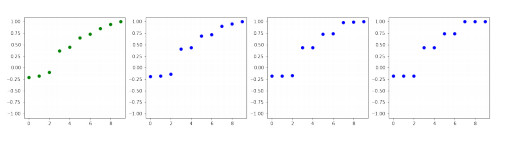
Initial distribution and opinions' evolution of system with (6) at steps 10, 30 and 70;
In this paper, we study a model of opinion dynamics based on the so-called "bounded confidence" principle introduced by Hegselmann and Krause. Following this principle, voters participating in an electoral decision with two options are influenced by individuals sharing an opinion similar to their own.
We consider a modification of this model where the operator generating the dynamical system which describes the process of formation the final distribution of opinions in the society is defined in two steps. First, to the opinion of an agent, a value proportional to opinions in his/her "influence group" is added, and then the elements of the resulting array are divided by the maximal absolute value of elements to keep the opinions in the prescribed interval. We show that under appropriate conditions, any trajectory tends to a fixed point, and all the remaining fixed points are Lyapunov stable.
Citation: Sergei Yu. Pilyugin, Maria S. Tarasova, Aleksandr S. Tarasov, Grigorii V. Monakov. A model of voting dynamics under bounded confidence with nonstandard norming[J]. Networks and Heterogeneous Media, 2022, 17(6): 917-931. doi: 10.3934/nhm.2022032
[1] | Sergei Yu. Pilyugin, M. C. Campi . Opinion formation in voting processes under bounded confidence. Networks and Heterogeneous Media, 2019, 14(3): 617-632. doi: 10.3934/nhm.2019024 |
[2] | Rainer Hegselmann, Ulrich Krause . Opinion dynamics under the influence of radical groups, charismatic leaders, and other constant signals: A simple unifying model. Networks and Heterogeneous Media, 2015, 10(3): 477-509. doi: 10.3934/nhm.2015.10.477 |
[3] | GuanLin Li, Sebastien Motsch, Dylan Weber . Bounded confidence dynamics and graph control: Enforcing consensus. Networks and Heterogeneous Media, 2020, 15(3): 489-517. doi: 10.3934/nhm.2020028 |
[4] | Michael Herty, Lorenzo Pareschi, Giuseppe Visconti . Mean field models for large data–clustering problems. Networks and Heterogeneous Media, 2020, 15(3): 463-487. doi: 10.3934/nhm.2020027 |
[5] | Clinton Innes, Razvan C. Fetecau, Ralf W. Wittenberg . Modelling heterogeneity and an open-mindedness social norm in opinion dynamics. Networks and Heterogeneous Media, 2017, 12(1): 59-92. doi: 10.3934/nhm.2017003 |
[6] | Sharayu Moharir, Ananya S. Omanwar, Neeraja Sahasrabudhe . Diffusion of binary opinions in a growing population with heterogeneous behaviour and external influence. Networks and Heterogeneous Media, 2023, 18(3): 1288-1312. doi: 10.3934/nhm.2023056 |
[7] | Juan Manuel Pastor, Javier García-Algarra, Javier Galeano, José María Iriondo, José J. Ramasco . A simple and bounded model of population dynamics for mutualistic networks. Networks and Heterogeneous Media, 2015, 10(1): 53-70. doi: 10.3934/nhm.2015.10.53 |
[8] | Aylin Aydoğdu, Sean T. McQuade, Nastassia Pouradier Duteil . Opinion Dynamics on a General Compact Riemannian Manifold. Networks and Heterogeneous Media, 2017, 12(3): 489-523. doi: 10.3934/nhm.2017021 |
[9] | Yuntian Zhang, Xiaoliang Chen, Zexia Huang, Xianyong Li, Yajun Du . Managing consensus based on community classification in opinion dynamics. Networks and Heterogeneous Media, 2023, 18(2): 813-841. doi: 10.3934/nhm.2023035 |
[10] | Sabrina Bonandin, Mattia Zanella . Effects of heterogeneous opinion interactions in many-agent systems for epidemic dynamics. Networks and Heterogeneous Media, 2024, 19(1): 235-261. doi: 10.3934/nhm.2024011 |
In this paper, we study a model of opinion dynamics based on the so-called "bounded confidence" principle introduced by Hegselmann and Krause. Following this principle, voters participating in an electoral decision with two options are influenced by individuals sharing an opinion similar to their own.
We consider a modification of this model where the operator generating the dynamical system which describes the process of formation the final distribution of opinions in the society is defined in two steps. First, to the opinion of an agent, a value proportional to opinions in his/her "influence group" is added, and then the elements of the resulting array are divided by the maximal absolute value of elements to keep the opinions in the prescribed interval. We show that under appropriate conditions, any trajectory tends to a fixed point, and all the remaining fixed points are Lyapunov stable.
Various models of opinion dynamics have been studied since 1950s ([6,7]). At present, opinion studies are a well-developed field of research (see, for example, the monographs [14,20] and the recent survey [17]). The main goal of opinion dynamics is to describe and analyze evolution of public opinion in social systems.
Mostly, models studied in opinion dynamics are linear, which allows one to apply more or less standard methods of linear dynamical systems. One of the first nonlinear models was suggested in [11,12], where the notion of "bounded confidence" has been introduced. This notion formalizes the fact that, in the course of formation of public opinion, a member of the society is mostly influenced by individuals sharing a similar opinion.
The first opinion model based on the notion of bounded confidence, introduced by Hegselmann and Krause, was later called the Hegselmann – Krause (HK) model; this model and its generalizations have been intensively studied by various authors, see, for example, [5,16,13,1,15,4,3,10,21,22,8]. Mostly, the results were based on computer simulations, and it was noticed that "rigorous analytical results are difficult to obtain [9].
In the paper [18], a modification of the HK model suggested by Campi was studied. Let us consider the dynamics of opinions in a society of voters who have to choose between two options, -1 and 1. Assume that the society is formed by
Fix a positive
J(vnk)={l∈{1,…,N}:|vnl−vnk|≤ε}. |
This is the set of indices of agents whose opinions influence agent
In the classical HK model, the dynamics of voters is based on the following procedure: at the step of the process of opinion formation at time
In the model studied in the paper [18], the average of values
Thus, when taking the average, the opinion of agent
Mathematically, this modification of the process may lead to the following consequence: some of the new values may be outside the interval
The dynamics of the appearing dynamical system has been completely described in [18]. It was shown that if
In this paper, we study a model similar to that considered in [18] but with a different norming. Instead of "cutting" the values obtained at the first step (when we add to
Our main results are as follows:
● we find a condition (see inequality (6)) under which any trajectory tends to a fixed point as time goes to infinity;
● we describe all fixed points in this case;
● we show that both fixed points
● we prove that all the remaining fixed points are Lyapunov stable (thus, the dynamics of our system is completely different from that of the system studied in [18]) but not attracting;
● we give an example of the system for which condition (6) is not satisfied and that has an unstable fixed point.
Of course, our reasoning in this paper essentially differs from that in [18].
The structure of the paper is as follows. Section 2 is devoted to the statement of the problem. In Section 3, basic properties of the system are described. In Section 4, we prove the convergence of trajectories to fixed points. In Section 5, stability of fixed points is analyzed. In Section 6, we give an example of a system with an unstable fixed point. Section 7 contains several examples of computer modeling.
We study a dynamical system modeling the following problem of opinion dynamics. A society consisting of
Vn=(vn1,…,vnN) |
be the array of opinions at time moment
Let us define the operator
V=(vk∈[−1,1]:k∈{1,…,N}). |
Introduce the sets
J(vk)={l∈{1,…,N}:|vl−vk|≤ε},k∈{1,…,N}. |
Denote by
Define an auxiliary array
W(V)=(w1(V),…,wN(V)), |
where
wk(V)=vk+hI(vk)∑l∈J(vk)vl,k=1,…,N. | (1) |
Now, assuming that
m(W(V))=maxl∈{1,…,N}|wl(V)| |
and
Φ(V)=(v′1,…,v′N), |
where
v′k=wk(V)m(W(V)). |
Clearly,
v′k∈[−1,1]. | (2) |
We can represent
W(V)=(E+hA)V, |
where
Consider an initial array of opinions
It follows from the above reasoning that if
For simplicity, we denote
Denote by
v1≤v2≤⋯≤vN. | (3) |
Lemma 3.1. If
Proof. It follows from [18,Corollary 1] that inequality (3) implies the inequalities
It is easily seen that the value
Let us note one important inequality. Without loss of generality, we may assume that, for given
m(W(V))=|vN+hI(vN)∑l∈J(vN)vl|≤1+h. | (4) |
Lemma 3.2. If
Proof. It is enough to prove the statement for
The inequality
vnk+hI(vnk)∑l∈J(vnk)vnl≤vnk(1+h) |
and
vnk+1+hI(vnk+1)∑l∈J(vnk+1)vnl≥vnk+1(1+h). |
Thus,
vn+1k+1−vn+1k≥(vnk+1−vnk)⋅(1+h)m(W(Vn))>ε(1+h)m(W(Vn)). | (5) |
Now inequality (4) implies the required inequality
vn+1k+1−vn+1k>ε. |
Remark 1. An analog of Lemma 3.2 does not necessarily hold for
Let
V0=(−1,−5/16,0,0,0,0,5/16,1), |
then
V1=(−1,−1/4,0,0,0,0,1/4,1). |
Hence,
In this section, we show that if
ε(N−1)<1, | (6) |
then any trajectory
Now we introduce the object which is the main tool in the following proofs.
Definition 4.1. For an array
(1) if
(2)
The value
In what follows, we often use the term band instead of band at time
Remark 2. Since we work with trajectories in
vnk≤…≤vnm |
for any band
It follows from Lemma 3.2 that if
Thus, for any band
{k,k+1,…,m}=r⋃j=1{kj,…,mj} | (7) |
with
Clearly, if
We introduce the following condition on the initial array
Condition A. The array
If
If
Thus, inequality (6) implies Condition A for any array
In the remaining part of this section, we assume that any initial array
Lemma 4.2. For any
limn→∞m(W(Vn))=1+h. | (8) |
Proof. Fix a band
Let us consider the behavior of
vn+1k=1m(W(Vn))(vnk+h1I(vnk)∑l∈J(vnk)vnl)≥(1+h)m(W(Vn))vnk. |
To get a contradiction, assume that relation (8) does not hold. Then there exists a subsequence
m(W(Vnk))≤1+h−α |
for some
Without loss of generality, we may assume that the above inequalities hold for all
vn+1k≥1+h1+h−αvnk=βvnk, |
where
β=1+h1+h−α>1. |
Thus,
vn+1k≥βnv0k→∞,n→∞, |
which contradicts the inequalities
Let us describe the behavior of a band of diameter not more than
Lemma 4.3. Assume that a band
limn→∞(vnm−vnk)=0. | (9) |
Proof. Without loss of generality, we assume that
m(W(Vn))≥γ>1,n≥0. |
It follows from our assumption (the band does not split) that
vn+1m−vn+1k=1m(W(Vn))(vnm+hI∑l∈Jvnl−vnk−hI∑l∈Jvnl)=1m(W(Vn))(vnm−vnk)≤1γ(vnm−vnk). |
This obviously implies the statement of our lemma.
Now we prove that the diameter of every band in decomposition (7) does not exceed
Lemma 4.4. For any initial array
First we fix some constants.
Fix a positive
δ≤εh3N(1+h), | (10) |
a positive
β≤δh3N(1+h), | (11) |
and a positive
α1+h−α≤β. | (12) |
We get Lemma 4.4 as a corollary of the following two lemmas.
Lemma 4.5. Assume that a band
Proof. Fix a time
By assumption, the value
vns−vnk≤ε |
it follows that
Then we have the following estimates for
vn+1s−vn+1k=1m(W(Vn))(vns+hI(vns)∑l∈J(vns)vnl−vnk−hI(vnk)∑l∈J(vnk)vnl)≥1m(W(Vn))(vns+h(I(vns)−1I(vns)vnk+1I(vns)vns+1)−vnk−hvns)≥1m(W(Vn))(vns+h(I(vns)−1I(vns)(vns−δ)++1I(vns)(vns+ε−δ))−vnk−h(vnk+δ))≥1+hm(W(Vn))(vns−vnk)+hm(W(Vn))⋅(εN−2δ)≥h1+h(εN−2δ)≥δ. |
In the last line, we apply inequality (10).
To get the upper bound, take a number
m(W(Vn))≥23+h,n≥n0. |
If
vn+1s−vn+1k=1m(W(Vn))(vns+hI(vns)∑l∈J(vns)vnl−vnk−hI(vnk)∑l∈J(vnk)vnl)≤1m(W(Vn))(vns+h(1I(vns)vnk+I(vns)−1I(vns)(vnk+ε+δ))−vnk−hvnk)≤1m(W(Vn))(vnk+δ+h(1I(vns)vnk+I(vns)−1I(vns)(vnk+ε+δ))−vnk−hvnk)=1m(W(Vn))(δ+h(I(vns)−1I(vns)(ε+δ)))≤123+h((1+h)δ+hε)≤123+h(2δ+hε)≤ε, |
where in the last line we take into account that
Lemma 4.6. Assume that a band
vn+2k≥vnk+β. |
Proof. We claim that there exists
vn+1k≥vnk−β,n≥n0, | (13) |
and if there exists an index
vn+1k≥vnk+2β,n≥n0. | (14) |
Then the statement of our lemma follows from Lemma 4.5. Indeed, there are two possible cases:
● There exists an index
Applying the inequalities (13) and (14), we get
vn+2k≥vn+1k−β≥(vnk+2β)−β=vnk+β. |
● For all
vn+2k≥vn+1k+2β≥(vnk−β)+2β=vnk+β |
which completes the proof.
To establish estimate (13), let us fix a positive
Consider an
m(W(Vn))≥1+h−α,n≥n0. |
If
vn+1k=1m(W(Vn))(vnk+hI(vnk)∑l∈J(vnk)vnl)≥1+hm(W(Vn))vnk=vnk+(1+hm(W(Vn))−1)vnk≥vnk−(1+hm(W(Vn))−1)≥vnk−α1+h−α≥vnk−β. |
Next, we assume that
vn+1k=1m(W(Vn))(vnk+hI(vnk)∑l∈J(vnk)vnl)≥1m(W(Vn))(vnk+h(I(vnk)−1I(vnk)vnk+1I(vnk)vns))≥1m(W(Vn))(vnk+h(I(vnk)−1I(vnk)vnk+1I(vnk)(vnk+δ)))=1+hm(W(Vn))vnk+δhI(vnk)⋅m(W(Vn))≥vnk−α1+h−α+δhN(1+h)≥vnk+2β. |
To prove Lemma 4.4, we assume that the diameter of the band
Theorem 4.7. If condition (6) is satisfied, thenany trajectory
Proof. It follows from Lemmas 4.3 and 4.4 that for any initial nonzero array
{1,…,N}=r⋃i=1{k:bi≤k≤ci}, |
where
0≤vnci−vnbi→0,n→∞,i=1,…,r. |
There are the following possible cases:
●
●
●
In any of these cases, there exist numbers
vnk→ai,bi≤k≤ci,n→∞, |
(and
It follows from the left-hand side of inequality (5) that
vn+1ci+1−vn+1bi≥vnci+1−vnbi,i=1,…,r−1; |
hence,
ai+1−ai>ε,i=1,…,r−1. |
In addition, either
A=(a1,…,a1,a2,…,a2,…,ar,…,ar) |
is a fixed point of
Vn→A,n→∞. |
Remark 3. In fact, the proofs of Lemmas 4.3 and 4.4 (and hence, of Theorem 4.7) are based not on condition (6) but on the assumption that for any
We refer to condition (6) in Theorem 4.7 since the above-formulated two assumptions are of "inner" character while condition (6) relates values from the statement of the problem.
In this section, we assume that relation (8) holds. As was noted, this assumption implies the conclusion of Theorem 4.7. Let us study the stability properties of the appearing fixed points of
First we introduce the following notation. Let
(B1(a1),…,Br(ar)) |
is called the scheme of the fixed point
Bj(aj)={bj,…,cj},for any j=1,…,r, |
where
pk=aj,k∈Bj(aj). |
Let us select two fixed points,
Theorem 5.1. If relation (8) holds, then
(1) both fixed points
(2) any fixed point
Proof. Let us first prove item (1). We consider the case of the fixed point
First we prove that
Let
vk∈[1−δ,1],k∈{1,…,N}. | (15) |
Then
wk(V)≥(1+h)(1−δ),k∈{1,…,N}, |
and
(Φ(V))k∈[1−δ,1],k∈{1,…,N}. |
This implies that
(Φn(V))k∈[1−Δ,1],k∈{1,…,N},n≥0. |
Thus,
As was said before introducing Condition A, we may assume that, for any
|vnk−1|→0,n→∞,k∈{1,…,N}. |
Thus,
Now we prove item (2). Consider two possible cases.
Case 1. The fixed point
Case 2. The fixed point
(B1(−1),…,Bl(al),…,Br(1)), |
where
Fix a
aj+1−aj>ε+2Δ,j=1,…,r−1. | (16) |
Without loss of generality, we assume that
|vk−pk|≤Δ,k∈{1,…,N}, |
then
Take a positive
2δ1−δ<Δ. | (17) |
Clearly, in this case
Introduce the following condition on the trajectory of an initial point
Condition C(
|vnk−pk|≤Δ,k∈{1,…,N},0≤n≤ν. |
We show that if
|v0k−pk|≤δ,k∈{1,…,N}, | (18) |
for an initial point
Thus, below we assume that inequalities (18) are satisfied.
Since
We start with
|vnk−1|≤δ,k∈Br(1),n≤ν+1. |
Denote
μn=m(W(Vn−1))×⋯×m(W(V1))×m(W(V0)). |
Since
(1+h)nμn(1−δ)≤vnk≤1,0≤n≤ν+1,k∈Br(1), |
and the inequalities
(1+h)nμn−1≤δ1−δ,0≤n≤ν+1, | (19) |
hold.
Now we consider indices
v0k≤al+δ,v1k≤1+hμ1(al+δ),…,vnk≤(1+h)nμn(al+δ) |
for
Hence, if
vν+1k−al≤(1+h)ν+1μν+1(al+δ)−al=((1+h)ν+1μν+1−1)al+(1+h)ν+1μν+1δ≤2δ1−δ<Δ. |
Similarly one shows that
vν+1k−al>−Δ, |
which proves that Condition C(
This completes the proof of Lyapunov stability of the fixed point
To prove that
(B1(−1),…,Bl(al+δ),…,Br(1)), |
where
The following example shows that if
Let
P=(−1,−1/2,0,0,1/2,1). |
Clearly,
W(P)=(−1−3h4,−12−3h8,0,0,12+3h8,1+3h4),m(W(P))=1+3h4, | (20) |
and
This fixed point is unstable; for any small
V0=(−1,−1/2,δ,δ,1/2,1) |
has a band
m(W(Vn))→1+h,n→∞, |
which, compared with relation (20) indicates that the fixed point
In Fig. 3 of the next section, the dynamics with
The first figure illustrates the dynamics of the system for which condition (6) holds. Figure 1 shows the initial distribution of opinions and the evolution of the system at times 10, 30, and 70. One can see that at time 70, the equilibrium is almost reached. The fixed points of this system are 4 groups of equal numbers.
The second example illustrates the evolution of the system with a larger number of agents. Here, condition (6) is not met, but Condition A holds. Figure 2 shows the initial distribution of such a system with
Figure 3 shows the dynamics of the band
V0=(−1,−1/2,δ,δ,1/2,1) |
which was mentioned in Section 6. The illustration shows the initial distribution of this system with
The authors are grateful to the reviewers for valuable comments which allowed to improve the presentation.
Initial distribution and opinions' evolution of system with (6) at steps 10, 30 and 70;
Initial distribution and opinions' evolution of system with Condition A at steps 20, 40 and 90;
Initial distribution and opinions' evolution for third example at steps 10, 30 and 70, when the equilibrium is reached;