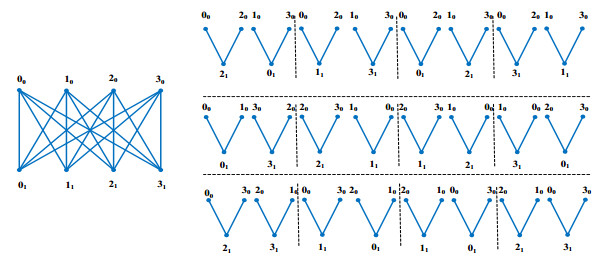
Modern medical diagnosis, treatment, or rehabilitation problems of the patient reach completely different levels due to the rapid development of artificial intelligence tools. Methods of machine learning and optimization based on the intersection of historical data of various volumes provide significant support to physicians in the form of accurate and fast solutions of automated diagnostic systems. It significantly improves the quality of medical services. This special issue deals with the problems of medical diagnosis and prognosis in the case of short datasets. The problem is not new, but existing machine learning methods do not always demonstrate the adequacy of prediction or classification models, especially in the case of limited data to implement the training procedures. That is why the improvement of existing and development of new artificial intelligence tools that will be able to solve it effectively is an urgent task. The special issue contains the latest achievements in medical diagnostics based on the processing of small numerical and image-based datasets. Described methods have a strong theoretical basis, and numerous experimental studies confirm the high efficiency of their application in various applied fields of Medicine.
Citation: Ivan Izonin, Nataliya Shakhovska. Special issue: informatics & data-driven medicine-2021[J]. Mathematical Biosciences and Engineering, 2022, 19(10): 9769-9772. doi: 10.3934/mbe.2022454
[1] | Tariq A. Alraqad, Hicham Saber . On the structure of finite groups associated to regular non-centralizer graphs. AIMS Mathematics, 2023, 8(12): 30981-30991. doi: 10.3934/math.20231585 |
[2] | Tao Cheng, Junchao Mao . A new class of directed strongly regular Cayley graphs over dicyclic groups. AIMS Mathematics, 2024, 9(9): 24184-24192. doi: 10.3934/math.20241176 |
[3] | Jiangmin Pan, Yingnan Zhang . On cubic semisymmetric bi-Cayley graphs on nonabelian simple groups. AIMS Mathematics, 2022, 7(7): 12689-12701. doi: 10.3934/math.2022702 |
[4] | Huani Li, Ruiqin Fu, Xuanlong Ma . Forbidden subgraphs in reduced power graphs of finite groups. AIMS Mathematics, 2021, 6(5): 5410-5420. doi: 10.3934/math.2021319 |
[5] | Chunqiang Cui, Jin Chen, Shixun Lin . Metric and strong metric dimension in TI-power graphs of finite groups. AIMS Mathematics, 2025, 10(1): 705-720. doi: 10.3934/math.2025032 |
[6] | Fawaz Aseeri, Julian Kaspczyk . The conjugacy diameters of non-abelian finite p-groups with cyclic maximal subgroups. AIMS Mathematics, 2024, 9(5): 10734-10755. doi: 10.3934/math.2024524 |
[7] | Huadong Su, Ling Zhu . Thickness of the subgroup intersection graph of a finite group. AIMS Mathematics, 2021, 6(3): 2590-2606. doi: 10.3934/math.2021157 |
[8] | Jean-Guy Caputo, Imene Khames, Arnaud Knippel . Nonlinear normal modes in a network with cubic couplings. AIMS Mathematics, 2022, 7(12): 20565-20578. doi: 10.3934/math.20221127 |
[9] | Nuttawoot Nupo, Sayan Panma . Certain structural properties for Cayley regularity graphs of semigroups and their theoretical applications. AIMS Mathematics, 2023, 8(7): 16228-16239. doi: 10.3934/math.2023830 |
[10] | Bilal A. Rather, Fawad Ali, Nasim Ullah, Al-Sharef Mohammad, Anwarud Din, Sehra . Aα matrix of commuting graphs of non-abelian groups. AIMS Mathematics, 2022, 7(8): 15436-15452. doi: 10.3934/math.2022845 |
Modern medical diagnosis, treatment, or rehabilitation problems of the patient reach completely different levels due to the rapid development of artificial intelligence tools. Methods of machine learning and optimization based on the intersection of historical data of various volumes provide significant support to physicians in the form of accurate and fast solutions of automated diagnostic systems. It significantly improves the quality of medical services. This special issue deals with the problems of medical diagnosis and prognosis in the case of short datasets. The problem is not new, but existing machine learning methods do not always demonstrate the adequacy of prediction or classification models, especially in the case of limited data to implement the training procedures. That is why the improvement of existing and development of new artificial intelligence tools that will be able to solve it effectively is an urgent task. The special issue contains the latest achievements in medical diagnostics based on the processing of small numerical and image-based datasets. Described methods have a strong theoretical basis, and numerous experimental studies confirm the high efficiency of their application in various applied fields of Medicine.
Many fundamental problems in combinatorics and related areas can be formulated as decomposition problems-where the aim is to decompose a large discrete structure into suitable smaller ones. Hence, problems of combinatorial design may be efficiently modeled from viewpoint of graph theory. In graph theory, the problems of edge decomposition and graph covering have a great attention as it is of immense importance for numerous applications to a wide range of areas [1,2,3]. Here we are concerned with the problem of detecting and correcting the error that may occur through the communication network (system). Despite communication systems may be robust, during the long process of transmitting data, external sources can coincide with the system and manipulate the data. Such manipulation leads to errors in transmitting data. The data received will be different from what was originally transmitted. Consequently, the ability to correct or at least detecting errors is an important issue to the system. The objective of the paper is to construct combinatorial designs which have efficient properties in detecting and correcting errors from viewpoint of coding theory. We call such designs Partially Balanced Network Design (PBNDs). Balanced incomplete block designs (BIBDs) were introduced in (1936). A BIBD is an arrangement of v objects (points) into b blocks each of size δ such that
1. Every object occurs at most once in each block.
2. Every object occurs in exactly α blocks. (α is the number of replications)
3. Every pair of objects occur together in exactly λ blocks.
Any BIBD has to satisfy the following conditions [3]:
b⩾v,vα=bδ,λ(v−1)=α(δ−1). |
v,b,α,δ,λ are called the parameters of a BIBD. A restriction on using the BIBD is that it is not available for all parametric combinations. Moreover, even if a BIBD is available for given v objects and block of size δ, it may require too many replications α. Another restriction that makes BIBD is not desirable for some experiments design is that BIBD is efficiency balanced, i.e., in BIBD every pair of distinct objects should occur together in exactly λ blocks. The partially balanced incomplete block designs (PBIBDs) compromise these restrictions up to some extent and help in reducing the number of replications. PBIBDs remain connected like BIBDs but no more balanced. Rather they are partially balanced in the sense that some pairs of objects have the same number of replications whereas some other pairs of objects have the same replications but are different from the replications of earlier pairs of objects. According to such enhancement, PBIBDs are applied for the design of experiments in many areas such as statistics [4], game theory [5], agriculture [6], cryptographic schemes [7], and many others. PBIBD was firstly studied by Bose in 1951. Bose et al., [8,9,10] introduced a class of binary, equireplicate and proper designs, which called PBIBD with m-associate classes. An associate class is a set of objects pairs where each pair from the set occur together with the same number of times, λi.
A PBIBD with m-associate classes is an arrangement of v objects in b blocks such that:
a) Each object from the set of objects occurs in α blocks.
b) Each block has δ objects (δ<v), and no object appears more than once in any block.
c) If two objects are i-th associates of each other then, they occur together in λi(i=1,2,…,m) blocks.
The number λi is independent of the particular pair of i-th associate chosen. λi doesn't need to all be different and some of λi's may be zero. PBIBD is studied and analyzed from viewpoint of graph theory. The relation between PBIBDs and strongly regular graphs is investigated in [8]. Bose and Nair [9] introduced the concept of association schemes in PBIBDs. Bose and Shimamoto [10] studied association schemes of PBIBDs using the graph-theoretic method. Regular graph design (RGD) is an important class of PBIBDs with two association schemes. An RGD (v,δ,α) is a collection of blocks of size δ on a v-set (with no restriction on repeated blocks) such that every object occurs in αblocks and any pair of objects occur together in either λ1 or λ2 blocks, where λ1 is some constant and λ2=λ1+1. There are extensive research for RGDs [11,12,13,14,15,16].
In this paper, we introduce a significant class of PBIBDs with two association classes with no repeated blocks. We call such a class a partially balanced network design (PBND). The aim of our paper is to show how PBND may be used to construct codes that process a high efficiency in detecting and correcting errors that may occur through the transmission process. The rest of the paper is organized as follows. Section 2 introduces the fundamentals and properties of PBND and the modeling of PBND as a graph design. Section 3 introduces the technique used to construct PBNDs that yield effective error detecting and correcting codes. Some direct constructions of PBNDs and general designs are constructed in Section 4. Section 5 studies the Cartesian product of designs and how that product will lead to codes able to detect and correct more errors. Section 6 shows the advantages of binary codes generated by PBNDs. In Section 7 we summarize the results and the conclusion of the paper.
Network Design (ND) is a pair (ω,τ), where ω={ω1,ω2,…,ωv} is the whole network (the universal set). The elements of ω are called points or objectives. τ={τ1,τ2,…,τb} is a collection of nonempty subsets of ω.Each element from τ is called a subnetwork. ND is described by five parameters (v,b,α,δ,λ), which are nonnegative integers and represent the following:
1) v the size of set ω.
2) b the number of subnetworks in τ.
3) α the number of subnetworks to which every point from ω belongs.
4) δ the size of each subnetwork.
5) λ the number of subnetworks that contain a pair of distinct points.
A network design (ND) is a complete-ND if δ=v, whenever δ<v it refers as incomplete-ND. Let λω1,ω2 be the number of subnetworks to which two points ω1,ω2 appear together. If λωi,ωj is unique for i,j with 1⩽i<j⩽v, we call such design as balanced design. If all λωi,ωj's are not identical for i,jwith 1⩽i<j⩽v, then the design is stated as partially balanced-ND. Here, we are interested in partially balanced incomplete network designs (PBNDs). For our designs we restrict λ⩽1. If λωi,ωj is a constant for i,j with 1⩽i<j⩽v, then the network design coincides with balanced incomplete block design (BIBD) and each subnetwork is viewed as a block of size δ. Through the paper we use (v,b,α,δ,λ)-ND for a network design (ND) with parameters (v,b,α,δ,λ).
Another form to express and determine a PBND is the incidence matrix. Let (ω,τ) be a PBND with parameters (v,b,α,δ,λ). The incidence matrix M=(mi,j) of a (v,b,α,δ,λ)-ND is a b×v binary matrix, where every row corresponds to a subnetwork, and every column corresponds to a point in the set ω.
mi,j={1;ωj∈τi,0;ωj∉τi. |
Theorem 1. Any PBND with parameters (v,b,α,δ,λ) should satisfy the following condition vα=bδ.
Proof. Consider the number of 1’s in the incidence matrix M of the given PBND. As matrix M inherited from its PBND then it has the following certain properties:
1) Every row has δ number of 1's.
2) Every column has α number of 1's.
3) Two distinct columns both have 1's in at most λ rows.
Since M has b rows and following property 1, then the number of 1's in the matrix is bδ. Now we are analyzing the columns of M. Property 2 and the number of columns of M imply that M has vα of 1's. Hence, vα=bδ.
We now review the connection between graph codes and PBND. When a PBND is transformed into an incidence matrix, the rows and the columns can be both viewed as binary codes. The binary code formed from the rows will be denoted as Crow, and the binary code formed from the columns will be referred as Ccolumn. The minimum distance in binary codes is a significant concept. The role of such a concept is to check whether the binary code can detect or correct errors [17,18,19,20].
Let C be a binary code where every element (coding word) belongs to C has length n. Let P=(p1,p2,...,pn) and Q=(q1,q2,...,qn) be two coding words from C. The minimum distance d(P,Q) between P and Q is calculated from the following relation:
d(P,Q)=n∑i=1d(pi,qi),d(pi,qi)={1;pi≠qi,0;pi=qi. | (1) |
According to relation (1), the minimum distance between two coding words P and Q defines the number of positions where P and Q differ i.e. pi≠qi for 1⩽i⩽n. The minimum distance θ of a code C is defined as:
θ(C)=min{d(P,Q);P,Q∈C,P≠Q}. | (2) |
In the following sections, we show how to use the minimum distance in detecting and correcting errors of constructed codes. All graphs described here are finite, undirected, without loops and multiple edges. Let V(H) and E(H) be the vertex set and edge set of the graph H, respectively. The degree of a vertex u∈V(H) is the number of vertices from V(H) that are adjacent to u. The graph is regular if and only if all vertices have a unique degree.
Hereafter, we illustrate the modeling of PBND in terms of graphs.
Definition 1. Let H be a s-regular graph of ordern. An (H,F,k,X)-graph design is a collection φ={F0,F1,…,Fb−1} of b subgraphs of H such that:
1) For every 0⩽i⩽b−1;Fi≅F.
2) For every 0⩽i⩽b−1;Fi is a spanning subgraph of H.
3) Every Fi contains at most s edges.
4) Every edge of H exists in exactly k subgraphs from φ.
5) For any i,j such that i≠j,Fi∩Fj≅X.
From Definition 1 an (H,F,k,X)-graph design is a PBDN. There is a correspondence between the parameters (H,F,k,X) of a graph design and the parameters for the corresponding PBDN. Namely, v=|E(H)|,b=|φ|,α=k,δ=|E(F)| and λ=|E(X)|. According to that correspondence, in the following we use (H,F,k,X)-ND for an (H,F,k,X)-graph design. Moreover, if the graph H is a complete bipartite graph Kn,n,X≅K1,1 and |E(F)|<n then (Kn,n,F,k,K2)-ND is a sub-orthogonal double cover (SODC) of Kn,nby F. The construction of SODCs of Kn,n and Kn by Ffor different classes of F has been studied in [21,22,23,24,25]. The design (Kn,n,F,k,K2)-ND is equivalent to an orthogonal double cover (ODC) of Kn,n by F if and only if |E(F)|=n, see [26,27,28,29,30,31,32,33,34,35]. If H is a Cayley graph and |E(F)|=s<n,then the constructed design (Kn,n,F,k,K2)-ND is considered as an ODC of Cayley graphs which have been studied in [36]. For the (H,F,k,X)-ND, if the graph H is a complete bipartite graph Kn,n,k⩾3,X≅K2,and |E(F)|=n, then (Kn,n,F,k,K2)-ND is a mutually orthogonal k cover (MOkC) of Kn,n by F, see [37,38,39,40,41,42,43,44]. In [45], the authors have constructed the circular intensely ODC design of balanced complete multipartite graphs. Additional background material for this field may be found in [46,47,48,49,50]. For many communication systems (networks), bipartite graphs are good tools in modeling such systems. In the next section, we are concerned with (H,F,k,X)-ND for a network H modeled as a bipartite graph and refer to it as a bipartite network.
The complete bipartite network Kn,n consists of two independent sets of nodes. The first set of the nodes is labeled by {0,1,...,n−1}×{0}, and the second set of the nodes is labeled by {0,1,...,n−1}×{1}. Each link u0v1 in the network has a weight equals to v−u (sums and differences are calculated modulon).
The network design (H,F,k,X)-ND is a collection G={Gij0,Gij1,...,Gijn−1};i∈{0,1,...,n−1},j∈{0,1,...,k−1} of kn isomorphic subnetworks (each of them is isomorphic to the networkF)of the complete bipartite network Kn,n such that:
a) For a certain j=η,j∈{0,1,...,k−1}, every link of the complete bipartite network Kn,n is found in exactly one subnetwork of {Giη0,Giη1,...,Giηn−1}.
b) For any two subnetworks Gawc,Gxyz from the collection G, if a≠x,w=y,c≠z, then there are no common links between these two subnetworks.
c) For any two subnetworks Gawc,Gxyz from the collection G, if w≠y, then these two subnetworks intersect in a common network which is isomorphic to the subnetwork X.
In the (H,F,k,X)-ND, we have v=|E(Kn,n)|=n2,b=k|E(F)|=kn,α=k,δ=|E(F)|=n,X≅K1,1. Then, vα=kn2,bδ=kn2⇒vα=bδ.
The incidence matrix I=(Iij) for the (Kn,n,F,k;K1,1)-ND is a kn×n2 binary matrix showing the relation between the links and the subnetworks of the complete bipartite network Kn,n,where every row in I corresponds to a subnetwork Fi in (Kn,n,F,k;K1,1)-ND and every column in I corresponds to a link lj in the complete bipartite network Kn,n,
Iij={1;lj∈Fi,0;lj∉Fi. |
Any code word from Crow represents a bipartite graph that is isomorphic to the graph F. In this case, the Crow is called a F-graph code. The rules of building the incidence matrix I=(Iij) of (H,F,k,X)-ND lead to the following lemma.
Lemma 1. An F-graph code exists if and only if the (H,F,k,X)-ND is available.
Moreover, since I is a binary matrix, then its rows or columns can be used as binary codes. For instance, the encoding function of the code Ccolumn is defined as; with W=Z2n, let encoding function E:W→Zkn2, be given by E(ij)=Cni+j(I) (the (ni+j)th column of the matrix I). Section 4 contains examples explain the methodology of the coding and decoding processes with the help of the minimum distance of the considered code.
Definition 2. ([18]) For m,λ∈Z+ and ε∈Zm2, the sphere of radius λ centered at ε is defined as S(ε,λ)={γ∈m2:d(ε,γ)≤λ}.
Theorem 2. ([18]) Supposeis E:W→C is an encoding function where W⊆Zn2 is the set of messages and E(W)=C⊆Zm2 is the set of code words with n<m. If the minimum distance between code words is at least λ+1,λ∈Z+, then we can detect all transmission errors of weight ≤λ.
Theorem 3. ([18]) Suppose E:W→C is an encoding function where W⊆Zn2 is the set of messages and E(W)=C⊆Zm2 is the set of code words with n<m. If the minimum distance between code words is at least 2λ+1,λ∈Z+, then we can construct a decoding function D:Zm2→W that corrects all transmission errors of weight ≤λ.
The following theorem gives the range of errors that can be detected or corrected when either Crowor Ccolumn is used.
Theorem 4. Let Crow, Ccolumn be the binary codes constructed from the rows and columns of the incidence matrix of (Kn,n,F,k;K1,1)-ND, respectively. We have the following:
1) For the binary code Crow, we can detect up to 2n−3 errors or correct up to ⌊2n−32⌋ errors.
2) For the binary code Ccolumn, we can detect up to 2k−3 errors or correct up to ⌊2k−32⌋ errors.
Proof. From the definition of the (Kn,n,F,k;K1,1)-ND, in the incidence matrix of (Kn,n,F,k;K1,1)-ND, there exists n number of 1's in every row. Any two rows have at most 1 position of 1's in common. Then the minimum distance of Crow is θ(Crow)=2(n−1)=2n−2. Therefore, for Crow, we can detect up to θ(Crow)−1=2n−3 errors and correct up to ⌊θ(Crow)−12⌋=⌊2n−32⌋. Considering Ccolumn there exists k number of 1's in every column. Any two columns have at most 1 position of 1's in common, then the minimum distance of Ccolumn is θ(Ccolumn)=2(k−1)=2k−2. Therefore, for Ccolumn we can detect up to (Ccolumn)−1=2k−3 and correct up to ⌊θ(Ccolumn)−12⌋=⌊2k−32⌋.
Method for constructing (Kn,n,F,k;K1,1)-ND
In what follows, we are concerned with the links of the network G. Consequently, we will use G for the links set of G.
Definition 3. If we have a subnetwork G={(u10,v11),(u20,v21),(u30,v31),...,(un0,vn1)} (the weights of these links are mutually distinct and equal to {0,1,…,n−1}) of the complete bipartite network Kn,n, then the translated subnetwork G+σ={(u10+σ,v11+σ),(u20+σ,v21+σ),(u30+σ,v31+σ),...,(un0+σ,vn1+σ)} is called the G-translate of the subnetwork G where σ∈{0,1,...,n−1}. The union of the subnetworks G+σ gives the complete bipartite network Kn,n. The subnetwork G will be called the basis of the complete bipartite network Kn,n.
In what follows, the basis G will be represented by a vector ϕ(G)=(φ0,φ1,…,φn−1) where ϕx∈{0,1,...,n−1} and (ϕx)0 is the unique node (ϕx,0)∈{0,1,...,n−1}×{0} that belongs to the unique link of weight x in the basis G Note that the links of the basis Gare {(ϕx)0,(ϕx+x)1}, which will be represented by ((ϕx)0,(ϕx+x)1).
Definition 4. The two bases G and H represented by the vectors ϕ(G) and ψ(H) are said to be intersected if {ϕi(G)−ψi(H):i∈{0,1,…,n−1}}={0,1,...,n−1}.
Definition 5. If the two bases vectors ϕ(G) and ψ(H) are intersected and G≅H, then the translated subnetworks G+σ and H+σ,σ∈{0,1,...,n−1} gives a (Kn,n,G,2;K1,1)-ND.
Definition 6. The subnetwork Hs of the complete bipartite network Kn,n is the symmetric subnetwork of the subnetwork H if H={(u10,v11),(u20,v21),(u30,v31),...,(un0,vn1)},Hs={(v10,u11),(v20,u21),(v30,u31),...,(vn0,un1)}. It is clear that if the subnetwork H is a basis, then the subnetwork Hs is also a basis. If the two bases H and Hs are intersected, then the basis H is called a symmetric basis.
Definition 7. The basis H represented by the vector ψ(H) is a symmetric basis if {ψτ(H)−ψn−τ(H)+τ:τ∈{0,1,...,n−1}}={0,1,…,n−1}.
In this section, we introduce some small PBNDs based on the direct construction method. Also, we introduce some generalized PBNDs.
Lemma 2. There exists a (K4,4,2K1,2,3;K1,1)-ND. Hence, there is a 2K1,2-code.
Proof. Let G={G000,G101,G202,G303,G010,G111,G212,G313,G020,G121,G222,G323}with G000={0021,2021,1001,3001},G101={0011,2011,1031,3031},G202={0001,2001,1021,3021}, G303={0031,2031,1011,3011},G010={0001,1001,3031,2031},G111={2021,3021,1011,0011}, G212={2011,3011,1021,0021},G313={1031,0031,2001,3001},G020={0021,3021,2031,1031},G121={0011,3011,2001,1001},G222={2011,1011,0001,3001},G322={2021,1021,0031,3031}, see Figure 1. All the subnetworks are isomorphic to 2K1,2. Let i0j1 be a link from the complete bipartite network K4,4. The constructed design forces every link i0j1 to occur in exactly 3 subnetworks from G. Moreover, such design fulfills the following total function ∩:A→{0,1}, where A={Gawc,Gxyz} be a set of 2-elements subsets of G and ∩({Gawc,Gxyz})={1;w≠y,0;a≠x,w=y,c≠z. Here, ∩({Gawc,Gxyz})=1 means that the intersection of two subnetworks Gawc,Gxyz is isomorphic to subnetwork K1,1, and ∩({Gawc,Gxyz})=0 refers that they have no subnetworks in common.
The incidence matrix Ifor (K4,4,2K1,2,3;K1,1)-ND is
I((K4,4,2K1,2,3;K1,1)−ND)=F0F1F2F3F4F5F6F7F8F9F10F11[001010000010010001000100100000101000000100010001100010000100000101000010001000100001010000011000001000010100010000100010100001000001000110001000100000010010000100100100001001001000010010000001]. |
Example 1. From Lemma 2,
W=Z24={0,1,2,3}×{0,1,2,3}={00,01,02,03,10,11,12,13,20,21,22,23,30,31,32,33},
Example 1. From Lemma 2, W=Z24={0,1,2,3}×{0,1,2,3}={00,01,02,03,10,11,12,13,20,21,22,23,30,31,32,33},E:W→Z122,E(ij)=C4i+j(I), hence,
E(00)=C0(I)=001010000010,E(01)=C1(I)=010001000100,E(02)=C2(I)=100000101000,E(03)=C3(I)=000100010001,E(10)=C4(I)=100010000100,E(11)=C5(I)=000101000010,E(12)=C6(I)=001000100001,E(13)=C7(I)=010000011000,E(20)=C8(I)=001000010100,E(21)=C9(I)=010000100010,E(22)=C10(I)=100001000001,E(23)=C11(I)=000110001000,E(30)=C12(I)=100000010010,E(31)=C13(I)=000100100100,E(32)=C14(I)=001001001000,E(33)=C15(I)=010010000001.
The minimum distance between code words is 4, so we can correct all single and double errors. For instance,
S(C0(I),2)={001010000010}∪{101010000010,011010000010,001110000010,001011000010,001010100010,001010010010,001010001010,001010000110,001010000011}∪{111010000010,101110000010,101011000010,101010100010,101010010010,101010001010,101010000110,101010000011,011110000010,011011000010,011010100010,011010010010,011010001010,011010000110,001110010010,001110001010,001110000110,011010000011,001111000010,001110100010,001110000011,001011100010,001011010010,001011001010,001011000110,001011000011,001010110010,001010101010,001010100110,001010100011,001010011010,001010010110,001010010011,001010001110,001010001011,001010001110,001010001011,001010000111}.
The decoding function D:Z122→W gives D(ε)=C0(I)=001010000010 for allε∈S(C0(I),2).
Lemma 3. There exists a (K4,4,K2,2,3;K1,1)-ND. Hence, there is a K2,2-code.
Proof. Let G={G000,G101,G202,G303,G010,G111,G212,G313,G020,G121,G222,G323} with G000={2001,2031,1001,1031},G101={3011,3021,0011,0021},G202={3031,3001,0031,0001}, G303={1011,1021,2011,2021},G010={1021,1001,3021,3001},G111={2011,2031,0011,0031}, G212={2021,2001,0021,0001},G313={1011,1031,3011,3031},G020={2021,2031,3021,3031}, G121={1021,1031,0021,0031},G222={2011,2001,3011,3001},G322={1011,1001,0011,0001}. All the subnetworks are isomorphic to K2,2. Let i0j1 be a link from the complete bipartite network K4,4.The constructed design forces every link i0j1 to occur in exactly 3 subnetworks from G. Moreover, such design fulfills the following total function ∩:A→{0,1}, where A={Gawc,Gxyz} be a set of 2-elements subsets of G and ∩({Gawc,Gxyz})={1;w≠y,0;a≠x,w=y,c≠z.
Here, ∩({Gawc,Gxyz})=1 means that the intersection of two subnetworks Gawc,Gxyz is isomorphic to subnetwork K1,1 and ∩({Gawc,Gxyz})=0 refers that they have no subnetworks in common.
Theorem 5. There exists a (Kn,n,Pn+1,n;K1,1)-ND where n is a prime number.
Proof. We define ψτ(Hx)=τ(x−τ) where τ,x∈{0,1,…,n−1}.Assume that p≠q∈{0,1,…,n−1},then ψτ(Hp)−ψτ(Hq)=τ(p−q);τ∈{0,1,…,n−1}. It is easy to check that the differences ψτ(Hp)−ψτ(Hq) give the group {0,1,…,n−1} since n is a prime and p−q is not equal to zero. Hence, Hp and Hq are intersected. Now, we will prove that all the bases Hp,p∈{0,1,…,n−1} are paths. The basis Hp={(τ(p−τ))0,(τ(p−τ)+τ)1:τ,p∈{0,1,…,n−1}}, it is trivial to show that Hp is a path at n=2,forn>2,assume that r=s/2, then for each λ∈{0,1,…,n−1},lλ=((λ+r)(s−r−λ))0,((λ+r)(s−r−λ)+λ+r)1), where lλ is the link of weight λ+r. Consider the two links lλ and lμ with λ≠μ, these two links are adjacent and incident in the node with zero subscript if (λ+r)(s−r−λ)=(μ+r)(s−r−μ). Putting s=2rgives (λ+μ)(λ−μ)=0. This means that λ+μ=0 since λ≠μ. Now, we can conclude that lλ=l−μ=ln−μ. Hence, there is only value of μ verifies the previous claim provided that λ≠0. Similarly, the two links lλ and lμ with λ≠μ are adjacent and incident in the node with one subscript if (λ+r)(s−r−λ)+(λ+r)=(μ+r)(s−r−μ)+(μ+r). This gives (λ+μ−1)(λ−μ)=0. Hence, lμ=l1−λ and there is only value of μ verifies the previous claim provided that λ≠1/2. Finally, the sequence l0,l1,ln−1,l2,ln−2,…,l(n−1)/2,ln−(n−1)/2represents a path.
Lemma 4. There exists a(K3,3,P4,3;K1,1)-ND. Hence, there is a P4-code, where P4 is a path graph with four vertices and three edges.
Proof. Let G={G000,G101,G202,G010,G111,G212,G020,G121,G222} with G000={0001,0011,1001}, G101={2001,2021,0021},G202={1021,1011,2011},G010={0001,0021,1021},G111={0011,2011,2021}, G212={1001,2001,1011},G020={0001,2001,2011},G121={0011,0021,1011},G222={1001,1021,2021}. All the subnetworks are isomorphic to P4. Let i0j1 be a link from the complete bipartite network K3,3.The constructed design forces every link i0j1 to occur in exactly 3 subnetworks from G. Moreover, such design fulfills the following total function ∩:A→{0,1}, where A={Gawc,Gxyz} be a set of 2-elements subsets of G and ∩({Gawc,Gxyz})={1;w≠y,0;a≠x,w=y,c≠z.
Here, ∩({Gawc,Gxyz})=1 means that the intersection of two subnetworks Gawc,Gxyz is isomorphic to subnetwork K1,1, and ∩({Gawc,Gxyz})=0 refers that they have no subnetworks in common.
In what follows, the link (a0,b1) will be written as (a,b) for simplification.
Theorem 6. There exists a (Kn,n,n−12P3∪K1,1,n;K1,1)-ND where n>2 is a prime number. Hence, there is a (n−12P3∪K1,1)-code.
Proof. Define all subnetworks of a (Kn,n,n−12P3∪K1,1,n;K1,1)-ND by the following table where x∈{0,1,…,n−1}.
From Table 1, we have a collection G={Gij0,Gij1,...,Gijn−1};i,j∈{0,1,...,n−1} of n2 subnetworks. Now, we want to prove the isomorphism of all subnetworks in Table 1. Suppose ε∈{0,1,...,n−1}is a fixed number and ξ∈{0,1,...,n−1}is an arbitrary number. By taking the subnetworks in the first column of Table 1, we have to prove that x2+εx=ξ has a unique solution for exactly one ξ, two solutions for n−12ξ's, and no solution for the remaining n−12ξ's. There is a numberh∈{0,1,...,n−1} with h=2ε since n>2 is a prime. Hence, (x+h)2=h2+ξ from the equation x2+εx=ξ. Now, we have a unique solution x=−h if h2+ξ=0.For any other h2+ξ, the desired property is verified. Similarly, the isomorphism for the remaining subnetworks in Table 1 can be proved. Hence, all subnetworks in Table 1 are isomorphic to n−12P3∪K1,1. It is clear that the subnetworks in the same row in Table 1 have no common links and any two subnetworks from two different rows have only one common link.
The elements of the collection G in Theorem 6 | |||
G000={(x,x2)} | G101={(x,x2+1)} | … | G(n−1)0n−1={(x,x2+n−1)} |
G010={(x,x2+x)} | G111={(x,x2+x+1)} | … | G(n−1)1n−1={(x,x2+x+n−1)} |
G020={(x,x2+2x)} | G121={(x,x2+2x+1)} | … | G(n−1)2n−1={(x,x2+2x+n−1)} |
⋮ | ⋮ | ⋮ | ⋮ |
G0(n−1)0={(x,x2+(n−1)x)} | G1(n−1)1={(x,x2+(n−1)x+1)} | … | G(n−1)(n−1)n−1={(x,x2+(n−1)x+n−1)} |
Lemma 5. There exists a (K3,3,P3∪K1,1,3;K1,1)-ND. Hence, there is a P3∪K1,1-code.
Proof. LetG={G000,G101,G202,G010,G111,G212,G020,G121,G222} with G000={0001,0011,2021}, G101={1001,1011,0021},G202={2001,2011,1021},G010={0001,0021,2011},G111={1001,1021,0011}, G212={2001,2021,1011},G020={1011,1021,0001},G121={2011,2021,1001},G222={0011,0021,2001}. All the subnetworks are isomorphic to P3∪K1,1. Let i0j1 be a link from the complete bipartite network K3,3.The constructed design forces every link i0j1 to occur in exactly 3 subnetworks from G. Moreover, such design fulfills the following total function ∩:A→{0,1}, where A={Gawc,Gxyz} be a set of 2-elements subsets of G and ∩({Gawc,Gxyz})={1;w≠y,0;a≠x,w=y,c≠z.
Here, ∩({Gawc,Gxyz})=1 means that the intersection of two subnetworks Gawc,Gxyz is isomorphic to subnetwork K1,1, and ∩({Gawc,Gxyz})=0 refers that they have no subnetworks in common.
Theorem 7. There exists a (Kn,n,nK1,1,n−1;K1,1)-ND where n is a prime number. Hence, there is a (nK1,1)-code.
Proof. Define all subnetworks of a (Kn,n,nK1,1,n−1;K1,1)-ND by the following table wherex∈{0,1,…,n−1}.
From Table 2, the collection G={Gij0,Gij1,...,Gijn−1};i∈{0,1,...,n−1},j∈{0,1,...,n−2} of n(n−1) subnetworks. Now, we want to prove the isomorphism of all subnetworks in Table 2. By taking the subnetworks in the first column of Table 2, for all ε∈{0,1,...,n−1}, we have gcd(ε,n)=1. Hence, the nodes εx are mutually distinct in {1,2,...,n−1} and all the subnetworks in the first column of Table 2 are isomorphic to nK1,1. Similarly, the isomorphism for the remaining subnetworks in Table 2 can be proved. Hence, all subnetworks in Table 2 are isomorphic to (nK1,1). It is clear that the subnetworks in the same row in Table 2 have no common links and any two subnetworks from two different rows have only one common link.
The elements of the collection G in Theorem 7 | |||
G000={(x,x)} | G101={(x,x+1)} | … | G(n−1)0n−1={(x,x+n−1)} |
G010={(x,2x)} | G111={(x,2x+1)} | … | G(n−1)1n−1={(x,2x+n−1)} |
G020={(x,3x)} | G121={(x,3x+1)} | … | G(n−1)2n−1={(x,3x+n−1)} |
⋮ | ⋮ | ⋮ | ⋮ |
G0(n−2)0={(x,(n−1)x)} | G1(n−2)1={(x,(n−1)x+1)} | … | G(n−1)(n−2)n−1={(x,(n−1)x+n−1)} |
Theorem 8. There exists a (Kn,n,(n−2)K1,1∪K1,2,n−1;K1,1)-ND where n is a prime number. Hence, there is a (n−2)K1,1∪K1,2-code.
Proof. Define all subnetworks of a (Kn,n,(n−2)K1,1∪K1,2,n−1;K1,1)-ND by the following table wherex∈{0,1,…,n−1}.
From Table 3, the collection G={Gij0,Gij1,...,Gijn−1};i∈{0,1,...,n−1},j∈{0,1,...,n−2} of n(n−1)subnetworks. Now, we want to prove the isomorphism of all subnetworks in Table 3. By taking the subnetworks in the first column of Table 3, for all ε∈{0,1,...,n−1}, we have gcd(ε,n)=1. Hence, the nodes εx are mutually distinct in {1,2,...,n−1} and the nodes εx+1 are mutually distinct in {1,2,...,n−1}. Together with the link (0,0) we conclude that every subnetwork in the first column of Table 3 is isomorphic to ((n−2)K1,1∪K1,2).Similarly, the isomorphism for the remaining subnetworks in Table 3 can be proved. Hence, all subnetworks in Table 3 are isomorphic to ((n−2)K1,1∪K1,2). It is clear that the subnetworks in the same row in Table 3 have no common links and any two subnetworks from two different rows have only one common link.
The elements of the collection G in Theorem 8 | |||
G000={(0,0),(x,x+1)} | G101={(0,1),(x,x+2)} | … | G(n−1)0n−1={(0,n−1),(x,x+n)} |
G010={(0,0),(x,2x+1)} | G111={(0,1),(x,2x+2)} | … | G(n−1)1n−1={(0,n−1),(x,2x+n)} |
G020={(0,0),(x,3x+1)} | G121={(0,1),(x,3x+2)} | … | G(n−1)2n−1={(0,n−1),(x,3x+n)} |
⋮ | ⋮ | ⋮ | ⋮ |
G0(n−2)0={(0,0),(x,(n−1)x+1)} | G1(n−2)1={(0,1),(x,(n−1)x+2)} | … | G(n−1)(n−2)n−1={(0,n−1),(x,(n−1)x+n)} |
Theorem 9. There exists a (K9,9,3K1,2∪K1,3,3;K1,1)-ND. Hence, there is a 3K1,2∪K1,3-code.
Proof. Define all subnetworks of a (K9,9,3K1,2∪K1,3,3;K1,1)-ND by the following table wherex∈{0,1,…,8}.
From Table 4, the collection G={Gij0,Gij1,...,Gij8};i∈{0,1,...,8},j∈{0,1,2} of 24 subnetworks. It is clear that all the subnetworks in the first column of Table 4 are isomorphic to the network (3K1,2∪K1,3). Similarly, the isomorphism for the remaining subnetworks in Table 4 can be proved. Hence, all subnetworks in Table 4 are isomorphic to (3K1,2∪K1,3). It is clear that the subnetworks in the same row in Table 4 have no common links and any two subnetworks from two different rows have only one common link.
The elements of the collection G in Theorem 9 | |||
G000={(x,x2)} | G101={(x,x2+1)} | … | G808={(x,x2+8)} |
G010={(x,x2+x)} | G111={(x,x2+x+1)} | … | G818={(x,x2+x+8)} |
G020={(x,x2+2x)} | G121={(x,x2+2x+1)} | … | G828={(x,x2+2x+8)} |
Theorem 10. There exists a (K12,12,2K1,2∪2K1,4,2;K1,1)-ND. Hence, there is a 2K1,2∪2K1,4-code.
Proof. Define all subnetworks of a (K12,12,2K1,2∪2K1,4,2;K1,1)-ND by the following table wherex∈{0,1,…,11}.
From Table 5, the collection G={Gij0,Gij1,...,Gij11};i∈{0,1,...,11},j∈{0,1} of 24 subnetworks. It is clear that all the subnetworks in the first column of Table 5 are isomorphic to the network (2K1,2∪2K1,4). Similarly, the isomorphism for the remaining subnetworks in Table 4 can be proved. Hence, all subnetworks in Table 5 are isomorphic to (2K1,2∪2K1,4). It is clear that the subnetworks in the same row in Table 5 have no common links and any two subnetworks from two different rows have only one common link.
The elements of the collection G in Theorem 10 | |||
G000={(x,x2)} | G101={(x,x2+1)} | … | G11,011={(x,x2+11)} |
G010={(x,x2+x)} | G111={(x,x2+x+1)} | … | G11,111={(x,x2+x+11)} |
Theorem 11. Let ϕ(G)=(φ0,φ1,…,φn−1) be a symmetric basis of (Kn,n,G,2;K1,1)-ND and ψ(H)=(ψ0,ψ1,…,ψm−1) be a symmetric basis of (Km,m,H,2;K1,1)-ND, then the Cartesian productϕ(G)×ψ(H)=(φ0,φ1,…,φn−1)×(ψ0,ψ1,…,ψm−1)=(φ0ψ0,φ0ψ1,…,φρψυ,…,φn−1ψm−1) where ρ∈{0,1,...,n−1},v∈{0,1,...,m−1} is a symmetric basis of (Kmn,mn,G×H,2;K1,1)-ND.
Proof. Since ϕ(G)=(φ0,φ1,…,φn−1) is a symmetric basis of (Kn,n,G,2;K1,1)-ND, then
{ϕρ(G)−ϕn−ρ(G)+ρ:ρ∈{0,1,...,n−1}}={0,1,...,n−1}. | (3) |
Since ψ(H)=(ψ0,ψ1,…,ψm−1)is a symmetric basis of (Km,m,H,2;K1,1)-ND, then
{ψv(H)−ψm−v(H)+v:v∈{0,1,...,m−1}}={0,1,...,m−1}. | (4) |
Then, ϕ(G)×ψ(H)=(φ0,φ1,…,φn−1)×(ψ0,ψ1,…,ψm−1)=(φ0ψ0,φ0ψ1,…,φρψυ,…,φn−1ψm−1) where ρ∈{0,1,...,n−1},v∈{0,1,...,m−1}.From (3) and (4), we can conclude {ϕρψv−ϕn−ρψm−v+ρv:ρv∈{0,1,...,n−1}×{0,1,...,m−1}}={(ϕρ(G)−ϕn−ρ(G)+ρ)(ψv(H)−ψm−v(H)+v):ρ∈{0,1,...,n−1},v∈{0,1,...,m−1}}={0,1,...,n−1}×{0,1,...,m−1}.Then, ϕ(G)×ψ(H) is a symmetric basis of (Kmn,mn,G×H,2;K1,1)-ND. The incidence matrix of (Kn,n,G,2;K1,1)-ND has 2n rows and n2 columns and the incidence matrix of (Km,m,H,2;K1,1)-ND has 2m rows and m2 columns. The incidence matrix of (Kmn,mn,G×H,2;K1,1)-ND has 2mn rows and n2×m2columns. Hence, |Ccolumn|=n2×m2 and |Crow|=2mn. When Crow is used for coding, we can detect up to θ(Crow)−1=2mn−3 errors and correct up to ⌊θ(Crow)−12⌋=⌊2mn−32⌋.
Example 2. Let ϕ(P3)=(0,0) be a symmetric basis of (K2,2,P3,2;K1,1)-ND and ψ(P4)=(0,1,1) be a symmetric basis of (K3,3,P4,2;K1,1)-ND, then the Cartesian product ϕ(P3)×ψ(P4)=(0,0)×(0,1,1)=(00,01,01,00,01,01) is a symmetric basis of (K6,6,P3×P4,2;K1,1)-ND. Note that if ij∈ϕ(P3)×ψ(P4),then f(ij)=3i+j gives a compact representation for the vector ϕ(P3)×ψ(P4) which is (0,1,1,0,1,1).
Combinatorial designs and several related structures can be used to generate codes based on the incidence matrix. Many interesting and useful results have been provided as a result of the interplay between designs and codes. For an excellent survey on the topic, see [51]. Recently there is more attention of codes generating from graphs. The connection of codes and graphs has been explored from different aspects in the literature. The main objective in these works is to take a special class of graphs and construct codes from the adjacency matrix of the graph. Nice codes can be generated by some strongly regular graphs [52,53]. Binary codes can be generated by different graphs such as Paley graphs, the block graphs of Steiner 2-designs, and the Latin square graphs [54]. Non-isomorphic codes have been produced by non-isomorphic graphs, see [54]. The structure of the graph may lead to different types of codes as a result, such as self-dual codes, self-orthogonal codes, etc. We refer the reader to [55,56,57,58,59,60,61] for some of these works. Higazy et al. [62] studied the group-generated graph designs for certain circulant graphs and the generation of codes from such designs. Here, we examine binary codes obtained from the row or column span of the incidence matrices of some graphs that occur as induced subgraphs of the complete bipartite graphs. In this paper, we introduce a method from which we can generate a special type of orthogonal codes. These codes are depending on the orthogonality of graphs found in the design (Kn,n,F,k;K1,1). Two graphs are orthogonal if they intersect in at most one edge. Our method constructs binary codes satisfying that the inner product of any two codewords ≤1. We introduce PBNDs that lead to efficient error detection and correction codes. In order to increase the dimension of code and keeping efficient properties for error detection and correction, we introduce a technique that generates codes based on the Cartesian product of earlier designs. We should notify the reader that our method for such a product differs from those studied in [63].
In this paper, we studied binary codes based on PBNDs, (Kn,n,F,k;K1,1). For instances, the advantages of binary codes generated by (Kn,n,F,2;K1,1) are as follows: From the binary codeword generator of length n2, we can get 2n−1 new binary codewords of length n2, this can be shown as follows, where these codewords represent the rows of the incidence matrix of (Kn,n,F,2;K1,1).
Definition 8. For the binary codeword with length n2,vij is called a binary codeword generator, if (j−i) gives {0,1,...,n−1} for ij with which vij=1.
Definition 9. If v0ij=1, then vx(i+x)(j+x)=1,x∈{0,1,...,n−1} is called x−translate of v0.
Example 3. Let x∈Zn,vxij={1;ij∈A={x}×Zn,0;ij∈Z2n∖A. and uxij={1;ij∈B=Zn×{x},0;ij∈Z2n∖B, the rows of the following matrix M represent 2n binary codewords of length n2,M=[v0ijv1ij⋮vn−1iju0iju1ij⋮un−1ij].
Put n=4, then x∈Z4,vxij={1;ij∈A={x}×Z4,0;ij∈Z24∖A. and uxij={1;ij∈B=Z4×{x},0;ij∈Z24∖B, the rows of the following matrix M represent 8 binary codewords of length 16. Also, if vxij=1, then there is a graph corresponding to this vector with the edge set E(Gx)={(i0,j1):i,j∈Z4}, see Figure 2.
M=[11110000000000000000111100000000000000001111000000000000000011111000100010001000010001000100010000100010001000100001000100010001]. |
Also we can add the following to the advantages of PBNDs: For the generalized binary codes generated by the Cartesian products of PBNDs. The Cartesian products of PBNDs is an easier method than the previously defined methods for constructing new binary codes from the old ones [64].
In the paper, we propose a novel technique for constructing a partially balanced network design (PBND). These networks are used to model the information transmission represented by strings of the signals zero and one. Certain problems appear in the transmission of information in digital communications when this information is transmitted in the form of strings of zeros and ones. The noise in the transmission channel changes the original transmitted signal to a different signal. Therefore, the receiver takes a wrong decision. The PBND is a helping tool that manages us to detect and correct the errors in the coded messages. A graphical model is introduced for the PBND. Also, the PBNDs are represented by their corresponding incidence matrices. These matrices compromise new and several codes. The nodes of these networks are labeled by the Cartesian product of additive abelian groups. Some direct constructions of small and generalized PBND are introduced. Finally, we prove that the Cartesian product of two small symmetric bases gives a larger symmetric basis that generates new codes with larger lengths. The Cartesian products of PBNDs is an easier method than the previously defined methods for constructing new binary codes from the old ones. The following table presents the summary of the results in the paper. It should be noted thatpis a prime number in Table 6.
Kn,n | F | k | X |
K3,3 | P4 | 3 | K1,1 |
K3,3 | P3∪K1,1 | 3 | K1,1 |
K4,4 | 2K1,2 | 3 | K1,1 |
K4,4 | K2,2 | 3 | K1,1 |
Kp,p | Pp+1 | p | K1,1 |
Kp,p | p−12P3∪K1,1 | p | K1,1 |
Kp,p | pK1,1 | p−1 | K1,1 |
Kp,p | (p−2)K1,1∪K1,2 | p−1 | K1,1 |
K9,9 | 3K1,2∪K1,3 | 3 | K1,1 |
K12,12 | 2K1,2∪2K1,4 | 2 | K1,1 |
This research was supported by Taif University Researchers Supporting Project Number (TURSP-2020/155), Taif University, Taif, Saudi Arabia.
The authors declare no conflict of interest.
[1] | N. Shakhovska, A. Salazar, I. Izonin, J. Campos, IDDM 2021: Proceedings of the 4th International Conference on Informatics & Data-Driven Medicine Valencia, Spain, November 19-21, 2021, CEUR-WS.org, 2021, 3038. Available from: http://ceur-ws.org/Vol-3038/ |
[2] |
X. Liu, V. Krylov, S. Jun, N. Volkova, A. Sachenko, G. Shcherbakova, et al., Segmentation and identification of spectral and statistical textures for computer medical diagnostics in dermatology, Math. Biosci. Eng., 19 (2022), 6923-6939. https://doi.org/10.3934/mbe.2022326 doi: 10.3934/mbe.2022326
![]() |
[3] |
Y. Bodyanskiy, O. Chala, N. Kasatkina, I. Pliss, Modified generalized neo-fuzzy system with combined online fast learning in medical diagnostic task for situations of information deficit, Math. Biosci. Eng., 19 (2022), 8003-8018. https://doi.org/10.3934/mbe.2022374 doi: 10.3934/mbe.2022374
![]() |
[4] |
R. Loula, L. H. A. Monteiro, On the criteria for diagnosing depression in bereaved individuals: a self-organizing map approach, Math. Biosci. Eng., 19 (2022), 5380-5392. https://doi.org/10.3934/mbe.2022252 doi: 10.3934/mbe.2022252
![]() |
[5] |
L-S. Lin, S. C. Hu, Y-S. Lin, D. C. Li, L. R. Siao, A new approach to generating virtual samples to enhance classification accuracy with small data—a case of bladder cancer, Math. Biosci. Eng., 19 (2022), 6204-6233. https://doi.org/10.3934/mbe.2022290 doi: 10.3934/mbe.2022290
![]() |
[6] |
N. Shakhovska, V. Yakovyna, V. Chopyak, A new hybrid ensemble machine-learning model for severity risk assessment and post-COVID prediction system, Math. Biosci. Eng., 19 (2022), 6102-6123. https://doi.org/10.3934/mbe.2022285 doi: 10.3934/mbe.2022285
![]() |
[7] |
Y. Hu, M. Wang, K. Wang, J. Gao, J. Tong, Z. Zhao, et al., A potential role for metastasis-associated in colon cancer 1 (MACC1) as a pan-cancer prognostic and immunological biomarker, Math. Biosci. Eng., 19 (2022), 8331-8353. https://doi.org/10.3934/mbe.2021413 doi: 10.3934/mbe.2021413
![]() |
[8] |
B. Ma, L. Cao, Y. Li, A novel 10-gene immune-related lncRNA signature model for the prognosis of colorectal cancer, Math. Biosci. Eng., 19 (2022), 9743-9760. https://doi.org/10.3934/mbe.2021477 doi: 10.3934/mbe.2021477
![]() |
[9] |
S. Shandilya, I. Izonin, S. K. Shandilya, K. K. Singh, Mathematical modelling of bio-inspired frog leap optimization algorithm for transmission expansion planning, Math. Biosci. Eng., 19 (2022), 7232-7247. https://doi.org/10.3934/mbe.2022341 doi: 10.3934/mbe.2022341
![]() |
The elements of the collection G in Theorem 6 | |||
G000={(x,x2)} | G101={(x,x2+1)} | … | G(n−1)0n−1={(x,x2+n−1)} |
G010={(x,x2+x)} | G111={(x,x2+x+1)} | … | G(n−1)1n−1={(x,x2+x+n−1)} |
G020={(x,x2+2x)} | G121={(x,x2+2x+1)} | … | G(n−1)2n−1={(x,x2+2x+n−1)} |
⋮ | ⋮ | ⋮ | ⋮ |
G0(n−1)0={(x,x2+(n−1)x)} | G1(n−1)1={(x,x2+(n−1)x+1)} | … | G(n−1)(n−1)n−1={(x,x2+(n−1)x+n−1)} |
The elements of the collection G in Theorem 7 | |||
G000={(x,x)} | G101={(x,x+1)} | … | G(n−1)0n−1={(x,x+n−1)} |
G010={(x,2x)} | G111={(x,2x+1)} | … | G(n−1)1n−1={(x,2x+n−1)} |
G020={(x,3x)} | G121={(x,3x+1)} | … | G(n−1)2n−1={(x,3x+n−1)} |
⋮ | ⋮ | ⋮ | ⋮ |
G0(n−2)0={(x,(n−1)x)} | G1(n−2)1={(x,(n−1)x+1)} | … | G(n−1)(n−2)n−1={(x,(n−1)x+n−1)} |
The elements of the collection G in Theorem 8 | |||
G000={(0,0),(x,x+1)} | G101={(0,1),(x,x+2)} | … | G(n−1)0n−1={(0,n−1),(x,x+n)} |
G010={(0,0),(x,2x+1)} | G111={(0,1),(x,2x+2)} | … | G(n−1)1n−1={(0,n−1),(x,2x+n)} |
G020={(0,0),(x,3x+1)} | G121={(0,1),(x,3x+2)} | … | G(n−1)2n−1={(0,n−1),(x,3x+n)} |
⋮ | ⋮ | ⋮ | ⋮ |
G0(n−2)0={(0,0),(x,(n−1)x+1)} | G1(n−2)1={(0,1),(x,(n−1)x+2)} | … | G(n−1)(n−2)n−1={(0,n−1),(x,(n−1)x+n)} |
The elements of the collection G in Theorem 9 | |||
G000={(x,x2)} | G101={(x,x2+1)} | … | G808={(x,x2+8)} |
G010={(x,x2+x)} | G111={(x,x2+x+1)} | … | G818={(x,x2+x+8)} |
G020={(x,x2+2x)} | G121={(x,x2+2x+1)} | … | G828={(x,x2+2x+8)} |
The elements of the collection G in Theorem 10 | |||
G000={(x,x2)} | G101={(x,x2+1)} | … | G11,011={(x,x2+11)} |
G010={(x,x2+x)} | G111={(x,x2+x+1)} | … | G11,111={(x,x2+x+11)} |
Kn,n | F | k | X |
K3,3 | P4 | 3 | K1,1 |
K3,3 | P3∪K1,1 | 3 | K1,1 |
K4,4 | 2K1,2 | 3 | K1,1 |
K4,4 | K2,2 | 3 | K1,1 |
Kp,p | Pp+1 | p | K1,1 |
Kp,p | p−12P3∪K1,1 | p | K1,1 |
Kp,p | pK1,1 | p−1 | K1,1 |
Kp,p | (p−2)K1,1∪K1,2 | p−1 | K1,1 |
K9,9 | 3K1,2∪K1,3 | 3 | K1,1 |
K12,12 | 2K1,2∪2K1,4 | 2 | K1,1 |
The elements of the collection G in Theorem 6 | |||
G000={(x,x2)} | G101={(x,x2+1)} | … | G(n−1)0n−1={(x,x2+n−1)} |
G010={(x,x2+x)} | G111={(x,x2+x+1)} | … | G(n−1)1n−1={(x,x2+x+n−1)} |
G020={(x,x2+2x)} | G121={(x,x2+2x+1)} | … | G(n−1)2n−1={(x,x2+2x+n−1)} |
⋮ | ⋮ | ⋮ | ⋮ |
G0(n−1)0={(x,x2+(n−1)x)} | G1(n−1)1={(x,x2+(n−1)x+1)} | … | G(n−1)(n−1)n−1={(x,x2+(n−1)x+n−1)} |
The elements of the collection G in Theorem 7 | |||
G000={(x,x)} | G101={(x,x+1)} | … | G(n−1)0n−1={(x,x+n−1)} |
G010={(x,2x)} | G111={(x,2x+1)} | … | G(n−1)1n−1={(x,2x+n−1)} |
G020={(x,3x)} | G121={(x,3x+1)} | … | G(n−1)2n−1={(x,3x+n−1)} |
⋮ | ⋮ | ⋮ | ⋮ |
G0(n−2)0={(x,(n−1)x)} | G1(n−2)1={(x,(n−1)x+1)} | … | G(n−1)(n−2)n−1={(x,(n−1)x+n−1)} |
The elements of the collection G in Theorem 8 | |||
G000={(0,0),(x,x+1)} | G101={(0,1),(x,x+2)} | … | G(n−1)0n−1={(0,n−1),(x,x+n)} |
G010={(0,0),(x,2x+1)} | G111={(0,1),(x,2x+2)} | … | G(n−1)1n−1={(0,n−1),(x,2x+n)} |
G020={(0,0),(x,3x+1)} | G121={(0,1),(x,3x+2)} | … | G(n−1)2n−1={(0,n−1),(x,3x+n)} |
⋮ | ⋮ | ⋮ | ⋮ |
G0(n−2)0={(0,0),(x,(n−1)x+1)} | G1(n−2)1={(0,1),(x,(n−1)x+2)} | … | G(n−1)(n−2)n−1={(0,n−1),(x,(n−1)x+n)} |
The elements of the collection G in Theorem 9 | |||
G000={(x,x2)} | G101={(x,x2+1)} | … | G808={(x,x2+8)} |
G010={(x,x2+x)} | G111={(x,x2+x+1)} | … | G818={(x,x2+x+8)} |
G020={(x,x2+2x)} | G121={(x,x2+2x+1)} | … | G828={(x,x2+2x+8)} |
The elements of the collection G in Theorem 10 | |||
G000={(x,x2)} | G101={(x,x2+1)} | … | G11,011={(x,x2+11)} |
G010={(x,x2+x)} | G111={(x,x2+x+1)} | … | G11,111={(x,x2+x+11)} |
Kn,n | F | k | X |
K3,3 | P4 | 3 | K1,1 |
K3,3 | P3∪K1,1 | 3 | K1,1 |
K4,4 | 2K1,2 | 3 | K1,1 |
K4,4 | K2,2 | 3 | K1,1 |
Kp,p | Pp+1 | p | K1,1 |
Kp,p | p−12P3∪K1,1 | p | K1,1 |
Kp,p | pK1,1 | p−1 | K1,1 |
Kp,p | (p−2)K1,1∪K1,2 | p−1 | K1,1 |
K9,9 | 3K1,2∪K1,3 | 3 | K1,1 |
K12,12 | 2K1,2∪2K1,4 | 2 | K1,1 |