be > 0.70 [69]. The outer loading value between 0.40 and 0.70 needs to be considered by looking at the AVE value and indicators with an outer loading value < 0.40 must be removed. If the outer loading value meets the criteria, the indicator reliability is said to be feasible, and it can be used to evaluate other measurement models in the future.
Factor loading is a discriminant parameter that indicates how well an item can distinguish itself from others. Based on the evaluation results on the outer loading value (Appendix 1 (1)), all items have exceeded the limit of 0.7 (except SN6 on subjective norms and PBC1 on perceived behavioral control) and are considered significant on the latent variable. It can be concluded that the internal consistency reliability has met the criteria. SN6 and PBC1 (with insufficient charge) are excluded from further analysis. Furthermore, an evaluation of the reliability of internal consistency is carried out which is known based on the composite reliability value. In contrast to Cronbach's alpha, the value of composite reliability does not assume that all indicators are reliable. Composite reliability values are interpreted in the same way as Cronbrach Alpha [69].
Table 3 presents that the composite reliability value is > 0.70. Therefore, it means that the internal consistency reliability has met the criteria. The average variance extracted (AVE) value is used to confirm the defined convergent validity of the next measurement model. The AVE value of all constructs in this study, as presented in Table 3, has exceeded the recommended value of 0.5. This is consistent with the criteria, implying that the latent variable accounted for more than half of the indicator variance [69]. Furthermore, an evaluation of the discriminant validity is carried out. One of the discriminant validity of the reflective model can be evaluated based on the value of cross loading and the value of Heterotrait-Monotrait Ratio (HTMT). Based on the results summarized in Appendix 1 (2), it is known that, following the evaluation, the cross-loading value of each indicator is greater than the outer loading value to other constructs, indicating that the discriminant validity, based on the cross-loading value, meets the criteria.
CR | AVE | 1 | 2 | 3 | 4 | 5 | 6 | 7 | |
1.Attitude | 0.953 | 0.649 | |||||||
2.Egoistic motive | 0.943 | 0.806 | 0.808 | ||||||
3.Intention to re-consume | 0.955 | 0.781 | 0.589 | 0.536 | |||||
4.PBC | 0.918 | 0.650 | 0.531 | 0.485 | 0.605 | ||||
5.Sesory appeal | 0.905 | 0.705 | 0.524 | 0.365 | 0.541 | 0.485 | |||
6.Subjecctive norms | 0.931 | 0.731 | 0.473 | 0.345 | 0.394 | 0.401 | 0.476 | ||
7.Warm Glow | 0.959 | 0.747 | 0.768 | 0.689 | 0.547 | 0.494 | 0.452 | 0.394 |
HTMT is the best and latest way to determine discriminant validity [62]. The threshold criteria for the HTM value is < 0.90. All variables are known to have HTMT values < 0.90, as presented in Table 3. Thus, it is possible to conclude that the discriminant validity based on the cross-loading and HTMT values is good and meets the criteria. Based on all evaluations of the measurement model that have been carried outperformed, it is known that the indicators in this research model are valid and reliable, allowing for further evaluation of the structural model.
A structural model for organic food consumption is evaluated in the second stage of analysis. To investigate the hypothesis of this study, the structural model is assessed by examining the coefficient of determination (R2) and the appropriate t-values through bootstrap with a 5000 repeat sample. In addition to these indicators, it is necessary to report the effect size (f2 value) and predictive relevance (Q2) [60].
The R-square coefficient represents the combined effect of exogenous variables on endogenous latent variables. R-Square values ranged between 0 and 1 with higher values indicating predictive accuracy. In this model, the R2 value of the endogenous variable of attitude is 0.704 and the endogenous variable of intention to consume organic food again in this model is 0.453 (Figure 2). This depicts that together egoistic motive, warm glow, subjective norms, and perceived sensory appeal account for 70.4% of the variance in attitudes. Meanwhile, attitudes, subjective norms, PBC, and perceived sensory appeal together explain 45.3% of the variance in the intention to consume organic food again in the future.
The R-Square value of 0.20 is said to be high for disciplines such as consumer behavior [60]. However, in research related to satisfaction and loyalty, it is expected to be higher, namely greater than 0.75. According to the researchers [70], the value of 0.63 is said to be substantial, the value of 0.33 is moderate, and 0.19 is weak. Referring to these criteria, the R-Square value of the attitude variable in this study is substantial while the intention variable is moderate.
Table 4 demonstrates the results of the structural equation path coefficients and summarizes the results of hypothesis testing as shown in Figure 2. It can be seen the direct relationship of each variable. Sensory appeal (β = 0.141, p = 0.006), egoistic motive (β = 0.457, p = 0.000), warm glow (β = 0.333, p = 0.000) are positively and significantly related to consumer attitudes. Therefore these path, SN→ATT, SEN_APP→ATT, E→ATT, and WG→ATT, are supported. Furthermore, attitude (β = 0.289, p = 0.000), PBC (β = 0.313, p = 0.000), sensory appeal (β = 0.204, p = 0.002) are positively and significantly related to the intention to re-consumption of organic food in the future. However, subjective norms (β = 0.042, p = 0.414) have no significant effect on the intention to re-consume organic food in the future. Thus, ATT→INT, PBC→INT, and SEN_APP→INT are supported, while SN→INT is rejected.
Hypothesis | Path | Std Beta | t-value | Result | f2 |
H1 | ATT → INT | 0.289 | 3.518* | Supported | 0.097 |
SN → ATT | 0.112 | 3.271* | Supported | 0.033 | |
SN → INT | 0.042 | 0.816ns | Rejected | 0.002 | |
PBC → INT | 0.313 | 4.738* | Supported | 0.125 | |
H2 | SEN_APP → ATT | 0.141 | 2.763* | Supported | 0.050 |
SEN_APP → INT | 0.204 | 3.045* | Supported | 0.052 | |
H3 | E → ATT | 0.457 | 8.594* | Supported | 0.405 |
WG → ATT | 0.333 | 7.542* | Supported | 0.197 | |
Note: *p < 0.05. |
In addition to evaluating the R-Square value of all endogenous variables, the change in the R-Square value when certain exogenous variables are removed from the model can be used to determine whether the omitted exogenous variables have a substantive impact on the endogenous variables. This measure is referred to as the F-Square effect value. The criteria for assessing the F-Square is a value of 0.02 which describes a small effect, a value of 0.15 which describes a moderate effect, and a value of 0.35 describes a large influence of the exogenous latent variable on the R-Square value of the endogenous latent variable [60]. In this study, it is known that egoistic motive has a large effect on consumer attitudes and warm glow also has a moderate effect. Thus, the addition of the two exogenous variables constructs explains the attitude variance well.
In addition, the predictive relevance of the model (Q2) using the blindfolding procedure was assessed. If the Q2 value is greater than 0 the model has predictive relevance for a particular endogenous construct. The relative measures of predictive relevance, values of 0.02, 0.15, and 0.35 indicate that exogenous constructs have small, medium, or large predictive relevance for certain endogenous constructs [60]. In this study, the value of Q2 attitude is 0.44 while the intention is 0.35. Thus, showing that the model has great predictive relevance.
The main objective of this study is to identify the factors that explain attitudes and intentions to re-consuming organic food in the future in the case of Indonesia. This study focuses on consumers already consumed organic products and want to know how this organic consumption practice can become a habit. In this study, the strongest antecedent of behavior, intention, is used to predict future behavior. TPB is applied as the theoretical framework for this study, which is then expanded to include new constructions. The findings have revealed that attitudes and perceived behavioral control have a significant positive effect on the future intentions to re-consume organic food, while subjective norms fail to show a significant effect on purchase intention. Furthermore, the addition of the perceived sensory appeal construct shows a significant positive effect on the intention.
Perceived behavioral control has emerged as the most significant determinant of intention to re-consume organic food in the future. This finding is consistent with the outcomes of the previous studies [1,9,63]. This shows that consumers can control their behavior through internal control over the extrinsic factors associated with purchasing organic food. According to the researcher [9], the greatest impact of PBC on intentions might suggest that the low consumption of organic food could be overcome by increasing product availability. Lack of availability is one of the main barriers to organic food consumption [10,68]. Furthermore, attitudes are identified to be better antecedents of intentions. This supports previous findings, which state that attitudes play a significant role in behavioral intentions [1,9,32,71]. Therefore, it is important to pay close attention to the factors that shape consumer attitudes towards organic food.
In this study, subjective norms are non-significant predictors of intention to re-consume organic food. This is consistent with several previous studies [1,16,30,35] that found no direct relationship between subjective norms and intentions in developing countries. This may be because of organic food is a product that has only been consumed by a small number of people, so the practice of consuming organic food has not become a social norm in society. Subjective norms and attitudes, on the other hand, have yielded different results, indicating a significant positive relationship. As a result, the influence of other people's opinions shapes consumers' positive attitudes toward organic food ingredients. These findings support the proposed new model [30,72] of the relationship. Consumers' preference for organic food is influenced by how the people important to them believe about the consumption behavior. Consumers' positive (or negative) attitudes toward organic food purchases are "downgraded". Thus, people who favor organic food ingredients can influence the formation of other consumer attitudes. However, this influence does not directly shape the intention.
Furthermore, our findings suggest that sensory attributes may contribute to consumers' intention to re-consume organic food in the future. This exemplifies that consumer experiences with appearance, taste, texture and other attributes encourage consumers to re-consume the product. Similarly, sensory attractiveness has been proven to have a positive and significant effect on consumer attitudes, supporting the research findings [18] that sensory attractiveness is a variable that affects both consumer attitudes and purchasing behavior related to organic products. This shows a positive evaluation of the experience of consuming organic food to encourage intention. The theoretical and managerial implications discovered in this study are discussed further.
Finally, egoistic motive (concern for health) and impure altruistic motive (warm glow) have been proven to have a strong influence on the attitudes of consumers of organic food. These results suggest that the addition of these two constructs can adequately explain the attitude variance. The theoretical and managerial implications of these findings will be discussed in further detail depth later.
This study provides theoretical contribution by introducing the concept of impure altruism, namely the warm glow obtained from pro-environmental and pro-social behavior. In this study, pro-environmental behavior is defined as organic food consumption motivated by environmental concerns, while pro-social behavior is defined as organic food consumption motivated by the welfare of local farmers. Altruistic motives may be considered detrimental because they tend to disregard personal well-being [21], but Andreoni [56] introduces the concept of a warm glow of giving, which suggests that consumers derive psychological benefits from these altruistic actions. By taking these motives into account, it is possible to predict attitudes (better than purely altruistic motives), in addition to self-interest (egoistic motives).
Only a few studies apply warm glow in the case of pro-environmental behavior [67,73,74,75], especially in the case of organic food consumption [17,51] and this study contributes to the gap. Furthermore, these findings provide new insight for TPB. The results of this study indicate that the addition of egoistic and warm glow motives increases the explanatory power of attitudes. Furthermore, the addition of perceived sensory appeal complements the TPB model, particularly to predict the consumption behavior of organic food, which can be used as a managerial basis.
This research may not generally be a description of the behavior of organic consumers in Indonesia given the narrow sample obtained. But, this study can be an alternative reference for marketers and public policy makers who promote pro-environmental and pro-social products and behaviors, especially for young consumers considering the distribution of these respondents who are mostly young organic consumers. This current study has discovered that attitudes and PBC influence intentions in TPB. Online sales can be initiated in developing countries such as Indonesia. Furthermore, by disseminating information through food campaigns and attempting to create a positive image of organic food, marketers can make positive evaluations of organic food.
Subjective norm is reported to influence consumer attitudes. Consequently, marketers and public policymakers can use reference groups such as presidents/bureaucrats, celebrities, environmentalists, teachers, as well as testimonials from organic food consumers to highlight organic food consumption, which is expected to be a positive evaluation of others. A large number of people are typically interested in adopting the lifestyle of the reference group. In Indonesia, social media has become a platform favored by young consumers. In line with these findings, brands through social media such as artists on Instagram who campaign for the health and environmental benefits of organic food can be a good choice in a marketing strategy. Following up on the findings of sensory appeal that shape consumer attitudes and intentions, it can be concluded that sensory branding strategy can be used as a foundation for marketers to use in marketing organic food.
Marketers can further emphasize sensory attributes in promoting organic food, which is considered superior to conventional products. Likewise, consumer perceptions of the quality of organic rice that are tastier and more durable can be used as ingredients to promote organic rice. As well as the sensory advantage possessed by organic vegetables, which is fresher. Consumer satisfaction with organic attributes is expected to result in positive evaluations that encourage consumers to become regular consumers of organic food.
On the other hand, the significance of egoistic motive and warm glow becomes the basis for not only emphasizing appearance, taste, and other physical attributes but also introducing the values of organic food, as well as campaigning for organic as a healthy and safe food. Given that egoistic motives are the most powerful factor in explaining attitudes, advocating for the attributes of the belief in nutritional content and the benefits for health help consumers evaluate organic food positively. Other promotions can also be carried out by creating a warm glow when consumers see organic food advertisements. Marketing strategy emphasizes deeply the inner values, such as organic product advertisements that lead consumers to feel good because they contribute to social welfare. Consuming organic food, for example, implies the contribution to the preservation of the Earth, whereas purchasing organic food means that you have contributed to environmental sustainability. The campaign then raises the awareness of emotional rewards.
This study is limited to measuring the intention to re-consume organic food in the future and there is no measurement of actual consumption behavior. Although previous findings have supported that intention is positively correlated with behavior, many empirical studies have shown gaps in these results. To better explain this relationship and reduce this gap, experts have suggested moderator variables [76]. In further research, the action that can be taken to overcome this gap is to add a moderating variable of price perception. This is because high prices can prevent consumers with high purchase intentions from actually buying organic food [12]. The moderating effect of perceived price might provide insight into the strength of the relationship between intention and behavior.
In addition, further research related to intention to re-consume may involve behavioral variables of past organic food consumption. Past behavior can be an input for someone to behave. Past behavior reflects the control of various factors, both internal and external, which in the long run form a repetitive behavior. Previous studies have suggested that instead of deliberately considering the benefits of engaging in certain types of behavior, individuals can base their intentions on previously encountered experiences [29,77,78].
This study is limited to measuring organic food in the raw food category so that the results of this study cannot be generalized to processed products, such as milk, yogurt, and others. In this study, organic food was measured in general terms whereas previous studies have shown that consumption of organic food differs among various food products. Future research may be able to compare consumer intentions and behavior towards various ranges of organic food products.
The limited number of samples and respondents' own selection bias may also be a problem in this study as those who are more health conscious, pro-environmental and pro-social about organic food may be mostly in this study resulting in overrepresentation of these people in the sample. In addition, the respondents in this study were dominated by young consumers with higher education. This allows for a bias towards answers because consumers already have knowledge of organic food. Therefore, future research can be carried out by considering the consumer knowledge variable.
In the case of a developing country, Indonesia, this study has proven the application of the theory of planned behavior model, which is then expanded by adding a novel construct to measure the intention to re-consume organic food in the future. This study supports the general findings of the TPB and confirms that in the countries where organic consumption is uncommon, establishing subjective norms does not affect behavior. This study has contributed to the literature on organic food consumer behavior, particularly in developing countries, by including additional constructs, such as the perception of sensory attractiveness, egoistic motive, and warm glow. The addition of these constructs has improved the predictions of the theoretical framework because all of them significantly influence consumer attitudes and intentions. Some of the gaps in this research can be used as material for the development of further research. The results of this study can be used as a reference choice in the strategy of developing organic consumption in Indonesia.
Items | Outer Loading | Cross Loading | ||||||||||||
ATT | E | INT | PBC | SEN_APP | SN | WG | ATT | E | INT | PBC | SEN_APP | SN | WG | |
SEN_APP.1 | 0.812 | 0.342 | 0.219 | 0.352 | 0.328 | 0.812 | 0.346 | 0.282 | ||||||
SEN_APP.2 | 0.842 | 0.356 | 0.247 | 0.390 | 0.302 | 0.842 | 0.387 | 0.296 | ||||||
SEN_APP.3 | 0.859 | 0.469 | 0.312 | 0.411 | 0.393 | 0.859 | 0.317 | 0.395 | ||||||
SEN_APP.4 | 0.845 | 0.418 | 0.314 | 0.490 | 0.407 | 0.845 | 0.362 | 0.400 | ||||||
WG.1 | 0.839 | 0.657 | 0.605 | 0.429 | 0.394 | 0.351 | 0.357 | 0.839 | ||||||
WG.2 | 0.837 | 0.590 | 0.511 | 0.468 | 0.423 | 0.353 | 0.311 | 0.837 | ||||||
WG.3 | 0.860 | 0.621 | 0.605 | 0.448 | 0.387 | 0.348 | 0.336 | 0.860 | ||||||
WG.4 | 0.902 | 0.648 | 0.579 | 0.468 | 0.410 | 0.381 | 0.314 | 0.902 | ||||||
WG.5 | 0.896 | 0.646 | 0.554 | 0.458 | 0.388 | 0.354 | 0.315 | 0.896 | ||||||
WG.6 | 0.879 | 0.644 | 0.618 | 0.425 | 0.383 | 0.335 | 0.271 | 0.879 | ||||||
WG.7 | 0.854 | 0.600 | 0.474 | 0.473 | 0.386 | 0.393 | 0.334 | 0.854 | ||||||
WG.8 | 0.847 | 0.625 | 0.512 | 0.422 | 0.363 | 0.359 | 0.315 | 0.847 | ||||||
SN.1 | 0.858 | 0.327 | 0.308 | 0.314 | 0.333 | 0.340 | 0.858 | 0.266 | ||||||
SN.2 | 0.889 | 0.370 | 0.291 | 0.342 | 0.356 | 0.372 | 0.889 | 0.304 | ||||||
SN.3 | 0.868 | 0.429 | 0.294 | 0.324 | 0.299 | 0.351 | 0.868 | 0.377 | ||||||
SN.4 | 0.781 | 0.326 | 0.156 | 0.234 | 0.245 | 0.356 | 0.781 | 0.275 | ||||||
SN.5 | 0.875 | 0.415 | 0.298 | 0.348 | 0.314 | 0.374 | 0.875 | 0.341 | ||||||
PBC.2 | 0.807 | 0.347 | 0.386 | 0.454 | 0.807 | 0.323 | 0.302 | 0.353 | ||||||
PBC.3 | 0.865 | 0.434 | 0.410 | 0.491 | 0.865 | 0.342 | 0.408 | 0.359 | ||||||
PBC.4 | 0.746 | 0.337 | 0.296 | 0.415 | 0.746 | 0.321 | 0.192 | 0.359 | ||||||
PBC.5 | 0.824 | 0.449 | 0.347 | 0.486 | 0.824 | 0.397 | 0.296 | 0.377 | ||||||
PBC.6 | 0.766 | 0.369 | 0.331 | 0.376 | 0.766 | 0.349 | 0.328 | 0.376 | ||||||
PBC.7 | 0.824 | 0.421 | 0.350 | 0.462 | 0.824 | 0.350 | 0.226 | 0.375 | ||||||
E.1 | 0.899 | 0.682 | 0.899 | 0.420 | 0.357 | 0.281 | 0.296 | 0.583 | ||||||
E.2 | 0.888 | 0.655 | 0.888 | 0.449 | 0.431 | 0.285 | 0.316 | 0.556 | ||||||
E.3 | 0.907 | 0.702 | 0.907 | 0.463 | 0.402 | 0.299 | 0.301 | 0.584 | ||||||
E.4 | 0.898 | 0.670 | 0.898 | 0.460 | 0.390 | 0.321 | 0.234 | 0.597 | ||||||
INT.1 | 0.900 | 0.462 | 0.481 | 0.900 | 0.504 | 0.407 | 0.318 | 0.448 | ||||||
INT.2 | 0.904 | 0.461 | 0.435 | 0.904 | 0.504 | 0.409 | 0.322 | 0.427 | ||||||
INT.3 | 0.886 | 0.507 | 0.466 | 0.886 | 0.476 | 0.448 | 0.335 | 0.469 | ||||||
INT.4 | 0.908 | 0.526 | 0.448 | 0.908 | 0.482 | 0.437 | 0.316 | 0.466 | ||||||
INT.5 | 0.896 | 0.517 | 0.462 | 0.896 | 0.495 | 0.420 | 0.315 | 0.459 | ||||||
INT.6 | 0.803 | 0.481 | 0.353 | 0.803 | 0.492 | 0.494 | 0.345 | 0.475 | ||||||
ATT.1 | 0.847 | 0.847 | 0.610 | 0.439 | 0.413 | 0.367 | 0.312 | 0.613 | ||||||
ATT.10 | 0.857 | 0.857 | 0.647 | 0.492 | 0.383 | 0.384 | 0.356 | 0.618 | ||||||
ATT.11 | 0.778 | 0.778 | 0.515 | 0.514 | 0.417 | 0.441 | 0.408 | 0.633 | ||||||
ATT.2 | 0.843 | 0.843 | 0.629 | 0.414 | 0.420 | 0.357 | 0.325 | 0.615 | ||||||
ATT.3 | 0.750 | 0.750 | 0.565 | 0.413 | 0.384 | 0.423 | 0.424 | 0.564 | ||||||
ATT.4 | 0.803 | 0.803 | 0.615 | 0.429 | 0.462 | 0.370 | 0.441 | 0.579 | ||||||
ATT.5 | 0.858 | 0.858 | 0.660 | 0.475 | 0.373 | 0.355 | 0.276 | 0.585 | ||||||
ATT.6 | 0.723 | 0.723 | 0.498 | 0.375 | 0.399 | 0.404 | 0.351 | 0.556 | ||||||
ATT.7 | 0.758 | 0.758 | 0.605 | 0.422 | 0.337 | 0.345 | 0.306 | 0.540 | ||||||
ATT.8 | 0.766 | 0.766 | 0.621 | 0.440 | 0.317 | 0.414 | 0.385 | 0.554 | ||||||
ATT.9 | 0.865 | 0.865 | 0.705 | 0.516 | 0.429 | 0.371 | 0.324 | 0.594 | ||||||
Description: ATT: Attitude; E: Egoistic; INT: Intention to re-consume; PBC: Perceived Behavioral Control; SEN_APP: Sensory Appeal Perceived; SN: Subjective norm; WG: Warm Glow. |
Mean | Standard Deviation | Matrix Correlations | ||||||||||
WG.1 | WG.2 | WG.3 | WG.4 | WG.5 | WG.6 | WG.7 | WG.8 | E.1 | E.2 | |||
WG.1 | 4.228 | 0.738 | 1.000 | |||||||||
WG.2 | 4.267 | 0.702 | 0.771 | 1.000 | ||||||||
WG.3 | 4.270 | 0.744 | 0.774 | 0.726 | 1.000 | |||||||
WG.4 | 4.285 | 0.740 | 0.690 | 0.693 | 0.755 | 1.000 | ||||||
WG.5 | 4.279 | 0.778 | 0.654 | 0.685 | 0.721 | 0.852 | 1.000 | |||||
WG.6 | 4.356 | 0.713 | 0.652 | 0.658 | 0.703 | 0.814 | 0.833 | 1.000 | ||||
WG.7 | 4.246 | 0.764 | 0.632 | 0.652 | 0.630 | 0.716 | 0.724 | 0.700 | 1.000 | |||
WG.8 | 4.208 | 0.792 | 0.620 | 0.621 | 0.635 | 0.704 | 0.715 | 0.705 | 0.867 | 1.000 | ||
E.1 | 4.513 | 0.702 | 0.564 | 0.450 | 0.570 | 0.535 | 0.499 | 0.571 | 0.401 | 0.432 | 1.000 | |
E.2 | 4.288 | 0.806 | 0.513 | 0.441 | 0.524 | 0.494 | 0.468 | 0.508 | 0.425 | 0.464 | 0.735 | 1.000 |
E.3 | 4.365 | 0.774 | 0.529 | 0.465 | 0.535 | 0.523 | 0.516 | 0.544 | 0.435 | 0.486 | 0.730 | 0.758 |
E.4 | 4.501 | 0.698 | 0.567 | 0.478 | 0.545 | 0.527 | 0.508 | 0.595 | 0.442 | 0.456 | 0.764 | 0.703 |
E.5 | 3.202 | 1.211 | 0.307 | 0.289 | 0.289 | 0.283 | 0.303 | 0.226 | 0.264 | 0.318 | 0.283 | 0.302 |
E.6 | 3.685 | 1.117 | 0.454 | 0.421 | 0.431 | 0.421 | 0.395 | 0.398 | 0.418 | 0.466 | 0.433 | 0.413 |
E.7 | 3.644 | 1.152 | 0.448 | 0.426 | 0.382 | 0.401 | 0.392 | 0.382 | 0.420 | 0.393 | 0.321 | 0.347 |
ATT.1 | 4.395 | 0.659 | 0.608 | 0.510 | 0.515 | 0.554 | 0.543 | 0.560 | 0.467 | 0.474 | 0.569 | 0.529 |
ATT.2 | 4.344 | 0.693 | 0.577 | 0.512 | 0.522 | 0.555 | 0.565 | 0.538 | 0.473 | 0.507 | 0.569 | 0.566 |
ATT.3 | 4.145 | 0.847 | 0.479 | 0.469 | 0.517 | 0.506 | 0.492 | 0.465 | 0.458 | 0.512 | 0.498 | 0.560 |
ATT.4 | 4.193 | 0.771 | 0.522 | 0.470 | 0.499 | 0.527 | 0.514 | 0.485 | 0.468 | 0.512 | 0.502 | 0.588 |
ATT.5 | 4.469 | 0.640 | 0.534 | 0.494 | 0.476 | 0.526 | 0.519 | 0.571 | 0.450 | 0.470 | 0.587 | 0.578 |
ATT.6 | 4.139 | 0.834 | 0.527 | 0.469 | 0.489 | 0.464 | 0.475 | 0.450 | 0.491 | 0.482 | 0.460 | 0.439 |
ATT.7 | 4.475 | 0.739 | 0.498 | 0.465 | 0.469 | 0.474 | 0.477 | 0.479 | 0.445 | 0.425 | 0.582 | 0.503 |
ATT.8 | 4.329 | 0.783 | 0.471 | 0.423 | 0.479 | 0.489 | 0.512 | 0.508 | 0.450 | 0.493 | 0.556 | 0.489 |
ATT.9 | 4.528 | 0.631 | 0.551 | 0.439 | 0.518 | 0.523 | 0.529 | 0.578 | 0.469 | 0.487 | 0.648 | 0.553 |
ATT.10 | 4.481 | 0.654 | 0.523 | 0.470 | 0.520 | 0.600 | 0.582 | 0.588 | 0.482 | 0.500 | 0.619 | 0.548 |
ATT.11 | 4.362 | 0.739 | 0.534 | 0.518 | 0.497 | 0.517 | 0.506 | 0.471 | 0.663 | 0.678 | 0.437 | 0.448 |
SN.1 | 3.706 | 0.953 | 0.281 | 0.237 | 0.292 | 0.220 | 0.247 | 0.171 | 0.193 | 0.199 | 0.301 | 0.303 |
SN.2 | 3.712 | 0.976 | 0.297 | 0.273 | 0.279 | 0.245 | 0.262 | 0.211 | 0.270 | 0.269 | 0.268 | 0.294 |
SN.3 | 3.742 | 0.972 | 0.384 | 0.319 | 0.363 | 0.333 | 0.327 | 0.282 | 0.325 | 0.274 | 0.268 | 0.265 |
SN.4 | 3.724 | 0.992 | 0.253 | 0.230 | 0.218 | 0.232 | 0.215 | 0.189 | 0.313 | 0.258 | 0.157 | 0.159 |
SN.5 | 3.733 | 0.990 | 0.299 | 0.261 | 0.271 | 0.298 | 0.282 | 0.286 | 0.323 | 0.336 | 0.261 | 0.312 |
SN.6 | 3.932 | 0.974 | 0.381 | 0.244 | 0.345 | 0.295 | 0.303 | 0.279 | 0.334 | 0.307 | 0.307 | 0.275 |
SN.7 | 2.861 | 1.315 | 0.131 | 0.108 | 0.087 | 0.074 | 0.108 | 0.043 | 0.132 | 0.159 | 0.058 | 0.074 |
PBC.1 | 4.318 | 0.733 | 0.310 | 0.331 | 0.327 | 0.271 | 0.298 | 0.306 | 0.274 | 0.244 | 0.289 | 0.252 |
PBC.2 | 4.181 | 0.680 | 0.314 | 0.309 | 0.267 | 0.304 | 0.286 | 0.314 | 0.325 | 0.321 | 0.302 | 0.349 |
PBC.3 | 4.160 | 0.713 | 0.325 | 0.336 | 0.293 | 0.330 | 0.326 | 0.273 | 0.309 | 0.293 | 0.322 | 0.431 |
PBC.4 | 4.228 | 0.726 | 0.329 | 0.335 | 0.309 | 0.277 | 0.303 | 0.302 | 0.321 | 0.310 | 0.271 | 0.258 |
PBC.5 | 4.240 | 0.679 | 0.323 | 0.388 | 0.312 | 0.360 | 0.322 | 0.307 | 0.321 | 0.277 | 0.270 | 0.356 |
PBC.6 | 4.039 | 0.841 | 0.287 | 0.349 | 0.363 | 0.349 | 0.337 | 0.323 | 0.295 | 0.300 | 0.243 | 0.364 |
PBC.7 | 4.291 | 0.657 | 0.328 | 0.334 | 0.343 | 0.367 | 0.312 | 0.343 | 0.301 | 0.266 | 0.313 | 0.324 |
SEN_APP.1 | 4.104 | 0.761 | 0.243 | 0.265 | 0.228 | 0.248 | 0.202 | 0.183 | 0.313 | 0.274 | 0.167 | 0.203 |
SEN_APP.2 | 4.053 | 0.745 | 0.242 | 0.251 | 0.269 | 0.263 | 0.266 | 0.249 | 0.248 | 0.258 | 0.203 | 0.212 |
SEN_APP.3 | 4.276 | 0.688 | 0.344 | 0.302 | 0.347 | 0.370 | 0.350 | 0.326 | 0.362 | 0.331 | 0.278 | 0.253 |
SEN_APP.4 | 4.172 | 0.689 | 0.331 | 0.353 | 0.309 | 0.375 | 0.348 | 0.340 | 0.382 | 0.331 | 0.277 | 0.279 |
INT.1 | 4.374 | 0.594 | 0.387 | 0.423 | 0.416 | 0.399 | 0.397 | 0.365 | 0.379 | 0.333 | 0.408 | 0.432 |
INT.2 | 4.374 | 0.604 | 0.354 | 0.416 | 0.376 | 0.399 | 0.359 | 0.359 | 0.379 | 0.315 | 0.359 | 0.388 |
INT.3 | 4.276 | 0.670 | 0.413 | 0.436 | 0.398 | 0.410 | 0.416 | 0.366 | 0.424 | 0.384 | 0.374 | 0.457 |
INT.4 | 4.350 | 0.603 | 0.380 | 0.438 | 0.411 | 0.421 | 0.431 | 0.393 | 0.392 | 0.357 | 0.395 | 0.366 |
INT.5 | 4.392 | 0.597 | 0.383 | 0.409 | 0.416 | 0.405 | 0.416 | 0.404 | 0.400 | 0.342 | 0.412 | 0.400 |
INT.6 | 4.326 | 0.658 | 0.354 | 0.357 | 0.353 | 0.442 | 0.402 | 0.359 | 0.525 | 0.496 | 0.279 | 0.337 |
E.3 | E.4 | E.5 | E.6 | E.7 | ATT.1 | ATT.2 | ATT.3 | ATT.4 | ATT.5 | ATT.6 | ATT.7 | |
E.3 | 1.000 | |||||||||||
E.4 | 0.759 | 1.000 | ||||||||||
E.5 | 0.320 | 0.221 | 1.000 | |||||||||
E.6 | 0.452 | 0.392 | 0.703 | 1.000 | ||||||||
E.7 | 0.362 | 0.303 | 0.660 | 0.741 | 1.000 | |||||||
ATT.1 | 0.537 | 0.556 | 0.268 | 0.390 | 0.376 | 1.000 | ||||||
ATT.2 | 0.578 | 0.544 | 0.320 | 0.415 | 0.425 | 0.858 | 1.000 | |||||
ATT.3 | 0.525 | 0.449 | 0.414 | 0.475 | 0.384 | 0.625 | 0.657 | 1.000 | ||||
ATT.4 | 0.612 | 0.504 | 0.359 | 0.432 | 0.425 | 0.650 | 0.697 | 0.656 | 1.000 | |||
ATT.5 | 0.601 | 0.603 | 0.192 | 0.331 | 0.307 | 0.757 | 0.720 | 0.597 | 0.677 | 1.000 | ||
ATT.6 | 0.445 | 0.446 | 0.372 | 0.429 | 0.438 | 0.591 | 0.579 | 0.534 | 0.618 | 0.567 | 1.000 | |
ATT.7 | 0.532 | 0.557 | 0.148 | 0.289 | 0.279 | 0.572 | 0.544 | 0.421 | 0.521 | 0.602 | 0.461 | 1.000 |
ATT.8 | 0.639 | 0.539 | 0.337 | 0.454 | 0.400 | 0.530 | 0.529 | 0.514 | 0.558 | 0.610 | 0.480 | 0.607 |
ATT.9 | 0.650 | 0.679 | 0.191 | 0.329 | 0.271 | 0.676 | 0.650 | 0.551 | 0.602 | 0.740 | 0.531 | 0.723 |
ATT.10 | 0.573 | 0.583 | 0.222 | 0.353 | 0.322 | 0.661 | 0.669 | 0.565 | 0.599 | 0.717 | 0.552 | 0.688 |
ATT.11 | 0.511 | 0.454 | 0.247 | 0.422 | 0.354 | 0.578 | 0.568 | 0.542 | 0.549 | 0.589 | 0.549 | 0.566 |
SN.1 | 0.278 | 0.226 | 0.386 | 0.298 | 0.302 | 0.227 | 0.247 | 0.299 | 0.344 | 0.197 | 0.268 | 0.244 |
SN.2 | 0.284 | 0.199 | 0.363 | 0.292 | 0.273 | 0.269 | 0.278 | 0.366 | 0.389 | 0.216 | 0.279 | 0.247 |
SN.3 | 0.283 | 0.239 | 0.372 | 0.371 | 0.416 | 0.326 | 0.343 | 0.388 | 0.407 | 0.285 | 0.312 | 0.315 |
SN.4 | 0.154 | 0.088 | 0.333 | 0.307 | 0.363 | 0.212 | 0.224 | 0.366 | 0.349 | 0.176 | 0.344 | 0.211 |
SN.5 | 0.270 | 0.228 | 0.347 | 0.370 | 0.349 | 0.284 | 0.281 | 0.390 | 0.390 | 0.287 | 0.308 | 0.279 |
SN.6 | 0.281 | 0.260 | 0.261 | 0.305 | 0.365 | 0.319 | 0.303 | 0.350 | 0.349 | 0.332 | 0.399 | 0.305 |
SN.7 | 0.065 | 0.021 | 0.396 | 0.330 | 0.314 | 0.101 | 0.114 | 0.242 | 0.167 | 0.032 | 0.229 | 0.044 |
PBC.1 | 0.287 | 0.309 | 0.122 | 0.111 | 0.148 | 0.287 | 0.270 | 0.208 | 0.228 | 0.265 | 0.219 | 0.209 |
PBC.2 | 0.365 | 0.371 | 0.161 | 0.196 | 0.219 | 0.244 | 0.233 | 0.238 | 0.329 | 0.282 | 0.280 | 0.260 |
PBC.3 | 0.383 | 0.339 | 0.217 | 0.216 | 0.214 | 0.358 | 0.375 | 0.335 | 0.446 | 0.323 | 0.337 | 0.329 |
PBC.4 | 0.253 | 0.283 | 0.241 | 0.220 | 0.207 | 0.277 | 0.262 | 0.255 | 0.266 | 0.255 | 0.295 | 0.224 |
PBC.5 | 0.301 | 0.321 | 0.161 | 0.182 | 0.223 | 0.418 | 0.398 | 0.367 | 0.416 | 0.342 | 0.381 | 0.322 |
PBC.6 | 0.325 | 0.260 | 0.287 | 0.259 | 0.256 | 0.288 | 0.359 | 0.354 | 0.409 | 0.248 | 0.293 | 0.214 |
PBC.7 | 0.316 | 0.303 | 0.169 | 0.214 | 0.227 | 0.399 | 0.399 | 0.313 | 0.364 | 0.339 | 0.337 | 0.266 |
SEN_APP.1 | 0.213 | 0.203 | 0.341 | 0.349 | 0.296 | 0.279 | 0.258 | 0.335 | 0.304 | 0.229 | 0.342 | 0.229 |
SEN_APP.2 | 0.244 | 0.228 | 0.347 | 0.359 | 0.278 | 0.253 | 0.263 | 0.364 | 0.282 | 0.246 | 0.294 | 0.223 |
SEN_APP.3 | 0.273 | 0.317 | 0.225 | 0.283 | 0.251 | 0.401 | 0.348 | 0.390 | 0.358 | 0.374 | 0.362 | 0.373 |
SEN_APP.4 | 0.266 | 0.308 | 0.250 | 0.329 | 0.335 | 0.288 | 0.317 | 0.333 | 0.295 | 0.322 | 0.356 | 0.312 |
INT.1 | 0.439 | 0.449 | 0.209 | 0.240 | 0.260 | 0.358 | 0.336 | 0.322 | 0.348 | 0.390 | 0.302 | 0.400 |
INT.2 | 0.400 | 0.414 | 0.221 | 0.214 | 0.268 | 0.382 | 0.352 | 0.317 | 0.323 | 0.407 | 0.303 | 0.367 |
INT.3 | 0.423 | 0.421 | 0.260 | 0.298 | 0.354 | 0.405 | 0.409 | 0.410 | 0.431 | 0.432 | 0.356 | 0.359 |
INT.4 | 0.431 | 0.414 | 0.200 | 0.247 | 0.260 | 0.406 | 0.400 | 0.376 | 0.416 | 0.451 | 0.351 | 0.412 |
INT.5 | 0.429 | 0.418 | 0.198 | 0.260 | 0.267 | 0.421 | 0.355 | 0.386 | 0.383 | 0.428 | 0.343 | 0.419 |
INT.6 | 0.331 | 0.322 | 0.237 | 0.305 | 0.286 | 0.353 | 0.339 | 0.372 | 0.367 | 0.405 | 0.328 | 0.279 |
ATT.8 | ATT.9 | ATT.10 | ATT.11 | SN.1 | SN.2 | SN.3 | SN.4 | SN.5 | SN.6 | SN.7 | PBC.1 | |
ATT.8 | 1.000 | |||||||||||
ATT.9 | 0.694 | 1.000 | ||||||||||
ATT.10 | 0.624 | 0.802 | 1.000 | |||||||||
ATT.11 | 0.620 | 0.641 | 0.672 | 1.000 | ||||||||
SN.1 | 0.289 | 0.243 | 0.274 | 0.273 | 1.000 | |||||||
SN.2 | 0.326 | 0.271 | 0.291 | 0.350 | 0.764 | 1.000 | ||||||
SN.3 | 0.357 | 0.334 | 0.354 | 0.382 | 0.687 | 0.697 | 1.000 | |||||
SN.4 | 0.274 | 0.200 | 0.241 | 0.311 | 0.552 | 0.568 | 0.653 | 1.000 | ||||
SN.5 | 0.382 | 0.316 | 0.345 | 0.412 | 0.672 | 0.750 | 0.653 | 0.636 | 1.000 | |||
SN.6 | 0.361 | 0.349 | 0.368 | 0.360 | 0.423 | 0.401 | 0.480 | 0.595 | 0.483 | 1.000 | ||
SN.7 | 0.154 | 0.067 | 0.116 | 0.196 | 0.467 | 0.461 | 0.422 | 0.524 | 0.489 | 0.421 | 1.000 | |
PBC.1 | 0.185 | 0.337 | 0.257 | 0.210 | 0.121 | 0.115 | 0.115 | 0.043 | 0.043 | 0.064 | -0.040 | 1.000 |
PBC.2 | 0.256 | 0.358 | 0.271 | 0.313 | 0.293 | 0.302 | 0.214 | 0.149 | 0.319 | 0.167 | 0.018 | 0.468 |
PBC.3 | 0.272 | 0.353 | 0.338 | 0.380 | 0.371 | 0.420 | 0.329 | 0.251 | 0.359 | 0.272 | 0.109 | 0.436 |
PBC.4 | 0.265 | 0.307 | 0.244 | 0.333 | 0.174 | 0.181 | 0.168 | 0.174 | 0.130 | 0.127 | 0.046 | 0.650 |
PBC.5 | 0.225 | 0.383 | 0.368 | 0.365 | 0.270 | 0.256 | 0.260 | 0.231 | 0.250 | 0.218 | 0.057 | 0.335 |
PBC.6 | 0.242 | 0.280 | 0.306 | 0.278 | 0.310 | 0.339 | 0.277 | 0.201 | 0.269 | 0.166 | 0.077 | 0.360 |
PBC.7 | 0.275 | 0.381 | 0.317 | 0.339 | 0.189 | 0.223 | 0.196 | 0.173 | 0.183 | 0.198 | 0.033 | 0.424 |
SEN_APP.1 | 0.281 | 0.207 | 0.228 | 0.355 | 0.308 | 0.316 | 0.269 | 0.309 | 0.289 | 0.226 | 0.213 | 0.138 |
SEN_APP.2 | 0.326 | 0.281 | 0.307 | 0.315 | 0.336 | 0.364 | 0.343 | 0.305 | 0.309 | 0.230 | 0.217 | 0.148 |
SEN_APP.3 | 0.394 | 0.376 | 0.385 | 0.399 | 0.255 | 0.273 | 0.284 | 0.290 | 0.261 | 0.299 | 0.121 | 0.238 |
SEN_APP.4 | 0.374 | 0.358 | 0.350 | 0.402 | 0.258 | 0.307 | 0.288 | 0.295 | 0.389 | 0.261 | 0.138 | 0.227 |
INT.1 | 0.348 | 0.471 | 0.408 | 0.395 | 0.288 | 0.308 | 0.260 | 0.195 | 0.296 | 0.301 | 0.082 | 0.382 |
INT.2 | 0.336 | 0.440 | 0.424 | 0.415 | 0.284 | 0.313 | 0.265 | 0.182 | 0.316 | 0.281 | 0.088 | 0.375 |
INT.3 | 0.392 | 0.428 | 0.422 | 0.440 | 0.290 | 0.307 | 0.292 | 0.213 | 0.317 | 0.338 | 0.128 | 0.341 |
INT.4 | 0.397 | 0.504 | 0.476 | 0.455 | 0.267 | 0.292 | 0.301 | 0.191 | 0.286 | 0.283 | 0.103 | 0.346 |
INT.5 | 0.428 | 0.498 | 0.467 | 0.432 | 0.270 | 0.285 | 0.281 | 0.208 | 0.292 | 0.296 | 0.077 | 0.360 |
INT.6 | 0.425 | 0.392 | 0.407 | 0.581 | 0.266 | 0.303 | 0.312 | 0.247 | 0.334 | 0.252 | 0.114 | 0.259 |
PBC.2 | PBC.3 | PBC.4 | PBC.5 | PBC.6 | PBC.7 | SEN_APP.1 | SEN_APP.2 | SEN_APP.3 | SEN_APP.4 | INT.1 | INT.2 | |
PBC.2 | 1.000 | |||||||||||
PBC.3 | 0.723 | 1.000 | ||||||||||
PBC.4 | 0.487 | 0.554 | 1.000 | |||||||||
PBC.5 | 0.593 | 0.643 | 0.533 | 1.000 | ||||||||
PBC.6 | 0.501 | 0.613 | 0.530 | 0.534 | 1.000 | |||||||
PBC.7 | 0.573 | 0.616 | 0.545 | 0.641 | 0.602 | 1.000 | ||||||
SEN_APP.1 | 0.233 | 0.275 | 0.236 | 0.307 | 0.267 | 0.266 | 1.000 | |||||
SEN_APP.2 | 0.215 | 0.230 | 0.197 | 0.285 | 0.271 | 0.265 | 0.686 | 1.000 | ||||
SEN_APP.3 | 0.293 | 0.309 | 0.320 | 0.360 | 0.289 | 0.328 | 0.586 | 0.603 | 1.000 | |||
SEN_APP.4 | 0.326 | 0.324 | 0.307 | 0.368 | 0.337 | 0.309 | 0.532 | 0.589 | 0.658 | 1.000 | ||
INT.1 | 0.420 | 0.461 | 0.366 | 0.424 | 0.351 | 0.406 | 0.295 | 0.324 | 0.336 | 0.401 | 1.000 | |
INT.2 | 0.420 | 0.440 | 0.381 | 0.425 | 0.334 | 0.429 | 0.322 | 0.318 | 0.323 | 0.402 | 0.868 | 1.000 |
INT.3 | 0.405 | 0.442 | 0.334 | 0.421 | 0.329 | 0.357 | 0.287 | 0.375 | 0.376 | 0.450 | 0.755 | 0.772 |
INT.4 | 0.366 | 0.428 | 0.360 | 0.432 | 0.307 | 0.424 | 0.308 | 0.374 | 0.339 | 0.433 | 0.794 | 0.773 |
INT.5 | 0.388 | 0.431 | 0.355 | 0.441 | 0.342 | 0.428 | 0.269 | 0.326 | 0.358 | 0.434 | 0.758 | 0.737 |
INT.6 | 0.405 | 0.401 | 0.403 | 0.428 | 0.331 | 0.405 | 0.382 | 0.346 | 0.443 | 0.471 | 0.606 | 0.648 |
INT.3 | INT.4 | INT.5 | INT.6 | |
INT.3 | 1.000 | |||
INT.4 | 0.766 | 1.000 | ||
INT.5 | 0.745 | 0.821 | 1.000 | |
INT.6 | 0.657 | 0.653 | 0.678 | 1.000 |
The authors declare no conflicts of interest.
[1] | Qayyum MU, Sherani AMK, Khan M, et al. (2023) Revolutionizing healthcare: the transformative impact of artificial intelligence in medicine. B Inform 1: 71-83. |
[2] |
Gill AY, Saeed A, Rasool S, et al. (2023) Revolutionizing healthcare: how machine learning is transforming patient diagnoses-a comprehensive review of AI's impact on medical diagnosis. J World Sci 2: 1638-1652. https://doi.org/10.58344/jws.v2i10.449 ![]() |
[3] |
Panayides AS, Amini A, Filipovic ND, et al. (2020) AI in medical imaging informatics: current challenges and future directions. IEEE J Biomed Health Inform 24: 1837-1857. https://doi.org/10.1109/JBHI.2020.2991043 ![]() |
[4] |
Ngiam KY, Khor IW (2019) Big data and machine learning algorithms for health-care delivery. Lancet Oncol 20: e262-e273. https://doi.org/10.1016/S1470-2045(19)30149-4 ![]() |
[5] |
Grant MJ, Booth A (2009) A typology of reviews: an analysis of 14 review types and associated methodologies. Health Info Libr J 26: 91-108. https://doi.org/10.1111/j.1471-1842.2009.00848.x ![]() |
[6] |
Jayaraman PP, Forkan ARM, Morshed A, et al. (2020) Healthcare 4.0: a review of frontiers in digital health. Wires Data Min Knowl 10: e1350. https://doi.org/10.1002/widm.1350 ![]() |
[7] | Ali M (2023) A comprehensive review of AI's impact on healthcare: revolutionizing diagnostics and patient care. Bullet Jurnal Multidisiplin Ilmu 2: 1163-1173. |
[8] | Najjar R (2024) Digital frontiers in healthcare: integrating mHealth, AI, and radiology for future medical diagnostics, In: Heston, T.F., Doarn, C.E. Editors. A Comprehensive Overview of Telemedicine. London: IntechOpen Limited. https://doi.org/10.5772/intechopen.114142 |
[9] |
Feretzakis G, Juliebø-Jones P, Tsaturyan A, et al. (2024) Emerging trends in AI and radiomics for bladder, kidney, and prostate cancer: a critical review. Cancers 16: 810. https://doi.org/10.3390/cancers16040810 ![]() |
[10] |
Dlamini Z, Francies FZ, Hull R, et al. (2020) Artificial intelligence (AI) and big data in cancer and precision oncology. Comput Struct Biotechnol J 18: 2300-2311. https://doi.org/10.1016/j.csbj.2020.08.019 ![]() |
[11] |
Cucchiara F, Petrini I, Romei C, et al. (2021) Combining liquid biopsy and radiomics for personalized treatment of lung cancer patients. State of the art and new perspectives. Pharmacol Res 169: 105643. https://doi.org/10.1016/j.phrs.2021.105643 ![]() |
[12] |
Ginghina O, Hudita A, Zamfir M, et al. (2022) Liquid biopsy and artificial intelligence as tools to detect signatures of colorectal malignancies: a modern approach in patient's stratification. Front Oncol 12: 856575. https://doi.org/10.3389/fonc.2022.856575 ![]() |
[13] |
Stenzinger A, Alber M, Allgäuer M, et al. (2022) Artificial intelligence and pathology: from principles to practice and future applications in histomorphology and molecular profiling. Semin Cancer Biol 84: 129-143. https://doi.org/10.1016/j.semcancer.2021.02.011 ![]() |
[14] |
Aminizadeh S, Heidari A, Dehghan M, et al. (2024) Opportunities and challenges of artificial intelligence and distributed systems to improve the quality of healthcare service. Artif Intell Med 149: 102779. https://doi.org/10.1016/j.artmed.2024.102779 ![]() |
[15] |
Pasrija P, Jha P, Upadhyaya P, et al. (2022) Machine learning and artificial intelligence: a paradigm shift in big data-driven drug design and discovery. Curr Top Med Chem 22: 1692-1727. https://doi.org/10.2174/1568026622666220701091339 ![]() |
[16] |
Akinola S, Telukdarie A (2023) Sustainable digital transformation in healthcare: advancing a digital vascular health innovation solution. Sustainability 15: 10417. https://doi.org/10.3390/su151310417 ![]() |
[17] |
Hassija V, Chamola V, Mahapatra A, et al. (2024) Interpreting black-box models: a review on explainable artificial intelligence. Cogn Comput 16: 45-74. https://doi.org/10.1007/s12559-023-10179-8 ![]() |
[18] | Schwartz R, Vassilev A, Greene K, et al. (2022) Towards a standard for identifying and managing bias in artificial intelligence. NIST Special Publication. https://doi.org/10.6028/NIST.SP.1270 |
[19] |
Rajkomar A, Hardt M, Howell MD, et al. (2018) Ensuring fairness in machine learning to advance health equity. Ann Intern Med 169: 866-872. https://doi.org/10.7326/M18-1990 ![]() |
[20] |
Ueda D, Kakinuma T, Fujita S, et al. (2024) Fairness of artificial intelligence in healthcare: review and recommendations. Jpn J Radiol 42: 3-15. https://doi.org/10.1007/s11604-023-01474-3 ![]() |
[21] |
Aminizadeh S, Heidari A, Toumaj S, et al. (2023) The applications of machine learning techniques in medical data processing based on distributed computing and the internet of things. Comput Methods Programs Biomed 241: 107745. https://doi.org/10.1016/j.cmpb.2023.107745 ![]() |
[22] |
Sahu M, Gupta R, Ambasta RK, et al. (2022) Artificial intelligence and machine learning in precision medicine: a paradigm shift in big data analysis. Prog Mol Biol Transl Sci 190: 57-100. https://doi.org/10.1016.pmbts.2022.03.002 ![]() |
[23] |
Schork NJ (2019) Artificial intelligence and personalized medicine, In: Von Hoff, D., Han, H. Editors. Precision medicine in Cancer therapy. 1 Ed., Switzerland: Springer, Cham, 265-283. https://doi.org/10.1007/978-3-030-16391-4_11 ![]() |
[24] |
Wang H, Xiong R, Lai L (2023) Rational drug design targeting intrinsically disordered proteins. Wires Comput Mol Sci 13: p.e1685. https://doi.org/10.1002/wcms.1685 ![]() |
[25] |
Pun FW, Ozerov IV, Zhavoronkov A (2023) AI-powered therapeutic target discovery. Trends Pharmacol Sci 44: 561-572. https://doi.org/10.1016/j.tips.2023.06.010 ![]() |
[26] |
Chen Y, Esmaeilzadeh P (2024) Generative AI in medical practice: in-depth exploration of privacy and security challenges. J Med Internet Res 26: e53008. https://doi.org/10.2196/53008 ![]() |
[27] |
Lainjo B (2024) A meta-study on optimizing healthcare performance with artificial intelligence and machine learning. J Autonom Intell 7: 1535. https://doi.org/10.32629/jai.v7i5.1535 ![]() |
[28] |
Tao WJ, Zeng Z, Dang HX, et al. (2020) Towards universal health coverage: lessons from 10 years of healthcare reform in China. BMJ Glob Health 5: e002086. https://doi.org/10.1136/bmjgh-2019-002086 ![]() |
[29] |
Haleem A, Javaid M, Singh RP, et al. (2022) Medical 4.0 technologies for healthcare: features, capabilities, and applications. Int Things Cyber Physic Syst 2: 12-30. https://doi.org/10.1016/j.iotcps.2022.04.001 ![]() |
[30] |
Wamba-Taguimdje SL, Wamba SF, Kamdjoug JRK, et al. (2020) Influence of artificial intelligence (AI) on firm performance: the business value of AI-based transformation projects. Bus Process Manag J 26: 1893-1924. https://doi.org/10.1108/BPMJ-10-2019-0411 ![]() |
[31] | Chowdhury S, Dey P, Joel-Edgar S, et al. (2023) Unlocking the value of artificial intelligence in human resource management through AI capability framework. Hum Resour Manag Rev 33: 100899. https://doi.org/10.1016/j.hrmr.2022.100899 |
[32] |
Akindote OJ, Adegbite AO, Omotosho A, et al. (2024) Evaluating the effectiveness of it project management in healthcare digitalization: a review. Int Med Sci Res J 4: 37-50. https://doi.org/10.51594/imsrj.v4i1.698 ![]() |
[33] | Rodrigues JJPC (2009) Health information systems: concepts, methodologies, tools, and applications: concepts, methodologies, tools, and applications. Igi Global. https://doi.org/10.4018/978-1-60566-988-5 |
[34] | World Health Organization (2021) Ethics and governance of artificial intelligence for health: WHO guidance. Available from: https://www.who.int/publications-detail-redirect/9789240029200 |
[35] |
Carter SM, Rogers W, Win KT, et al. (2020) The ethical, legal and social implications of using artificial intelligence systems in breast cancer care. Breast 49: 25-32. https://doi.org/10.1016/j.breast.2019.10.001 ![]() |
[36] |
Smith H (2021) Clinical AI: opacity, accountability, responsibility and liability. AI Soc 36: 535-545. https://doi.org/10.1007/s00146-020-01019-6 ![]() |
[37] |
Reddy S, Allan S, Coghlan S, et al. (2020) A governance model for the application of AI in health care. J Am Med Inform Assoc 27: 491-497. https://doi.org/10.1093/jamia/ocz192 ![]() |
[38] |
Williamson SM, Prybutok V (2024) Balancing privacy and progress: a review of privacy challenges, systemic oversight, and patient perceptions in AI-driven healthcare. Appl Sci 14: 675. https://doi.org/10.3390/app14020675 ![]() |
[39] |
Ali A, Al-Rimy BAS, Tin TT, et al. (2023) Empowering precision medicine: unlocking revolutionary insights through blockchain-enabled federated learning and electronic medical records. Sensors 23: 7476. https://doi.org/10.3390/s23177476 ![]() |
[40] |
Floridi L, Cowls J, Beltrametti M, et al. (2018) AI4People—an ethical framework for a good AI society: opportunities, risks, principles, and recommendations. Minds Mach 28: 689-707. https://doi.org/10.1007/s11023-018-9482-5 ![]() |
[41] |
Bhidayasiri R, Goetz CG (2024) Embracing the promise of artificial intelligence to improve patient care in movement disorders, In: Bhidayasiri, R., Maetzler, W. Editors. Handbook of Digital Technologies in Movement Disorders. 1 Ed., Massachusetts: Academic Press, 11-23. https://doi.org/10.1016/B978-0-323-99494-1.00015-0 ![]() |
[42] | Kalmady SV, Paul AK, Narayanaswamy JC, et al. (2022) Prediction of obsessive-compulsive disorder: importance of neurobiology-aided feature design and cross-diagnosis transfer learning. Biol Psychiatry Cogn Neurosci Neuroimaging 7: 735-746. https://doi.org/10.1016/j.bpsc.2021.12.003 |
[43] |
Harerimana G, Jang B, Kim JW, et al. (2018) Health big data analytics: a technology survey. IEEE Access 6: 65661-65678. https://doi.org/10.1109/ACCESS.2018.2878254 ![]() |
[44] |
Morley J, Floridi L (2020) An ethically mindful approach to AI for health care. Lancet 395: 254-255. https://doi.org/10.1016/S0140-6736(19)32975-7 ![]() |
[45] |
Morley J, Floridi L (2021) How to design a governable digital health ecosystem, In: Cowls, J., Morley, J. Editors. The 2020 Yearbook of the Digital Ethics Lab. 1 Ed., Netherland: Springer, Cham, 69-88. https://doi.org/10.1007/978-3-030-80083-3_8 ![]() |
[46] |
Cleret de Langavant L, Bayen E, Yaffe K (2018) Unsupervised machine learning to identify high likelihood of dementia in population-based surveys: development and validation study. J Med Internet Res 20: e10493. https://doi.org/10.2196/10493 ![]() |
[47] |
Moscoso A, Silva-Rodríguez J, Aldrey JM, et al. (2019) Prediction of Alzheimer's disease dementia with MRI beyond the short-term: implications for the design of predictive models. Neuroimage Clin 23: 101837. https://doi.org/10.1016/j.nicl.2019.101837 ![]() |
[48] |
López-Martínez F, Núñez-Valdez ER, Gomez JL, et al. (2019) A neural network approach to predict early neonatal sepsis. Comput Electr Eng 76: 379-388. https://doi.org/10.1016/j.compeleceng.2019.04.015 ![]() |
[49] | Morley J, Machado C, Burr C, et al. (2019) The debate on the ethics of AI in health care: a reconstruction and critical review. Available at SSRN 3486518. https://doi.org/10.2139/ssrn.3486518 |
[50] | Morley J, Machado CCV, Burr C, et al. (2021) The ethics of AI in health care: a mapping, In: Floridi, L., Editor. Ethics, Governance, and Policies in Artificial Intelligence. 1 Ed., Netherland: Springer, Cham, 313-346. https://doi.org/10.1007/978-3-030-81907-1_18 |
[51] |
Karimian G, Petelos E, Evers SMAA (2022) The ethical issues of the application of artificial intelligence in healthcare: a systematic scoping review. AI Ethics 2: 539-551. https://doi.org/10.1007/s43681-021-00131-7 ![]() |
1. | Giuseppe Timpanaro, Paolo Guarnaccia, Silvia Zingale, Vera Teresa Foti, Alessandro Scuderi, The sustainability role in the purchasing choice of agri-food products in the United Arab Emirates and Italy, 2022, 7, 2471-2086, 212, 10.3934/agrfood.2022014 | |
2. | Costa Synodinos, Gustavo Hermínio Salati Marcondes de Moraes, Nágela Bianca do Prado, Green food purchasing behaviour: a multi-method approach of Generation Y in a developing country, 2023, 0007-070X, 10.1108/BFJ-09-2022-0769 | |
3. | Adhi Prakosa, Leny Wulandari, Arif Sudaryana, Consumer Intention to Purchase Organic Ice Cream: How Health Awareness and Product Knowledge Support SDG 3 on Good Health and Well-Being, 2024, 4, 2965-730X, e02143, 10.47172/2965-730X.SDGsReview.v4.n03.pe02143 | |
4. | D D Aisyah, D Cahyasita, A D Nugroho, A. Loch, R.C. Joshi, A. Munawar, M.T. Multazam, Willingness to Pay and Barriers in Gen Z’s Pro-Environmental Product Consumption, 2023, 69, 2117-4458, 04024, 10.1051/bioconf/20236904024 |
Variable | Item | Measurement Item | Source |
Intention to re-consume (INT) | INT1 | I intend to repurchase organic foods. | [17,35] |
INT2 | I intend to eat more organically processed foods in the future. | ||
INT3 | I would like to repurchase organic food because it is the best option. | ||
INT4 | I would like to reintroduce organic food, particularly environmentally friendly products. | ||
INT5 | I would like to re-consume organic food, preferably healthy and nutritious food. | ||
INT6 | For the welfare of farmers, I would like to re-consume organic food. | ||
Attitude (ATT) | ATT1 | Buying organic food is a good idea. | [17,35,62] |
ATT2 | Buying organic food is a wise decision. | ||
ATT3 | Buying organic food is important. | ||
ATT4 | Consuming organic food is advantageous. | ||
ATT5 | Consuming organic food is valuable. | ||
ATT6 | Consuming organic food is interesting. | ||
ATT7 | I like organic food because it is processed without the use of chemicals. | ||
ATT8 | I like organic food because it is more nutritious. | ||
ATT9 | I like organic food because it is healthier to consume. | ||
ATT10 | I like organic food because it is environmentally friendly. | ||
ATT11 | I like organic food because it allows me to contribute to the well-being of farmers. | ||
Subjective norms (SN) | SN1 | Most of the people important to me think that I should eat organic food. | [62,63,64] |
SN2 | My family believes that I should consume organic food. | ||
SN3 | People whose opinions I value believe that I should consume organic food. | ||
SN4 | My friend's positive feedback encourages me to consume organic food. | ||
SN5 | My loved ones expect me to consume organic food. | ||
SN6 | Because of external pressure (social, environmental, network, etc.), I choose organic food. | ||
Perceived Behavior Control (PBC) | PBC1 | It is entirely up to me whether or not I buy organic food. | [17,62,63,64] |
PBC2 | Consumers believe they can buy organic food. | ||
PBC3 | Consumers believe they have the resources, time, and willingness to buy organic food. | ||
PBC4 | Customers believe that they have control over whether or not they consume organic food. | ||
PBC5 | Consumers believe that there will be numerous opportunities for them to consume organic food. | ||
PBC6 | Consumers find it simple to consume organic food. | ||
PBC7 | Consumers believe they can consume organic food if they desire to do so. | ||
Sensory Appeal Perceived (SEN_APP) | SEN_APP1 | In my opinion, organic food is more attractive. | [18,40,62,65] |
SEN_APP2 | In my opinion, organic food appears to be tasty. | ||
SEN_APP3 | In my opinion, organic food looks fresher. (especial for rice, in question is natural / no over-processing after harvest) | ||
SEN_APP4 | In my opinion, organic food has a pleasant texture. | ||
Warm Glow (WG) | WG1 | Buying organic food gives me a pleasant feeling. | [17,21,51,66,67] |
WG2 | Consuming organic food makes me happy because it makes me feel that I have done something good. | ||
WG3 | I am happy when I can make choices to consume organic food, for myself and my family. | ||
WG4 | Every time I consume organic food, I feel good about myself because I have protected the environment. | ||
WG5 | I am satisfied because I have contributed to the efficiency of natural resources by consuming organic food. | ||
WG6 | Consuming organic food makes me feel good because it contributes to the preservation of the quality of the Earth (soil, water, etc.). | ||
WG7 | Purchasing organic food makes me happy because I am contributing to the well-being of farmers. | ||
WG8 | I am pleased that I have contributed to the growth of the local economy by purchasing organic food. | ||
Egoistic motive (E) | E1 | I eat organic food to better maintain my health. | [1,23,45,68] |
E2 | Organic food is the food that my family consumes for the sake of our family's health. | ||
E3 | I eat organic food to provide my body with more nutrition. | ||
E4 | I choose organic foods to obtain products that are safer to consume. |
Demographic Variable | N | % | |
Age (years old) | 17–25 | 179 | 53.12 |
26–35 | 97 | 28.78 | |
36–45 | 25 | 7.42 | |
56–55 | 31 | 9.20 | |
> 56 | 5 | 1.48 | |
Gender | Male | 95 | 28.19 |
Female | 242 | 71.81 | |
Education qualification | Higher secondary | 52 | 15.43 |
Graduate | 212 | 62.91 | |
Postgraduate | 71 | 21.07 | |
Any other | 2 | 0.59 | |
Marital status | Single | 210 | 62.31 |
Married | 127 | 37.69 | |
Employment status | Employed | 171 | 50.74 |
Unemployed | 12 | 3.56 | |
Retired | 2 | 0.59 | |
Homemaker | 37 | 10.98 | |
Student | 115 | 34.12 |
CR | AVE | 1 | 2 | 3 | 4 | 5 | 6 | 7 | |
1.Attitude | 0.953 | 0.649 | |||||||
2.Egoistic motive | 0.943 | 0.806 | 0.808 | ||||||
3.Intention to re-consume | 0.955 | 0.781 | 0.589 | 0.536 | |||||
4.PBC | 0.918 | 0.650 | 0.531 | 0.485 | 0.605 | ||||
5.Sesory appeal | 0.905 | 0.705 | 0.524 | 0.365 | 0.541 | 0.485 | |||
6.Subjecctive norms | 0.931 | 0.731 | 0.473 | 0.345 | 0.394 | 0.401 | 0.476 | ||
7.Warm Glow | 0.959 | 0.747 | 0.768 | 0.689 | 0.547 | 0.494 | 0.452 | 0.394 |
Hypothesis | Path | Std Beta | t-value | Result | f2 |
H1 | ATT → INT | 0.289 | 3.518* | Supported | 0.097 |
SN → ATT | 0.112 | 3.271* | Supported | 0.033 | |
SN → INT | 0.042 | 0.816ns | Rejected | 0.002 | |
PBC → INT | 0.313 | 4.738* | Supported | 0.125 | |
H2 | SEN_APP → ATT | 0.141 | 2.763* | Supported | 0.050 |
SEN_APP → INT | 0.204 | 3.045* | Supported | 0.052 | |
H3 | E → ATT | 0.457 | 8.594* | Supported | 0.405 |
WG → ATT | 0.333 | 7.542* | Supported | 0.197 | |
Note: *p < 0.05. |
Items | Outer Loading | Cross Loading | ||||||||||||
ATT | E | INT | PBC | SEN_APP | SN | WG | ATT | E | INT | PBC | SEN_APP | SN | WG | |
SEN_APP.1 | 0.812 | 0.342 | 0.219 | 0.352 | 0.328 | 0.812 | 0.346 | 0.282 | ||||||
SEN_APP.2 | 0.842 | 0.356 | 0.247 | 0.390 | 0.302 | 0.842 | 0.387 | 0.296 | ||||||
SEN_APP.3 | 0.859 | 0.469 | 0.312 | 0.411 | 0.393 | 0.859 | 0.317 | 0.395 | ||||||
SEN_APP.4 | 0.845 | 0.418 | 0.314 | 0.490 | 0.407 | 0.845 | 0.362 | 0.400 | ||||||
WG.1 | 0.839 | 0.657 | 0.605 | 0.429 | 0.394 | 0.351 | 0.357 | 0.839 | ||||||
WG.2 | 0.837 | 0.590 | 0.511 | 0.468 | 0.423 | 0.353 | 0.311 | 0.837 | ||||||
WG.3 | 0.860 | 0.621 | 0.605 | 0.448 | 0.387 | 0.348 | 0.336 | 0.860 | ||||||
WG.4 | 0.902 | 0.648 | 0.579 | 0.468 | 0.410 | 0.381 | 0.314 | 0.902 | ||||||
WG.5 | 0.896 | 0.646 | 0.554 | 0.458 | 0.388 | 0.354 | 0.315 | 0.896 | ||||||
WG.6 | 0.879 | 0.644 | 0.618 | 0.425 | 0.383 | 0.335 | 0.271 | 0.879 | ||||||
WG.7 | 0.854 | 0.600 | 0.474 | 0.473 | 0.386 | 0.393 | 0.334 | 0.854 | ||||||
WG.8 | 0.847 | 0.625 | 0.512 | 0.422 | 0.363 | 0.359 | 0.315 | 0.847 | ||||||
SN.1 | 0.858 | 0.327 | 0.308 | 0.314 | 0.333 | 0.340 | 0.858 | 0.266 | ||||||
SN.2 | 0.889 | 0.370 | 0.291 | 0.342 | 0.356 | 0.372 | 0.889 | 0.304 | ||||||
SN.3 | 0.868 | 0.429 | 0.294 | 0.324 | 0.299 | 0.351 | 0.868 | 0.377 | ||||||
SN.4 | 0.781 | 0.326 | 0.156 | 0.234 | 0.245 | 0.356 | 0.781 | 0.275 | ||||||
SN.5 | 0.875 | 0.415 | 0.298 | 0.348 | 0.314 | 0.374 | 0.875 | 0.341 | ||||||
PBC.2 | 0.807 | 0.347 | 0.386 | 0.454 | 0.807 | 0.323 | 0.302 | 0.353 | ||||||
PBC.3 | 0.865 | 0.434 | 0.410 | 0.491 | 0.865 | 0.342 | 0.408 | 0.359 | ||||||
PBC.4 | 0.746 | 0.337 | 0.296 | 0.415 | 0.746 | 0.321 | 0.192 | 0.359 | ||||||
PBC.5 | 0.824 | 0.449 | 0.347 | 0.486 | 0.824 | 0.397 | 0.296 | 0.377 | ||||||
PBC.6 | 0.766 | 0.369 | 0.331 | 0.376 | 0.766 | 0.349 | 0.328 | 0.376 | ||||||
PBC.7 | 0.824 | 0.421 | 0.350 | 0.462 | 0.824 | 0.350 | 0.226 | 0.375 | ||||||
E.1 | 0.899 | 0.682 | 0.899 | 0.420 | 0.357 | 0.281 | 0.296 | 0.583 | ||||||
E.2 | 0.888 | 0.655 | 0.888 | 0.449 | 0.431 | 0.285 | 0.316 | 0.556 | ||||||
E.3 | 0.907 | 0.702 | 0.907 | 0.463 | 0.402 | 0.299 | 0.301 | 0.584 | ||||||
E.4 | 0.898 | 0.670 | 0.898 | 0.460 | 0.390 | 0.321 | 0.234 | 0.597 | ||||||
INT.1 | 0.900 | 0.462 | 0.481 | 0.900 | 0.504 | 0.407 | 0.318 | 0.448 | ||||||
INT.2 | 0.904 | 0.461 | 0.435 | 0.904 | 0.504 | 0.409 | 0.322 | 0.427 | ||||||
INT.3 | 0.886 | 0.507 | 0.466 | 0.886 | 0.476 | 0.448 | 0.335 | 0.469 | ||||||
INT.4 | 0.908 | 0.526 | 0.448 | 0.908 | 0.482 | 0.437 | 0.316 | 0.466 | ||||||
INT.5 | 0.896 | 0.517 | 0.462 | 0.896 | 0.495 | 0.420 | 0.315 | 0.459 | ||||||
INT.6 | 0.803 | 0.481 | 0.353 | 0.803 | 0.492 | 0.494 | 0.345 | 0.475 | ||||||
ATT.1 | 0.847 | 0.847 | 0.610 | 0.439 | 0.413 | 0.367 | 0.312 | 0.613 | ||||||
ATT.10 | 0.857 | 0.857 | 0.647 | 0.492 | 0.383 | 0.384 | 0.356 | 0.618 | ||||||
ATT.11 | 0.778 | 0.778 | 0.515 | 0.514 | 0.417 | 0.441 | 0.408 | 0.633 | ||||||
ATT.2 | 0.843 | 0.843 | 0.629 | 0.414 | 0.420 | 0.357 | 0.325 | 0.615 | ||||||
ATT.3 | 0.750 | 0.750 | 0.565 | 0.413 | 0.384 | 0.423 | 0.424 | 0.564 | ||||||
ATT.4 | 0.803 | 0.803 | 0.615 | 0.429 | 0.462 | 0.370 | 0.441 | 0.579 | ||||||
ATT.5 | 0.858 | 0.858 | 0.660 | 0.475 | 0.373 | 0.355 | 0.276 | 0.585 | ||||||
ATT.6 | 0.723 | 0.723 | 0.498 | 0.375 | 0.399 | 0.404 | 0.351 | 0.556 | ||||||
ATT.7 | 0.758 | 0.758 | 0.605 | 0.422 | 0.337 | 0.345 | 0.306 | 0.540 | ||||||
ATT.8 | 0.766 | 0.766 | 0.621 | 0.440 | 0.317 | 0.414 | 0.385 | 0.554 | ||||||
ATT.9 | 0.865 | 0.865 | 0.705 | 0.516 | 0.429 | 0.371 | 0.324 | 0.594 | ||||||
Description: ATT: Attitude; E: Egoistic; INT: Intention to re-consume; PBC: Perceived Behavioral Control; SEN_APP: Sensory Appeal Perceived; SN: Subjective norm; WG: Warm Glow. |
Mean | Standard Deviation | Matrix Correlations | ||||||||||
WG.1 | WG.2 | WG.3 | WG.4 | WG.5 | WG.6 | WG.7 | WG.8 | E.1 | E.2 | |||
WG.1 | 4.228 | 0.738 | 1.000 | |||||||||
WG.2 | 4.267 | 0.702 | 0.771 | 1.000 | ||||||||
WG.3 | 4.270 | 0.744 | 0.774 | 0.726 | 1.000 | |||||||
WG.4 | 4.285 | 0.740 | 0.690 | 0.693 | 0.755 | 1.000 | ||||||
WG.5 | 4.279 | 0.778 | 0.654 | 0.685 | 0.721 | 0.852 | 1.000 | |||||
WG.6 | 4.356 | 0.713 | 0.652 | 0.658 | 0.703 | 0.814 | 0.833 | 1.000 | ||||
WG.7 | 4.246 | 0.764 | 0.632 | 0.652 | 0.630 | 0.716 | 0.724 | 0.700 | 1.000 | |||
WG.8 | 4.208 | 0.792 | 0.620 | 0.621 | 0.635 | 0.704 | 0.715 | 0.705 | 0.867 | 1.000 | ||
E.1 | 4.513 | 0.702 | 0.564 | 0.450 | 0.570 | 0.535 | 0.499 | 0.571 | 0.401 | 0.432 | 1.000 | |
E.2 | 4.288 | 0.806 | 0.513 | 0.441 | 0.524 | 0.494 | 0.468 | 0.508 | 0.425 | 0.464 | 0.735 | 1.000 |
E.3 | 4.365 | 0.774 | 0.529 | 0.465 | 0.535 | 0.523 | 0.516 | 0.544 | 0.435 | 0.486 | 0.730 | 0.758 |
E.4 | 4.501 | 0.698 | 0.567 | 0.478 | 0.545 | 0.527 | 0.508 | 0.595 | 0.442 | 0.456 | 0.764 | 0.703 |
E.5 | 3.202 | 1.211 | 0.307 | 0.289 | 0.289 | 0.283 | 0.303 | 0.226 | 0.264 | 0.318 | 0.283 | 0.302 |
E.6 | 3.685 | 1.117 | 0.454 | 0.421 | 0.431 | 0.421 | 0.395 | 0.398 | 0.418 | 0.466 | 0.433 | 0.413 |
E.7 | 3.644 | 1.152 | 0.448 | 0.426 | 0.382 | 0.401 | 0.392 | 0.382 | 0.420 | 0.393 | 0.321 | 0.347 |
ATT.1 | 4.395 | 0.659 | 0.608 | 0.510 | 0.515 | 0.554 | 0.543 | 0.560 | 0.467 | 0.474 | 0.569 | 0.529 |
ATT.2 | 4.344 | 0.693 | 0.577 | 0.512 | 0.522 | 0.555 | 0.565 | 0.538 | 0.473 | 0.507 | 0.569 | 0.566 |
ATT.3 | 4.145 | 0.847 | 0.479 | 0.469 | 0.517 | 0.506 | 0.492 | 0.465 | 0.458 | 0.512 | 0.498 | 0.560 |
ATT.4 | 4.193 | 0.771 | 0.522 | 0.470 | 0.499 | 0.527 | 0.514 | 0.485 | 0.468 | 0.512 | 0.502 | 0.588 |
ATT.5 | 4.469 | 0.640 | 0.534 | 0.494 | 0.476 | 0.526 | 0.519 | 0.571 | 0.450 | 0.470 | 0.587 | 0.578 |
ATT.6 | 4.139 | 0.834 | 0.527 | 0.469 | 0.489 | 0.464 | 0.475 | 0.450 | 0.491 | 0.482 | 0.460 | 0.439 |
ATT.7 | 4.475 | 0.739 | 0.498 | 0.465 | 0.469 | 0.474 | 0.477 | 0.479 | 0.445 | 0.425 | 0.582 | 0.503 |
ATT.8 | 4.329 | 0.783 | 0.471 | 0.423 | 0.479 | 0.489 | 0.512 | 0.508 | 0.450 | 0.493 | 0.556 | 0.489 |
ATT.9 | 4.528 | 0.631 | 0.551 | 0.439 | 0.518 | 0.523 | 0.529 | 0.578 | 0.469 | 0.487 | 0.648 | 0.553 |
ATT.10 | 4.481 | 0.654 | 0.523 | 0.470 | 0.520 | 0.600 | 0.582 | 0.588 | 0.482 | 0.500 | 0.619 | 0.548 |
ATT.11 | 4.362 | 0.739 | 0.534 | 0.518 | 0.497 | 0.517 | 0.506 | 0.471 | 0.663 | 0.678 | 0.437 | 0.448 |
SN.1 | 3.706 | 0.953 | 0.281 | 0.237 | 0.292 | 0.220 | 0.247 | 0.171 | 0.193 | 0.199 | 0.301 | 0.303 |
SN.2 | 3.712 | 0.976 | 0.297 | 0.273 | 0.279 | 0.245 | 0.262 | 0.211 | 0.270 | 0.269 | 0.268 | 0.294 |
SN.3 | 3.742 | 0.972 | 0.384 | 0.319 | 0.363 | 0.333 | 0.327 | 0.282 | 0.325 | 0.274 | 0.268 | 0.265 |
SN.4 | 3.724 | 0.992 | 0.253 | 0.230 | 0.218 | 0.232 | 0.215 | 0.189 | 0.313 | 0.258 | 0.157 | 0.159 |
SN.5 | 3.733 | 0.990 | 0.299 | 0.261 | 0.271 | 0.298 | 0.282 | 0.286 | 0.323 | 0.336 | 0.261 | 0.312 |
SN.6 | 3.932 | 0.974 | 0.381 | 0.244 | 0.345 | 0.295 | 0.303 | 0.279 | 0.334 | 0.307 | 0.307 | 0.275 |
SN.7 | 2.861 | 1.315 | 0.131 | 0.108 | 0.087 | 0.074 | 0.108 | 0.043 | 0.132 | 0.159 | 0.058 | 0.074 |
PBC.1 | 4.318 | 0.733 | 0.310 | 0.331 | 0.327 | 0.271 | 0.298 | 0.306 | 0.274 | 0.244 | 0.289 | 0.252 |
PBC.2 | 4.181 | 0.680 | 0.314 | 0.309 | 0.267 | 0.304 | 0.286 | 0.314 | 0.325 | 0.321 | 0.302 | 0.349 |
PBC.3 | 4.160 | 0.713 | 0.325 | 0.336 | 0.293 | 0.330 | 0.326 | 0.273 | 0.309 | 0.293 | 0.322 | 0.431 |
PBC.4 | 4.228 | 0.726 | 0.329 | 0.335 | 0.309 | 0.277 | 0.303 | 0.302 | 0.321 | 0.310 | 0.271 | 0.258 |
PBC.5 | 4.240 | 0.679 | 0.323 | 0.388 | 0.312 | 0.360 | 0.322 | 0.307 | 0.321 | 0.277 | 0.270 | 0.356 |
PBC.6 | 4.039 | 0.841 | 0.287 | 0.349 | 0.363 | 0.349 | 0.337 | 0.323 | 0.295 | 0.300 | 0.243 | 0.364 |
PBC.7 | 4.291 | 0.657 | 0.328 | 0.334 | 0.343 | 0.367 | 0.312 | 0.343 | 0.301 | 0.266 | 0.313 | 0.324 |
SEN_APP.1 | 4.104 | 0.761 | 0.243 | 0.265 | 0.228 | 0.248 | 0.202 | 0.183 | 0.313 | 0.274 | 0.167 | 0.203 |
SEN_APP.2 | 4.053 | 0.745 | 0.242 | 0.251 | 0.269 | 0.263 | 0.266 | 0.249 | 0.248 | 0.258 | 0.203 | 0.212 |
SEN_APP.3 | 4.276 | 0.688 | 0.344 | 0.302 | 0.347 | 0.370 | 0.350 | 0.326 | 0.362 | 0.331 | 0.278 | 0.253 |
SEN_APP.4 | 4.172 | 0.689 | 0.331 | 0.353 | 0.309 | 0.375 | 0.348 | 0.340 | 0.382 | 0.331 | 0.277 | 0.279 |
INT.1 | 4.374 | 0.594 | 0.387 | 0.423 | 0.416 | 0.399 | 0.397 | 0.365 | 0.379 | 0.333 | 0.408 | 0.432 |
INT.2 | 4.374 | 0.604 | 0.354 | 0.416 | 0.376 | 0.399 | 0.359 | 0.359 | 0.379 | 0.315 | 0.359 | 0.388 |
INT.3 | 4.276 | 0.670 | 0.413 | 0.436 | 0.398 | 0.410 | 0.416 | 0.366 | 0.424 | 0.384 | 0.374 | 0.457 |
INT.4 | 4.350 | 0.603 | 0.380 | 0.438 | 0.411 | 0.421 | 0.431 | 0.393 | 0.392 | 0.357 | 0.395 | 0.366 |
INT.5 | 4.392 | 0.597 | 0.383 | 0.409 | 0.416 | 0.405 | 0.416 | 0.404 | 0.400 | 0.342 | 0.412 | 0.400 |
INT.6 | 4.326 | 0.658 | 0.354 | 0.357 | 0.353 | 0.442 | 0.402 | 0.359 | 0.525 | 0.496 | 0.279 | 0.337 |
E.3 | E.4 | E.5 | E.6 | E.7 | ATT.1 | ATT.2 | ATT.3 | ATT.4 | ATT.5 | ATT.6 | ATT.7 | |
E.3 | 1.000 | |||||||||||
E.4 | 0.759 | 1.000 | ||||||||||
E.5 | 0.320 | 0.221 | 1.000 | |||||||||
E.6 | 0.452 | 0.392 | 0.703 | 1.000 | ||||||||
E.7 | 0.362 | 0.303 | 0.660 | 0.741 | 1.000 | |||||||
ATT.1 | 0.537 | 0.556 | 0.268 | 0.390 | 0.376 | 1.000 | ||||||
ATT.2 | 0.578 | 0.544 | 0.320 | 0.415 | 0.425 | 0.858 | 1.000 | |||||
ATT.3 | 0.525 | 0.449 | 0.414 | 0.475 | 0.384 | 0.625 | 0.657 | 1.000 | ||||
ATT.4 | 0.612 | 0.504 | 0.359 | 0.432 | 0.425 | 0.650 | 0.697 | 0.656 | 1.000 | |||
ATT.5 | 0.601 | 0.603 | 0.192 | 0.331 | 0.307 | 0.757 | 0.720 | 0.597 | 0.677 | 1.000 | ||
ATT.6 | 0.445 | 0.446 | 0.372 | 0.429 | 0.438 | 0.591 | 0.579 | 0.534 | 0.618 | 0.567 | 1.000 | |
ATT.7 | 0.532 | 0.557 | 0.148 | 0.289 | 0.279 | 0.572 | 0.544 | 0.421 | 0.521 | 0.602 | 0.461 | 1.000 |
ATT.8 | 0.639 | 0.539 | 0.337 | 0.454 | 0.400 | 0.530 | 0.529 | 0.514 | 0.558 | 0.610 | 0.480 | 0.607 |
ATT.9 | 0.650 | 0.679 | 0.191 | 0.329 | 0.271 | 0.676 | 0.650 | 0.551 | 0.602 | 0.740 | 0.531 | 0.723 |
ATT.10 | 0.573 | 0.583 | 0.222 | 0.353 | 0.322 | 0.661 | 0.669 | 0.565 | 0.599 | 0.717 | 0.552 | 0.688 |
ATT.11 | 0.511 | 0.454 | 0.247 | 0.422 | 0.354 | 0.578 | 0.568 | 0.542 | 0.549 | 0.589 | 0.549 | 0.566 |
SN.1 | 0.278 | 0.226 | 0.386 | 0.298 | 0.302 | 0.227 | 0.247 | 0.299 | 0.344 | 0.197 | 0.268 | 0.244 |
SN.2 | 0.284 | 0.199 | 0.363 | 0.292 | 0.273 | 0.269 | 0.278 | 0.366 | 0.389 | 0.216 | 0.279 | 0.247 |
SN.3 | 0.283 | 0.239 | 0.372 | 0.371 | 0.416 | 0.326 | 0.343 | 0.388 | 0.407 | 0.285 | 0.312 | 0.315 |
SN.4 | 0.154 | 0.088 | 0.333 | 0.307 | 0.363 | 0.212 | 0.224 | 0.366 | 0.349 | 0.176 | 0.344 | 0.211 |
SN.5 | 0.270 | 0.228 | 0.347 | 0.370 | 0.349 | 0.284 | 0.281 | 0.390 | 0.390 | 0.287 | 0.308 | 0.279 |
SN.6 | 0.281 | 0.260 | 0.261 | 0.305 | 0.365 | 0.319 | 0.303 | 0.350 | 0.349 | 0.332 | 0.399 | 0.305 |
SN.7 | 0.065 | 0.021 | 0.396 | 0.330 | 0.314 | 0.101 | 0.114 | 0.242 | 0.167 | 0.032 | 0.229 | 0.044 |
PBC.1 | 0.287 | 0.309 | 0.122 | 0.111 | 0.148 | 0.287 | 0.270 | 0.208 | 0.228 | 0.265 | 0.219 | 0.209 |
PBC.2 | 0.365 | 0.371 | 0.161 | 0.196 | 0.219 | 0.244 | 0.233 | 0.238 | 0.329 | 0.282 | 0.280 | 0.260 |
PBC.3 | 0.383 | 0.339 | 0.217 | 0.216 | 0.214 | 0.358 | 0.375 | 0.335 | 0.446 | 0.323 | 0.337 | 0.329 |
PBC.4 | 0.253 | 0.283 | 0.241 | 0.220 | 0.207 | 0.277 | 0.262 | 0.255 | 0.266 | 0.255 | 0.295 | 0.224 |
PBC.5 | 0.301 | 0.321 | 0.161 | 0.182 | 0.223 | 0.418 | 0.398 | 0.367 | 0.416 | 0.342 | 0.381 | 0.322 |
PBC.6 | 0.325 | 0.260 | 0.287 | 0.259 | 0.256 | 0.288 | 0.359 | 0.354 | 0.409 | 0.248 | 0.293 | 0.214 |
PBC.7 | 0.316 | 0.303 | 0.169 | 0.214 | 0.227 | 0.399 | 0.399 | 0.313 | 0.364 | 0.339 | 0.337 | 0.266 |
SEN_APP.1 | 0.213 | 0.203 | 0.341 | 0.349 | 0.296 | 0.279 | 0.258 | 0.335 | 0.304 | 0.229 | 0.342 | 0.229 |
SEN_APP.2 | 0.244 | 0.228 | 0.347 | 0.359 | 0.278 | 0.253 | 0.263 | 0.364 | 0.282 | 0.246 | 0.294 | 0.223 |
SEN_APP.3 | 0.273 | 0.317 | 0.225 | 0.283 | 0.251 | 0.401 | 0.348 | 0.390 | 0.358 | 0.374 | 0.362 | 0.373 |
SEN_APP.4 | 0.266 | 0.308 | 0.250 | 0.329 | 0.335 | 0.288 | 0.317 | 0.333 | 0.295 | 0.322 | 0.356 | 0.312 |
INT.1 | 0.439 | 0.449 | 0.209 | 0.240 | 0.260 | 0.358 | 0.336 | 0.322 | 0.348 | 0.390 | 0.302 | 0.400 |
INT.2 | 0.400 | 0.414 | 0.221 | 0.214 | 0.268 | 0.382 | 0.352 | 0.317 | 0.323 | 0.407 | 0.303 | 0.367 |
INT.3 | 0.423 | 0.421 | 0.260 | 0.298 | 0.354 | 0.405 | 0.409 | 0.410 | 0.431 | 0.432 | 0.356 | 0.359 |
INT.4 | 0.431 | 0.414 | 0.200 | 0.247 | 0.260 | 0.406 | 0.400 | 0.376 | 0.416 | 0.451 | 0.351 | 0.412 |
INT.5 | 0.429 | 0.418 | 0.198 | 0.260 | 0.267 | 0.421 | 0.355 | 0.386 | 0.383 | 0.428 | 0.343 | 0.419 |
INT.6 | 0.331 | 0.322 | 0.237 | 0.305 | 0.286 | 0.353 | 0.339 | 0.372 | 0.367 | 0.405 | 0.328 | 0.279 |
ATT.8 | ATT.9 | ATT.10 | ATT.11 | SN.1 | SN.2 | SN.3 | SN.4 | SN.5 | SN.6 | SN.7 | PBC.1 | |
ATT.8 | 1.000 | |||||||||||
ATT.9 | 0.694 | 1.000 | ||||||||||
ATT.10 | 0.624 | 0.802 | 1.000 | |||||||||
ATT.11 | 0.620 | 0.641 | 0.672 | 1.000 | ||||||||
SN.1 | 0.289 | 0.243 | 0.274 | 0.273 | 1.000 | |||||||
SN.2 | 0.326 | 0.271 | 0.291 | 0.350 | 0.764 | 1.000 | ||||||
SN.3 | 0.357 | 0.334 | 0.354 | 0.382 | 0.687 | 0.697 | 1.000 | |||||
SN.4 | 0.274 | 0.200 | 0.241 | 0.311 | 0.552 | 0.568 | 0.653 | 1.000 | ||||
SN.5 | 0.382 | 0.316 | 0.345 | 0.412 | 0.672 | 0.750 | 0.653 | 0.636 | 1.000 | |||
SN.6 | 0.361 | 0.349 | 0.368 | 0.360 | 0.423 | 0.401 | 0.480 | 0.595 | 0.483 | 1.000 | ||
SN.7 | 0.154 | 0.067 | 0.116 | 0.196 | 0.467 | 0.461 | 0.422 | 0.524 | 0.489 | 0.421 | 1.000 | |
PBC.1 | 0.185 | 0.337 | 0.257 | 0.210 | 0.121 | 0.115 | 0.115 | 0.043 | 0.043 | 0.064 | -0.040 | 1.000 |
PBC.2 | 0.256 | 0.358 | 0.271 | 0.313 | 0.293 | 0.302 | 0.214 | 0.149 | 0.319 | 0.167 | 0.018 | 0.468 |
PBC.3 | 0.272 | 0.353 | 0.338 | 0.380 | 0.371 | 0.420 | 0.329 | 0.251 | 0.359 | 0.272 | 0.109 | 0.436 |
PBC.4 | 0.265 | 0.307 | 0.244 | 0.333 | 0.174 | 0.181 | 0.168 | 0.174 | 0.130 | 0.127 | 0.046 | 0.650 |
PBC.5 | 0.225 | 0.383 | 0.368 | 0.365 | 0.270 | 0.256 | 0.260 | 0.231 | 0.250 | 0.218 | 0.057 | 0.335 |
PBC.6 | 0.242 | 0.280 | 0.306 | 0.278 | 0.310 | 0.339 | 0.277 | 0.201 | 0.269 | 0.166 | 0.077 | 0.360 |
PBC.7 | 0.275 | 0.381 | 0.317 | 0.339 | 0.189 | 0.223 | 0.196 | 0.173 | 0.183 | 0.198 | 0.033 | 0.424 |
SEN_APP.1 | 0.281 | 0.207 | 0.228 | 0.355 | 0.308 | 0.316 | 0.269 | 0.309 | 0.289 | 0.226 | 0.213 | 0.138 |
SEN_APP.2 | 0.326 | 0.281 | 0.307 | 0.315 | 0.336 | 0.364 | 0.343 | 0.305 | 0.309 | 0.230 | 0.217 | 0.148 |
SEN_APP.3 | 0.394 | 0.376 | 0.385 | 0.399 | 0.255 | 0.273 | 0.284 | 0.290 | 0.261 | 0.299 | 0.121 | 0.238 |
SEN_APP.4 | 0.374 | 0.358 | 0.350 | 0.402 | 0.258 | 0.307 | 0.288 | 0.295 | 0.389 | 0.261 | 0.138 | 0.227 |
INT.1 | 0.348 | 0.471 | 0.408 | 0.395 | 0.288 | 0.308 | 0.260 | 0.195 | 0.296 | 0.301 | 0.082 | 0.382 |
INT.2 | 0.336 | 0.440 | 0.424 | 0.415 | 0.284 | 0.313 | 0.265 | 0.182 | 0.316 | 0.281 | 0.088 | 0.375 |
INT.3 | 0.392 | 0.428 | 0.422 | 0.440 | 0.290 | 0.307 | 0.292 | 0.213 | 0.317 | 0.338 | 0.128 | 0.341 |
INT.4 | 0.397 | 0.504 | 0.476 | 0.455 | 0.267 | 0.292 | 0.301 | 0.191 | 0.286 | 0.283 | 0.103 | 0.346 |
INT.5 | 0.428 | 0.498 | 0.467 | 0.432 | 0.270 | 0.285 | 0.281 | 0.208 | 0.292 | 0.296 | 0.077 | 0.360 |
INT.6 | 0.425 | 0.392 | 0.407 | 0.581 | 0.266 | 0.303 | 0.312 | 0.247 | 0.334 | 0.252 | 0.114 | 0.259 |
PBC.2 | PBC.3 | PBC.4 | PBC.5 | PBC.6 | PBC.7 | SEN_APP.1 | SEN_APP.2 | SEN_APP.3 | SEN_APP.4 | INT.1 | INT.2 | |
PBC.2 | 1.000 | |||||||||||
PBC.3 | 0.723 | 1.000 | ||||||||||
PBC.4 | 0.487 | 0.554 | 1.000 | |||||||||
PBC.5 | 0.593 | 0.643 | 0.533 | 1.000 | ||||||||
PBC.6 | 0.501 | 0.613 | 0.530 | 0.534 | 1.000 | |||||||
PBC.7 | 0.573 | 0.616 | 0.545 | 0.641 | 0.602 | 1.000 | ||||||
SEN_APP.1 | 0.233 | 0.275 | 0.236 | 0.307 | 0.267 | 0.266 | 1.000 | |||||
SEN_APP.2 | 0.215 | 0.230 | 0.197 | 0.285 | 0.271 | 0.265 | 0.686 | 1.000 | ||||
SEN_APP.3 | 0.293 | 0.309 | 0.320 | 0.360 | 0.289 | 0.328 | 0.586 | 0.603 | 1.000 | |||
SEN_APP.4 | 0.326 | 0.324 | 0.307 | 0.368 | 0.337 | 0.309 | 0.532 | 0.589 | 0.658 | 1.000 | ||
INT.1 | 0.420 | 0.461 | 0.366 | 0.424 | 0.351 | 0.406 | 0.295 | 0.324 | 0.336 | 0.401 | 1.000 | |
INT.2 | 0.420 | 0.440 | 0.381 | 0.425 | 0.334 | 0.429 | 0.322 | 0.318 | 0.323 | 0.402 | 0.868 | 1.000 |
INT.3 | 0.405 | 0.442 | 0.334 | 0.421 | 0.329 | 0.357 | 0.287 | 0.375 | 0.376 | 0.450 | 0.755 | 0.772 |
INT.4 | 0.366 | 0.428 | 0.360 | 0.432 | 0.307 | 0.424 | 0.308 | 0.374 | 0.339 | 0.433 | 0.794 | 0.773 |
INT.5 | 0.388 | 0.431 | 0.355 | 0.441 | 0.342 | 0.428 | 0.269 | 0.326 | 0.358 | 0.434 | 0.758 | 0.737 |
INT.6 | 0.405 | 0.401 | 0.403 | 0.428 | 0.331 | 0.405 | 0.382 | 0.346 | 0.443 | 0.471 | 0.606 | 0.648 |
INT.3 | INT.4 | INT.5 | INT.6 | |
INT.3 | 1.000 | |||
INT.4 | 0.766 | 1.000 | ||
INT.5 | 0.745 | 0.821 | 1.000 | |
INT.6 | 0.657 | 0.653 | 0.678 | 1.000 |
Variable | Item | Measurement Item | Source |
Intention to re-consume (INT) | INT1 | I intend to repurchase organic foods. | [17,35] |
INT2 | I intend to eat more organically processed foods in the future. | ||
INT3 | I would like to repurchase organic food because it is the best option. | ||
INT4 | I would like to reintroduce organic food, particularly environmentally friendly products. | ||
INT5 | I would like to re-consume organic food, preferably healthy and nutritious food. | ||
INT6 | For the welfare of farmers, I would like to re-consume organic food. | ||
Attitude (ATT) | ATT1 | Buying organic food is a good idea. | [17,35,62] |
ATT2 | Buying organic food is a wise decision. | ||
ATT3 | Buying organic food is important. | ||
ATT4 | Consuming organic food is advantageous. | ||
ATT5 | Consuming organic food is valuable. | ||
ATT6 | Consuming organic food is interesting. | ||
ATT7 | I like organic food because it is processed without the use of chemicals. | ||
ATT8 | I like organic food because it is more nutritious. | ||
ATT9 | I like organic food because it is healthier to consume. | ||
ATT10 | I like organic food because it is environmentally friendly. | ||
ATT11 | I like organic food because it allows me to contribute to the well-being of farmers. | ||
Subjective norms (SN) | SN1 | Most of the people important to me think that I should eat organic food. | [62,63,64] |
SN2 | My family believes that I should consume organic food. | ||
SN3 | People whose opinions I value believe that I should consume organic food. | ||
SN4 | My friend's positive feedback encourages me to consume organic food. | ||
SN5 | My loved ones expect me to consume organic food. | ||
SN6 | Because of external pressure (social, environmental, network, etc.), I choose organic food. | ||
Perceived Behavior Control (PBC) | PBC1 | It is entirely up to me whether or not I buy organic food. | [17,62,63,64] |
PBC2 | Consumers believe they can buy organic food. | ||
PBC3 | Consumers believe they have the resources, time, and willingness to buy organic food. | ||
PBC4 | Customers believe that they have control over whether or not they consume organic food. | ||
PBC5 | Consumers believe that there will be numerous opportunities for them to consume organic food. | ||
PBC6 | Consumers find it simple to consume organic food. | ||
PBC7 | Consumers believe they can consume organic food if they desire to do so. | ||
Sensory Appeal Perceived (SEN_APP) | SEN_APP1 | In my opinion, organic food is more attractive. | [18,40,62,65] |
SEN_APP2 | In my opinion, organic food appears to be tasty. | ||
SEN_APP3 | In my opinion, organic food looks fresher. (especial for rice, in question is natural / no over-processing after harvest) | ||
SEN_APP4 | In my opinion, organic food has a pleasant texture. | ||
Warm Glow (WG) | WG1 | Buying organic food gives me a pleasant feeling. | [17,21,51,66,67] |
WG2 | Consuming organic food makes me happy because it makes me feel that I have done something good. | ||
WG3 | I am happy when I can make choices to consume organic food, for myself and my family. | ||
WG4 | Every time I consume organic food, I feel good about myself because I have protected the environment. | ||
WG5 | I am satisfied because I have contributed to the efficiency of natural resources by consuming organic food. | ||
WG6 | Consuming organic food makes me feel good because it contributes to the preservation of the quality of the Earth (soil, water, etc.). | ||
WG7 | Purchasing organic food makes me happy because I am contributing to the well-being of farmers. | ||
WG8 | I am pleased that I have contributed to the growth of the local economy by purchasing organic food. | ||
Egoistic motive (E) | E1 | I eat organic food to better maintain my health. | [1,23,45,68] |
E2 | Organic food is the food that my family consumes for the sake of our family's health. | ||
E3 | I eat organic food to provide my body with more nutrition. | ||
E4 | I choose organic foods to obtain products that are safer to consume. |
Demographic Variable | N | % | |
Age (years old) | 17–25 | 179 | 53.12 |
26–35 | 97 | 28.78 | |
36–45 | 25 | 7.42 | |
56–55 | 31 | 9.20 | |
> 56 | 5 | 1.48 | |
Gender | Male | 95 | 28.19 |
Female | 242 | 71.81 | |
Education qualification | Higher secondary | 52 | 15.43 |
Graduate | 212 | 62.91 | |
Postgraduate | 71 | 21.07 | |
Any other | 2 | 0.59 | |
Marital status | Single | 210 | 62.31 |
Married | 127 | 37.69 | |
Employment status | Employed | 171 | 50.74 |
Unemployed | 12 | 3.56 | |
Retired | 2 | 0.59 | |
Homemaker | 37 | 10.98 | |
Student | 115 | 34.12 |
CR | AVE | 1 | 2 | 3 | 4 | 5 | 6 | 7 | |
1.Attitude | 0.953 | 0.649 | |||||||
2.Egoistic motive | 0.943 | 0.806 | 0.808 | ||||||
3.Intention to re-consume | 0.955 | 0.781 | 0.589 | 0.536 | |||||
4.PBC | 0.918 | 0.650 | 0.531 | 0.485 | 0.605 | ||||
5.Sesory appeal | 0.905 | 0.705 | 0.524 | 0.365 | 0.541 | 0.485 | |||
6.Subjecctive norms | 0.931 | 0.731 | 0.473 | 0.345 | 0.394 | 0.401 | 0.476 | ||
7.Warm Glow | 0.959 | 0.747 | 0.768 | 0.689 | 0.547 | 0.494 | 0.452 | 0.394 |
Hypothesis | Path | Std Beta | t-value | Result | f2 |
H1 | ATT → INT | 0.289 | 3.518* | Supported | 0.097 |
SN → ATT | 0.112 | 3.271* | Supported | 0.033 | |
SN → INT | 0.042 | 0.816ns | Rejected | 0.002 | |
PBC → INT | 0.313 | 4.738* | Supported | 0.125 | |
H2 | SEN_APP → ATT | 0.141 | 2.763* | Supported | 0.050 |
SEN_APP → INT | 0.204 | 3.045* | Supported | 0.052 | |
H3 | E → ATT | 0.457 | 8.594* | Supported | 0.405 |
WG → ATT | 0.333 | 7.542* | Supported | 0.197 | |
Note: *p < 0.05. |
Items | Outer Loading | Cross Loading | ||||||||||||
ATT | E | INT | PBC | SEN_APP | SN | WG | ATT | E | INT | PBC | SEN_APP | SN | WG | |
SEN_APP.1 | 0.812 | 0.342 | 0.219 | 0.352 | 0.328 | 0.812 | 0.346 | 0.282 | ||||||
SEN_APP.2 | 0.842 | 0.356 | 0.247 | 0.390 | 0.302 | 0.842 | 0.387 | 0.296 | ||||||
SEN_APP.3 | 0.859 | 0.469 | 0.312 | 0.411 | 0.393 | 0.859 | 0.317 | 0.395 | ||||||
SEN_APP.4 | 0.845 | 0.418 | 0.314 | 0.490 | 0.407 | 0.845 | 0.362 | 0.400 | ||||||
WG.1 | 0.839 | 0.657 | 0.605 | 0.429 | 0.394 | 0.351 | 0.357 | 0.839 | ||||||
WG.2 | 0.837 | 0.590 | 0.511 | 0.468 | 0.423 | 0.353 | 0.311 | 0.837 | ||||||
WG.3 | 0.860 | 0.621 | 0.605 | 0.448 | 0.387 | 0.348 | 0.336 | 0.860 | ||||||
WG.4 | 0.902 | 0.648 | 0.579 | 0.468 | 0.410 | 0.381 | 0.314 | 0.902 | ||||||
WG.5 | 0.896 | 0.646 | 0.554 | 0.458 | 0.388 | 0.354 | 0.315 | 0.896 | ||||||
WG.6 | 0.879 | 0.644 | 0.618 | 0.425 | 0.383 | 0.335 | 0.271 | 0.879 | ||||||
WG.7 | 0.854 | 0.600 | 0.474 | 0.473 | 0.386 | 0.393 | 0.334 | 0.854 | ||||||
WG.8 | 0.847 | 0.625 | 0.512 | 0.422 | 0.363 | 0.359 | 0.315 | 0.847 | ||||||
SN.1 | 0.858 | 0.327 | 0.308 | 0.314 | 0.333 | 0.340 | 0.858 | 0.266 | ||||||
SN.2 | 0.889 | 0.370 | 0.291 | 0.342 | 0.356 | 0.372 | 0.889 | 0.304 | ||||||
SN.3 | 0.868 | 0.429 | 0.294 | 0.324 | 0.299 | 0.351 | 0.868 | 0.377 | ||||||
SN.4 | 0.781 | 0.326 | 0.156 | 0.234 | 0.245 | 0.356 | 0.781 | 0.275 | ||||||
SN.5 | 0.875 | 0.415 | 0.298 | 0.348 | 0.314 | 0.374 | 0.875 | 0.341 | ||||||
PBC.2 | 0.807 | 0.347 | 0.386 | 0.454 | 0.807 | 0.323 | 0.302 | 0.353 | ||||||
PBC.3 | 0.865 | 0.434 | 0.410 | 0.491 | 0.865 | 0.342 | 0.408 | 0.359 | ||||||
PBC.4 | 0.746 | 0.337 | 0.296 | 0.415 | 0.746 | 0.321 | 0.192 | 0.359 | ||||||
PBC.5 | 0.824 | 0.449 | 0.347 | 0.486 | 0.824 | 0.397 | 0.296 | 0.377 | ||||||
PBC.6 | 0.766 | 0.369 | 0.331 | 0.376 | 0.766 | 0.349 | 0.328 | 0.376 | ||||||
PBC.7 | 0.824 | 0.421 | 0.350 | 0.462 | 0.824 | 0.350 | 0.226 | 0.375 | ||||||
E.1 | 0.899 | 0.682 | 0.899 | 0.420 | 0.357 | 0.281 | 0.296 | 0.583 | ||||||
E.2 | 0.888 | 0.655 | 0.888 | 0.449 | 0.431 | 0.285 | 0.316 | 0.556 | ||||||
E.3 | 0.907 | 0.702 | 0.907 | 0.463 | 0.402 | 0.299 | 0.301 | 0.584 | ||||||
E.4 | 0.898 | 0.670 | 0.898 | 0.460 | 0.390 | 0.321 | 0.234 | 0.597 | ||||||
INT.1 | 0.900 | 0.462 | 0.481 | 0.900 | 0.504 | 0.407 | 0.318 | 0.448 | ||||||
INT.2 | 0.904 | 0.461 | 0.435 | 0.904 | 0.504 | 0.409 | 0.322 | 0.427 | ||||||
INT.3 | 0.886 | 0.507 | 0.466 | 0.886 | 0.476 | 0.448 | 0.335 | 0.469 | ||||||
INT.4 | 0.908 | 0.526 | 0.448 | 0.908 | 0.482 | 0.437 | 0.316 | 0.466 | ||||||
INT.5 | 0.896 | 0.517 | 0.462 | 0.896 | 0.495 | 0.420 | 0.315 | 0.459 | ||||||
INT.6 | 0.803 | 0.481 | 0.353 | 0.803 | 0.492 | 0.494 | 0.345 | 0.475 | ||||||
ATT.1 | 0.847 | 0.847 | 0.610 | 0.439 | 0.413 | 0.367 | 0.312 | 0.613 | ||||||
ATT.10 | 0.857 | 0.857 | 0.647 | 0.492 | 0.383 | 0.384 | 0.356 | 0.618 | ||||||
ATT.11 | 0.778 | 0.778 | 0.515 | 0.514 | 0.417 | 0.441 | 0.408 | 0.633 | ||||||
ATT.2 | 0.843 | 0.843 | 0.629 | 0.414 | 0.420 | 0.357 | 0.325 | 0.615 | ||||||
ATT.3 | 0.750 | 0.750 | 0.565 | 0.413 | 0.384 | 0.423 | 0.424 | 0.564 | ||||||
ATT.4 | 0.803 | 0.803 | 0.615 | 0.429 | 0.462 | 0.370 | 0.441 | 0.579 | ||||||
ATT.5 | 0.858 | 0.858 | 0.660 | 0.475 | 0.373 | 0.355 | 0.276 | 0.585 | ||||||
ATT.6 | 0.723 | 0.723 | 0.498 | 0.375 | 0.399 | 0.404 | 0.351 | 0.556 | ||||||
ATT.7 | 0.758 | 0.758 | 0.605 | 0.422 | 0.337 | 0.345 | 0.306 | 0.540 | ||||||
ATT.8 | 0.766 | 0.766 | 0.621 | 0.440 | 0.317 | 0.414 | 0.385 | 0.554 | ||||||
ATT.9 | 0.865 | 0.865 | 0.705 | 0.516 | 0.429 | 0.371 | 0.324 | 0.594 | ||||||
Description: ATT: Attitude; E: Egoistic; INT: Intention to re-consume; PBC: Perceived Behavioral Control; SEN_APP: Sensory Appeal Perceived; SN: Subjective norm; WG: Warm Glow. |
Mean | Standard Deviation | Matrix Correlations | ||||||||||
WG.1 | WG.2 | WG.3 | WG.4 | WG.5 | WG.6 | WG.7 | WG.8 | E.1 | E.2 | |||
WG.1 | 4.228 | 0.738 | 1.000 | |||||||||
WG.2 | 4.267 | 0.702 | 0.771 | 1.000 | ||||||||
WG.3 | 4.270 | 0.744 | 0.774 | 0.726 | 1.000 | |||||||
WG.4 | 4.285 | 0.740 | 0.690 | 0.693 | 0.755 | 1.000 | ||||||
WG.5 | 4.279 | 0.778 | 0.654 | 0.685 | 0.721 | 0.852 | 1.000 | |||||
WG.6 | 4.356 | 0.713 | 0.652 | 0.658 | 0.703 | 0.814 | 0.833 | 1.000 | ||||
WG.7 | 4.246 | 0.764 | 0.632 | 0.652 | 0.630 | 0.716 | 0.724 | 0.700 | 1.000 | |||
WG.8 | 4.208 | 0.792 | 0.620 | 0.621 | 0.635 | 0.704 | 0.715 | 0.705 | 0.867 | 1.000 | ||
E.1 | 4.513 | 0.702 | 0.564 | 0.450 | 0.570 | 0.535 | 0.499 | 0.571 | 0.401 | 0.432 | 1.000 | |
E.2 | 4.288 | 0.806 | 0.513 | 0.441 | 0.524 | 0.494 | 0.468 | 0.508 | 0.425 | 0.464 | 0.735 | 1.000 |
E.3 | 4.365 | 0.774 | 0.529 | 0.465 | 0.535 | 0.523 | 0.516 | 0.544 | 0.435 | 0.486 | 0.730 | 0.758 |
E.4 | 4.501 | 0.698 | 0.567 | 0.478 | 0.545 | 0.527 | 0.508 | 0.595 | 0.442 | 0.456 | 0.764 | 0.703 |
E.5 | 3.202 | 1.211 | 0.307 | 0.289 | 0.289 | 0.283 | 0.303 | 0.226 | 0.264 | 0.318 | 0.283 | 0.302 |
E.6 | 3.685 | 1.117 | 0.454 | 0.421 | 0.431 | 0.421 | 0.395 | 0.398 | 0.418 | 0.466 | 0.433 | 0.413 |
E.7 | 3.644 | 1.152 | 0.448 | 0.426 | 0.382 | 0.401 | 0.392 | 0.382 | 0.420 | 0.393 | 0.321 | 0.347 |
ATT.1 | 4.395 | 0.659 | 0.608 | 0.510 | 0.515 | 0.554 | 0.543 | 0.560 | 0.467 | 0.474 | 0.569 | 0.529 |
ATT.2 | 4.344 | 0.693 | 0.577 | 0.512 | 0.522 | 0.555 | 0.565 | 0.538 | 0.473 | 0.507 | 0.569 | 0.566 |
ATT.3 | 4.145 | 0.847 | 0.479 | 0.469 | 0.517 | 0.506 | 0.492 | 0.465 | 0.458 | 0.512 | 0.498 | 0.560 |
ATT.4 | 4.193 | 0.771 | 0.522 | 0.470 | 0.499 | 0.527 | 0.514 | 0.485 | 0.468 | 0.512 | 0.502 | 0.588 |
ATT.5 | 4.469 | 0.640 | 0.534 | 0.494 | 0.476 | 0.526 | 0.519 | 0.571 | 0.450 | 0.470 | 0.587 | 0.578 |
ATT.6 | 4.139 | 0.834 | 0.527 | 0.469 | 0.489 | 0.464 | 0.475 | 0.450 | 0.491 | 0.482 | 0.460 | 0.439 |
ATT.7 | 4.475 | 0.739 | 0.498 | 0.465 | 0.469 | 0.474 | 0.477 | 0.479 | 0.445 | 0.425 | 0.582 | 0.503 |
ATT.8 | 4.329 | 0.783 | 0.471 | 0.423 | 0.479 | 0.489 | 0.512 | 0.508 | 0.450 | 0.493 | 0.556 | 0.489 |
ATT.9 | 4.528 | 0.631 | 0.551 | 0.439 | 0.518 | 0.523 | 0.529 | 0.578 | 0.469 | 0.487 | 0.648 | 0.553 |
ATT.10 | 4.481 | 0.654 | 0.523 | 0.470 | 0.520 | 0.600 | 0.582 | 0.588 | 0.482 | 0.500 | 0.619 | 0.548 |
ATT.11 | 4.362 | 0.739 | 0.534 | 0.518 | 0.497 | 0.517 | 0.506 | 0.471 | 0.663 | 0.678 | 0.437 | 0.448 |
SN.1 | 3.706 | 0.953 | 0.281 | 0.237 | 0.292 | 0.220 | 0.247 | 0.171 | 0.193 | 0.199 | 0.301 | 0.303 |
SN.2 | 3.712 | 0.976 | 0.297 | 0.273 | 0.279 | 0.245 | 0.262 | 0.211 | 0.270 | 0.269 | 0.268 | 0.294 |
SN.3 | 3.742 | 0.972 | 0.384 | 0.319 | 0.363 | 0.333 | 0.327 | 0.282 | 0.325 | 0.274 | 0.268 | 0.265 |
SN.4 | 3.724 | 0.992 | 0.253 | 0.230 | 0.218 | 0.232 | 0.215 | 0.189 | 0.313 | 0.258 | 0.157 | 0.159 |
SN.5 | 3.733 | 0.990 | 0.299 | 0.261 | 0.271 | 0.298 | 0.282 | 0.286 | 0.323 | 0.336 | 0.261 | 0.312 |
SN.6 | 3.932 | 0.974 | 0.381 | 0.244 | 0.345 | 0.295 | 0.303 | 0.279 | 0.334 | 0.307 | 0.307 | 0.275 |
SN.7 | 2.861 | 1.315 | 0.131 | 0.108 | 0.087 | 0.074 | 0.108 | 0.043 | 0.132 | 0.159 | 0.058 | 0.074 |
PBC.1 | 4.318 | 0.733 | 0.310 | 0.331 | 0.327 | 0.271 | 0.298 | 0.306 | 0.274 | 0.244 | 0.289 | 0.252 |
PBC.2 | 4.181 | 0.680 | 0.314 | 0.309 | 0.267 | 0.304 | 0.286 | 0.314 | 0.325 | 0.321 | 0.302 | 0.349 |
PBC.3 | 4.160 | 0.713 | 0.325 | 0.336 | 0.293 | 0.330 | 0.326 | 0.273 | 0.309 | 0.293 | 0.322 | 0.431 |
PBC.4 | 4.228 | 0.726 | 0.329 | 0.335 | 0.309 | 0.277 | 0.303 | 0.302 | 0.321 | 0.310 | 0.271 | 0.258 |
PBC.5 | 4.240 | 0.679 | 0.323 | 0.388 | 0.312 | 0.360 | 0.322 | 0.307 | 0.321 | 0.277 | 0.270 | 0.356 |
PBC.6 | 4.039 | 0.841 | 0.287 | 0.349 | 0.363 | 0.349 | 0.337 | 0.323 | 0.295 | 0.300 | 0.243 | 0.364 |
PBC.7 | 4.291 | 0.657 | 0.328 | 0.334 | 0.343 | 0.367 | 0.312 | 0.343 | 0.301 | 0.266 | 0.313 | 0.324 |
SEN_APP.1 | 4.104 | 0.761 | 0.243 | 0.265 | 0.228 | 0.248 | 0.202 | 0.183 | 0.313 | 0.274 | 0.167 | 0.203 |
SEN_APP.2 | 4.053 | 0.745 | 0.242 | 0.251 | 0.269 | 0.263 | 0.266 | 0.249 | 0.248 | 0.258 | 0.203 | 0.212 |
SEN_APP.3 | 4.276 | 0.688 | 0.344 | 0.302 | 0.347 | 0.370 | 0.350 | 0.326 | 0.362 | 0.331 | 0.278 | 0.253 |
SEN_APP.4 | 4.172 | 0.689 | 0.331 | 0.353 | 0.309 | 0.375 | 0.348 | 0.340 | 0.382 | 0.331 | 0.277 | 0.279 |
INT.1 | 4.374 | 0.594 | 0.387 | 0.423 | 0.416 | 0.399 | 0.397 | 0.365 | 0.379 | 0.333 | 0.408 | 0.432 |
INT.2 | 4.374 | 0.604 | 0.354 | 0.416 | 0.376 | 0.399 | 0.359 | 0.359 | 0.379 | 0.315 | 0.359 | 0.388 |
INT.3 | 4.276 | 0.670 | 0.413 | 0.436 | 0.398 | 0.410 | 0.416 | 0.366 | 0.424 | 0.384 | 0.374 | 0.457 |
INT.4 | 4.350 | 0.603 | 0.380 | 0.438 | 0.411 | 0.421 | 0.431 | 0.393 | 0.392 | 0.357 | 0.395 | 0.366 |
INT.5 | 4.392 | 0.597 | 0.383 | 0.409 | 0.416 | 0.405 | 0.416 | 0.404 | 0.400 | 0.342 | 0.412 | 0.400 |
INT.6 | 4.326 | 0.658 | 0.354 | 0.357 | 0.353 | 0.442 | 0.402 | 0.359 | 0.525 | 0.496 | 0.279 | 0.337 |
E.3 | E.4 | E.5 | E.6 | E.7 | ATT.1 | ATT.2 | ATT.3 | ATT.4 | ATT.5 | ATT.6 | ATT.7 | |
E.3 | 1.000 | |||||||||||
E.4 | 0.759 | 1.000 | ||||||||||
E.5 | 0.320 | 0.221 | 1.000 | |||||||||
E.6 | 0.452 | 0.392 | 0.703 | 1.000 | ||||||||
E.7 | 0.362 | 0.303 | 0.660 | 0.741 | 1.000 | |||||||
ATT.1 | 0.537 | 0.556 | 0.268 | 0.390 | 0.376 | 1.000 | ||||||
ATT.2 | 0.578 | 0.544 | 0.320 | 0.415 | 0.425 | 0.858 | 1.000 | |||||
ATT.3 | 0.525 | 0.449 | 0.414 | 0.475 | 0.384 | 0.625 | 0.657 | 1.000 | ||||
ATT.4 | 0.612 | 0.504 | 0.359 | 0.432 | 0.425 | 0.650 | 0.697 | 0.656 | 1.000 | |||
ATT.5 | 0.601 | 0.603 | 0.192 | 0.331 | 0.307 | 0.757 | 0.720 | 0.597 | 0.677 | 1.000 | ||
ATT.6 | 0.445 | 0.446 | 0.372 | 0.429 | 0.438 | 0.591 | 0.579 | 0.534 | 0.618 | 0.567 | 1.000 | |
ATT.7 | 0.532 | 0.557 | 0.148 | 0.289 | 0.279 | 0.572 | 0.544 | 0.421 | 0.521 | 0.602 | 0.461 | 1.000 |
ATT.8 | 0.639 | 0.539 | 0.337 | 0.454 | 0.400 | 0.530 | 0.529 | 0.514 | 0.558 | 0.610 | 0.480 | 0.607 |
ATT.9 | 0.650 | 0.679 | 0.191 | 0.329 | 0.271 | 0.676 | 0.650 | 0.551 | 0.602 | 0.740 | 0.531 | 0.723 |
ATT.10 | 0.573 | 0.583 | 0.222 | 0.353 | 0.322 | 0.661 | 0.669 | 0.565 | 0.599 | 0.717 | 0.552 | 0.688 |
ATT.11 | 0.511 | 0.454 | 0.247 | 0.422 | 0.354 | 0.578 | 0.568 | 0.542 | 0.549 | 0.589 | 0.549 | 0.566 |
SN.1 | 0.278 | 0.226 | 0.386 | 0.298 | 0.302 | 0.227 | 0.247 | 0.299 | 0.344 | 0.197 | 0.268 | 0.244 |
SN.2 | 0.284 | 0.199 | 0.363 | 0.292 | 0.273 | 0.269 | 0.278 | 0.366 | 0.389 | 0.216 | 0.279 | 0.247 |
SN.3 | 0.283 | 0.239 | 0.372 | 0.371 | 0.416 | 0.326 | 0.343 | 0.388 | 0.407 | 0.285 | 0.312 | 0.315 |
SN.4 | 0.154 | 0.088 | 0.333 | 0.307 | 0.363 | 0.212 | 0.224 | 0.366 | 0.349 | 0.176 | 0.344 | 0.211 |
SN.5 | 0.270 | 0.228 | 0.347 | 0.370 | 0.349 | 0.284 | 0.281 | 0.390 | 0.390 | 0.287 | 0.308 | 0.279 |
SN.6 | 0.281 | 0.260 | 0.261 | 0.305 | 0.365 | 0.319 | 0.303 | 0.350 | 0.349 | 0.332 | 0.399 | 0.305 |
SN.7 | 0.065 | 0.021 | 0.396 | 0.330 | 0.314 | 0.101 | 0.114 | 0.242 | 0.167 | 0.032 | 0.229 | 0.044 |
PBC.1 | 0.287 | 0.309 | 0.122 | 0.111 | 0.148 | 0.287 | 0.270 | 0.208 | 0.228 | 0.265 | 0.219 | 0.209 |
PBC.2 | 0.365 | 0.371 | 0.161 | 0.196 | 0.219 | 0.244 | 0.233 | 0.238 | 0.329 | 0.282 | 0.280 | 0.260 |
PBC.3 | 0.383 | 0.339 | 0.217 | 0.216 | 0.214 | 0.358 | 0.375 | 0.335 | 0.446 | 0.323 | 0.337 | 0.329 |
PBC.4 | 0.253 | 0.283 | 0.241 | 0.220 | 0.207 | 0.277 | 0.262 | 0.255 | 0.266 | 0.255 | 0.295 | 0.224 |
PBC.5 | 0.301 | 0.321 | 0.161 | 0.182 | 0.223 | 0.418 | 0.398 | 0.367 | 0.416 | 0.342 | 0.381 | 0.322 |
PBC.6 | 0.325 | 0.260 | 0.287 | 0.259 | 0.256 | 0.288 | 0.359 | 0.354 | 0.409 | 0.248 | 0.293 | 0.214 |
PBC.7 | 0.316 | 0.303 | 0.169 | 0.214 | 0.227 | 0.399 | 0.399 | 0.313 | 0.364 | 0.339 | 0.337 | 0.266 |
SEN_APP.1 | 0.213 | 0.203 | 0.341 | 0.349 | 0.296 | 0.279 | 0.258 | 0.335 | 0.304 | 0.229 | 0.342 | 0.229 |
SEN_APP.2 | 0.244 | 0.228 | 0.347 | 0.359 | 0.278 | 0.253 | 0.263 | 0.364 | 0.282 | 0.246 | 0.294 | 0.223 |
SEN_APP.3 | 0.273 | 0.317 | 0.225 | 0.283 | 0.251 | 0.401 | 0.348 | 0.390 | 0.358 | 0.374 | 0.362 | 0.373 |
SEN_APP.4 | 0.266 | 0.308 | 0.250 | 0.329 | 0.335 | 0.288 | 0.317 | 0.333 | 0.295 | 0.322 | 0.356 | 0.312 |
INT.1 | 0.439 | 0.449 | 0.209 | 0.240 | 0.260 | 0.358 | 0.336 | 0.322 | 0.348 | 0.390 | 0.302 | 0.400 |
INT.2 | 0.400 | 0.414 | 0.221 | 0.214 | 0.268 | 0.382 | 0.352 | 0.317 | 0.323 | 0.407 | 0.303 | 0.367 |
INT.3 | 0.423 | 0.421 | 0.260 | 0.298 | 0.354 | 0.405 | 0.409 | 0.410 | 0.431 | 0.432 | 0.356 | 0.359 |
INT.4 | 0.431 | 0.414 | 0.200 | 0.247 | 0.260 | 0.406 | 0.400 | 0.376 | 0.416 | 0.451 | 0.351 | 0.412 |
INT.5 | 0.429 | 0.418 | 0.198 | 0.260 | 0.267 | 0.421 | 0.355 | 0.386 | 0.383 | 0.428 | 0.343 | 0.419 |
INT.6 | 0.331 | 0.322 | 0.237 | 0.305 | 0.286 | 0.353 | 0.339 | 0.372 | 0.367 | 0.405 | 0.328 | 0.279 |
ATT.8 | ATT.9 | ATT.10 | ATT.11 | SN.1 | SN.2 | SN.3 | SN.4 | SN.5 | SN.6 | SN.7 | PBC.1 | |
ATT.8 | 1.000 | |||||||||||
ATT.9 | 0.694 | 1.000 | ||||||||||
ATT.10 | 0.624 | 0.802 | 1.000 | |||||||||
ATT.11 | 0.620 | 0.641 | 0.672 | 1.000 | ||||||||
SN.1 | 0.289 | 0.243 | 0.274 | 0.273 | 1.000 | |||||||
SN.2 | 0.326 | 0.271 | 0.291 | 0.350 | 0.764 | 1.000 | ||||||
SN.3 | 0.357 | 0.334 | 0.354 | 0.382 | 0.687 | 0.697 | 1.000 | |||||
SN.4 | 0.274 | 0.200 | 0.241 | 0.311 | 0.552 | 0.568 | 0.653 | 1.000 | ||||
SN.5 | 0.382 | 0.316 | 0.345 | 0.412 | 0.672 | 0.750 | 0.653 | 0.636 | 1.000 | |||
SN.6 | 0.361 | 0.349 | 0.368 | 0.360 | 0.423 | 0.401 | 0.480 | 0.595 | 0.483 | 1.000 | ||
SN.7 | 0.154 | 0.067 | 0.116 | 0.196 | 0.467 | 0.461 | 0.422 | 0.524 | 0.489 | 0.421 | 1.000 | |
PBC.1 | 0.185 | 0.337 | 0.257 | 0.210 | 0.121 | 0.115 | 0.115 | 0.043 | 0.043 | 0.064 | -0.040 | 1.000 |
PBC.2 | 0.256 | 0.358 | 0.271 | 0.313 | 0.293 | 0.302 | 0.214 | 0.149 | 0.319 | 0.167 | 0.018 | 0.468 |
PBC.3 | 0.272 | 0.353 | 0.338 | 0.380 | 0.371 | 0.420 | 0.329 | 0.251 | 0.359 | 0.272 | 0.109 | 0.436 |
PBC.4 | 0.265 | 0.307 | 0.244 | 0.333 | 0.174 | 0.181 | 0.168 | 0.174 | 0.130 | 0.127 | 0.046 | 0.650 |
PBC.5 | 0.225 | 0.383 | 0.368 | 0.365 | 0.270 | 0.256 | 0.260 | 0.231 | 0.250 | 0.218 | 0.057 | 0.335 |
PBC.6 | 0.242 | 0.280 | 0.306 | 0.278 | 0.310 | 0.339 | 0.277 | 0.201 | 0.269 | 0.166 | 0.077 | 0.360 |
PBC.7 | 0.275 | 0.381 | 0.317 | 0.339 | 0.189 | 0.223 | 0.196 | 0.173 | 0.183 | 0.198 | 0.033 | 0.424 |
SEN_APP.1 | 0.281 | 0.207 | 0.228 | 0.355 | 0.308 | 0.316 | 0.269 | 0.309 | 0.289 | 0.226 | 0.213 | 0.138 |
SEN_APP.2 | 0.326 | 0.281 | 0.307 | 0.315 | 0.336 | 0.364 | 0.343 | 0.305 | 0.309 | 0.230 | 0.217 | 0.148 |
SEN_APP.3 | 0.394 | 0.376 | 0.385 | 0.399 | 0.255 | 0.273 | 0.284 | 0.290 | 0.261 | 0.299 | 0.121 | 0.238 |
SEN_APP.4 | 0.374 | 0.358 | 0.350 | 0.402 | 0.258 | 0.307 | 0.288 | 0.295 | 0.389 | 0.261 | 0.138 | 0.227 |
INT.1 | 0.348 | 0.471 | 0.408 | 0.395 | 0.288 | 0.308 | 0.260 | 0.195 | 0.296 | 0.301 | 0.082 | 0.382 |
INT.2 | 0.336 | 0.440 | 0.424 | 0.415 | 0.284 | 0.313 | 0.265 | 0.182 | 0.316 | 0.281 | 0.088 | 0.375 |
INT.3 | 0.392 | 0.428 | 0.422 | 0.440 | 0.290 | 0.307 | 0.292 | 0.213 | 0.317 | 0.338 | 0.128 | 0.341 |
INT.4 | 0.397 | 0.504 | 0.476 | 0.455 | 0.267 | 0.292 | 0.301 | 0.191 | 0.286 | 0.283 | 0.103 | 0.346 |
INT.5 | 0.428 | 0.498 | 0.467 | 0.432 | 0.270 | 0.285 | 0.281 | 0.208 | 0.292 | 0.296 | 0.077 | 0.360 |
INT.6 | 0.425 | 0.392 | 0.407 | 0.581 | 0.266 | 0.303 | 0.312 | 0.247 | 0.334 | 0.252 | 0.114 | 0.259 |
PBC.2 | PBC.3 | PBC.4 | PBC.5 | PBC.6 | PBC.7 | SEN_APP.1 | SEN_APP.2 | SEN_APP.3 | SEN_APP.4 | INT.1 | INT.2 | |
PBC.2 | 1.000 | |||||||||||
PBC.3 | 0.723 | 1.000 | ||||||||||
PBC.4 | 0.487 | 0.554 | 1.000 | |||||||||
PBC.5 | 0.593 | 0.643 | 0.533 | 1.000 | ||||||||
PBC.6 | 0.501 | 0.613 | 0.530 | 0.534 | 1.000 | |||||||
PBC.7 | 0.573 | 0.616 | 0.545 | 0.641 | 0.602 | 1.000 | ||||||
SEN_APP.1 | 0.233 | 0.275 | 0.236 | 0.307 | 0.267 | 0.266 | 1.000 | |||||
SEN_APP.2 | 0.215 | 0.230 | 0.197 | 0.285 | 0.271 | 0.265 | 0.686 | 1.000 | ||||
SEN_APP.3 | 0.293 | 0.309 | 0.320 | 0.360 | 0.289 | 0.328 | 0.586 | 0.603 | 1.000 | |||
SEN_APP.4 | 0.326 | 0.324 | 0.307 | 0.368 | 0.337 | 0.309 | 0.532 | 0.589 | 0.658 | 1.000 | ||
INT.1 | 0.420 | 0.461 | 0.366 | 0.424 | 0.351 | 0.406 | 0.295 | 0.324 | 0.336 | 0.401 | 1.000 | |
INT.2 | 0.420 | 0.440 | 0.381 | 0.425 | 0.334 | 0.429 | 0.322 | 0.318 | 0.323 | 0.402 | 0.868 | 1.000 |
INT.3 | 0.405 | 0.442 | 0.334 | 0.421 | 0.329 | 0.357 | 0.287 | 0.375 | 0.376 | 0.450 | 0.755 | 0.772 |
INT.4 | 0.366 | 0.428 | 0.360 | 0.432 | 0.307 | 0.424 | 0.308 | 0.374 | 0.339 | 0.433 | 0.794 | 0.773 |
INT.5 | 0.388 | 0.431 | 0.355 | 0.441 | 0.342 | 0.428 | 0.269 | 0.326 | 0.358 | 0.434 | 0.758 | 0.737 |
INT.6 | 0.405 | 0.401 | 0.403 | 0.428 | 0.331 | 0.405 | 0.382 | 0.346 | 0.443 | 0.471 | 0.606 | 0.648 |
INT.3 | INT.4 | INT.5 | INT.6 | |
INT.3 | 1.000 | |||
INT.4 | 0.766 | 1.000 | ||
INT.5 | 0.745 | 0.821 | 1.000 | |
INT.6 | 0.657 | 0.653 | 0.678 | 1.000 |