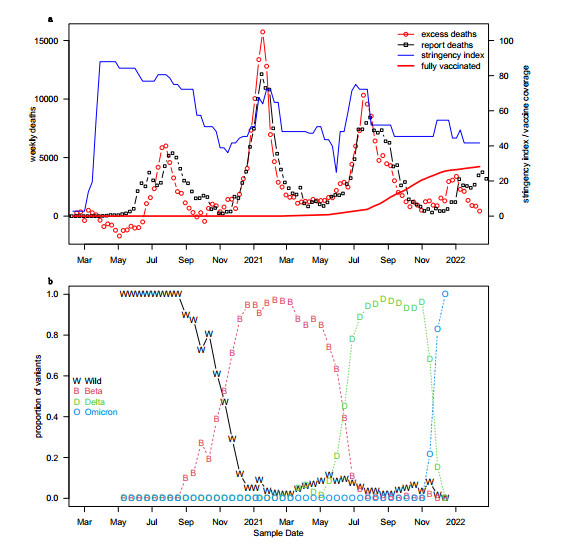
The COVID-19 pandemic caused multiple waves of mortality in South Africa, where three genetic variants of SARS-COV-2 and their ancestral strain dominated consecutively. State-of-the-art mathematical modeling approach was used to estimate the time-varying transmissibility of SARS-COV-2 and the relative transmissibility of Beta, Delta, and Omicron variants. The transmissibility of the three variants were about 73%, 87%, and 276% higher than their preceding variants. To the best of our knowledge, our model is the first simple model that can simulate multiple mortality waves and three variants' replacements in South Africa. The transmissibility of the Omicron variant is substantially higher than that of previous variants.
Citation: Yangyang Yu, Yuan Liu, Shi Zhao, Daihai He. A simple model to estimate the transmissibility of the Beta, Delta, and Omicron variants of SARS-COV-2 in South Africa[J]. Mathematical Biosciences and Engineering, 2022, 19(10): 10361-10373. doi: 10.3934/mbe.2022485
[1] | Kimihito Ito, Chayada Piantham, Hiroshi Nishiura . Estimating relative generation times and reproduction numbers of Omicron BA.1 and BA.2 with respect to Delta variant in Denmark. Mathematical Biosciences and Engineering, 2022, 19(9): 9005-9017. doi: 10.3934/mbe.2022418 |
[2] | Rahat Zarin, Usa Wannasingha Humphries, Amir Khan, Aeshah A. Raezah . Computational modeling of fractional COVID-19 model by Haar wavelet collocation Methods with real data. Mathematical Biosciences and Engineering, 2023, 20(6): 11281-11312. doi: 10.3934/mbe.2023500 |
[3] | Fang Wang, Lianying Cao, Xiaoji Song . Mathematical modeling of mutated COVID-19 transmission with quarantine, isolation and vaccination. Mathematical Biosciences and Engineering, 2022, 19(8): 8035-8056. doi: 10.3934/mbe.2022376 |
[4] | Yuta Okada, Taishi Kayano, Asami Anzai, Tong Zhang, Hiroshi Nishiura . Protection against SARS-CoV-2 BA.4 and BA.5 subvariants via vaccination and natural infection: A modeling study. Mathematical Biosciences and Engineering, 2023, 20(2): 2530-2543. doi: 10.3934/mbe.2023118 |
[5] | Yuan Liu, Anyin Feng, Shi Zhao, Weiming Wang, Daihai He . Large-scale synchronized replacement of Alpha (B.1.1.7) variant by the Delta (B.1.617.2) variant of SARS-COV-2 in the COVID-19 pandemic. Mathematical Biosciences and Engineering, 2022, 19(4): 3591-3596. doi: 10.3934/mbe.2022165 |
[6] | Sarafa A. Iyaniwura, Rabiu Musa, Jude D. Kong . A generalized distributed delay model of COVID-19: An endemic model with immunity waning. Mathematical Biosciences and Engineering, 2023, 20(3): 5379-5412. doi: 10.3934/mbe.2023249 |
[7] | Salman Safdar, Calistus N. Ngonghala, Abba B. Gumel . Mathematical assessment of the role of waning and boosting immunity against the BA.1 Omicron variant in the United States. Mathematical Biosciences and Engineering, 2023, 20(1): 179-212. doi: 10.3934/mbe.2023009 |
[8] | Ugo Avila-Ponce de León, Angel G. C. Pérez, Eric Avila-Vales . Modeling the SARS-CoV-2 Omicron variant dynamics in the United States with booster dose vaccination and waning immunity. Mathematical Biosciences and Engineering, 2023, 20(6): 10909-10953. doi: 10.3934/mbe.2023484 |
[9] | Yi Yin, Ting Zeng, Miao Lai, Zemin Luan, Kai Wang, Yuhang Ma, Zhiliang Hu, Kai Wang, Zhihang Peng . Impact of antibody-level on viral shedding in B.1.617.2 (Delta) variant-infected patients analyzed using a joint model of longitudinal and time-to-event data. Mathematical Biosciences and Engineering, 2023, 20(5): 8875-8891. doi: 10.3934/mbe.2023390 |
[10] | Kun Wang, Peng Wang, Zhengang Jiang, Lu Wang, Linhua Zhou, Dequan Qi, Weishi Yin, Pinchao Meng . Data-driven assessment of immune evasion and dynamic Zero-COVID policy on fast-spreading Omicron in Changchun. Mathematical Biosciences and Engineering, 2023, 20(12): 21692-21716. doi: 10.3934/mbe.2023960 |
The COVID-19 pandemic caused multiple waves of mortality in South Africa, where three genetic variants of SARS-COV-2 and their ancestral strain dominated consecutively. State-of-the-art mathematical modeling approach was used to estimate the time-varying transmissibility of SARS-COV-2 and the relative transmissibility of Beta, Delta, and Omicron variants. The transmissibility of the three variants were about 73%, 87%, and 276% higher than their preceding variants. To the best of our knowledge, our model is the first simple model that can simulate multiple mortality waves and three variants' replacements in South Africa. The transmissibility of the Omicron variant is substantially higher than that of previous variants.
The coronavirus disease 2019 (COVID-19) spread rapidly and ravaged the world in a short time. As of December 18, 2021,271,963,258 confirmed cases and 5,331,019 deaths had been recorded (WHO, https://covid19.who.int/), seriously affecting global public health. The rapid mutation rate of the virus is an important reason for its huge and long-lasting impact. Currently, several variants of the virus have emerged, causing multiple peaks of COVID-19 infection worldwide. In South Africa, the Wild strain, Beta variant, Delta variant, and Omicron variant have emerged and dominated. Basically, the newly emerged variant viruses show stronger infectivity and weaken the effectiveness of vaccines [1,2].
In October 2020, the Beta variant was first discovered in South Africa. It triggered the second wave of outbreaks in the country [3]. Compared to the Wild strain, the Beta variant spread rapidly in South Africa and increased infectivity and immunity evasion. Additionally, the effect of the ChAdOx1 nCoV-19 vaccine on the Beta variant was severely weakened [4,5]. Therefore, the Beta variant has higher reinfection characteristics than the Wild strain [6]. However, compared to the Beta variant, the Delta variant is much more fatal.
In December 2020, the Delta variant was first detected in Maharashtra, India. It spread rapidly to other countries and regions [7]. Five studies estimated the basic reproductive number, R0, of the Delta variant and indicated that the range of R0 of the Delta variant is 3.2–8, with an average value of 5.08 [8], which is significantly higher than those of the Alpha variant [7] and Wild strain [8]. Some studies indicated that the increased replication suitability and decreased sensitivity to neutralizing antibodies of the Delta variant have led to a greatly increased infectivity of the Delta variant [9]. However, the Omicron variant, as the mutant strain with the most mutation sites currently during the COVID-19 pandemic, seems to have a higher transmission rate, lower vaccine efficiency, and higher reinfection risk [10]. In an effort to explore the transmissibility of the Delta variant, Ito et al. reported the predominance of the Delta variant in the run-up to the July 2021 Olympics in Tokyo, Japan. The authors used renewal-equation-based model which is different from our model to describe the adaptive evolution of multiple variants in Japan and demonstrated that the Delta variant was more transmissible than its predecessor, with a transmittance 1.4 times higher than that of the Alpha variant [11].
The Omicron variant was first discovered in South Africa on November 9, 2021, and was classified variant of concern by WHO on November 26, 2021 [12]. As of December 16, 2021, the Omicron variant has existed in 89 countries and regions, and it is spreading at an unprecedented speed. In South Africa, the variant quickly replaced the Delta variant and caused a rapid increase in the number of infections [13]. The number of daily cases rose rapidly from 273 cases on November 17, 2021 to above 26,389 cases on December 16, 2021 (WHO, https://covid19.who.int/).
Presently, several studies have been conducted on the Omicron variant. Some of these studies investigated Omicron's vaccine breakthrough rate and antibody resistance through in vitro experiments and clinical research. In an in vitro experimental study on the SARS-CoV-2 variants, Wilhelm et al. demonstrated that the neutralizing effect of the vaccine against the Omicron variant was severely reduced, compared to the Delta variant [14]. Furthermore, Zhang et al. demonstrated that the Omicron variant may lead to more obvious evasion of immunity in an in vitro study [15]. Karim et al. compared the neutralizing titers of the Omicron variant with those of the Victoria, Beta, and Delta variants, and indicated that the Omicron variant will cause more breakthrough infections, which may trigger further infection waves [1,16]. Mohiuddin et al. used the reduction in neutralizing antibody titers to infer vaccine effectiveness. The study reported that the effect of the vaccine on the Omicron variant was severely reduced, the effectiveness of vaccines against severe illnesses was significantly reduced for frail individuals, and the protection against infection, mild illness, and transmission was almost eliminated [17]. Kuhlmann et al. revealed that three doses of the mRNA vaccine may not be enough to prevent infection and symptomatic diseases of the Omicron variant based on clinical studies on patients [18]. Additionally, Nishiura et al. reported the relative reproductive numbers of Omicron and Delta variants in South Africa by a mathematical model different from ours [19]. The authors assumed that the effective reproduction number of the Omicron variant, ROmicron(t) was given by multiplying a constant factor k to that of Delta variant, RDelta(t). This research paper reported that the effective reproduction number of the Omicron variant was estimated to be 4.2 times more than Delta variant, and 3.3 times more transmissible than the Delta variant.
Some studies used theoretical models to investigate the effects of the Omicron variant. Bai et al. analyzed the population movement data obtained from both flights in South Africa and the Omicron case report data, and estimated that the probability of the Omicron variant being introduced into the studied country before November 28, 2021 was higher than 50% [20]. Kumar et al. studied the spike proteins of the Omicron and Delta variants using several computational tools and a computational saturation mutagenesis model. They found that the Omicron variant has a higher affinity for human angiotensin-converting enzyme 2 (ACE2) receptors than the Delta variant, indicating that the Omicron variant has a higher transmission potential [21]. In another study on the Omicron variant's infectiousness, vaccine breakthrough, and antibody resistance using an artificial intelligence model, the variant's infectivity was found to be above 10 times higher than that of the Wild virus or about twice that of the Delta variant. Vaccine breakthrough was twice that of the Delta variant, and antibody resistance had been weakened [22]. Kuhlmann et al. [18] used the meta-analysis method to predict that after 6 months of initial immunization with mRNA vaccines, the vaccine's efficacy on the symptoms of patients infected by the Omicron variant was estimated to have reduced to about 40%. Additionally, the efficacy on severe diseases had decreased to about 80% [23]. Furthermore, the OpenCOVID individual-based model was used to compare the infectiousness, severity, and immune evasion properties of the Omicron and Delta variants. The model indicated that the Omicron variant could become the new dominant variant [24].
Figure 1a shows the weekly reported deaths and excess deaths with stringency index and vaccination coverage in South Africa. According to the figure, the number of excess deaths was about three-fold that of the reported deaths, the number of weekly deaths varies largely with the stringency index and variant invasion, and vaccination could effectively reduce the number of deaths. Figure 1b shows the trend of infection by the four COVID-19 virus variants over time in South Africa. The Beta variant gradually replaced the wild strain from September 2020 to January 2021, then the Delta variant gradually replaced the Beta variant from May to August 2021. After November, the Omicron variant replaced the Beta variant and became the dominant variant within a month.
In this work, we propose a new model and fit the model to the adjusted COVID-19 deaths and the proportion of variants in South Africa to estimate the relative transmission rates of the Beta, Delta, and Omicron variants, compared to their preceding dominant strains, which were the Wild strain, Beta variant, and Delta variant, respectively.
The susceptible-exposed-infectious-hospitalized-recovered-death (SEIHRD) model from our previous studies [31,32] was adopted and extended to simulate the alternative dominance of the Wild strain, Beta variant, Delta variant, and Omicron variant. Based on the assumption that the variant replacement only affected the overall transmission rate of COVID-19 and only transmission changes but no variant changes occurred, the SEIHRD model with a flexible time-varying transmission rate could simulate COVID-19 deaths (or excess deaths) [33]. To simulate the replacement of the proceeding strain by a variant, an additional set of (EIHRD) equations for the variant were included. Since the replacements occurred thrice in South Africa, one set of the SEIHRD model for the Wild strain and three additional sets of EIHRD models for the Beta, Delta variant, and Omicron variants, amounting to 21 equations, were used. However, one set of SEIHRD and one additional set of EIHRD were sufficient. Therefore, only 8 equations were used if further merging HRD classes for two successive variants. At any moment, at most two strains (or variants) dominated. Therefore, a system of two groups of models was sufficient to simulate the replacement.
The dominance of the variants could be divided into several time intervals:
(1) Before the emergence of the Beta variant, (SEIHRD)1 was used to model the dynamics of the wild strain;
(2) After the emergence of the Beta variant, (EIHRD)2 was used to model the dynamics of the Beta variant;
(3) After the emergence of the Delta variant, by which the Wild stain had almost been replaced, (SEIHRD)1 was reused to model the dynamics of the Delta variant;
(4) After the emergence of the Omicron variant, by which the Beta variant had been replaced, (SEIHRD)2 was reused to model dynamics of the Omicron variant.
Therefore, only 8 equations were used to simulate the successive replacements of one Wild strain and three variants. This system can be used to model further replacements in principle. To the best of our knowledge, our model is the simplest model for this purpose.
Our model reads as follows:
˙S=−ε1βSI1N−ε2βSI2N, | (1) |
˙E1=ε1βSI1N−σE1, | (2) |
˙E2=ε2βSI2N−σE2, | (3) |
˙I1=σE1−γI1, | (4) |
˙I2=σE2−γI2, | (5) |
˙H=πγ(I1+I2)−κH, | (6) |
˙D=θκH, | (7) |
˙R=(1−π)γI1+(1−π)γI2+(1−θ)κH. | (8) |
If t∈[0,t1]∪[t2,t3] and E1<1, then E1=1, S=S−1. If t∈[0,t1]∪[t2,t3] and E1<1, then E1=1, S=S−1. If t∈[0,t1], ε1=ε2=1, If t∈[t1,t3], ε2=η1, If t∈[t2,t4], ε1=η1η2, If t∈[t3,t4], ε2=η1η2η3. We simulate weekly deaths Dt+Δt as
Dt+Δt=∫t+ΔttθκHdt | (9) |
And we denote the weekly reported deaths as Zt+Δt. We assume
Zt+Δt∼NegativeBinominal(mean=Dt+Δt,variance=Dt+Δt(1+τDt+Δt)) | (10) |
LogLikelihood=∑ni=1logf(Zi|Z1:(i−1),Θ) | (11) |
We assumed transmission rate was exponential cubic spline function, we used nβ = 13, which meant there were 13 nodes in the cubic spline evenly distributed over the study period. We denote these 13 nodes as (ti,bi), where i = 1 to 13, ti=tstart+(i−1)12∗(tend−tstart), bi were positive values to be estimated via fitting model to data. Given our model and parameter setting (including fixed parameter and unknown parameters) and data, we used standard iterated filtering to achieve the maximum log likelihood estimates of all unknown parameters which included these 13 bi. The transmission rate was shown in the Figure 2 as dashed blue curve.
According to Figure 1, the number of excess deaths was about three-fold that of the reported deaths and excess deaths had negative values. Therefore, reported COVID-19 deaths was multiplied by a factor of 3, resulting in the adjusted COVID-19 deaths.
The plug-and-play likelihood-based inference framework [34] was adopted and implemented in the R package POMP [35] to fit the model.
For COVID-19 deaths, a negative binomial measurement model was used to link the simulated model and adjusted reported deaths. For the variant proportions, out of several options, a simple approach was adopted. For the three time-intervals, based on the replacement process of the variants, the sum of squared errors between the simulated and reported proportions was calculated. The simulated proportion was defined as either E1/(E1+E2) or E2/(E1+E2), which is the ratio of exposed cases of the variant to the exposed cases of both the variant and the preceding strain or variant. The transmissibility of the Beta, Delta, and Omicron variants was denoted as η1-fold of that of the Wild strain, η2-fold of that of the Beta strain, and η3-fold of that of the Delta strain, respectively.
A comparison of the fitting performance of models with different number of nodes in the transmission rate and the second-order Akaike Information Criterion (AICc) revealed that nβ=13 yielded the smallest AICc. The emergence times of the three variants were fixed on June 12, 2020, February 23, 2021, and September 27, 2021. These dates were 1–2 months ahead the first time these variants were reported on August 31, 2020, March 22, 2021 and November 1, 2021. We introduce one exposed case of the dominant variants if there is no exposed case of the dominant variants in a day to mimic continuous importation of cases.
Aggregated variant proportion data, vaccination data and stringency index data were obtained from the complied data source in The Our World in Data [25] and original from the GISAID [26,27,28,29,30].
Figure 2 shows the results of model fitting. Panel 2a shows the model of the simulated deaths and adjusted COVID-19 deaths. Our model simulation largely matched the observed three-fold adjusted COVID-19 deaths in South Africa, with an estimated infection fatality rate of about 1%. Panels b–d show the simulated proportions of the Beta, Delta, and Omicron variants and the observed proportions. The simulated and observed proportions were closely matched. The estimated relative transmission rate factor is shown in Panels b–d.
Simulated and observed proportions were closely matched. The estimated η1=1.73, η2=1.87, and η3=3.76 are similar to the estimates for the Beta, Delta, and Omicron variants, reported by previous studies at 1.69-fold [2], 1.65-fold [37], and 3.8-fold of their preceding strain or variant, respectively. In Figure 3, 200 groups of (η1, η2, η3) values were sampled, and the model was refitted. The sum of squared errors between observed and simulated proportion time series were calculated. The results in Figure 3 suggest that our estimates of η1, η2, and η3 are robust.
In our model, we ignored the effect of re-infection and effects of vaccination because the coverage of fully vaccinated individuals was relatively low and the invasion of the Omicron variant occurred at the end of the study period. Allowing re-infection would mean a proportion of recovered individuals would become susceptible, leading to an increase in the susceptible pool, i.e., S→S+∆S. However, concurrently, the transmission rate was increased due to the increased transmissibility of the Omicron variant, i.e., β→β+∆β. Therefore, the mass action term in our model became βSI→(β+∆β)(S+∆S)I = βSI + (∆βS+∆Sβ+∆β∆S)I. If possible, disentangling ∆β and ∆S from fitting this type of model to aggregated death data would be difficult, when the time interval covering the Omicron variant was short. The effects of ∆β and ∆S are exchangeable. Therefore, ignoring the immunity evasion-induced ∆S, i.e., assuming ∆S = 0, and synthesis of all effects into ∆β to estimate ∆β was appropriate. Additionally, in interpreting ∆β, ∆β should be emphasized to include both effects from ∆β and ∆S. Furthermore, we sought to estimate how fast the Omicron variant transmits relative to the Delta variant. Other types of study, such as case-control studies, are needed to reveal the underlying mechanism. The sizes of the susceptible pools for a variant, e.g., Omicron and its preceding variant, e.g., Delta, may be assumed to be the same (i.e., if ∆S = 0, we have R0(Omicron) = η3*R0(Delta)).
We argue that R0(Omicron) could be further categorized into three components: R0 (reinfection), R0 (breakthrough), and R0(natural). Each of these three components has its own exclusive susceptible pool. R0 (reinfection) is in the pool of those infected by previous strains/variants. R0 (breakthrough) is in the pool of the vaccinated population, and R0 (natural) is in the pool of unvaccinated susceptible individuals.
For the Delta and Omicron variants, the re-infection risks are 15% and 81% [38]. The vaccine breakthrough risk for the Delta variant is 40%. The vaccine efficacy is 60% 3 months after administration of the second vaccine dose, while the vaccine breakthrough risk is high for the Omicron variant. However, the vaccination coverage in South Africa is only 25.96% (fully vaccinated by December 16, 2021). Given the high infection attack rate, the susceptible pool in South Africa is probably 20–30% currently.
Assuming that the susceptible, fully vaccinated, and recovered individuals were 20%, 20%, and 60% of the population in November 2021, in reality the vaccinated and recovered individuals could overlap. In this study, we assigned the group that overlapped (e.g., infected but vaccinated as well, due to unawareness of infection status) to one of the two groups. Given the above information, the composition of the "susceptible pool" for infection with the Delta and Omicron variants is indicated in Table 1.
Susceptible | Vaccinated | Recovered | Total | |
Delta | 20% | 8% (20*40%) | 9% (60*15%) | 88.6% |
Omicron | 20% | 20% (20*100%) | 48.6% (60*81%) | 37% |
Therefore, the susceptible pool of the Omicron variant was about 2.39-fold of that of the Delta variant in November 2021, and the observed transmission advantage was partly due to this difference in the sizes of susceptible pools of the two variants. The natural increase in the transmissibility was 1.57 (1.57*2.39 = 3.76).
As for the spread of the new variant, there were several preceding studies that have reported the relative transmissibility of new variants compared to the preceding one. Kathy Leung et al. reported the early transmissibility of the N501Y mutant strain in the UK between October and November 2020 from a bioinformatics and public health perspective. The authors extracted all viral genomes carrying 501Y from translated spike proteins and analysed them with other closely related viral strains in global phylogeny. Two 501Y variants were identified. By extending the competitive transmission model for the two viruses, the 501Y variant 2 (also known as Alpha variant) 𝑅0 was estimated to be 1.75 times higher than 501N, implying a 75% improvement in transmissible ability compared to 501N strain [39]. Additionally, Roquebert et al. compared the transmissibility of Beta variant and Alpha variant in parts of France. The authors used sequencing maps and reverse transcription PCR results to determine the differences in gene sequence and regional distribution of the two variants. Using multinomial log-linear model and generalized linear model, they found that Beta variant had a spread advantage of 15.8% (95% confidence interval: 15.5-16.2%) in Ile-de-France and 17.3% (95% confidence interval: 15.5-16.2%) in Hauts-de-France [40]. For Omicron variant, Kimihito Ito et al. reported its relative instantaneous reproduction number compared with Delta variant in Denmark. A method was developed to estimate the relative instantaneous regeneration number of one variant relative to another, and the effective (instantaneous) reproductive number of Omicron variant was estimated to be 3.19 times that of Delta variant (95% CI 2.82-3.61) under the same epidemiological conditions [41].
In terms of mathematical modelling part, Chu introduced a dynamics of fractional order COVID-19 model with a case study of Saudi Arabia [42]. In order to formulate the model, they used the classical Caputo type derivative of fractional order. They considered the transmission of infection through the environment and the data since March 02, 2020 to July 31, 2020 which was the second wave of novel pandemic were considered for estimation of parameters. The authors estimated that the basic reproduction number R0 for this data is 1.29. Additionally, Li showed the SEIARD mathematical model to calculate the basic reproduction number. They utilized data which includes the reported cases from March 06, 2021 to April 30, 2021 since they considered the third wave of pandemic and to determine the peak of infection curve. The basic reproduction number calculated to be 1.2044. Moreover, the parameters sensitive to the basic regeneration number were shown, and the effects on model variables were graphically shown. And the model also predicted that the peak of infections was to be May 06, 2021 [43].
We formulated a simple model of 8 equations to simulate the multiple wave patterns in the COVID-19 deaths and three replacements of variants in South Africa. Concurrently, the time varying transmission rate and three relative transmission rate factors were estimated for three variants. The relative transmissibility rates of the Beta, Delta, and Omicron variants were 73%, 87%, and 276% higher than their preceding dominant strain/variants, respectively. This change from the Omicron variant has two sources.
First, the increase in the susceptible pool of Omicron variants was due to enhanced ability of immunity evasion and intrinsic increase in transmission. Mathematical models are important tools for mitigation of pandemics. However, many models are too complicated to be useful. In study, 8 equations were used to simulate a complicated situation of multiple waves and three replacements of variants. Our model can be further simplified if the two exposed classes are removed. This reduction will not significantly change the results, as demonstrated in previous studies [31].
This study had some limitations. First, the possible shortened generation intervals (GI) for the Delta and Omicron variants were ignored, which will lead to an overestimate of the relative transmission rate than if a shortened GI is used. Our estimated relative transmissibility could contain a contribution from the shortened GI (thus more generations in a given time period). Second, reinfection was not considered in model simulation, which will lead an overestimate of the relative transmission rate than if reinfection is considered. Thus, our estimated synthesized relative transmission rate contains contributions from a shortened GI and immunity escaping feature of the variant. Reinfection risk for other variants was low; for instance, for the Beta variant, 10% of cases could be cases of reinfection. However, infectivity and severity were reduced in cases of reinfection. Therefore, consideration of reinfection in the model should include reduced infectivity and severity. With reduced infectivity and severity, the overall effect of reinfection before the Omicron variant must be limited. Our estimate relative transmission rate was under the situation in South Africa, which may be true in other countries/regions with the similar situation (infection attack rate), whereas may not be true in countries/regions with a low infection attack rate. Nevertheless, our modelling approach can be readily used. Simple mathematical framework can be used to estimate the relative transmission rate of multiple variants.
Conceptualization, D.H., Y.Y., Y.L.; methodology, D.H., Y.Y., Y.L.; software, D.H.; validation, D.H., and Y.Y.; formal analysis, D.H.; investigation, Y.L.; resources, S.Z.; data curation, D.H., and Y.L.; writing—original draft preparation, D.H., and Y.Y.; writing—review and editing, D.H., and Y.Y.; visualization, D.H.; supervision, D.H.; funding acquisition, D.H. All authors have read and agreed to the published version of the manuscript.
This study was funded by a grant from the Research Grants Council of the Hong Kong Special Administrative Region, China (HKU C7123-20G) and two grants from the Otto Poon Charitable Foundation Smart Cities Research Institute (SCRI) (#Q-CDAV & Q-CDBA).
All data are publicly available.
The authors declare that they have no known competing financial interests or personal relationships that could affect the design and outcome of the study.
[1] |
K. S. S. Abdool, K.Q. Adbool, Omicron SARS-CoV-2 variant: A new chapter in the COVID-19 pandemic. Lancet, 398 (2021), 2126–2128. https://doi.org/10.1016/S0140-6736(21)02758-6 doi: 10.1016/S0140-6736(21)02758-6
![]() |
[2] |
H. Tegally, E. Wilkinson, C. L. Althaus, M. Giovanetti, J. E. San, J. Giandhari, et al., Rapid replacement of the Beta variant by the Delta variant in South Africa, medRxiv, 2021. https://doi.org/10.1101/2021.09.23.21264018 doi: 10.1101/2021.09.23.21264018
![]() |
[3] |
S. Cele, I. Gazy, L. Jackson, S.-H. Hwa, H. Tegally, G. Lustig, et al., Escape of SARS-CoV-2 501Y. V2 from neutralization by convalescent plasma, Nature, 593 (2021), 142–146. https://doi.org/10.1038/s41586-021-03471-w doi: 10.1038/s41586-021-03471-w
![]() |
[4] |
S. A. Madhi, V. Baillie, C. L. Cutland, M. Voysey, A. L. Koen, L. Fairlie, et al., Efficacy of the ChAdOx1 nCoV-19 Covid-19 vaccine against the B. 1.351 variant, N Engl. J. Med., 384 (2021), 1885–1898. https://doi.org/10.1056/NEJMoa2102214 doi: 10.1056/NEJMoa2102214
![]() |
[5] |
H. Tegally, E. Wikinson, M. Giovanetti, A. Iranzadeh, V. Fonseca, J. Giandhari, et al., Detection of a SARS-CoV-2 variant of concern in South Africa, Nature, 592 (2021), 438–443. https://doi.org/10.1038/s41586-021-03402-9 doi: 10.1038/s41586-021-03402-9
![]() |
[6] |
H. Chemaitelly, R. Bertollini, L. J. Abu-Raddad, Efficacy of natural immunity against SARS-CoV-2 reinfection with the Beta variant, N Engl. J. Med., 385 (2021), 2585–2586. https://doi.org/10.1056/NEJMc2110300 doi: 10.1056/NEJMc2110300
![]() |
[7] |
C. Del Rio, P. N. Malani, S. B. Omer, Confronting the delta variant of SARS-CoV-2, summer 2021, JAMA, 326 (2021), 1001–1002. https://doi.org/10.1001/jama.2021.14811 doi: 10.1001/jama.2021.14811
![]() |
[8] |
Y. Liu, J. Rocklöv, The reproductive number of the Delta variant of SARS-CoV-2 is far higher compared to the ancestral SARS-CoV-2 virus, J. Travel Med., 2021. https://doi.org/10.1093/jtm/taab124 doi: 10.1093/jtm/taab124
![]() |
[9] |
P. Mlcochova, S. A. Kemp, M. S. Dhar, G. Papa, B. Meng, I. A. T. M. Ferreira, et al., SARS-CoV-2 B. 1.617. 2 Delta variant replication and immune evasion, Nature, 599 (2021), 114–119. https://doi.org/10.1038/s41586-021-03944-y doi: 10.1038/s41586-021-03944-y
![]() |
[10] | I. Torjesen, Covid-19: Omicron may be more transmissible than other variants and partly resistant to existing vaccines, scientists fear, BMJ. https://doi.org/10.1136/bmj.n2943 |
[11] |
K. Ito, C. Piantham, H. Nishiura, Predicted dominance of variant Delta of SARS-CoV-2 before Tokyo Olympic Games, Japan, July 2021, Euro. Surveill., 26 (2021), 2100570. https://doi.org/10.2807/1560-7917.ES.2021.26.27.2100570 doi: 10.2807/1560-7917.ES.2021.26.27.2100570
![]() |
[12] |
H. Gu, P. Krishnan, D. Y. Ng, L. D. J. Chang, G. Y. Z. Liu, S. S. M. Cheng, et al., Probable transmission of SARS-CoV-2 omicron variant in quarantine hotel, Hong Kong, China, November 2021, Emerg. Infect. Dis., 28 (2022), 460. https://doi.org/10.3201/eid2802.212422 doi: 10.3201/eid2802.212422
![]() |
[13] |
O. Dyer, Covid-19: Peru's official death toll triples to become world's highest, BMJ, 373 (2021), n1442. https://doi.org/10.1136/bmj.n1442 doi: 10.1136/bmj.n1442
![]() |
[14] |
A. Wilhelm, M. Widera, K. Grikscheit, T. Toptan, B. Schenk, C. Pallas, et al., Reduced neutralization of SARS-CoV-2 omicron variant by vaccine sera and monoclonal antibodies, MedRxiv, 2021. https://doi.org/10.1101/2021.12.07.21267432 doi: 10.1101/2021.12.07.21267432
![]() |
[15] |
L. Zhang, Q. Li, Z. Liang, T. Li, S. Liu, Q. Q. Cui, et al., The significant immune escape of pseudotyped SARS-CoV-2 Variant Omicron, Emerg. Microbes Infect., 11 (2022), 1–5. https://doi.org/10.1080/22221751.2021.2017757 doi: 10.1080/22221751.2021.2017757
![]() |
[16] |
W. Dejnirattisai, R. H. Shaw, P. Supasa, C. Liu, A. S. V. Stuart, A. J. Pollard, et al., Reduced neutralisation of SARS-CoV-2 omicron B. 1.1. 529 variant by post-immunisation serum. Lancet, 399 (2022), 234–236. https://doi.org/10.1016/S0140-6736(21)02844-0 doi: 10.1016/S0140-6736(21)02844-0
![]() |
[17] |
B. J. Gardner, A. M. Kilpatrick, Estimates of reduced vaccine effectiveness against hospitalization, infection, transmission and symptomatic disease of a new SARS-CoV-2 variant, Omicron (B. 1.1. 529), using neutralizing antibody titers, MedRxiv, 2021. https://doi.org/10.1101/2021.12.10.21267594 doi: 10.1101/2021.12.10.21267594
![]() |
[18] |
C. Kuhlmann, C. K. Mayer, M. Claassen, T. G. Maponga, A. D. Sutherland, T. Suliman, et al., Breakthrough infections with SARS-CoV-2 Omicron variant despite booster dose of mRNA vaccine, Available at SSRN 3981711, 2021. https://dx.doi.org/10.2139/ssrn.3981711 doi: 10.2139/ssrn.3981711
![]() |
[19] |
H. Nishiura, K. Ito, A. Anzai, T. Kobayashi, C. Piantham, A. J. Rodriguez-Morales, Relative reproduction number of SARS-CoV-2 Omicron (B. 1.1. 529) compared with Delta variant in South Africa, J. Clin. Med., 11 (2021), 30. https://doi.org/10.3390/jcm11010030 doi: 10.3390/jcm11010030
![]() |
[20] |
Y. Bai, Z. Du, M. Xu, L. Wang, P. Wu, E. H. Y. Lau, et al., International risk of SARS-CoV-2 Omicron variant importations originating in South Africa, medRxiv, 2021. https://doi.org/10.1101/2021.12.07.21267410 doi: 10.1101/2021.12.07.21267410
![]() |
[21] |
S. Kumar, T. S. Thambiraja, K. Karuppanan, G. Subramaniam, Omicron and Delta variant of SARS‐CoV‐2: a comparative computational study of spike protein. J. Med. Virol., 2021. https://doi.org/10.1002/jmv.27526 doi: 10.1002/jmv.27526
![]() |
[22] |
J. Chen, R. Wang, N. B. Gilby, G. W. Wei, Omicron (B. 1.1. 529): Infectivity, vaccine breakthrough, and antibody resistance, J. Chem. Inf. Model., 62 (2022), 412–422. https://doi.org/10.1021/acs.jcim.1c01451 doi: 10.1021/acs.jcim.1c01451
![]() |
[23] |
D. S. Khoury, M. Steain, J. Triccas, A. Sigal, M. P. Davenport, D. Cromer, Analysis: A meta-analysis of Early Results to predict Vaccine efficacy against Omicron, medRxiv, 2021. https://doi.org/10.1101/2021.12.13.21267748 doi: 10.1101/2021.12.13.21267748
![]() |
[24] |
E. A. Le Rutte, A. J. Shattock, N. Chitnis, S. L. Kelly, M. A. Penny, Assessing impact of Omicron on SARS-CoV-2 dynamics and public health burden, medRxiv, 2021. https://doi.org/10.1101/2021.12.12.21267673 doi: 10.1101/2021.12.12.21267673
![]() |
[25] | H. Ritchie, E. Mathieu, L. Rodés-Guirao, C. Appel, C. Giattino, E. Ortiz-Ospina, et al., Coronavirus Pandemic (COVID-19), 2020 [cited 2022 Feb 28]. Available from: https://ourworldindata.org/coronavirus |
[26] |
Y. Shu, J. McCauley, GISAID: Global initiative on sharing all influenza data–from vision to reality, Euro. Surveill., 22 (2017), 30494. https://doi.org/10.2807/1560-7917.ES.2017.22.13.30494 doi: 10.2807/1560-7917.ES.2017.22.13.30494
![]() |
[27] |
S. Khare, C. Gurry, L. Freitas, M. B. Schultz, G. Bach, A, Diallo, et al., GISAID's Role in Pandemic Response, China CDC Wkly, 3 (2021), 1049. https://doi.org/10.46234/ccdcw2021.255 doi: 10.46234/ccdcw2021.255
![]() |
[28] |
S. Elbe, G. Buckland‐Merrett, Data, disease and diplomacy: GISAID's innovative contribution to global health, Global Challenges, 1 (2017), 33–46. https://doi.org/10.1002/gch2.1018 doi: 10.1002/gch2.1018
![]() |
[29] |
E. Mathieu, H. Ritchie, E. Ortiz-Ospina, M. Roser, J. Hasell, C. Appel, et al., A global database of COVID-19 vaccinations, Nat. Hum. Behav., 5 (2021), 947–953. https://doi.org/10.1038/s41562-021-01122-8 doi: 10.1038/s41562-021-01122-8
![]() |
[30] |
T. Hale, N. Angrist, R. Goldszmidt, B. Kira, A. Petherick, T. Phillips, et al., A global panel database of pandemic policies (Oxford COVID-19 Government Response Tracker), Nat. Hum. Behav., 5 (2021), 529–538. https://doi.org/10.1038/s41562-021-01079-8 doi: 10.1038/s41562-021-01079-8
![]() |
[31] |
H. Song, G. Fan, S. Zhao, H. Li, Q. Huang, D. He, Forecast of the COVID-19 trend in India: A simple modelling approach, Math. Biosci. Eng., 18 (2021), 9775–9786. https://doi.org/10.3934/mbe.2021479 doi: 10.3934/mbe.2021479
![]() |
[32] |
H. Song, G. Fan, Y. Liu, X. Wang, D. He, The second wave of COVID-19 in South and Southeast Asia and the effects of vaccination, Front. Med., 8 (2021). https://doi.org/10.3389/fmed.2021.773110 doi: 10.3389/fmed.2021.773110
![]() |
[33] |
S. S. Musa, X. Wang, S. Zhao, S. Li, N. Hussaini, W. Wang, D. He, The heterogeneous severity of COVID-19 in African countries: A modeling approach, Bull. Math. Biol., 84 (2022), 1–16. https://doi.org/10.1007/s11538-022-00992-x doi: 10.1007/s11538-022-00992-x
![]() |
[34] |
D. He, E. L. Ionides, A. A. King, Plug-and-play inference for disease dynamics: Measles in large and small populations as a case study, J. R. Soc. Interf., 7 (2010), 271–283. https://doi.org/10.1098/rsif.2009.0151 doi: 10.1098/rsif.2009.0151
![]() |
[35] |
E. L. Ionides, C. Bretó, A. A. King, Inference for nonlinear dynamical systems, PNAS, 103 (2006), 18438-18443. https://doi.org/10.1073/pnas.0603181103 doi: 10.1073/pnas.0603181103
![]() |
[36] | W. T. Vetterling, W. H. Press, S. A. Teukolsky, B. P. Flannery, Numerical recipes: Example book C (The Art of Scientific Computing), 1992, Press Syndicate of the University of Cambridge. |
[37] |
H. Campbell, P. Gustafson, Inferring the COVID-19 IFR with a simple Bayesian evidence synthesis of seroprevalence study data and imprecise mortality data, medRxiv, 2021. https://doi.org/10.1101/2021.05.12.21256975 doi: 10.1101/2021.05.12.21256975
![]() |
[38] | N. Ferguson, A. Ghani, A. Cori, A. Hogan, W. Hinsley, E. Volz, Report 49: Growth, population distribution and immune escape of Omicron in England, Imperial College London. https://doi.org/10.25561/93038 |
[39] |
K. Leung, M. H. Shum, G. M. Leung, T. T. Lam, J. T. Wu, Early transmissibility assessment of the N501Y mutant strains of SARS-CoV-2 in the United Kingdom, October to November 2020, Euro. Surveill., 26 (2021), 2002106. https://doi.org/10.2807/1560-7917.ES.2020.26.1.2002106 doi: 10.2807/1560-7917.ES.2020.26.1.2002106
![]() |
[40] |
B. Roquebert, S. Trombert-Paolantoni, S. Haim-Boukobza, E. Lecorche, L. Verdurme, V. Foulongne, et al., The SARS-CoV-2 B. 1.351 lineage (VOC β) is outgrowing the B. 1.1. 7 lineage (VOC α) in some French regions in April 2021, Euro. Surveill., 26 (2021), 2100447. https://doi.org/10.2807/1560-7917.ES.2021.26.23.2100447 doi: 10.2807/1560-7917.ES.2021.26.23.2100447
![]() |
[41] |
K. Ito, C. Piantham, H. Nishiura, Relative instantaneous reproduction number of Omicron SARS‐CoV‐2 variant with respect to the Delta variant in Denmark, J. Med. Virol., 94 (2022), 2265–2268. https://doi.org/10.1002/jmv.27560 doi: 10.1002/jmv.27560
![]() |
[42] |
Y. M. Chu, A. Ali, M. A. Khan, S. Islam, S. Ullah, Dynamics of fractional order COVID-19 model with a case study of Saudi Arabia, Results Phys., 21 (2021), 103787. https://doi.org/10.1016/j.rinp.2020.103787 doi: 10.1016/j.rinp.2020.103787
![]() |
[43] |
X. P. Li, Y. Wang, M. A. Khan, M. Y. Alshahrani, T. Muhammad, A dynamical study of SARS-COV-2: A study of third wave, Results Phys., 29 (2021), 104705. https://doi.org/10.1016/j.rinp.2021.104705 doi: 10.1016/j.rinp.2021.104705
![]() |
1. | Xueze Yang, Zhiyang Dou, Yuqing Ding, Boni Su, Hua Qian, Nan Zhang, Analysis of SARS-CoV-2 transmission in airports based on real human close contact behaviors, 2024, 82, 23527102, 108299, 10.1016/j.jobe.2023.108299 | |
2. | Farideh Mostafavi, Mansour Bahardoust, Francesco Sera, Alireza Amirabadizadeh, Sepehr Allahyari, Paddy Ssentongod, Manochehr Karami, Seyed Saeed Hashemi Nazari, COVID-19 Vaccine Effectiveness of Booster Doses Against Delta and Omicron Variants Over Follow-up Times Using Longitudinal Meta-analysis, 2024, 24, 2228-7795, e00626, 10.34172/jrhs.2024.161 | |
3. | Juan Ma, Shaojuan Ma, Xinyu Bai, Jinhua Ran, Ergodic stationary distribution of stochastic virus mutation model with time delay, 2023, 8, 2473-6988, 21371, 10.3934/math.20231089 | |
4. | Ugo Avila-Ponce de León, Angel G. C. Pérez, Eric Avila-Vales, Modeling the SARS-CoV-2 Omicron variant dynamics in the United States with booster dose vaccination and waning immunity, 2023, 20, 1551-0018, 10909, 10.3934/mbe.2023484 | |
5. | Tianqi Song, Yishi Wang, Chuncheng Wang, Qi An, Modeling and analyzing COVID-19 infections in South Africa, 2025, 18, 1793-5245, 10.1142/S1793524523501036 |
Susceptible | Vaccinated | Recovered | Total | |
Delta | 20% | 8% (20*40%) | 9% (60*15%) | 88.6% |
Omicron | 20% | 20% (20*100%) | 48.6% (60*81%) | 37% |