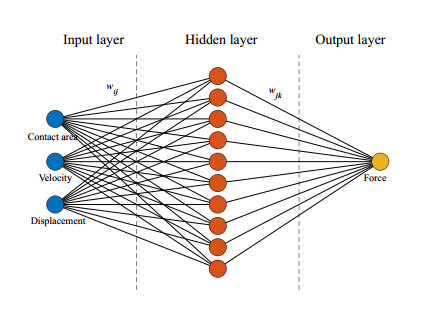
This study reviews the literature focused on nation brands and brand culture through the innovative combination of latent Dirichlet allocation with a multinomial and unordered discrete choice analysis. Unlike a narrow perspective of bibliometric work, which confines itself to reviewing existing literature within a specific research domain, a broader viewpoint leverages bibliometric analysis to pinpoint potential research opportunities indicative of emerging trends in related fields. Adopting this comprehensive paradigm, the current study scrutinizes 60 articles spanning the timeframe from 1992 to 2021. The analysis discerns six prospective marketing strategies instrumental in propelling a country to global brand prominence: the synergistic integration of country-of-origin and city brands, consumption branding, materialistic branding, green branding, ideological branding, and scientific branding. Notably, environmental branding has assumed a pivotal global role post–2015, while ideological branding represents a more recent trend centered on diligent efforts to invigorate national identity systems. Empirical insights underscore the need of a multidisciplinary approach in the creation of nation brands, suggesting that distinct strategies need not be mutually exclusive. Quantitatively, it is found evidence that covering one additional environmental topic in a study increases (decreases) its likelihood of belonging to the consumption (ideology) cluster by 50.8 (50.6) percentage points, respectively. Strategic recommendations for future national endeavors emphasize the significance of becoming a Stackelberg leader in the race to generate added value. Collectively, these findings underscore that the bibliometric analysis employed to elucidate the evolution of nation brands and brand culture, typically associated with international marketing, unveils two promising niche areas for future research in green finance: green nation brands and green brand culture. The former pertains to asset allocations within green enterprises and environmental sectors, enhancing a country's symbolic commitment to the burgeoning green paradigm. Meanwhile, the later delves into the internalization of fintech development's growth and intermediary effects, fostering green innovation, energy efficiency, and green supply chains. This bottom-up approach is geared towards meeting community-based needs and presents valuable avenues for future exploration in the field of green finance.
Citation: Vitor Miguel Ribeiro. Pioneering paradigms: unraveling niche opportunities in green finance through bibliometric analysis of nation brands and brand culture[J]. Green Finance, 2024, 6(2): 287-347. doi: 10.3934/GF.2024012
[1] | Teng Fei, Hongjun Wang, Lanxue Liu, Liyi Zhang, Kangle Wu, Jianing Guo . Research on multi-strategy improved sparrow search optimization algorithm. Mathematical Biosciences and Engineering, 2023, 20(9): 17220-17241. doi: 10.3934/mbe.2023767 |
[2] | Akim Kapsalyamov, Shahid Hussain, Prashant K. Jamwal . A novel compliant surgical robot: Preliminary design analysis. Mathematical Biosciences and Engineering, 2020, 17(3): 1944-1958. doi: 10.3934/mbe.2020103 |
[3] | Liwei Deng, Zhen Liu, Tao Zhang, Zhe Yan . Study of visual SLAM methods in minimally invasive surgery. Mathematical Biosciences and Engineering, 2023, 20(3): 4388-4402. doi: 10.3934/mbe.2023203 |
[4] | Xiangyang Ren, Shuai Chen, Kunyuan Wang, Juan Tan . Design and application of improved sparrow search algorithm based on sine cosine and firefly perturbation. Mathematical Biosciences and Engineering, 2022, 19(11): 11422-11452. doi: 10.3934/mbe.2022533 |
[5] | Anlu Yuan, Tieyi Zhang, Lingcong Xiong, Zhipeng Zhang . Torque control strategy of electric racing car based on acceleration intention recognition. Mathematical Biosciences and Engineering, 2024, 21(2): 2879-2900. doi: 10.3934/mbe.2024128 |
[6] | Kai Cheng, Lixia Li, Yanmin Du, Jiangtao Wang, Zhenghua Chen, Jian Liu, Xiangsheng Zhang, Lin Dong, Yuanyuan Shen, Zhenlin Yang . A systematic review of image-guided, surgical robot-assisted percutaneous puncture: Challenges and benefits. Mathematical Biosciences and Engineering, 2023, 20(5): 8375-8399. doi: 10.3934/mbe.2023367 |
[7] | Xinran Zhang, Yongde Zhang, Jianzhi Yang, Haiyan Du . A prostate seed implantation robot system based on human-computer interactions: Augmented reality and voice control. Mathematical Biosciences and Engineering, 2024, 21(5): 5947-5971. doi: 10.3934/mbe.2024262 |
[8] | Xiaoguang Liu, Jiawei Wang, Tie Liang, Cunguang Lou, Hongrui Wang, Xiuling Liu . SE-TCN network for continuous estimation of upper limb joint angles. Mathematical Biosciences and Engineering, 2023, 20(2): 3237-3260. doi: 10.3934/mbe.2023152 |
[9] | Zhen Yang, Junli Li, Liwei Yang, Qian Wang, Ping Li, Guofeng Xia . Path planning and collision avoidance methods for distributed multi-robot systems in complex dynamic environments. Mathematical Biosciences and Engineering, 2023, 20(1): 145-178. doi: 10.3934/mbe.2023008 |
[10] | Liwei Yang, Lixia Fu, Ping Li, Jianlin Mao, Ning Guo, Linghao Du . LF-ACO: an effective formation path planning for multi-mobile robot. Mathematical Biosciences and Engineering, 2022, 19(1): 225-252. doi: 10.3934/mbe.2022012 |
This study reviews the literature focused on nation brands and brand culture through the innovative combination of latent Dirichlet allocation with a multinomial and unordered discrete choice analysis. Unlike a narrow perspective of bibliometric work, which confines itself to reviewing existing literature within a specific research domain, a broader viewpoint leverages bibliometric analysis to pinpoint potential research opportunities indicative of emerging trends in related fields. Adopting this comprehensive paradigm, the current study scrutinizes 60 articles spanning the timeframe from 1992 to 2021. The analysis discerns six prospective marketing strategies instrumental in propelling a country to global brand prominence: the synergistic integration of country-of-origin and city brands, consumption branding, materialistic branding, green branding, ideological branding, and scientific branding. Notably, environmental branding has assumed a pivotal global role post–2015, while ideological branding represents a more recent trend centered on diligent efforts to invigorate national identity systems. Empirical insights underscore the need of a multidisciplinary approach in the creation of nation brands, suggesting that distinct strategies need not be mutually exclusive. Quantitatively, it is found evidence that covering one additional environmental topic in a study increases (decreases) its likelihood of belonging to the consumption (ideology) cluster by 50.8 (50.6) percentage points, respectively. Strategic recommendations for future national endeavors emphasize the significance of becoming a Stackelberg leader in the race to generate added value. Collectively, these findings underscore that the bibliometric analysis employed to elucidate the evolution of nation brands and brand culture, typically associated with international marketing, unveils two promising niche areas for future research in green finance: green nation brands and green brand culture. The former pertains to asset allocations within green enterprises and environmental sectors, enhancing a country's symbolic commitment to the burgeoning green paradigm. Meanwhile, the later delves into the internalization of fintech development's growth and intermediary effects, fostering green innovation, energy efficiency, and green supply chains. This bottom-up approach is geared towards meeting community-based needs and presents valuable avenues for future exploration in the field of green finance.
With the progression of technology, minimally invasive surgical (MISR) robots have incrementally supplanted traditional surgical approaches, demonstrating superior performance in terms of operative clarity, user-friendliness, and operational adaptability [1]. Moreover, the implementation of MIS robots is commonly associated with enhanced patient outcomes, reduced pain levels, minimized blood loss and scarring, as well as shortened recovery time [2]. However, due to the lack of direct access to the surgical site, surgeons tend to exert excessive force, resulting in tissue damage [3]. The absence of haptic feedback is widely recognized as a significant challenge in current robot-assisted laparoscopic surgery [4,5]. The accuracy of force feedback is crucial for determining tissue properties and executing delicate surgical procedures. The accuracy of force feedback is crucial for the identification of tissue properties and the execution of precise surgical procedures.
Force feedback plays a pivotal role in surgical procedures, facilitating surgeons to perceive the mechanical characteristics of tissues, assess their anatomical structure, and execute precise force-control actions for ensuring safe tissue manipulation [6,7]. To demonstrate the feasibility and efficacy of force feedback in laparoscopic surgery, Wagner and Semere incorporated a commercially available force/torque sensor at the distal end of the surgical instrument to precisely measure tool-tissue forces. The measured forces were then fed back to the master robot through a teleoperation system, enabling the effective execution of blunt dissection tasks. Remarkably, this implementation of force feedback resulted in a significant 50% reduction in applied force and an impressive 66% decrease in tissue damage error [8,9].
To date, extensive research has been conducted on the force feedback of minimally invasive surgical robots. The existing methods for estimating forces in surgical robots can be broadly categorized into direct force sensing and sensorless force estimation [10,11]. In the direct force sensing method, the force exerted by the surgical tool on the tissue is quantified through an integrated sensor positioned either on or in close proximity to the tool [12,13]. Using piezoresistive sensors, Abiri et al. [14] developed a device that can be affixed to the tip of a surgical instrument for measuring interaction forces. Furthermore, Reiley et al. [15] incorporated strain gauges onto the shafts of surgical tools for measuring interaction forces. However, challenges such as biocompatibility, sterilization, and miniaturization impose limitations on the feasibility of this approach [16,17]. The exposure to high temperatures, pressures, and humidity during sterilization procedures may potentially inflict damage upon electronic components [18]. Zarrin et al. [19] and Carotenuto et al. [20] proposed a laparoscopic sensing instrument based on Fiber Bragg grating (FBG) that demonstrates resistance to high-temperature sterilization. However, the durability of the fiber is inadequate and it exhibits mechanical limitations during complex movements.
In contrast, sensorless force estimation methods enable the prediction of forces using existing resources, obviating the need for additional electronics. Haghighipanah et al. [21] and Wang et al. [22], employed a cable tension disturbance observer on the surgical robot's end effector to quantify external forces. Tholey et al. [23] and Zhao et al. [24] utilized the driving motor's current as an estimation of the force exerted between the gripper and tissue at the distal end of the surgical robot. However, the utilization of these motion-based methods poses challenges in acquiring precise modeling information for surgical tools or robotic operators, thereby hindering the establishment of an accurate method for predicting interaction forces. In addition, there are vision-based force estimation methods. When force is applied, the deformation of the soft tissues is captured by the camera. This information is then used to predict the actual force exerted on the object [25,26,27]. Noohi et al. [28] propose a virtual template-based approach for estimating tool-soft tissue interactions using monocular camera images. In [25], relying exclusively on endoscopic images acquired by the stereo camera, Haouchine employed a dynamic biomechanical model based on organ shape to accurately estimate the contact force at the tool tip. To enhance force estimation accuracy, Mozaffari et al. [29] introduced a neural fuzzy inference system for identifying tool-tissue forces and maximum local stress in laparoscopic surgery. Despite extensive research on replacing force sensors with image sensors, occlusion, optical noise, and camera motion can still introduce interference in the image, thereby impacting the accuracy of force estimation. These studies demonstrate that both approaches relying on dynamic motion information and strategies based on advanced visual analysis exhibit a certain level of stability, robustness, and efficacy in estimating applied forces. Although these methods have inherent limitations in terms of predictive accuracy, they possess significant potential for wide-ranging applications in the field of force estimation.
Building upon the aforementioned research, this study aims to further investigate and advance the new trajectory of force estimation technology. We propose a novel approach for clamping force prediction based on mechanical clamp blade motion parameters. In this methodology, a backpropagation (BP) neural network is employed to construct a soft tissue force prediction model by considering the interplay between soft tissue pressure, instrument displacement, motion speed, and indenter contact area. The BP neural network exhibits exceptional capabilities in nonlinear analysis, generalization, and fault tolerance, rendering it suitable for effectively capturing the intricate mapping relationship between the clamping force of soft tissue and the motion parameters of the clamp blade. However, the convergence speed of BP neural networks is often slow and they are prone to getting trapped in local optima. Therefore, this study proposes the integration of the sparrow search algorithm (SSA) to enhance the predictive performance of the model for clamping force in dynamic and non-linear surgical environments by optimizing the weights and thresholds of BP neural networks. The proposed algorithm surpasses existing algorithms, such as the genetic algorithm (GA), in terms of search accuracy, convergence speed, and stability [30,31]. This is crucial for facilitating rapid and precise decision-making in surgical applications. The model's prediction performance was validated through an in vitro compression experiment on pig kidney tissue. This model not only enables real-time clamping force prediction, but also facilitates more accurate estimation of the force between the instrument and tissue during future surgical procedures, thereby enhancing surgical safety and efficacy.
The remaining sections of the paper are organized as follows: Section 2 provides a detailed description of the design and implementation of soft tissue compression experiments. Section 3 elaborates on the algorithms employed for predicting soft tissue gripping force, along with their theoretical foundations. In Section 4, we present experimental results and conduct an in-depth analysis of the performance of our proposed prediction model. Finally, Section 5 summarizes the research findings and discusses potential avenues for future investigation.
Soft tissues exhibit intricate nonlinear and stage-dependent characteristics, posing challenges for traditional modeling approaches to accurately capture their behavior. Therefore, in this study, we propose a mechanical model based on the SSA-optimized BP neural network to precisely simulate the dynamic mechanical response of soft tissues under continuous compression. The proposed approach integrates the SSA's capability in global optimization with the efficiency of the BP neural network for function approximation and simulation of complex system behavior, enabling a comprehensive understanding of soft tissue's mechanical response under compression.
In our study, we employ BP neural networks to forecast the mechanical properties of soft tissues. BP networks are renowned for their inherent capability to automatically discern intricate relationships between input and output data, a crucial aspect in comprehending the nonlinear mechanical response exhibited by soft tissues. Through the network's self-learning and adaptive capabilities, we can encode the mechanical behavior of soft tissue into its weights, thereby achieving more accurate simulation and prediction of its response.
The BP neural network is comprised of multiple layers, typically including an input layer, one or more hidden layers, and an output layer. In this study, the structure of the soft tissue force prediction model based on the BP neural network is illustrated in Figure 1, enabling the network to effectively capture data patterns ranging from simple to intricate.
The operation process of the BP neural network is primarily divided into two stages: forward propagation and backpropagation. During the forward propagation phase, the input signal undergoes a sequential pass through each layer of the network. At each node, the input signal is multiplied by its corresponding weight, added to the bias term, and then transformed into an output signal using an activation function. The input s(l)j of each neuron j in each layer l is obtained through a linear combination of the activation values o(l−1)j, weights w(l)y, and biases b(l)j from the previous layer, which can be calculated as follows:
s(l)j=∑iw(l)ijo(l−1)j+b(l)j | (1) |
The activation value o(l)j of neuron j corresponds to the output obtained by applying the input signal through the activation function:
o(l)j=f(s(l)j) | (2) |
where f(⋅) is the activation function, and the sigmoid function f(x)=11+e−x is usually chosen.
In the backpropagation phase, the network's weight and threshold are updated through error minimization between the network output and target output. Specifically, the network error is defined as the sum of squared differences between the desired output and actual output:
E=12∑Nk−1(yk−o(L)k)2 | (3) |
where yk is the target output value, o(L)k is the actual output value of the network, L represents the output layer, and k traverses all output neurons. Therefore, the error gradient is calculated as follows:
The error gradient for neuron k in the output layer L is as follows:
δ(L)k=(o(L)k−yk)−f′(s(L)k) | (4) |
The error gradient for neuron j in hidden layer l (l < L) is as follows:
δ(l)j=(∑kw(l+1)jkδ(l+1)k)⋅f′(s(l)j) | (5) |
The weight and threshold adjustments of the neural network are updated using the gradient descent method:
w(l)ij=w(l)ij−η⋅o(l)iδ(l)j) | (6) |
blj=blj−η⋅δlj | (7) |
where, η is the learning rate, a positive number less than 1.
The formula for determining the number of neurons in the hidden layer is:
m=√n+0+a | (8) |
where m is the number of nodes in the hidden layer, n is the number of nodes in the input layer, o is the number of nodes in the output layer, and a is a constant between 1 and 10.
The BP neural network demonstrates exceptional performance in automatically extracting association rules between input and output data, while effectively retaining the acquired knowledge within its network weights, thereby exhibiting remarkable self-learning and adaptive characteristics. However, when confronted with complex nonlinear data such as soft tissue mechanics, BP networks encounter specific challenges. These include relatively slow convergence rates and high sensitivity to initial weight settings, which may result in the network getting trapped in local minima during the learning process and failing to accurately approximate intricate real-world models. This limitation becomes particularly evident when processing soft tissue data characterized by complex mechanical behavior, necessitating optimization of relevant parameters to overcome these challenges.
The SSA, proposed by Xue and Shen in 2020 [32], is based on the behavioral patterns observed in sparrow populations, encompassing the distinct roles of producers, followers, and scouts. By simulating the foraging and predator avoidance strategies of these characters, SSA demonstrated superior capabilities in global search. We integrate this algorithm with a BP neural network to optimize the initialization and adjustment process of the BP network by leveraging SSA's global search abilities. This integration not only enhances the convergence speed of the model, but also improves its adaptability in tackling complex and nonlinear problems, particularly in simulating the mechanical behavior of soft tissues.
A population of n sparrows can be represented as:
X=[X1,1X1,2⋯X1,dX2,1X2,2⋯X2,dXn,1Xn,2⋯Xn,d] | (9) |
where d represents the dimensionality of the problem variable to be optimized and n denotes the number of sparrows. In the subsequent optimization problem, d signifies the number of BP parameters that need to be optimized, that is, the total number of weights and biases in the BP neural network. Consequently, all sparrows' fitness values can be expressed as:
Fx=[f([X1,1X1,2⋯X1,d])f([X2,1X2,2⋯X2,d])f([Xn,1Xn,2⋯Xn,d])] | (10) |
where f is the fitness value. According to the above SSA principle, the optimization objective function can be established as:
f=1NN∑k=1(yk−o(L)k)2 | (11) |
where N is the total number of training sets. yk and o(L)k are the true and predicted values of the kth data, respectively. The fitness function indicates that we ultimately want to get a network with a small training set error. The producer position is updated during the process of population iteration as follows:
Xt+1i,j={Xti,j∗exp(−i∂⋅itemmax)R2<STXti,j+Q⋅LR2>ST | (12) |
where t represents the current iteration value, j represents the dimension between 1 and d, Xti,j represents the value of the jth dimension when the ith sparrow iterates t times, and itemmax is the number with the most iterations. R2∈[0,1] and ST∈[0.5,1.0] represent the alarm value and safety threshold, respectively. The variable Q is a random number that conforms to a normal distribution, while L represents an 1*d matrix consisting of all elements equal to 1. If R2<ST, then the sparrow population is safe. Otherwise, some sparrows perceive danger, and all sparrows must quickly move to a safe area.
The follower's position is continuously updated, ensuring constant monitoring of the producer. In the event that the follower detects a superior food source, it engages in competitive interactions to acquire it. If successful, the follower obtains access to the food resource; otherwise, it continues its vigilant surveillance.
Xt+1i,j={Q⋅exp(Xtworst−Xti,jj2)i>n/2Xt+1p+|Xti,j−Xt+1p|⋅A+⋅Lotherwise | (13) |
where Xtp represents the best position occupied by the producer, and Xtworst indicates the current worst position. A represents an 1*d matrix where the elements are randomly assigned values of either 1 or -1, and A∗=AT(AAT)−1. When i>n/2, the ith follower exhibits a suboptimal fitness value attributed to its state of hunger.
The sparrow position is updated in the event of danger as follows:
Xt+1i,j={Xtbest+β⋅|Xti,j−Xtbest|fi>fgXti,j+K⋅(Xti,j−Xtworst(fi−fw)+ε)fi=fg | (14) |
Scouts constitute 10–20 percent of the population, with Xttest representing the current optimal proportion. The variable β follows a standard normal distribution with a mean of 0 and a variance of 1. K∈[−1,1] is a random number. fi represents the current fitness value of the sparrow. fg and fw are the current best and worst fitness values. The constant ε represents the minimum value required to prevent zero partitioning error. The value of fi>fg indicates that the sparrow is positioned at the periphery of the population, rendering it susceptible to predation by hazardous factors. The condition fi=fg indicates that the sparrow positioned in the center of the population possesses awareness of potential threats and necessitates repositioning closer to other sparrows. K represents both the direction of movement for the sparrow and serves as a control coefficient for determining step size [33].
The present study introduces a novel prediction model that combines the SSA algorithm with the BP neural network, aiming to enhance the precision of soft tissue gripping force prediction in micro-invasive surgery. During the training process, we initially determine the specific structural parameters of the input layer, hidden layer, and output layer. Subsequently, SSA is employed to explore optimal weights and biases. The initialization of sparrow population parameters includes specifying the number of sparrows in the population, proportion of discoverers, warning value threshold, and maximum iteration count. In order to precisely guide the optimization process, the fitness function of SSA is defined as the error function of the neural network for evaluating and adjusting the search strategy. Subsequently, based on optimal weight and optimal deviation, prediction of clamping force in soft tissue is performed. The flow chart illustrating the entire optimization process is presented in Figure 2. The key steps of the SSA-BP prediction model are outlined as follows:
1) Construct the initial BP neural network and initialize the weights and biases of the network.
2) Configure the parameters of the SSA algorithm, including sparrow population size, alarm value, safety threshold, and iteration number. Evaluate and rank the fitness of each individual sparrow, identify the location of current optimal and worst solutions, and update the sparrow's fitness in subsequent iterations.
3) Employ SSA to optimize the BP prediction model and iterate through the optimization process until reaching either a predetermined error range or maximum number of iterations.
4) Upon completion of iteration, apply the optimized weights and deviations obtained by SSA to the BP network.
5) Utilize the optimized BP network model for predicting and analyzing collected soft tissue pressure data.
The evaluation indices employed in this study encompass root mean square error (RMSE), mean square error (MSE), mean absolute error (MAE), sum of squares of error (SSE), and coefficient of determination (R2). The evaluation of model prediction error and prediction effect primarily relies on RMSE, MSE, MAE, SSE, and R2. A smaller value for RMSE, MSE, MAE, and SSE indicates a better prediction effect, while a larger value for R2 suggests an improved prediction effect. The following equations represent the calculation formulas for RMSE, MSE, MAE, SSE, and R2. In these equations, o(L)k denotes the predicted output value, yk represents the actual value, ¯y signifies the mean value of yk, and N corresponds to the number of samples.
RMSE=√1NN∑k=1|yk−o(L)k|2 | (15) |
MSE=1NN∑k=1|yk−o(L)k|2 | (16) |
MAE=1NN∑k=1|yk−σ(L)k| | (17) |
SSE=N∑k=1(yk−o(L)k)2 | (18) |
R2=1−N∑k=1(o(L)k−yk)2N∑k=1(yk−ˉy)2 | (19) |
In this study, a series of in vitro experiments were conducted to simulate surgical procedures and construct a model that accurately reflects the mechanical response of biological soft tissues. The experimental design was rigorous and the data obtained were highly accurate, ensuring the reliability of the developed model.
The primary equipment utilized in the experiment is the SHIMADZU (EZ-LX) universal mechanical testing machine, manufactured by Shimadzu Corporation (Japan). This device features a high-precision force sensor, and its mechanical arm is equipped with a specially designed clamp blade to facilitate swift replacement of experimental instruments without compromising the data reading accuracy of the sensor during experimentation. The experimental setup, as depicted in Figure 3, was utilized as the test platform. Its operation involved controlling the clamp blades with varying contact areas to achieve a uniform compression at low speed until reaching the predetermined compression depth. Subsequently, compression was halted and the force sensor reading was recorded.
Considering the influence of the contact area between the clamp leaf and soft tissue during surgical procedures, three types of indenters were designed to simulate various operation scenarios, each with different contact areas. The indenter utilized in the experiment, as depicted in Figure 4, exhibits respective contact areas of 45.76, 29.29, and 16.47 mm2. Detailed dimensions of the indenter are provided in Table 1. This variation allows for an assessment of the impact that contact area has on pressure.
Type | Scale | Profile size (mm) | Slot size (mm) | Contact area (mm2) | Gear height (mm) | Gear angle (°) |
YT1 | 1 | 15.12*5.0 | 12.60*2.40 | 45.76 | 0.2 | 90 |
YT2 | 0.8 | 12.16*4.0 | 10.08*1.92 | 29.29 | ||
YT3 | 0.6 | 9.12*3.0 | 7.56*1.44 | 16.47 |
The pig kidney was selected as the experimental sample to simulate laparoscopic surgery due to its high availability and similar tissue characteristics to human abdominal organs. Specifically, pig kidneys offer an ideal model for simulating urinary system surgery. Following removal from pigs, the samples were cut into standardized chunks (30 ± 1 mm × 15 ± 1 mm, thickness 8 ± 1 mm) and stored in portable coolers to maintain freshness and biomechanical stability. The experiment commenced within 5 hours after removal to ensure preservation of the tissue's biomechanical properties.
The experimental parameters encompass compression depth and loading speed to replicate the clamping procedure in real surgical scenarios.
1) Compression depth: To prevent direct contact between the forceps leaf and the support platform beneath the tissue sample during experimentation, which could potentially overload the sensor, a strict upper limit of compression depth was imposed equal to the thickness of the sample. Considering that the treated pig kidney tissue sample had an approximate thickness of 8mm, a maximum compression depth of 3mm was set during experimentation to ensure test process safety and data reliability.
2) Loading speed: The study revealed that the average clamping velocity of the laparoscopic clamp on soft tissue during the operation ranged from 1.2 to 2.4 mm/s [34,35,36]. To accurately simulate surgical conditions, forceps blades were subjected to four different loading speeds in this experiment: 0.5, 1, 1.5, and 2 mm/s, aiming to investigate the impact of varying speeds on compression efficacy.
In order to ensure the stability of the biological properties of the soft tissues throughout the experiment, we opted for a controlled temperature range of 24 ± 2 ℃ and a humidity range of 45–55%. Prior to the commencement of the experiment, the sample was extracted from the cooler and allowed to cool down at room temperature. Subsequently, the clamp blade indenter was affixed to the sensor of the mechanical testing machine ensuring proper alignment with the sample on the test platform. Compression experiments were performed at four different loading speeds, each compression reaching its predetermined maximum depth, while real-time monitoring and recording of force variations were facilitated by dedicated software integrated into the testing apparatus. Figure 5 illustrates an experimental scenario depicting pig kidney tissue compression using forceps leaves.
Based on the obtained experimental data on compression, a preliminary analysis was conducted on the mechanical properties of pig kidney tissue. The temporal pressure curve is depicted in Figure 6.
As illustrated in Figure 6, the stress of pig kidney tissue under static load can be categorized into two distinct stages. In the initial stage, namely from O to 𝐺0, the pressure exhibits a non-linear increase over time until it reaches its peak value 𝐺0. This initial phase of rapid growth reflects the immediate elastic response of the organization and is referred to as the stress response phase. In the transition stage from 𝐺0 to 𝐺1, the pressure gradually decreases over time and eventually reaches a stable state. At 𝐺0𝐺1, the curve demonstrates a decreasing pressure trend with respect to time, indicating that the tissue undergoes relaxation under continuous stress. This stage signifies the gradual adaptation of the material to applied stress and is characteristic of stress relaxation. Although the relaxation process can theoretically extend for several hours until complete tissue relaxation is achieved, this study specifically focuses on the initial phase of relaxation, i.e., the stress variations over a brief time period. During the experiment, the duration of the relaxation stage was less than 60 seconds, yet it yielded a significant effect on tissue relaxation.
This study focuses on the modeling and optimization of stress segments exhibiting hyperelastic behavior during compression in the stress response phase. The influence of experimental parameters, such as press speed and indenter's accessible area, on the applied pressure during interaction with soft tissue is thoroughly investigated and analyzed. By constructing a mechanical model based on collected experimental data, we accurately describe and predict the behavior of soft tissue under compressive forces.
During the compression experiment, precise control of indenter movement speed was achieved using computer software. The compression speeds were set at 0.5, 1, 1.5, and 2 mm/s to simulate the compression of diverse biological tissues with different contact areas. Each indenter continuously compressed pig kidney tissue at its corresponding speed until reaching a preset compression depth, after which the compression was halted to record data. This allowed for obtaining the relationship between pressure exerted by three distinct forceps indenters on pig kidney tissue and compression depth under various loading speeds, as depicted in Figure 7.
As shown in Figure 7, the relationship between the pressure exerted by the clamp indenter with different contact area and the compression depth under constant speed is analyzed. The findings demonstrate a consistent increase in pressure with an expanding contact area of the indenter, indicating a discernible pattern. Specifically, larger contact areas result in higher peak pressures applied to pig kidney tissue. This phenomenon can be attributed to increased deformation volume of pig kidney tissue during compression due to larger contact areas, leading to greater strain. Considering the viscoelastic properties inherent in pig kidney tissue, this translates into a substantial increase in pressure when interacting with an indenter featuring a larger contact area at equivalent compression depths.
To accurately assess the influence of pressing speed on compression force, we maintained a constant contact area for the indenter while varying the compression speed, as depicted in Figure 8.
The pressure-compression depth relation curve in Figure 8 reveals that, under identical conditions, an increase in compression speed results in a strengthened interaction between the indenter and pig kidney tissue, consequently elevating the peak force value. This phenomenon signifies that higher compression speeds amplify the impact force exerted by the indenter on the structure, leading to more pronounced structural deformation within a shorter duration and subsequently generating augmented pressure.
Through comparative analysis of the data presented in Figures 7 and 8, it was observed that porcine kidney tissue exhibited similar rates of force change when pressure was applied by indenters with different contact areas at a fixed compression speed. However, altering the compression speed while maintaining a constant contact area resulted in significant differences in the rate of force change. This finding suggests that during the force-response phase of the compression experiment, the impact of compression speed on the force experienced by pig kidney tissue is more critical than that of contact area size.
In this study, to assess the prediction efficiency of the SSA-BP neural network model, we employed two classical neural network prediction models for comparison: the standard BP neural network and the GA-BP (Genetic Algorithm optimized BP) neural network. All models were evaluated on an identical dataset to ensure impartiality.
As a model capable of mapping multi-dimensional functions, the BP neural network possesses inherent advantages in addressing complex relationship analysis. In our experimental setup, the input layer comprises three neurons corresponding to the compression depth, compression speed, and contact area of the indenter. The number of neurons in the hidden layer was determined using an empirical formula, resulting in a final network structure of 3-10-10-1. The learning rate is set at 0.0001 with an expected prediction accuracy of 10e-8 and a maximum iteration limit of 5000. Moreover, we specify the tansig function as the transfer function between the input layer and hidden layer while employing the purelin function as the transfer function between hidden layer and output layer. During the training process, we utilize the traindx algorithm as our chosen training method.
The GA-BP neural network models employ genetic algorithms to optimize the initial weights and thresholds of the network. Key parameters encompass a population size of 45 individuals, a maximum number of genetic iterations set at 55, a generation gap value of 0.85, a crossover probability of 0.6, and a mutation probability amounting to 0.022.
The SSA-BP neural network model employs the SSA for optimizing the network parameters. The parameter configuration of the model includes a population size of 20, a maximum iteration count of 30, a discoverer's share in the population set at 15%, and a maximum safety threshold limited to 0.7.
The present study compares and analyzes the root mean square error (RMSE) iteration of the three distinct models during the training process, with the corresponding results presented in Figures 9–11. The error curve of the SSA-BP model (Figure 11) reveals that it achieves the lowest RMSE on both the validation and test sets at the 36th iteration, indicating superior convergence performance compared to GA-BP and standard BP models. The error curve of the GA-BP model, in contrast, exhibits a tendency to plateau after 15 iterations, implying a potential convergence towards a local optimum solution. This outcome underscores the efficacy of SSA optimization strategies in expediting the training process of BP neural networks and showcasing notable advantages in achieving rapid convergence towards lower error levels.
In order to evaluate the training efficacy of the aforementioned prediction model, we compare the predicted data from the experimental sample with the actual experimental data. Figure 12 illustrates the test results obtained using SSA-BP, GA-BP, and BP neural network models. It is evident that optimizing the BP neural network with SSA has significantly enhanced its predictive performance, resulting in a higher correlation between optimized predictions and actual data compared to those generated by the GA-BP model. The comparison of absolute error (AE) among SSA-BP, GA-BP, and BP neural networks is illustrated in Figure 13. It can be observed that the initial network error is below 0.15 N, while the GA-optimized BP network achieves an error rate below 0.1 N and the SSA-optimized BP network attains an even lower error rate below 0.05 N. These results demonstrate that the SSA-BP neural network model exhibits excellent predictive performance and successfully meets the desired target.
To ensure the reliability of simulation results, three prediction models were compared and analyzed based on their performance indexes, which included RMSE, MSE, MAE and SSE. Generally speaking, lower values of these indicators indicate better predictive performance and higher model accuracy. The GA-BP and SSA-BP neural network models exhibit significant improvement in all error indicators when compared to the standard BP model, as depicted in Figure 14. Notably, the SSA-BP model demonstrates the most substantial reduction in errors. The coefficient of determination (R2) serves as a crucial metric for assessing the efficacy of model fitting to a given dataset. Notably, the SSA-BP neural network exhibits the highest R2 value, indicating superior data fitting capability. Consequently, the SSA-BP model demonstrates enhanced predictive capability compared to other comparative models.
The present study aims to investigate the force feedback in minimally invasive surgery, with a specific focus on the intricate interaction between surgical instruments and delicate biological soft tissues. In order to address the issue of inadequate clamping force feedback mechanisms in existing minimally invasive surgical robot systems, this study proposes a clamping force detection scheme based on clamp blade parameters. Through a series of compression tests conducted on isolated pig kidney tissue using a universal test machine, the relationship between pressure and compression depth, compression speed, and contact area of the indenter is thoroughly analyzed, leading to the establishment of a mechanical model. This model utilizes an SSA-optimized BP neural network for accurate estimation and prediction of clamping force during surgery. A comparative analysis with traditional BP models and GA-BP models reveals that the SSA-BP model outperforms both in terms of prediction accuracy and convergence speed. Notably, when considering key performance indicators such as the RMSE, MSE, MAE, and SSE, the SSA-BP model demonstrates superior predictive ability and higher degree of fit.
In summary, the main conclusions of this study are as follows:
1) The key factors influencing the clamping force of surgical instruments were determined through rigorous experimentation, providing a solid empirical foundation for the development of mechanical models.
2) The proposed SSA-BP neural network model exhibits remarkable advantages in accurately predicting force feedback, thereby enhancing its predictive accuracy.
3) Simulation results validate the potential of the SSA-BP model in significantly improving the precision of clamping force prediction within minimally invasive surgical robot systems.
Future work will primarily focus on enhancing the model's generalization capabilities and validating it across a broader range of biological soft tissues. Furthermore, there will be an exploration of integrating this predictive model into existing minimally invasive surgical robot systems to enhance their force-feedback mechanisms and improve overall surgical safety and efficiency.
The authors declare they have not used Artificial Intelligence (AI) tools in the creation of this article.
This paper is supported by National Natural Science Foundation of China under grant No. 52305066.
The authors declare there is no conflict of interest.
[1] |
Aaker DA (1996) Measuring brand equity across products and markets. Calif Manag Rev 38: 102–120. http://dx.doi.org/10.2307/41165845 doi: 10.2307/41165845
![]() |
[2] |
Abramo G, D'Angelo CA (2011) Evaluating research: from informed peer review to bibliometrics. Scientometrics 87: 499–514. https://doi.org/10.1007/s11192-011-0352-7 doi: 10.1007/s11192-011-0352-7
![]() |
[3] |
Adriaanse LS, Rensleigh C (2013) Web of Science, Scopus and Google Scholar: A content comprehensiveness comparison. The Electronic Library 31: 727–744. https://doi.org/10.1108/EL-12-2011-0174 doi: 10.1108/EL-12-2011-0174
![]() |
[4] |
Aguilar-Moreno JA, Palos-Sanchez PR, Pozo-Barajas R (2024). Sentiment analysis to support business decision-making. A bibliometric study. AIMS Mathematics 9: 4337–4375. https://doi.org/10.3934/math.2024215 doi: 10.3934/math.2024215
![]() |
[5] | Aldridge S, Halpern D, Fitzpatrick S (2002) Social capital: A discussion paper. Cabinet Office, London. https://ntouk.files.wordpress.com/2020/07/socialcapital.pdf |
[6] |
Alserhan BA, Halkias D, Boulanouar AW, et al. (2015) Expressing herself through brands: the arab woman's perspective. J Res Mark Entrep 17: 36–53. https://doi.org/10.1108/JRME-09-2014-0024 doi: 10.1108/JRME-09-2014-0024
![]() |
[7] | Anderson B (1983) Imagined communities: Reflections on the origin and spread of nationalism Verso books. Available from: https://www.taylorfrancis.com/chapters/edit/10.4324/9781003060963-46/imagined-communities-benedict-anderson. |
[8] | Anholt S (2002) Nation branding: A continuing theme. J Brand Manag 10: 59–59. Available from: https://www.elibrary.ru/item.asp?id = 6247609. |
[9] |
Anholt S (2005) Anholt nation brands index: how does the world see America? J Advertising Res 45: 296–304. https://doi.org/10.1017/S0021849905050336 doi: 10.1017/S0021849905050336
![]() |
[10] |
Appio FP, Martini A, Massa S, et al. (2016) Unveiling the intellectual origins of social media-based innovation: insights from a bibliometric approach. Scientometrics 108: 355–388. https://doi.org/10.1007/s11192-016-1955-9 doi: 10.1007/s11192-016-1955-9
![]() |
[11] |
Appio FP, Cesaroni F, Minin AD (2014) Visualizing the structure and bridges of the intellectual property management and strategy literature: A document co-citation analysis. Scientometrics 101: 623–661. https://doi.org/10.1007/s11192-014-1329-0 doi: 10.1007/s11192-014-1329-0
![]() |
[12] |
Aria M, Cuccurullo C (2017) bibliometrix: An R-tool for comprehensive science mapping analysis. J Informetr 11: 959-975. https://doi.org/10.1016/j.joi.2017.08.007 doi: 10.1016/j.joi.2017.08.007
![]() |
[13] |
Aria M, Misuraca M, Spano M (2020) Mapping the evolution of social research and data science on 30 years of Social Indicators Research. Soc Indic Res 149: 803–831. https://doi.org/10.1007/s11205-020-02281-3 doi: 10.1007/s11205-020-02281-3
![]() |
[14] |
Aria M, Cuccurullo C, D'Aniello L, et al. (2022) Thematic Analysis as a New Culturomic Tool: The Social Media Coverage on COVID-19 Pandemic in Italy. Sustainability 14: 3643. https://doi.org/10.3390/su14063643 doi: 10.3390/su14063643
![]() |
[15] | Aronczyk M (2008) Living the brand: Nationality, globality, and the identity strategies of nation branding consultants. Int J Commun 2: 25. https://ijoc.org/index.php/ijoc/article/view/218 |
[16] |
Bartikowski B, Fastoso F, Gierl H (2021) How nationalistic appeals affect foreign luxury brand reputation: A study of ambivalent effects. J Bus Ethics 169: 261–277. https://doi.org/10.1007/s10551-020-04483-8 doi: 10.1007/s10551-020-04483-8
![]() |
[17] |
Batra R (2019) Creating brand meaning: a review and research agenda. J Consum Psychol 29: 535–546. https://doi.org/10.1002/jcpy.1122 doi: 10.1002/jcpy.1122
![]() |
[18] | Belk RW (1984) Three scales to measure constructs related to materialism: Reliability, validity, and relationships to measures of happiness. ACR North Am Adv 1: 1-14. Available from: https://www.jrconsumers.com/academic_articles/issue_1/Belk_.pdf. |
[19] |
Bellostas A, Del Río C, González-Álvarez K (2023) Cultural context, organizational performance and Sustainable Development Goals: A pending task. Green Financ 5: 211–239. https://doi.org/10.3934/GF.2023009 doi: 10.3934/GF.2023009
![]() |
[20] |
Benneworth P, Charles D (2005) University spin-off policies and economic development in less successful regions: Learning from two decades of policy practice. Eur Plan Stud 13: 537–557. https://doi.org/10.1080/09654310500107175 doi: 10.1080/09654310500107175
![]() |
[21] |
Bennink H (2023) Prospects of green financing in democratic societies. Green Financ 5: 154–194. https://doi.org/10.3934/GF.2023007 doi: 10.3934/GF.2023007
![]() |
[22] |
Bilkey WJ, Nes E (1982) Country-of-origin effects on product evaluations. J Int Bus Stud 13: 89–100. https://doi.org/10.1057/palgrave.jibs.8490539 doi: 10.1057/palgrave.jibs.8490539
![]() |
[23] | Blei DM, Ng AY, Jordan MI (2003) Latent dirichlet allocation. J Mach Learn Res 3: 993–1022. Available from: https://www.jmlr.org/papers/volume3/blei03a/blei03a.pdf?ref = https://githubhelp.com. |
[24] | Boell SK, Cecez-Kecmanovic D (2015) On being 'systematic' in literature reviews. Formulating Research Methods for Information Systems, 48–78. Available from: https://link.springer.com/chapter/10.1057/9781137509888_3. |
[25] |
Bulmer S, Buchanan-Oliver M (2010) Experiences of brands and national identity. Australas Mark J 18: 199–205. https://doi.org/10.1016/j.ausmj.2010.07.002 doi: 10.1016/j.ausmj.2010.07.002
![]() |
[26] | Caffrey J, Isaacs HH (1971) Estimating the impact of a college or university on the local economy. EXXON Education Foundation, New York. Available from: https://eric.ed.gov/?id = ED252100. |
[27] |
Carlsen L (2023) The state of the 'Prosperity' pillar by 2022: A partial ordering-based analysis of the sustainable development goals 7–11. Green Financ 5: 89–101. https://doi.org/10.3934/GF.2023005 doi: 10.3934/GF.2023005
![]() |
[28] |
Chabowski BR, Samiee S, Hult GTM (2013) A bibliometric analysis of the global branding literature and a research agenda. J Int Bus Stud 44: 622-634. https://doi.org/10.1057/jibs.2013.20 doi: 10.1057/jibs.2013.20
![]() |
[29] |
Chappin EJL, Ligtvoet A (2014) Transition and transformation: A bibliometric analysis of two scientific networks researching socio-technical change. Renew Sust Energ Rev 30: 715–723. https://doi.org/10.1016/j.rser.2013.11.013 doi: 10.1016/j.rser.2013.11.013
![]() |
[30] |
Clayton R, Pontusson J (1998) Welfare-state retrenchment revisited: entitlement cuts, public sector restructuring, and inegalitarian trends in advanced capitalist societies. World Polit 51: 67–98. https://doi.org/10.1017/S0043887100007796 doi: 10.1017/S0043887100007796
![]() |
[31] |
Clifton N (2014) Towards a holistic understanding of county of origin effects? branding of the region, branding from the region. J Destin Mark Manage 3: 122–132. https://doi.org/10.1016/j.jdmm.2014.02.003 doi: 10.1016/j.jdmm.2014.02.003
![]() |
[32] |
Cohen J (1992) Statistical power analysis. Curr Dir Psychol Sci 1: 98–101. https://doi.org/10.1111/1467-8721.ep10768783 doi: 10.1111/1467-8721.ep10768783
![]() |
[33] | Connor W (1978) A nation is a nation, is a state, is an ethnic group is a... Ethnic Racial Stud 1: 377–400. https://doi.org/10.1080/01419870.1978.9993240 |
[34] |
Cobo MJ, Lopez-Herrera AG, Herrera-Viedma E, et al. (2011) An approach for detecting, quantifying, and visualizing the evolution of a research field: A practical application to the fuzzy sets theory field. J Informetr 5: 146-166. https://doi.org/10.1016/j.joi.2010.10.002 doi: 10.1016/j.joi.2010.10.002
![]() |
[35] |
D'Angelo CA, Giuffrida C, Abramo G (2011) A heuristic approach to author name disambiguation in bibliometrics databases for large-scale research assessments. J Am Soc Inf Sci Tec 62: 257–269. https://doi.org/10.1002/asi.21460 doi: 10.1002/asi.21460
![]() |
[36] |
De Nooy W (2011) Networks of action and events over time: a multilevel discrete-time event history model for longitudinal network data. Soc Networks 33: 31–40. https://doi.org/10.1016/j.socnet.2010.09.003 doi: 10.1016/j.socnet.2010.09.003
![]() |
[37] |
Demangeot C, Broderick AJ, Craig CS (2015) Multicultural marketplaces: New territory for international marketing and consumer research. Int Market Rev 32: 118—140. https://doi.org/10.1108/IMR-01-2015-0017 doi: 10.1108/IMR-01-2015-0017
![]() |
[38] |
Demir G, Chatterjee P, Pamucar D (2024) Sensitivity analysis in multi-criteria decision making: A state-of-the-art research perspective using bibliometric analysis. Expert Syst Appl 237: 121660. https://doi.org/10.1016/j.eswa.2023.121660 doi: 10.1016/j.eswa.2023.121660
![]() |
[39] |
Demir G, Chatterjee P, Zakeri S, et al. (2024) Mapping the Evolution of Multi-Attributive Border Approximation Area Comparison Method: A Bibliometric Analysis. Decis Making Appl Manag Eng 7: 290-314. https://doi.org/10.31181/dmame7120241037 doi: 10.31181/dmame7120241037
![]() |
[40] |
Desalegn G (2023) Insuring a greener future: How green insurance drives investment in sustainable projects in developing countries? Green Financ 5: 195–210. https://doi.org/10.3934/GF.2023008 doi: 10.3934/GF.2023008
![]() |
[41] |
Dinnie K (2004a) Place branding: Overview of an emerging literature. Place Branding 1: 106–110. https://doi.org/10.1057/palgrave.pb.5990010 doi: 10.1057/palgrave.pb.5990010
![]() |
[42] |
Dinnie K (2004b) Country-of-origin 1965-2004: A literature review. J Customer Behav 3: 165–213. https://doi.org/10.1362/1475392041829537 doi: 10.1362/1475392041829537
![]() |
[43] |
Dinnie K, Melewar TC, Seidenfuss KU, et al. (2010) Nation branding and integrated marketing communications: an ASEAN perspective. Int Market Rev 27: 388–403. https://doi.org/10.1108/02651331011058572 doi: 10.1108/02651331011058572
![]() |
[44] |
Donato H, Donato M (2019) Etapas na condução de uma revisão sistemática. Acta Médica Port 32: 227–235. https://doi.org/10.20344/amp.11923 doi: 10.20344/amp.11923
![]() |
[45] |
Druckman D (1994) Nationalism, patriotism, and group loyalty: A social psychological perspective. Mershon Int Stud Rev 38: 43–68. https://doi.org/10.2307/222610 doi: 10.2307/222610
![]() |
[46] |
Ductor L (2015) Does co‐authorship lead to higher academic productivity?. Oxf B Econ Stat 77: 385–407. https://doi.org/10.1111/obes.12070 doi: 10.1111/obes.12070
![]() |
[47] | Dwivedi Y, Williams MD, Lal B, et al. (2008) Profiling adoption, acceptance and diffusion research in the information systems discipline. ECIS 2008 Proceedings, 112. Available from: http://aisel.aisnet.org/ecis2008/112. |
[48] |
Dzhengiz T (2020) Bridging the gap: organisational value frames and sustainable alliance portfolios. Dzhengiz, T. Bridging the Gap: Organisational Value Frames and Sustainable Alliance Portfolios. Green Financ 2: 323–343. https://doi.org/10.3934/GF.2020018 doi: 10.3934/GF.2020018
![]() |
[49] | Everett DH, Whitton WI (1952) A general approach to hysteresis. Trans Faraday Soc 48: 749–757. Available from: https://pubs.rsc.org/en/content/articlelanding/1952/tf/tf9524800749/unauth. |
[50] |
Ezuma REMR, Matthew NK (2022) The perspectives of stakeholders on the effectiveness of green financing schemes in Malaysia. Green Financ 4: 450–473. https://doi.org/10.3934/GF.2022022 doi: 10.3934/GF.2022022
![]() |
[51] |
Failler P, Li Z (2019) Welcome to Green Finance: A new open access journal for sustainable research professionals. Green Financ 1: 1–3. https://doi.org/10.3934/GF.2019.1.1 doi: 10.3934/GF.2019.1.1
![]() |
[52] |
Falagas ME, Pitsouni EI, Malietzis GA, et al. (2008) Comparison of Pubmed, Scopus, Web of Science, and Google Scholar: strengths and weaknesses. FASEB J 22: 338–342. https://doi.org/10.1096/fj.07-9492LSF doi: 10.1096/fj.07-9492LSF
![]() |
[53] |
Fan Y (2010) Branding the nation: Towards a better understanding. Place Branding Publi 6: 97–103. https://doi.org/10.1057/pb.2010.16 doi: 10.1057/pb.2010.16
![]() |
[54] |
Fetscherin M (2010) The determinants and measurement of a country brand: the country brand strength index. Int Market Rev 27: 466–479. https://doi.org/10.1108/02651331011058617 doi: 10.1108/02651331011058617
![]() |
[55] |
Foscht T, Maloles C, Swoboda B, et al. (2008) The impact of culture on brand perceptions: a six-nation study. J Prod Brand Manag 17: 131–142. https://doi.org/10.1108/10610420810875052 doi: 10.1108/10610420810875052
![]() |
[56] | Frankenberger-Graham KD, Smith MC, Kahle LR, et al. (2015) Exploring the relations between consumer perceptions of marketing, materialism and life satisfaction. Proceedings of the 1993 Academy of Marketing Science (AMS) Annual Conference Springer, 15–19. Available from: https://link.springer.com/chapter/10.1007/978-3-319-13159-7_4. |
[57] |
Freeman LC (1977) A set of measures of centrality based on betweenness. Sociometry 40: 35–41. https://doi.org/10.2307/3033543 doi: 10.2307/3033543
![]() |
[58] | Friedman M, Friedman R (1980) Free to Choose: A Personal Statement. London, Secker and Warburg. Available from: https://www.proglocode.unam.mx/sites/proglocode.unam.mx/files/docencia/Milton%20y%20Rose%20Friedman%20-%20Free%20to%20Choose.pdf. |
[59] |
Gaggiotti H, Cheng P, Yunak O (2008) City brand managemen (CBM): The case of Kazakhstan. Place Branding Publi 4: 115–123. https://doi.org/10.1057/palgrave.pb.6000081 doi: 10.1057/palgrave.pb.6000081
![]() |
[60] |
Gan C, Wang W (2015) Research characteristics and status on social media in China: A bibliometric and co-word analysis. Scientometrics 105: 1167–1182. https://doi.org/10.1007/s11192-015-1723-2 doi: 10.1007/s11192-015-1723-2
![]() |
[61] |
Geary D (2013) Incredible India in a global age: The cultural politics of image branding in tourism. Tourist Stud 13: 36–61. https://doi.org/10.1177/1468797612471105 doi: 10.1177/1468797612471105
![]() |
[62] | Gellner E (1994) Encounters with nationalism. Philos Quart 47. Available from: https://www.wiley.com/en-cn/Encounters+with+Nationalism-p-9780631194811. |
[63] |
Gielens K, Steenkamp J-BEM (2019) Branding in the era of digital (dis) intermediation. Int J Res Mark 36: 367–384. https://doi.org/10.1016/j.ijresmar.2019.01.005 doi: 10.1016/j.ijresmar.2019.01.005
![]() |
[64] |
Gong W, Stump RL, Li ZG (2014) Global use and access of social networking web sites: a national culture perspective. J Res Interact Mark 8: 37–55. https://doi.org/10.1108/JRIM-09-2013-0064 doi: 10.1108/JRIM-09-2013-0064
![]() |
[65] |
Gorelick J, Walmsley N (2020) The greening of municipal infrastructure investments: Technical assistance, instruments, and city champions. Green Financ 2: 114–134. https://doi.org/10.3934/GF.2020007 doi: 10.3934/GF.2020007
![]() |
[66] |
Gössling S, Humpe A (2020) The global scale, distribution and growth of aviation: Implications for climate change. Global Environ Chang 65: 102194. https://doi.org/10.1016/j.gloenvcha.2020.102194 doi: 10.1016/j.gloenvcha.2020.102194
![]() |
[67] |
Gould M, Skinner H (2007) Branding on ambiguity? place branding without a national identity: Marketing northern Ireland as a post-conflict society in the USA. Place Branding Publi 3: 100–113. https://doi.org/10.1057/palgrave.pb.6000051 doi: 10.1057/palgrave.pb.6000051
![]() |
[68] |
Griffiths TL, Steyvers M (2004) Finding scientific topics. P Natl Acad Sci 101: 5228–5235. https://doi.org/10.1073/pnas.030775210 doi: 10.1073/pnas.030775210
![]() |
[69] |
Grimson A (2010) Culture and identity: two different notions. Soc Ident 16: 61–77. https://doi.org/10.1080/13504630903465894 doi: 10.1080/13504630903465894
![]() |
[70] |
Gupta N (2011) Globalization does lead to change in consumer behavior: An empirical evidence of impact of globalization on changing materialistic values in Indian consumers and its aftereffects. Asia Pac J Market Lo 23: 251–269. https://doi.org/10.1108/13555851111143204 doi: 10.1108/13555851111143204
![]() |
[71] | Guzman F (2005). A brand building literature review. ICFAI J Brand Manag 2: 30–48. https://pt.scribd.com/document/137034169/A-Brand-Building-Literature-Review. |
[72] |
Haas R, Ajanovic A, Ramsebner J, et al. (2021) Financing the future infrastructure of sustainable energy systems. Green Financ 3: 90–118. https://doi.org/10.3934/GF.2021006 doi: 10.3934/GF.2021006
![]() |
[73] | Hahn ED, Soyer R (2005) Probit and logit models: Differences in the multivariate realm. J R Stat Soc Series B: 1–12. Available from: https://citeseerx.ist.psu.edu/document?repid = rep1 & type = pdf & doi = c45e142a45851c8b4da074ac38fd56bb5ff78749. |
[74] |
Hao A W, Paul J, Trott S, Guo C, Wu HH (2021). Two decades of research on nation branding: A review and future research agenda. Int Market Rev 38: 46–69. https://doi.org/10.1108/IMR-01-2019-0028 doi: 10.1108/IMR-01-2019-0028
![]() |
[75] |
Hanson K, O'Dwyer E (2019) Patriotism and nationalism, left and right: A methodology study of American national identity. Polit Psychol 40: 777–795. https://doi.org/10.1111/pops.12561 doi: 10.1111/pops.12561
![]() |
[76] |
He J, Wang CL, Wu Y (2021) Building the connection between nation and commercial brand: an integrative review and future research directions. Int Market Rev 38: 19–35. https://doi.org/10.1108/IMR-11-2019-0268 doi: 10.1108/IMR-11-2019-0268
![]() |
[77] |
Hewett K, Hult GTM, Mantrala MK, Nim N, Pedada K (2022) Cross-border marketing ecosystem orchestration: A conceptualization of its determinants and boundary conditions. Int J Res Mark 39: 619–638. https://doi.org/10.1016/j.ijresmar.2021.09.003 doi: 10.1016/j.ijresmar.2021.09.003
![]() |
[78] |
Hicks D (1999) The difficulty of achieving full coverage of international social science literature and the bibliometric consequences. Scientometrics 44: 193–215. https://doi.org/10.1007/BF02457380 doi: 10.1007/BF02457380
![]() |
[79] |
Hill MO (1973) Diversity and evenness: a unifying notation and its consequences. Ecology 54: 427–432. https://doi.org/10.2307/1934352 doi: 10.2307/1934352
![]() |
[80] |
Hong ST, Wyer Jr RS (1989) Effects of country-of-origin and product-attribute information on product evaluation: An information processing perspective. J Consum Res16: 175–187. https://doi.org/10.1086/209206 doi: 10.1086/209206
![]() |
[81] |
Hsieh MH, Lindridge A (2005) Universal appeals with local specifications. J Prod Brand Manag 14: 14–28. https://doi.org/10.1108/10610420510583716 doi: 10.1108/10610420510583716
![]() |
[82] |
Huang Y, Yu K, Huang C (2023) Green finance engagement: An empirical study of listed companies on Chinese main board. Green Financ 5: 1–17. https://doi.org/10.3934/GF.2023001 doi: 10.3934/GF.2023001
![]() |
[83] | Hutcheson K (1970) A test for comparing diversities based on the Shannon formula. J Theor Biol 29: 151–154. Available from: https://cir.nii.ac.jp/crid/1571135650903988352. |
[84] |
Ilić B, Stojanovic D, Djukic G (2019) Green economy: mobilization of international capital for financing projects of renewable energy sources. Green Financ 1: 94–109. https://doi.org/10.3934/GF.2019.2.94 doi: 10.3934/GF.2019.2.94
![]() |
[85] |
Johansson JK, Douglas SP, Nonaka I (1985) Assessing the impact of country of origin on product evaluations: a new methodological perspective. J Marketing Res 22: 388–396. https://doi.org/10.1177/002224378502200404 doi: 10.1177/002224378502200404
![]() |
[86] |
Kalamova MM, Konrad KA (2010) Nation brands and foreign direct investment. Kyklos 63: 400–431. https://doi.org/10.1111/j.1467-6435.2010.00480.x doi: 10.1111/j.1467-6435.2010.00480.x
![]() |
[87] |
Karrh JA (1998) Brand placement: A review. J Curr Iss Res Ad 20: 31–49. https://doi.org/10.1080/10641734.1998.10505081 doi: 10.1080/10641734.1998.10505081
![]() |
[88] |
Kaufmann HR, Durst S (2008) Developing inter-regional brands. Euromed J Bus 3: 38–62. https://doi.org/10.1108/14502190810873812 doi: 10.1108/14502190810873812
![]() |
[89] |
Keillor BD, Hult TGM (1999) A five-country study of national identity: Implications for international marketing research and practice. Int Market Rev 16: 65–84. https://doi.org/10.1108/02651339910257656 doi: 10.1108/02651339910257656
![]() |
[90] |
Kemfert C, Schmalz S (2019) Sustainable finance: Political challenges of development and implementation of framework conditions. Green Financ 1: 237–248. https://doi.org/10.3934/GF.2019.3.237 doi: 10.3934/GF.2019.3.237
![]() |
[91] |
Khasseh AA, Soheili F, Moghaddam HS, et al. (2017) Intellectual structure of knowledge in metrics: A co-word analysis. Inform Process Manag 53: 705–720. https://doi.org/10.1016/j.ipm.2017.02.001 doi: 10.1016/j.ipm.2017.02.001
![]() |
[92] |
Kirloskar-Steinbach M (2019) Diversifying philosophy: The art of non-domination. Educ Philos Theory 51: 1490–1503. https://doi.org/10.1080/00131857.2018.1562850 doi: 10.1080/00131857.2018.1562850
![]() |
[93] | Klein LR (1978) The supply side. Am Econ Rev 68: 1–7. https://www.jstor.org/stable/1809682 |
[94] |
Klein JG, Ettensoe R (1999) Consumer animosity and consumer ethnocentrism: An analysis of unique antecedents. J Int Consum Mark 11: 5–24. https://doi.org/10.1300/J046v11n04_02 doi: 10.1300/J046v11n04_02
![]() |
[95] |
Kosterman R, Feshbach S (1989) Toward a measure of patriotic and nationalistic attitudes. Polit Psychol 10: 257–274. https://doi.org/10.2307/3791647 doi: 10.2307/3791647
![]() |
[96] |
Kotler P, Gertner D (2002) Country as brand, product, and beyond: A place marketing and brand management perspective. J Brand Manag 9: 249–261. https://doi.org/10.1057/palgrave.bm.2540076 doi: 10.1057/palgrave.bm.2540076
![]() |
[97] | Lantz G, Loeb S (1998) An examination of the community identity and purchase preferences using the social identity approach. ACR North Am Adv 25: 486–491.Available from: https://www.acrwebsite.org/volumes/8199/volumes/v25/NA-25. |
[98] |
Lewison G (1998) New bibliometric techniques for the evaluation of medical schools. Scientometrics 41: 5–16. https://doi.org/10.1007/bf02457962 doi: 10.1007/bf02457962
![]() |
[99] |
Li WK, Wyer Jr RS (1994) The role of country of origin in product evaluations: Informational and standard-of-comparison effects. J Consum Psychol 3: 187–212. https://doi.org/10.1016/S1057-7408(08)80004-6 doi: 10.1016/S1057-7408(08)80004-6
![]() |
[100] |
Loo T, Davies G (2006) Branding China: The ultimate challenge in reputation management? Corp Reput Rev 9: 198–210. https://doi.org/10.1057/palgrave.crr.1550025 doi: 10.1057/palgrave.crr.1550025
![]() |
[101] |
Maciel FA, Rocha A, Silva JF (2013) Brand personality of global quick-service restaurants in emerging and developed markets: A comparative study in Brazil and the US. Latin Am Bus Rev 14: 139–161. https://doi.org/10.1080/10978526.2013.808950 doi: 10.1080/10978526.2013.808950
![]() |
[102] | Magurran AE (1988) Why diversity? Ecological Diversity and its Measurement Princeton University Press. Available from: https://link.springer.com/book/10.1007/978-94-015-7358-0. |
[103] | Magurran AE, McGill BJ (2011) Biological diversity: frontiers in measurement and assessment Oxford University Press. Available from: https://www.journals.uchicago.edu/doi/abs/10.1086/666756. |
[104] |
Maheswaran D (1994) Country of origin as a stereotype: Effects of consumer expertise and attribute strength on product evaluations. J Consum Res 21: 354–365. https://doi.org/10.1086/209403 doi: 10.1086/209403
![]() |
[105] | Margalef R (1972) Homage to Evelyn Hutchinson, or why there is an upper limit to diversity, Connecticut Academy of Arts and Sciences. Available from: https://digital.csic.es/handle/10261/166281. |
[106] | Marino-Romero JA, Palos-Sanchez PR, Velicia-Martin FA (2023) Evolution of digital transformation in SMEs management through a bibliometric analysis. Technol Forecast Soc 199: 123014. http://doi.org/10.1016/j.techfore.2023.123014 |
[107] |
Martínez NM (2016) Towards a network place branding through multiple stakeholders and based on cultural identities. J Place Manag Dev 9: 73–90. https://doi.org/10.1108/JPMD-11-2015-0052 doi: 10.1108/JPMD-11-2015-0052
![]() |
[108] |
Matzler K, Strobl A, Stokburger-Sauer N, et al. (2016) Brand personality and culture: The role of cultural differences on the impact of brand personality perceptions on tourists' visit intentions. Tourism Manage 52: 507–520. https://doi.org/10.1016/j.tourman.2015.07.017 doi: 10.1016/j.tourman.2015.07.017
![]() |
[109] | May RM (1979) Patterns of species abundance and diversity. Ecol Evol Communities 1: 81–120. https://cir.nii.ac.jp/crid/1573668924704110208 |
[110] |
McFadden D (1974) The measurement of urban travel demand. J Public Econ 3: 303–328. https://doi.org/10.1016/0047-2727(74)90003-6 doi: 10.1016/0047-2727(74)90003-6
![]() |
[111] | McFadden D, Tye WB, Train K (1977) An application of diagnostic tests for the independence from irrelevant alternatives property of the multinomial logit model. Institute of Transportation Studies, University of California Berkeley, CA. Available from: https://onlinepubs.trb.org/Onlinepubs/trr/1977/637/637-007.pdf. |
[112] | Mohan K, Ahlemann F (2011) What methodology attributes are critical for potential users? understanding the effect of human needs. In: Mouratidis, H., Rolland, C. (eds) Advanced Information Systems Engineering. CAiSE 2011. Lecture Notes in Computer Science, 6741. Springer, Berlin, Heidelberg. https://doi.org/10.1007/978-3-642-21640-4_24 |
[113] |
Mooij M (2003) Convergence and divergence in consumer behaviour: implications for global advertising. Int J Advert 22: 183–202. https://doi.org/10.1080/02650487.2003.11072848 doi: 10.1080/02650487.2003.11072848
![]() |
[114] |
Moura J, Soares I (2023) Financing low-carbon hydrogen: The role of public policies and strategies in the EU, UK and USA. Green Financ 5: 265–297. https://doi.org/10.3934/GF.2023011 doi: 10.3934/GF.2023011
![]() |
[115] | Nielsen K (1999) Cosmopolitan nationalism. Monist 82: 446–468. https://www.jstor.org/stable/27903647 |
[116] |
Norris M, Oppenheim C (2007) Comparing alternatives to the web of science for coverage of the social sciences' literature. J Informetr 1: 161–169. https://doi.org/10.1016/j.joi.2006.12.001 doi: 10.1016/j.joi.2006.12.001
![]() |
[117] |
Osareh F (1996) Bibliometrics, citation analysis and co-citation analysis: A review of literature. Libri 46: 149–158. https://doi.org/10.1515/libr.1996.46.3.149 doi: 10.1515/libr.1996.46.3.149
![]() |
[118] |
Ouellet JF (2007) Consumer racism and its effects on domestic cross-ethnic product purchase: An empirical test in the United States, Canada, and France. J Marketing 71: 113–128. https://doi.org/10.1509/jmkg.71.1.113 doi: 10.1509/jmkg.71.1.113
![]() |
[119] |
Özsomer A (2012) The interplay between global and local brands: A closer look at perceived brand globalness and local iconness. J Int Marketing 20: 72–95. https://doi.org/10.1509/jim.11.0105 doi: 10.1509/jim.11.0105
![]() |
[120] |
Özsomer A, Batra R, Chattopadhyay A, et al. (2012) A global brand management roadmap. Int J Res Mark 29: 1–4. https://doi.org/10.1016/j.ijresmar.2012.01.001 doi: 10.1016/j.ijresmar.2012.01.001
![]() |
[121] |
Papadopoulos N (2004) Place branding: Evolution, meaning and implications. Place Branding 1: 36–49. https://doi.org/10.1057/palgrave.pb.5990003 doi: 10.1057/palgrave.pb.5990003
![]() |
[122] |
Papadopoulos N, Hamzaoui-Essoussi L, El Banna A (2016) Nation branding for foreign direct investment: an Integrative review and directions for research and strategy. J Prod Brand Manag 25: 615–628. https://doi.org/10.1108/JPBM-09-2016-1320 doi: 10.1108/JPBM-09-2016-1320
![]() |
[123] |
Papadopoulos N, Cleveland M, Bartikowski B, et al. (2018) Of countries, places and product/brand place associations: an inventory of dispositions and issues relating to place image and its effects. J Prod Brand Manag 27: 735–753. https://doi.org/10.1108/JPBM-09-2018-2035 doi: 10.1108/JPBM-09-2018-2035
![]() |
[124] |
Pato ML, Teixeira AAC (2016) Twenty years of rural entrepreneurship: A bibliometric survey. Sociol Ruralis 56: 3–28. https://doi.org/10.1111/soru.12058 doi: 10.1111/soru.12058
![]() |
[125] |
Pearson D, Pearson T (2017) Branding food culture: UNESCO creative cities of gastronomy. J Food Prod Mark 23: 342–355. https://doi.org/10.1080/10454446.2014.1000441 doi: 10.1080/10454446.2014.1000441
![]() |
[126] | Perez ME, Padgett D, Burgers W (2011) Intergenerational influence on brand preferences. J Prod Brand Manag 20: 5–13. https://doi.org/10.1108/10610421111107978 |
[127] |
Peterson RA, Jolibert AJP (1995) A meta-analysis of country-of-origin effects. J Int Bus Stud 26: 883–900. https://doi.org/10.1057/palgrave.jibs.8490824 doi: 10.1057/palgrave.jibs.8490824
![]() |
[128] | Pielou EC (1969) An introduction to mathematical ecology. Queen's University, Kigston, Ontario, Canada. Available from: https://www.cabdirect.org/cabdirect/abstract/19729701428. |
[129] |
Ribeiro HCM, Cirani CBS (2013) Análise da produção científica da Revista de Administração e Inovação. Revista De Administração e Inovação 10: 208–228. https://doi.org/10.5773/rai.v10i4.1139 doi: 10.5773/rai.v10i4.1139
![]() |
[130] |
Ribeiro VM, Correia‐da‐Silva J, Resende J (2016) Nesting vertical and horizontal differentiation in two‐sided markets. B Econ Res 68: 133–145. https://doi.org/10.1111/boer.12084 doi: 10.1111/boer.12084
![]() |
[131] |
Rojas-Méndez J (2016) The nation brand molecule. J Prod Brand Manag 131: 891–941. https://doi.org/10.1093/qje/qjw005 doi: 10.1093/qje/qjw005
![]() |
[132] |
Rojas-Méndez J, Khoshnevis M (2023) Conceptualizing nation branding: the systematic literature review. J Prod Brand Manag 32: 107–123. https://doi.org/10.1108/JPBM-04-2021-3444 doi: 10.1108/JPBM-04-2021-3444
![]() |
[133] | Roztocki N, Weistroffer HR (2008) Event studies in information systems research: a review, Proceedings of the Fourteenth Americas Conference on Information Systems 1: 1–11. Available from: https://papers.ssrn.com/sol3/papers.cfm?abstract_id = 1235562. |
[134] |
Samiee S (1994) Customer evaluation of products in a global market. J Int Bus Stud 25: 579–604. https://doi.org/10.1057/palgrave.jibs.8490213 doi: 10.1057/palgrave.jibs.8490213
![]() |
[135] |
Schwarz C (2018) ldagibbs: A command for topic modeling in stata using latent dirichlet allocation. Stata J 18: 101–117. https://doi.org/10.1177/1536867X1801800107 doi: 10.1177/1536867X1801800107
![]() |
[136] | Seo J (2008) The politics of historiography in China: Contextualizing the Koguryo controversy. Asian Perspect 32: 39–58. https://www.jstor.org/stable/42704640 |
[137] |
Seo J (2021) Diagnosing Korea–Japan relations through thick description: Revisiting the national identity formation process. Third World Q 1: 1–16. https://doi.org/10.1080/01436597.2021.1937098 doi: 10.1080/01436597.2021.1937098
![]() |
[138] |
Shimp TA, Sharma S (1987) Consumer ethnocentrism: Construction and validation of the cetscale. J Marketing Res 24: 280–289. https://doi.org/10.1177/002224378702400304 doi: 10.1177/002224378702400304
![]() |
[139] |
Shugurov M (2023) International cooperation on climate research and green technologies in the face of sanctions: The case of Russia. Green Financ 5: 102–153. https://doi.org/10.3934/GF.2023006 doi: 10.3934/GF.2023006
![]() |
[140] |
Silva ST, Teixeira AAC (2009) On the divergence of evolutionary research paths in the past 50 years: a comprehensive bibliometric account. J Evol Econ 19: 605–642. https://doi.org/10.1007/s00191-008-0121-9 doi: 10.1007/s00191-008-0121-9
![]() |
[141] |
Smith PM, West CD (1994) The globalization of furniture industries/markets. J Glob Mark 7: 103–132. https://doi.org/10.1300/J042v07n03_06 doi: 10.1300/J042v07n03_06
![]() |
[142] |
Södergren J (2021) Brand authenticity: 25 Years of research. Int J Consum Stud 45: 645–663. https://doi.org/10.1111/ijcs.12651 doi: 10.1111/ijcs.12651
![]() |
[143] |
Song YA, Sung Y (2013) Antecedents of nation brand personality. Corp Reput Rev 16: 80–94. https://doi.org/10.1057/crr.2012.24 doi: 10.1057/crr.2012.24
![]() |
[144] | Stalnaker S (2002) Hub culture: The next wave of urban consumers Wiley. Available from: https://cir.nii.ac.jp/crid/1130282269900840704. |
[145] |
Steenkamp J-BEM (2019) Building strong nation brands. Int Market Rev 38: 6–18. https://doi.org/10.1108/IMR-10-2019-0253 doi: 10.1108/IMR-10-2019-0253
![]() |
[146] |
Steenkamp J-BEM, Hofstede FT, Wedel M (1999) A cross-national investigation into the individual and national cultural antecedents of consumer innovativeness. J Marketing 63: 55–69. ttps://doi.org/10.1177/002224299906300204 doi: 10.1177/002224299906300204
![]() |
[147] |
Steenkamp J-BEM, Heerde HJ, Geyskens I (2010) What makes consumers willing to pay a price premium for national brands over private labels? J Marketing Res 47: 1011–1024. https://doi.org/10.1509/jmkr.47.6.1011 doi: 10.1509/jmkr.47.6.1011
![]() |
[148] | Tajfel H, Turner J (1979) An integrative theory of intergroup conflict, Oxford Management Readers. Available from: https://psycnet.apa.org/record/2010-11535-008. |
[149] |
Teixeira AAC, Vieira PC, Abreu AP (2017) Sleeping beauties and their princes in innovation studies. Scientometrics 110: 541–580. https://doi.org/10.1007/s11192-016-2186-9 doi: 10.1007/s11192-016-2186-9
![]() |
[150] |
Therkelsen A, Gram M (2010) Branding Europe–between nations, regions and continents. Scand J Hosp Tour 10: 107–128. https://doi.org/10.1080/15022250903561903 doi: 10.1080/15022250903561903
![]() |
[151] | Tuan YF (1990) Topophilia: A study of environmental perception, attitudes, and values Columbia University Press. Available from: https://www.harvard.com/book/topophilia_a_study_of_environmental_perception_attitudes_and_values/. |
[152] | Turner G (1994a) Making it national: Australian nationalism and popular culture. https://doi.org/10.4324/9781003116363 |
[153] | Turner G (1994b) Whatever happened to national identity?: Film and the nation in the 1990s. Metro Magazine: Media and Education Magazine 1: 32–35. Available from: https://search.informit.org/doi/abs/10.3316/ielapa.153836366722575. |
[154] |
Eck NJV, Waltman L (2009) How to normalize cooccurrence data? an analysis of some well-known similarity measures. J Am Soc Inf Sci Tec 60: 1635–1651. https://doi.org/10.1002/asi.21075 doi: 10.1002/asi.21075
![]() |
[155] |
Eck NJV, Waltman L (2010) Software survey: VosViewer, a computer program for bibliometric mapping. Scientometrics 84: 523–538. https://doi.org/10.1007/s11192-009-0146-3 doi: 10.1007/s11192-009-0146-3
![]() |
[156] | Eck NJV, Waltman L (2011) Text mining and visualization using VosViewer. arXiv preprint arXiv. https://arXiv.org/abs/1109.2058 |
[157] |
Verlegh PWJ, Steenkamp J-BEM (1999) A review and meta-analysis of country-of-origin research. J Econ Psychol 20: 521–546. https://doi.org/10.1016/S0167-4870(99)00023-9 doi: 10.1016/S0167-4870(99)00023-9
![]() |
[158] | Volcic Z (2009) Television in the Balkans: The rise of commercial nationalism. Television studies after TV Routledge. Available from: https://research.monash.edu/en/publications/volcic-z-television-in-the-balkans-the-rise-of-commercial-nationa. |
[159] | Volcic Z, Andrejevic M (2011) Nation branding in the era of commercial nationalism. Int J Commun 5: 21. https://ijoc.org/index.php/ijoc/article/view/849 |
[160] |
Wakkee I, Sijde PV, Vaupell C, et al. (2019) The university's role in sustainable development: Activating entrepreneurial scholars as agents of change. Technol Forecast Soc 141: 195–205. https://doi.org/10.1016/j.techfore.2018.10.013 doi: 10.1016/j.techfore.2018.10.013
![]() |
[161] |
Wallström A, Steyn P, Pitt L (2010) Expressing herself through brands: a comparative study of women in six Asia-Pacific nations. J Brand Manag 18: 228–237. https://doi.org/10.1057/bm.2010.45 doi: 10.1057/bm.2010.45
![]() |
[162] |
Waltman L, Eck NJV, Noyons ECM (2010) A unified approach to mapping and clustering of bibliometric networks. J Informetr 4: 629–635. https://doi.org/10.1016/j.joi.2010.07.002 doi: 10.1016/j.joi.2010.07.002
![]() |
[163] |
Wang Y, Liu J, Yang X, et al. (2023) The mechanism of green finance's impact on enterprises' sustainable green innovation. Green Financ 5: 452–478. https://doi.org/10.3934/GF.2023018 doi: 10.3934/GF.2023018
![]() |
[164] |
White L (2009) The man from Snowy River: Australia's bush legend and commercial nationalism. Tour Rev Int 13: 139–146. https://doi.org/10.3727/154427209789604633 doi: 10.3727/154427209789604633
![]() |
[165] |
White GO, Guldiken O, Hemphill TA, et al. (2016) Trends in international strategic management research from 2000 to 2013: Text mining and bibliometric analyses. Manage Int Rev 56: 35–65. https://doi.org/10.1007/s11575-015-0260-9 doi: 10.1007/s11575-015-0260-9
![]() |
[166] |
Wilson JAJ, Hollensen S (2010) Saipa Group, Iran–using strategic brand extensions to build relationships. J Islamic Mark 1: 177–188. https://doi.org/10.1108/17590831011055905 doi: 10.1108/17590831011055905
![]() |
[167] |
Winit W, Gregory G, Cleveland M, et al. (2014) Global vs local brands: how home country bias and price differences impact brand evaluations. Int Market Rev 31: 102–128. https://doi.org/10.1108/IMR-01-2012-0001 doi: 10.1108/IMR-01-2012-0001
![]() |
[168] | Wodak R, Cillia R, Reisigl M (1999) The discursive construction of national identities. Discourse Soc 10: 149–173. https://eprints.lancs.ac.uk/id/eprint/1726/ |
[169] |
Wolfswinkel JF, Furtmueller E, Wilderom CP (2013) Using grounded theory as a method for rigorously reviewing literature. Eur J Inform Syst 22: 45–55. https://doi.org/10.1057/ejis.2011.51 doi: 10.1057/ejis.2011.51
![]() |
[170] |
Zong QJ, Shen HZ, Yuan QJ, et al. (2013) Doctoral dissertations of library and information science in China: A co-word analysis. Scientometrics 94: 781–799. https://doi.org/10.1007/s11192-012-0799-1 doi: 10.1007/s11192-012-0799-1
![]() |
Type | Scale | Profile size (mm) | Slot size (mm) | Contact area (mm2) | Gear height (mm) | Gear angle (°) |
YT1 | 1 | 15.12*5.0 | 12.60*2.40 | 45.76 | 0.2 | 90 |
YT2 | 0.8 | 12.16*4.0 | 10.08*1.92 | 29.29 | ||
YT3 | 0.6 | 9.12*3.0 | 7.56*1.44 | 16.47 |